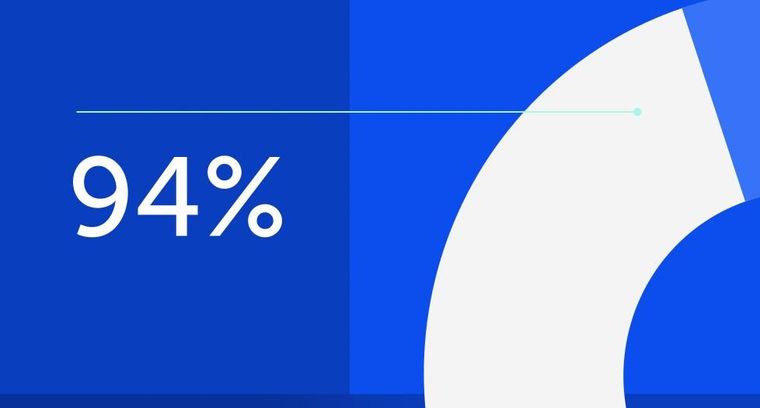
94% of researchers rate our articles as excellent or good
Learn more about the work of our research integrity team to safeguard the quality of each article we publish.
Find out more
ORIGINAL RESEARCH article
Front. Genet., 23 July 2024
Sec. Applied Genetic Epidemiology
Volume 15 - 2024 | https://doi.org/10.3389/fgene.2024.1403509
Background: Colorectal cancer is influenced by several factors such as unhealthy habits and genetic factors. C1QB has been linked to a number of malignancies. However, uncertainty surrounds the connection between C1QB and CRC. Therefore, this study aimed to explore a bidirectional causal relationship of C1QB as a drug target in CRC through Mendelian randomization (MR) analysis.
Methods: The GWASs for C1QB and CRC were obtained from the Integrative Epidemiology Unit Open GWAS database. There were five strategies to investigate MR. Sensitivity analysis was carried out via tests for heterogeneity, horizontal pleiotropy and leave-one-out effects to evaluate the dependability of the MR analysis results. Furthermore, colocalization analysis of C1QB and CRC, protein-protein interaction network and drug prediction according to exposure factors as well as phenotype scanning were performed.
Results: The results of forward MR analysis demonstrated that C1QB was a risk factor for CRC (OR = 1.104, p = 0.033). However, we did not find a causal relationship between CRC and C1QB (reverse MR). Rs294180 and rs291985 corresponded to the same linkage interval and had the potential to influence C1QB and CRC, respectively. The PPI results demonstrated that C1QB interacted with 10 genes (C1QA, C1QC, C1R, C1S, C2, C4A, C4B, CALR, SERPING1, and VSIG4). Additionally, 21 medications were predicted to match C1QB. Molecular docking data, including for benzo(a)pyrene, 1-naphthylisothiocyanate, calcitriol and medroxyprogesterone acetate, revealed excellent binding for drugs and proteins. Moreover, we identified 29 diseases that were associated with C1QB and related medicines via disease prediction and intersection methods. As a therapeutic target for CRC, phenotypic scanning revealed that C1QB does not significantly affect weight loss, liver cirrhosis, or nonalcoholic fatty liver disease, but might have protective impacts on ovarian cancer and melanoma.
Conclusion: The results highlight a causal relationship between C1QB and CRC and imply an oncogenic role for C1QB in CRC, as potential drug targets. Drugs designed to target C1QB have a greater chance of success in clinical trials and are expected to help prioritize CRC drug development and reduce drug development costs. That provided a theoretical foundation and reference for research on CRC and C1QB in MR.
According to the GLOBOCAN report, colorectal cancer (CRC) ranks third in incidence among malignant tumors and is the second leading cause of cancer death worldwide, affecting both genders (Sung et al., 2021). The variables associated with CRC risk include obesity, unhealthy dietary habits, smoking, aging, chronic inflammation and genetic predisposition (Hossain et al., 2022). In 2020, more than 1.9 million new colorectal cancer cases were diagnosed each year, with approximately 930,000 deaths globally. The burden of CRC is projected to increase to 3.2 million new cases and 1.6 million deaths by the year 2040 (Morgan et al., 2023). Despite existing treatment for CRC patients have achieved great advances, such as in surgery, chemotherapy, radiotherapy, molecularly targeted therapy and immunotherapy, barriers remain: high postoperative recurrence, chemotherapy drug resistance, and severe adverse effects limit therapeutic efficacy and result in poor prognosis. Therefore, further searching for potential biomarkers and treatment methods for CRC is crucial for enhancing the detection and longevity of individuals with CRC.
Complement is a class of peptides with enzyme activity and self-regulation. And the complement system represents an important component of the inflammatory response, which regulates both innate and adaptive immune responses. C1q is the first subcomponent of the complement classical pathway, which can activate the complement cascade when associated with C1r and C1s (Lu and Kishore, 2017). Human C1q is a hexameric molecule, assembled from 18 polypeptide chains of three different types, A (28 kDa), B (25 kDa), and C (24 kDa). Each of the three polypeptide chains consists of a C-terminal globular domain (gC1q), linked to a N-terminal collagen-like domain (cC1q) (Nayak et al., 2012). The role of the cC1q mainly mediates the immune effect by interacting with C1r and C1s proteases to activate the complement. And the gC1q domain is responsible for target recognition. It includes binding to the Fc region of immunoglobulin, recognition of surface proteins of bacteria and viruses (Kishore et al., 2004). In addition to binding IgG and IgM containing immune complexes and activating the complement classical pathway, there is emerging evidence to suggest that C1q plays crucial role in a wide range of physiological and pathological processes, such as placental development (Agostinis et al., 2017a), wound healing (Bossi et al., 2014), autoimmunity (Son et al., 2015) and cancer (Ghebrehiwet et al., 2024). C1q is locally synthesized by macrophages and dendritic cells, and has recently entered the limelight due to its immunoregulatory functions in the tumor microenvironment (TME). The notion of C1q involvement in the pathogenesis of cancer is still evolving. C1q appears to exert a dual role in cancer: tumor promoting as well as tumor-protective, depending on types of human tumors. Bioinformatics analysis in various carcinomas reported that high levels of C1q have a favorable prognostic index in basal-like breast cancer (Mangogna et al., 2019a), HER-2 positive breast cancer (Mangogna et al., 2019a), and skin cutaneous melanoma (Liang et al., 2022; Yang et al., 2022). However, C1q paly a pro-tumorigenic role in lung adenocarcinoma (Mangogna et al., 2019a), clear cell renal cell carcinoma (CCRCC) (Mangogna et al., 2019a) and Glioma (Mangogna et al., 2019b). C1QA, C1QB and C1QC genes encode the three C1q chains, respectively. Although the three genes are interdependent and often transcribed synchronously (Chen et al., 2011). Their expression levels seem to be not completely consistent across different pathological types of cancer. For example, the expression of the three C1q chains was higher in CCRCC as compared to normal kidney. However, in the case of papillary renal cell carcinoma (PRCC), this trend was evident only for C1QA and C1QB mRNA expression (Mangogna et al., 2019a). Junjie Jiang et al. reported that higher mRAN expression of C1QB, instead of C1QA and C1QC was in gastric cancer tissues than that in adjacent normal tissues (Jiang et al., 2020). The iTRAQ-based quantitative proteomic analysis reveals that the protein expression levels of C1QB and C1QC were upregulated in serum sample of patients with CCRCC and were significantly associated with the grade and stage of CCRCC (Zhang et al., 2016). Recently, Huiming Deng et al. showed that the protein expression of C1QC in CRC tissues was higher than that in non-cancer tissue by immunohistochemical staining. And a high protein expression of C1QC in CRC patients led to a poor prognosis (Deng et al., 2022). However, the associations between C1QB and the prognosis of CRC remain unclear due to insufficient sample size.
The statistical technique of Mendelian randomization (MR) has emerged as a robust method for evaluating causal connections through the use of genetic variables as instrumental factors in recent years (Holmes et al., 2017). Owing to the abundance of summary data from genome-wide association studies (GWAS), MR can be used to effectively and affordably determine the causal link between exposure and disease outcome (Liu et al., 2021). Drug-targeting MRs are new research designs that can be developed for drug repurposing by employing genetic tools near or within target genes to mimic the potential effects and dangers of drug targets. In the present study, we utilized a bidirectional two-sample MR design and used data from GWASs, to examine the causal relationship between C1QB and CRC. Subsequently, co-localization analysis of the C1QB gene and colorectal cancer tissue was performed to identify the expression quantitative trait locus (eQTL) associated with colorectal cancer. Finally, a protein-protein interaction (PPI) network was constructed, and drug prediction according to exposure factors and phenotype scanning were performed to provide new ideas for drug development in colorectal cancer. The study design is shown in Figure 1.
Figure 1. Overview of the study design. MR analyses depend on three core assumptions: ①Relevance (G is associated with the X).②Independence (G is not related to any confounding factors of the exposure-outcome association).③Exclusion restriction (G does not affect Y except through its potential effect on the X. The red represented the forward MR analyses, with C1QB as exposure and CRC as outcome. The blue represented the reverse MR analyses, with CRC as exposure and C1QB as outcome. Abbreviations: CRC, Colorectal cancer; MR, Mendelian randomization; SNPs, single-nucleotide polymorphisms.
The GWASs for colorectal cancer (CRC) (finn-b-C3_COLORECTAL) and C1QB (eqtl-a-ENSG00000173369) were obtained from the Integrative Epidemiology Unit (IEU) Open GWAS database (https://gwas.mrcieu.ac.uk/). The CRC dataset included 16,380,466 single nucleotide polymorphisms (SNPs). There were 18,308 SNPs and 31,684 samples for C1QB. Utilizing the “Two Sample MR” R program (version 4.3.1), SNPs (instrumental variables (IVs)) were subsequently chosen (forward Mendelian randomization (MR): p < 5*10^8; reverse MR: p < 5*10^6) (Huang et al., 2023). Six independent SNPs closely associated with C1QB and 3 independent SNPs closely associated with CRC were subsequently filtered out (Supplementary Table S1). In forward MR, IVs of the exposure factor (C1QB, clump = TRUE, instrumental variable for linkage disequilibrium (LD) removed; r2 = 0.001; kb = 10,000) and outcome (CRC, proxy = TRUE; rsq = 0.8) were screened separately. The same procedure was applied for screening in reverse MR images. The dataset GSE87211, which is associated with colorectal cancer, was obtained from the Gene Expression Omnibus data database, and box-and-line plots were drawn to determine the differential expression of C1QB.
The three underlying hypotheses that served as the foundation for the MR investigation were as follows: 1) that there is a strong and remarkable relationship between IVs and exposure factors; 2) that IVs are not affected by confounders; and 3) that the impact of IVs on outcomes is solely determined by exposure factors, not other channels. To investigate the connection between C1QB and CRC, we used bidirectional MR analysis. There were five strategies to investigate MR: MR- Egger, inverse variance weighted (IVW), weighted median, simple mode and weighted mode. p < 0.05 was considered to indicate statistical significance in the MR analyses. Then, odds ratios (ORs) were computed, with ORs larger than 1 denoting a hazardous factor and ORs less than 1 denoting a protective factor. Scatter plots, forest plots, and funnel plots were created to display the results. Sensitivity analysis was carried out via tests for heterogeneity, horizontal pleiotropy and leave-one-out (LOO) effects to evaluate the dependability of the MR analysis results.
Bayesian colocalization analyses were used to assess the probability that two traits share the same causal variant using the “coloc” package (https://github.com/chr1swallace/coloc) with default arguments. To clarify how significant signaling loci affect outcomes, “coloc” was used to analyze the colocalization of CRC cells with C1QB. The joint analysis involves four hypothetical scenarios: The first scenario H0 suggests that phenotype 1 (GWAS) and phenotype 2 (e.g., eQTL) are not significantly associated with all SNP loci in a genomic region. The second scenario H1/H2 suggests that either phenotype 1 (GWAS) or phenotype 2 (e.g., eQTL) is significantly associated with SNP loci in a genomic region. The third scenario H3 suggests that both phenotype 1 (GWAS) and phenotype 2 (e.g., eQTL) are significantly associated with SNP loci in a genomic region, driven by different causal variant loci. The fourth scenario H4 suggests that both phenotype 1 (GWAS) and phenotype 2 (e.g., eQTL) are significantly associated with SNP loci in a genomic region, driven by the same causal variant locus. Based on these four scenarios, we aim to establish a higher probability for the fourth scenario H4 in statistical terms, enabling an explanation of how significant signal loci affect phenotypes. H0, H1/H2, H3, and H4 indicate that phenotypes 1 (GWAS) and two (in the case of eQTLs) are not substantially correlated with each other, are significantly correlated with each other but driven by distinct causal variant loci, are significantly correlated with each other and are driven by the same causal variant locus, respectively. The threshold of significance for colocalization was set at PP.H4 >0.75. The colocalization results were visualized via the “gwasglue” and “gassocplot” R programs (version 4.3.1).
Moreover, the protein-protein interaction (PPI) network of C1QB was constructed using a tool to search for recurring instances of neighboring genes (STRING, https://string-db.org) (confidence > 0.4). The Comparative Toxicogenomics Database (CTD, http://ctdbase.org/) was utilized to predict drugs that bind to C1QB. Cytoscape was used to display the PPI and drug prediction findings.
To further understand the effect of drug small molecules on C1QB chain and the druggability of target genes, this study further performed molecular docking at the atomic level. The 3D structures of the drugs were obtained from the PubChem Compound Database (https://pubchem.ncbi.nlm.nih.gov/), after which the energy needed to minimize further docking was minimized. The structure of C1QB chain (PDB ID:2wnv) was downloaded from the PDB (Protein Data Bank, http://www.rcsb.org/). The target includes ligand and water removal, hydrogen addition, and amino acid optimization and patching and were saved in pdbqt format. We subsequently used Autodock Vina 1.2.0 (http://autodock.scripps.edu/), a computerized protein–ligand docking software package, to examine and confirm the strength of the bond between the compound and target. The binding models were visualized with PyMol 2.3.0 software and Discovery Studio 3.5 software.
The thresholds were set at an inference score >50 to predict diseases connected to C1QB and C1QB-drug via the CTD, respectively. The intersecting diseases were identified via a Venn diagram to pinpoint the intersection of C1QB-related and C1QB drug-related diseases. Finally, the intersecting diseases were identified in the GWAS database, and MR analysis was carried out with C1QB as the exposure factor and intersecting diseases as the outcome. In the present MR analysis, the inverse variance weighted (IVW) method was used as the primary analysis to combine the variant-specific Wald estimators by taking the inverse of their approximate variances as the corresponding weights.
After screening, we selected six independent SNPs as IVs. The forward MR analyses are listed in Supplementary Table S2. The IVW findings revealed that C1QB (p = 0.033) was causally associated with CRC. Tracking of the ORs (OR = 1.104) revealed that C1QB was a risk factor for CRC (Figure 2). The other models did not reveal any significant associations. The scatter plot illustrated that the C1QB line’s slope was positive, indicating that an increase in C1QB led to an increased risk of CRC (Supplementary Figure S1A). According to the forest plot, when the MR effect size exceeded 0, C1QB was a risk factor for CRC (Supplementary Figure S1B). Additionally, the funnel plot showed that the forward-looking MR images adhered to Mendel’s second law of random grouping (Supplementary Figure S1C).
Figure 2. Forest plot of fiveMR estimators of the association between C1QB and CRC. Abbreviations: CRC, Colorectal cancer; MR, Mendelian randomization; OR, odds ratio; CI, confidence interval; SNPs, single-nucleotide polymorphisms.
After the screening of IVs, a total of 3 IVs (SNPs) were obtained. The reverse MR analyses are listed in Supplementary Table S3. Figure 2 illustrates that none of the five algorithms were statistically significant. The scatter, forest, and funnel diagrams of the reverse MR images also confirmed the IVW results (Supplementary Figures S1D–F).
Several tests were conducted to assess the precision of the analysis. There was no indication of heterogeneity in the sample, according to the Q-value of the heterogeneity test (for both forward and reverse MR images, the heterogeneity was greater than 0.05) (Supplementary Table S4). Similarly, there was no impact of horizontal pleiotropy between C1QB and CRC, according to the results of the test (forward MR: p = 0.747; reverse MR: p = 0.396) (Supplementary Table S4). LOO analysis supported IVW and demonstrated the accuracy of the MR analysis (Supplementary Figure S2).
By utilizing co-localization analysis, researchers can determine whether a particular SNP is responsible for both exposure and outcome, as long as the SNP is strongly linked to both variables. Studies have shown that proteins subjected to MR and colocalization testing may serve as potential drug targets. According to the findings of the colocalization study, there was a 78.08% probability that two characteristics (C1QB and CRC) were impacted by interlocking SNP mutations. Rs294180 and rs291985 corresponded to the same linkage interval and had the potential to influence C1QB and CRC, respectively (Figure 3). In addition, we did gene expression level analysis of C1QB in CRC and normal groups based on the dataset GSE87211, and found that CIQB expression was higher in the normal group (Supplementary Figure S3).
Figure 3. Co-localization analysis. The Results of co-localisation analysis based on eqtl−a−ENSG00000173369 and finn−b−C3_COLORECTAL with different coloured circles representing Recomb Rate.
C1QB was loaded into the STRING (https://cn.string-db.org/) database for network creation, and the resulting files were imported into Cytoscape for visualization. As shown in Figure 4A, the PPI analysis demonstrated that C1QB interacted with 10 proteins (C1QA, C1QC, C1R, C1S, C2, C4A, C4B, CALR, SERPING1, and VSIG4), whereas C1QA and C1QC interacted most strongly with C1QB. Drug prediction results demonstrated that twenty-one medications were associated with C1QB (Figure 4B). The binding affinity of the drug candidates for C1QB chain was assessed through molecular docking to gain a better understanding of the potential of the drug target for being targeted by drugs. The binding sites and interactions of the ten drug candidates with C1QB chain, which are encoded by their respective genes, were obtained using AutoDock Vina v.1.2.2. The binding energy for each interaction was also generated, achieving valid docking results using the prescribed drugs (Table 1). The greater the absolute value of the docking affinity was, the stronger the binding ability between the compound and the active site of the target was. According to the docking data, most of the binding complexes exhibited high binding affinities, with an average of −6.85 kcal/mol. The modes of the top four binding complexes are displayed in Figure 4C, and included C1QB-Benzo(a)pyrene docking (−9.5 kcal/mol), C1QB-1-naphthyl isothiocyanate docking (−7.3 kcal/mol), C1QB-calcitriol docking (−7.2 kcal/mol), and C1QB-medroxyprogesterone acetate (−7.2 kcal/mol). Benzo(a)pyrene exhibited the lowest binding energy, indicating extremely stable binding. Based on the molecular docking simulation results, four drugs that fit precisely into the same binding pocket of the target protein were observed. The four drugs all mainly interacted with the residues TYR:142 and LEU:220.
Figure 4. (A) PPI network built with STRING. The red circles represent C1QB, and the green rectangles represent the associated genes (B) Drug prediction results of C1QB. The red circle represents genes, and the blue rectangle represents drugs (C) Docking results of small molecules. C1QB docking Benzo(a)pyrene, C1QB docking 1-Naphthyl isothiocyanate, C1QB docking calcitriol, C1QB docking medroxyprogesterone acetate. The four drugs all mainly interacted with the residues TYR:142 and LEU:220.
We predicted 110 and 92 C1QB-related and C1QB drug-related diseases, respectively, with 29 diseases satisfying both the C1QB and C1QB-drug associations (Figures 5A–C). Using the GWAS database, and searching for GWAS id data related to 29 diseases, not all the diseases could be searched for relevant data. Based on the data retrieved, only weight loss, liver cirrhosis and nonalcoholic fatty liver disease were found among the nonneoplastic disease patients. We subsequently conducted Mendelian randomization analysis of C1QB for the three diseases, As shown in Figure 6, MR analyses revealed no causal role of C1QB in weight loss, liver cirrhosis or nonalcoholic fatty liver disease risk. MR analysis was subsequently performed for C1QB and 12 other cancers. Using the random model IVW, we found that C1QB seems to be negatively associated with ovarian cancer (OR = 0.9987, 95% CI = 0.9992–1.0003, p < 0.0023) and melanoma cancer (OR = 0.9988, 95% CI = 0.9980–0.9996, p < 0.0024) risk. Therefore, we conclude that as a therapeutic target for CRC, phenotypic scanning revealed that C1QB does not significantly affect weight loss, liver cirrhosis, or nonalcoholic fatty liver disease, but might have protective impacts on ovarian cancer and melanoma.
Figure 5. (A) Disease prediction results for C1QB. Red circles represent C1QB, green rectangles represent diseases (B) Disease prediction results of drugs. Green rectangles represent diseases, blue rectangles represent drugs (C) Venn diagram intersection of C1QB predicted diseases and drug predicted disease.
Figure 6. Associations of genetically predicted C1QB with predicted diseases. Abbreviations: CRC, Colorectal cancer; MR, Mendelian randomization; OR, odds ratio; CI, confidence interval; SNPs, single-nucleotide polymorphisms.
This study indicated that C1QB was a risk factor for CRC based on several MR methods (IVW, MR-Egger, weighted median, simple mode, weighted mode, horizontal multiplicity test and Cochran’s Q heterogeneity test), which are capable of eliminating any influencing variables during measurement. Additionally, colocalization served as significant supporting evidence. The above results guarantee and explain the reliability of the MR analysis results. By pinpointing small-molecule drugs with exceptional binding strength and beneficial interaction characteristics, we can prioritize four drugs (benzo(a)pyrene, 1-naphthyl isothiocyanate, calcitriol and medroxyprogesterone acetate) as specific target drugs for C1QB chain for further experimental validation. Additionally, a phenome-wide association analysis was conducted to demonstrate the potential pleiotropy of the target gene and the possible side effects of the drugs.
C1QB, together with C1QA and C1QC, controls the protein translation of C1q. C1QA, C1QB, and C1QC are three genes with strong interactions (Chen et al., 2011). Our PPI network also confirmed this point. Currently, many bioinformatics analyses have supported the impact of the three genes on tumor development and play protective or harmful roles in tumor progression. However, the effects of these three genes on different tumors are not entirely consistent. C1QB is associated with many cancers. According to the latest studies, the expression of C1QB was upregulated in CCRCC patients (Zhang et al., 2016), glioma patients (Mangogna et al., 2019b), gastric cancer patients (Jiang et al., 2020), CRC patients (Deng et al., 2022), PRCC(Mangogna et al., 2019a), and breast cancer (Mangogna et al., 2019a). And it was downregulated in nasopharyngeal carcinoma patients (Wu et al., 2020), skin cutaneous melanoma patients (Liang et al., 2022), lung adenocarcinoma (Mangogna et al., 2019a) and esophageal cancer (Yu et al., 2019). Moreover, high levels of C1QB expressions were associated with favorable prognosis in basal-like breast cancer (Mangogna et al., 2019a), HER2-positive breast cancer (Mangogna et al., 2019a), skin cutaneous melanoma patients (Liang et al., 2022), and osteosarcoma (Chen et al., 2021). And there was a negative correlation between higher levels of C1QB expression and unfavorable prognosis of patients with CCRCC(Mangogna et al., 2019a), lung adenocarcinoma (Mangogna et al., 2019a), glioma patients (Mangogna et al., 2019b), and gastric cancer patients (Jiang et al., 2020).
C1QB is the core promoter of the C1q molecule. An intact B chain is required for production and secretion of a serum C1q molecule (McAdam et al., 1988). The post transcriptional regulation of C1QB gene may affect the synthesis rate, stability and functional regulation of C1q protein, thus affecting the response process of the immune system (Yang et al., 2022). C1q is mainly produced by macrophages and dendritic cells (Verneret et al., 2014) and diffusely present in the stroma and vascular endothelium of several human malignant tumor (Bulla et al., 2016). C1q can get involved in a range of biological processes by binding to specific receptors, immune complexes, or other activators, such as angiogenesis (Bossi et al., 2014), immune modulation (Clarke and Tenner, 2014), and cell migration, adhesion, survival, and differentiation (Nayak et al., 2010). In malignant pleural mesothelioma, C1q can bind to hyaluronic acid (HA) exerts pro-tumorigenic effects (Agostinis et al., 2017b) and impacts on HA synthesis (Vidergar et al., 2021) and metabolism (Balduit et al., 2023). On the contrary, Hong et al. (2009) reported that C1q may induce apoptosis of prostate cancer cells by activating WOX1 and destabilizing cell adhesion, thus acting as an anti-tumor humoral factor. In ovarian cancer, Kaur et al. have reported that exogenous treatment with C1q and the recombinant globular head modules (gC1q) can induce apoptosis in SKOV3 cell line via TNF-α induced apoptosis pathway involving upregulation of Bax and Fas (Kaur et al., 2016). These rather two set of contradicting studies appear to suggest that the function of C1q in various tumor is complex and is strongly dependent on the TME. Recently, increasing studies based on scRNA-seq analysis have identified a distinct subset of tumor-associated macrophages (TAMs) that expresses C1q in various types of cancer. And the presence of C1q + TAM often correlates with poor prognosis. Proposed mechanisms by which C1q+ TAM drive cancer progression may correlate with exhausted T cells(Revel et al., 2022). In CRC, C1q + TAM can interact with T cell subsets via CXCL10-CXCR3 axis. This binding will activate and recruit T cells, favoring T cell exhaustion (Zhang et al., 2020). TAMs mainly originate from peripheral blood monocytes and tissue resident macrophages (Christofides et al., 2022). Thus, we infer that genetic variation in the C1QB may affect tissue infiltration and C1q secretion of peripheral blood monocytes, as well as the function of C1q interacting with different receptors that can influence CRC tumor progression.
With complement-targeted therapy becoming a hot topic in antitumor drug research following the detection of substantial amounts of this C component in the tumor microenvironment (Lu et al., 2021). C1q is capable of engaging a broad range of ligands via its globular domain (gC1q) which is composed of C-terminal halves of the A (ghA), B (ghB) and C (ghC) chains. Recent studies using recombinant forms of ghA, ghB and ghC have suggested that each module within the gC1q domain has a fair degree of structural and each chain can have differential ligand specificity (Kishore et al., 2004). Many C1q ligands have been found to interact in a significant way with different subunits of its globular domain (Kishore et al., 2004). In the resulting C1q model, the ghB lies on the outer part of the molecule, whereas A and C are positioned inside. It is very likely that this particular configuration has direct implications in terms of ligand recognition and C1 activation (Gaboriaud et al., 2011). For example, the ghB is considered as the principal IgG-binding module of C1q (Kishore et al., 2004). In this study, the drug target prediction and molecular docking experiments showed that the four ligands (benzo(a)pyrene, 1-naphthyl isothiocyanate, calcitriol and medroxyprogesterone acetate) were the most likely prospective drug to target the ghB. And the residues TYR:142 and LEU:220 have been implicated in the interaction. Benzo(a)pyrene, a polyaromatic hydrocarbon compound, is considered to be involved primarily in mutagenesis, carcinogenesis, and cancer metastasis promotion (Walle et al., 2006; Guo et al., 2015; Huang et al., 2020). Benzo(a)pyrene is also known to have xenoestrogenic action (Santodonato, 1997) and is currently considered to be an endocrine disruptor (Hoyer, 2001). Benzo(a)pyrene has been shown to induce apoptosis in intestinal porcine epithelial cells (Li et al., 2023a), neuronal cells (Nie et al., 2022), and endometrial cells (Yi et al., 2019). 1-Naphthyl isothiocyanate is the most widely used chemical for causing cholestasis both in vitro and in vivo according to toxicological studies (Li et al., 2016). It is secreted into bile by multidrug resistance-related proteins after hepatocyte metabolism and binding with glutathione, which has toxic effects on bile duct cells. There is currently no research on the relationship between 1-naphthyl isothiocyanate and cancer. Given the multiple side effects of benzo(a)pyrene and 1-naphthyl isothiocyanate, these agents may not be the preferred drugs for targeting C1q protein. Calcitriol is the most common biological metabolite derived from vitamin D. Deficiency of calcitriol is an epidemic that is predominantly caused by inadequate sun exposure. Calcitriol is widely used as a dietary supplement worldwide due to its beneficial effects on human health. Epidemiological studies have shown that reduced serum calcitriol levels are associated with an increased risk of CRC and a worse prognosis in CRC patients (Sluyter et al., 2021; Li et al., 2023b). Preclinical studies have revealed that the combined high-dose administration of calcitriol and certain chemotherapeutic drugs can potentiate their antitumor effects with mild side effects (Sookprasert et al., 2012; Ozgen et al., 2023). However, well-designed clinical trials are needed to optimize vitamin D administration as an anticancer therapeutic agent based on different levels of vitamin D (Henn et al., 2022). Numerous epidemiological and experimental studies have suggested the possible protective effect of medroxyprogesterone acetate on CRC risk (Chlebowski et al., 2004; Tanaka et al., 2008; Meijer et al., 2018). However, progestogens in continuous or sequential therapy used in women may be responsible for increased adverse effects both for breast cancer (Li et al., 2012), weight gain (Sims et al., 2020) and the cardiovascular system (Lizarelli et al., 2009).
This study has several advantages. First, genetic variants in C1QB associated with CRC were first screened, extracting data from the largest CRC risk GWAS available to the public. Second, multiple sensitivity analyses were performed to verify the dependability and coherence of the findings. Third, PPI analysis presented a promising avenue for the advancement of CRC medications via bypass. The high binding activity of the molecular docking data indicated the strong potential of C1QB as a drug target. Calcitriol may have the greatest advantage in targeting C1QB among the four drugs due to its fewer side effects.
Our study is not without limitations. There is an inconsistency between the results of MR and the analysis of the dataset GSE87211. Huiming Deng et al. reported there were significant differences in the mRNA expression of C1QA, C1QB, and C1QC in the TCGA colon cancer dataset. Meanwhile, the immunohistochemical staining results showed that the expression score of C1QC in CRC tissues was higher than that in non-cancer tissue. Kaplan-Meier survival analysis showed that high C1QC expression was significantly correlated with poor overall survival, which is consistent with our MR results (Deng et al., 2022). The reasons for consistent results may be as follows. Firstly, these gene variations not only affect the exposure factor CIQB, but may also directly or indirectly affect the outcome CRC, deviating from the actual causal relationship. Secondly, unique genetic regulatory mechanisms may exist among various tissues and the reliance on blood eQTLs for MR testing might not offer a thorough understanding of the illness and available treatment options. Thirdly, the dataset GSE87211 may contain a wider range of environmental and socio-economic factors, as well as differences in demographic variables such as race, gender, and age, leading to differences in results. Our research focused exclusively on European populations to establish genetic uniformity, this inevitably led to some limitations of the findings. Therefore, additional research and validation are needed to extend the findings to other ethnic groups with unique genetic backgrounds and confirm their wider relevance. Finally, the precision of molecular docking analysis does not necessarily translate to its effectiveness in clinical applications. Further research and verification are needed.
In conclusion, after performing MR analysis, we found that genetically predicted inhibition of C1QB may reduce the risk of CRC, which was supported by colocalization analysis. Relevant studies support the antitumor function of C1QB, suggesting that it is a potential drug target for CRC treatment. Additionally, drug prediction and molecular docking were used to validate the medicinal value of C1QB. These findings could lead to more effective CRC treatments, potentially reducing drug development costs and advancing personalized medicine approaches. However, MR analysis revealed that targeted inhibition of C1QB may increase the risk of ovarian cancer and melanoma. Further research and clinical trials on drugs targeting C1QB are warranted.
The data presented in the study are deposited in the IEU Open GWAS repository (https://gwas.mrcieu.ac.uk/datasets/), accession number: finn-b-C3_COLORECTAL and eqtl-a-ENSG00000173369.
MJ: Conceptualization, Software, Writing–original draft. YC: Writing–original draft. XQ: Formal Analysis, Writing–original draft. XL: Data curation, Writing–review and editing. JL: Writing–original draft. CG: Supervision, Visualization, Writing–original draft. HT: Funding acquisition, Writing–review and editing.
The author(s) declare that financial support was received for the research, authorship, and/or publication of this article. This work was funded by National Natural Science Foundation of China Grants (No. 82105044).
The authors sincerely thank the researchers and participants of the original GWAS for collecting and managing the large-scale data resources.
The authors declare that the research was conducted in the absence of any commercial or financial relationships that could be construed as a potential conflict of interest.
All claims expressed in this article are solely those of the authors and do not necessarily represent those of their affiliated organizations, or those of the publisher, the editors and the reviewers. Any product that may be evaluated in this article, or claim that may be made by its manufacturer, is not guaranteed or endorsed by the publisher.
The Supplementary Material for this article can be found online at: https://www.frontiersin.org/articles/10.3389/fgene.2024.1403509/full#supplementary-material
Agostinis, C., Tedesco, F., and Bulla, R. (2017a). Alternative functions of the complement protein C1q at embryo implantation site. J. Reprod. Immunol. 119, 74–80. doi:10.1016/j.jri.2016.09.001
Agostinis, C., Vidergar, R., Belmonte, B., Mangogna, A., Amadio, L., Geri, P., et al. (2017b). Complement protein C1q binds to hyaluronic acid in the malignant pleural mesothelioma microenvironment and promotes tumor growth. Front. Immunol. 8, 1559. doi:10.3389/fimmu.2017.01559
Balduit, A., Vidergar, R., Zacchi, P., Mangogna, A., Agostinis, C., Grandolfo, M., et al. (2023). Complement protein C1q stimulates hyaluronic acid degradation via gC1qR/HABP1/p32 in malignant pleural mesothelioma. Front. Immunol. 14, 1151194. doi:10.3389/fimmu.2023.1151194
Bossi, F., Tripodo, C., Rizzi, L., Bulla, R., Agostinis, C., Guarnotta, C., et al. (2014). C1q as a unique player in angiogenesis with therapeutic implication in wound healing. Proc. Natl. Acad. Sci. U.S.A. 111 (11), 4209–4214. doi:10.1073/pnas.1311968111
Bulla, R., Tripodo, C., Rami, D., Ling, G. S., Agostinis, C., Guarnotta, C., et al. (2016). C1q acts in the tumour microenvironment as a cancer-promoting factor independently of complement activation. Nature Commun. 7 (1), 10346. doi:10.1038/ncomms10346
Chen, G., Tan, C. S., Teh, B. K., and Lu, J. (2011). Molecular mechanisms for synchronized transcription of three complement C1q subunit genes in dendritic cells and macrophages. J. Biol. Chem. 286 (40), 34941–34950. doi:10.1074/jbc.M111.286427
Chen, L. H., Liu, J. F., Lu, Y., He, X. Y., Zhang, C., and Zhou, H. H. (2021). Complement C1q (C1qA, C1qB, and C1qC) may Be a potential prognostic factor and an index of tumor microenvironment remodeling in osteosarcoma. Front. Oncol. 11, 642144. doi:10.3389/fonc.2021.642144
Chlebowski, R. T., Wactawski-Wende, J., Ritenbaugh, C., Hubbell, F. A., Ascensao, J., Rodabough, R. J., et al. (2004). Estrogen plus progestin and colorectal cancer in postmenopausal women. N. Engl. J. Med. 350 (10), 991–1004. doi:10.1056/NEJMoa032071
Christofides, A., Strauss, L., Yeo, A., Cao, C., Charest, A., and Boussiotis, V. A. (2022). The complex role of tumor-infiltrating macrophages. Nat. Immunol. 23 (8), 1148–1156. doi:10.1038/s41590-022-01267-2
Clarke, E. V., and Tenner, A. J. (2014). Complement modulation of T cell immune responses during homeostasis and disease. J. Leukoc. Biol. 96 (5), 745–756. doi:10.1189/jlb.3MR0214-109R
Deng, H., Chen, Y., Liu, Y., Liu, L., and Xu, R. (2022). Complement C1QC as a potential prognostic marker and therapeutic target in colon carcinoma based on single-cell RNA sequencing and immunohistochemical analysis. Bosn. J. Basic Med. Sci. 22 (6), 912–922. doi:10.17305/bjbms.2022.7309
Gaboriaud, C., Frachet, P., Thielens, N. M., and Arlaud, G. J. (2011). The human c1q globular domain: structure and recognition of non-immune self ligands. Front. Immunol. 2, 92. doi:10.3389/fimmu.2011.00092
Ghebrehiwet, B., Zaniewski, M., Fernandez, A., DiGiovanni, M., Reyes, T. N., Ji, P., et al. (2024). The C1q and gC1qR axis as a novel checkpoint inhibitor in cancer. Front. Immunol. 15, 1351656. doi:10.3389/fimmu.2024.1351656
Guo, J., Xu, Y., Ji, W., Song, L., Dai, C., and Zhan, L. (2015). Effects of exposure to benzo[a]pyrene on metastasis of breast cancer are mediated through ROS-ERK-MMP9 axis signaling. Toxicol. Lett. 234 (3), 201–210. doi:10.1016/j.toxlet.2015.02.016
Henn, M., Martin-Gorgojo, V., and Martin-Moreno, J. M. (2022). Vitamin D in cancer prevention: gaps in current knowledge and room for hope. Nutrients 14 (21), 4512. doi:10.3390/nu14214512
Holmes, M. V., Ala-Korpela, M., and Smith, G. D. (2017). Mendelian randomization in cardiometabolic disease: challenges in evaluating causality. Nat. Rev. Cardiol. 14 (10), 577–590. doi:10.1038/nrcardio.2017.78
Hong, Q., Sze, C. I., Lin, S. R., Lee, M. H., He, R. Y., Schultz, L., et al. (2009). Complement C1q activates tumor suppressor WWOX to induce apoptosis in prostate cancer cells. PLoS One 4 (6), e5755. doi:10.1371/journal.pone.0005755
Hossain, M. S., Karuniawati, H., Jairoun, A. A., Urbi, Z., Ooi, J., John, A., et al. (2022). Colorectal cancer: a review of carcinogenesis, global Epidemiology, current challenges, risk factors, preventive and treatment strategies. Cancers (Basel) 14 (7), 1732. doi:10.3390/cancers14071732
Hoyer, P. B. (2001). Reproductive toxicology: current and future directions. Biochem. Pharmacol. 62 (12), 1557–1564. doi:10.1016/s0006-2952(01)00814-0
Huang, L., Xiao, X., Yao, Y., Yu, J., Chen, Q., Liang, P., et al. (2020). Benzo[a]pyrene promotes progression in tongue squamous cell carcinoma. Oral Dis. 26 (8), 1649–1658. doi:10.1111/odi.13489
Huang, P., Zhang, P. F., and Li, Q. (2023). Causal relationship between cannabis use and cancer: a genetically informed perspective. J. Cancer Res. Clin. Oncol. 149 (11), 8631–8638. doi:10.1007/s00432-023-04807-x
Jiang, J., Ding, Y., Wu, M., Lyu, X., Wang, H., Chen, Y., et al. (2020). Identification of TYROBP and C1QB as two novel key genes with prognostic value in gastric cancer by network analysis. Front. Oncol. 10, 1765. doi:10.3389/fonc.2020.01765
Kaur, A., Sultan, S. H., Murugaiah, V., Pathan, A. A., Alhamlan, F. S., Karteris, E., et al. (2016). Human C1q induces apoptosis in an ovarian cancer cell line via tumor necrosis factor pathway. Front. Immunol. 7, 599. doi:10.3389/fimmu.2016.00599
Kishore, U., Ghai, R., Greenhough, T. J., Shrive, A. K., Bonifati, D. M., Gadjeva, M. G., et al. (2004). Structural and functional anatomy of the globular domain of complement protein C1q. Immunol. Lett. 95 (2), 113–128. doi:10.1016/j.imlet.2004.06.015
Li, C. I., Beaber, E. F., Tang, M. T., Porter, P. L., Daling, J. R., and Malone, K. E. (2012). Effect of depo-medroxyprogesterone acetate on breast cancer risk among women 20 to 44 years of age. Cancer Res. 72 (8), 2028–2035. doi:10.1158/0008-5472.CAN-11-4064
Li, J., Bai, J., Si, X., Jia, H., and Wu, Z. (2023a). Benzo[a]pyrene induces epithelial tight junction disruption and apoptosis via inhibiting the initiation of autophagy in intestinal porcine epithelial cells. Chem. Biol. Interact. 374, 110386. doi:10.1016/j.cbi.2023.110386
Li, J., Qin, S., Zhang, S., Lu, Y., Shen, Q., Cheng, L., et al. (2023b). Serum vitamin D concentration, vitamin D-related polymorphisms, and colorectal cancer risk. Int. J. Cancer. 153 (2), 278–289. doi:10.1002/ijc.34521
Li, X., Liu, R., Yu, L., Yuan, Z., Sun, R., Yang, H., et al. (2016). Alpha-naphthylisothiocyanate impairs bile acid homeostasis through AMPK-FXR pathways in rat primary hepatocytes. Toxicology. 370, 106–115. doi:10.1016/j.tox.2016.09.020
Liang, Z., Pan, L., Shi, J., and Zhang, L. (2022). C1QA, C1QB, and GZMB are novel prognostic biomarkers of skin cutaneous melanoma relating tumor microenvironment. Sci. Rep. 12 (1), 20460. doi:10.1038/s41598-022-24353-9
Liu, L., Zeng, P., Xue, F., Yuan, Z., and Zhou, X. (2021). Multi-trait transcriptome-wide association studies with probabilistic Mendelian randomization. Am. J. Hum. Genet. 108 (2), 240–256. doi:10.1016/j.ajhg.2020.12.006
Lizarelli, P. M., Martins, W. P., Vieira, C. S., Soares, G. M., Franceschini, S. A., Ferriani, R. A., et al. (2009). Both a combined oral contraceptive and depot medroxyprogesterone acetate impair endothelial function in young women. Contraception 79 (1), 35–40. doi:10.1016/j.contraception.2008.07.024
Lu, J., and Kishore, U. (2017). C1 complex: an adaptable proteolytic module for complement and non-complement functions. Front. Immunol. 8, 592. doi:10.3389/fimmu.2017.00592
Lu, P., Ma, Y., Wei, S., and Liang, X. (2021). The dual role of complement in cancers, from destroying tumors to promoting tumor development. Cytokine 143, 155522. doi:10.1016/j.cyto.2021.155522
Mangogna, A., Agostinis, C., Bonazza, D., Belmonte, B., Zacchi, P., Zito, G., et al. (2019a). Is the complement protein C1q a pro- or anti-tumorigenic factor? Bioinformatics analysis involving human carcinomas. Front. Immunol. 10, 865. doi:10.3389/fimmu.2019.00865
Mangogna, A., Belmonte, B., Agostinis, C., Zacchi, P., Iacopino, D. G., Martorana, A., et al. (2019b). Prognostic implications of the complement protein C1q in gliomas. Front. Immunol. 10, 2366. doi:10.3389/fimmu.2019.02366
McAdam, R. A., Goundis, D., and Reid, K. B. (1988). A homozygous point mutation results in a stop codon in the C1q B-chain of a C1q-deficient individual. Immunogenetics 27 (4), 259–264. doi:10.1007/BF00376120
Meijer, B. J., Wielenga, M. C. B., Hoyer, P. B., Amos-Landgraf, J. M., Hakvoort, T. B. M., Muncan, V., et al. (2018). Colorectal tumor prevention by the progestin medroxyprogesterone acetate is critically dependent on postmenopausal status. Oncotarget. 9 (55), 30561–30567. doi:10.18632/oncotarget.25703
Morgan, E., Arnold, M., Gini, A., Lorenzoni, V., Cabasag, C. J., Laversanne, M., et al. (2023). Global burden of colorectal cancer in 2020 and 2040: incidence and mortality estimates from GLOBOCAN. Gut. 72 (2), 338–344. doi:10.1136/gutjnl-2022-327736
Nayak, A., Ferluga, J., Tsolaki, A. G., and Kishore, U. (2010). The non-classical functions of the classical complement pathway recognition subcomponent C1q. Immunol. Lett. 131 (2), 139–150. doi:10.1016/j.imlet.2010.03.012
Nayak, A., Pednekar, L., Reid, K. B., and Kishore, U. (2012). Complement and non-complement activating functions of C1q: a prototypical innate immune molecule. Innate Immun. 18 (2), 350–363. doi:10.1177/1753425910396252
Nie, J., Zhang, Y., Ning, L., Yan, Z., Duan, L., Xi, H., et al. (2022). Phosphorylation of p53 by Cdk5 contributes to benzo[a]pyrene-induced neuronal apoptosis. Environ. Toxicol. 37 (1), 17–27. doi:10.1002/tox.23374
Ozgen, O., Ozen Eroglu, G., Kucukhuseyin, O., Akdeniz, N., Hepokur, C., Kuruca, S., et al. (2023). Vitamin D increases the efficacy of cisplatin on bladder cancer cell lines. Mol. Biol. Rep. 50 (1), 697–706. doi:10.1007/s11033-022-08044-2
Revel, M., Sautes-Fridman, C., Fridman, W. H., and Roumenina, L. T. (2022). C1q+ macrophages: passengers or drivers of cancer progression. Trends Cancer. 8 (7), 517–526. doi:10.1016/j.trecan.2022.02.006
Santodonato, J. (1997). Review of the estrogenic and antiestrogenic activity of polycyclic aromatic hydrocarbons: relationship to carcinogenicity. Chemosphere. 34 (4), 835–848. doi:10.1016/s0045-6535(97)00012-x
Sims, J., Lutz, E., Wallace, K., Kassahun-Yimer, W., Ngwudike, C., and Shwayder, J. (2020). Depo-medroxyprogesterone acetate, weight gain and amenorrhea among obese adolescent and adult women. Eur. J. Contracept. Reprod. Health Care. 25 (1), 54–59. doi:10.1080/13625187.2019.1709963
Sluyter, J. D., Manson, J. E., and Scragg, R. (2021). Vitamin D and clinical cancer outcomes: a review of meta-analyses. JBMR Plus. 5 (1), e10420. doi:10.1002/jbm4.10420
Son, M., Diamond, B., and Santiago-Schwarz, F. (2015). Fundamental role of C1q in autoimmunity and inflammation. Immunol. Res. 63 (1-3), 101–106. doi:10.1007/s12026-015-8705-6
Sookprasert, A., Pugkhem, A., Khuntikeo, N., Chur-in, S., Chamadol, N., Prawan, A., et al. (2012). Evaluation of efficacy, safety and tolerability of high dose-intermittent calcitriol supplementation to advanced intrahepatic cholangiocarcinoma patients--a pilot study. Asian Pac J. Cancer Prev. 13 (Suppl. l), 161–167.
Sung, H., Ferlay, J., Siegel, R. L., Laversanne, M., Soerjomataram, I., Jemal, A., et al. (2021). Global cancer statistics 2020: GLOBOCAN estimates of incidence and mortality worldwide for 36 cancers in 185 countries. CA Cancer J. Clin. 71 (3), 209–249. doi:10.3322/caac.21660
Tanaka, Y., Kato, K., Mibu, R., Uchida, S., Asanoma, K., Hashimoto, K., et al. (2008). Medroxyprogesterone acetate inhibits proliferation of colon cancer cell lines by modulating cell cycle-related protein expression. Menopause. 15 (3), 442–453. doi:10.1097/gme.0b013e318156fb77
Verneret, M., Tacnet-Delorme, P., Osman, R., Awad, R., Grichine, A., Kleman, J. P., et al. (2014). Relative contribution of c1q and apoptotic cell-surface calreticulin to macrophage phagocytosis. J. Innate Immun. 6 (4), 426–434. doi:10.1159/000358834
Vidergar, R., Balduit, A., Zacchi, P., Agostinis, C., Mangogna, A., Belmonte, B., et al. (2021). C1q-HA matrix regulates the local synthesis of hyaluronan in malignant pleural mesothelioma by modulating HAS3 expression. Cancers (Basel). 13 (3), 416. doi:10.3390/cancers13030416
Walle, T., Walle, U. K., Sedmera, D., and Klausner, M. (2006). Benzo[A]pyrene-induced oral carcinogenesis and chemoprevention: studies in bioengineered human tissue. Drug Metab. Dispos. 34 (3), 346–350. doi:10.1124/dmd.105.007948
Wu, Z. H., Zhou, T., and Sun, H. Y. (2020). DNA methylation-based diagnostic and prognostic biomarkers of nasopharyngeal carcinoma patients. Med. Baltim. 99 (24), e20682. doi:10.1097/MD.0000000000020682
Yang, H., Che, D., Gu, Y., and Cao, D. (2022). Prognostic and immune-related value of complement C1Q (C1QA, C1QB, and C1QC) in skin cutaneous melanoma. Front. Genet. 13, 940306. doi:10.3389/fgene.2022.940306
Yi, T., Liu, M., Li, X., Liu, X., Ding, Y., He, J., et al. (2019). Benzo(a)pyrene inhibits endometrial cell apoptosis in early pregnant mice via the WNT5A pathway. J. Cell Physiol. 234 (7), 11119–11129. doi:10.1002/jcp.27762
Yu, D., Ruan, X., Huang, J., Hu, W., Chen, C., Xu, Y., et al. (2019). Comprehensive analysis of competitive endogenous RNAs network, being associated with esophageal squamous cell carcinoma and its emerging role in head and neck squamous cell carcinoma. Front. Oncol. 9, 1474. doi:10.3389/fonc.2019.01474
Zhang, L., Jiang, H., Xu, G., Chu, N., Xu, N., Wen, H., et al. (2016). iTRAQ-based quantitative proteomic analysis reveals potential early diagnostic markers of clear-cell Renal cell carcinoma. Biosci. Trends. 10 (3), 210–219. doi:10.5582/bst.2016.01055
Keywords: C1QB, colorectal cancer, drug target Mendelian randomization, molecular docking, phenotype scanning
Citation: Jiao M, Cui Y, Qiu X, Liang X, Li J, Guo C and Tian H (2024) Causal relationship between complement C1QB and colorectal cancer: a drug target Mendelian randomization study. Front. Genet. 15:1403509. doi: 10.3389/fgene.2024.1403509
Received: 22 March 2024; Accepted: 02 July 2024;
Published: 23 July 2024.
Edited by:
Rui Medeiros, Portuguese Oncology Institute of Porto (IPO Porto)/Porto Comprehensive Cancer Centre (Porto.CCC), PortugalReviewed by:
Youjie Zeng, Central South University, ChinaCopyright © 2024 Jiao, Cui, Qiu, Liang, Li, Guo and Tian. This is an open-access article distributed under the terms of the Creative Commons Attribution License (CC BY). The use, distribution or reproduction in other forums is permitted, provided the original author(s) and the copyright owner(s) are credited and that the original publication in this journal is cited, in accordance with accepted academic practice. No use, distribution or reproduction is permitted which does not comply with these terms.
*Correspondence: Congcong Guo, Z3VvY29uZ2NvbmdAc2RmbXUuZWR1LmNu; Hu Tian, dGlhbmh1NjU4NUAxNjMuY29t
Disclaimer: All claims expressed in this article are solely those of the authors and do not necessarily represent those of their affiliated organizations, or those of the publisher, the editors and the reviewers. Any product that may be evaluated in this article or claim that may be made by its manufacturer is not guaranteed or endorsed by the publisher.
Research integrity at Frontiers
Learn more about the work of our research integrity team to safeguard the quality of each article we publish.