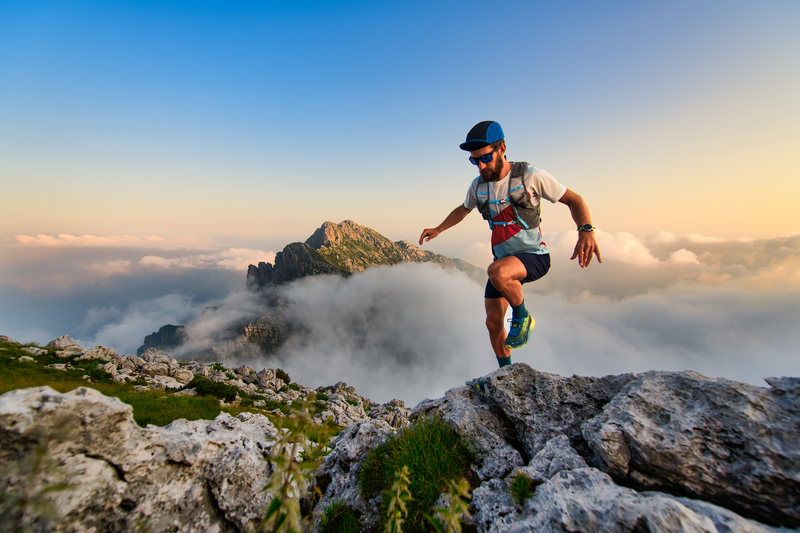
95% of researchers rate our articles as excellent or good
Learn more about the work of our research integrity team to safeguard the quality of each article we publish.
Find out more
ORIGINAL RESEARCH article
Front. Genet. , 07 February 2024
Sec. Cancer Genetics and Oncogenomics
Volume 15 - 2024 | https://doi.org/10.3389/fgene.2024.1270302
Background: Emerging evidence points to the exceptional importance and value of m7G alteration in the diagnosis and prognosis of cancers. Nonetheless, a biomarker for precise screening of various cancer types has not yet been developed based on serum m7G-harboring miRNAs.
Methods: A total of 20,702 serum samples, covering 12 cancer types and consisting of 7,768 cancer samples and 12,934 cancer-free samples were used in this study. A m7G target miRNA diagnostic signature (m7G-miRDS) was established through the least absolute shrinkage and selection operator (LASSO) analyses in a training dataset (n = 10,351), and validated in a validation dataset (n = 10,351).
Results: The m7G-miRDS model, a 12 m7G-target-miRNAs signature, demonstrated high accuracy and was qualified for cancer detection. In the training and validation cohort, the area under the curve (AUC) reached 0.974 (95% CI 0.971–0.977) and 0.972 (95% CI 0.969–0.975), respectively. The m7G-miRDS showed superior sensitivity in each cancer type and had a satisfactory AUC in identifying bladder cancer, lung cancer and esophageal cancer. Additionally, the diagnostic performance of m7G-miRDS was not interfered by the gender, age and benign disease.
Conclusion: Our results greatly extended the value of serum circulating miRNAs and m7G in cancer detection, and provided a new direction and strategy for the development of novel biomarkers with high accuracy, low cost and less invasiveness for mass cancer screening, such as ncRNA modification.
Early cancer detection is crucial for reducing cancer-caused mortality, prolonging patient survival and lessening the social burden (Bray et al., 2018). Due to the defects of high cost, invasiveness, poor compliance, and especially low accuracy, large-scale cancer screening is neither possible nor cost-effective based on present existing methods (So et al., 2021). A novel biomarker with greater effectiveness and less invasiveness for large-scale cancer screening is urgently needed due to the fact that early diagnosis of cancer could greatly extend patient survival.
N7-methylguanosine (m7G), a prevalent posttranscriptional modifications of RNA, is widely observed in both mRNAs and non-coding RNAs (e.g., tRNAs; micro-RNAs, miRNAs) (Luo et al., 2021; Wang et al., 2023), and plays essential roles in RNA metabolism, processing and function (Chen et al., 2022). m7G modification is dynamical and mainly regulated by the methyltransferase-like 1 (METTL1)/WD repeat domain 4 (WDR4) (Alexandrov et al., 2002), Williams–Beuren syndrome chromosome region 22 (WBSCR22)/tRNA methyltransferase activator subunit 11–2 (TRMT112) (Haag et al., 2015), and RNA guanine-7 methyltransferase (RNMT)/RNMT-activating miniprotein (RAM) (Trotman et al., 2017). Aberrant m7G modification was closely related to tumor occurrence and progression (Ma et al., 2021a; Luo et al., 2022). As the m7G methyltransferase of miRNAs, METTL1 exhibited hyperactivity in lung and bladder cancer, positively correlating with advanced clinical stages and high tumor grades (Pandolfini et al., 2019; Ying et al., 2021), while in colon cancer, it is significantly downregulated, actively participating in multiple tumor progression-related processes through its involvement in mediating miRNA maturation or in an m7G-tRNA codon-dependent manner (Ma et al., 2021b; Chen and Liu, 2021). These findings suggest that m7G plays a dual role in tumors and may unveil novel therapeutic targets for cancer patients.
miRNA is increasingly acknowledged as a valuable biomarker in various cancers. Recent studies have identified a subset of miRNAs with a modified m7G cap structure via different m7G sequencing techniques (Chen et al., 2022; Wang et al., 2023), such as miRNA let-7 (Chen and Liu, 2021) and miR-149-3p (Liu et al., 2019a). m7G can promote miRNA maturation or modulate miRNA binding to target genes, leading to alternations in the expression of their targets, including cancer-relevant genes. Previous studies have explored the prognostic value of m7G-related miRNAs and their association with immune infiltration in various cancer types (Yang et al., 2018; Hong et al., 2022; Zhang et al., 2022). In addition, Zhang et al. (2021) demonstrated the diagnostic performance of m6A target miRNAs in serum, with an area under the curve (AUC) of 0.979. Circulating miRNA, known for its highly stable as well as limited vulnerability to long-term storage at room temperature and freeze-thawing, is increasingly recognized as a valuable biomarker for cancer detection (Matsuzaki et al., 2021). However, the exploration of utilizing m7G target miRNAs (m7G-miRNAs) for cancer detection has not yet been undertaken.
Here, we utilized a total of 20,702 serum samples, covering 12 different cancer types and cancer-free controls, to establish a m7G-miRNAs diagnostic signature (m7G-miRDS) for cancer detection. The signature exhibited high accuracy in both the training and validation sets, with an AUC of 0.974 and 0.972, respectively. In addition, the m7G-miRDS showed excellent sensitivity in a particular cancer type, including bladder cancer, lung cancer and esophageal cancer. The diagnostic performance of m7G-miRDS was scarcely influenced by gender, age, or tumor stage. In summary, our study revealed the value of serum circulating m7G target miRNAs for cancer detection, and yielded a new path for developing novel biomarkers for large-scale cancer screening that are highly accurate, low cost and non-invasive for mass cancer screening, such as ncRNA modification.
We retrieved serum miRNA expression data and corresponding clinical information from the NCBI Gene Expression Omnibus (GEO) database, applying the following inclusion criteria: 1) Samples from cancer groups were collected from treatment-naive patients with clear diagnoses of malignant tumors; 2) Samples from control groups were obtained from healthy volunteers or individuals with benign diseases; 3) No duplicate samples were included. In total, 20,702 samples from ten individual GEO datasets (GSE106817, GSE112264, GSE113486, GSE113740, GSE122497, GSE137140, GSE139031, GSE85589, GSE164174, GSE124158), were enrolled in this study (Supplementary Table S1). Those datasets included 12,934 cancer-free and 7,768 cancer samples across 12 cancer types, including bladder urothelial carcinoma (BLCA), breast invasive carcinoma (BRCA), colorectal cancer (CRC), esophageal carcinoma (ESCA), glioma (GBM), gastric cancer (GC), hepatocellular carcinoma (HCC), lung cancer (LC), pancreatic adenocarcinoma (PAAD), prostate adenocarcinoma (PRAD), sarcoma (SARC), and ovarian cancer (OV).
The Affymetrix datasets along with corresponding annotation files were downloaded individually. When the probes were converted to gene symbols, if multiple probes corresponded to the same gene symbol and their mean value was taken as the final value. All miRNA expression were integrated once every dataset was processed, normalized, and log2 transformed. To detect and remove the batch effect caused by non-biotechnological bias, we conducted batch correction utilizing the ComBat function from the R package sva (v.3.38.0) (Leek et al., 2012). In total, an expression matrix consisting of 20,702 samples and 2,525 miRNAs was utilized for further analysis.
In this study, a total of 33 m7G target miRNAs were extracted from previously published studies (Liu et al., 2019b; Pandolfini et al., 2019) (Supplementary Table S2). To investigate the biological processes in which these miRNAs are involved, we initially predicted their potential targets using the miRWalk3.0 database (http://mirwalk.umm.uni-heidelberg.de/search_genes), with binding probability >95% and binding site position 3′UTR (Sticht et al., 2018). Only experimentally validated miRNA-target interactions from miRTarBase (https://mirtarbase.cuhk.edu.cn/), were considered for miRNA targets.
Differentially expressed m7G-harboring miRNAs between the cancer-free and cancer groups were identified using the R package limma (v3.10.3). The false discovery rate (FDR) was controlled using the Benjamini and Hochberg method. miRNAs with FDR <0.05 and |log2FC| >1, were considered significantly differentially expressed. Gene ontology (GO) enrichment and KEGG pathway enrichment analyses were performed to the biological functions and pathways associated with these genes using R package ClusterProfile (v3.6.0) (Yu et al., 2012).
To build a diagnostic signature for cancer detection, all enrolled samples (N = 20,702) were randomly equally divided into training and validation sets using the createDataPartition function of the R package caret (v6.0.88). Each group consisted of 10,351 samples, with 6,467 cancer-free controls and 3,884 cancers in each set. Details on those samples were presented in the Supplementary Table S3. Based on 22 differentially expressed m7G-harboring miRNAs (Supplementary Table S4), the least absolute shrinkage and selection operator (LASSO) analyses (100-fold cross-validation) was performed to determine the candidate m7G target miRNAs by using R package glmnet (v4.0.2). A diagnostic signature for cancer detection was established using 12 m7G target miRNAs (Supplementary Table S4). The model calculation formula is:
where Coefi is the LASSO coefficient of each miRNA, and
All statistical analyses and picture drawing were implemented in R statistical software (v4.0.5). The diagnostic performance of the m7G-miRNAs signature was evaluated by receiver operating characteristic (ROC) curve analysis, including the area under the curve (AUC), sensitivity, specificity and accuracy. The relationship of m7G-miRNAs and each miRNA was determined using Spearman correlation analysis. Statistical significance between groups was calculated by a Wilcoxon test or Kruskal‒Wallis test at a confidence interval (CI) of 95%, with p < 0.05 considered statistically significant.
A schematic diagram of the study design was presented in Figure 1A. In total, an integrative dataset of 20,702 serum samples and 33 m7G target miRNAs was utilized to build a m7G target microRNAs diagnostic signature (m7G-miRDS) for cancer detection. In the training and validation cohort, the average age of participants in the both was 62.5 years, and the proportion of female was 30.7% and 31.3%, respectively.
FIGURE 1. Identification of candidate m7G target miRNAs in serum. (A) Workflow illustrating the establishment of the serum m7G-miRNAs signature for cancer detection. (B) Boxplot showing 22 differentially expressed m7G target miRNAs between the cancer-free and cancer groups, according to the criteria of FDR <0.05 and log2|fold change| > 1. Statistical significance values correspond to p values as follows: **** <0.0001. (C) Heatmap of the expression of 12 candidate m7G target miRNAs identified by LASSO in the cancer-free and cancer groups. (D) Principal component analysis between cancer-free and cancer groups based on the 12 candidate m7G target miRNAs. (E) ROC curve showing the performance of each candidate m7G target miRNA individually detecting cancer patients in the training set. The area under curve (AUC) of a single miRNA ranged from 0.742 to 0.955. (F) Radar chart showing the AUC of each candidate m7G target miRNA individually detecting cancer patients in the validation set.
GO and KEGG enrichment analysis were performed to explore the biological processes regulated by m7G target miRNAs. The results underscored the predominant enrichment of these genes in pathways related to cancer and RNA metabolism, including TGF-β receptor signaling pathway, RNA metabolism process and RNA localization (Supplementary Figures S1D–SE; Supplementary Table S5).
Utilizing expression profiles of 33 pan-cancer datasets from The Cancer Genome Atlas (TCGA) project, we observed aberrant expression of METTL1 and WDR4, known as m7G methyltransferase, across multiple cancer types. Specially, the two genes were downregulated in colon adenocarcinoma compared to controls, while upregulated in diffuse large B-cell lymphoma and thymoma. In addition, they demonstrated significant independent prognostic value in cancers such as BLCA, ESCA and OV, as illustrated in Supplementary Figures S1A-C. These findings implied that m7G dysregulation was closely related to tumor occurrence and progression.
In the training set, 22 of 33 m7G target miRNAs were significantly differentially expressed when comparing the cancer group with the cancer-free controls (FDR<0.05 and log2|fold change| > 1). Among these, 21miRNAs exhibited significant upregulation in cancers, and only miR-125a-3p was significantly downregulated (Figure 1B and Supplementary Table S4). Then, we identified a total of 12 candidate m7G target miRNAs using the LASSO (Supplementary Figures S1F,G). Supplementary Table S4 listed 12 m7G target miRNAs and their corresponding coefficients. Based on the expression profiles of the 12 candidate miRNAs, unsupervised hierarchical clustering demonstrated a distinct separation between cancers and cancer-free controls (Figure 1C). This observation was further supported by principal component analysis (Figure 1D). These results underscored significant differences in expression patterns between two groups, providing valuable insights for the development of a diagnostic signature for cancer detection.
The diagnostic performance of each candidate miRNA was assessed individually for cancer detection. Each miRNA exhibited a significant diagnostic capability in distinguishing cancers from cancer-free controls, with AUC values ranging from 0.742 for miR-193a-5p to 0.955 for miR-663a (Figure 1E). The similar finding was also observed in the validation cohort (Figure 1F). Those results suggested that identified candidate m7G target miRNAs hold promise as biomarkers for cancer detection.
Utilizing the 12 identified m7G target miRNAs, we built a diagnostic signature for cancer detection, named m7G-miRDS. The m7G-miRNAs score in cancers was significantly higher than that in cancer-free controls (Figure 2A). The distribution of m7G-miRNA scores in each cancer type showed that BRCA patients had the lowest median m7G-miRNA scores, while BLCA patients had the highest median m7G-miRNA scores (Figure 2B). The m7G-miRDS model, a 12-m7G-miRNAs set, showed a higher diagnostic power than each candidate miRNA alone in distinguishing cancer samples from cancer-free controls in the training cohort, with an AUC and 95% confidence interval (CI) of 0.974 (0.971–0.977) (Figure 2C). Similar to the training cohort, the m7G-miRDS model also showed a high diagnostic performance in the validation cohort, with an AUC of 0.972% and 95% CI, 0.969 to 0.975 (Figure 2D). Additionally, the m7G-miRDS demonstrated a satisfactory diagnostic value in the entire set (Figure 2E).
FIGURE 2. Construction of a serum m7G target miRNA signature for cancer detection. (A) Violin plot showing differences in m7G-miRNAs scores between the cancer-free and cancer groups. (B) Plot displaying differences in m7G-miRNAs scores across different cancer types. (C–E) The ROC curve showing the diagnostic performance of m7G-RDS in the training set (C), validation set (D) and entire set (E). (F) Circle plot depicting the relationship between the m7G-miRNA score and the expression of each miRNA using Spearman statistical analysis. Green represents a negative correlation, and red represents a positive correlation. (G,H) The decision curve showing differences in decision threshold probability between m7G-RDS and other serum biomarkers in the training set (G) and validation set (H).
By Spearman correlation analysis, we observed a remarkable positive correlation between m7G-miRNAs and 12 miRNAs, except hsa-miR-125a-3p (Figure 2F and Supplementary Table S6). Previously published studies reported the important value of hsa-miR-93 and hsa-miR-122 in the diagnosis and prognosis of various cancer types (Luna et al., 2017; Gao et al., 2019; Faramin Lashkarian et al., 2023). In decision curve analyses, m7G-miRNAs demonstrated an absolute superiority net benefit within a wide range of decision-making threshold probabilities compared to hsa-miR-93 and hsa-miR-122 neither in training set not validation set (Figures 2G,H).
Considering the effect of patient clinicopathological characteristics on diagnostic efficacy, such as gender, age and pathological stage, we tested the diagnostic performance of m7G-miRDS stratified by patient gender. No significant difference in m7G-miRNA scores was observed between female and male patients (p = 0.45, Figure 3A). Similar to gender, we also did not observe a significant correlation between m7G-miRNA scores and patient age (R = 0.07, Figure 3B). According to the subgroups classified by tumor stage, there was no significant difference in the m7G-miRNA scores between different pathological stages, except for stage I and stage III (Figure 3C). In conclusion, m7G-miRDS was not interfered by the gender and age of patients, underscoring its robustness as a biomarker for distinguishing cancers from controls.
FIGURE 3. Diagnostic performance of m7G-RDS in different clinical features and cancer types. (A) Differences in m7G-miRNAs scores between female and male individuals. (B) Correlation between m7G-miRNAs score and age through Spearman analysis. (C) Violin plot illustrating differences in m7G-miRNAs scores among different stages. (D) The radar chart showing the diagnostic ability of m7G-RDS in each cancer type of the training set (purple polyline) and the validation set (green polyline). (E) The ROC curve showing the diagnostic value of m7G-RDS in early gastric cancer. (F) Plot of differences in m7G-RDS between patients with hepatocellular carcinoma (HCC) and patients with liver cirrhosis or chronic hepatitis. (G) ROC curve showing the ability of m7G-RDS to distinguish HCC patients from liver cirrhosis/chronic hepatitis patients. (H) Principal component analysis for the 12 candidate m7G target miRNAs in HCC and liver cirrhosis/chronic hepatitis patients.
To evaluate the discriminative ability of m7G-miRNAs across various cancer types, we mixed each cancer type separately with cancer-free controls. As expected, m7G-miRDS showed superior discriminatory capability, with the highest AUC and 95% CI for bladder cancer in the training and validation cohort (0.982, 0.979–0.986 and 0.981, 0.978–0.985, respectively), and the lowest AUC and 95% CI for breast cancer in both sets (0.954, 0.946–0.963 and 0.955, 0.947–0.963, respectively) (Figure 3D and Supplementary Table S7). Despite a slight diminish in diagnostic ability of m7G-miRDS for individual cancer type, it maintained very high sensitivity. The results suggested that m7G-miRDS could identify over 92% of patients with a given cancer type, resulting in a lower incidence of missed diagnosis.
Among ten datasets, the dataset GSE164174 included samples derived from early gastric cancer, and the training and validation cohort consisted of 698 cancer-free controls and 711 samples from patients with early gastric cancer, with 705 stage I and 5 stage II samples. In Figure 3E, the m7G-miRDS demonstrated promising performance in diagnosing early gastric cancer, with an AUC of 0.973 (95% CI, 0.970–0.975), a specificity of 0.910, a sensitivity of 0.984 and an accuracy of 0.947.
In addition, the dataset GSE113740 included 46 chronic hepatitis and 93 liver cirrhosis serum samples, thus we also investigated the capability of m7G-miRDS in distinguishing between cancer and non-tumor diseases. The model exhibited a remarkable accuracy in discriminating HCC individuals from patients with chronic hepatitis or liver cirrhosis (Figures 3F–H), with an AUC of 0.846 (95% CI: 0.805–0.888), surpassing the performance of traditional biomarkers, such as alpha-fetoprotein (AUC, 0.65; specificity, 51.4%; sensitivity, 73.3%) (Yamamoto et al., 2020). The above results indicated that chronic disorders might not significantly affect the diagnostic performance of m7G-miRDS.
Growing evidence indicates a critical role of m7G in the development of human disease, especially cancer (Wang et al., 2023; Xia et al., 2023). The m7G modification can occur in miRNAs, and aberrant m7G levels are closely associated with tumorigenesis and progression via regulation of the expression of downstream targets, such as multiple oncogenes and tumor suppressor genes (Ma et al., 2021a; Luo et al., 2022). miRNAs derived from blood have recognized as valuable biomarker for the diagnosis and prognosis of cancer, owing to their noninvasive and cost-effective nature (Bagnoli et al., 2016; Hussen et al., 2021). However, utilizing a specific set of m7G target miRNAs as a diagnostic signature and its relationship with cancer detection largely unexplored. Here, utilizing a total of 20,702 serum samples across 12 distinct cancer types, we identified a novel 12-m7G-target-miRNA biosignature for cancer detection. The m7G-miRDS demonstrated effective discrimination between cancers from cancer-free controls, independent of patient gender and age.
There have been studies focusing on the development and validation of signatures for cancer prognosis or diagnosis by utilizing m7G regulators (e.g., m7G methyltransferase) or RNA modification related miRNAs (e.g., m6A target miRNAs), demonstrating high specificity and sensitivity (Zhang et al., 2021; Hong et al., 2022; Li et al., 2022; Ying et al., 2023). Early detection of cancer leads to better treatment and increased survival rates (Crosby et al., 2022). However, existing studies has not extensively explored the relationship between m7G and cancer detection, especially concerning the diagnostic performance of m7G target miRNAs in serum. In this study, a set of 12 target miRNAs, namely, miR-320e, let-7a-5p, miR-320a, miR-320d, miR-320b, miR-320c, miR-92b-3p, miR-23b-5p, miR-296-3p, miR-663a, miR-193a-5p, and miR-125a-3p, was identified as a diagnostic signature to discriminate cancer from cancer-free controls. Among these 12 m7G-miRNAs, four belong to the miR-320 family, known for anti-oncogene target miRNA for cancer therapy (Liang et al., 2021). Notably, miR-320b and miR-320d were identified as prediction biomarkers for platinum resistance in ovarian cancer patients (Liu et al., 2022). Aberrant expression of miR-320e, let-7a-5p and miR-92b-3p have been acknowledged as a prognostic biomarker in colorectal cancer (Perez-Carbonell et al., 2015; Pichler et al., 2017; Bustos et al., 2021). Additionally, we discovered that miR-320b and miR-320e, which can be modified by m6A and m7G, were identified as diagnostic signatures for cancer detection in both Zhang et al., 2021 study and our research. Those findings enhance our understanding of the molecular intricacies involving miRNAs as well as their RNA modifications, presenting a new avenue for cancer detection via using distinct specific biosignatures, especially modified non-coding RNAs.
However, our study has a number of drawbacks: 1) Due to the lack of corresponding stage information except gastric cancer, the performance of m7G-miRDS in diagnosing other cancers with early stages still needs to be further investigated. 2) The function of several m7G target miRNAs still requires further validation through low-throughput experiments. 3) There aren't enough m7G-RIP-seq data from the serum of cancer patients, and more m7G target miRNAs could be candidates for the development of a signature. Together, these results suggest a diverse potential of m7A target miRNAs in cancer detection and the creation of cutting-edge therapeutic approaches.
A 12 m7G-miRNAs biosignature to diagnose cancer was identified. Our results suggest that the importance of serum circulating m7G target miRNAs in cancer, and further large prospective cohort studies are warranted to validate our findings.
All serum miRNA expression profiles with corresponding clinical information were available in GEO (https://www.ncbi.nlm.nih.gov/geo/), and the related accession number was provided in the supplementary materials.
Ethical review and approval was not required for the study on human participants in accordance with the local legislation and institutional requirements. Written informed consent from the patients/ participants OR patients/participants legal guardian/next of kin was not required to participate in this study in accordance with the national legislation and the institutional requirements.
DL: conceptualization, supervision, funding acquisition, writing-review and editing. WL: conceptualization, supervision, funding acquisition, writing-review and editing. YC: conceptualization, supervision, funding acquisition, project administration, investigation, methodology, formal analysis, validation, writing-original draft, writing-review and editing. YX: project administration, investigation, methodology, formal analysis, validation, writing-original draft and writing-review. LB: validation, methodology and data curation, writing-original draft. HC: data curation, visualization, writing-review and editing.
The author(s) declare financial support was received for the research, authorship, and/or publication of this article. This study was supported by National Natural Science Foundation of China (92159302 to WL; 82173182 to DL), Post-Doctor Research Project, West China Hospital, Sichuan University (2021HXBH051 to YC), the Fundamental Research Funds for the Central Universities (2022SCU12047 to YC) and the Sichuan Science and Technology Support Project (2022NSFSC1516 to YC).
The authors declare that the research was conducted in the absence of any commercial or financial relationships that could be construed as a potential conflict of interest.
All claims expressed in this article are solely those of the authors and do not necessarily represent those of their affiliated organizations, or those of the publisher, the editors and the reviewers. Any product that may be evaluated in this article, or claim that may be made by its manufacturer, is not guaranteed or endorsed by the publisher.
The Supplementary Material for this article can be found online at: https://www.frontiersin.org/articles/10.3389/fgene.2024.1270302/full#supplementary-material
SUPPLEMENTARY FIGURE S1 | The expression level and survival analysis of m7G methyltransferase and the function annotation of m7G target miRNAs. (A) Heatmap illustrating the prognosis implications of METTL1 and WDR4 genes across 33 cancer types. (B,C). Differential expression analysis of the METTL1 (B) and WDR4 (C) genes in TCGA pan-cancer cohort. (D,E) Barplot displaying functional annotation of the included 33 m7G target miRNAs using gene ontology (GO) (D) and KEGG pathway enrichment analysis (E). (F) The tuning parameters (log(lambda)) of m7G target miRNAs were selected to cross-verify the error curve. According to the minimal criterion and 1-se criterion, perpendicular imaginary lines were drawn at the optimal value. (G) The LASSO coefficient profile of 12 candidate m7G target miRNAs and perpendicular imaginary lines were drawn at the value chosen by 100-fold cross-validation.
SUPPLEMENTARY TABLE S1 | Ten GEO datasets used in this study and corresponding sample sizes and clinical features of each dataset.
SUPPLEMENTARY TABLE S2 | A list of m7G-harboring miRNAs derived from previously published studies. Functional annotation for 33 m7G target miRNAs. A list of. List of 12 m7G target miRNA obtained by using the LASSO method.
SUPPLEMENTARY TABLE S3 | Details on samples in the training set and validation set.
SUPPLEMENTARY TABLE S4 | A list of significantly differentially expressed m7G target miRNAs (FDR < 0.05 and |log2(FC)| > 1) and 12 candidate m7G-miRNAs.
SUPPLEMENTARY TABLE S5 | Functional annotation for 33 m7G target miRNAs.
SUPPLEMENTARY TABLE S6 | Spearman correlation analysis between m7G-miRNAs and 12 miRNAs.
SUPPLEMENTARY TABLE S7 | Summary of AUC, sensitivity, specificity and accuracy of m7G-miRDS for each cancer type in the training and validation cohorts. AUC: area under the curve.
Alexandrov, A., Martzen, M. R., and Phizicky, E. M. (2002). Two proteins that form a complex are required for 7-methylguanosine modification of yeast trna. Rna 8 (10), 1253–1266. doi:10.1017/s1355838202024019
Bagnoli, M., Canevari, S., Califano, D., Losito, S., Maio, M. D., Raspagliesi, F., et al. (2016). Development and validation of a microrna-based signature (mirovar) to predict early relapse or progression of epithelial ovarian cancer: a cohort study. Lancet Oncol. 17 (8), 1137–1146. doi:10.1016/S1470-2045(16)30108-5
Bray, F., Ferlay, J., Soerjomataram, I., Siegel, R. L., Torre, L. A., and Jemal, A. (2018). Global cancer statistics 2018: globocan estimates of incidence and mortality worldwide for 36 cancers in 185 countries. CA Cancer J. Clin. 68 (6), 394–424. doi:10.3322/caac.21492
Bustos, M., Gross, R., Dejenie, R., Suyeon, R., Rahimzadeh, N., Tran, L., et al. (2021). Diagnostic ability of four cell-free mirna signatures pre- and post-nephrectomy concordantly found in the tumor and blood from patients with renal cell carcinoma. J. Clin. Oncol. 39 (15), e16577. doi:10.1200/JCO.2021.39.15_suppl.e16577
Chen, Y., Lin, H., Miao, L., and He, J. (2022). Role of N7-methylguanosine (M(7)G) in cancer. Trends Cell Biol. 32 (10), 819–824. doi:10.1016/j.tcb.2022.07.001
Chen, Z., and Liu, J. (2021). Microrna-Let-7 targets Hmga2 to regulate the proliferation, migration, and invasion of colon cancer cell Hct116. Evid. Based Complement. Altern. Med. 2021, 2134942. doi:10.1155/2021/2134942
Crosby, D., Bhatia, S., Brindle, K. M., Coussens, L. M., Dive, C., Emberton, M., et al. (2022). Early detection of cancer. Science 375 (6586), eaay9040. doi:10.1126/science.aay9040
Faramin Lashkarian, M., Hashemipour, N., Niaraki, N., Soghala, S., Moradi, A., Sarhangi, S., et al. (2023). Microrna-122 in human cancers: from mechanistic to clinical perspectives. Cancer Cell Int. 23 (1), 29. doi:10.1186/s12935-023-02868-z
Gao, Y., Deng, K., Liu, X., Dai, M., Chen, X., Chen, J., et al. (2019). Molecular mechanism and role of microrna-93 in human cancers: a study based on bioinformatics analysis, meta-analysis, and quantitative polymerase chain reaction validation. J. Cell Biochem. 120 (4), 6370–6383. doi:10.1002/jcb.27924
Haag, S., Kretschmer, J., and Bohnsack, M. T. (2015). Wbscr22/Merm1 is required for late nuclear pre-ribosomal rna processing and mediates N7-methylation of G1639 in human 18s rrna. RNA 21 (2), 180–187. doi:10.1261/rna.047910.114
Hong, P., Du, H., Tong, M., Cao, Q., Hu, D., Ma, J., et al. (2022). A novel m7g-related micrornas risk signature predicts the prognosis and tumor microenvironment of kidney renal clear cell carcinoma. Front. Genet. 13, 922358. doi:10.3389/fgene.2022.922358
Hussen, B. M., Hidayat, H. J., Salihi, A., Sabir, D. K., Taheri, M., and Ghafouri-Fard, S. (2021). Microrna: a signature for cancer progression. Biomed. Pharmacother. 138, 111528. doi:10.1016/j.biopha.2021.111528
Leek, J. T., Johnson, W. E., Parker, H. S., Jaffe, A. E., and Storey, J. D. (2012). The sva package for removing batch effects and other unwanted variation in high-throughput experiments. Bioinformatics 28 (6), 882–883. doi:10.1093/bioinformatics/bts034
Li, X. Y., Zhao, Z. J., Wang, J. B., Shao, Y. H., Hui, L., You, J. X., et al. (2022). M7g methylation-related genes as biomarkers for predicting overall survival outcomes for hepatocellular carcinoma. Front. Bioeng. Biotechnol. 10, 849756. doi:10.3389/fbioe.2022.849756
Liang, Y., Li, S., and Tang, L. (2021). Microrna 320, an anti-oncogene target mirna for cancer therapy. Biomedicines 9 (6), 591. doi:10.3390/biomedicines9060591
Liu, Y., Yang, C., Zhao, Y., Chi, Q., Wang, Z., and Sun, B. (2019b). Overexpressed methyltransferase-like 1 (Mettl1) increased chemosensitivity of colon cancer cells to cisplatin by regulating mir-149-3p/S100a4/P53 Axis. Aging (Albany NY) 11 (24), 12328–12344. doi:10.18632/aging.102575
Liu, Y., Zhao, Y., Chi, Q., Wang, Z., and Sun, B. (2019a). Overexpressed MethyltransFerase-like 1 (Mettl1) increased chemosensitivity of colon cancer cells to cisplatin by regulating mir-149-3p/S100a4/P53 Axis. Aging (Albany NY) 11 (24), 17. doi:10.18632/aging.102575
Liu, Y. Y., Zhao, R. F., Liu, C., Zhou, J., Yang, L., and Li, L. (2022). Mir-320b and mir-320d as biomarkers to predict and participate in the formation of platinum resistance in ovarian cancer patients. Front. Oncol. 12, 881496. doi:10.3389/fonc.2022.881496
Luna, J. M., Barajas, J. M., Teng, K. Y., Sun, H. L., Moore, M. J., Rice, C. M., et al. (2017). Argonaute clip defines a deregulated mir-122-bound transcriptome that correlates with patient survival in human liver cancer. Mol. Cell 67 (3), 400–410. doi:10.1016/j.molcel.2017.06.025
Luo, X., Li, H., Liang, J., Zhao, Q., Xie, Y., Ren, J., et al. (2021). Rmvar: an updated database of functional variants involved in rna modifications. Nucleic Acids Res. 49 (D1), D1405–D1412. doi:10.1093/nar/gkaa811
Luo, Y., Yao, Y., Wu, P., Zi, X., Sun, N., and He, J. (2022). The potential role of N(7)-methylguanosine (M7g) in cancer. J. Hematol. Oncol. 15 (1), 63. doi:10.1186/s13045-022-01285-5
Ma, J., Han, H., Huang, Y., Yang, C., Zheng, S., Cai, T., et al. (2021b). Mettl1/Wdr4-Mediated M(7)G trna modifications and M(7)G codon usage promote mrna translation and lung cancer progression. Mol. Ther. 29 (12), 3422–3435. doi:10.1016/j.ymthe.2021.08.005
Ma, J., Zhang, L., Chen, J., Song, B., Zang, C., and Liu, H. (2021a). M(7)Gdisai: N7-methylguanosine (M(7)G) sites and diseases associations inference based on heterogeneous network. BMC Bioinforma. 22 (1), 152. doi:10.1186/s12859-021-04007-9
Matsuzaki, J., Yamamoto, Y., Yi, O., Ayyar, S., Miyajima, R., Takeda, Y., et al. (2021). Machine learning-based multiple cancer detections with circulating mirna profiles in the blood. J. Clin. Oncol. 39 (15), 3037. doi:10.1200/JCO.2021.39.15_suppl.3037
Pandolfini, L., Barbieri, I., Bannister, A. J., Hendrick, A., Andrews, B., Webster, N., et al. (2019). Mettl1 promotes let-7 microrna processing via M7g methylation. Mol. Cell 74 (6), 1278–1290. doi:10.1016/j.molcel.2019.03.040
Perez-Carbonell, L., Sinicrope, F. A., Alberts, S. R., Oberg, A. L., Balaguer, F., Castells, A., et al. (2015). Mir-320e Is a Novel Prognostic Biomarker in Colorectal Cancer. Br. J. Cancer 113 (1), 83–90. doi:10.1038/bjc.2015.168
Pichler, M., Stiegelbauer, V., Vychytilova-Faltejskova, P., Ivan, C., Ling, H., Winter, E., et al. (2017). Genome-wide mirna analysis identifies mir-188-3p as a novel prognostic marker and molecular factor involved in colorectal carcinogenesis. Clin. Cancer Res. 23 (5), 1323–1333. doi:10.1158/1078-0432.CCR-16-0497
So, J. B. Y., Kapoor, R., Zhu, F., Koh, C., Zhou, L., Zou, R., et al. (2021). Development and validation of a serum microrna biomarker panel for detecting gastric cancer in a high-risk population. Gut 70 (5), 829–837. doi:10.1136/gutjnl-2020-322065
Sticht, C., De La Torre, C., Parveen, A., and Gretz, N. (2018). Mirwalk: an online resource for prediction of microrna binding sites. PLoS One 13 (10), e0206239. doi:10.1371/journal.pone.0206239
Trotman, J. B., Giltmier, A. J., Mukherjee, C., and Schoenberg, D. R. (2017). Rna guanine-7 methyltransferase catalyzes the methylation of cytoplasmically recapped rnas. Nucleic Acids Res. 45 (18), 10726–10739. doi:10.1093/nar/gkx801
Wang, X., Zhang, Y., Chen, K., Liang, Z., Ma, J., Xia, R., et al. (2023). M7ghub V2.0: an updated database for decoding the N7-methylguanosine (M7g) epitranscriptome. Nucleic Acids Res. 52, D203–D212. doi:10.1093/nar/gkad789
Xia, X., Wang, Y., and Zheng, J. C. (2023). Internal M7g methylation: a novel epitranscriptomic contributor in brain development and diseases. Mol. Ther. Nucleic Acids 31, 295–308. doi:10.1016/j.omtn.2023.01.003
Yamamoto, Y., Kondo, S., Matsuzaki, J., Esaki, M., Okusaka, T., Shimada, K., et al. (2020). Highly sensitive circulating microrna panel for accurate detection of hepatocellular carcinoma in patients with liver disease. Hepatol. Commun. 4 (2), 284–297. doi:10.1002/hep4.1451
Yang, Y., Qu, A., Zhao, R., Hua, M., Zhang, X., Dong, Z., et al. (2018). Genome-wide identification of a novel mirna-based signature to predict recurrence in patients with gastric cancer. Mol. Oncol. 12 (12), 2072–2084. doi:10.1002/1878-0261.12385
Ying, X., Liu, B., Yuan, Z., Huang, Y., Chen, C., Jiang, X., et al. (2021). Mettl1-M(7) G-egfr/efemp1 Axis promotes the bladder cancer development. Clin. Transl. Med. 11 (12), e675. doi:10.1002/ctm2.675
Ying, Y., Zhang, W., Zhu, H., Luo, J., Xu, X., Yang, S., et al. (2023). A novel M7g regulator-based methylation patterns in head and neck squamous cell carcinoma. Mol. Carcinog. 62 (12), 1902–1917. doi:10.1002/mc.23624
Yu, G., Wang, L. G., Han, Y., and He, Q. Y. (2012). Clusterprofiler: an R package for comparing biological themes among gene clusters. OMICS 16 (5), 284–287. doi:10.1089/omi.2011.0118
Zhang, B., Chen, Z., Tao, B., Yi, C., Lin, Z., Li, Y., et al. (2021). M(6)a target micrornas in serum for cancer detection. Mol. Cancer 20 (1), 170. doi:10.1186/s12943-021-01477-6
Keywords: m7G, microRNA, liquid biopsy, cancer diagnosis, pan-cancer
Citation: Chen Y, Xie Y, Bi L, Ci H, Li W and Liu D (2024) A novel serum m7G-harboring microRNA signature for cancer detection. Front. Genet. 15:1270302. doi: 10.3389/fgene.2024.1270302
Received: 06 August 2023; Accepted: 22 January 2024;
Published: 07 February 2024.
Edited by:
Elisa Frullanti, University of Siena, ItalyReviewed by:
Bowen Song, Nanjing University of Chinese Medicine, ChinaCopyright © 2024 Chen, Xie, Bi, Ci, Li and Liu. This is an open-access article distributed under the terms of the Creative Commons Attribution License (CC BY). The use, distribution or reproduction in other forums is permitted, provided the original author(s) and the copyright owner(s) are credited and that the original publication in this journal is cited, in accordance with accepted academic practice. No use, distribution or reproduction is permitted which does not comply with these terms.
*Correspondence: Weimin Li, d2VpbWkwMDNAc2N1LmVkdS5jbg==; Dan Liu, TGl1ZGFuMTA5NjVAd2Noc2N1LmNu
†These authors have contributed equally to this work
Disclaimer: All claims expressed in this article are solely those of the authors and do not necessarily represent those of their affiliated organizations, or those of the publisher, the editors and the reviewers. Any product that may be evaluated in this article or claim that may be made by its manufacturer is not guaranteed or endorsed by the publisher.
Research integrity at Frontiers
Learn more about the work of our research integrity team to safeguard the quality of each article we publish.