- 1School of Physical Education And Health, Guangxi Medical University, Nanning, China
- 2Department of Bone and Joint Surgery, The First Affiliated Hospital of Guangxi Medical University, Nanning, China
- 3Department of Spinal Surgery, The First Affiliated Hospital of Guangxi University of Chinese Medicine, Nanning, China
Background: Clinical observations indicate that blood lipids may be risk factors for lateral epicondylitis (LE) of the humerus, and lipid-lowering drugs are also used for the prevention and treatment of tendon diseases, but these lack high-quality clinical trial evidence and remain inconclusive. Mendelian randomization (MR) analyses can overcome biases in traditional observational studies and offer more accurate inference of causal relationships. Therefore, we employed this approach to investigate whether blood lipids are risk factors for LE and if lipid-lowering drugs can prevent it.
Methods: Genetic variations associated with lipid traits, including low-density lipoprotein cholesterol (LDL-C), triglycerides (TG), and total cholesterol (TC), were obtained from the UK Biobank and the Global Lipids Genetics Consortium (GLGC). Data on genetic variation in LE were sourced from FinnGen, including 24,061 patients and 275,212 controls. Subsequently, MR analyses were conducted to assess the potential correlation between lipid traits and LE. Additionally, drug-target Mendelian randomization analyses were performed on 10 drug targets relevant to LE. For those drug targets that yielded significant results, further analysis was conducted using colocalization techniques.
Results: No correlation was found between three blood lipid traits and LE. Lipoprotein lipase (LPL) enhancement is significantly associated with a decreased risk of LE (OR = 0.76, [95% CI, 0.65–0.90], p = 0.001). The expression of LPL in the blood is associated with LE and shares a single causal variant (12.07%), greatly exceeding the probability of different causal variations (1.93%), with a colocalization probability of 86.2%.
Conclusion: The three lipid traits are not risk factors for lateral epicondylitis. LPL is a potential drug target for the prevention and treatment of LE.
1 Introduction
Lateral epicondylitis (LE) of the humerus, also known as “tennis elbow,” is primarily linked to overuse of the elbow, particularly affecting the extensor carpi radialis brevis muscle (Bisset and Vicenzino, 2015; Herquelot et al., 2013; Sayampanathan et al., 2020). Among adults, the incidence rate ranges from 1%–3%, while tennis players exhibit a significantly higher incidence, reaching 40%–50% (Sanders et al., 2015; Cutts et al., 2020; Stegink-Jansen et al., 2021). Surgical intervention is reserved for patients who resist conventional treatments (Knutsen et al., 2015; Xu et al., 2023). Typically, patients undergo conservative treatment, which includes rest, oral nonsteroidal anti-inflammatory drugs (NSAIDs), and localized corticosteroid injections. Prolonged NSAID usage may result in gastrointestinal complications (Wolf, 2023), whereas extended corticosteroid injections can lead to local skin and muscle atrophy, and pigmentation changes, among other concerns (Titchener et al., 2015; Branson et al., 2017; Kheiran et al., 2021; Kim et al., 2021). Therefore, there is an urgent need to develop new drugs to treat this disease.
Previous studies have shown a correlation between tendon diseases and hyperlipidemia (Tilley et al., 2015; Lee et al., 2019). The mechanism may involve lipid effects on tendon homeostasis, tendon remodeling, and inflammation activation (Lee et al., 2019; Steplewski et al., 2019; Chen et al., 2023). However, findings regarding the association between LE and blood lipids vary, while a meta-analysis indicates that high cholesterol levels are risk factors for LE (Chen et al., 2023), other studies suggest no clear association (Sayampanathan et al., 2020). Currently, research on the relationship between hyperlipidemia and LE remains limited. Observational studies, which dominate the available research, are prone to limitations and confounding factors, underscoring the incompleteness of our understanding of this relationship and the need for further exploration.
Statins are commonly used for cardiovascular diseases. Recent literature also suggests their potential in preventing tendon disorders (Yang and Qu, 2018; Lee et al., 2023). A systematic review and a cohort study with over 10 years of follow-up have shown that statins can reduce the risk of tendon disorders in patients with hyperlipidemia (Lin et al., 2015; Teichtahl et al., 2016). However, the gold standard for determining drug efficacy is randomized controlled trials (RCT), and currently, no relevant RCT studies exist. Therefore, further exploration of the relationship between lipid-lowering drugs and LE is necessary.
Mendelian randomization is a method that utilizes genetic variations as instrumental variables (IVs). The distribution of these variations in human populations is random, akin to the ideal conditions of an RCT. This randomness helps in investigating the causal relationship between exposure and disease, reducing confounding biases inherent in traditional observational studies, and thereby enhancing the accuracy of causal inference (Larsson et al., 2023). Drug target Mendelian randomization studies represent a straightforward application of this method. In these studies, genetic variants encoding protein targets have the potential to affect the expression of target genes. Furthermore, certain drugs can act on these targets, thereby influencing gene expression (Schmidt et al., 2020). In this study, we apply MR analysis to investigate the causal relationship between blood lipids and LE. We also examine the impact of lipid-lowering drugs on LE, aiming to discover new medications for the treatment of this condition.
2 Materials and methods
Our study is structured as follows: 1) We perform a two-sample MR analysis to investigate the potential causal relationship between three lipid traits and LE; 2) We undertake a drug-target MR analysis involving 10 genes associated with blood lipids and LE, aiming to explore the potential link between these target genes and the condition; 3) For genes identified as significant in the drug-target analysis, we proceed with further colocalization analysis. Our comprehensive research approach is illustrated in Figure 1. The foundation of MR lies in three critical assumptions. Firstly, Relevance: The genetic variants chosen must be strongly associated with the exposure factor, serving effectively as proxies for exposure. Secondly, Independence: These genetic variations should not be linked to other confounding factors that may influence the outcome, thus ensuring the accuracy of the results. Lastly, Exclusion Restriction: The influence of genetic variations on the outcome should occur exclusively through the exposure factor, eliminating any other direct influence pathways. These essential principles are detailed in Figure 2.
We obtained the genome-wide association study (GWAS) data for lipid traits from the IEU Open GWAS database (https://gwas.mrcieu.ac.uk/), which includes data from the UK Biobank and the Global Lipids Genetics Consortium (GLGC). The sample sizes for LDL-C, TG, and TC were 440,546, 441,016, and 187,365, respectively. For our analysis, instrumental variables were carefully chosen based on stringent criteria: a linkage disequilibrium (LD) clumping threshold of r2 < 0.001, a p-value less than 5 × 10−8, and a physical distance threshold not exceeding 10,000 kb. We then utilized the PhenoScanner database (http://www.phenoscanner.medschl.cam.ac.uk/) to identify and exclude SNPs that could be associated with potential confounding factors, such as overuse and diabetes (Ranger et al., 2016; Cannata et al., 2021; Park et al., 2021). Ultimately, for our two-sample MR analysis, we included 127, 223, and 74 SNPs for LDL-C, TG, and TC, respectively. Outcome data on LE were obtained from the FinnGen(R9), which included 4,278 patients and 275,212 healthy controls. Detailed information on the GWAS data is provided in Supplementary Table S1, and the SNPs for each of the three lipid traits are listed in Supplementary Tables S2–S4.
We retrieved lipid-lowering drugs and their associated coding genes from the DrugBank database (https://go.drugbank.com/). From this database, we identified 10 relevant genes, including six genes associated with lowering LDL-C and four genes involved in reducing TG levels. Our methodology for selecting genetic variants was in line with established research practices. Within a 100 kb range surrounding these target genes, we identified single-nucleotide polymorphisms (SNPs) linked to LDL-C, TG, and TC levels. These SNPs demonstrated genome-wide significant associations in a GWAS meta-analysis conducted by the GLGC (p < 5 × 10−8). To further refine our selection, we clumped these SNPs at a linkage disequilibrium (LD) threshold of r2 < 0.20 and a physical distance threshold of 250 kb, effectively choosing them as proxies for the targets of lipid-lowering drugs. Following these steps, we conducted drug-target MR studies, adhering to similar principles. We selected coronary heart disease as a positive control in these studies to validate the effectiveness of the genetic instrumental variables we identified.
Colocalization analysis was a key component in verifying the validity of instrumental variable assumptions. This analysis was crucial for confirming that the observed associations between exposure and outcome were not confounded by different genetic variants in linkage disequilibrium. Out of the five hypotheses generated by this method, two warrant special attention: H3, which posits that the two traits, although correlated, are influenced by distinct causal variations; and H4, which suggests that these traits are not only correlated but also share a common causal variation. We estimated the colocalization probability by calculating the ratio H4/(H3 + H4). A ratio exceeding 80% was interpreted as a positive result (Zuber et al., 2022; Bi et al., 2023).
2.1 Statistical analysis
In our MR study, we utilize five methods to calculate associations. The core methods include Inverse Variance Weighted (IVW), MR Egger, and Weighted Median, which serve as the primary reference methods for our analysis. We assess heterogeneity using Weighted Cochran’s Q and MR Egger. Additionally, the egger-intercept parameter is employed to detect the presence of pleiotropy. The MR-PRESSO method plays a crucial role in identifying outliers, and it allows us to provide a causal estimate after excluding these outliers (Verbanck et al., 2018). To ensure statistical rigor, we apply the Bonferroni correction to adjust significance levels. For the analysis of three lipid traits, a p-value of less than 0.016 (0.05/3) is considered statistically significant. In the case of the ten lipid target genes, a p-value of less than 0.005 (0.05/10) is indicative of statistical significance. “Two-Sample MR,” and “coloc” in R (version 4.2.2) were used for all statistical analyses.
3 Results
3.1 The relationship between lipid traits and LE
Among the lipid traits studied, LDL-C, TG, and TC were associated with 127, 223, and 74 independent SNPs, respectively, all exhibiting F-statistics values greater than 10 (ranging from 26 to 3,279), thus confirming their statistical validity. In our MR study investigating the relationship between these three lipid traits and LE, all applied analytical methods yielded p-values greater than 0.05. This suggests no evidence of a causal association between the lipid traits and LE. Moreover, the absence of heterogeneity as indicated by the Cochran Q test and the MR-Egger heterogeneity test, along with the MR-Egger intercept not showing signs of horizontal pleiotropy, further substantiates the robustness of our findings. The results of the MR analysis are detailed in Supplementary Table S5, while the assessments for heterogeneity and pleiotropy are documented in Supplementary Table S6. Additionally, the Forest plot illustrating the MR results is presented in Figure 3.
3.2 The relationship between lipid-lowering drug targets and LE
This study identified ten target genes—HMGCR, PCSK9, ABCG5, APOB, NPC1L1, LDLR, ANGPTL3, LPL, APOC3, and PPARA—associated with LE. Post-screening, all SNPs related to these genes demonstrated F-values greater than 10, ranging from 34 to 138,055, ensuring robust statistical significance. We employed coronary heart disease (CHD) as a positive control in our MR analysis. The results indicated that eight of the drug targets were significantly correlated with a decreased risk of CHD, thereby validating the instrumental variables used in the study. While the associations between NPC1L1, ANGPTL3, and coronary heart disease did not reach statistical significance, an overall protective trend against CHD was observed. Notably, the MR-Egger heterogeneity test revealed heterogeneity in the NPC1L1 target (p = 0.02). In contrast, LPL showed a significant association with a reduced risk of LE (OR = 0.76, [95% CI, 0.65–0.90], p = 0.001). However, no significant associations were found between LE and the other nine drug targets. The Cochran Q test and MR-Egger heterogeneity test for all drug targets yielded p-values greater than 0.05, suggesting a lack of heterogeneity across the study. The MR-Egger intercept test identified pleiotropy in the PCSK9 target (p = 0.02), but PCSK9 was not deemed a positive drug target. Detailed data on the ten target genes are presented in Supplementary Tables S7, S8. Supplementary Tables S9, S10 contain the MR results for drug targets, including tests for heterogeneity and pleiotropy. Supplementary Tables S11, S12 display the MR results for the positive control, along with checks for heterogeneity and pleiotropy. The IVW method’s drug-targeted results are shown in Figure 4. Figure 5 illustrates the MR results for the positive control using the IVW method. Figure 6 shows the visual graph of the drug target MR results for LPL-LE. (A) Scatter plot. (B) Funnel plot. (C) Forest plot. (D) Leave-one-out sensitivity analysis.
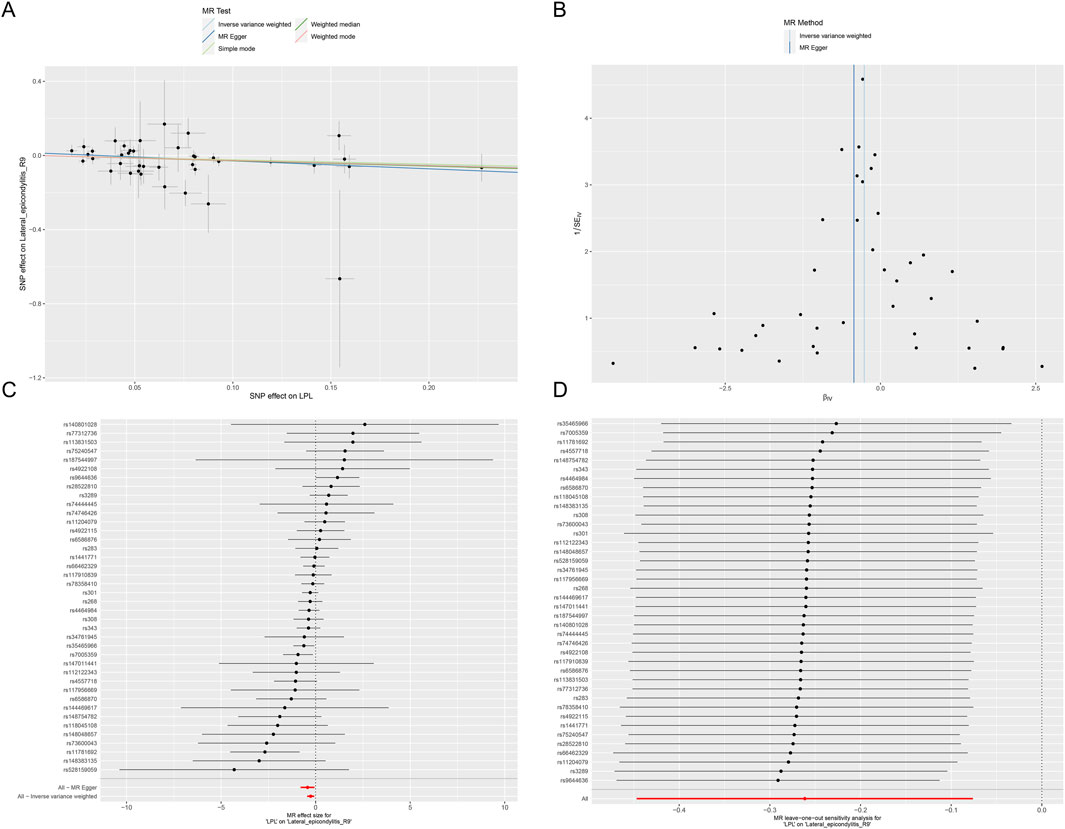
Figure 6. The visual graph of the drug target MR results for LPL-LE. (A) Scatter plot. (B) Funnel plot. (C) Forest plot. (D) Leave-one-out sensitivity analysis.
3.3 Colocalization analysis
In the study of the LPL target, we extended our analysis to include colocalization. This analysis revealed that the likelihood of a shared causal relationship between LPL expression in the blood and LE stands at 12.07% (H4). Conversely, the probability of there being different causal variants was found to be 1.93% (H3). The overall probability of colocalization, calculated as 86.2% (H4/H3+H4), suggests a strong likelihood of shared causality. Detailed results of this colocalization analysis are presented in Supplementary Table S13.
4 Discussion
The findings of this MR study suggest that blood lipids are not causally linked to LE. Furthermore, LPL emerges as a promising drug target for treating LE. Intriguingly, the protective role of LPL in LE seems independent of its lipid-lowering properties, indicating a potential alternative mechanism of action.
Current research findings have not established LDL-C, TG, and TC as risk factors for LE, aligning with conclusions from earlier studies (Scott et al., 2015; Sayampanathan et al., 2020; Park et al., 2021). Furthermore, recent MR studies indicate that TG is not a risk factor for conditions such as rotator cuff syndrome and achilles tendinopathy (He et al., 2022; Cao et al., 2023). Given that LE is categorized as a type of tendinopathy, these findings imply a lack of causal link between TG and LE. Our MR study specifically addresses this research gap, providing valuable insights into the relationship between these factors and LE.
The LPL gene is located on human chromosome 8p22, encoding 448 amino acids and comprising 10 exons (spanning 30 kb) interspersed with nine introns (spanning 6 kb) (He et al., 2018). LPL is ubiquitously expressed in the capillary endothelium of all tissues, where its primary function is to hydrolyze TG into glycerol and free fatty acids (FFA), which are then absorbed by tissues (Geldenhuys et al., 2017b). The gene is most abundantly expressed and active in adipose tissue, skeletal muscle, and the heart, while its expression is comparatively lower in other tissues (Chang, 2019). LPL exhibits tissue-specific physiological roles: in skeletal muscle, it facilitates the breakdown of plasma lipoproteins, reducing plasma TG and providing FFA for oxidative energy production, whereas in adipose tissue, the FFA generated is preferentially directed toward lipid storage (Li et al., 2014). Environmental factors such as fasting/feeding, exercise, and cold exposure can modulate LPL protein levels and activity, thereby channeling fatty acids to specific cells according to their energy requirements (Wu et al., 2021). Furthermore, LPL expression is tightly regulated at both the transcriptional and translational levels through multiple complex mechanisms (Kersten, 2014; Geldenhuys et al., 2017b).
In our study, we discovered that LPL is linked to a lower risk of LE. This contrasts with ANGPTL3 and APOC3, which, despite also lowering TG, do not show a similar association. This leads us to hypothesize that the protective effect of LPL against LE might not be related to its lipid-lowering properties but rather to its role in reducing inflammation. Recent research over the past decade has emphasized the significant involvement of inflammatory cells and mediators in the progression of tendinopathy (Dakin et al., 2014; Millar et al., 2017). The literature describes the initial phase of LE as an acute inflammatory response, which precedes changes in tissue structure, such as angiogenesis and disordered collagen fiber alignment (Ackermann and Renström, 2012; Ahmad et al., 2013). Confirming this, a color Doppler ultrasound study on LE identified the presence of inflammation (Torp-Pedersen et al., 2008). Cells associated with inflammation, including macrophages, mast cells, B lymphocytes, and T lymphocytes, heavily infiltrate the affected area, releasing inflammatory factors like Interleukin-1 (IL-1), Interleukin-6 (IL-6), Tumor Necrosis Factor-alpha (TNF-α), and Tumor Necrosis Factor-beta (TNF-β). This leads to increased activity in the Nuclear Factor-kappa B (NF-κB) pathway, triggering an inflammatory cascade (Han et al., 2012; Millar et al., 2021). Beyond its triglyceride-lowering function, LPL has been reported to suppress the release of inflammatory factors such as TNF-α, IL-6, and Cyclooxygenase-2 (COX-2) by inhibiting NF-κB activation, thereby mitigating inflammation (Kota et al., 2005; Takasu et al., 2012). This inhibition of inflammation may be a key mechanism through which LPL treats tendinopathies.
Discovered in the early 1990s, NO – 1886 (Ibrolipim) is an established LPL activator. It effectively increases LPL mRNA levels in tissues, boosts the concentration of LPL protein in plasma following heparin administration, and enhances LPL activity. In addition, NO – 1886 has been shown to lower plasma triglyceride levels in animals with lipid disorders (Yin et al., 2004; Geldenhuys et al., 2017a). Animal studies further reveal that this compound significantly reduces 29 types of cellular inflammatory factors, including IL-1, IL-6, and TNF-β, among others. It also suppresses the expression of most pro-inflammatory cytokines in adipose tissue, leading to reduced tissue inflammation (Cai et al., 2006). Evidence suggests that NO – 1886 primarily targets LPL in skeletal muscle (Kusunoki et al., 2005), indicating its potential to mitigate tendon inflammation. Our study findings also demonstrate that ANGPTL3 inhibitors display a protective trend against LE, with an odds ratio of 0.54 ([95% CI, 0.35–0.84], p = 0.006). Moreover, the literature indicates that ANGPTL inhibitors enhance LPL activity, urging further exploration of the link between ANGPTL3 and LE. The newly reported drug molecule C10d exhibits at least double the LPL activation efficiency of N0-1886 and can reverse the inhibitory effect of ANGPTL on LPL, increasing its investigative potential (Geldenhuys et al., 2014; 2017b). In conclusion, drugs that activate LPL may prevent LE by inhibiting the release of inflammatory factors. However, further cellular and molecular-level studies are needed to explain this result, and high-quality clinical research is also crucial.
It is important to note that drug-target MR results suggest an association between PCSK9 inhibition and an increased risk of LE (OR = 1.36, [95% CI, 1.06–1.75], p = 0.014), a finding that may appear counterintuitive. However, this result demonstrates pleiotropy (p = 0.02, Supplementary Table S10), implying the presence of confounding factors that invalidate the conclusion. The relationship between PCSK9 inhibition and inflammation remains a subject of debate. Seidah et al. reported that PCSK9 inhibition suppresses TLR4/NF-κB signaling, leading to the inhibition of inflammatory mediators (Seidah and Prat, 2021). Similarly, Ding et al. found that PCSK9 inhibition reduces the inflammatory response by suppressing toll-like receptors (TLRs), the NLRP3 inflammasome, and the NF-κB pathway (Ding et al., 2022). Conversely, Pradhan et al. observed that in a cohort of 9,738 patients treated with PCSK9 inhibitors for 14 weeks, 47.2% exhibited residual inflammatory risk, with high-sensitivity C-reactive protein (hs-CRP) levels ≥2 mg/L during treatment (Pradhan et al., 2018). Given the inconclusive relationship between PCSK9 inhibition and inflammation, coupled with the lack of a positive drug target MR result in this study, further research is needed to clarify the connection between PCSK9 inhibition and LE.
This study possesses several limitations. Primarily, our reliance on data from FinnGen as the exclusive outcome may lead to certain biases. Additionally, the absence of RCTs focusing on the risk factors for lateral epicondylitis limits the breadth of our analysis. In excluding confounding SNPs, we specifically targeted those associated with overuse and diabetes, which could introduce bias stemming from subjective selection. Finally, as our study population was predominantly European, it is important to consider that the findings might not be universally applicable across different ethnic groups.
5 Conclusion
Low-density lipoprotein cholesterol, triglycerides, and total cholesterol are not risk factors for lateral epicondylitis. Moreover, we identified lipoprotein lipase (LPL) as a potential therapeutic target, suggesting its importance in preventing and treating this condition.
Data availability statement
The original contributions presented in the study are included in the article/Supplementary Material, further inquiries can be directed to the corresponding author.
Author contributions
M-ML: Visualization, Writing–original draft. XC: Writing–original draft, Software. X-HB: Data curation, Writing–review and editing. B-HH: Supervision, Writing–review and editing.
Funding
The author(s) declare that no financial support was received for the research, authorship, and/or publication of this article.
Acknowledgments
We want to extend our gratitude to the participants and authors of various Genome-Wide Association Studies (GWASs), such as the UK Biobank, FinnGen, GLGC, and CARDIoGRAMplusC4D, for generously providing the data used in this summary.
Conflict of interest
The authors declare that the research was conducted in the absence of any commercial or financial relationships that could be construed as a potential conflict of interest.
Publisher’s note
All claims expressed in this article are solely those of the authors and do not necessarily represent those of their affiliated organizations, or those of the publisher, the editors and the reviewers. Any product that may be evaluated in this article, or claim that may be made by its manufacturer, is not guaranteed or endorsed by the publisher.
Supplementary material
The Supplementary Material for this article can be found online at: https://www.frontiersin.org/articles/10.3389/fgene.2024.1437712/full#supplementary-material
References
Ackermann, P. W., and Renström, P. (2012). Tendinopathy in sport. Sports Health 4, 193–201. doi:10.1177/1941738112440957
Ahmad, Z., Siddiqui, N., Malik, S. S., Abdus-Samee, M., Tytherleigh-Strong, G., and Rushton, N. (2013). Lateral epicondylitis: a review of pathology and management. Bone and Jt. J. 95-B, 1158–1164. doi:10.1302/0301-620x.95b9.29285
Bi, Y., Zhu, Y., Tang, S., and Huang, Y. (2023). Lipids, lipid-modifying drug target genes, and migraine: a Mendelian randomization study. J. Headache Pain 24, 112. doi:10.1186/s10194-023-01633-x
Bisset, L. M., and Vicenzino, B. (2015). Physiotherapy management of lateral epicondylalgia. J. Physiother. 61, 174–181. doi:10.1016/j.jphys.2015.07.015
Branson, R., Naidu, K., du Toit, C., Rotstein, A. H., Kiss, R., McMillan, D., et al. (2017). Comparison of corticosteroid, autologous blood, or sclerosant injections for chronic tennis elbow. J. Sci. Med. Sport 20, 528–533. doi:10.1016/j.jsams.2016.10.010
Cai, M., Yin, W., Li, Q., Liao, D., Tsutsumi, K., Hou, H., et al. (2006). Effects of NO-1886 on inflammation-associated cytokines in high-fat/high-sucrose/high-cholesterol diet-fed miniature pigs. Eur. J. Pharmacol. 540, 139–146. doi:10.1016/j.ejphar.2006.04.032
Cannata, F., Vadalà, G., Ambrosio, L., Napoli, N., Papalia, R., Denaro, V., et al. (2021). The impact of type 2 diabetes on the development of tendinopathy. Diabetes Metab. Res. Rev. 37, e3417. doi:10.1002/dmrr.3417
Cao, Z., Li, Q., Li, Y., and Wu, J. (2023). The association of metabolic syndrome with rotator cuff tendinopathy: a two-sample Mendelian randomization study. Diabetol. Metab. Syndr. 15, 211. doi:10.1186/s13098-023-01189-5
Chang, C. L. (2019). Lipoprotein lipase: new roles for an “old” enzyme. Curr. Opin. Clin. Nutr. metabolic care 22, 111–115. doi:10.1097/MCO.0000000000000536
Chen, Q., Shen, P., Zhang, B., Chen, Y., and Zheng, C. (2023). A meta-analysis of the risk factors for lateral epicondylitis. J. Hand Ther. 37, 44–52. doi:10.1016/j.jht.2023.05.013
Cutts, S., Gangoo, S., Modi, N., and Pasapula, C. (2020). Tennis elbow: a clinical review article. J. Orthop. 17, 203–207. doi:10.1016/j.jor.2019.08.005
Dakin, S. G., Dudhia, J., and Smith, R. K. W. (2014). Resolving an inflammatory concept: the importance of inflammation and resolution in tendinopathy. Vet. Immunol. Immunopathol. 158, 121–127. doi:10.1016/j.vetimm.2014.01.007
Ding, Z., Pothineni, N. V. K., Goel, A., Lüscher, T. F., and Mehta, J. L. (2022). Corrigendum to: PCSK9 and inflammation: role of shear stress, pro-inflammatory cytokines, and LOX-1. Cardiovasc Res. 118, 2031. doi:10.1093/cvr/cvab352
Geldenhuys, W. J., Aring, D., and Sadana, P. (2014). A novel Lipoprotein lipase (LPL) agonist rescues the enzyme from inhibition by angiopoietin-like 4 (ANGPTL4). Bioorg. and Med. Chem. Lett. 24, 2163–2167. doi:10.1016/j.bmcl.2014.03.021
Geldenhuys, W. J., Caporoso, J., Leeper, T. C., Lee, Y.-K., Lin, L., Darvesh, A. S., et al. (2017a). Structure-activity and in vivo evaluation of a novel lipoprotein lipase (LPL) activator. Bioorg. and Med. Chem. Lett. 27, 303–308. doi:10.1016/j.bmcl.2016.11.053
Geldenhuys, W. J., Lin, L., Darvesh, A. S., and Sadana, P. (2017b). Emerging strategies of targeting lipoprotein lipase for metabolic and cardiovascular diseases. Drug Discov. Today 22, 352–365. doi:10.1016/j.drudis.2016.10.007
Han, S. H., An, H. J., Song, J. Y., Shin, D. E., Kwon, Y. D., Shim, J. S., et al. (2012). Effects of corticosteroid on the expressions of neuropeptide and cytokine mRNA and on tenocyte viability in lateral epicondylitis. J. Inflamm. (Lond) 9, 40. doi:10.1186/1476-9255-9-40
He, L., Yu, T., Zhang, W., Wang, B., Ma, Y., and Li, S. (2022). Causal associations of obesity with achilles tendinopathy: a two-sample mendelian randomization study. Front. Endocrinol. (Lausanne) 13, 902142. doi:10.3389/fendo.2022.902142
He, P.-P., Jiang, T., OuYang, X.-P., Liang, Y.-Q., Zou, J.-Q., Wang, Y., et al. (2018). Lipoprotein lipase: biosynthesis, regulatory factors, and its role in atherosclerosis and other diseases. Clin. Chim. Acta 480, 126–137. doi:10.1016/j.cca.2018.02.006
Herquelot, E., Guéguen, A., Roquelaure, Y., Bodin, J., Sérazin, C., Ha, C., et al. (2013). Work-related risk factors for incidence of lateral epicondylitis in a large working population. Scand. J. Work Environ. Health 39, 578–588. doi:10.5271/sjweh.3380
Kersten, S. (2014). Physiological regulation of lipoprotein lipase. Biochimica Biophysica Acta (BBA) - Mol. Cell Biol. Lipids 1841, 919–933. doi:10.1016/j.bbalip.2014.03.013
Kheiran, A., Pandey, A., and Pandey, R. (2021). Common tendinopathies around the elbow; what does current evidence say? J. Clin. Orthop. Trauma 19, 216–223. doi:10.1016/j.jcot.2021.05.021
Kim, Y. J., Wood, S. M., Yoon, A. P., Howard, J. C., Yang, L. Y., and Chung, K. C. (2021). Efficacy of nonoperative treatments for lateral epicondylitis: a systematic review and meta-analysis. Plastic Reconstr. Surg. 147, 112–125. doi:10.1097/PRS.0000000000007440
Knutsen, E. J., Calfee, R. P., Chen, R. E., Goldfarb, C. A., Park, K. W., and Osei, D. A. (2015). Factors associated with failure of nonoperative treatment in lateral epicondylitis. Am. J. Sports Med. 43, 2133–2137. doi:10.1177/0363546515590220
Kota, R. S., Ramana, C. V., Tenorio, F. A., Enelow, R. I., and Rutledge, J. C. (2005). Differential effects of lipoprotein lipase on tumor necrosis factor-alpha and interferon-gamma-mediated gene expression in human endothelial cells. J. Biol. Chem. 280, 31076–31084. doi:10.1074/jbc.M412189200
Kusunoki, M., Tsutsumi, K., Iwata, K., Yin, W., Nakamura, T., Ogawa, H., et al. (2005). NO-1886 (ibrolipim), a lipoprotein lipase activator, increases the expression of uncoupling protein 3 in skeletal muscle and suppresses fat accumulation in high-fat diet-induced obesity in rats. Metabolism 54, 1587–1592. doi:10.1016/j.metabol.2005.06.005
Larsson, S. C., Butterworth, A. S., and Burgess, S. (2023). Mendelian randomization for cardiovascular diseases: principles and applications. Eur. Heart J. 44, 4913–4924. doi:10.1093/eurheartj/ehad736
Lee, S., Lee, N., and Shin, S.-J. (2023). Relationship of missed statin therapy and 10-year atherosclerotic cardiovascular disease risk score to retear rate after arthroscopic rotator cuff repair. Am. J. Sports Med. 51, 1988–1996. doi:10.1177/03635465231175476
Lee, S. H., Gong, H. S., Kim, S., Kim, J., and Baek, G. H. (2019). Is there a relation between lateral epicondylitis and total cholesterol levels? Arthrosc. J. Arthrosc. and Relat. Surg. 35, 1379–1384. doi:10.1016/j.arthro.2019.01.048
Li, Y., He, P.-P., Zhang, D.-W., Zheng, X.-L., Cayabyab, F. S., Yin, W.-D., et al. (2014). Lipoprotein lipase: from gene to atherosclerosis. Atherosclerosis 237, 597–608. doi:10.1016/j.atherosclerosis.2014.10.016
Lin, T. T.-L., Lin, C.-H., Chang, C.-L., Chi, C.-H., Chang, S.-T., and Sheu, W. H.-H. (2015). The effect of diabetes, hyperlipidemia, and statins on the development of rotator cuff disease: a nationwide, 11-year, longitudinal, population-based follow-up study. Am. J. Sports Med. 43, 2126–2132. doi:10.1177/0363546515588173
Millar, N. L., Murrell, G. A. C., and McInnes, I. B. (2017). Inflammatory mechanisms in tendinopathy - towards translation. Nat. Rev. Rheumatol. 13, 110–122. doi:10.1038/nrrheum.2016.213
Millar, N. L., Silbernagel, K. G., Thorborg, K., Kirwan, P. D., Galatz, L. M., Abrams, G. D., et al. (2021). Tendinopathy. Nat. Rev. Dis. Prim. 7, 1–21. doi:10.1038/s41572-020-00234-1
Park, H. B., Gwark, J.-Y., Im, J.-H., and Na, J.-B. (2021). Factors associated with lateral epicondylitis of the elbow. Orthop. J. Sports Med. 9, 23259671211007734. doi:10.1177/23259671211007734
Pradhan, A. D., Aday, A. W., Rose, L. M., and Ridker, P. M. (2018). Residual inflammatory risk on treatment with PCSK9 inhibition and statin therapy. Circulation 138, 141–149. doi:10.1161/CIRCULATIONAHA.118.034645
Ranger, T. A., Wong, A. M. Y., Cook, J. L., and Gaida, J. E. (2016). Is there an association between tendinopathy and diabetes mellitus? A systematic review with meta-analysis. Br. J. Sports Med. 50, 982–989. doi:10.1136/bjsports-2015-094735
Sanders, T. L., Maradit Kremers, H., Bryan, A. J., Ransom, J. E., Smith, J., and Morrey, B. F. (2015). The epidemiology and health care burden of tennis elbow: a population-based study. Am. J. Sports Med. 43, 1066–1071. doi:10.1177/0363546514568087
Sayampanathan, A. A., Basha, M., and Mitra, A. K. (2020). Risk factors of lateral epicondylitis: a meta-analysis. Surgeon 18, 122–128. doi:10.1016/j.surge.2019.08.003
Schmidt, A. F., Finan, C., Gordillo-Marañón, M., Asselbergs, F. W., Freitag, D. F., Patel, R. S., et al. (2020). Genetic drug target validation using Mendelian randomisation. Nat. Commun. 11, 3255. doi:10.1038/s41467-020-16969-0
Scott, A., Zwerver, J., Grewal, N., de Sa, A., Alktebi, T., Granville, D. J., et al. (2015). Lipids, adiposity and tendinopathy: is there a mechanistic link? Critical review. Br. J. Sports Med. 49, 984–988. doi:10.1136/bjsports-2014-093989
Seidah, N. G., and Prat, A. (2021). The multifaceted biology of PCSK9. Endocr. Rev. 43, 558–582. doi:10.1210/endrev/bnab035
Stegink-Jansen, C. W., Bynum, J. G., Lambropoulos, A. L., Patterson, R. M., and Cowan, A. C. (2021). Lateral epicondylosis: a literature review to link pathology and tendon function to tissue-level treatment and ergonomic interventions. J. Hand Ther. 34, 263–297. doi:10.1016/j.jht.2021.05.005
Steplewski, A., Fertala, J., Tomlinson, R., Hoxha, K., Han, L., Thakar, O., et al. (2019). The impact of cholesterol deposits on the fibrillar architecture of the Achilles tendon in a rabbit model of hypercholesterolemia. J. Orthop. Surg. Res. 14, 172. doi:10.1186/s13018-019-1217-7
Takasu, S., Mutoh, M., Takahashi, M., and Nakagama, H. (2012). Lipoprotein lipase as a candidate target for cancer prevention/therapy. Biochem. Res. Int. 2012, 398697. doi:10.1155/2012/398697
Teichtahl, A. J., Brady, S. R. E., Urquhart, D. M., Wluka, A. E., Wang, Y., Shaw, J. E., et al. (2016). Statins and tendinopathy: a systematic review. Med. J. Aust. 204, 115–121. doi:10.5694/mja15.00806
Tilley, B. J., Cook, J. L., Docking, S. I., and Gaida, J. E. (2015). Is higher serum cholesterol associated with altered tendon structure or tendon pain? A systematic review. Br. J. Sports Med. 49, 1504–1509. doi:10.1136/bjsports-2015-095100
Titchener, A. G., Booker, S. J., Bhamber, N. S., Tambe, A. A., and Clark, D. I. (2015). Corticosteroid and platelet-rich plasma injection therapy in tennis elbow (lateral epicondylalgia): a survey of current U.K. specialist practice and a call for clinical guidelines. Br. J. Sports Med. 49, 1410–1413. doi:10.1136/bjsports-2013-092674
Torp-Pedersen, T. E., Torp-Pedersen, S. T., Qvistgaard, E., and Bliddal, H. (2008). Effect of glucocorticosteroid injections in tennis elbow verified on colour Doppler ultrasonography: evidence of inflammation. Br. J. Sports Med. 42, 978–982. doi:10.1136/bjsm.2007.041285
Verbanck, M., Chen, C.-Y., Neale, B., and Do, R. (2018). Detection of widespread horizontal pleiotropy in causal relationships inferred from Mendelian randomization between complex traits and diseases. Nat. Genet. 50, 693–698. doi:10.1038/s41588-018-0099-7
Wolf, J. M. (2023). Lateral epicondylitis. N. Engl. J. Med. 388, 2371–2377. doi:10.1056/NEJMcp2216734
Wu, S. A., Kersten, S., and Qi, L. (2021). Lipoprotein lipase and its regulators: an unfolding story. Trends Endocrinol. Metab. 32, 48–61. doi:10.1016/j.tem.2020.11.005
Xu, J., Chen, M., Xue, X., Zhou, W., and Luo, X. (2023). Global research trends and hotspots in lateral epicondylitis during the past 30 Years: a bibliometric and visualization study. Med. Sci. Monit. 29, e939309. doi:10.12659/MSM.939309
Yang, Y., and Qu, J. (2018). The effects of hyperlipidemia on rotator cuff diseases: a systematic review. J. Orthop. Surg. Res. 13, 204. doi:10.1186/s13018-018-0912-0
Yin, W., Liao, D., Wang, Z., Xi, S., Tsutsumi, K., Koike, T., et al. (2004). NO-1886 inhibits size of adipocytes, suppresses plasma levels of tumor necrosis factor-alpha and free fatty acids, improves glucose metabolism in high-fat/high-sucrose-fed miniature pigs. Pharmacol. Res. 49, 199–206. doi:10.1016/j.phrs.2003.09.008
Keywords: lipids, lipid-lowering drugs, lateral epicondylitis, Mendelian randomization, drug target
Citation: Liu M-M, Chen X, Bao X-H and Huang B-H (2024) Lipids, lipid-lowering drugs and lateral epicondylitis of the humerus: a drug-targeted Mendelian randomization study. Front. Genet. 15:1437712. doi: 10.3389/fgene.2024.1437712
Received: 24 May 2024; Accepted: 22 August 2024;
Published: 02 September 2024.
Edited by:
Luiz Sérgio Fernandes de Carvalho, Clarity Healthcare Intelligence, BrazilReviewed by:
Hengyi Xu, The University of Texas at Austin, United StatesXinyi Zhang, Yale University, United States
Luyang Liu, Shandong Provincial Hospital, China
Copyright © 2024 Liu, Chen, Bao and Huang. This is an open-access article distributed under the terms of the Creative Commons Attribution License (CC BY). The use, distribution or reproduction in other forums is permitted, provided the original author(s) and the copyright owner(s) are credited and that the original publication in this journal is cited, in accordance with accepted academic practice. No use, distribution or reproduction is permitted which does not comply with these terms.
*Correspondence: Bao-Hua Huang, 20hbh@163.com