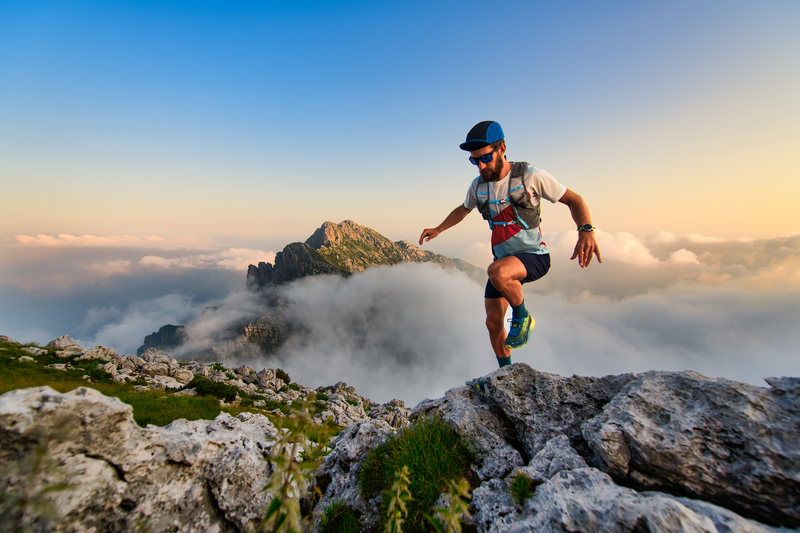
94% of researchers rate our articles as excellent or good
Learn more about the work of our research integrity team to safeguard the quality of each article we publish.
Find out more
ORIGINAL RESEARCH article
Front. Genet. , 02 September 2024
Sec. Genetics of Aging
Volume 15 - 2024 | https://doi.org/10.3389/fgene.2024.1392061
Introduction: Large genome-wide association studies (GWASs) using case–control study designs have now identified tens of loci associated with ischemic stroke (IS). As a complement to these studies, we performed GWAS in a case-only design to identify loci influencing the age at onset (AAO) of ischemic stroke.
Methods: Analyses were conducted in a discovery cohort of 10,857 ischemic stroke cases using a linear regression framework. We meta-analyzed all SNPs with p-value <1 x 10−5 in a sexcombined or sex-stratified analysis using summary data from two additional replication cohorts.
Results: In the women-only meta-analysis, we detected significant evidence for the association of AAO with rs429358, an exonic variant in apolipoprotein E (APOE) that encodes for the APOE-Є4 allele. Each copy of the rs429358:T>C allele was associated with a 1.29-year earlier stroke AAO (meta p-value = 2.48 x 10−11). This APOE variant has previously been associated with increased mortality and ischemic stroke AAO. We hypothesized that the association with AAO may reflect a survival bias attributable to an age-related decrease in mortality among APOE-Є4 carriers and have no association to stroke AAO per se. A simulation study showed that a variant associated with overall mortality might indeed be detected with an AAO analysis. A variant with a 2-fold increase in mortality risk would lead to an observed effect of AAO that is comparable to what we found.
Discussion: In conclusion, we detected a robust association of the APOE locus with stroke AAO and provided simulations to suggest that this association may be unrelated to ischemic stroke per se but related to a general survival bias.
Genetic association analysis and, in particular, genome-wide genetic association studies (GWASs) have become standard tools for identifying genetic contributions to complex diseases, including ischemic stroke (IS). Although such studies are typically framed as case–control study designs, case-only designs have also been used, for example, for the purpose of identifying variants that are associated with the age at disease onset, i.e., timing of disease. Such associations may reflect variants that predispose to earlier forms of disease or modify the effects of other disease-predisposing variants. Case-only age at onset association analyses have been carried out on multiple traits, including Alzheimer’s disease (Naj et al., 2014), Parkinson’s disease (Blauwendraat et al., 2019), and stroke (Lagging et al., 2019). Case–control approaches that condition on clinical covariates, such as the age at onset (AAO), have also been used to boost the power to detect risk loci for complex diseases (Zaitlen et al., 2012; Traylor et al., 2014).
To identify variants associated with ischemic stroke AAO, we performed a two-stage meta-analysis of GWAS for AAO in 10,857 stroke cases from the Stroke Genetics Network (SiGN) (Meschia et al., 2013), followed by a replication of all associated SNPs (p-value <5 × 10−5) in two independent studies, FinnGen Data Freeze 57 and the Women’s Health Initiative (WHI) (The WHI Study Group, 1998). We performed sex-combined and sex-stratified analyses. From these analyses, we identified a variant in the apolipoprotein E (APOE) locus, encoding the ApoE-Є4 allele, that was significantly associated with earlier AAO in women (rs429358:T>C, meta p-value = 2.48 × 10−11, beta = 1.29 ± 0.38 years) but not in men. This SNP has previously been associated with stroke AAO in a prior candidate gene study (Lagging et al., 2019), yet, it is not associated with the risk of ischemic stroke (OR = 1.0, 95% confidence interval [CI], 0.97–1.03, and p = 0.97; ncases = 33,936 vs ncontrols = 391,114 in European populations (Malik et al., 2018)). Thus, we hypothesized that the association with earlier stroke AAO may reflect an overall association of this variant with earlier death (Pilling et al., 2017). To test this hypothesis, we performed a simulation study in which we simulated loci that are associated with overall mortality unrelated to stroke to characterize parameters that would lead to age-related differences in allele frequencies that could be misinterpreted as being related to the age at disease onset.
A summary of the characteristics of the discovery (SiGN) and follow-up replication cohorts (FinnGen Data Freeze 5 and WHI) is shown in Table 1. The mean age of stroke onset was 67.6, 66.7, and 76.6 years, in SiGN, FinnGen, and WHI (the latter includes only women), respectively.
Table 1. Characteristics of the study populations. Percentages have been calculated on the subset of individuals with no missing values for the variable in question. Hypertension: as derived from multiple administrative registers. CAD, coronary artery disease. T2D, type 2 diabetes. DF5, FinnGen Data Freeze 57. Stratification was based on self-reported sex.
In the SiGN discovery cohorts, genome-wide association analyses revealed 61 individual loci associated with stroke AAO in the sex-combined analysis at a significance threshold of p <1.0 × 10−5 (see Methods), 144 individual loci in the men-only analysis, and 37 individual loci in the women-only analysis (Supplementary Figure S1; Supplementary Figure S2). There was one genome-wide significant hit (rs6051656) in the men-only analyses in SiGN spanning a 14-kb region at chromosome 20:364,295–378,978 near TRIB3 (Supplementary Figure S3; Supplementary Table S1), which did not replicate in the men-only meta-analysis of SiGN with FinnGen (Supplementary Table S1). Further meta-analyses of all SNPs associated at p <1.0 × 10−5 in SiGN with FinnGen did not yield genome-wide significant results for the sex-combined analysis but did reveal a genome-wide significant association in the women-only analysis with rs429358 on chromosome 19 at the APOE locus (pmeta,SiGN-FinnGen-value = 2.4 × 10−8, beta = −1.63 years ±0.29; Table 2; Supplementary Figure S4). This SNP was further replicated in the WHI (pmeta,SiGN-FinnGen-WHI = 2.48 × 10−11, beta = −1.29 ± 0.38). APOE-rs429358 is associated with stroke AAO in both sexes combined, although the magnitude of association is stronger in women than in men (unequal variance t-test, p = 4.3 × 10−4; Figure 1). Conditional analysis (using the SiGN summary statistics) indicated no secondary associated SNPs at this locus.
Table 2. Meta-analysis results, total sample and sex-specific, of the association of APOE-rs429358 (chr19:45,411,941, b37) with the ischemic stroke age at onset in discovery (SiGN) and replication (FinnGen and WHI) cohorts. The sample size is for the total sample and for men/women. The alleles are the effect (C) allele and other (T) allele with the corresponding effect allele frequency (EAF). The beta (effect size) and se (standard error) correspond to the difference in the age at onset (in years) associated with each copy of the risk allele (C). As the WHI includes only women, the results are presented for “women-only.”
Figure 1. Association of APOE rs429358 with the age at stroke onset in women (red), men (blue), and sex-combined meta-analyses. Point estimate represents the effect of each copy of the minor (C) allele on the AAO (in years); 95% confidence intervals are indicated by error bars.
APOE rs429358 is a missense variant for which the minor allele, C, changes the amino acid at the 112th position of the ApoE protein from a cysteine to an arginine, thus altering the protein conformation. In combination with a second SNP (rs7412:C>T) in APOE, rs429358 encodes the ApoE isoform of an individual, with the rs429358-C allele associated with the ApoE-Є4 allele. The ApoE-Є4 allele (frequency of 0.16 in SiGN) has been associated with numerous adverse health outcomes, including hyperlipidemia (Khalil et al., 2021) and lipid metabolism (Graham et al., 2021), Alzheimer’s disease (Serrano-Pozo et al., 2021) and dementia, and coronary disease (Aragam et al., 2022). However, this variant is neither associated with ischemic stroke susceptibility in MEGASTROKE (OR = 1.00; 95% CI: 0.96–1.03; p = 0.77) nor with any other ischemic stroke subtype (Supplementary Table S2) (Malik et al., 2018).
An association of APOE rs429358 with stroke AAO has been reported previously (Deelen et al., 2011), and this variant has also been associated with longevity (Pilling et al., 2017) and the age of parental death (Lew, 1985). These observations, coupled with the association we observed between APOE rs429358 and stroke AAO, albeit genome-wide significant in women only, prompted us to investigate via simulation whether the stroke AAO association could be a manifestation of a survival bias attributable to a higher overall mortality among ApoE-Є4 carriers.
We simulated a population of individuals who were followed from birth until death based on age-specific mortality rates obtained from the Social Security Administration Actuarial Life Tables (Figure 2) (Lew, 1985). Birthdates for the simulated subjects were randomly drawn between 1 January 1900 and 1 January 2020. Each individual was assigned a genotype for three SNPs, GISmultiplicative, GISadditive, and Gdeath. GISmultiplicative and GISadditive increased the risk of ischemic stroke only, and Gdeath increased the risk of death only but not through IS. Stroke was assumed to increase the risk of death as a function of the time since the event. We performed association analyses for each simulated SNP and two phenotypes: logistic regression of case–control status and linear regression of the age at onset. Methods provides the simulation details.
Figure 2. Data generating model for the simulation study. Five variables were generated at birth (DOB, GENO, and SEX), and the subsequent risks of stroke and death were estimated annually. The date of death and date of stroke (if applicable) are outputted from the model. The genotypic effect varied from 1.0 (null) to 2.0 by increments of 0.1. The probability draw parameters for the Markov chain Monte Carlo simulations are given with B(p|c) notation, indicating a draw from the binomial distribution (B) with a probability (p) of stroke, given the conditions (C), i.e., sex, age, and geno(types).
As expected, both the additive and multiplicative loci simulated to influence the risk of stroke were identifiable via a case–control design (1,000 cases and 1,000 controls), with power increasing with larger effect sizes (Figure 3A). Whether they were associated with AAO depended on the amount of risk conferred by the allele (Figure 3B). Loci with a relative increase in risk were not associated with AAO at all, but those with an additive increase in risk saw proportionally more strokes at early ages, and thus, the risk allele was associated with a lower AAO of IS. The simulated locus that was associated with mortality via mechanisms unrelated to stroke was not associated with stroke risk. However, that locus was associated with the AAO. A locus with a 2-fold increase in mortality would display an association with a ∼1.5-year decrease in the age at onset, an effect size similar to that identified for the APOE locus in our GWAS. In other words, our results indicate that the observed SNP association in women is biased by an association with earlier death, assuming that the SNP effect is indeed independent of ischemic stroke.
Figure 3. Estimated power to detect an association between the genotype and stroke according to the effect size for variants associated with age at death (green), additive effect on stroke susceptibility (red), and multiplicative effect on stroke susceptibility (blue) for (left) case–control analysis and (right) age-at-onset analysis. The x-axis shows the effect size in the relative risk for a given genetic variant. The y-axis shows the power, given a genetic effect size.
We found that APOE-rs429358, encoding the ApoE-Є4 haplotype, is associated with an earlier age at ischemic stroke in women. Although this SNP has not been associated with an increased risk of ischemic stroke in previous GWASs, there are several lines of evidence supporting that the association we observed with stroke AAO is real. First, this association is statistically robust and replicated in two independent populations. Second, this SNP has been associated previously in a candidate gene study with stroke AAO (Gerdes et al., 2000; Sebastiani et al., 2012; Brooks-Wilson, 2013). Third, this SNP is biologically compelling as it encodes the ApoE-Є4 allele, which has been associated previously with a shorter lifespan (Mahley, 2016) and several common age-related conditions, including Alzheimer’s disease (Wilson et al., 1996; Bennet et al., 2007; Mahley, 2016; Li et al., 2021) and ischemic heart disease (Garatachea et al., 2014; Caruso et al., 2022).
Although the association between rs429358 and ischemic stroke AAO appears to be robust, the interpretation of this association can be debated. One possibility is that this variant, while not associated with stroke susceptibility per se, is associated with the age at which stroke occurs in individuals more likely (either through genetic or environmental factors) to experience a stroke. A second possibility is that the association of this variant with stroke AAO is a more general consequence of the APOE risk allele at this locus being associated with a shortened lifespan in general, i.e., an age-related decrease in the frequency of the risk allele. Our simulation study supports the latter interpretation. In other words, the association we detected between APOE and stroke AAO may be due to a survival bias. Our simulations suggest that a locus exerting a two-fold increase in mortality would also be associated with a ∼1.5-year decrease in the age at onset, an effect size similar to that identified for the APOE locus in our GWAS and is consistent with previous findings (Martin et al., 2021).
Although previous simulation studies have assessed the power of AAO studies (Gamache et al., 2020), these previous studies have failed to account for the competing risk of mortality and potential bias that may play a role in these studies. Our simulations utilize a prospective data generating model, even though a retrospective case–control or case-only analytic model is fit to the data. This allows our simulations to estimate associations (either OR from a case–control study or year difference in AAO studies) that are more likely to represent those found in the natural population.
The reason for the predominantly larger effect on stroke AAO we observed among females compared to male stroke cases is not apparent. Possible explanations include a disproportionate effect of ApoE-Є4 on survival in women compared to men, a direct effect of ApoE-Є4 on stroke AAO, or chance. Modest differences in the associations of the APOE genotype have been reported between men and women for ischemic heart disease (Joshi et al., 2016), Alzheimer’s disease (Corbo and Scacchi, 1999), and lifespan (Finch and Morgan, 2007; Yassine and Finch, 2020), although the reasons underlying these differences are not known. An alternative hypothesis may be more plausible and seems supported by our simulations and GWAS results. It is thought that ApoE-Є4 is the proinflammatory ancestral allele in humans and adaptive to reducing mortality under conditions of infections, food scarcity, and (paradoxically) a shortened life expectancy (NINDS Stroke Genetics Network et al., 2016; Caruso et al., 2022). Several lines of evidence support this notion. ApoE-Є4 shows less severe liver damage during hepatitis C infections, the allele frequency remained high in populations where food was scarce until recently, and the allele is associated with higher circulating cholesterol levels (Caruso et al., 2022). As the human population expanded and thrived, the ApoE-Є3 and ApoE-Є2 alleles spread, while the ApoE-Є4 allele was maintained by balancing selection (NINDS Stroke Genetics Network et al., 2016; Caruso et al., 2022). Yet, as conditions for our species continued to improve, diets and lifespan changed, thus rendering ApoE-Є4 susceptible to cardiovascular disease (CVD) and more pronounced effects in women (Caruso et al., 2022).
Although there is no doubt that the APOE locus is an important determinant of atherosclerosis, the largest GWAS, to date, of stroke and its subtypes has revealed no evidence of an association of this locus with stroke risk (Malik et al., 2018). Moreover, our simulation analyses suggest that a plausible explanation of the association of this locus with stroke AAO can be entirely explained by survival bias, an explanation that is entirely consistent with the robust association reported by multiple studies of the APOE locus with lifespan.
Strengths of our study include the large number of well-characterized ischemic stroke cases with a wide range of ages at onset, as well as the prospective design of our simulation study. Nevertheless, our study is not without limitations. One notable result is that the effect size in WHI women (−1.02) is in the same direction but smaller in magnitude, possibly reflecting the fact these women, on average, experienced stroke onset at substantially older ages with a higher prevalence of hypertension and lower current smoking than the other female participants and the men. True as this may be, if anything, the overall meta-analysis effect estimate is smaller, which does not preclude the main interpretation that results are consistent despite variations across studies. However, foremost among the limitations, none of the studies included in our meta-analysis were birth cohorts, so only individuals who survived until the age of their recruitment are part of our GWAS. If case-fatality rates differed between early- and late-onset strokes, then variants specifically associated with early (or late)-onset stroke could go undetected. Likewise, our study may be affected by an ascertainment bias as cases were drawn from case–control studies, i.e., people were ascertained on a phenotype that may introduce confounding effects, such as some strokes that are more likely to be detected by clinicians or the severity of stroke. However, the replication in FinnGen sidesteps this issue because people are randomly ascertained in this study.
In conclusion, we detected a robust association of the APOE locus with stroke AAO and provided simulations to suggest that this association may be unrelated to ischemic stroke per se but related to a general survival bias.
Analyses were performed on subjects of the European ancestry from SiGN, FinnGen study, and WHI. SiGN is an international collaboration that includes ischemic stroke cases recruited from multiple sites in the United States and Europe (United Kingdom, Poland, Belgium, Spain, Austria, and Sweden) (Meschia et al., 2013). The discovery phase of this analysis included 10,857 SiGN participants, while the replication phase included 8,124 participants from FinnGen Data Freeze 532 and 3,415 participants from the WHI (Wassertheil-Smoller et al., 2003). Participants provided (written) informed consent, and all studies upheld the ethical standards according to the Declaration of Helsinki.
For each study included in the SiGN dataset, ischemic stroke was confirmed by neuroimaging. Details, including inclusion criteria, for each study are given in the original SiGN GWAS publication (Adams et al., 1993). In the WHI, all incident strokes, other vascular events, and deaths were identified through a self-report at annual (OS) and semi-annual (CT) participant contacts and through third-party reports by family members and proxies. Medical records were obtained for potential strokes, and adjudication was performed by trained physician adjudicators who assigned a diagnosis. Stroke diagnosis requiring and/or occurring during hospitalization was based on the rapid onset of a neurological deficit attributable to an obstruction or rupture of an arterial vessel system. The deficit was not known to be secondary to brain trauma, tumor, infection, or other causes and must have lasted more than 24 h, unless death supervened or a lesion compatible with acute stroke was evident on computed tomography or a magnetic resonance imaging scan (NINDS Stroke Genetics Network et al., 2016). Strokes were classified as ischemic, hemorrhagic, or unknown/missing. Ischemic stroke subtypes were further classified using Trial of Org 10172 in Acute Stroke Treatment (TOAST) criteria (Regier et al., 2018).
The SiGN and WHI participants were genotyped on different Illumina platforms. The dataset was divided into different study strata based on similar genetic ancestry and genotyping platforms. Genotypes that were not measured were imputed against 1000G phase 3 for SiGN (Chang et al., 2015) and TOPMed for WHI (1000 Genomes Project Consortium et al., 2015). The FinnGen cohort and methods are comprehensively described elsewhere (Kurki et al., 2023).
Genome-wide analyses of stroke AAO were carried out in SiGN using BOLT-LMM (1000 Genomes Project Consortium et al., 2015). We used PLINK 1.938 to “hard call” and subset the imputed SNPs to build the genetic relationship matrix (GRM). SNPs with an INFO <0.8, genotyping rate <95%, or missing genotype rate >5% were excluded from the analysis. Covariates in the association analysis included the study stratum, the GRM to correct for subtle population stratification, and sex (in the sex-combined analysis). After the analysis, we filtered out SNPs with minimum minor allele frequency (MAF) <5%.
To identify independent signals for the three main analyses in the discovery, we used PLINK 1.9 with 1000G phase 1 (version 3) as a reference (1000 Genomes Project Consortium et al., 2015). We set the minimal p-value threshold at 0.05 and defined a clumped region as ±500 kb, with a minimum linkage disequilibrium r2 at 0.05 (--clump-p1 5e-8 --clump-p2 0.05 --clump-kb 500 --clump-r2 0.05 --clump-best --clump-verbose). To identify independent signals to take forward in the replication phase, we included clumps at p < 1.0 × 10−5 (--clump-p1 1e-5 --clump-p2 0.05 --clump-kb 500 --clump-r2 0.05 --clump-best --clump-verbose).
We used GCTA-COJO (Yang et al., 2012) to investigate whether there were additional associated SNPs at the discovered loci. We used the stepwise model selection procedure (--cojo-slct) and used the imputed genotype data (converted to :hard call,” as described for the GWAS) as input (--bfile).
We performed a look-up of SNPs that were associated at p < 1.0 × 10−5 in any of the three analyses (sex-combined, men-only, and women-only) in FinnGen and the Women’s Health Initiative (women-only and for rs429358 only) (Winkler et al., 2015). Meta-analyses of SiGN with FinnGen were performed in METAL using the inverse-variance weighted approach (Lew, 1985). A p-value threshold of p < 5 × 10−8 was considered to be significant in the meta-analysis. Baseline characteristics for the replication datasets are given in Table 1.
To test how likely the differences in effect size between the women and men analyses are, under the null hypothesis of no difference, we used a t-test for unequal variance. The test we used is similar to Welch’s t-test, but we additionally corrected for Spearman’s rank correlation r between all women and men effect sizes (filtered on MAF >0.05 and INFO >0.8)40; r was equal to 0.018.
The data generating model is shown in Figure 1. Pseudo-men and women were simulated, drawing a date of birth at random from 1 January 1900 to 1 January 2020. Each pseudo-individual was followed over the course of 120 years or until their death, whichever came first. At birth, genotypes were assigned at three loci, each having a minor allele frequency of 10%. Two genotypes, GenoStrokeMult and GenoStrokeAdd, incurred a risk on stroke only, and the other, GenoDeath, incurred a risk on death via an unspecified pathway independent of stroke. The annual stroke risk was a function of sex, age, and genotypes given by
The genetic effect of the genotype,
The resulting annual mortality risk was given by
The given data generating model resulted in observations with seven features: date of birth, sex,
Two genotypic models were simulated. The first modeled a constant relative risk over the lifespan, given by
Given the above data generating model, it is trivial to determine the age at stroke for each pseudo-individual (date of stroke–date of birth). The target parameter was defined as the difference in the age of stroke between genotypes among cases.
Estimates of this target parameter were obtained using linear regression controlling for sex to approximate a common GWAS strategy. Genotypes were coded as 0, 1, and 2 to estimate the additive genetic model. Models were run for each of the simulated loci separately.
The data presented in the study are deposited in the GWAS Catalog repository, accession numbers GCST90301368, GCST90301369, and GCST90301370.
For the studies involved in the SiGN consortium and the Women Health Initiative the respective instititional Ethics Committees approved the studies. These studies were conducted in accordance with the local legislation and institutional requirements. The participants provided their written informed consent to participate in this study. Patients and control subjects in FinnGen provided informed consent for biobank research, based on the Finnish Biobank Act. Alternatively, separate research cohorts, collected prior the Finnish Biobank Act came into effect (in September 2013) and start of FinnGen (August 2017), were collected based on study-specific consents and later transferred to the Finnish biobanks after approval by Fimea, the National Supervisory Authority for Welfare and Health. Recruitment protocols followed the biobank protocols approved by Fimea. The Coordinating Ethics Committee of the Hospital District of Helsinki and Uusimaa (HUS) approved the FinnGen study protocol Nr HUS/990/2017. The FinnGen study is approved by Finnish Institute for Health and Welfare (THL), approval number THL/2031/6.02.00/2017, amendments THL/1101/5.05.00/2017, THL/341/6.02.00/2018, THL/2222/6.02.00/2018, THL/283/6.02.00/2019, THL/1721/5.05.00/2019, Digital and population data service agency VRK43431/2017-3, VRK/6909/2018-3, VRK/4415/2019-3 the Social Insurance Institution (KELA) KELA 58/522/2017, KELA 131/522/2018, KELA 70/522/2019, KELA 98/522/2019, and Statistics Finland TK-53-1041-17. The Biobank Access Decisions for FinnGen samples and data utilized in FinnGen Data Freeze 5 include: THL Biobank BB2017_55, BB2017_111, BB2018_19, BB_2018_34, BB_2018_67, BB2018_71, BB2019_7, BB2019_8, BB2019_26, Finnish Red Cross Blood Service Biobank 7.12.2017, Helsinki Biobank HUS/359/2017, Auria Biobank AB17-5154, Biobank Borealis of Northern Finland_2017_1013, Biobank of Eastern Finland 1186/2018, Finnish Clinical Biobank Tampere MH0004, Central Finland Biobank 1-2017, and Terveystalo Biobank STB 2018001.
JvB: conceptualization, data curation, formal analysis, investigation, methodology, supervision, visualization, writing–original draft, and writing–review and editing. PM: conceptualization, formal analysis, methodology, writing–original draft, and writing–review and editing. PH: resources and writing–review and editing. JH: resources and writing–review and editing. CK: resources and writing–review and editing. RL: resources and writing–review and editing. AP: resources and writing–review and editing. VT: resources and writing–review and editing. SP: conceptualization, data curation, formal analysis, funding acquisition, investigation, methodology, project administration, resources, supervision, visualization, writing–original draft, and writing–review and editing. SK: conceptualization, resources, and writing–review and editing. BM: conceptualization, resources, and writing–review and editing. JdR: data curation, investigation, methodology, supervision, and writing–review and editing. SWvdL: conceptualization, formal analysis, methodology, project administration, resources, supervision, visualization, writing–original draft, and writing–review and editing.
Utilizing a case-only GWAS design, we identified a significant association between the APOE locus, specifically the rs429358 variant, and AAO of ischemic stroke. This exonic variant in APOE, linked to the APOE-Є4 allele, exhibited a compelling correlation, with each copy of the allele associated with a 1.29-year earlier stroke onset. Notably, our findings, supported by a robust discovery cohort of 10,857 ischemic stroke cases and reinforced through meta-analysis with replication cohorts, shed light on a potential survival bias influencing the AAO. Although APOE-Є4 has previously been linked to increased mortality and stroke AAO, our simulation study suggests that this association may be independent of ischemic stroke itself, emphasizing the need for a nuanced interpretation in understanding the genetic factors influencing the age at onset in stroke cases.
The author(s) declare that financial support was received for the research, authorship, and/or publication of this article. This work was supported by NIH grants R01 NS100178 and R01 NS105150 from the US National Institutes of Health. JdR was supported by a Vidi fellowship (639.072.715) from the Dutch Organization for Scientific Research (Nederlandse Organisatie voor Wetenschappelijk Onderzoek, NWO). SK was additionally supported by the Department of Veterans Affairs RR&D N1699-R and BX004672-01A1. SWvdL was funded through EU H2020 TO_AITION (grant number: 848146), EU HORIZON NextGen (grant number: 101136962), EU HORIZON MIRACLE (grant number: 101115381), and Health-Holland PPP Allowance “Getting the Perfect Image.” The Women’s Health Initiatives (WHI) program was funded by the National Heart, Lung, and Blood Institute; National Institutes of Health; US Department of Health and Human Services, through 75N92021D00001, 75N92021D00002, 75N92021D00003, 75N92021D00004, and 75N92021D00005. The FinnGen project was funded by two grants from Business Finland (HUS 4685/31/2016 and UH 4386/31/2016) and the following industry partners: AbbVie Inc., AstraZeneca United Kingdom Ltd.; Biogen MA Inc.; Bristol Myers Squibb (and Celgene Corporation & Celgene International II Sàrl); Genentech Inc.; Merck Sharp & Dohme LCC, Pfizer Inc.; GlaxoSmithKline Intellectual Property Development Ltd.; Sanofi US Services Inc.; Maze Therapeutics Inc., Janssen Biotech Inc.; Novartis AG; and Boehringer Ingelheim International GmbH. The FinnGen project was not involved in the study design, collection, analysis, interpretation of data, the writing of this article, or the decision to submit it for publication.
The authors thank the Netherlands CardioVascular Research Initiative of the Netherlands Heart Foundation (CVON 2011/B019 and CVON 2017-20: Generating the best evidence-based pharmaceutical targets for atherosclerosis [GENIUS I&II]), the ERA-CVD program “druggable-MI-targets” (grant number: 01KL1802), the Leducq Foundation “PlaqOmics,” (grant number: 101136962). The authors thank the participants and investigators of the FinnGen study. The authors thank the following biobanks for delivering biobank samples to FinnGen: Auria Biobank (www.auria.fi/biopankki), THL Biobank (www.thl.fi/biobank), Helsinki Biobank (www.helsinginbiopankki.fi), Biobank Borealis of Northern Finland (https://www.ppshp.fi/Tutkimus-ja-opetus/Biopankki/Pages/Biobank-Borealis-briefly-in-English.aspx), Finnish Clinical Biobank Tampere (www.tays.fi/en-US/Research_and_development/Finnish_Clinical_Biobank_Tampere), Biobank of Eastern Finland (www.ita-suomenbiopankki.fi/en), Central Finland Biobank (www.ksshp.fi/fi-FI/Potilaalle/Biopankki), Finnish Red Cross Blood Service Biobank (www.veripalvelu.fi/verenluovutus/biopankkitoiminta), Terveystalo Biobank (www.terveystalo.com/fi/Yritystietoa/Terveystalo-Biopankki/Biopankki/), and Arctic Biobank (https://www.oulu.fi/en/university/faculties-and-units/faculty-medicine/northern-finland-birth-cohorts-and-arctic-biobank). All Finnish biobanks are members of the BBMRI.fi infrastructure (www.bbmri.fi). Finnish Biobank Cooperative FINBB (https://finbb.fi/) is the coordinator of BBMRI-ERIC operations in Finland. The authors thank SP for supervision and mentoring during the initiation of this study.
SWvdL received funding from Roche Diagnostics International Ltd for unrelated work. JdR is co-founder and director of Cyclomics, a genomics company.
The remaining authors declare that the research was conducted in the absence of any commercial or financial relationships that could be construed as a potential conflict of interest.
The author(s) declared that they were an editorial board member of Frontiers, at the time of submission. This had no impact on the peer review process and the final decision
All claims expressed in this article are solely those of the authors and do not necessarily represent those of their affiliated organizations, or those of the publisher, the editors, and the reviewers. Any product that may be evaluated in this article, or claim that may be made by its manufacturer, is not guaranteed or endorsed by the publisher.
The Supplementary Material for this article can be found online at: https://www.frontiersin.org/articles/10.3389/fgene.2024.1392061/full#supplementary-material
1000 Genomes Project Consortium Auton, A., Brooks, L. D., Durbin, R. M., Garrison, E. P., Kang, H. M., et al. (2015). A global reference for human genetic variation. Nature 526 (7571), 68–74. doi:10.1038/nature15393
Adams, H. P., Bendixen, B. H., Kappelle, L. J., Biller, J., Love, B. B., Gordon, D. L., et al. (1993). Classification of subtype of acute ischemic stroke. Definitions for use in a multicenter clinical trial. TOAST. Trial of Org 10172 in Acute Stroke Treatment. Stroke 24, 35–41. doi:10.1161/01.str.24.1.35
Aragam, K. G., Jiang, T., Goel, A., Kanoni, S., Wolford, B. N., Atri, D. S., et al. (2022). Discovery and systematic characterization of risk variants and genes for coronary artery disease in over a million participants. Nat. Genet. 54, 1803–1815. doi:10.1038/s41588-022-01233-6
Bennet, A. M., Di Angelantonio, E., Ye, Z., Wensley, F., Dahlin, A., Ahlbom, A., et al. (2007). Association of apolipoprotein E genotypes with lipid levels and coronary risk. JAMA 298, 1300–1311. doi:10.1001/jama.298.11.1300
Blauwendraat, C., Heilbron, K., Vallerga, C. L., Bandres-Ciga, S., von Coelln, R., Pihlstrøm, L., et al. (2019). Parkinson’s disease age at onset genome-wide association study: defining heritability, genetic loci, and α-synuclein mechanisms. Mov. Disord. 34, 866–875. doi:10.1002/mds.27659
Brooks-Wilson, A. R. (2013). Genetics of healthy aging and longevity. Hum. Genet. 132, 1323–1338. doi:10.1007/s00439-013-1342-z
Caruso, C., Ligotti, M. E., Accardi, G., Aiello, A., Duro, G., Galimberti, D., et al. (2022). How important are genes to achieve longevity? Int. J. Mol. Sci. 23, 5635. doi:10.3390/ijms23105635
Chang, C. C., Chow, C. C., Tellier, L. C., Vattikuti, S., Purcell, S. M., and Lee, J. J. (2015). Second-generation PLINK: rising to the challenge of larger and richer datasets. Gigascience 4, 7. doi:10.1186/s13742-015-0047-8
Corbo, R. M., and Scacchi, R. (1999). Apolipoprotein E (APOE) allele distribution in the world. Is APOE*4 a “thrifty” allele? Ann. Hum. Genet. 63, 301–310. doi:10.1046/j.1469-1809.1999.6340301.x
Deelen, J., Beekman, M., Uh, H. W., Helmer, Q., Kuningas, M., Christiansen, L., et al. (2011). Genome-wide association study identifies a single major locus contributing to survival into old age; the APOE locus revisited. Aging Cell. 10, 686–698. doi:10.1111/j.1474-9726.2011.00705.x
Finch, C. E., and Morgan, T. E. (2007). Systemic inflammation, infection, ApoE alleles, and Alzheimer disease: a position paper. Curr. Alzheimer Res. 4, 185–189. doi:10.2174/156720507780362254
Gamache, J., Yun, Y., and Chiba-Falek, O. (2020). Sex-dependent effect of APOE on Alzheimer’s disease and other age-related neurodegenerative disorders. Dis. Model. Mech. 13, dmm045211. doi:10.1242/dmm.045211
Garatachea, N., Emanuele, E., Calero, M., Fuku, N., Arai, Y., Abe, Y., et al. (2014). ApoE gene and exceptional longevity: insights from three independent cohorts. Exp. Gerontol. 53, 16–23. doi:10.1016/j.exger.2014.02.004
Gerdes, L. U., Jeune, B., Ranberg, K. A., Nybo, H., and Vaupel, J. W. (2000). Estimation of apolipoprotein E genotype-specific relative mortality risks from the distribution of genotypes in centenarians and middle-aged men: apolipoprotein E gene is a “frailty gene,” not a “longevity gene.”. Genet. Epidemiol. 19, 202–210. doi:10.1002/1098-2272(200010)19:3<202::AID-GEPI2>3.0.CO;2-Q
Graham, S. E., Clarke, S. L., Wu, K. H. H., Kanoni, S., Zajac, G. J. M., Ramdas, S., et al. (2021). The power of genetic diversity in genome-wide association studies of lipids. Nature 600, 675–679. doi:10.1038/s41586-021-04064-3
Joshi, P. K., Fischer, K., Schraut, K. E., Campbell, H., Esko, T., and Wilson, J. F. (2016). Variants near CHRNA3/5 and APOE have age- and sex-related effects on human lifespan. Nat. Commun. 7, 11174. doi:10.1038/ncomms11174
Khalil, Y. A., Rabès, J.-P., Boileau, C., and Varret, M. (2021). APOE gene variants in primary dyslipidemia. Atherosclerosis 328, 11–22. doi:10.1016/j.atherosclerosis.2021.05.007
Kurki, M. I., Karjalainen, J., Palta, P., Sipilä, T. P., Kristiansson, K., Donner, K. M., et al. (2023). FinnGen provides genetic insights from a well-phenotyped isolated population. Nature 613, 508–518. doi:10.1038/s41586-022-05473-8
Lagging, C., Lorentzen, E., Stanne, T. M., Pedersen, A., Söderholm, M., Cole, J. W., et al. (2019). APOE ε4 is associated with younger age at ischemic stroke onset but not with stroke outcome. Neurology 93, 849–853. doi:10.1212/WNL.0000000000008459
Lew, E. A. (1985). Actuarial contributions to life table analysis. Natl. Cancer Inst. Monogr. 67, 29–36.
Li, M., Zhao, J. V., Kwok, M. K., and Schooling, C. M. (2021). Age and sex specific effects of APOE genotypes on ischemic heart disease and its risk factors in the UK Biobank. Sci. Rep. 11, 9229. doi:10.1038/s41598-021-88256-x
Mahley, R. W. (2016). Apolipoprotein E: from cardiovascular disease to neurodegenerative disorders. J. Mol. Med. 94, 739–746. doi:10.1007/s00109-016-1427-y
Malik, R., Chauhan, G., Traylor, M., Sargurupremraj, M., Okada, Y., Mishra, A., et al. (2018). Multiancestry genome-wide association study of 520,000 subjects identifies 32 loci associated with stroke and stroke subtypes. Nat. Genet. 50, 524–537. doi:10.1038/s41588-018-0058-3
Martin, E. R., Gao, X. R., and Li, Y.-J. (2021). An exploration of genetic association tests for disease risk and age at onset. Genet. Epidemiol. 45, 249–279. doi:10.1002/gepi.22368
Meschia, J. F., Arnett, D. K., Ay, H., Brown, R. D., Benavente, O. R., Cole, J. W., et al. (2013). Stroke Genetics Network (SiGN) study: design and rationale for a genome-wide association study of ischemic stroke subtypes. Stroke 44, 2694–2702. doi:10.1161/STROKEAHA.113.001857
Naj, A. C., Jun, G., Reitz, C., Kunkle, B. W., Perry, W., Park, Y. S., et al. (2014). Effects of multiple genetic loci on age at onset in late-onset Alzheimer disease: a genome-wide association study. JAMA Neurol. 71, 1394–1404. doi:10.1001/jamaneurol.2014.1491
NINDS Stroke Genetics Network (SiGN), (2016). Loci associated with ischaemic stroke and its subtypes (SiGN): a genome-wide association study. Lancet Neurol. 15, 174–184. doi:10.1016/S1474-4422(15)00338-5
Pilling, L. C., Kuo, C. L., Sicinski, K., Tamosauskaite, J., Kuchel, G. A., Harries, L. W., et al. (2017). Human longevity: 25 genetic loci associated in 389,166 UK biobank participants. Aging (Albany NY) 9, 2504–2520. doi:10.18632/aging.101334
Regier, A. A., Farjoun, Y., Larson, D. E., Krasheninina, O., Kang, H. M., Howrigan, D. P., et al. (2018). Functional equivalence of genome sequencing analysis pipelines enables harmonized variant calling across human genetics projects. Nat. Commun. 9, 4038. doi:10.1038/s41467-018-06159-4
Sebastiani, P., Solovieff, N., Dewan, A. T., Walsh, K. M., Puca, A., Hartley, S. W., et al. (2012). Genetic signatures of exceptional longevity in humans. PLoS One 7, e29848. doi:10.1371/journal.pone.0029848
Serrano-Pozo, A., Das, S., and Hyman, B. T. (2021). APOE and Alzheimer’s disease: advances in genetics, pathophysiology, and therapeutic approaches. Lancet Neurol. 20, 68–80. doi:10.1016/S1474-4422(20)30412-9
The WHI Study Group (1998). Design of the Women’s health initiative clinical trial and observational study. The Women’s health initiative study Group. Control. Clin. Trials 19, 61–109. doi:10.1016/s0197-2456(97)00078-0
Traylor, M., Mäkelä, K. M., Kilarski, L. L., Holliday, E. G., Devan, W. J., Nalls, M. A., et al. (2014). A novel MMP12 locus is associated with large artery atherosclerotic stroke using a genome-wide age-at-onset informed approach. PLoS Genet. 10, e1004469. doi:10.1371/journal.pgen.1004469
Wassertheil-Smoller, S., Hendrix, S. L., Limacher, M., Heiss, G., Kooperberg, C., Baird, A., et al. (2003). Effect of estrogen plus progestin on stroke in postmenopausal women: the Women’s Health Initiative: a randomized trial. JAMA 289, 2673–2684. doi:10.1001/jama.289.20.2673
Wilson, P. W., Schaefer, E. J., Larson, M. G., and Ordovas, J. M. (1996). Apolipoprotein E alleles and risk of coronary disease. A meta-analysis. Arterioscler. Thromb. Vasc. Biol. 16, 1250–1255. doi:10.1161/01.atv.16.10.1250
Winkler, T. W., Justice, A. E., Graff, M., Barata, L., Feitosa, M. F., Chu, S., et al. (2015). The influence of age and sex on genetic associations with adult body size and shape: a large-scale genome-wide interaction study. PLoS Genet. 11, e1005378. doi:10.1371/journal.pgen.1005378
Yang, J., Ferreira, T., Morris, A. P., Medland, S. E., et al. (2012). Conditional and joint multiple-SNP analysis of GWAS summary statistics identifies additional variants influencing complex traits. Nat. Genet. 44, 369–S3. doi:10.1038/ng.2213
Yassine, H. N., and Finch, C. E. (2020). APOE alleles and diet in brain aging and Alzheimer’s disease. Front. Aging Neurosci. 12, 150. doi:10.3389/fnagi.2020.00150
Keywords: genome-wide association study, stroke, age at onset, APOE, atherosclerosis
Citation: von Berg J, McArdle PF, Häppölä P, Haessler J, Kooperberg C, Lemmens R, Pezzini A, Thijs V, Pulit SL, Kittner SJ, Mitchell BD, de Ridder J and van der Laan SW (2024) Evidence of survival bias in the association between APOE-Є4 and age at ischemic stroke onset. Front. Genet. 15:1392061. doi: 10.3389/fgene.2024.1392061
Received: 26 February 2024; Accepted: 18 June 2024;
Published: 02 September 2024.
Edited by:
Paolina Crocco, University of Calabria, ItalyReviewed by:
Yin Wang, China Medical University, ChinaCopyright © 2024 von Berg, McArdle, Häppölä, Haessler, Kooperberg, Lemmens, Pezzini, Thijs, Pulit, Kittner, Mitchell, de Ridder, van der Laan and FinnGen. This is an open-access article distributed under the terms of the Creative Commons Attribution License (CC BY). The use, distribution or reproduction in other forums is permitted, provided the original author(s) and the copyright owner(s) are credited and that the original publication in this journal is cited, in accordance with accepted academic practice. No use, distribution or reproduction is permitted which does not comply with these terms.
*Correspondence: Sander W. van der Laan, cy53LnZhbmRlcmxhYW4tMkB1bWN1dHJlY2h0Lm5s
†ORCID: Sander W. van der Laan, orcid.org/0000-0001-6888-1404
Disclaimer: All claims expressed in this article are solely those of the authors and do not necessarily represent those of their affiliated organizations, or those of the publisher, the editors and the reviewers. Any product that may be evaluated in this article or claim that may be made by its manufacturer is not guaranteed or endorsed by the publisher.
Research integrity at Frontiers
Learn more about the work of our research integrity team to safeguard the quality of each article we publish.