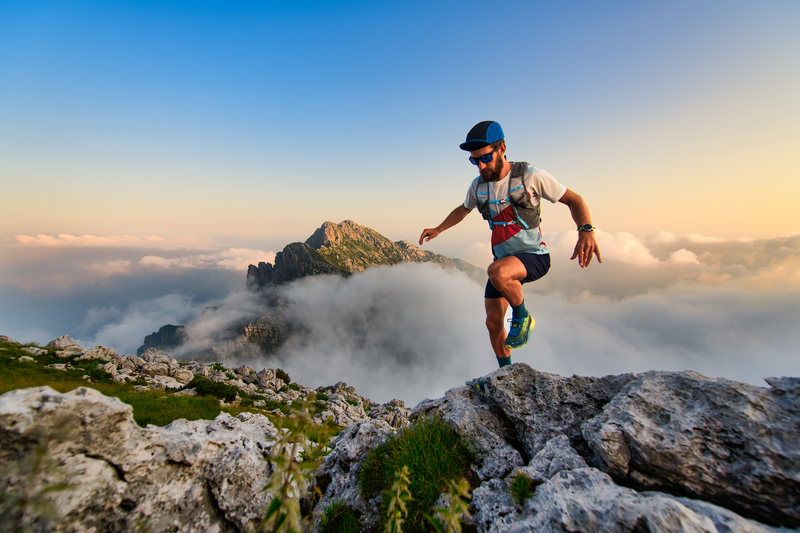
95% of researchers rate our articles as excellent or good
Learn more about the work of our research integrity team to safeguard the quality of each article we publish.
Find out more
ORIGINAL RESEARCH article
Front. Genet. , 17 June 2024
Sec. Behavioral and Psychiatric Genetics
Volume 15 - 2024 | https://doi.org/10.3389/fgene.2024.1380544
Introduction: Sleep is associated with psychiatric disorders. However, their causality remains unknown.
Methods: The study explored the causal relationship between seven sleep parameters (sleep duration, insomnia, sleep apnea, chronotype, daytime dozing, napping during the day, and snoring) and three psychiatric disorders including major depressive disorder (MDD), schizophrenia, and attention-deficit/hyperactivity disorder (ADHD) using two-sample Mendelian randomization (MR). Genome-wide association study (GWAS) summary data for sleep parameters were obtained from the United Kingdom biobank, FinnGen biobank, and EBI databases. MR-Egger, weighted median, inverse-variance weighted (IVW), simple mode, weighted mode, maximum likelihood, penalized weighted median, and IVW(fixed effects) were used to perform the MR analysis. The heterogeneity was detected by Cochran’s Q statistic. The horizontal pleiotropy was detected by MR Egger. The sensitivity was investigated by the leave-one-out analysis.
Results: Insomnia (OR = 2.02, 95%CI = 1.34–3.03, p = 0.001, False-discovery rate (FDR) corrected p-value = 0.011) and napping during the day (OR = 1.81, 95%CI = 1.34–2.44, FDR corrected p-value<0.001) were associated with an increased risk of MDD. Longer sleep duration (OR = 2.20, 95%CI = 1.24–3.90, FDR corrected p-value = 0.049) had an association with the increased risk of schizophrenia, while daytime dozing (OR = 4.44, 95%CI = 1.20–16.41, corrected p-value = 0.088)and napping during the day (OR = 2.11, 95%CI = 1.11–4.02, FDR corrected p-value = 0.088) had a suggestive association with an increased risk of schizophrenia. Longer sleep duration had a suggestive association with a decreased risk of ADHD (OR = 0.66, 95%CI = 0.42–0.93, FDR corrected p-value = 0.088).
Conclusion: This study provides further evidence for a complex relationship between sleep and psychiatric disorders. Our findings highlight the potential benefits of addressing sleep problems in the prevention of psychiatric disorders.
Psychiatric disorders represent a significant public health concern, as they are implicated in approximately 70% of suicides (Baldessarini and Tondo, 2020; Pooja et al., 2021). Among these, major depressive disorder (MDD) has been the most common psychiatric disorder, affecting around 163 million people worldwide (Hashmi et al., 2022). Meanwhile, 73 million people were affected by attention-deficit/hyperactivity disorder (ADHD) and 19 million people suffered from schizophrenia (GBD, 2017 Disease and Injury Incidence and Prevalence Collaborators, 2018), bringing huge burdens. Psychiatric disorders, prominently MDD, ADHD, and schizophrenia, impose significant public health challenges, affecting millions globally. The profound impacts of these conditions on both personal and societal levels underscore the critical need for identifying modifiable risk factors that can be targeted through therapeutic interventions (Murray et al., 2012).
Psychiatric disorders are closely associated with sleep (Sutton, 2014), including individual sleep dimensions (e.g., sleep duration, sleep quality, and sleep timing) and sleep disturbances (e.g., insomnia, sleep apnea). On the one hand, sleep disruptions (e.g., insomnia or excessive sleep) are the defining features and diagnostic criteria of depression (Battle, 2013). Based on an epidemiological study conducted in the US, among individuals with depression, 92% reported at least one type of sleep-related complaint, 85% experienced insomnia, and 48% experienced hypersomnia (Geoffroy et al., 2018). On the other hand, sleep disturbances may serve as potential risk factors for the onset of psychiatric disorders. According to a prospective cohort study, insomnia symptoms were predictors of depression, (Dong and Yang, 2019). Therapeutic interventions targeting sleep disturbances (e.g., insomnia and sleep apnea) alleviated symptoms related to depression (Hertenstein et al., 2022), schizophrenia (Mylonas et al., 2020), and ADHD (Liu et al., 2023). Given these insights, our study has meticulously selected seven sleep parameters: sleep duration, insomnia, sleep apnea, chronotype, daytime dozing, napping during the day, and snoring. These parameters were specifically chosen for their documented impacts on psychiatric health, as evidenced by their diagnostic relevance and frequent alteration in psychiatric patients, supported by both clinical observations and epidemiological studies. This comprehensive examination of selected sleep parameters across major depressive disorder, schizophrenia, and ADHD not only aims to elucidate the causal pathways involved but also seeks to identify potential therapeutic interventions that could significantly improve outcomes for patients suffering from these debilitating disorders.
Mendelian randomization (MR) is an epidemiological method that uses genetic variants as instrumental variables to investigate the causal association between exposures and outcomes. MR could be considered conceptually as natural randomized controlled trial studies (RCTs) because genetic variants are randomly inherited (Emdin et al., 2017). MR is an alternative approach to explore the potential causal relationship between an exposure and an outcome when RCTs are not feasible due to practical reasons (e.g., expensive financial investment and ethical issues). In addition, MR studies can overcome limitations of observational studies (e.g., inability to control for potential confounding factors), and thus provide stronger evidence of causation. For instance, certain genetic variants have been associated with sleep characteristics (e.g., sleep duration, propensity for insomnia, and circadian rhythms) (Sun et al., 2022). These genetic variants are fixed at birth and are rarely influenced by acquired environment and behaviors. Therefore, by analyzing associations between the underlying genetic variants of sleep characteristics with psychiatric disorders, causality can be reliably inferred.
Recent advances in the field of MR have significantly contributed to our understanding of the intricate causal relationships between various sleep traits and psychiatric disorders. A pivotal bidirectional Mendelian Randomization (MR) study meticulously examined the complex interactions between crucial sleep characteristics, including insomnia, chronotype, and sleep duration, and a spectrum of psychiatric disorders such as MDD, ADHD, Post-Traumatic Stress Disorder (PTSD), Bipolar Disorder (BD), and Schizophrenia (Sun et al., 2022). The findings of this study revealed distinct associations: insomnia was linked to MDD, ADHD, and PTSD; chronotype showed associations with MDD and Schizophrenia; and sleep duration was correlated with bipolar disorder. These associations underscore the multifaceted impact of sleep patterns on mental health, highlighting specific sleep-related factors contributing to the etiology of diverse psychiatric conditions. A seminal study by Huang et al. is particularly illuminating in this regard, revealing a robust association between insomnia and increased susceptibility to ADHD and MDD (Huang et al., 2021). This finding is corroborated by subsequent research, which further underscores the link between insomnia and an elevated risk of MDD development. Another study adds another layer of complexity, suggesting a protective effect of morning diurnal preference against schizophrenia (Wang et al., 2022). In this study, longer sleep durations and habitual daytime napping are implicated in an increased risk of developing schizophrenia. Moreover, other MR investigations have shed light on the broader implications of diurnal preferences, linking late diurnal preference to poorer mental health outcomes (O’Loughlin et al., 2021). A particularly targeted study provides compelling evidence that, among the various sleep disturbances, insomnia specifically, as opposed to short or long sleep durations, exerts a causal influence on anxiety disorders (Huang et al., 2020). Those studies primarily focused on a specific sleep parameter (e.g., insomnia or sleep duration) or targeted individual psychiatric disorders (e.g., schizophrenia). This selective focus, despite its contributions, has resulted in a narrow scope of evidence. There is a need to include a wide range of sleep parameters and examine their effects on common psychiatric disorders to address existing gaps and enhance our understanding of these complex relationships. Such an inclusive and expansive approach is essential to deeper address the existing gaps in the literature and to deepen our holistic understanding of the complex interplay between sleep traits and psychiatric health.
In the present study, we employed a two-sample MR approach to systematically explore the causal relationship between a comprehensive set of sleep parameters—sleep duration, insomnia, sleep apnea, chronotype, daytime dozing, napping during the day, and snoring—and three major psychiatric disorders: MDD, schizophrenia, and ADHD. This method offers a robust alternative to traditional observational studies by mitigating issues related to confounding factors and reverse causality. Our investigation aims to enhance our understanding of the etiology of these psychiatric disorders and could lead to the development of novel preventative strategies.
A two-sample MR design was selected to investigate the causal associations of sleep (sleep duration, insomnia, sleep apnea, chronotype, daytime dozing, napping during the day, and snoring) with psychiatric disorders including MDD, schizophrenia, and ADHD. Sleep was the exposure and psychiatric disorders were the outcomes. We thus performed a total of 21 MR analyses.
Instrumental variables (IV) for both sleep and psychiatric disorders were based on the following three key assumptions (Woolf et al., 2022): (a) a relevance assumption--the genetic variants should be strongly associated with the exposure; (b) an independence assumption--the genetic variants should be independent of potential confounders; and (c) an exclusion restriction--the genetic variants should be associated with the outcome only through the exposure.
All individuals included in the genome-wide association study (GWAS) were of European ethnic origin (Table 1). For each exposure factor, the single-nucleotide polymorphisms (SNPs) were filtered according to the three main MR assumptions. To satisfy the “relevance assumption”, SNPs that achieved p < 5 × 10−8 were selected. To meet the “independence assumption”, we searched the curated SNP-to-phenotype databases of PhenoScanner (Version 2; http://www.phenoscanner.medschl.cam.ac.uk/) for other significant (p < 5 × 10−8) genome-wide phenotypes related to the chosen SNPs and their correlated SNPs, which may affect the outcomes via paths other than the exposures of interest (Kamat et al., 2019). Additionally, we performed linkage disequilibrium (LD) calculation across SNPs to select independent SNPs based on LD r2 < 0.001 and clump distance >10,000 kb (Purcell et al., 2007). We used these carefully chosen SNPs as the final genetic IVs for the subsequent MR analysis.
For sleep duration, insomnia, chronotype, daytime dozing, and napping during the day, we used the largest published GWAS, which had been conducted in a population of European descent (Elsworth et al., 2020). These data were from an MRC Integrative Epidemiology Unit (IEU) at the University of Bristol analysis of United Kingdom Biobank phenotypes (UK Biobank, 2017). The United Kingdom Biobank is a comprehensive database, encompassing data from approximately 500,000 volunteers, who were aged between 40 and 69 years at the time of recruitment. This database includes not only genetic data and physiological measurements but also detailed health records and information about the lifestyle and environment. SNPs were selected to serve as instrumental variables for various sleep parameters. It included 71 SNPs associated with sleep duration (refer to Supplementary Table S1), 42 SNPs linked to insomnia (see Supplementary Table S2), and 161 SNPs related to chronotype (outlined in Supplementary Table S3). Additionally, 31 SNPs were chosen for their relevance to daytime dozing (detailed in Supplementary Table S4), while 94 SNPs were selected for their association with napping during the day (listed in Supplementary Table S5).
Sleep duration was measured by a standardized question “About how many hours of sleep do you get in every 24 h? (please include naps)” with responses in integer hours. Two binary variables were derived from self-reported sleep duration, i.e., short sleep (<7 h, the number of cases was 106,192) and long sleep (<7 h, the number of cases was 34,184) (Cappuccio et al., 2010). Insomnia was assessed by the question “do you have trouble falling asleep at night or do you wake up in the middle of the night?”, with 129,720 insomnia patients and 237,627 controls. Chronotype was measured by a question “Do you consider yourself to be definitely a morning person/more a morning than evening/more an evening than morning/definitely an evening person/do not know/prefer not to answer”, identifying 252,287 as morning person and 150,908 as evening person. Daytime dozing was assessed by a question “How likely are you to doze off or fall asleep during the daytime when you do not mean to? E.g., when working, reading or driving”, resulting in 104,786 daytime dozing and 356,127 controls. Napping during the day was measured by a question “Do you have a nap during the day” while the numbers of cases was not avaliable.
For sleep apnea, we used the largest number of SNPs of GWAS which was conducted in a population of European descent. These data were from FinnGen biobank, which included data for 16,761 sleep apnea patients and 201,194 controls. Sleep apnea were defined using participant-reported diagnosis or ICD diagnostic codes available in electronic health records (ICD-9: 327.23 and ICD-10: G47.3) (Campos et al., 2023). FinnGen is a large biobank study that aims to genotype 500,0000 Finns, which included data for including prospective and retrospective epidemiological and disease-based cohorts as well as hospital biobank samples. In total, five SNPs that showed genome-wide significance were selected as instrumental variables (Supplementary Table S6).
For snoring, we used the largest and most recently published GWAS which was conducted in a population of European descent, including 152,302 snoring cases and 256,015 controls. Snoring was measured by a question “Does your partner or a close relative or friend complain about your snoring”. These data were from the European Bioinformatics Institute (EBI) databases which is a hub for research and services in bioinformatics (Cook et al., 2020; Cantelli et al., 2022). In total, 38 SNPs that showed genome-wide significance were selected as instrumental variables for snoring (Supplementary Table S7).
We performed the statistical analyses using R version 3.6.3 (R Foundation for Statistical Computing, Vienna, Austria) and R package TwoSampleMR. We compared eight different MR methods: MR-Egger, weighted median, inverse-variance weighted (IVW), simple mode, weighted mode, maximum likelihood, penalized weighted median, and inverse-variance weighted (fixed effects). All these methods are based on similar assumptions about the nature of pleiotropy (Boef et al., 2015). We conducted the primary analysis using the IVW method with multiplicative random effects. This method is optimal for two-sample MR, provided that SNPs strictly adhere to essential assumptions (Burgess et al., 2019). The differences between these 8 MR methods (Sanderson et al., 2022) were shown in Table 2. The Cochran’s Q statistic (IVW) and Rucker’s Q statistic (MR Egger) were used to detect the heterogeneity of our MR analysis, and p-values> 0.05 indicated no heterogeneity (Hemani et al., 2018). The intercept test of MR Egger was used to detect the horizontal pleiotropy, and p-values>0.05 indicated no horizontal pleiotropy (Shu et al., 2022). The leave-one-out analysis was used to investigate whether the causal relationship between sleep and psychiatric disorders was influenced by a single SNP (Lee, 2019). The main parameters included sample size, type I error rate, proportion of cases, odds ratio (OR) of the outcome, and proportion of the variance explained for the association between the SNPs and the exposure variable (r2). The statistical significance was set at a two-sided p-values<0.05. To avoid the inflation of false-positive findings, we calculated the false-discovery rate (FDR) corrected p-values for the main analyses (Korthauer et al., 2019). Significance was determined as FDR-corrected p-values<0.05, whereas p-values <0.05 that did not meet the FDR-corrected threshold were regarded as suggestive evidence of an association. The overview of the study design, illustrating the various sleep parameters and psychiatric disorders analyzed, the MR methods employed, and the sensitivity analyses conducted was shown in Figure 1.
All cited genome-wide association studies, epigenome-wide association studies and summary-level data had been approved by a relevant review board.
In this study, a total of 21 MR analyses were conducted. These analyses encompassed a range of MR methodologies, including but not limited to MR-Egger, Weighted Median, and IVW. Each method was rigorously applied and evaluated to explore various aspects of the genetic associations under investigation. We used the IVW method as the main method for all MR analyses. The IVW model, which generates a stable causal inference even in the presence of horizontal pleiotropy, provided a weighted regression of the IV-specific causal estimation. The results of IVW were shown in Table 3. To maintain clarity and conciseness in the presentation of our findings, the detailed outcomes of all MR analyses have been compiled in the (Supplementary Table S8) accompanying this manuscript.
Table 3. Summary of IVW results for the association between sleep parameters and psychiatric disorders.
Insomnia was significantly associated with an increased risk of MDD, according to the results of IVW (number of SNPs = 32, OR = 2.017, 95%CI = 1.344–3.026, SE = 0.207, corrected p-value = 0.011). Napping during the day also showed a significant association with an increased risk of MDD, according to the results or IVW (number of SNPs = 83, OR = 1.807, 95%CI = 1.336–2.443, SE = 0.154, corrected p-value<0.001). The increased risk of schizophrenia was linked to sleep duration (number of SNPs = 57, OR = 2.200, 95%CI = 1.241–3.901, SE = 0.292, corrected p-value = 0.049). Daytime dozing was associated with schizophrenia, but the association was not statistically significant (number of SNPs = 23, OR = 4.439, 95%CI = 1.201–16.407, SE = 0.667, corrected p-value = 0.088). Similarly, the association between napping during the day and schizophrenia was not significant (number of SNPs = 82, OR = 2.111, 95%CI = 1.109–4.018, SE = 0.328, corrected p-value = 0.088). Neither was the association between sleep duration and ADHD (number of SNPs = 52, OR = 0.625, 95%CI = 0.417–0.934, SE = 0.206, corrected p-value = 0.088). IVW results of the causal relationship between sleep parameters and the risk of psychiatric disorders are shown in Figure 2.
No causal association was found of sleep duration (number of SNPs = 56, OR = 0.855, 95%CI = 0.627, 1.166, SE = 0.158, corrected p-value = 0.564), sleep apnea (number of SNPs = 5, OR = 1.038, 95%CI = 0.863, 1.249, SE = 0.094, corrected p-value = 0.855), chronotype (number of SNPs = 133, OR = 1.079, 95%CI = 0.949–1.228, SE = 0.066, corrected p-value = 0.564), day time dozing (number of SNPs = 28, OR = 1.185, 95%CI = 0.683–2.054, SE = 0.281, corrected p-value = 0.835), and snoring (number of SNPs = 23, OR = 0.836, 95%CI = 0.428–1.632, SE = 0.341, corrected p-value = 0.835) with MDD. Additionally, there was no causal relationship of insomnia (number of SNPs = 34, OR = 1.006, 95%CI = 0.454–2.231, SE = 0.406, corrected p-value = 0.988), sleep apnea (number of SNPs = 5, OR = 1.015, 95%CI = 0.865–1.191, SE = 0.081, corrected p-value = 0.908), chronotype (number of SNPs = 142, OR = 1.233, 95%CI = 0.943–1.613, SE = 0.137, corrected p-value = 0.328), and snoring (number of SNPs = 23, OR = 0.514, 95%CI = 0.146–1.813, SE = 0.643, corrected p-value = 0.564) with schizophrenia. There was no causal relationship of insomnia (number of SNPs = 31, OR = 1.430, 95%CI = 0.729–2.804, SE = 0.344, corrected p-value = 0.564), sleep apnea (number of SNPs = 4, OR = 1.015, 95%CI = 0.854–1.206, SE = 0.088, corrected p-value = 0.908), chronotype (number of SNPs = 129, OR = 0.936, 95%CI = 0.723–1.212, SE = 0.132, corrected p-value = 0.835), day time dozing (number of SNPs = 30, OR = 0.896, 95%CI = 0.300–2.675, SE = 0.558, corrected p-value = 0.908), napping during the day (number of SNPs = 78, OR = 1.455, 95%CI = 0.901–2.350, SE = 0.245, corrected p-value = 0.328), and snoring (number of SNPs = 29, OR = 1.265, 95%CI = 0.479–3.338, SE = 0.495, corrected p-value = 0.835) with ADHD. More details are shown in Supplementary Table S8 and Supplementary Figure S1–S21.
Sensitivity analyses revealed the robustness of the findings (Supplementary Table S9; Supplementary Figure S22–S63). Heterogeneity was found only in the test of sleep duration and ADHD. There was no heterogeneity among other tests. Horizontal pleiotropy was unlikely to skew the causality of sleep-related factors with psychiatric disorders according to the MR-Egger regression (Supplementary Table S10). Leave-one-out analysis indicated that the causal estimates of sleep and psychiatric disorders were not driven by any single SNP (Supplementary Figure S64–S84).
In this MR study, we explored the causal associations between seven sleep parameters and three common psychiatric disorders. Our findings indicate that insomnia and napping during the day were significantly associated with increased MDD risk. Additionally, sleep duration, daytime dozing, and napping during the day showed an effect on increasing the risk of schizophrenia, while sleep duration showed an effect on decreasing the risk of ADHD.
Epidemiological evidence has revealed associations between insomnia, daytime dozing, and napping during the day. However, the extent to which the underlying genetics are shared is unknown (Lane et al., 2017). Our results confirmed daytime dozing and napping during the day shared the same SNP rs12140153 and rs13284688 which could be considered evidence for underlying genetics. The mutations in SNP rs12140153 have a profound effect on the protein Pals1-associated tight junction (PATJ). The PATJ gene encodes a membrane-associated protein that plays a crucial role in the formation of tight junctions, essential for maintaining the integrity of various cellular structures and functions. Research has implicated this protein in photoreception in model organisms such as mice and Drosophila, suggesting that it may play a role in sleep-wake cycle regulation via the entrainment of circadian rhythms to light-dark cycles (Peirson et al., 2007). The exact mechanism through which mutations in rs12140513 influence PATJ function and, in turn, affect sleep patterns warrants further investigation.
Our findings indicate that insomnia and napping during the day are significantly associated with an increased MDD risk. This finding consistent with findings from recent studies (Baranova et al., 2022; Sun et al., 2022; Yao et al., 2022). The potential underlying mechanisms are likely due to neurotransmitter imbalance, such as enhanced cholinergic or diminished aminergic neurotransmissions, brain activation abnormalities in emotion regulation areas, dysregulation of the hypothalamic–pituitary–adrenal axis, and increased inflammation (Freeman et al., 2020). Chronic insomnia may cause overactivity in brain regions responsible for mood regulation, leading to more frequent mood swings and contributing to the low mood characteristic of depression. Furthermore, insomnia might reduce brain’s sensitivity to neurotransmitters like serotonin. A reduction in serotonin receptor sensitivity and serotonin neurotransmission has often been implicated in the pathophysiology of major depressive disorder (Meerlo et al., 2008). Interventions targeting sleep have showed an effect on reducing depressive symptoms, underscoring the connection between sleep and mood (Gee et al., 2019).
This link is crucial for understanding the broader implications of sleep disorders on mental health and underscores the importance of addressing sleep disturbances within mental health treatment paradigms (Baranova et al., 2022). In addition to psychiatric disorders, insomnia has demonstrated associations with systemic diseases such as cardiovascular disease, as discussed in recent studies (Cao et al., 2024). This connection suggests that the scope of insomnia’s impact extends well beyond mental health, influencing overall physical health and potentially complicating treatment approaches for related physical ailments. Daytime napping may disrupt normal circadian rhythms, affecting hormone production and the body clock, and is associated with symptoms of depression (e.g., a lack of energy and low mood). While a moderate amount of napping may help reduced sleep pressure and restore energy, too much may lead to decreased quality of sleep at night, further exacerbating depressive symptoms (Crouse et al., 2021). Currently, evidence about the relationship between napping and MDD is scarce. In the future, more studies using a longitudinal or interventional design are needed to confirm their association.
Our study showed that sleep duration was associated with an increased risk for schizophrenia, while daytime dozing and napping during the day had a suggestive association with the risk of schizophrenia, consistent with Wang et al. study (Wang et al., 2022). The underlying mechanisms that link sleep with schizophrenia are complex and multifaceted and may involve disturbances in the sleep structure and circadian rhythm system in patients with schizophrenia (Waite et al., 2020). When sleep deprivation occurs, it can cause an increase in dopamine release in the brain. This increase is particularly significant in individuals with schizophrenia, as it can intensify positive symptoms (Ferrarelli, 2021). Moreover, slow-wave sleep (or deep sleep) plays a pivotal role in cognitive processing and emotional stabilization (Walker, 2009). A lack of sleep can result in a decrease in the proportion of slow-wave sleep, which may affect cognitive processing, making individuals more susceptible to hallucinations and delusions (Walker, 2009). Our study used Mendelian randomization to establish a causal link between sleep and schizophrenia, adding to the current evidence base.
Our study also revealed that sleep duration had a suggestive association with the risk of ADHD. The crucial role of sleep in promoting the optimal development and organization of neural circuitry is well-known (Lunsford-Avery et al., 2016). Both insufficient and excessive sleep can disrupt the equilibrium of neurotransmitters in the brain, particularly those involved in regulating attention, behavior, and emotions, such as dopamine (Tononi and Cirelli, 2012). This disruption could elevate the risk of developing ADHD. In addition, the quality of sleep, often compromised by either too little or too much sleep, may result in daytime fatigue, decreased attention span, and emotional instability (Tononi and Cirelli, 2012). These findings provide an important direction for future research about the relationship between sleep and ADHD.
A major strength of this study was the MR design, which minimized the residual confounding and reverse causality inherent in observational studies and allowed us to interrogate the potential causality between sleep and common psychiatric disorders. Additionally, we included a variety of sleep parameters and psychiatric disorders. Sensitivity analyses supported the validity of the estimates. The IVs used in this study were extracted from the largest GWAS of sleep with the largest sample sizes and were strongly associated with the exposure of interest. This approach minimized weak-instrument bias and increased statistical power in our analyses. However, our study also has limitations. First, the study’s reliance on genetic instruments from GWAS summary data may not fully capture the multifaceted nature of sleep parameters and psychiatric disorders. Genetic variants used as instruments may not comprehensively represent the biological mechanisms underlying these conditions. Second, although horizontal pleiotropy was assessed using MR-Egger, the potential for unrecognized pleiotropic effects remains a concern. MR assumptions such as the instrument’s exclusive effect through the exposure (exclusion restriction) and the absence of unmeasured confounders between the instrument and the outcome are critical for unbiased causal inference. Any violations of these assumptions can lead to biased estimates, limiting the conclusiveness of our findings. Moreover, the detected heterogeneity, as indicated by Cochran’s Q statistic, suggests variability in the effects across different genetic instruments, which could further complicate the causal interpretation. Third, the GWAS summary data derived from the United Kingdom Biobank, FinnGen Biobank, and EBI databases may limit the generalizability of the findings. These populations may not fully represent the global diversity, particularly in terms of ethnic and genetic backgrounds, which could influence the sleep-psychiatry relationship. Lastly, while this study focused on three specific psychiatric disorders (MDD, schizophrenia, and ADHD), the causal relationship between sleep parameters and other psychiatric conditions remains to be explored. This suggests a need for broader investigation into how sleep disturbances may influence a wider range of psychiatric conditions, thereby extending the relevance of our findings to other disorders and potentially uncovering novel therapeutic targets or preventative strategies.
Our study found a causal association between insomnia and napping during the day with the risk of MDD. Additionally, sleep duration was associated with an increased risk for schizophrenia, while daytime dozing and napping during the day had a suggestive association with the risk of schizophrenia. Furthermore, our findings also revealed that sleep duration had a suggestive association with the risk of ADHD.
The original contributions presented in the study are included in the article/Supplementary Material, further inquiries can be directed to the corresponding author.
The studies involving humans were approved by all cited genome-wide association studies, epigenome-wide association studies and summary-level data had been approved by a relevant review board. The studies were conducted in accordance with the local legislation and institutional requirements. Written informed consent for participation in this study was provided by the participants’ legal guardians/next of kin. No potentially identifiable images or data are presented in this study.
PC: Writing–review and editing, Writing–original draft, Visualization, Validation, Software, Resources, Project administration, Methodology, Investigation, Formal Analysis, Data curation, Conceptualization. JQ: Writing–review and editing, Writing–original draft, Visualization, Software, Resources, Project administration, Methodology, Investigation, Formal Analysis, Data curation, Conceptualization, Validation. YW: Conceptualization, Writing–review and editing, Writing–original draft, Validation, Software, Resources, Formal Analysis, Data curation. JY: Writing–review and editing, Writing–original draft, Software, Methodology, Formal Analysis, Data curation. YP: Writing–review and editing, Writing–original draft, Visualization, Validation, Supervision, Software, Resources, Methodology, Formal Analysis, Data curation. BZ: Writing–review and editing, Writing–original draft, Visualization, Validation, Supervision, Software, Resources, Project administration, Methodology, Investigation, Funding acquisition, Formal Analysis, Data curation, Conceptualization.
The author(s) declare that financial support was received for the research, authorship, and/or publication of this article. BZ was supported by the “Shanghai Jiao Tong University School of Medicine-Nursing Development Program”.
The authors are grateful to all the investigators for making available their GWAS data.
The authors declare that the research was conducted in the absence of any commercial or financial relationships that could be construed as a potential conflict of interest.
All claims expressed in this article are solely those of the authors and do not necessarily represent those of their affiliated organizations, or those of the publisher, the editors and the reviewers. Any product that may be evaluated in this article, or claim that may be made by its manufacturer, is not guaranteed or endorsed by the publisher.
The Supplementary Material for this article can be found online at: https://www.frontiersin.org/articles/10.3389/fgene.2024.1380544/full#supplementary-material
Baldessarini, R. J., and Tondo, L. (2020). Suicidal risks in 12 DSM-5 psychiatric disorders. J. Affect Disord. 271, 66–73. doi:10.1016/j.jad.2020.03.083
Baranova, A., Cao, H., and Zhang, F. (2022). Shared genetic liability and causal effects between major depressive disorder and insomnia. Hum. Mol. Genet. 31 (8), 1336–1345. doi:10.1093/hmg/ddab328
Battle, D. E. (2013). Diagnostic and statistical manual of mental disorders (DSM). Codas 25 (2), 191–192. doi:10.1590/s2317-17822013000200017
Boef, A. G., Dekkers, O. M., and le Cessie, S. (2015). Mendelian randomization studies: a review of the approaches used and the quality of reporting. Int. J. Epidemiol. 44 (2), 496–511. doi:10.1093/ije/dyv071
Bowden, J., Davey Smith, G., Haycock, P. C., and Burgess, S. (2016). Consistent estimation in mendelian randomization with some invalid instruments using a weighted median estimator. Genet. Epidemiol. 40 (4), 304–314. doi:10.1002/gepi.21965
Burgess, S., Davey Smith, G., Davies, N. M., Dudbridge, F., Gill, D., Glymour, M. M., et al. (2019). Guidelines for performing Mendelian randomization investigations: update for summer 2023. Wellcome Open Res. 4, 186. doi:10.12688/wellcomeopenres.15555.2
Burgess, S., and Thompson, S. G. (2017). Interpreting findings from Mendelian randomization using the MR-Egger method. Eur. J. Epidemiol. 32 (5), 377–389. doi:10.1007/s10654-017-0255-x
Campos, A. I., García-Marín, L. M., Byrne, E. M., Martin, N. G., Cuéllar-Partida, G., and Rentería, M. E. (2020). Insights into the aetiology of snoring from observational and genetic investigations in the UK Biobank. Nat. Commun. 11 (1), 817. doi:10.1038/s41467-020-14625-1
Campos, A. I., Ingold, N., Huang, Y., Mitchell, B. L., Kho, P. F., Han, X., et al. (2023). Discovery of genomic loci associated with sleep apnea risk through multi-trait GWAS analysis with snoring. Sleep 46 (3), zsac308. doi:10.1093/sleep/zsac308
Cantelli, G., Bateman, A., Brooksbank, C., Petrov, A. I., Malik-Sheriff, R. S., Ide-Smith, M., et al. (2022). The European bioinformatics Institute (EMBL-EBI) in 2021. Nucleic Acids Res. 50 (D1), D11–D19. doi:10.1093/nar/gkab1127
Cao, H., Baranova, A., Zhao, Q., and Zhang, F. (2024). Bidirectional associations between mental disorders, antidepressants and cardiovascular disease. BMJ Ment. Health 27 (1), e300975. doi:10.1136/bmjment-2023-300975
Cappuccio, F. P., D’Elia, L., Strazzullo, P., and Miller, M. A. (2010). Sleep duration and all-cause mortality: a systematic review and meta-analysis of prospective studies. Sleep 33 (5), 585–592. doi:10.1093/sleep/33.5.585
Cook, C. E., Stroe, O., Cochrane, G., Birney, E., and Apweiler, R. (2020). The European Bioinformatics Institute in 2020: building a global infrastructure of interconnected data resources for the life sciences. Nucleic Acids Res. 48 (D1), D17–D23. doi:10.1093/nar/gkz1033
Crouse, J. J., Carpenter, J. S., Song, Y. J. C., Hockey, S. J., Naismith, S. L., Grunstein, R. R., et al. (2021). Circadian rhythm sleep-wake disturbances and depression in young people: implications for prevention and early intervention. Lancet Psychiatry 8 (9), 813–823. doi:10.1016/S2215-0366(21)00034-1
Dashti, H. S., Jones, S. E., Wood, A. R., Lane, J. M., van Hees, V. T., Wang, H., et al. (2019). Genome-wide association study identifies genetic loci for self-reported habitual sleep duration supported by accelerometer-derived estimates. Nat. Commun. 10 (1), 1100. doi:10.1038/s41467-019-08917-4
Dong, Y., and Yang, F. M. (2019). Insomnia symptoms predict both future hypertension and depression. Prev. Med. 123, 41–47. doi:10.1016/j.ypmed.2019.02.001
Elsworth, B., Lyon, M., Alexander, T., Liu, Y., Matthews, P., Hallett, J., et al. (2020) The MRC IEU OpenGWAS data infrastructure.
Emdin, C. A., Khera, A. V., and Kathiresan, S. (2017). Mendelian randomization. Jama. 318 (19), 1925–1926. doi:10.1001/jama.2017.17219
Ferrarelli, F. (2021). Sleep abnormalities in schizophrenia: state of the art and next steps. Am. J. Psychiatry 178 (10), 903–913. doi:10.1176/appi.ajp.2020.20070968
Freeman, D., Sheaves, B., Waite, F., Harvey, A. G., and Harrison, P. J. (2020). Sleep disturbance and psychiatric disorders. Lancet Psychiatry 7 (7), 628–637. doi:10.1016/S2215-0366(20)30136-X
GBD 2017 Disease and Injury Incidence and Prevalence Collaborators (2018). Global, regional, and national incidence, prevalence, and years lived with disability for 354 diseases and injuries for 195 countries and territories, 1990-2017: a systematic analysis for the Global Burden of Disease Study 2017. Lancet 392 (10159), 1789–1858. doi:10.1016/S0140-6736(18)32279-7
Gee, B., Orchard, F., Clarke, E., Joy, A., Clarke, T., and Reynolds, S. (2019). The effect of non-pharmacological sleep interventions on depression symptoms: a meta-analysis of randomised controlled trials. Sleep. Med. Rev. 43, 118–128. doi:10.1016/j.smrv.2018.09.004
Geoffroy, P. A., Hoertel, N., Etain, B., Bellivier, F., Delorme, R., Limosin, F., et al. (2018). Insomnia and hypersomnia in major depressive episode: prevalence, sociodemographic characteristics and psychiatric comorbidity in a population-based study. J. Affect Disord. 226, 132–141. doi:10.1016/j.jad.2017.09.032
Hashmi, A. N., Qamar, R., Taj, R., Zubair, U. B., Agha, Z., Abbasi, S. A., et al. (2022). Contributing risk factors of common psychiatric disorders in the Pakistani population. Eur. Arch. Psychiatry Clin. Neurosci. 273, 963–981. doi:10.1007/s00406-022-01545-y
Hemani, G., Zheng, J., Elsworth, B., Wade, K. H., Haberland, V., Baird, D., et al. (2018). The MR-Base platform supports systematic causal inference across the human phenome. Elife 7, e34408. doi:10.7554/eLife.34408
Hertenstein, E., Trinca, E., Wunderlin, M., Schneider, C. L., Züst, M. A., Fehér, K. D., et al. (2022). Cognitive behavioral therapy for insomnia in patients with mental disorders and comorbid insomnia: a systematic review and meta-analysis. Sleep. Med. Rev. 62, 101597. doi:10.1016/j.smrv.2022.101597
Huang, J., Zuber, V., Matthews, P. M., Elliott, P., Tzoulaki, J., and Dehghan, A. (2020). Sleep, major depressive disorder, and Alzheimer disease: a Mendelian randomization study. Neurology 95 (14), e1963–e1970. doi:10.1212/WNL.0000000000010463
Huang, P., Zou, Y., Zhang, X., Ye, X., Wang, Y., Yu, R., et al. (2021). The causal effects of insomnia on bipolar disorder, depression, and schizophrenia: a two-sample mendelian randomization study. Front. Genet. 12, 763259. doi:10.3389/fgene.2021.763259
Jiang, S., Zheng, L., and Miao, Z. (2022). Gastroesophageal reflux disease and oral symptoms: a two-sample Mendelian randomization study. Front. Genet. 13, 1061550. doi:10.3389/fgene.2022.1061550
Jones, S. E., Lane, J. M., Wood, A. R., van Hees, V. T., Tyrrell, J., Beaumont, R. N., et al. (2019). Genome-wide association analyses of chronotype in 697,828 individuals provides insights into circadian rhythms. Nat. Commun. 10 (1), 343. doi:10.1038/s41467-018-08259-7
Kamat, M. A., Blackshaw, J. A., Young, R., Surendran, P., Burgess, S., Danesh, J., et al. (2019). PhenoScanner V2: an expanded tool for searching human genotype-phenotype associations. Bioinformatics 35 (22), 4851–4853. doi:10.1093/bioinformatics/btz469
Korthauer, K., Kimes, P. K., Duvallet, C., Reyes, A., Subramanian, A., Teng, M., et al. (2019). A practical guide to methods controlling false discoveries in computational biology. Genome Biol. 20 (1), 118. doi:10.1186/s13059-019-1716-1
Lane, J. M., Jones, S. E., Dashti, H. S., Wood, A. R., Aragam, K. G., van Hees, V. T., et al. (2019). Biological and clinical insights from genetics of insomnia symptoms. Nat. Genet. 51 (3), 387–393. doi:10.1038/s41588-019-0361-7
Lane, J. M., Liang, J., Vlasac, I., Anderson, S. G., Bechtold, D. A., Bowden, J., et al. (2017). Genome-wide association analyses of sleep disturbance traits identify new loci and highlight shared genetics with neuropsychiatric and metabolic traits. Nat. Genet. 49 (2), 274–281. doi:10.1038/ng.3749
Lee, Y. H. (2019). Causal association between smoking behavior and the decreased risk of osteoarthritis: a Mendelian randomization. Z Rheumatol. 78 (5), 461–466. doi:10.1007/s00393-018-0505-7
Liu, H. L. V., Sun, F., and Tse, C. Y. A. (2023). Examining the impact of physical activity on sleep quality in children with ADHD. J. Atten. Disord. 27 (10), 1099–1106. doi:10.1177/10870547231171723
Lunsford-Avery, J. R., Krystal, A. D., and Kollins, S. H. (2016). Sleep disturbances in adolescents with ADHD: a systematic review and framework for future research. Clin. Psychol. Rev. 50, 159–174. doi:10.1016/j.cpr.2016.10.004
Meerlo, P., Sgoifo, A., and Suchecki, D. (2008). Restricted and disrupted sleep: effects on autonomic function, neuroendocrine stress systems and stress responsivity. Sleep. Med. Rev. 12 (3), 197–210. doi:10.1016/j.smrv.2007.07.007
Murray, C. J., Vos, T., Lozano, R., Naghavi, M., Flaxman, A. D., Michaud, C., et al. (2012). Disability-adjusted life years (DALYs) for 291 diseases and injuries in 21 regions, 1990-2010: a systematic analysis for the Global Burden of Disease Study 2010. Lancet 380 (9859), 2197–2223. doi:10.1016/S0140-6736(12)61689-4
Mylonas, D., Baran, B., Demanuele, C., Cox, R., Vuper, T. C., Seicol, B. J., et al. (2020). The effects of eszopiclone on sleep spindles and memory consolidation in schizophrenia: a randomized clinical trial. Neuropsychopharmacology 45 (13), 2189–2197. doi:10.1038/s41386-020-00833-2
O’Loughlin, J., Casanova, F., Jones, S. E., Hagenaars, S. P., Beaumont, R. N., Freathy, R. M., et al. (2021). Using Mendelian Randomisation methods to understand whether diurnal preference is causally related to mental health. Mol. Psychiatry 26 (11), 6305–6316. doi:10.1038/s41380-021-01157-3
Peirson, S. N., Oster, H., Jones, S. L., Leitges, M., Hankins, M. W., and Foster, R. G. (2007). Microarray analysis and functional genomics identify novel components of melanopsin signaling. Curr. Biol. 17 (16), 1363–1372. doi:10.1016/j.cub.2007.07.045
Pooja, F., Chhabria, P., Kumar, P., Kalpana, F., Kumar, P., Iqbal, A., et al. (2021). Frequency of psychiatric disorders in suicide attempters: a cross-sectional study from low-income country. Cureus 13 (4), e14669. doi:10.7759/cureus.14669
Purcell, S., Neale, B., Todd-Brown, K., Thomas, L., Ferreira, M. A., Bender, D., et al. (2007). PLINK: a tool set for whole-genome association and population-based linkage analyses. Am. J. Hum. Genet. 81 (3), 559–575. doi:10.1086/519795
Sanderson, E., Glymour, M. M., Holmes, M. V., Kang, H., Morrison, J., Munafò, M. R., et al. (2022). Mendelian randomization. Nat. Rev. Methods Prim. 2 (1), 6. doi:10.1038/s43586-021-00092-5
Shu, M. J., Li, J. R., Zhu, Y. C., and Shen, H. (2022). Migraine and ischemic stroke: a mendelian randomization study. Neurol. Ther. 11 (1), 237–246. doi:10.1007/s40120-021-00310-y
Slob, E. A., and Burgess, S. (2020). A comparison of robust Mendelian randomization methods using summary data. Genet. Epidemiol. 44 (4), 313–329. doi:10.1002/gepi.22295
Strausz, S., Ruotsalainen, S., Ollila, H. M., Karjalainen, J., Kiiskinen, T., Reeve, M., et al. (2021). Genetic analysis of obstructive sleep apnoea discovers a strong association with cardiometabolic health. Eur. Respir. J. 57 (5), 2003091. doi:10.1183/13993003.03091-2020
Sun, X., Liu, B., Liu, S., Wu, D. J. H., Wang, J., Qian, Y., et al. (2022). Sleep disturbance and psychiatric disorders: a bidirectional Mendelian randomisation study. Epidemiol. Psychiatr. Sci. 31, e26. doi:10.1017/S2045796021000810
Sutton, E. L. (2014). Psychiatric disorders and sleep issues. Med. Clin. North Am. 98 (5), 1123–1143. doi:10.1016/j.mcna.2014.06.009
Tononi, G., and Cirelli, C. (2012). Time to be SHY? Some comments on sleep and synaptic homeostasis. Neural Plast. 2012, 415250. doi:10.1155/2012/415250
UK Biobank (2017). Rapid GWAS of thousands of phenotypes for 337,000 samples in the UK biobank. Available at: http://www.nealelab.is/blog/2017/7/19/rapid-gwas-of-thousands-of-phenotypes-for-337000-samples-in-the-uk-biobank.
Waite, F., Sheaves, B., Isham, L., Reeve, S., and Freeman, D. (2020). Sleep and schizophrenia: from epiphenomenon to treatable causal target. Schizophr. Res. 221, 44–56. doi:10.1016/j.schres.2019.11.014
Walker, M. P. (2009). The role of slow wave sleep in memory processing. J. Clin. Sleep. Med. 5 (Suppl. l), S20–S26. doi:10.5664/jcsm.5.2s.s20
Wang, H., Lane, J. M., Jones, S. E., Dashti, H. S., Ollila, H. M., Wood, A. R., et al. (2019). Genome-wide association analysis of self-reported daytime sleepiness identifies 42 loci that suggest biological subtypes. Nat. Commun. 10 (1), 3503. doi:10.1038/s41467-019-11456-7
Wang, Z., Chen, M., Wei, Y. Z., Zhuo, C. G., Xu, H. F., Li, W. D., et al. (2022). The causal relationship between sleep traits and the risk of schizophrenia: a two-sample bidirectional Mendelian randomization study. BMC Psychiatry 22 (1), 399. doi:10.1186/s12888-022-03946-8
Woolf, B., Di Cara, N., Moreno-Stokoe, C., Skrivankova, V., Drax, K., Higgins, J. P. T., et al. (2022). Investigating the transparency of reporting in two-sample summary data Mendelian randomization studies using the MR-Base platform. Int. J. Epidemiol. 51 (6), 1943–1956. doi:10.1093/ije/dyac074
Xu, J., Zhang, S., Tian, Y., Si, H., Zeng, Y., Wu, Y., et al. (2022). Genetic causal association between iron status and osteoarthritis: a two-sample mendelian randomization. Nutrients 14 (18), 3683. doi:10.3390/nu14183683
Keywords: sleep, psychiatric disorder, major depressive disorder, schizophrenia, attention-deficit/hyperactivity disorder, mendelian randomization, causal relationship
Citation: Chen P, Qin J, Wang Y, Yuan J, Pan Y and Zhu B (2024) The causal relationship between sleep and risk of psychiatric disorders: A two-sample mendelian randomization study. Front. Genet. 15:1380544. doi: 10.3389/fgene.2024.1380544
Received: 01 February 2024; Accepted: 31 May 2024;
Published: 17 June 2024.
Edited by:
Sergi Papiol, Ludwig Maximilian University of Munich, GermanyReviewed by:
Fuquan Zhang, Nanjing Medical University, ChinaCopyright © 2024 Chen, Qin, Wang, Yuan, Pan and Zhu. This is an open-access article distributed under the terms of the Creative Commons Attribution License (CC BY). The use, distribution or reproduction in other forums is permitted, provided the original author(s) and the copyright owner(s) are credited and that the original publication in this journal is cited, in accordance with accepted academic practice. No use, distribution or reproduction is permitted which does not comply with these terms.
*Correspondence: Bingqian Zhu, emh1YnFAc2hzbXUuZWR1LmNu
†These authors have contributed equally to this work and share first authorship
Disclaimer: All claims expressed in this article are solely those of the authors and do not necessarily represent those of their affiliated organizations, or those of the publisher, the editors and the reviewers. Any product that may be evaluated in this article or claim that may be made by its manufacturer is not guaranteed or endorsed by the publisher.
Research integrity at Frontiers
Learn more about the work of our research integrity team to safeguard the quality of each article we publish.