Identification of optimal reference genes for gene expression normalization in human osteosarcoma cell lines under proliferative conditions
- 1Programa de Pós-Graduação em Patologia, Universidade Federal do Ceará, Fortaleza, Brazil
- 2Fundação Oswaldo Cruz, Rio de Janeiro, Brazil
A Commentary on
Identification of optimal reference genes for gene expression normalization in human osteosarcoma cell lines under proliferative conditions
by Dong X, Yang Q, Du Z, Zhang G, Shi C, Qin X and Song Y (2022) Identification of optimal reference genes for gene expression normalization in human osteosarcoma cell lines under proliferative conditions. Front. Genet. 13:989990. doi: 10.3389/fgene.2022.989990
To the Editor—We have read with interest the paper by Dong et al. (2022), an essay on identification of ideal reference genes (RGs) for expression analysis, which showed that using three algorithms plus an in silico prediction software application, the ribosomal RNA (18S rRNA) was among the genes with the most stable expression. Preceding that, Silveira et al. (2021) highlighted the significance of assessing the expression stability of RGs systematically when performing real-time quantitative polymerase chain reaction (qRT-PCR) analysis under different experimental conditions. Because qRT-PCR requires stable and reliable genes for normalization, it is crucial to select and validate, and to compare and reproduce meaningful analyses of expression changes of the genes of interest (Dundas and Ling, 2012).
Regarding this latter topic, we would like to comment on important fresh, restricted data. When assaying the human acute monocytic leukemia THP-1 cell line stimulated with 1 mM of Brefeldin A (Hypofarma, Brazil) for 16 h, we uncovered significant information concerning the determination of the expression stability, leading to the choice of the best housekeeping genes for gene expression analysis in a real-time qRT-PCR study. For this, we selected and concomitantly tested in duplicates the stability of four most commonly used RGs in immunological studies using the GoTaq qPCR Master Mix kit (Promega Biosciences, United States) in ABI 7500 Fast equipment (Applied Biosystem, United States): GAPDH, β-actin, 18S rRNA, and β-2-microglobulin [(Dundas and Ling, 2012; Chapman and Waldenström, 2015)]. The details are given in Table 1. As determined previously [(Freitas et al., 2019; Vandesompele et al., 2002)], RG with a lower geNorm mean value of expression stability (M) was considered trustworthy. Hence, three candidate RGs, namely, β-actin, β-2-microglobulin, and GAPDH, displayed satisfactory results (M-values <1.5), except for the 18S rRNA gene, which was considered the least stable (M-value = 2.4) under our test conditions (Figure 1A). This finding replicated other studies [(Molomjamts and Ingolfsland, 2023; Lewczuk et al., 2023; Gubern et al., 2009; Julian et al., 2014; Zhang et al., 2018)]. Based on this, we realized that the 18S rRNA gene performed worst during human mRNA analysis in vitro upon drug stimulus due to its unstable expression. Concordantly, all three prior candidate RGs were again deemed to be potentially mostly stable by RefFinder, with ranking values very close to each other (Figure 1B). Once more, the 18S rRNA gene was considered poorly stable by that algorithm. Despite rRNA comprising roughly 80% of the total RNA available in the cell, being less prone to degradation, our data are in contrast with Dong et al. (2022) and two other controversial studies [(Song et al., 2022; Köhsler et al., 2020)]. Importantly, to ensure the specificity of the amplified products in real-time qRT-PCR assays, the fluorescence signals obtained after the melting curve analysis for each amplification cycle confirmed to yield unique and reproducible peaks in the different replicates analyzed (data not shown). In addition, the cDNA samples presented the parameters considered reliable for application in real-time qRT-PCR assays, ranging from 1.8 to 2.2 in efficiency values (data not shown).
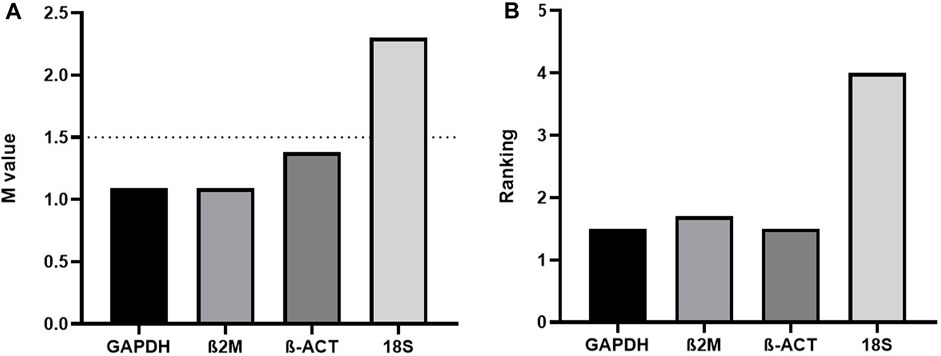
FIGURE 1. (A) geNorm and (B) RefFinder expression stability (M) and ranking values of four reference genes tested in the THP-1 cell line stimulated with Brefeldin A for 16 h (n = 13). β2M=β-2-microglobulin; β-ACT=β-actin.
Identifying and validating optimal internal RNA standards for a real-time qRT-PCR essay, either in constitutive or stimulatory expression patterns under different conditions, is essential for the accurate and reproducible measurement of gene expression abundance in a specific cell or tissue (Yi et al., 2020; Bustin et al., 2010). Furthermore, it is critical to screen for stable endogenous control genes as there are no universally applicable RGs with invariant expression available; therefore, more than one RG should be employed in order to avoid such a bias in the interpretation of results (Kozera and Rapacz, 2013). Therefore, normalizing a qRT-PCR dataset to 18S rRNA may result in faulty data, at least when measuring mRNA levels from the THP-1 cell line model, whereas RG amplified products employing β-actin, GAPDH, and β-2-microglobulin mRNA might lead to more reliable datasets. GAPDH, a commonly used RG in several studies, has been found to be more suitable (Dundas and Ling, 2012). Alternatively, β-actin was the most appropriate RG to be used as an endogenous control for mRNA quantification in cell line studies (Bronkhorst et al., 2016).
In summary, it is crucial to carefully evaluate ahead for appropriate RG in expression studies for the accurate detection of meaningful changes in targeted gene transcript levels in human in vitro real-time qRT-PCR studies since normalization with unsuitable housekeeping gene products led to significant misinterpretation of expression profiles and final results (Köhsler et al., 2020). Our data not only support those previous ones (Molomjamts and Ingolfsland, 2023; Lewczuk et al., 2023; Gubern et al., 2009; Julian et al., 2014; Zhang et al., 2018) but also add another round of information regarding the selection and validation of ideal RGs for expression analysis. Finally, the ideal RG should have minimal regulation across the disease spectrum and be minimally influenced by patient heterogeneity (Kumar et al., 2022). Thus, other follow-up studies are warranted to better clarify this important issue.
Author contributions
PRCS: data curation, formal analysis, investigation, methodology, software, validation, writing–original draft, and writing–review and editing. ASMS: investigation, methodology, and writing–original draft. CGGP: data curation, formal analysis, investigation, methodology, supervision, validation, and writing–original draft. LLN: conceptualization, data curation, formal analysis, investigation, methodology, supervision, validation, writing–original draft, and writing–review and editing. CCF: formal analysis, funding acquisition, investigation, supervision, validation, writing–original draft, and writing–review and editing. PRZA: conceptualization, data curation, formal analysis, funding acquisition, methodology, project administration, resources, supervision, writing–original draft, and writing–review and editing.
Funding
The author(s) declare financial support was received for the research, authorship, and/or publication of this article. This work received the specific APQ-1 grant from the FAPERJ state funding agency. This work is part of master’s theses (PRCS & ASMS), which were supported by the CAPES funding agency. CGGP is in debt to INCT-TB/CNPq for kindly granting access to the scholarship.
Conflict of interest
The authors declare that the research was conducted in the absence of any commercial or financial relationships that could be construed as a potential conflict of interest.
Publisher’s note
All claims expressed in this article are solely those of the authors and do not necessarily represent those of their affiliated organizations, or those of the publisher, the editors, and the reviewers. Any product that may be evaluated in this article, or claim that may be made by its manufacturer, is not guaranteed or endorsed by the publisher.
References
Bronkhorst, A. J., Aucamp, J., Wentzel, J. F., and Pretorius, P. J. (2016). Reference gene selection for in vitro cell-free DNA analysis and gene expression profiling. Clin. Biochem. 49 (7-8), 606–608. doi:10.1016/j.clinbiochem.2016.01.022
Bustin, S. A., Beaulieu, J. F., Huggett, J., Jaggi, R., Kibenge, F. S., Olsvik, P. A., et al. (2010). MIQE précis: practical implementation of minimum standard guidelines for fluorescence-based quantitative real-time PCR experiments. BMC Mol. Biol. 11, 74. doi:10.1186/1471-2199-11-74
Chapman, J. R., and Waldenström, J. (2015). With reference to reference genes: a systematic review of endogenous controls in gene expression studies. PLoS One 10 (11), e0141853. doi:10.1371/journal.pone.0141853
Dong, X., Yang, Q., Du, Z., Zhang, G., Shi, C., Qin, X., et al. (2022). Identification of optimal reference genes for gene expression normalization in human osteosarcoma cell lines under proliferative conditions. Front. Genet. 13, 989990. doi:10.3389/fgene.2022.989990
Dundas, J., and Ling, M. (2012). Reference genes for measuring mRNA expression. Theory Biosci. 131 (4), 215–223. doi:10.1007/s12064-012-0152-5
Freitas, F. C. P., Depintor, T. S., Agostini, L. T., Luna-Lucena, D., Nunes, F. M. F., Bitondi, M. M. G., et al. (2019). Evaluation of reference genes for gene expression analysis by real-time quantitative PCR (qPCR) in three stingless bee species (Hymenoptera: apidae: Meliponini). Sci. Rep. 9 (1), 17692. doi:10.1038/s41598-019-53544-0
Gubern, C., Hurtado, O., Rodríguez, R., Morales, J. R., Romera, V. G., Moro, M. A., et al. (2009). Validation of housekeeping genes for quantitative real-time PCR in in-vivo and in-vitro models of cerebral ischaemia. BMC Mol. Biol. 10, 57. doi:10.1186/1471-2199-10-57
Julian, G. S., de Oliveira, R. W., Perry, J. C., Tufik, S., and Chagas, J. R. (2014). Validation of housekeeping genes in the brains of rats submitted to chronic intermittent hypoxia, a sleep apnea model. PLoS One 9 (10), e109902. doi:10.1371/journal.pone.0109902
Köhsler, M., Leitsch, D., Müller, N., and Walochnik, J. (2020). Validation of reference genes for the normalization of RT-qPCR gene expression in Acanthamoeba spp. Sci. Rep. 10 (1), 10362. doi:10.1038/s41598-020-67035-0
Kozera, B., and Rapacz, M. (2013). Reference genes in real-time PCR. J. Appl. Genet. 54 (4), 391–406. doi:10.1007/s13353-013-0173-x
Kumar, S., Ahmad, A., Kushwaha, N., Shokeen, N., Negi, S., Gautam, K., et al. (2022). Selection of ideal reference genes for gene expression analysis in COVID-19 and mucormycosis. Microbiol. Spectr. 10 (6), e0165622. doi:10.1128/spectrum.01656-22
Lewczuk, A., Boratyńska-Jasińska, A., and Zabłocka, B. (2023). Validation of the reference genes for expression analysis in the Hippocampus after transient ischemia/reperfusion injury in gerbil brain. Int. J. Mol. Sci. 24 (3), 2756. doi:10.3390/ijms24032756
Molomjamts, M., and Ingolfsland, E. C. (2023). Identification of reference genes for the normalization of retinal mRNA expression by RT-qPCR in oxygen induced retinopathy, anemia, and erythropoietin administration. PLoS One 18 (4), e0284764. doi:10.1371/journal.pone.0284764
Silveira, G. O., Amaral, M. S., Coelho, H. S., Maciel, L. F., Pereira, A. S. A., Olberg, G. G. O., et al. (2021). Assessment of reference genes at six different developmental stages of Schistosoma mansoni for quantitative RT-PCR. Sci. Rep. 11 (1), 16816. doi:10.1038/s41598-021-96055-7
Song, J., Cho, J., Park, J., and Hwang, J. H. (2022). Identification and validation of stable reference genes for quantitative real time PCR in different minipig tissues at developmental stages. BMC Genomics 23 (1), 585. doi:10.1186/s12864-022-08830-z
Vandesompele, J., De Preter, K., Pattyn, F., Poppe, B., Van Roy, N., De Paepe, A., et al. (2002). Accurate normalization of real-time quantitative RT-PCR data by geometric averaging of multiple internal control genes. Genome Biol. 3 (7), RESEARCH0034. doi:10.1186/gb-2002-3-7-research0034
Yi, S., Lin, Q., Zhang, X., Wang, J., Miao, Y., and Tan, N. (2020). Selection and validation of appropriate reference genes for quantitative RT-PCR analysis in rubia yunnanensis diels based on transcriptome data. Biomed. Res. Int. 2020, 5824841. doi:10.1155/2020/5824841
Keywords: real-time quantitative polymerase chain reaction, reference genes, 18S rRNA analysis, THP-1 cell line, in silico prediction software
Citation: de Sousa PRC, da Silva ASM, de Ponte CGG, Nogueira LL, Frota CC and Antas PRZ (2024) Commentary: Identification of optimal reference genes for gene expression normalization in human osteosarcoma cell lines under proliferative conditions. Front. Genet. 15:1342447. doi: 10.3389/fgene.2024.1342447
Received: 21 November 2023; Accepted: 02 January 2024;
Published: 25 January 2024.
Edited by:
Xiongwen Lv, Anhui Medical University, ChinaReviewed by:
John Hildyard, Royal Veterinary College (RVC), United KingdomHassan Dastsooz, University of Turin, Italy
Copyright © 2024 de Sousa, da Silva, de Ponte, Nogueira, Frota and Antas. This is an open-access article distributed under the terms of the Creative Commons Attribution License (CC BY). The use, distribution or reproduction in other forums is permitted, provided the original author(s) and the copyright owner(s) are credited and that the original publication in this journal is cited, in accordance with accepted academic practice. No use, distribution or reproduction is permitted which does not comply with these terms.
*Correspondence: Paulo R. Z. Antas, paulo.zuquim@fiocruz.br