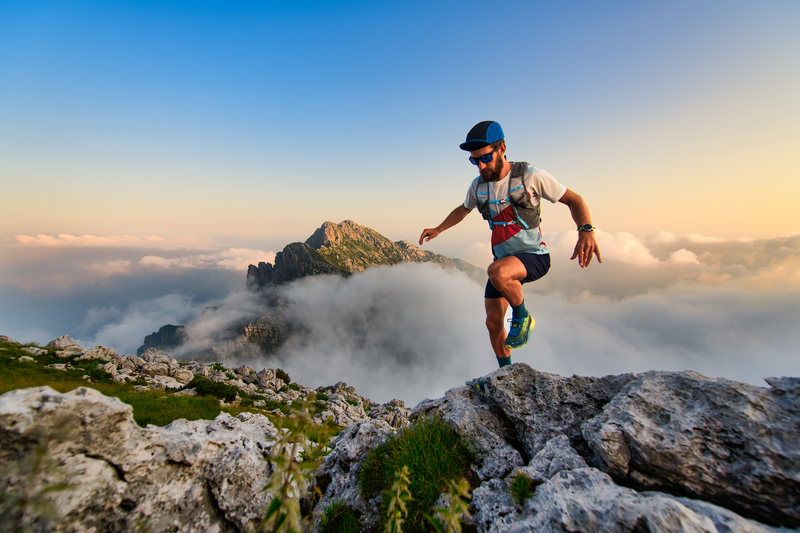
94% of researchers rate our articles as excellent or good
Learn more about the work of our research integrity team to safeguard the quality of each article we publish.
Find out more
ORIGINAL RESEARCH article
Front. Genet. , 23 November 2023
Sec. Statistical Genetics and Methodology
Volume 14 - 2023 | https://doi.org/10.3389/fgene.2023.1309069
This article is part of the Research Topic Advances in Dissection of Biomolecule Variation and their Effects on Complex Diseases based on High-throughput Omics Data View all 3 articles
To explore the correlation and causality between multidimensional sleep traits and pan-cancer incidence and mortality among patients with cancer. The multivariable Cox regression, linear and nonlinear Mendelian randomization (MR), and survival curve analyses were conducted to assess the impacts of chronotype, sleep duration, and insomnia symptoms on pan-cancer risk (N = 326,417 from United Kingdom Biobank) and mortality (N = 23,956 from United Kingdom Biobank). In the Cox regression, we observed a linear and J-shaped association of sleep duration with pan-cancer incidence and mortality among cancer patients respectively. In addition, there was a positive association of insomnia with pan-cancer incidence (HR, 1.03, 95% CI: 1.00–1.06, p = 0.035), all-cause mortality (HR, 1.17, 95% CI: 1.06–1.30, p = 0.002) and cancer mortality among cancer patients (HR, 1.25, 95% CI: 1.11–1.41, p < 0.001). In the linear MR, there was supporting evidence of positive associations between long sleep duration and pan-cancer incidence (OR, 1.41, 95% CI: 1.08–1.84, p = 0.012), and there was a positive association between long sleep duration and all-cause mortality in cancer patients (OR, 5.56, 95% CI: 3.15–9.82, p = 3.42E-09). Meanwhile, a strong association between insomnia and all-cause mortality in cancer patients (OR, 1.41, 95% CI: 1.27–1.56, p = 4.96E-11) was observed in the linear MR. These results suggest that long sleep duration and insomnia play important roles in pan-cancer risk and mortality among cancer patients. In addition to short sleep duration and insomnia, our findings highlight the effect of long sleep duration in cancer prevention and prognosis.
In 2019, the World Health Organization (WHO) reported that cancer had become the first or second leading cause of death in more than 100 countries globally (Sung et al., 2021). Unhealthy lifestyle behaviors are associated with an increased risk of cancer. Emerging evidence also suggests that poor sleep is associated with the risk of cancer (Xiao et al., 2017; Wang J. et al., 2021), and poor sleep quality is persistent in cancer patients and survivors (Davidson et al., 2002; Irwin et al., 2013; Garland et al., 2014; Yoon et al., 2015). Several studies have shown that short and long sleep durations are associated with a higher risk of several cancers (Richmond et al., 2019; Wang J. et al., 2021; Tao et al., 2021; Xie et al., 2021; Lv et al., 2022; Peeri et al., 2022). Although several studies have explored the relationship between sleep duration and cancer mortality, inconsistent results have been found in observational studies (Chien et al., 2010; Phipps et al., 2016; Marinac et al., 2017; Trudel-Fitzgerald et al., 2017; Wong et al., 2017; Khan et al., 2018; Svensson et al., 2021; Tao et al., 2021). Some studies showed a U-shaped relationship (Wong et al., 2017), while others only found a relationship for long sleep duration (Marinac et al., 2017; Trudel-Fitzgerald et al., 2017) or no relationship (Phipps et al., 2016). In addition, no study has explored sleep traits and 5-year cancer mortality in cancer survivors, which is an important survival indicator among cancer patients. Because of self-reporting and residual confounding effects in observational studies, causal relationships are still unclear.
Mendelian randomization (MR) analysis provides a method to help clarify causal association by utilizing genetic variants of sleep traits as instrumental variables to improve inference in observational studies (Smith and Ebrahim, 2003; Davey Smith and Hemani, 2014; Davies et al., 2018; Sanderson, 2021). MR could overcome some limitations of traditional observational studies, because genetic variants are typically not associated with confounders. Thus differences between those who do not carry the variant and those who do can be attributed to the difference in the risk factor (Emdin et al., 2017). To date, several genome-wide association studies (GWAS) have found genetic variants that are robustly associated with sleep traits, including chronotype, sleep duration and insomnia symptoms (Dashti et al., 2019; Jansen et al., 2019; Jones et al., 2019). Recent studies have explored the causal associations between sleep duration and several cancers (Richmond et al., 2019; Wang J. et al., 2021; Hayes et al., 2022). However, sleep health is a multidimensional issue; several sleep traits are associated with disease risk and should be considered together. It is not convincing to consider only the causal association between one factor of sleep traits (e.g., chronotype, sleep duration, or insomnia symptoms) and the risk and mortality of cancer. In addition, we could accurately clarify the effects of sleep traits on cancer mortality only if we focused on cancer patients. Thus, there is a need to explore the potential causal associations between sleep traits and pan-cancer incidence and mortality among patients with cancer.
In the present study, we aimed to systematically explore the correlation and causality between multidimensional sleep traits and pan-cancer incidence and mortality among patients with cancer. We first used multivariable Cox proportional hazard models to estimate the associations between sleep traits and pan-cancer incidence. Furthermore, we investigated whether there was a causal relationship using linear and nonlinear MR analysis. We also analyzed the associations and causal relationship between sleep straits and mortality among cancer patients, including all-cause mortality of cancer patients, 5-year cancer mortality and cancer mortality, using multivariable Cox proportional hazard models and MR analyses. Finally, Kaplan‒Meier estimation was used to compare survival analyses across different groups of cancer patients.
The United Kingdom Biobank is a prospective cohort of more than 500,000 adults aged 37–63 years, recruited from 22 centers across the United Kingdom between 2006 and 2010 (Fry et al., 2017). We extracted all information needed for this study from the United Kingdom Biobank (Fry et al., 2017). Considering the confounding factor of ancestry, we analyzed only unrelated participants of European ancestry. Overall, 326,417 participants were included in pan-cancer incidence analysis. The detailed study protocol is shown in Figure 1. Among those participants, the average follow-up duration was 11.28 ± 2.64 years, in which 283,516 participants did not develop cancer and 42,901 participants developed cancer. In our study, 23,956 cancer patients were included in the mortality analysis. The detailed study protocol is shown in Supplementary Table S8. Among those with cancer, the average follow-up duration was 11.63 ± 2.70 years, in which 3,687 cancer patients were died (2,864 with cancer deaths and 823 with noncancer deaths). Descriptions and sources of the selected covariates, genotyping process and sample quality control in the United Kingdom Biobank are shown in Supplementary Table S1.
The chronotype, sleep duration, and insomnia symptoms were assessed. Chronotype was assessed using the standardized question “Do you consider yourself to be?” with one of the six possible answers: “Definitely a ‘morning’ person,” “More a ‘morning’ than ‘evening’ person,” “More an ‘evening’ than ‘morning’ person,” “Definitely an ‘evening’ person,” “Do not know,” or “Prefer not to answer.” In the present study, we classified “Definitely a ‘morning’ person,” “More a ‘morning’ than ‘evening’ person,” “More an ‘evening’ than ‘morning’ person,” “Definitely an ‘evening’ person,” as definite morning, more morning, more evening and definite evening, respectively, and “Do not know” and “Prefer not to answer” responses were considered as missing data. Sleep duration was assessed by asking: “About how many hours of sleep do you get every 24 h (Please include naps).” The answer can contain only integer values. “Do not know” and “Prefer not to answer” responses were considered missing data. Binary variables for ≤5h, 6h, 7h, 8h, and ≥9 h were also derived. Insomnia symptoms were assessed using a single item: “Do you have trouble falling asleep at night or do you wake up in the middle of the night?” with answers: “Never/rarely,” “Sometimes,” “Usually,” or “Prefer not to answer.” Participants who chose “Prefer not to answer” were also considered missing data. Binary variables for insomnia symptoms were generated as “Never/rarely”, “Sometimes”, and “Usually”.
In this study, pan-cancer was defined as malignant neoplasms according to the International Classification of Diseases edition 10 (ICD-10), excluding in situ (ICD code: D00-D09), benign (ICD code: D10-D36), uncertain or non-well-defined cancers (ICD code: D37-D48), which is the same approach as that used by other studies (Huang et al., 2022; Zhou et al., 2022; Zhu et al., 2022). Thus, our study focused on malignant tumors (ICD code: C00-C97). A record of cancer diagnosis was needed, and the endpoints were the first diagnosis of these cancers using the ICD-10 codes. In the pan-cancer risk analysis, we excluded all participants with any cancer diagnosis records at baseline, and participants were excluded if they had missing data on covariates. The incident cases were defined as participants who were diagnosed with cancer during the follow-up period. Cancer case records were available through the National Health Service central and death registries. In the mortality analysis, we included participants with a cancer diagnosis (ICD code: C00-C97) at baseline and cancer patients were excluded if they had missing data on covariates. The primary and contributory causes of death were defined based on the ICD-10. All-cause mortality of cancer patients, 5-year cancer mortality, and cancer mortality were defined as the percentage of deaths caused by all-causes, cancer within 5 years (cancer patients with a diagnosis within 2 years prior to enrollment were included, and noncancer deaths were excluded) and cancer within follow-up years (excluding noncancer deaths) among cancer patients, respectively. Participants were followed up from their date of enrollment until the date of diagnosis of malignant tumors, date of withdrawal from the study, date of death, or until the end of follow-up, whichever came first. The definitions and sources of information for outcomes in the United Kingdom Biobank of cancer are shown in Supplementary Table S2.
Multivariable Cox proportional hazard models were used to investigate the prospective associations of sleep traits with the pan-cancer incidence and mortality among patients with cancer. To minimize the interference of confounding factors, we adjusted for age, sex, assessment center, and the top 10 genetic principal components in the basic model of analyzing pan-cancer incidence and mortality. Additionally, we further adjusted for body mass index, employment status, Townsend deprivation index, smoking status, drinking status and mental health issues, vegetables and fruits intake, sedentary behavior, comorbidity, total physical activity, education, ethnicity and family history in the further adjusted model of analyzing pan-cancer incidence. Similarly, we further adjusted for body mass index, employment status, Townsend deprivation index, smoking status, drinking status, mental health issues, vegetables and fruit intake, sedentary behavior, comorbidity, total physical activity, education, ethnicity, family history, number of self-reported cancers, operation and treatments in a further adjusted model of analyzing mortality. To avoid interference from other sleep traits, we also adjusted for chronotype, sleep duration, and insomnia symptoms in the full model of analyzing pan-cancer incidence and mortality.
We used 12 SNPs associated with an evening chronotype (Lane et al., 2016). We also used 27 SNPs, 8 SNPs and 78 SNPs that were associated with short sleep duration, long sleep duration and continuous sleep duration, respectively (Dashti et al., 2019). In addition, 57 SNPs were associated with insomnia symptoms (Lane et al., 2019). Detailed information on the SNPs is provided in Supplementary Tables S3–S7. The unweighted GRS (Genetic risk score) was calculated as a summary of the number of risk alleles (0, 1, and 2) across the genetic variants of different sleep traits for each participant (Wang W. et al., 2021).
For linear MR analysis, the genetic variants used were extracted from the United Kingdom Biobank imputation dataset. We used a two-stage method (Richmond et al., 2019; Ai et al., 2021) to assess the causal associations between genetically predicted sleep traits and outcomes by MR analysis. We first regressed the sleep traits on the GRS and then regressed cancer or death status on the fitted values of sleep traits from the first-stage regression, with adjustment for age, sex, assessment centers, top 10 genetic principal components, and genotyping arrays (basic model) in both stages. To eliminate the potential violation of MR assumptions, we tested the associations between potential confounders and GRSs. Then, we repeated our MR analyses with an adjustment of these included confounders in the fully adjusted model. In addition, to avoid the potential interference of other sleep traits, we also analyzed the causal associations after excluding participants with interferential sleep traits, which had strong associations (p < 0.05/groups) with the GRSs. For example, we found that insomnia symptoms were strongly associated with the GRSs of short sleep duration, and we excluded participants with insomnia symptoms while exploring the causal association between genetically predicted short sleep duration and the outcomes.
We used nonlinear MR to assess the potential nonlinear J- or U-shaped associations between genetically predicted continuous sleep durations and outcomes. We first divided our sample into three strata based on the residual variation in continuous sleep duration regressed on the GRS (Ai et al., 2021). We then calculated piecewise linear MR effects in each stratum and generated localized average causal effects in these strata. The p values from the quadratic test and Cochran’s Q test for nonlinearity are reported (Staley and Burgess, 2017).
Time-to-event clinical outcomes are common in medical research because they offer more information than simply an event. We used survival analysis methods to handle pan-cancer mortality and censored observations during follow-up. Kaplan‒Meier estimation was used to create survival curves, and the log-rank test was used to compare survival across different groups. Survival curves were also conducted from the Cox models across different groups after adjusting for all covariates.
We first performed a sensitivity analysis by removing participants who reported currently working shifts (sometimes, usually, and always) in the multivariable Cox proportional hazard models. Then, participants who had extreme sleep duration (<4 h or >11 h) were removed. We also conducted sensitivity analysis in the multivariable Cox proportional hazard models and survival curve analysis based on age, which only included participants aged >50 years. A quadratic fit test of the results was performed on the association between sleep duration and mortality in cancer patients. To verify the MR assumption that the genetic variants should not be associated with relevant confounders, we investigated associations between allele scores and potential confounders in United Kingdom Biobank. In addition, we used the MR‒Egger, weighted median, and radial MR methods (Bowden et al., 2015; Bowden et al., 2016; Bowden et al., 2018; Ai et al., 2021) to evaluate potential pleiotropy and outliers in the sensitivity analysis. Once outliers were found, they were removed, and the results were reanalyzed. For further sensitivity analysis, we used a Bonferroni-corrected threshold of p < 0.05/groups in the MR analysis. p values were considered between the Bonferroni-corrected threshold and 0.05 as suggestive evidence in the MR analysis. All statistical analyses were performed using R software (version 4.0.2).
A total of 326,417 United Kingdom Biobank participants without cancer diagnosis at baseline were included in the pan-cancer incidence analysis, and the detailed screening process is shown in Figure 1. The baseline characteristics of the sample are presented in Table 1. Participants with a cancer diagnosis during the follow-up period were more likely to be male and older, and half of those with a cancer diagnosis were retired or not in the workforce. They were also likely to be previous or current smokers and to have a family history and more comorbidities. Moreover, they had a higher percentage of long sleep duration and frequent insomnia symptoms.
TABLE 1. Baseline characteristics of people who had and had not developed cancer during the follow-up period in United Kingdom Biobank (n = 326,417).
To analyze the relationship between sleep traits and the mortality of cancer patients, a total of 23,956 United Kingdom Biobank participants with a cancer diagnosis at baseline were included in the study, and the detailed screening process is shown in Supplementary Table S8. Their baseline characteristics are listed in Table 2. Patients with cancer who died during the follow-up period were likely to be male, older and smokers, and they had a relatively higher prevalence of mental health issues and comorbidities. They were also likely to be retired or not in the workforce, and had lower vegetables and fruits intakes, increased sedentary behavior, higher BMI, and more treatments. In addition, they had a higher percentage of definite evening chronotype, unfavorable sleep duration and frequent insomnia symptoms.
TABLE 2. Baseline characteristics of non-deaths and deaths among cancer patients during the follow-up period in United Kingdom Biobank (n = 23,956).
There was no association between definite evening chronotype and pan-cancer incidence after adjusting for demographic information and other sleep traits (HR, 1.03, 95% CI: 0.99–1.07, p = 0.137; Supplementary Table S9). However, we observed a positive association between sleep duration and pan-cancer incidence after adjusting for all the covariates (HR, 0.94, 95% CI: 0.89–0.99, p = 0.016, sleep duration≤5 h; HR, 0.97, 95% CI: 0.94–1.00, p = 0.038, sleep duration = 6 h; HR, 1.03, 95% CI: 1.00–1.05, p = 0.021, sleep duration = 8 h; HR, 1.04, 95% CI: 1.00–1.08, p = 0.031, sleep duration≥9 h; Supplementary Table S9). Thus, there could be a linear association between sleep duration and pan-cancer incidence (Figure 2A). In addition, there was a positive association between frequent insomnia symptoms and pan-cancer incidence in the fully adjusted model (HR, 1.03, 95% CI: 1.00–1.06, p = 0.035; Supplementary Table S9).
FIGURE 2. Association of sleep duration with pan-cancer incidence and mortality among cancer patients across different hours. (A). Pan-cancer incidence (n = 326,417), (B). All-cause mortality of cancer patients (n = 23,956), (C). 5-Year cancer mortality (n = 4,962), and (D). Cancer-caused mortality (n = 23,133). HRs across hours of habitual sleep duration were presented with 7 h serving as a reference group. Error bars are 95% CI. Basic model: adjusted for age, sex, assessment center, top 10 genetic principal components and genotyping array. Further adjusted model: adjusted for age, sex, assessment center, top 10 genetic principal components, genotyping array, body mass index, employment status, Townsend deprivation index, smoking status, drinking status and mental health issues, vegetables and fruit intake, sedentary behavior, comorbidity, total physical activity, education, ethnicity, family history, number of self-reported cancers, operation and treatments. Full adjusted model: adjusted for age, sex, assessment center, top 10 genetic principal components, genotyping array, body mass index, employment status, Townsend deprivation index, smoking status, drinking status and mental health issues, vegetables and fruit intake, sedentary behavior, comorbidity, total physical activity, education, ethnicity, family history, number of self-reported cancers, operation, treatments and other sleep traits. Statistical significance was defined as p < 0.05.
Regarding the relationship between chronotype and mortality (all-cause mortality, 5-year cancer mortality, and cancer mortality) among cancer patients, no association between definite evening chronotype and mortality of cancer patients was found (HR, 0.95, 95% CI: 0.83–1.08, p = 0.450, all-cause mortality, Supplementary Table S10; HR, 0.89, 95% CI: 0.56–1.43, p = 0.638, 5-year cancer mortality, Supplementary Table S11; HR, 0.91, 95% CI: 0.79–1.06, p = 0.241, cancer mortality, Supplementary Table S12). A positive association between shorter sleep duration (6 h) and all-cause mortality (HR, 1.13, 95% CI: 1.02–1.25, p = 0.019, Supplementary Table S10), 5-year cancer mortality (HR, 1.50, 95% CI: 1.06–2.22, p = 0.022, Supplementary Table S11) and cancer mortality (HR, 1.13, 95% CI: 1.01–1.27, p = 0.033, Supplementary Table S12) among cancer patients was observed in this study. Meanwhile, we also found a positive association between long sleep duration (≥9 h) and all-cause mortality (HR, 1.30, 95% CI: 1.16–1.45, p < 0.001, Supplementary Table S10), 5-year cancer mortality (HR, 1.84, 95% CI: 1.25–2.70, p = 0.002, Supplementary Table S11) and cancer mortality (HR, 1.35, 95% CI: 1.18–1.53, p < 0.001, Supplementary Table S12) among cancer patients. This indicated that there was a J-shaped association between sleep duration and mortality among cancer patients (Figures 2B–D). In addition, there was a positive association between frequent insomnia symptoms and all-cause mortality (HR, 1.17, 95% CI: 1.06–1.30, p = 0.002, Supplementary Table S10) and cancer mortality among cancer patients (HR, 1.25, 95% CI: 1.11–1.41, p < 0.001, Supplementary Table S12). However, there was no association between frequent insomnia symptoms and 5-year cancer mortality among cancer patients (HR, 1.14, 95% CI: 0.80–1.63, p = 0.475, Supplementary Table S11).
We used a two-stage least squares method to assess the associations between genetically predicted sleep traits and outcomes produced by the linear MR analyses. We first regressed the exposures on the genetic risk scores (GRSs) of sleep traits (Supplementary Tables S13–16), and then we regressed the outcome on the fitted values of the exposure from the first-stage regression (Supplementary Tables S17, 18). In the first-stage regression, no obvious associations of evening chronotype GRSs with other sleep traits were found (Supplementary Table S13), and we observed that there was no association between definite evening chronotype and pan-cancer incidence in the fully adjusted model of linear MR analysis (OR, 1.09, 95% CI: 0.92–1.30 per category increase, p = 0.306) (Supplementary Table S17 and Figure 3). However, a strong association (p < 0.05/24 groups = 2.08E-03) of short sleep duration GRSs with insomnia symptoms (Supplementary Table S14) was found in the pan-cancer incidence samples in the first-stage regression. To avoid the potential interference of other sleep traits, we excluded participants with insomnia symptoms and then repeated the MR analyses in the pan-cancer incidence samples and found that there was no association between short sleep duration and pan-cancer incidence in the fully adjusted model of linear MR analysis (OR, 0.86, 95% CI: 0.74–1.00 per category increase, p = 0.053) (Supplementary Table S18 and Figure 3). Meanwhile, the strong association (p < 0.05/24 groups = 2.08E-03) of long sleep duration GRSs with evening chronotype (Supplementary Table S16) was also found in the pan-cancer incidence samples in the first-stage regression. Hence, we excluded participants with the evening chronotype and then repeated the MR analyses in the pan-cancer incidence samples (Supplementary Table S18). After excluding participants with the evening chronotype, there was still supporting evidence of positive associations between long sleep duration and pan-cancer incidence (OR, 1.41, 95% CI: 1.08–1.84 per category increase, p = 0.012) (Supplementary Table S18 and Figure 3) in the fully adjusted model of linear MR analysis. In addition, a strong association (p < 0.05/24 groups = 2.08E-03) of insomnia symptom GRSs with short sleep duration (Supplementary Table S16) was found in the pan-cancer incidence samples in the first-stage regression. After excluding participants with short sleep duration, there was also no association between insomnia symptoms and pan-cancer incidence in the fully adjusted model of linear MR analysis (OR, 1.02, 95% CI: 0.98–1.07 per category increase, p = 0.242) (Supplementary Table S18 and Figure 3).
FIGURE 3. Linear-MR estimates for association between sleep traits and pan-cancer incidence and mortality among cancer patients. Odds ratios are per category in evening chronotype (definite morning [incidence, n = 79,023; all-cause mortality, n = 5,914; 5-year mortality, n = 1,235; cancer mortality, n = 5,703], intermediate morning [106,528; 8,047; 1,711; 7,813], intermediate evening [83,534; 6,045; 1,209; 5,811], and definite evening [27,034; 1,813; 358; 1,738]), per category in sleep duration (≤5 h [1,349; 1,099; 216; 1,046], 6 h [9,997; 3,845; 774; 3,714], 7 h [31,159; 7,905; 6,800; 2,170], 8 h [27,403; 6,800; 1,454; 6,565], ≥9 h [6,401; 2,170; 438; 2,056] in short sleep duration MR analysis and ≤5 h [9,161; 1,099; 216; 1,046], 6 h [35,570; 3,845; 774; 3,714], 7 h [73,791; 7,905; 6,800; 2,170], 8 h [54,817; 6,800; 1,454; 6,565], ≥9 h [12,212; 2,170; 438; 2,056] in long sleep duration analysis) and per category in insomnia risk (no [64,963; 4,729; 1,003; 4,566], some [115,628; 10,192; 2,127; 9,868], and frequent [45,165; 6,898; 1,383; 6,631] insomnia symptoms). ■*p < 0.05 and ■**P < bonferroni-corrected threshold of 0.05/groups. Further adjusted model adjusted for age, sex, assessment centers, top 10 genetic principal components, genotyping array and GRS association factors.
Similarly, we tested the associations between potential confounders and the sleep trait GRSs in cancer mortality samples, and no obvious association of sleep trait GRSs with other sleep traits in the cancer mortality samples was found (Supplementary Tables S19–22). Linear MR analyses suggested that there was a positive association between long sleep duration and all-cause mortality in cancer patients (OR, 5.56, 95% CI: 3.15–9.82 per category increase, p = 3.42E-09, Supplementary Table S24 and Figure 3) and a strong association between frequent insomnia symptoms and all-cause mortality in cancer patients (OR, 1.41, 95% CI: 1.27–1.56 per category increase, p = 4.96E-11, Supplementary Table S25 and Figure 3) in the fully adjusted model. We also observed a positive association between long sleep duration and cancer mortality in cancer patients (OR, 4.89, 95% CI: 2.66–9.82 per category increase, p = 3.08E-07, Supplementary Table S24 and Figure 3) and a strong association between frequent insomnia symptoms and cancer mortality in cancer patients (OR, 1.48, 95% CI: 1.32–1.67 per category increase, p = 7.29E-11, Supplementary Table S25 and Figure 3) in the fully adjusted linear MR analysis. However, we found that there was no association between long sleep duration (OR, 11.11, 95% CI: 0.38–321 per category increase, p = 0.161, Supplementary Table S24 and Figure 3), insomnia symptoms (OR, 1.31, 95% CI: 0.96–1.77 per category increase, p = 0.085, Supplementary Table S24 and Figure 3) and 5-year cancer mortality in the fully adjusted model of linear MR analysis. In addition, we found that there was no association between definite evening chronotype (OR, 1.26, 95% CI: 0.71–2.24 per category increase, p = 0.423, all-cause mortality, Supplementary Table S23 and Figure 3; OR, 0.56, 95% CI: 0.07–4.78 per category increase, p = 0.595,5-year cancer mortality, Supplementary Table S23 and Figure 3; OR, 0.85, 95% CI: 0.45–1.61 per category increase, p = 0.610, cancer mortality, Supplementary Table S23 and Figure 3) and the mortality of cancer patients in the fully adjusted model of linear MR analysis. There was also no association between short sleep duration (OR, 0.85, 95% CI: 0.73–1.00 per category increase, p = 0.050, all-cause mortality, Supplementary Table S24 and Figure 3; OR, 0.85, 95% CI: 0.57–1.26 per category increase, p = 0.419, 5-year cancer mortality, Supplementary Table S24 and Figure 3; OR, 0.86, 95% CI: 0.73–1.02 per category increase, p = 0.088, cancer mortality, Supplementary Table S24 and Figure 3) and mortality of cancer patients in the fully adjusted model of the linear MR analysis.
We observed no evidence favoring a nonlinear relationship between genetically continuous sleep duration and the study outcomes (pan-cancer incidence, all-cause mortality in cancer patients, 5-year cancer mortality, and cancer mortality; Supplementary Table S26). To avoid the potential interference of extreme sleep duration (<4 h or >11 h), there was still no statistical evidence for nonlinear associations between genetically predicted continuous sleep durations and the risk of cancer and mortality using the piecewise linear method after excluding participants with extreme sleep duration (Supplementary Table S27). This means that there were no potential nonlinear J- or U-shaped associations between genetically predicted continuous sleep duration and outcomes.
We also conducted an analysis of mortality among cancer patients (all-cause mortality of cancer patients, 5-year cancer mortality, and cancer mortality) associated with sleep traits and found that the highest mortality occurred in the definite evening chronotype, long sleep duration (≥9 h), and insomnia symptom subgroups (Supplementary Table S28). In addition, Figure 4 shows the analysis of the survival probability of sleep traits with p < 0.05 across different groups. The lowest survival probability occurred in the definite evening chronotype, long sleep duration (≥9 h), and insomnia symptoms subgroups in all-cause mortality (Figure 4A), 5-year cancer mortality (Figure 4B) and cancer-cause mortality (Figure 4C) among cancer patients, respectively. We also observed a similar survival curve from the Cox models by adjusting for additional covariates (Supplementary Table S29).
FIGURE 4. The analysis of survival probability of sleep traits on pan-cancer patients in the UKB cohort. Kaplan–Meier plots for the survival probability of pan-cancer patients in different group of sleep traits. (A) all-cause mortality (n = 23,956); (B) 5-Year cancer mortality (n = 4,962); (C). Cancer-cause mortality (n = 23,133) in the UKB cohort. P calculated by the log-rank test, and p < 0.05 for trend across different groups. (a). Chronotype; (b). Sleep duration; (c). Insomnia.
In the sensitivity analyses, we first removed participants who reported currently working shifts (sometimes, usually, and always) and participants who had extreme sleep durations (<4 h or >11 h). Then, we obtained the same results as in the fully adjusted model before the removal (Supplementary Tables S30, S31). We also conducted a sensitivity analysis based on age>50, and the multivariable Cox regression results for pan-cancer incidence and mortality associated with sleep traits were similar (Supplementary Table S32). Considering the underlying impact of age on survival probability, we conducted a stratified analysis and obtained similar results in the survival probability of sleep traits in cancer patients (age>50 years) (Supplementary Table S33, S34). After adjusting for potential confounders and other sleep traits that were potentially associated with GRSs (Supplementary Tables S13–S16 and Supplementary Tables S19–S22), the results were generally consistent with the basic model in the linear MR analysis (Supplementary Tables S17, S18 and Supplementary Tables S23–S25). Furthermore, we excluded those with interferential sleep traits that had strong associations with GRSs (p < 0.05/24 groups = 2.08E-03) and then repeated the linear and nonlinear MR analyses (Supplementary Tables S18–S27). In addition, we used a Bonferroni-corrected threshold in our MR analysis to indicate a strong association. We also performed the test for a quadratic fit of the results on the association between sleep duration and mortality of cancer patients (Figures 2B–D) (Supplementary Tables S35–S37). Scatter plots of individual single nucleotide polymorphisms (SNPs) of sleep traits and SNP effects on pan-cancer incidence and mortality among patients with cancer are shown in Supplementary Tables S38–S41. Radial MR analysis (Bowden et al., 2015; Bowden et al., 2016; Bowden et al., 2018; Ai et al., 2021) was used to identify outlying genetic variants in pan-cancer incidence and mortality among cancer patients (Supplementary Tables S42–S45). An MR leave-one-out sensitivity analysis for the effect of the sleep trait SNPs on outcomes was also conducted (Supplementary Tables S46–S49). Moreover, we used MR‒Egger and weighted median analyses to account for any potential pleiotropy (Supplementary Tables S50–S52), and the results showed that the SNPs used for instrumental variables had no obvious pleiotropy.
The present study found that long sleep duration and frequent insomnia symptoms were associated with a higher pan-cancer incidence, and long sleep duration had a causal relationship with the incidence of pan-cancer. Among cancer patients, we observed that long sleep duration and frequent insomnia symptoms were relevant risk factors and even potential causal risk factors for mortality. The highest mortality could occur in the definite evening chronotype, long sleep duration, and frequent insomnia symptoms subgroups. The survival analysis confirmed that the lowest survival probability could occur in the definite evening chronotype, long sleep duration, and frequent insomnia symptoms subgroups. In summary, this study provided convincing evidence of the causal relationships between multiple sleep traits and pan-cancer incidence and mortality among cancer patients through the use of multiple methods (Graphical Abstract) and might help in the management of cancer based on sleep behaviors.
Sleep duration has been found to be associated with cancer risk, including breast, lung, and prostate cancer (Richmond et al., 2019; Wang J. et al., 2021; Tao et al., 2021; Xie et al., 2021; Peeri et al., 2022; Wilunda et al., 2022). A U-shaped association was observed between sleep duration and lung cancer risk (Xie et al., 2021). A MR study observed suggestive evidence of a causal association between both short and long sleep duration and risk of some site-specific cancers (Titova et al., 2021). However, our study found a covariate relationship between short sleep duration and frequent insomnia symptoms, and therefore, we adjusted for multiple sleep traits with possible covariates instead of individual sleep trait in the analysis model. Finally, our study confirmed that long sleep duration was associated with a higher cancer incidence, which was consistent with several studies (Tao et al., 2021; Peeri et al., 2022; Wilunda et al., 2022). Furthermore, this study (Titova et al., 2021) only explored the causal relationship between sleep duration and cancer risk, and it did not consider the influence of other sleep traits on cancer risk and mortality. Thus, our study provided more evidence for the relationship between several sleep traits and cancer risk. In addition, inconsistent results have been reported regarding the relationship between insomnia and cancer risk (Sen et al., 2017; Richmond et al., 2019; Wang J. et al., 2021; Huo et al., 2021; Peeri et al., 2022). In the present study, we found that frequent insomnia symptoms were associated with a higher pan-cancer incidence in the Cox proportional hazard analysis, which was also confirmed by other studies (Sen et al., 2017; Peeri et al., 2022). However, the causal association of frequent insomnia symptoms with pan-cancer incidence was not found by linear MR analysis, which was consistent with the findings of another study (Richmond et al., 2019). This indicates that there is no causal relationship between insomnia symptoms and pan-cancer risk and that insomnia symptoms might be related to the increased pan-cancer incidence by interfering with multiple factors. Many conventional observational studies suggest that the evening chronotype is associated with an increased risk of some types of cancer (Erren et al., 2015; Hurley et al., 2019; Richmond et al., 2019; Wang J. et al., 2021; Sun et al., 2021; Von Behren et al., 2021; Xie et al., 2021; Costas et al., 2022; Peeri et al., 2022; Yu et al., 2022; Yuan et al., 2023). However, only a few studies analysed the causal association between chronotype and cancer risk (Richmond et al., 2019; Wang J. et al., 2021; Sun et al., 2021; Yu et al., 2022; Yuan et al., 2023). Our study explored the correlations and causal associations between evening chronotype and pan-cancer risk using multivariable Cox proportional hazard and MR analyses simultaneously. However, definite evening chronotype could not increase the pan-cancer incidence in the multivariable Cox proportional hazard analysis, which was also confirmed by the MR analysis.
Our study found consistent results for the association between unfavorable sleep duration, especially long sleep duration and mortality among cancer patients in both the multivariable Cox proportional hazard and MR analyses. Several cohort studies have revealed a relationship between sleep duration and mortality (Yeo et al., 2013; Xiao et al., 2014; Tao et al., 2021; Wilunda et al., 2022). However, no study has focused on the population with cancer. Only when focusing on patients with cancer can we accurately elucidate the effects of sleep duration on cancer mortality. Other sleep traits, such as insomnia (Li et al., 2014; Bertisch et al., 2018; Garfield et al., 2019; Hedstrom et al., 2019; Sogawa et al., 2022), are associated with all-cause mortality, cardiovascular disease mortality, and cancer mortality, which could support our findings that frequent insomnia symptoms were a relevant risk factor and even a potential causal risk factor for mortality among cancer patients. However, the results were usually confounded by self-reported exposures and residual confounding effects in previous studies, and MR analysis methods could overcome this limitation. In addition, although several studies have explored the association between chronotype and mortality (Dickerman et al., 2016; Knutson and von Schantz, 2018; Quinn et al., 2022), few studies have focused on the causal relationship between chronotype and cancer mortality. Our study observed the phenotypic association between the definite evening chronotype and mortality, although no causal association of definite evening chronotype with mortality was found.
The current study integrated multiple analyses, including multivariable Cox proportional hazard analysis, survival curve analysis, linear MR analysis, and nonlinear MR analysis, to explore the correlations and causal relationships of multiple sleep traits (chronotype, sleep duration, and insomnia symptoms) on pan-cancer incidence and mortality in the United Kingdom Biobank cohort. In the multivariable Cox proportional hazard analysis, we used the basic, further adjusted, and fully adjusted models. However, some confounding factors, including measurement error, selection bias, and unmeasured factors, might reverse the effect estimates. Therefore, we used MR analysis to mitigate potential bias. We used genetic instruments of sleep traits identified in the GWAS with a Bonferroni-corrected statistical significance threshold as to repeat the results. Considering the inconsistent results of sleep duration and the study outcomes, we also used nonlinear MR analysis to further explore the causal relationship between sleep duration and pan-cancer incidence and mortality among cancer patients. Additionally, we conducted a series of sensitivity analyses to assess the main assumptions and results.
This study had several limitations. First, the baseline characteristics of the stage, grade, and treatment of cancers were unavailable, which could restrict the exploration of their potential influence on the results. However, we included several relevant variables, including the number of treatments/medications and operations among cancer patients to decrease bias. Second, self-reported measures were used in the multivariable Cox proportional hazard analysis rather than objective measures. However, previous studies suggest good concordance between self-reported measures and accelerometer measures in United Kingdom Biobank (Dashti et al., 2019; Jones et al., 2019). Third, the pan-cancer risk increased was small with p values close to borderline in the multivariable Cox proportional hazard analysis. However, it is still statistically significant, and therefore, it is still relevant. Fourth, the genetic variants are associated with some confounders, such as body mass index, which might be affected by pleiotropy. However, the results were consistent with those of the primary analyses after further adjustment for potential confounders. In addition, we repeated the analysis after excluding participants with interfering sleep traits. However, the limited number of SNPs associated with a definite evening chronotype and long sleep duration may cause these results to be biased due to weak instrumental variables. Weak instrumental variables could affect the direction of the observational association (Burgess et al., 2016). In most observational studies, the associations of being an evening chronotype or having a long sleep duration with cancer were largely consistent across different methods (Xie et al., 2021; Lotti et al., 2022; Peeri et al., 2022). Therefore, bias should be minimal. Finally, we used unweighted genetic risk scores to minimize bias caused by potentially weak instruments in the MR analysis.
In summary, long sleep duration, and frequent insomnia symptoms were associated with a higher pan-cancer risk, and long sleep duration had a causal relationship with pan-cancer incidence. Among cancer patients, long sleep duration and frequent insomnia symptoms were relevant and potential causal risk factors for mortality, respectively. The present study also detected the highest risk of death and the lowest survival probability in unfavorable sleep trait subgroups, which further emphasizes the effect of unfavorable sleep traits on mortality outcomes in cancer patients. Therefore, attention should be given not only to the relationship between sleep issues and the risk of cancer in society but also to cancer patients with sleep issues to manage the prognosis of patients with cancer.
The datasets presented in this study can be found in online repositories. The names of the repository/repositories and accession number(s) can be found in the article/Supplementary Material.
The studies involving humans were approved by United Kingdom National Health Service’s National Research Ethics Service (ref 11/NW/0382). The studies were conducted in accordance with the local legislation and institutional requirements. Written informed consent for participation in this study was provided by the participants’ legal guardians/next of kin.
ST: Data curation, Formal Analysis, Investigation, Methodology, Writing–original draft. LH: Data curation, Formal Analysis, Investigation, Methodology, Writing–original draft. YB: Writing–review and editing. SA: Investigation, Methodology, Writing–review and editing. SC: Methodology, Writing–review and editing. QW: Data curation, Investigation, Writing–review and editing. XZ: Data curation, Writing–review and editing. WY: Data curation, Formal Analysis, Writing–review and editing. JS: Funding acquisition, Investigation, Writing–review and editing. LS: Supervision, Writing–review and editing. JD: Funding acquisition, Supervision, Writing–review and editing. LL: Funding acquisition, Supervision, Writing–review and editing.
The authors declare financial support was received for the research, authorship, and/or publication of this article. This work was supported by the National Natural Science Foundation of China (no. 82203579, 82271528 and 82271527), the Peking University Sixth Hospital Scientific Research Cultivation Fund (no. PY21003), the National Key Research and Development Program of China (no. 2021YFC0863700), National Programs for Brain Science and Brain-like Intelligence Technology of China (no. 2021ZD0200800, 2021ZD0200700) and Science Foundation of Peking University Cancer Hospital (JC202304).
This study was conducted using the United Kingdom Biobank resource (Application Number 48344). We are grateful to all participants and researchers involved in this prospective cohort.
The authors declare that the research was conducted in the absence of any commercial or financial relationships that could be construed as a potential conflict of interest.
All claims expressed in this article are solely those of the authors and do not necessarily represent those of their affiliated organizations, or those of the publisher, the editors and the reviewers. Any product that may be evaluated in this article, or claim that may be made by its manufacturer, is not guaranteed or endorsed by the publisher.
The Supplementary Material for this article can be found online at: https://www.frontiersin.org/articles/10.3389/fgene.2023.1309069/full#supplementary-material
Ai, S., Zhang, J., Zhao, G., Wang, N., Li, G., So, H. C., et al. (2021). Causal associations of short and long sleep durations with 12 cardiovascular diseases: linear and nonlinear Mendelian randomization analyses in UK Biobank. Eur. Heart J. 42 (34), 3349–3357. doi:10.1093/eurheartj/ehab170
Bertisch, S. M., Pollock, B. D., Mittleman, M. A., Buysse, D. J., Bazzano, L. A., Gottlieb, D. J., et al. (2018). Insomnia with objective short sleep duration and risk of incident cardiovascular disease and all-cause mortality: sleep Heart Health Study. Sleep 41 (6), zsy047. doi:10.1093/sleep/zsy047
Bowden, J., Davey Smith, G., and Burgess, S. (2015). Mendelian randomization with invalid instruments: effect estimation and bias detection through Egger regression. Int. J. Epidemiol. 44 (2), 512–525. doi:10.1093/ije/dyv080
Bowden, J., Davey Smith, G., Haycock, P. C., and Burgess, S. (2016). Consistent estimation in mendelian randomization with some invalid instruments using a weighted median estimator. Genet. Epidemiol. 40 (4), 304–314. doi:10.1002/gepi.21965
Bowden, J., Spiller, W., Del Greco, M. F., Sheehan, N., Thompson, J., Minelli, C., et al. (2018). Improving the visualization, interpretation and analysis of two-sample summary data Mendelian randomization via the Radial plot and Radial regression. Int. J. Epidemiol. 47 (6), 2100. doi:10.1093/ije/dyy265
Burgess, S., Davies, N. M., and Thompson, S. G. (2016). Bias due to participant overlap in two-sample Mendelian randomization. Genet. Epidemiol. 40 (7), 597–608. doi:10.1002/gepi.21998
Chien, K. L., Chen, P. C., Hsu, H. C., Su, T. C., Sung, F. C., Chen, M. F., et al. (2010). Habitual sleep duration and insomnia and the risk of cardiovascular events and all-cause death: report from a community-based cohort. Sleep 33 (2), 177–184. doi:10.1093/sleep/33.2.177
Costas, L., Frias-Gomez, J., Benavente Moreno, Y., Peremiquel-Trillas, P., Carmona, A., de Francisco, J., et al. (2022). Night work, chronotype and risk of endometrial cancer in the Screenwide case-control study. Occup. Environ. Med. 79, 624–627. doi:10.1136/oemed-2021-108080
Dashti, H. S., Jones, S. E., Wood, A. R., Lane, J. M., van Hees, V. T., Wang, H., et al. (2019). Genome-wide association study identifies genetic loci for self-reported habitual sleep duration supported by accelerometer-derived estimates. Nat. Commun. 10 (1), 1100. doi:10.1038/s41467-019-08917-4
Davey Smith, G., and Hemani, G. (2014). Mendelian randomization: genetic anchors for causal inference in epidemiological studies. Hum. Mol. Genet. 23 (R1), R89–R98. doi:10.1093/hmg/ddu328
Davidson, J. R., MacLean, A. W., Brundage, M. D., and Schulze, K. (2002). Sleep disturbance in cancer patients. Soc. Sci. Med. 54 (9), 1309–1321. doi:10.1016/s0277-9536(01)00043-0
Davies, N. M., Holmes, M. V., and Davey Smith, G. (2018). Reading Mendelian randomisation studies: a guide, glossary, and checklist for clinicians. BMJ 362, k601. doi:10.1136/bmj.k601
Dickerman, B. A., Markt, S. C., Koskenvuo, M., Hublin, C., Pukkala, E., Mucci, L. A., et al. (2016). Sleep disruption, chronotype, shift work, and prostate cancer risk and mortality: a 30-year prospective cohort study of Finnish twins. Cancer Causes Control 27 (11), 1361–1370. doi:10.1007/s10552-016-0815-5
Emdin, C. A., Khera, A. V., and Kathiresan, S. (2017). Mendelian randomization. JAMA 318 (19), 1925–1926. doi:10.1001/jama.2017.17219
Erren, T. C., Morfeld, P., and Gross, V. J. (2015). Night shift work, chronotype, and prostate cancer risk: incentives for additional analyses and prevention. Int. J. Cancer 137 (7), 1784–1785. doi:10.1002/ijc.29524
Fry, A., Littlejohns, T. J., Sudlow, C., Doherty, N., Adamska, L., Sprosen, T., et al. (2017). Comparison of sociodemographic and health-related characteristics of UK biobank participants with those of the general population. Am. J. Epidemiol. 186 (9), 1026–1034. doi:10.1093/aje/kwx246
Garfield, V., Joshi, R., Garcia-Hernandez, J., Tillin, T., and Chaturvedi, N. (2019). The relationship between sleep quality and all-cause, CVD and cancer mortality: the Southall and Brent REvisited study (SABRE). Sleep. Med. 60, 230–235. doi:10.1016/j.sleep.2019.03.012
Garland, S. N., Johnson, J. A., Savard, J., Gehrman, P., Perlis, M., Carlson, L., et al. (2014). Sleeping well with cancer: a systematic review of cognitive behavioral therapy for insomnia in cancer patients. Neuropsychiatr. Dis. Treat. 10, 1113–1124. doi:10.2147/NDT.S47790
Hayes, B. L., Robinson, T., Kar, S., Ruth, K. S., Tsilidis, K. K., Frayling, T., et al. (2022). Do sex hormones confound or mediate the effect of chronotype on breast and prostate cancer? A Mendelian randomization study. PLoS Genet. 18 (1), e1009887. doi:10.1371/journal.pgen.1009887
Hedstrom, A. K., Bellocco, R., Ye, W., Trolle Lagerros, Y., and Akerstedt, T. (2019). Association between insomnia and mortality is only evident among long sleepers. Nat. Sci. Sleep. 11, 333–342. doi:10.2147/NSS.S222049
Huang, B. H., Duncan, M. J., Cistulli, P. A., Nassar, N., Hamer, M., and Stamatakis, E. (2022). Sleep and physical activity in relation to all-cause, cardiovascular disease and cancer mortality risk. Br. J. Sports Med. 56 (13), 718–724. doi:10.1136/bjsports-2021-104046
Huo, Z., Ge, F., Li, C., Cheng, H., Lu, Y., Wang, R., et al. (2021). Genetically predicted insomnia and lung cancer risk: a Mendelian randomization study. Sleep. Med. 87, 183–190. doi:10.1016/j.sleep.2021.06.044
Hurley, S., Goldberg, D., Von Behren, J., Clague DeHart, J., Wang, S., and Reynolds, P. (2019). Chronotype and postmenopausal breast cancer risk among women in the California Teachers Study. Chronobiol Int. 36 (11), 1504–1514. doi:10.1080/07420528.2019.1658113
Irwin, M. R., Olmstead, R. E., Ganz, P. A., and Haque, R. (2013). Sleep disturbance, inflammation and depression risk in cancer survivors. Brain Behav. Immun. 30 (Suppl. l), S58–S67. doi:10.1016/j.bbi.2012.05.002
Jansen, P. R., Watanabe, K., Stringer, S., Skene, N., Bryois, J., Hammerschlag, A. R., et al. (2019). Genome-wide analysis of insomnia in 1,331,010 individuals identifies new risk loci and functional pathways. Nat. Genet. 51 (3), 394–403. doi:10.1038/s41588-018-0333-3
Jones, S. E., Lane, J. M., Wood, A. R., van Hees, V. T., Tyrrell, J., Beaumont, R. N., et al. (2019). Genome-wide association analyses of chronotype in 697,828 individuals provides insights into circadian rhythms. Nat. Commun. 10 (1), 343. doi:10.1038/s41467-018-08259-7
Khan, H., Kella, D., Kunutsor, S. K., Savonen, K., and Laukkanen, J. A. (2018). Sleep duration and risk of fatal coronary heart disease, sudden cardiac death, cancer death, and all-cause mortality. Am. J. Med. 131 (12), 1499–1505. doi:10.1016/j.amjmed.2018.07.010
Knutson, K. L., and von Schantz, M. (2018). Associations between chronotype, morbidity and mortality in the UK Biobank cohort. Chronobiol Int. 35 (8), 1045–1053. doi:10.1080/07420528.2018.1454458
Lane, J. M., Jones, S. E., Dashti, H. S., Wood, A. R., Aragam, K. G., van Hees, V. T., et al. (2019). Biological and clinical insights from genetics of insomnia symptoms. Nat. Genet. 51 (3), 387–393. doi:10.1038/s41588-019-0361-7
Lane, J. M., Vlasac, I., Anderson, S. G., Kyle, S. D., Dixon, W. G., Bechtold, D. A., et al. (2016). Genome-wide association analysis identifies novel loci for chronotype in 100,420 individuals from the UK Biobank. Nat. Commun. 7, 10889. doi:10.1038/ncomms10889
Li, Y., Zhang, X., Winkelman, J. W., Redline, S., Hu, F. B., Stampfer, M., et al. (2014). Association between insomnia symptoms and mortality: a prospective study of U.S. men. Circulation 129 (7), 737–746. doi:10.1161/CIRCULATIONAHA.113.004500
Lotti, S., Pagliai, G., Colombini, B., Sofi, F., and Dinu, M. (2022). Chronotype differences in energy intake, cardiometabolic risk parameters, cancer, and depression: a systematic review with meta-analysis of observational studies. Adv. Nutr. 13 (1), 269–281. doi:10.1093/advances/nmab115
Lv, X., Li, Y., Li, R., Guan, X., Li, L., Li, J., et al. (2022). Relationships of sleep traits with prostate cancer risk: a prospective study of 213,999 UK Biobank participants. Prostate 82 (9), 984–992. doi:10.1002/pros.24345
Marinac, C. R., Nelson, S. H., Flatt, S. W., Natarajan, L., Pierce, J. P., and Patterson, R. E. (2017). Sleep duration and breast cancer prognosis: perspectives from the Women's Healthy Eating and Living Study. Breast Cancer Res. Treat. 162 (3), 581–589. doi:10.1007/s10549-017-4140-9
Peeri, N. C., Tao, M. H., Demissie, S., and Nguyen, U. D. T. (2022). Sleep duration, chronotype, and insomnia and the risk of lung cancer: United Kingdom biobank cohort. Cancer Epidemiol. Biomarkers Prev. 31 (4), 766–774. doi:10.1158/1055-9965.EPI-21-1093
Phipps, A. I., Bhatti, P., Neuhouser, M. L., Chen, C., Crane, T. E., Kroenke, C. H., et al. (2016). Pre-diagnostic sleep duration and sleep quality in relation to subsequent cancer survival. J. Clin. Sleep. Med. 12 (4), 495–503. doi:10.5664/jcsm.5674
Quinn, L. M., Hadjiconstantinou, M., Brady, E. M., Bodicoat, D. H., Henson, J. J., Hall, A. P., et al. (2022). Chronotype and well-being in adults with established type 2 diabetes: a cross-sectional study. Diabet. Med. 39 (3), e14690. doi:10.1111/dme.14690
Richmond, R. C., Anderson, E. L., Dashti, H. S., Jones, S. E., Lane, J. M., Strand, L. B., et al. (2019). Investigating causal relations between sleep traits and risk of breast cancer in women: mendelian randomisation study. BMJ 365, l2327. doi:10.1136/bmj.l2327
Sanderson, E. (2021). Multivariable mendelian randomization and mediation. Cold Spring Harb. Perspect. Med. 11 (2), a038984. doi:10.1101/cshperspect.a038984
Sen, A., Opdahl, S., Strand, L. B., Vatten, L. J., Laugsand, L. E., and Janszky, I. (2017). Insomnia and the risk of breast cancer: the HUNT study. Psychosom. Med. 79 (4), 461–468. doi:10.1097/PSY.0000000000000417
Smith, G. D., and Ebrahim, S. (2003). Mendelian randomization': can genetic epidemiology contribute to understanding environmental determinants of disease? Int. J. Epidemiol. 32 (1), 1–22. doi:10.1093/ije/dyg070
Sogawa, R., Shimanoe, C., Tanaka, K., Hara, M., Nishida, Y., Furukawa, T., et al. (2022). Sex- and age-specific all-cause mortality in insomnia with hypnotics: findings from Japan multi-institutional Collaborative Cohort Study. Sleep. Med. 100, 410–418. doi:10.1016/j.sleep.2022.09.020
Staley, J. R., and Burgess, S. (2017). Semiparametric methods for estimation of a nonlinear exposure-outcome relationship using instrumental variables with application to Mendelian randomization. Genet. Epidemiol. 41 (4), 341–352. doi:10.1002/gepi.22041
Sun, X., Ye, D., Jiang, M., Qian, Y., and Mao, Y. (2021). Genetically proxied morning chronotype was associated with a reduced risk of prostate cancer. Sleep 44 (10), zsab104. doi:10.1093/sleep/zsab104
Sung, H., Ferlay, J., Siegel, R. L., Laversanne, M., Soerjomataram, I., Jemal, A., et al. (2021). Global cancer statistics 2020: GLOBOCAN estimates of incidence and mortality worldwide for 36 cancers in 185 countries. CA Cancer J. Clin. 71 (3), 209–249. doi:10.3322/caac.21660
Svensson, T., Saito, E., Svensson, A. K., Melander, O., Orho-Melander, M., Mimura, M., et al. (2021). Association of sleep duration with all- and major-cause mortality among adults in Japan, China, Singapore, and korea. JAMA Netw. Open 4 (9), e2122837. doi:10.1001/jamanetworkopen.2021.22837
Tao, F., Cao, Z., Jiang, Y., Fan, N., Xu, F., Yang, H., et al. (2021). Associations of sleep duration and quality with incident cardiovascular disease, cancer, and mortality: a prospective cohort study of 407,500 UK biobank participants. Sleep. Med. 81, 401–409. doi:10.1016/j.sleep.2021.03.015
Titova, O. E., Michaelsson, K., Vithayathil, M., Mason, A. M., Kar, S., Burgess, S., et al. (2021). Sleep duration and risk of overall and 22 site-specific cancers: a Mendelian randomization study. Int. J. Cancer 148 (4), 914–920. doi:10.1002/ijc.33286
Trudel-Fitzgerald, C., Zhou, E. S., Poole, E. M., Zhang, X., Michels, K. B., Eliassen, A. H., et al. (2017). Sleep and survival among women with breast cancer: 30 years of follow-up within the Nurses' Health Study. Br. J. Cancer 116 (9), 1239–1246. doi:10.1038/bjc.2017.85
Von Behren, J., Hurley, S., Goldberg, D., Clague DeHart, J., Wang, S. S., and Reynolds, P. (2021). Chronotype and risk of post-menopausal endometrial cancer in the California Teachers Study. Chronobiol Int. 38 (8), 1151–1161. doi:10.1080/07420528.2021.1912073
Wang, J., Tang, H., Duan, Y., and Yang, S. (2021a). Association between sleep traits and lung cancer: a mendelian randomization study. J. Immunol. Res. 2021, 1893882. doi:10.1155/2021/1893882
Wang, W., Jiang, H., Zhang, Z., Duan, W., Han, T., and Sun, C. (2021b). Interaction between dietary branched-chain amino acids and genetic risk score on the risk of type 2 diabetes in Chinese. Genes Nutr. 16 (1), 4. doi:10.1186/s12263-021-00684-6
Wilunda, C., Abe, S. K., Svensson, T., Sawada, N., Tsugane, S., Wada, K., et al. (2022). Sleep duration and risk of cancer incidence and mortality: a pooled analysis of six population-based cohorts in Japan. Int. J. Cancer 151 (7), 1068–1080. doi:10.1002/ijc.34133
Wong, J. Y., Bassig, B. A., Vermeulen, R., Hu, W., Ning, B., Seow, W. J., et al. (2017). Sleep duration across the adult lifecourse and risk of lung cancer mortality: a cohort study in xuanwei, China. Cancer Prev. Res. (Phila). 10 (6), 327–336. doi:10.1158/1940-6207.CAPR-16-0295
Xiao, Q., Arem, H., Pfeiffer, R., and Matthews, C. (2017). Prediagnosis sleep duration, napping, and mortality among colorectal cancer survivors in a large US cohort. Sleep 40 (4), zsx010. doi:10.1093/sleep/zsx010
Xiao, Q., Keadle, S. K., Hollenbeck, A. R., and Matthews, C. E. (2014). Sleep duration and total and cause-specific mortality in a large US cohort: interrelationships with physical activity, sedentary behavior, and body mass index. Am. J. Epidemiol. 180 (10), 997–1006. doi:10.1093/aje/kwu222
Xie, J., Zhu, M., Ji, M., Fan, J., Huang, Y., Wei, X., et al. (2021). Relationships between sleep traits and lung cancer risk: a prospective cohort study in UK Biobank. Sleep 44 (9). doi:10.1093/sleep/zsab089
Yeo, Y., Ma, S. H., Park, S. K., Chang, S. H., Shin, H. R., Kang, D., et al. (2013). A prospective cohort study on the relationship of sleep duration with all-cause and disease-specific mortality in the Korean Multi-center Cancer Cohort study. J. Prev. Med. Public Health 46 (5), 271–281. doi:10.3961/jpmph.2013.46.5.271
Yoon, H. S., Yang, J. J., Song, M., Lee, H. W., Lee, Y., Lee, K. M., et al. (2015). Short sleep duration and its correlates among cancer survivors in korea: the korea national health and nutrition examination surveys. Asian Pac J. Cancer Prev. 16 (11), 4705–4710. doi:10.7314/apjcp.2015.16.11.4705
Yu, Y., Hou, L., Shi, X., Sun, X., Liu, X., Yu, Y., et al. (2022). Impact of nonrandom selection mechanisms on the causal effect estimation for two-sample Mendelian randomization methods. PLoS Genet. 18 (3), e1010107. doi:10.1371/journal.pgen.1010107
Yuan, S., Mason, A. M., Titova, O. E., Vithayathil, M., Kar, S., Chen, J., et al. (2023). Morning chronotype and digestive tract cancers: mendelian randomization study. Int. J. Cancer 152 (4), 697–704. doi:10.1002/ijc.34284
Zhou, T., Yuan, Y., Xue, Q., Li, X., Wang, M., Ma, H., et al. (2022). Adherence to a healthy sleep pattern is associated with lower risks of all-cause, cardiovascular and cancer-specific mortality. J. Intern Med. 291 (1), 64–71. doi:10.1111/joim.13367
Keywords: sleep traits, pan-cancer incidence, mortality, mendelian randomization, causal relationships
Citation: Tian S, Huangfu L, Bao Y, Ai S, Chang S, Wang Q, Zhu X, Yan W, Shi J, Shi L, Deng J and Lu L (2023) Causal associations of sleep traits with cancer incidence and mortality. Front. Genet. 14:1309069. doi: 10.3389/fgene.2023.1309069
Received: 07 October 2023; Accepted: 13 November 2023;
Published: 23 November 2023.
Edited by:
Yilong Li, First Affiliated Hospital of Harbin Medical University, ChinaReviewed by:
Xianxian Wu, Chinese Academy of Medical Sciences and Peking Union Medical College, ChinaCopyright © 2023 Tian, Huangfu, Bao, Ai, Chang, Wang, Zhu, Yan, Shi, Shi, Deng and Lu. This is an open-access article distributed under the terms of the Creative Commons Attribution License (CC BY). The use, distribution or reproduction in other forums is permitted, provided the original author(s) and the copyright owner(s) are credited and that the original publication in this journal is cited, in accordance with accepted academic practice. No use, distribution or reproduction is permitted which does not comply with these terms.
*Correspondence: Lin Lu, bGlubHVAYmptdS5lZHUuY24=; Jiahui Deng, amlhaHVpZGVuZzIwMTJAYmptdS5lZHUuY24=; Le Shi, bGVzaGlAYmptdS5lZHUuY24=
Disclaimer: All claims expressed in this article are solely those of the authors and do not necessarily represent those of their affiliated organizations, or those of the publisher, the editors and the reviewers. Any product that may be evaluated in this article or claim that may be made by its manufacturer is not guaranteed or endorsed by the publisher.
Research integrity at Frontiers
Learn more about the work of our research integrity team to safeguard the quality of each article we publish.