- 1Department of Gastrointestinal Surgery, The First Affiliated Hospital of Wenzhou Medical University, Wenzhou, China
- 2Department of Medical Oncology, Sir Run Run Shaw Hospital, School of Medicine, Graduate School, Zhejiang University, Hangzhou, China
- 3The First School of Medicine, School of Information and Engineering, Wenzhou Medical University, Wenzhou, China
- 4School of Public Health and Management, Wenzhou Medical University, Wenzhou, China
- 5School of Basic Medicine, Wenzhou Medical University, Wenzhou, China
Background: Previous studies have suggested an association between Type 1 diabetes (T1D) and autoimmune diseases (AIDs), but the causal relationship remains unclear. Therefore, this study utilizes publicly available Genome-Wide Association Studies (GWAS) databases and employs a two-sample Mendelian Randomization (MR) approach to explore the causal relationships between T1D and systemic lupus erythematosus (SLE), rheumatoid arthritis (RA), and inflammatory bowel disease (IBD).
Methods: Summary GWAS data for T1D, SLE, RA, and IBD were downloaded from open GWAS databases and the International Inflammatory Bowel Disease Genetics Consortium (IIBDGC). We employed a series of methods to select instrumental variables closely related to T1D. To enhance the reliability of our conclusions, we applied multiple robust analytical methods, with the inverse variance weighted (IVW) method as the primary approach. Validation and meta-analysis were conducted using the FinnGen consortium. Additionally, we assessed heterogeneity, pleiotropy, and sensitivity to ensure the robustness of our conclusions.
Results: A potential causal association was found between T1D and SLE (OR = 1.37, 95% CI = 1.26 – 1.49, P < 0.001), which was further confirmed by meta-analysis. Similarly, a potential causal association was found between T1D and RA (OR = 1.32, 95% CI = 1.17 – 1.50, P < 0.001), and this was also confirmed by meta-analysis. Although the association between T1D and IBD showed P < 0.05, the leave-one-out test did not pass, and further meta-analysis indicated no significant statistical association between them.
Conclusion: Our study reveals the relationships between T1D and three clinically common autoimmune diseases (SLE, RA, and IBD). This research supplements previous studies and provides a reference for future clinical work.
Introduction
Autoimmune diseases (AIDs) are a group of complex chronic diseases of unknown etiology characterized by defects in immune tolerance. Common autoimmune diseases include systemic lupus erythematosus (SLE), rheumatoid arthritis (RA), and inflammatory bowel disease (IBD) (Gao et al., 2021). In the United States, autoimmune diseases are one of the leading causes of death among young and middle-aged women (Cooper and Stroehla, 2003). Additionally, because these conditions are often lifelong, they impose a significant burden on both society and individuals (Roberts and Erdei, 2020; Rose, 2016).
Type 1 diabetes (T1D) is an autoimmune disease characterized by insulin deficiency and resultant hyperglycemia (DiMeglio et al., 2018). It commonly occurs in individuals aged 10–14 years (DiMeglio et al., 2018; Maahs et al., 2010). The current understanding is that its pathogenesis may be related to a T-cell-mediated autoimmune process targeting pancreatic β-cells, with its incidence increasing globally (Vehik and Dabelea, 2011). The relationship between T1D and autoimmune diseases has long been both intriguing and perplexing. Clinically, it has been observed that patients with T1D often have other autoimmune diseases, such as dermatological and rheumatic conditions (Popoviciu et al., 2023). Research indicates that T1D and other autoimmune diseases may share certain pathways or genes (Szymczak et al., 2021). However, the causal relationship between T1D and other autoimmune diseases remains unclear.
Observational studies may struggle to correctly determine causality or may produce spurious associations due to the presence of some unavoidable biases (Boyko, 2013). Therefore, in this study, we use Mendelian Randomization (MR) to further investigate the causal relationship between T1D and three clinically common autoimmune diseases (SLE RA and IBD). Mendelian Randomization uses genetic variation as an instrumental variable for the exposure, thereby determining the causal relationship between the exposure and the outcome (Davey Smith and Hemani, 2014; Yarmolinsky et al., 2018). This method can avoid reverse causation and potential confounding biases, making the results more convincing (Zoccali et al., 2006).
Materials and method
Study Design
Mendelian Randomization (MR) studies typically use single nucleotide polymorphisms (SNPs) as instrumental variables (IVs). Conducting an MR analysis requires meeting the following three assumptions (Figure 1): (1) the IVs are strongly associated with the exposure; (2) the IVs are not associated with potential confounders; (3) the IVs influence the outcome only through the exposure. The data used in this study are publicly available and free, thus no further ethical review or patient consent is required.
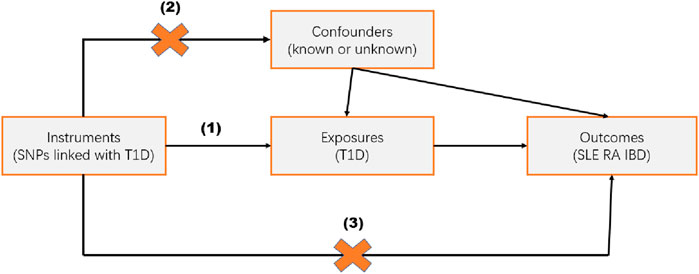
Figure 1. Study Design. Mendelian randomization studies are based on three assumptions: (1) the instrumental variable (IV) is strongly associated with the exposure; (2) the IV is independent of known or unknown confounders; (3) the IV influences the outcome only through the exposure.
Data sources
To ensure the robustness of the results and the generalizability of the conclusions, we selected databases from two different sources for each outcome. Details of the data are shown in Table 1.
SNPs related to T1D were obtained from a large Genome-Wide Association Studies (GWAS) study, which included 9,266 cases and 15,574 controls (Forgetta et al., 2020).
SNPs related to SLE were obtained from a large GWAS study that included 5,201 cases and 9,066 controls (Bentham et al., 2015). Moreover, SLE data from the Finnish database (FinnGen) included 538 cases and 213,145 controls.
SNPs related to RA were obtained from a large GWAS study that included 14,361 cases and 43,923 controls (Okada et al., 2014). Moreover, RA data from the Finnish database (FinnGen) included 6,236 cases and 147,221 controls.
SNPs related to IBD were obtained from a study by the International Inflammatory Bowel Disease Genetics Consortium (IIBDGC), which is the largest genetic database for IBD globally. This study included 31,665 cases and 33,977 controls after quality control (QC) (Liu et al., 2015). In addition, IBD data from the Finnish database (FinnGen) included 5,673 cases and 213,119 controls.
When multiple GWAS databases were available, we prioritized those with larger sample sizes, more SNPs, and greater citation frequency by researchers.
Meta-analysis
To validate the robustness of the results, we further verified the outcomes within the FinnGen consortium. Subsequently, we conducted a meta-analysis to further ascertain the relationship between T1D and the different autoimmune diseases. In the meta-analysis, a random effects model was used if heterogeneity (p < 0.05) was present; if no heterogeneity was detected (p > 0.05), a fixed effects model was employed.
Selection of genetic instruments
To ensure adherence to the assumptions of Mendelian Randomization, we selected instrumental variables based on the following criteria (Gagliano Taliun and Evans, 2021): we used a threshold of p < 5 × 10^ -8 as the primary filter to ensure that the SNPs were strongly associated with the characteristics of T1D. Moreover, we excluded SNPs in linkage disequilibrium (LD) (R^ 2 < 0.001, clumped at 10,000 kb). We also calculated the F-statistic to test for bias due to weak instruments, using the formula: F = β ^2/se^ 2 (Wang et al., 2022; Zhao et al., 2023; Li and Martin, 2002). An F-statistic greater than 10 was required to minimize bias from weak instruments (Burgess et al., 2011).
Statistical analysis
In this study, MR analysis was conducted using the TwoSampleMR package (version 0.5.6) and R software (version 4.2.1) (Yavorska and Burgess, 2017). Meta-analysis was performed using Review Manager (version 5.4). The primary analysis method was the Inverse Variance Weighted (IVW) approach, which combines the Wald ratio estimates of each SNP to produce a pooled estimate (Pierce and Burgess, 2013). Supplementary analyses included: (1). Weighted Median (Bowden et al., 2016). This method can provide consistent estimates of causal effects even if up to 50% of the instruments are invalid; (2). MR Egger (Bowden et al., 2015). This method offers consistent estimates of pleiotropy even if all instruments are invalid; 3. MR-PRESSO (Verbanck et al., 2018). This method identifies outliers with horizontal pleiotropy and is most effective when less than 50% of the instruments exhibit horizontal pleiotropy. Cochran’s Q test was used to detect heterogeneity (Greco et al., 2015). The intercept test from MR Egger regression was employed to evaluate horizontal pleiotropy (Bowden et al., 2015).
Results
Selection of instrumental variables
We selected IVs based on the criteria outlined above. Ultimately, we identified 44 SNPs to be used as IVs for T1D. Moreover, all F-statistics were greater than 10, indicating the absence of weak instrument bias (Supplementary Table S1).
Relationship between T1D and SLE
In this study, we found that T1D exhibited a positive association with SLE (OR = 1.37, 95% CI = 1.26–1.49, p < 0.001). This result remained robust even after removing outliers using the MR-PRESSO method (OR = 1.11, 95% CI = 1.02–1.20, p = 0.018) (Figures 2, 4; Table 2). Within the FinnGen consortium, T1D continued to show a positive association with SLE (OR = 1.18, 95% CI = 1.10–1.27, p < 0.001) (Figures 3, 4; Table 2). Meta-analysis further confirmed the relationship between the two (OR = 1.27, 95% CI = 1.10–1.46, p = 0.001) (Figure 5).
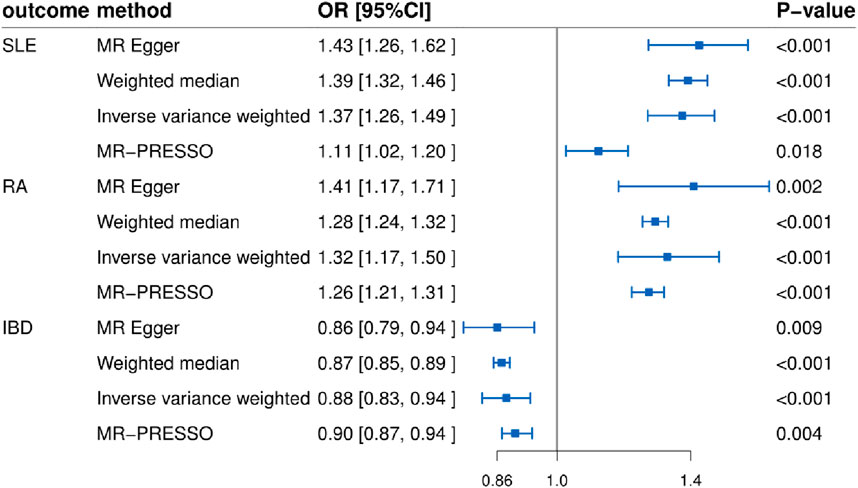
Figure 2. Forest plots showing the relationship between T1D and SLE, RA, and IBD (non-FinnGen databases).
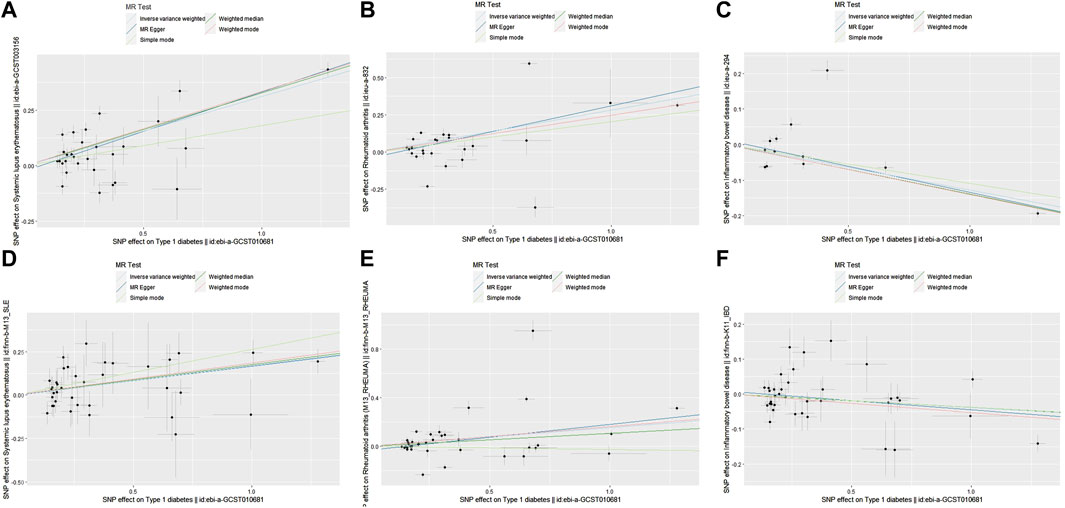
Figure 4. Scatter plots showing the relationship between T1D and SLE, RA, IBD. (A) T1D and SLE (non-FinnGen databases); (B) T1D and RA (non-FinnGen databases) (C) T1D and IBD (non-FinnGen databases); (D) T1D and SLE (FinnGen database) (E) T1D and RA (FinnGen database); (F) T1D and IBD (FinnGen database).
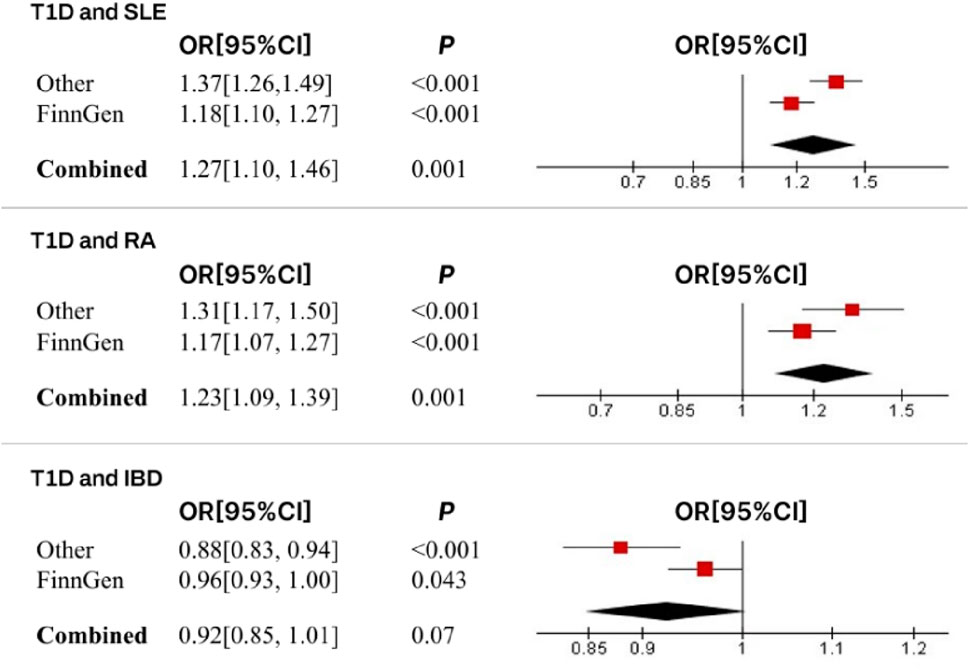
Figure 5. Meta-analysis results and forest plots for the relationship between T1D and SLE, RA, and IBD.
Relationship between T1D and RA
In our study, we found a positive association between T1D and RA (OR = 1.32, 95% CI = 1.17–1.50, p < 0.001). This positive association persisted even after removing outliers using the MR-PRESSO method (OR = 1.26, 95% CI = 1.21–1.31, p < 0.001) (Figures 2, 4; Table 2). This conclusion was also validated using data from the FinnGen consortium (OR = 1.17, 95% CI = 1.07–1.27, p < 0.001) (Figures 3, 4; Table 2). Meta-analysis further confirmed the relationship between T1D and RA (OR = 1.23, 95% CI = 1.09–1.39, p = 0.001) (Figure 5).
Relationship between T1D and IBD
In this study, our analysis indicated a negative association between T1D and IBD (OR = 0.88, 95% CI = 0.83–0.94, p < 0.001) (Figures 2, 4; Table 2). However, it is noteworthy that the leave-one-out analysis suggested that this relationship might be disproportionately influenced by a single SNP, indicating potential bias in the results (Supplementary Figure S1). In the FinnGen consortium, a similar trend was observed between T1D and IBD (OR = 0.96, 95% CI = 0.93–1.00, p = 0.043) (Figures 3, 4; Table 2), but the leave-one-out analysis still indicated potential bias (Supplementary Figure S1). Meta-analysis showed no significant statistical difference (OR = 0.92, 95% CI = 0.85–1.01, p = 0.07) (Figure 5).
MR sensitivity analysis results
The MR Egger regression intercept indicated limited evidence of horizontal pleiotropy (Table 3). For T1D and SLE, as well as T1D and RA, the leave-one-out analysis demonstrated that the causal associations were not unduly influenced by any single SNP. However, the leave-one-out analysis suggested that the causal association between T1D and IBD might be disproportionately affected by a single SNP. Heterogeneity tests for each group are presented in Table 3. The forest plots and volcano plots provide a more visual representation of the heterogeneity (Supplementary Figures S2, S3).
Discussion
In this study, we used MR to evaluate the causal relationships between T1D and several clinically common autoimmune diseases. Our research indicated that genetic susceptibility to T1D was associated with an increased risk of both SLE and RA, but not with IBD.
T1D is a complex chronic disease that is often found to co-occur with other autoimmune diseases in clinical settings (Zeglaoui et al., 2010; Çetın et al., 2013). A study from Sweden involving 3,093 participants demonstrated a significant association between T1D and RA (OR = 4.9, 95% CI = 1.8, 13.1), which is consistent with our findings (Liao et al., 2009). Although previous views suggested that T1D is not an independent risk factor for RA (Popoviciu et al., 2023), our analysis indicated a possible causal relationship, and Zhernakova et al. have also identified shared genetic risk loci between T1D and RA (Zhernakova et al., 2007). Similarly, a study based on the HealthFacts database showed that patients with T1D are more likely to develop SLE, another rheumatic disease (1325/158865) (Bao et al., 2019), compared to an incidence rate of approximately 23.2 per 100,000 in the general North American population (Popoviciu et al., 2023). Additionally, both RA and SLE are more commonly co-morbid in female T1D patients than in males (Bao et al., 2019; Bao et al., 2018). Therefore, clinicians should be vigilant in preventing rheumatic diseases in T1D patients, especially in females, to reduce potential risks and economic burdens on patients.
Although SLE and RA are distinct diseases, they both fall under the category of rheumatic diseases. Previous researches have shown that RA and SLE share familial aggregation (Cardenas-Roldan et al., 2013), genetic (Cui et al., 2013; Orozco et al., 2011; Marquez et al., 2017), molecular mechanisms (Higgs et al., 2011), and targeted therapies (Petitdemange et al., 2020), which might partially explain why both are associated with T1D. Studies have indicated that the interleukin two receptor subunit alpha (IL2RA) gene is closely related to the onset of T1D (Pahkuri et al., 2023), and IL2RA is also implicated in the pathogenesis of SLE and RA (Gorji et al., 2019; van Steenbergen et al., 2015). Our study also identified that mutations in the IL2RA gene (rs12722495) might contribute to the associations observed between these conditions.
The causal relationship between T1D and IBD has long been debated. A study from Denmark indicated a significant association between T1D and IBD (Halling et al., 2017). However, other studies have found no significant association between the two (Cohen et al., 2008; Lu et al., 2020), which aligns with our findings. Although our results confirm some previous clinical studies, several important points deserve attention: Firstly, T1D commonly occurs in individuals aged 10–14 years (DiMeglio et al., 2018; Maahs et al., 2010), whereas IBD tends to develop in young and middle-aged adults (He et al., 2022). This study targeted an adult population. For the pediatric population, a study involving 1,200 cases found an association between IBD and diabetes (Kappelman et al., 2011). Additionally, research from Austria and Germany observed a higher incidence of IBD in children with T1D compared to their age-matched peers (Jasser-Nitsche et al., 2021). Therefore, the relationship between T1D and early-onset IBD in children warrants further investigation. Secondly, although our study did not find a statistically significant relationship between T1D and IBD, the P-value was close to 0.05, suggesting a potential negative association trend. Previous studies have shown that protein tyrosine phosphatase non-receptor type 22 (PTPN22) plays an opposing role in Crohn’s disease compared to T1D (Barrett et al., 2008). Research indicates that PTPN22 knockdown activates inflammatory signaling pathways, leading to Crohn’s disease (Spalinger et al., 2013). Conversely, PTPN22 knockdown does not increase the risk of T1D and may even confer protective effects (Zheng and Kissler, 2013). Similarly, risk alleles for T1D, such as Interleukin 27 (IL-27), Interleukin 10 (IL-10), and interleukin-18 receptor 1 (IL18RA), have been found to prevent Crohn’s disease. Major histocompatibility complex (MHC) alleles strongly associated with T1D risk also appear to prevent both Crohn’s disease and ulcerative colitis (Wang et al., 2010). In contrast, PTPN22 is implicated in promoting the development of RA and SLE (Liao et al., 2009; Deng and Tsao, 2010). This intriguing phenomenon may relate to the “direction” of genetic variants: if a variant is associated with multiple autoimmune diseases but in opposite directions, it is more likely to be involved in pathways related to immune function, exhibiting contrasting characteristics (Wang et al., 2010).
Compared with traditional research methods, our study has several advantages. Firstly, we used Mendelian Randomization to evaluate the relationship between T1D and other autoimmune diseases. This method significantly reduces the impact of confounding bias and reverse causation. Secondly, our instrumental variables were derived from large-scale GWAS, providing reliable and robust SNP data, which helps avoid bias from weak instruments. Additionally, we conducted meta-analyses to further assess the reliability of our results.
However, our study has some limitations. Firstly, although we used various methods to analyze horizontal pleiotropy, we cannot entirely rule out the presence of potential horizontal pleiotropy. Secondly, Mendelian Randomization itself may face new issues, such as the “winner’s curse” (Yarmolinsky et al., 2018). Lastly, our study was limited by its focus on a specific ethnic group, so the conclusions might not be generalizable to other populations.
Conclusion
In summary, our study further substantiated the causal relationships between T1D and both RA and SLE, while no association was found between T1D and IBD. These findings suggested that in managing patients with T1D, attention should be given to preventing RA and SLE to reduce potential complications and economic burdens for patients.
Data availability statement
The original contributions presented in the study are included in the article/Supplementary Material, further inquiries can be directed to the corresponding authors.
Ethics statement
Ethical approval was not required for the study involving humans in accordance with the local legislation and institutional requirements. Written informed consent to participate in this study was not required from the participants or the participant’s legal guardians/next of kin in accordance with the national legislation and the institutional requirements.
Author contributions
WX: Supervision, Writing–original draft, Writing–review and editing. HJ: Conceptualization, Investigation, Methodology, Project administration, Software, Writing–review and editing. YC: Conceptualization, Project administration, Validation, Writing–review and editing. ZY: Validation, Writing–review and editing. YS: Validation, Writing–review and editing. HZ: Validation, Writing–review and editing. SL: Writing–review and editing, Funding acquisition. SH: Writing–review and editing, Project administration. NL: Writing–review and editing, Funding acquisition, Project administration.
Funding
The author(s) declare that financial support was received for the research, authorship, and/or publication of this article. This project was sponsored by the Zhejiang Medical and Health Project (Key Talents) (2014RCA014), Wenzhou Municipal Science and Bureau (Y20210184), Zhejiang Provincial Health Department Medical Support Discipline–Nutrition (11-ZC24), Natural Science Foundation of Zhejiang Province (LQ20H020002), General Research Project of Zhejiang Provincial Department of Education (Y201942047) and Wenzhou Science and Technology Program (Y20180060).
Acknowledgments
We are grateful to all organizations that provide publicly available data.
Conflict of interest
The authors declare that the research was conducted in the absence of any commercial or financial relationships that could be construed as a potential conflict of interest.
Publisher’s note
All claims expressed in this article are solely those of the authors and do not necessarily represent those of their affiliated organizations, or those of the publisher, the editors and the reviewers. Any product that may be evaluated in this article, or claim that may be made by its manufacturer, is not guaranteed or endorsed by the publisher.
Supplementary material
The Supplementary Material for this article can be found online at: https://www.frontiersin.org/articles/10.3389/fgene.2024.1335839/full#supplementary-material
References
Bao, Y. K., Salam, M., Parks, D., McGill, J. B., and Hughes, J. (2018). High prevalence of systemic rheumatic diseases in women with type 1 diabetes. J. Diabetes Complicat. 32 (8), 737–739. doi:10.1016/j.jdiacomp.2018.06.001
Bao, Y. K., Weide, L. G., Ganesan, V. C., Jakhar, I., McGill, J. B., Sahil, S., et al. (2019). High prevalence of comorbid autoimmune diseases in adults with type 1 diabetes from the HealthFacts database. J. Diabetes 11 (4), 273–279. doi:10.1111/1753-0407.12856
Barrett, J. C., Hansoul, S., Nicolae, D. L., Cho, J. H., Duerr, R. H., Rioux, J. D., et al. (2008). Genome-wide association defines more than 30 distinct susceptibility loci for Crohn's disease. Nat. Genet. 40 (8), 955–962. doi:10.1038/ng.175
Bentham, J., Morris, D. L., Graham, D. S. C., Pinder, C. L., Tombleson, P., Behrens, T. W., et al. (2015). Genetic association analyses implicate aberrant regulation of innate and adaptive immunity genes in the pathogenesis of systemic lupus erythematosus. Nat. Genet. 47 (12), 1457–1464. doi:10.1038/ng.3434
Bowden, J., Davey Smith, G., and Burgess, S. (2015). Mendelian randomization with invalid instruments: effect estimation and bias detection through Egger regression. Int. J. Epidemiol. 44 (2), 512–525. doi:10.1093/ije/dyv080
Bowden, J., Davey Smith, G., Haycock, P. C., and Burgess, S. (2016). Consistent estimation in mendelian randomization with some invalid instruments using a weighted median estimator. Genet. Epidemiol. 40 (4), 304–314. doi:10.1002/gepi.21965
Boyko, E. J. (2013). Observational research-opportunities and limitations. J. Diabetes Complicat. 27 (6), 642–648. doi:10.1016/j.jdiacomp.2013.07.007
Burgess, S., and Thompson, S. G.CRP CHD Genetics Collaboration (2011). Avoiding bias from weak instruments in Mendelian randomization studies. Int. J. Epidemiol. 40 (3), 755–764. doi:10.1093/ije/dyr036
Cardenas-Roldan, J., Rojas-Villarraga, A., and Anaya, J. M. (2013). How do autoimmune diseases cluster in families? A systematic review and meta-analysis. BMC Med. 11, 73. doi:10.1186/1741-7015-11-73
Çetın, D., Ünübol, M., Güney, E., Karaoğlu, A. Ö., Meteoğlu, İ., and Bozkurt, G. (2013). Coexistence of type 1 diabetes mellitus and Crohn's disesae. Turk J. Gastroenterol. 24 (5), 451–452. doi:10.4318/tjg.2013.0513
Cohen, R., Robinson, D., Paramore, C., Fraeman, K., Renahan, K., and Bala, M. (2008). Autoimmune disease concomitance among inflammatory bowel disease patients in the United States, 2001-2002. Inflamm. Bowel Dis. 14 (6), 738–743. doi:10.1002/ibd.20406
Cooper, G. S., and Stroehla, B. C. (2003). The epidemiology of autoimmune diseases. Autoimmun. Rev. 2 (3), 119–125. doi:10.1016/s1568-9972(03)00006-5
Cui, Y., Sheng, Y., and Zhang, X. (2013). Genetic susceptibility to SLE: recent progress from GWAS. J. Autoimmun. 41, 25–33. doi:10.1016/j.jaut.2013.01.008
Davey Smith, G., and Hemani, G. (2014). Mendelian randomization: genetic anchors for causal inference in epidemiological studies. Hum. Mol. Genet. 23 (R1), R89–R98. doi:10.1093/hmg/ddu328
Deng, Y., and Tsao, B. P. (2010). Genetic susceptibility to systemic lupus erythematosus in the genomic era. Nat. Rev. Rheumatol. 6 (12), 683–692. doi:10.1038/nrrheum.2010.176
DiMeglio, L. A., Evans-Molina, C., and Oram, R. A. (2018). Type 1 diabetes. Lancet 391 (10138), 2449–2462. doi:10.1016/S0140-6736(18)31320-5
Forgetta, V., Manousaki, D., Istomine, R., Ross, S., Tessier, M. C., Marchand, L., et al. (2020). Rare genetic variants of large effect influence risk of type 1 diabetes. Diabetes 69 (4), 784–795. doi:10.2337/db19-0831
Gagliano Taliun, S. A., and Evans, D. M. (2021). Ten simple rules for conducting a mendelian randomization study. PLoS Comput. Biol. 17 (8), e1009238. doi:10.1371/journal.pcbi.1009238
Gao, Z. W., Wang, X., Zhang, H. Z., Lin, F., Liu, C., and Dong, K. (2021). The roles of adenosine deaminase in autoimmune diseases. Autoimmun. Rev. 20 (1), 102709. doi:10.1016/j.autrev.2020.102709
Gorji, A. E., Roudbari, Z., Alizadeh, A., and Sadeghi, B. (2019). Investigation of systemic lupus erythematosus (SLE) with integrating transcriptomics and genome wide association information. Gene 706, 181–187. doi:10.1016/j.gene.2019.05.004
Greco, M. F., Minelli, C., Sheehan, N. A., and Thompson, J. R. (2015). Detecting pleiotropy in Mendelian randomisation studies with summary data and a continuous outcome. Stat. Med. 34 (21), 2926–2940. doi:10.1002/sim.6522
Halling, M. L., Kjeldsen, J., Knudsen, T., Nielsen, J., and Hansen, L. K. (2017). Patients with inflammatory bowel disease have increased risk of autoimmune and inflammatory diseases. World J. Gastroenterol. 23 (33), 6137–6146. doi:10.3748/wjg.v23.i33.6137
He, B. J., Liu, Z. K., Shen, P., Sun, Y. X., Chen, B., Zhan, S. Y., et al. (2022). Epidemiological study on the incidence of inflammatory bowel disease in Yinzhou District, Ningbo City from 2011 to 2020. Beijing Da Xue Xue Bao Yi Xue Ban. 54 (3), 511–519. doi:10.19723/j.issn.1671-167X.2022.03.017
Higgs, B. W., Liu, Z., White, B., Zhu, W., White, W. I., Morehouse, C., et al. (2011). Patients with systemic lupus erythematosus, myositis, rheumatoid arthritis and scleroderma share activation of a common type I interferon pathway. Ann. Rheum. Dis. 70 (11), 2029–2036. doi:10.1136/ard.2011.150326
Jasser-Nitsche, H., Bechtold-Dalla Pozza, S., Binder, E., Bollow, E., Heidtmann, B., Lee-Barkley, Y. H., et al. (2021). Comorbidity of inflammatory bowel disease in children and adolescents with type 1 diabetes. Acta Paediatr. 110 (4), 1353–1358. doi:10.1111/apa.15643
Kappelman, M. D., Galanko, J. A., Porter, C. Q., and Sandler, R. S. (2011). Association of paediatric inflammatory bowel disease with other immune-mediated diseases. Arch. Dis. Child. 96 (11), 1042–1046. doi:10.1136/archdischild-2011-300633
Li, B., and Martin, E. B. (2002). An approximation to the F distribution using the chi-square distribution. Comput. statistics and data analysis 40 (1), 21–26. doi:10.1016/s0167-9473(01)00097-4
Liao, K. P., Gunnarsson, M., Källberg, H., Ding, B., Plenge, R. M., Padyukov, L., et al. (2009). Specific association of type 1 diabetes mellitus with anti-cyclic citrullinated peptide-positive rheumatoid arthritis. Arthritis Rheum. 60 (3), 653–660. doi:10.1002/art.24362
Liu, J. Z., van Sommeren, S., Huang, H., Ng, S. C., Alberts, R., Takahashi, A., et al. (2015). Association analyses identify 38 susceptibility loci for inflammatory bowel disease and highlight shared genetic risk across populations. Nat. Genet. 47 (9), 979–986. doi:10.1038/ng.3359
Lu, S., Gong, J., Tan, Y., and Liu, D. (2020). Epidemiologic association between inflammatory bowel diseases and type 1 diabetes mellitus: a meta-analysis. J. Gastrointestin Liver Dis. 29 (3), 407–413. doi:10.15403/jgld-798
Maahs, D. M., West, N. A., Lawrence, J. M., and Mayer-Davis, E. J. (2010). Epidemiology of type 1 diabetes. Endocrinol. Metab. Clin. North Am. 39 (3), 481–497. doi:10.1016/j.ecl.2010.05.011
Marquez, A., Vidal-Bralo, L., Rodríguez-Rodríguez, L., González-Gay, M. A., Balsa, A., González-Álvaro, I., et al. (2017). A combined large-scale meta-analysis identifies COG6 as a novel shared risk locus for rheumatoid arthritis and systemic lupus erythematosus. Ann. Rheum. Dis. 76 (1), 286–294. doi:10.1136/annrheumdis-2016-209436
Okada, Y., Wu, D., Trynka, G., Raj, T., Terao, C., Ikari, K., et al. (2014). Genetics of rheumatoid arthritis contributes to biology and drug discovery. Nature 506 (7488), 376–381. doi:10.1038/nature12873
Orozco, G., Eyre, S., Hinks, A., Bowes, J., Morgan, A. W., Wilson, A. G., et al. (2011). Study of the common genetic background for rheumatoid arthritis and systemic lupus erythematosus. Ann. Rheum. Dis. 70 (3), 463–468. doi:10.1136/ard.2010.137174
Pahkuri, S., Ekman, I., Vandamme, C., Näntö-Salonen, K., Toppari, J., Veijola, R., et al. (2023). DNA methylation differences within INS, PTPN22 and IL2RA promoters in lymphocyte subsets in children with type 1 diabetes and controls. Autoimmunity 56 (1), 2259118. doi:10.1080/08916934.2023.2259118
Petitdemange, A., Blaess, J., Sibilia, J., Felten, R., and Arnaud, L. (2020). Shared development of targeted therapies among autoimmune and inflammatory diseases: a systematic repurposing analysis. Ther. Adv. Musculoskelet. Dis. 12, 1759720X20969261. doi:10.1177/1759720X20969261
Pierce, B. L., and Burgess, S. (2013). Efficient design for Mendelian randomization studies: subsample and 2-sample instrumental variable estimators. Am. J. Epidemiol. 178 (7), 1177–1184. doi:10.1093/aje/kwt084
Popoviciu, M. S., Kaka, N., Sethi, Y., Patel, N., Chopra, H., and Cavalu, S. (2023). Type 1 diabetes mellitus and autoimmune diseases: a critical review of the association and the application of personalized medicine. J. Pers. Med. 13 (3), 422. doi:10.3390/jpm13030422
Roberts, M. H., and Erdei, E. (2020). Comparative United States autoimmune disease rates for 2010-2016 by sex, geographic region, and race. Autoimmun. Rev. 19 (1), 102423. doi:10.1016/j.autrev.2019.102423
Rose, N. R. (2016). Prediction and prevention of autoimmune disease in the 21st century: a review and preview. Am. J. Epidemiol. 183 (5), 403–406. doi:10.1093/aje/kwv292
Spalinger, M. R., Lang, S., Weber, A., Frei, P., Fried, M., Rogler, G., et al. (2013). Loss of protein tyrosine phosphatase nonreceptor type 22 regulates interferon-γ-induced signaling in human monocytes. Gastroenterology 144 (5), 978–988. doi:10.1053/j.gastro.2013.01.048
Szymczak, F., Colli, M. L., Mamula, M. J., Evans-Molina, C., and Eizirik, D. L. (2021). Gene expression signatures of target tissues in type 1 diabetes, lupus erythematosus, multiple sclerosis, and rheumatoid arthritis. Sci. Adv. 7 (2), eabd7600. doi:10.1126/sciadv.abd7600
van Steenbergen, H. W., van Nies, J. A. B., Ruyssen-Witrand, A., Huizinga, T. W. J., Cantagrel, A., Berenbaum, F., et al. (2015). IL2RA is associated with persistence of rheumatoid arthritis. Arthritis Res. Ther. 17 (1), 244. doi:10.1186/s13075-015-0739-6
Vehik, K., and Dabelea, D. (2011). The changing epidemiology of type 1 diabetes: why is it going through the roof? Diabetes Metab. Res. Rev. 27 (1), 3–13. doi:10.1002/dmrr.1141
Verbanck, M., Chen, C. Y., and Neale, B. (2018). Detection of widespread horizontal pleiotropy in causal relationships inferred from Mendelian randomization between complex traits and diseases. Nat. Genet. 50 (5), 693–698. doi:10.1038/s41588-018-0099-7
Wang, B., Liu, D., Song, M., Wang, W., Guo, B., and Wang, Y. (2022). Immunoglobulin G N-glycan, inflammation and type 2 diabetes in East Asian and European populations: a Mendelian randomization study. Mol. Med. 28 (1), 114. doi:10.1186/s10020-022-00543-z
Wang, K., Baldassano, R., Zhang, H., Qu, H. Q., Imielinski, M., Kugathasan, S., et al. (2010). Comparative genetic analysis of inflammatory bowel disease and type 1 diabetes implicates multiple loci with opposite effects. Hum. Mol. Genet. 19 (10), 2059–2067. doi:10.1093/hmg/ddq078
Yarmolinsky, J., Wade, K. H., Richmond, R. C., Langdon, R. J., Bull, C. J., Tilling, K. M., et al. (2018). Causal inference in cancer epidemiology: what is the role of mendelian randomization? Cancer Epidemiol. Biomarkers Prev. 27 (9), 995–1010. doi:10.1158/1055-9965.EPI-17-1177
Yavorska, O. O., and Burgess, S. (2017). MendelianRandomization: an R package for performing Mendelian randomization analyses using summarized data. Int. J. Epidemiol. 46 (6), 1734–1739. doi:10.1093/ije/dyx034
Zeglaoui, H., Landolsi, H., Mankai, A., Ghedira, I., and Bouajina, E. (2010). Type 1 diabetes mellitus, celiac disease, systemic lupus erythematosus and systemic scleroderma in a 15-year-old girl. Rheumatol. Int. 30 (6), 793–795. doi:10.1007/s00296-009-0988-2
Zhao, S. S., Yiu, Z. Z. N., Barton, A., and Bowes, J. (2023). Association of lipid-lowering drugs with risk of psoriasis: a mendelian randomization study. JAMA Dermatol 159 (3), 275–280. doi:10.1001/jamadermatol.2022.6051
Zheng, P., and Kissler, S. (2013). PTPN22 silencing in the NOD model indicates the type 1 diabetes-associated allele is not a loss-of-function variant. Diabetes 62 (3), 896–904. doi:10.2337/db12-0929
Zhernakova, A., Alizadeh, B. Z., Bevova, M., van Leeuwen, M. A., Coenen, M. J. H., Franke, B., et al. (2007). Novel association in chromosome 4q27 region with rheumatoid arthritis and confirmation of type 1 diabetes point to a general risk locus for autoimmune diseases. Am. J. Hum. Genet. 81 (6), 1284–1288. doi:10.1086/522037
Keywords: systemic lupus erythematosus (SLE), rheumatoid arthritis (RA), inflammatory bowel disease (IBD), type 1 diabetes(T1D), Mendelian randomization(MR)
Citation: Xie W, Jiang H, Chen Y, Yu Z, Song Y, Zhang H, Li S, Han S and Liu N (2024) Relationship between type 1 diabetes and autoimmune diseases in european populations: A two-sample Mendelian randomization study. Front. Genet. 15:1335839. doi: 10.3389/fgene.2024.1335839
Received: 15 January 2024; Accepted: 22 August 2024;
Published: 16 September 2024.
Edited by:
Alex Tsoi, University of Michigan, United StatesReviewed by:
Yuxiang Fei, China Pharmaceutical University, ChinaJing-guo Chen, The Second Affiliated Hospital of Xi’an Jiaotong University, China
Copyright © 2024 Xie, Jiang, Chen, Yu, Song, Zhang, Li, Han and Liu. This is an open-access article distributed under the terms of the Creative Commons Attribution License (CC BY). The use, distribution or reproduction in other forums is permitted, provided the original author(s) and the copyright owner(s) are credited and that the original publication in this journal is cited, in accordance with accepted academic practice. No use, distribution or reproduction is permitted which does not comply with these terms.
*Correspondence: Naxin Liu, bGl1bmF4aW42OEB3emhvc3BpdGFsLmNu; Shaoliang Han, c2xoYW44OEAxMjYuY29t; Sen Li, bHp6MTg0MEB3bXUuZWR1LmNu