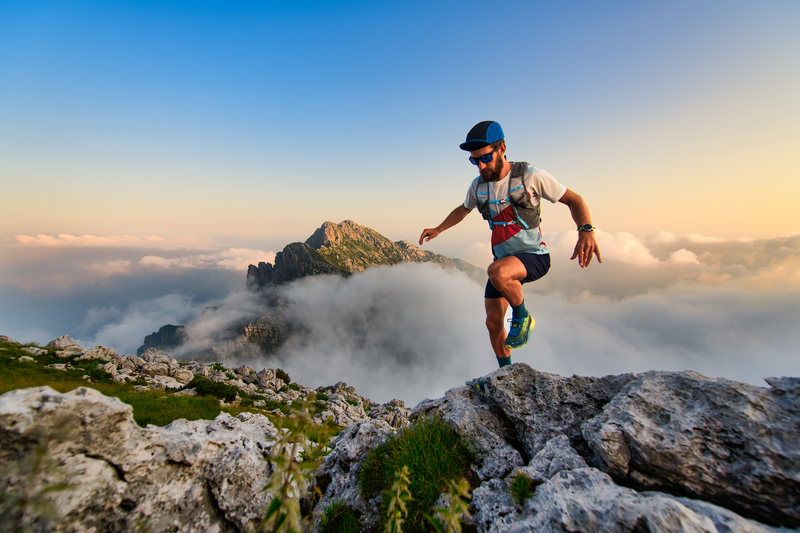
95% of researchers rate our articles as excellent or good
Learn more about the work of our research integrity team to safeguard the quality of each article we publish.
Find out more
ORIGINAL RESEARCH article
Front. Genet. , 08 January 2024
Sec. Behavioral and Psychiatric Genetics
Volume 14 - 2023 | https://doi.org/10.3389/fgene.2023.1301150
Background: The relationship between genotype and phenotype is governed by numerous genetic interactions (GIs), and the mapping of GI networks is of interest for two main reasons: 1) By modelling biological robustness, GIs provide a powerful opportunity to infer compensatory biological mechanisms via the identification of functional relationships between genes, which is of interest for biological discovery and translational research. Biological systems have evolved to compensate for genetic (i.e., variations and mutations) and environmental (i.e., drug efficacy) perturbations by exploiting compensatory relationships between genes, pathways and biological processes; 2) GI facilitates the identification of the direction (alleviating or aggravating interactions) and magnitude of epistatic interactions that influence the phenotypic outcome. The generation of GIs for human diseases is impossible using experimental biology approaches such as systematic deletion analysis. Moreover, the generation of disease-specific GIs has never been undertaken in humans.
Methods: We used our Indian schizophrenia case-control (case-816, controls-900) genetic dataset to implement the workflow. Standard GWAS sample quality control procedure was followed. We used the imputed genetic data to increase the SNP coverage to analyse epistatic interactions across the genome comprehensively. Using the odds ratio (OR), we identified the GIs that increase or decrease the risk of a disease phenotype. The SNP-based epistatic results were transformed into gene-based epistatic results.
Results: We have developed a novel approach by conducting gene-based statistical epistatic analysis using an Indian schizophrenia case-control genetic dataset and transforming these results to infer GIs that increase the risk of schizophrenia. There were ∼9.5 million GIs with a p-value
Conclusion: Unlike model organisms, this approach is specifically viable in humans due to the availability of abundant disease-specific genome-wide genotype datasets. The study exclusively identified brain/nervous system-related processes, affirming the findings. This computational approach fills a critical gap by generating practically non-existent heritable disease-specific human GIs from human genetic data. These novel datasets can train innovative deep-learning models, potentially surpassing the limitations of conventional GWAS.
Schizophrenia, a debilitating mental disorder with a lifetime prevalence of around 1% and notable morbidity and mortality, presents a significant public health challenge. Currently, the Psychiatric Genomics Consortium (PGC) has identified over 250 schizophrenia risk loci, among more than 300 unique association loci linked to major psychiatric disorders, necessitating urgent exploration (Lam et al., 2019; Periyasamy et al., 2019; Ripke et al., 2020). The discovery of these loci has been greatly facilitated by Genome-wide Association Studies (GWAS), a cornerstone in the field, having successfully unveiled numerous loci associated with various complex traits and diseases (Visscher et al., 2017). The GWAS Catalogue of September 2023 (Marees et al., 2018) catalogues a remarkable 552,116 significant variant-trait associations from 6,566 publications. Despite GWAS’s remarkable success in identifying this multitude of loci, only a relatively small fraction of single nucleotide polymorphisms (SNPs) associated with diseases/traits have been functionally characterised. This limitation stems from diverse challenges encompassing scientific, technical, methodological, and funding aspects. Additionally, a significant majority of low penetrance risk variants identified by GWAS are situated in non-coding regions, impacting gene regulation, thereby presenting substantial methodological hurdles in accurately pinpointing the causal variant, identifying the genes it influences, and deducing the molecular mechanisms of diseases (Egervari et al., 2018). The unravelling of disease mechanisms and gaining fundamental biological insights are pivotal for offering improved evidence-based and personalised treatments. Existing in vivo models need increased sensitivity to observe phenotypes associated with low penetrance risk variants, given that the biological robustness inherent in these systems can complicate results. Consequently, novel approaches are imperative for swiftly translating GWAS discoveries into viable medical interventions.
The complexity of biological systems raises many scientific challenges as they have evolved to be robust in the face of environmental and genetic perturbations. Systemic properties such as extreme-polygenicity (Boyle et al., 2017), pleiotropy and robustness are a result of redundant and compensatory mechanisms that have evolved between molecular and organismic resolutions (Periyasamy et al., 2009; Periyasamy et al., 2013), contributing to nonadditive/nonlinear effects in biological systems. These compensatory mechanisms regulated by various molecular activities can contribute to biological epistasis (Moore, 2005; Sackton and Hartl, 2016). Epistasis/GI manifests when the effects of mutations/variants depend on the genetic background on which they occur (Domingo et al., 2019). Such dependency on genetic background/context can cause phenotypic diversity between individuals and, on evolutionary timescales, incompatibilities between different species. Such issues raise concern when using in-vivo models to represent humans, as evidenced by the alarming drug failure rates (∼96%) when translating from preclinical to clinical trials (Hingorani et al., 2019). Technologies that generate data to study the above systemic properties are required to bridge the genotype-phenotype gap. Fortunately, some high-throughput technologies in genomics could be advantageous to infer GIs, where they are thought to be one of the causes of missing heritability (Eichler et al., 2010; Zuk et al., 2012). GIs frequently connect genes between compensatory pathways, so they confound our ability to predict phenotype from gene-environment interactions. This major challenge must be addressed to realise the potential of precision medicine. Causalities can be modelled by identifying GIs that enhance or suppress a phenotype (Costanzo et al., 2016). Currently, there is a yeast GI (Costanzo et al., 2016) and a database containing ∼223,000 GIs for the human cancer cell lines (Horlbeck et al., 2018; Mair et al., 2019), a mere fraction of the estimated number of gene-gene combinations (∼200 million) for humans. These datasets were generated by observing cellular phenotypes for many combinations of double gene-silencing experiments, analogous to generating gene-based statistical epistasis data. However, this laborious experimental approach becomes an improbable task when considering human endophenotypes, let alone human disease phenotypes such as schizophrenia. Hence, the only solution will be to use in silico approaches. Although there are computational approaches to predicting GIs (Sun et al., 2014; Wei et al., 2014; Niel et al., 2015; Fang et al., 2019), genome-wide disease-specific human-level GI data have not been generated to date.
All participants provided written informed consent, and the study received ethical approval from each participating institution’s institutional review boards. This research adhered to the Strengthening the Reporting of Genetic Association Studies (STREGA) reporting guideline. Our participant cohort was drawn from The Schizophrenia Research Foundation in Chennai, India, and overwhelmingly comprised individuals of Tamil ethnicity, accounting for more than 97% of the sample. We employed standardised assessment tools, which were administered in Tamil when necessary. These tools included the Diagnostic Interview for Genetic Studies (Nurnberger et al., 1994), the Family Interview for Genetic Studies (Maxwell, 1992), the DSM-IV diagnostic criteria, and a consensus diagnostic procedure.
The study population encompassed two main groups: 1) a family dataset that was assembled based on the presence of multiple affected family members and 2) a case-control dataset. Our study involved 1,716 participants, of which 816 were diagnosed with schizophrenia. The recruitment, genotyping, and data analysis were carried out in consecutive phases, commencing on 1 January 2001.
To estimate the study power, we used a dedicated R package powerGWASInteraction (Kooperberg and LeBlanc, 2008) to conduct power analysis for genetic interactions. We used the default parameters for the GxG interaction model and conducted the analysis using powerGG (). The power was set at 80%, alpha at 0.05, case-control ratio at 0.5, disease prevalence at 0.01 and the number of genes tested was set to 20,000. The sample size was estimated to be approximately 8,000. In contrast, the false discovery rate (FDR) method is widely utilised in situations with numerous tests where the expense of false positives is minimal and employing the Bonferroni procedure is unfeasible due to the sheer volume of simultaneous tests (i.e., ∼200 million in this case). It maintains strict control over the family-wise error rate and becomes more conservative as the number of comparisons increases. This means that as the number of tests grows, Bonferroni correction becomes increasingly stringent, potentially leading to a higher likelihood of false negatives. FDR, on the other hand, focuses on the proportion of false positives among the declared significant findings. It controls the rate of false discoveries and, in many cases, allows for a higher discovery rate than Bonferroni, especially in situations with many hypotheses being tested. Hence, we also used the r package pwrFDR (Jung, 2005; Liu and Hwang, 2007), a dedicated application to calculate power based on FDR. The average power was 100% for an effect size of 1.5, a sample size of 1716 and an alpha of 0.05.
The study sample was assembled and underwent genotyping in sequential phases spanning 15 years. The family sample, consisting of 658 individuals, was subjected to genotyping utilising the Illumina CNV370 Beadchip array. Concurrently, a group of unrelated controls, totalling 199 individuals, was initially enrolled in the study and subsequently genotyped using Illumina OmniExpress-12 arrays. In the later stages of the study, additional cases and controls were brought on board. They underwent genotyping in two distinct batches: the first wave included 1,370 individuals, and the second encompassed 1,008 individuals. It is important to note that the figures provided here represent the initial sample sizes before the quality control process. The standard GWAS sample quality control procedures have been followed to check for ancestry and relatedness (Peterson et al., 2019).
We conducted a multi-dimensional scaling (MDS) analysis with the objectives of assessing potential array-related effects and determining the genetic similarity of the Indian samples to the South Asian (SAS) population in the 1,000 Genomes (1 KG) phase 3 reference dataset. The MDS analysis was performed using all common SNPs found in both the Indian family and case-control datasets and the 1,000 Genomes dataset, comprising a total of 2,504 samples and 26,231 SNPs after pruning. We excluded SNPs with ambiguous strand information to avoid any potential issues related to strand orientation. The absence of outliers in the analysis confirmed that our samples exhibited Indian ancestry (See Figure 1).
FIGURE 1. MDS analysis using 1,000G SAS population. Super populations include African (AFR), Admixed American (AMR), European (EUR), East Asian (EAS) and South Asian (SAS). The figure shows the Indian case-control datasets (In-House) clustering with the SAS population.
To ensure the identification of genetically related individuals, we compiled a set of 26,939 single nucleotide polymorphisms (SNPs) that were in linkage disequilibrium independently and had a minor allele frequency exceeding 0.05. These SNPs were consistently present across all genotyping platforms employed in the study. In the family samples, we employed identity by descent (IBD) analysis and cross-referenced the results with the clinical records associated with the four datasets. This allowed us to detect and eliminate duplicate entries and incorrectly specified relationships. Individuals demonstrating relatedness beyond second-degree (as indicated by PI-HAT≥0.1875) were excluded from the case-control datasets. Subsequently, we integrated the four datasets to identify discrepancies in relatedness within and between families. This process led to the removal of 143 individuals who exhibited such discrepancies. Before proceeding with the imputation process, the cleaned datasets underwent further filtering based on specific parameters, including --geno 0.02, --maf 0.01, --hwe 0.001, and --mind 0.1.
As previously described, we applied imputation to each of our four datasets using the 1,000 Genomes (1 KG) phase 3 reference dataset. During this process, we updated the SNP coordinates to align with human genome build 37 and ensured they were oriented on the positive strand. To achieve accurate phasing, we employed SHAPEIT v2.72720. Subsequently, we carried out an imputation using IMPUTE v2.3.021. For the imputation procedure, each chromosome was divided into segments of 5 megabases with a 250-kilobase overlap. As recommended by the authors, we utilised all available 1 KG populations, encompassing approximately 2,504 individuals, as the reference dataset. The resulting imputed data was then converted into ‘best guess’ genotypes and saved in binary PLINK format for further analysis.
From an initial set of 81,177,102 imputed single nucleotide polymorphisms (SNPs), we applied a series of stringent filters and quality control measures to reduce the dataset to approximately 6.2–6.5 million SNPs. These filters included: 1) Removing SNPs with an INFO score less than 0.8. 2) Extracting of unrelated individuals. 3) Eliminating SNPs with a missing data rate exceeding 0.05. 4) Discarding SNPs with a Hardy-Weinberg equilibrium (HWE) p-value less than 1 × 10−5 in both cases and controls. 5) Applying a stricter HWE threshold, with a p-value less than 1 × 10−6 in controls. 6) Employing an even more stringent HWE threshold, with a p-value less than 1 × 10−10 in cases. After applying these quality control criteria, a total of 5.5 million genetic variants and 1716 individuals (comprising 816 cases and 900 controls) remained in the dataset, having successfully met all filtering and quality control requirements.
Imputed data increased the SNP coverage for comprehensive analysis of epistatic interactions across the genome. We mapped the genes with promoter regions from the FANTOM5 project (https://fantom.gsc.riken.jp/) (Lizio et al., 2015; Noguchi et al., 2017), which had robust experimentally validated promotor regions for GENCODE genes. All SNPs within the promotor and their genes, considered to represent a gene, were included in the analysis. Only intergenic SNP-SNP interactions were considered for generating GIs. The generation of GIs using the standard epistatic analysis method required significant investment in time (i.e., many weeks), computational and storage resources as it generated results for billions of SNP-SNP combinations. Initially, we used the fast epistatic analysis method, BOOST (Wan et al., 2010), on the SNPs located in genes and their regulatory regions to identify the most relevant SNPs and then used the standard epistatic analysis method on nominally significant epistatic SNP-SNP combinations to generate OR for GIs (Steps 1-6 in Figure 2). Plink 1.9 (Purcell et al., 2007) was used to conduct genome-wide epistasis analysis. The multiple testing burden was further reduced by collapsing the p-values and ORs of SNP-SNP to gene-gene combinations. The SNP-based epistatic results were transformed into gene-based epistatic results. The most significant SNP-SNP p-value combination representing the two genes was transformed into p-values representing the gene-gene combinations. By using the odds ratio (OR), we identified the GIs that increase (OR >1) or decrease (OR <1) the risk of a disease phenotype (i.e., schizophrenia). The number of gene-gene combinations for multiple tests was estimated to be ∼200 million, assuming 20,000 protein-coding genes. The Bonferroni threshold was set at 2.5 × 10−10 (i.e. 0.05/200,000,000), and the false discovery rate threshold (FDR value of 0.05) for significant GIs was set at 2.375 × 10−3 for FDR significant GIs, assuming 200 million gene-gene combinations.
FIGURE 2. The high-performance computing (HPC) bioinformatics workflow for generating GIs from disease-specific case-control datasets; Genes and regulatory regions data: FANTOM5 and GENCODE project.
A subset of GIs with OR>2 and OR<0.5 was validated using spatial analysis of functional enrichment (SAFE) (Baryshnikova, 2016; Baryshnikova et al., 2018) and REVIGO’s (Supek et al., 2011) GO disease specificity analyses to confirm the relevance of the generated data (Steps 7–9). SAFE is a systematic method for annotating biological networks and examining functional organisation. Due to the hierarchical nature of GO biological processes, the resulting lists of GO terms can be large and highly redundant. REVIGO summarises the non-redundant GO terms using a semantic similarity algorithm that uses the ‘most informative common ancestor’ approach to identify precise GO biological process terms. We applied SAFE to annotate the schizophrenia GI similarity network with GO terms and used REVIGO to validate the GIs of schizophrenia. We used REVIGO to reduce redundancy and summarise incomprehensible lists of Gene Ontology terms by finding a representative subset of the terms by clustering using semantic similarity measures. We used GOATOOLS (https://github.com/tanghaibao/goatools) (Klopfenstein et al., 2018) to plot the GO biological process hierarchy. GO release 2023-07-27 (http://current.geneontology.org/ontology/go-basic.obo) was used for this analysis.
We considered all intragenic genetic interactions with nominal p-values (1 × 10−5) to identify the hub genes and the potential drives of schizophrenia. We considered a two-fold increase or decrease in ORs for this analysis. We considered OR > 2 for all increased-risk GIs, and for all decreased-risk GIs, we considered OR<0.5. From this list, we tabulated the genetic interactions that showed an increased risk for schizophrenia and decreased or no risk for schizophrenia.
We have generated a global schizophrenia GI dataset comprising ∼9.5 million GIs (1 × 10−5). These data include intragenic and as well as promoter/enhancer regions.
We have developed a novel approach by conducting gene-based statistical epistatic analysis using an Indian schizophrenia case-control (case-816, controls-900) genetic dataset and transforming these results to infer GIs. Although underpowered, we successfully generated meaningful GI data with a small sample size 1716. The SAFE and REVIGO analysis confirmed this by identifying brain/nervous system-related biological processes exclusively. None of the GIs reached the Bonferroni threshold of 2.5 × 10−10. However, all 9.5 million GIs surpassed the FDR significance threshold.
There were ∼9.5 million GIs with a nominal p-value
There were ∼110,000 GIs with OR >2 or OR <0.5. SAFE and REVIGO analysis exclusively identified brain/nervous system-related biological processes, affirming the findings. The genetic interactions of schizophrenia were functionally enriched in brain/nervous system-related biological processes. Most biological processes were semantically related to nervous system development, with synaptic transmission being the next largest cluster, followed by synapse organisation. Figure 3 shows the enriched biological processes identified by SAFE. The lowest processes in the GO hierarchy are all related to the nervous system (see Supplementary Figure S1).
FIGURE 3. Spatial analysis of functional enrichment of GI data generated using the Indian schizophrenia genetic data. The x-axis on the bottom shows the enrichment score of biological processes, and the x-axis on the top shows the specificity of biological processes. The y-axis shows the GO biological process enriched after multiple testing corrections. The biological processes are organised in the ascending order of specificity.
Notable genes are hub genes, which can be considered drivers of schizophrenia. Figure 4 shows the top hub genes involved in schizophrenia. CSMD1 had the highest GIs, followed by KCNIP4, WWOX and NRXN3. Each hub gene increases the risk for schizophrenia when interacting with a subset of genes and shows decreased or no risk for schizophrenia with another subset of genes. Supplementary Table S1 shows the complete list of hub genes and their functions based on existing literature.
FIGURE 4. The hub genes of schizophrenia. The x-axis shows the number of gene interactions of hub genes that increase or decrease the risk of schizophrenia. The y-axis shows the hub genes of schizophrenia.
We observed the nervous system development to have the highest enrichment, and neurogenesis to have the highest specificity. The schizophrenia GIs were primarily enriched in biological processes involved in the nervous system and neuron-related processes. In contrast to model organisms, this approach is specifically viable in humans due to the availability of abundant disease-specific genome-wide genotype datasets. Despite limited power, meaningful GI data was generated with a small sample. SAFE and REVIGO analyses exclusively identified brain/nervous system-related processes, affirming the reliability of the generated GIs. This computational approach fills a critical gap by generating practically non-existent heritable disease-specific human GIs from human genetic data. These novel datasets can train innovative deep-learning models for post-GWAS functional characterisation, potentially surpassing the limitations of conventional GWAS.
Biologists have used double gene silencing approaches for over two decades to study GIs (Mani et al., 2008). Generating GI data has been daunting as it involves establishing cell/animal lines to observe relevant phenotypes, which often takes weeks to months per GI experiment. However, this laborious experimental approach becomes an improbable task when considering human endophenotypes, let alone complex human disease phenotypes such as schizophrenia. Moreover, this is neither ethical nor practical in humans, and observational studies investigating phenotypes in any organism are subject to a range of unknown confounding effects that are difficult to control. We have proposed a novel approach by conducting analogous gene-based statistical epistatic analysis using human genetic data and transforming these results to infer gene-based GIs. This approach is only feasible in humans because, unlike model organisms, abundant GWAS data are available, including large-scale publicly available biobank data. Compared to traditional observational experiments, our strategy is robust to confounding, rapid, and cost-effective and can be performed using existing GWAS data. The novel contribution of our approach consists in how we generate and utilise the GI information. OR is an often-ignored parameter. This information is useful in detecting the direction and magnitude of GIs. While existing in silico approaches focus on the presence or absence of GIs, we also include the effect size and direction of disease-specific GIs to infer increased or decreased disease risk.
Only two cellular-level GI datasets are available for the research community: a wet-lab generated comprehensive yeast GI dataset (∼8 million GIs) and a cancer GI dataset (∼230,000) generated using human cancer cell lines with cell growth/viability as the phenotype. Our approach addresses this problem by developing a novel in silico method to generate heritable disease-specific GIs such as schizophrenia. The significant short-term outcomes will include: 1) Producing a schizophrenia GI resource for the global biomedical science community. 2) A contribution to the modelling of biological robustness, thereby providing a powerful opportunity to infer compensatory biological mechanisms and to study genetic (i.e., variations and mutations) and environmental (i.e., drug efficacy) perturbations. 3) The generation of GIs for other human heritable diseases. In addition to contributing to basic research in schizophrenia, disease-specific GIs are highly important for translational research. The ubiquitous nature of biological robustness is a major problem for pharmaceutical drug discovery, as humans can develop resistance to therapeutic agents (Boucher and Jenna, 2013). GIs can map the elusive relationship between genotype and phenotype and are thought to underlie many common heritable diseases. Furthermore, exploring GI networks may help to realise the gene networks underlying phenotypical variation and polygenic diseases.
While GWAS has successfully unveiled numerous risk loci, it faces ongoing critique due to its limited ability to elucidate many molecular mechanisms influencing human phenotypes that contribute to disease risk. GWAS loci often prove challenging to decipher because linkage disequilibrium frequently masks the causal variant during association, and identifying the specific gene responsible for mediating variant effects on the trait is seldom achievable solely through GWAS data. Moreover, a significant proportion of GWAS risk variants with low penetrance reside in non-coding regions that impact the gene regulation (Egervari et al., 2018), presenting considerable methodological hurdles in accurately pinpointing the causal variant, determining the genes it governs, and deducing disease molecular mechanisms (Schaid et al., 2018). These obstacles are unique to variant-trait associations within the realm of GWAS. However, our approach remains unhindered by these challenges as it can directly predict the causal genes and molecular mechanisms from human genetic data, aligning with one of GWAS’s primary objectives—to identify causal genes, molecular mechanisms, and potential interventions.
We plan to use the workflow to generate GIs for a larger dataset, such as the PGC dataset. We anticipate large datasets could reveal more significant GIs for schizophrenia. These datasets can be used for various systems biology studies, such as developing explainable deep learning models to predict disease mechanisms (Ma et al., 2018).
The original contributions presented in the study are publicly available. This data can be found here: https://espace.library.uq.edu.au/view/UQ:24c3e5e.
The studies involving humans were approved by West Moreton Hospital and Health Service HREC and Research Ethics and Integrity, The University of Queensland. The studies were conducted in accordance with the local legislation and institutional requirements. Written informed consent for participation in this study was provided by the participants’ legal guardians/next of kin.
SP: Conceptualization, Data curation, Formal Analysis, Investigation, Methodology, Project administration, Resources, Software, Supervision, Validation, Visualization, Writing–original draft, Writing–review and editing. PY: Writing–review and editing, Data curation, Formal Analysis, Methodology, Software, Visualization, Writing–original draft. SJ: Project administration, Resources, Supervision, Writing–review and editing, Conceptualization, Data curation. RT: Data curation, Funding acquisition, Project administration, Resources, Writing–review and editing, Conceptualization, Supervision. BM: Funding acquisition, Project administration, Resources, Supervision, Writing–review and editing, Conceptualization.
The author(s) declare financial support was received for the research, authorship, and/or publication of this article. This study was supported by grants 143027, 339454, 613602, and 1047956 from the Australian NHMRC (BM and RT).
We thank the patients and their family members who participated in this study. Additionally, we acknowledge the valuable support from the staff at Sowmanasya Hospital in Tiruchirappalli, Athma Hospitals and Research Private Ltd. in Tiruchirappalli, Asha Hospital in Hyderabad, Vazhikatti Mental Health Centre and Research Institute in Coimbatore, and Manthin Maiyam Hospital in Erode.
The authors declare that the research was conducted in the absence of any commercial or financial relationships that could be construed as a potential conflict of interest.
All claims expressed in this article are solely those of the authors and do not necessarily represent those of their affiliated organizations, or those of the publisher, the editors and the reviewers. Any product that may be evaluated in this article, or claim that may be made by its manufacturer, is not guaranteed or endorsed by the publisher.
The Supplementary Material for this article can be found online at: https://www.frontiersin.org/articles/10.3389/fgene.2023.1301150/full#supplementary-material
SUPPLEMENTARY FIGURE S1 | The Gene Ontology biological processes enriched in schizophrenia. The yellow nodes indicate the enriched process, and the white nodes indicate the processes linking the enriched nodes to higher- level biological processes.
SUPPLEMENTARY TABLE S1 | Tabs one and two show the number of hub gene interactions that increase or decrease the risk of schizophrenia. Tab three contains the list of top hup genes and their functions.
Baryshnikova, A. (2016). Systematic functional annotation and visualization of biological networks. Cell Syst. 2 (6), 412–421. doi:10.1016/j.cels.2016.04.014
Baryshnikova, A. (2018). Spatial analysis of functional enrichment (SAFE) in large biological networks. Methods Mol. Biol. 1819, 249–268. doi:10.1007/978-1-4939-8618-7_12
Boucher, B., and Jenna, S. (2013). Genetic interaction networks: better understand to better predict. Front. Genet. 4 (290), 290. doi:10.3389/fgene.2013.00290
Boyle, E. A., Li, Y. I., and Pritchard, J. K. (2017). An expanded view of complex traits: from polygenic to omnigenic. Cell 169 (7), 1177–1186. doi:10.1016/j.cell.2017.05.038
Costanzo, M., VanderSluis, B., Koch, E. N., Baryshnikova, A., Pons, C., Tan, G., et al. (2016). A global genetic interaction network maps a wiring diagram of cellular function. Science 353 (6306), aaf1420. doi:10.1126/science.aaf1420
Domingo, J., Baeza-Centurion, P., and Lehner, B. (2019). The causes and consequences of genetic interactions (epistasis). Annu. Rev. Genomics Hum. Genet. 20 (1), 433–460. doi:10.1146/annurev-genom-083118-014857
Egervari, G., Kozlenkov, A., Dracheva, S., and Hurd, Y. L. (2018). Molecular windows into the human brain for psychiatric disorders. Mol. Psychiatry 24, 653–673. doi:10.1038/s41380-018-0125-2
Eichler, E. E., Flint, J., Gibson, G., Kong, A., Leal, S. M., Moore, J. H., et al. (2010). Missing heritability and strategies for finding the underlying causes of complex disease. Nat. Rev. Genet. 11 (6), 446–450. doi:10.1038/nrg2809
Fang, G., Wang, W., Paunic, V., Heydari, H., Costanzo, M., Liu, X., et al. (2019). Discovering genetic interactions bridging pathways in genome-wide association studies. Nat. Commun. 10 (1), 4274. doi:10.1038/s41467-019-12131-7
Hingorani, A. D., Kuan, V., Finan, C., Kruger, F. A., Gaulton, A., Chopade, S., et al. (2019). Improving the odds of drug development success through human genomics: modelling study. Sci. Rep. 9 (1), 18911. doi:10.1038/s41598-019-54849-w
Horlbeck, M. A., Xu, A., Wang, M., Bennett, N. K., Park, C. Y., Bogdanoff, D., et al. (2018). Mapping the genetic landscape of human cells. Cell 174 (4), 953–967. doi:10.1016/j.cell.2018.06.010
Jung, S.-H. (2005). Sample size for FDR-control in microarray data analysis. Bioinformatics 21 (14), 3097–3104. doi:10.1093/bioinformatics/bti456
Klopfenstein, D. V., Zhang, L., Pedersen, B. S., Ramírez, F., Warwick Vesztrocy, A., Naldi, A., et al. (2018). GOATOOLS: a Python library for Gene Ontology analyses. Sci. Rep. 8 (1), 10872. doi:10.1038/s41598-018-28948-z
Kooperberg, C., and LeBlanc, M. (2008). Increasing the power of identifying gene x gene interactions in genome-wide association studies. Genet. Epidemiol. 32 (3), 255–263. doi:10.1002/gepi.20300
Lam, M., Chen, C.-Y., Li, Z., Martin, A. R., Bryois, J., Ma, X., et al. (2019). Comparative genetic architectures of schizophrenia in East Asian and European populations. Nat. Genet. 51 (12), 1670–1678. doi:10.1038/s41588-019-0512-x
Liu, P., and Hwang, J. T. G. (2007). Quick calculation for sample size while controlling false discovery rate with application to microarray analysis. Bioinformatics 23 (6), 739–746. doi:10.1093/bioinformatics/btl664
Lizio, M., Harshbarger, J., Shimoji, H., Severin, J., Kasukawa, T., Sahin, S., et al. (2015). Gateways to the FANTOM5 promoter level mammalian expression atlas. Genome Biol. 16 (1), 22. doi:10.1186/s13059-014-0560-6
Ma, J., Yu, M. K., Fong, S., Ono, K., Sage, E., Demchak, B., et al. (2018). Using deep learning to model the hierarchical structure and function of a cell. Nat. Methods 15, 290–298. doi:10.1038/nmeth.4627
Mair, B., Moffat, J., Boone, C., and Andrews, B. J. (2019). Genetic interaction networks in cancer cells. Curr. Opin. Genet. Dev. 54, 64–72. doi:10.1016/j.gde.2019.03.002
Mani, R., Onge, R. P.St., Hartman, J. L., Giaever, G., and Roth, F. P. (2008). Defining genetic interaction. Proc. Natl. Acad. Sci. 105 (9), 3461–3466. doi:10.1073/pnas.0712255105
Marees, A. T., de Kluiver, H., Stringer, S., Vorspan, F., Curis, E., Marie-Claire, C., et al. (2018). A tutorial on conducting genome-wide association studies: quality control and statistical analysis. Int. J. Methods Psychiatric Res. 27 (2), e1608. doi:10.1002/mpr.1608
Maxwell, M. (1992). Family Interview for genetic studies (FIGS): manual for FIGS. Bethesda, MD: National Institute of Mental Health.
Niel, C., Sinoquet, C., Dina, C., and Rocheleau, G. (2015). A survey about methods dedicated to epistasis detection. Front. Genet. 6, 285. doi:10.3389/fgene.2015.00285
Noguchi, S., Arakawa, T., Fukuda, S., Furuno, M., Hasegawa, A., Hori, F., et al. (2017). FANTOM5 CAGE profiles of human and mouse samples. Sci. Data 4 (1), 170112. doi:10.1038/sdata.2017.112
Nurnberger, J. I., Blehar, M. C., Kaufmann, C. A., York-Cooler, C., Simpson, S. G., Harkavy-Friedman, J., et al. (1994). Diagnostic Interview for genetic studies: rationale, unique features, and training. Archives General Psychiatry 51 (11), 849–859. doi:10.1001/archpsyc.1994.03950110009002
Periyasamy, S., Gray, A., and Kille, P. (2009). “Multiscale adaptive dynamics from molecules to cells,” in Foundations of systems biology in engineering (Denver, Colorado, USA: Omnipress).
Periyasamy, S., Gray, A., and Kille, P. (2013). The bottom-up approach to defining life: deciphering the functional organization of biological cells via multi-objective representation of biological complexity from molecules to cells. Front. Physiology 4, 369. doi:10.3389/fphys.2013.00369
Periyasamy, S., John, S., Padmavati, R., Rajendren, P., Thirunavukkarasu, P., Gratten, J., et al. (2019). Association of schizophrenia risk with disordered niacin metabolism in an Indian genome-wide association study. JAMA Psychiatry 76 (10), 1026–1034. doi:10.1001/jamapsychiatry.2019.1335
Peterson, R. E., Kuchenbaecker, K., Walters, R. K., Chen, C.-Y., Popejoy, A. B., Periyasamy, S., et al. (2019). Genome-wide association studies in ancestrally diverse populations: opportunities, methods, pitfalls, and recommendations. Cell 179 (3), 589–603. doi:10.1016/j.cell.2019.08.051
Purcell, S., Neale, B., Todd-Brown, K., Thomas, L., Ferreira, M. A., Bender, D., et al. (2007). PLINK: a tool set for whole-genome association and population-based linkage analyses. Am. J. Hum. Genet. 81 (3), 559–575. doi:10.1086/519795
Ripke, S., Walters, J. T., and O’Donovan, M. C. (2020). Mapping genomic loci prioritises genes and implicates synaptic biology in schizophrenia. medRxiv.
Sackton, T. B., and Hartl, D. L. (2016). Genotypic context and epistasis in individuals and populations. Cell 166 (2), 279–287. doi:10.1016/j.cell.2016.06.047
Schaid, D. J., Chen, W., and Larson, N. B. (2018). From genome-wide associations to candidate causal variants by statistical fine-mapping. Nat. Rev. Genet. 19 (8), 491–504. doi:10.1038/s41576-018-0016-z
Sun, X., Lu, Q., Mukherjee, S., Crane, P. K., Elston, R., Ritchie, M. D., et al. (2014). Analysis pipeline for the epistasis search - statistical versus biological filtering. Front. Genet. 5, 106. doi:10.3389/fgene.2014.00106
Supek, F., Bošnjak, M., Škunca, N., and Šmuc, T. (2011). REVIGO summarizes and visualizes long lists of gene Ontology terms. PLOS ONE 6 (7), e21800. doi:10.1371/journal.pone.0021800
Visscher, P. M., Wray, N. R., Zhang, Q., Sklar, P., McCarthy, M. I., Brown, M. A., et al. (2017). 10 years of GWAS discovery: biology, function, and translation. Am. J. Hum. Genet. 101 (1), 5–22. doi:10.1016/j.ajhg.2017.06.005
Wan, X., Yang, C., Yang, Q., Xue, H., Fan, X., Tang, N. L. S., et al. (2010). BOOST: a fast approach to detecting gene-gene interactions in genome-wide case-control studies. Am. J. Hum. Genet. 87 (3), 325–340. doi:10.1016/j.ajhg.2010.07.021
Wei, W.-H., Hemani, G., and Haley, C. S. (2014). Detecting epistasis in human complex traits. Nat. Rev. Genet. 15, 722–733. doi:10.1038/nrg3747
Keywords: epistasis, schizophrenia, genetic interaction analysis, GWAS, hub genes, systems biology
Citation: Periyasamy S, Youssef P, John S, Thara R and Mowry BJ (2024) Genetic interactions of schizophrenia using gene-based statistical epistasis exclusively identify nervous system-related pathways and key hub genes. Front. Genet. 14:1301150. doi: 10.3389/fgene.2023.1301150
Received: 24 September 2023; Accepted: 12 December 2023;
Published: 08 January 2024.
Edited by:
Tychele Turner, Washington University in St. Louis, United StatesReviewed by:
Sheng Wang, University of California, San Francisco, United StatesCopyright © 2024 Periyasamy, Youssef, John, Thara and Mowry. This is an open-access article distributed under the terms of the Creative Commons Attribution License (CC BY). The use, distribution or reproduction in other forums is permitted, provided the original author(s) and the copyright owner(s) are credited and that the original publication in this journal is cited, in accordance with accepted academic practice. No use, distribution or reproduction is permitted which does not comply with these terms.
*Correspondence: Sathish Periyasamy, cy5wZXJpeWFzYW15QHVxLmVkdS5hdQ==
Disclaimer: All claims expressed in this article are solely those of the authors and do not necessarily represent those of their affiliated organizations, or those of the publisher, the editors and the reviewers. Any product that may be evaluated in this article or claim that may be made by its manufacturer is not guaranteed or endorsed by the publisher.
Research integrity at Frontiers
Learn more about the work of our research integrity team to safeguard the quality of each article we publish.