- 1Division of Human Genetics, Department of Pathology, University of Cape Town, Cape Town, South Africa
- 2Botswana Harvard AIDS Institute Partnership, Gaborone, Botswana
- 3Institute of Infectious Disease and Molecular Medicine, University of Cape Town, Cape Town, South Africa
- 4UCT/SAMRC Platform for Pharmacogenomics Research and Translation (PREMED) Unit, South African Medical Research Council, Cape Town, South Africa
- 5Laboratory of Genomics Diversity, Center for Computer Technologies, ITMO University, St. Petersburg, Russia
- 6Guy Harvey Oceanographic Center Halmos College of Arts and Sciences, Nova Southeastern University, Fort Lauderdale, FL, United States
- 7Department of Immunology and Infectious Diseases, Harvard T. H. Chan School of Public Health AIDS Initiative, Harvard T. H. Chan School of Public Health, Boston, MA, United States
- 8Department of Applied Sciences, Faculty of Health and Life Sciences, Northumbria University, Newcastle, United Kingdom
HIV infection continues to be a major global public health issue. The population heterogeneity in susceptibility or resistance to HIV-1 and progression upon infection is attributable to, among other factors, host genetic variation. Therefore, identifying population-specific variation and genetic modifiers of HIV infectivity can catapult the invention of effective strategies against HIV-1 in African populations. Here, we investigated whole genome sequences of 390 unrelated HIV-positive and -negative individuals from Botswana. We report 27.7 million single nucleotide variations (SNVs) in the complete genomes of Botswana nationals, of which 2.8 million were missing in public databases. Our population structure analysis revealed a largely homogenous structure in the Botswana population. Admixture analysis showed elevated components shared between the Botswana population and the Niger-Congo (65.9%), Khoe-San (32.9%), and Europeans (1.1%) ancestries in the population of Botswana. Statistical significance of the mutational burden of deleterious and loss-of-function variants per gene against a null model was estimated. The most deleterious variants were enriched in five genes: ACTRT2 (the Actin Related Protein T2), HOXD12 (homeobox D12), ABCB5 (ATP binding cassette subfamily B member 5), ATP8B4 (ATPase phospholipid transporting 8B4) and ABCC12 (ATP Binding Cassette Subfamily C Member 12). These genes are enriched in the glycolysis and gluconeogenesis (p < 2.84e-6) pathways and therefore, may contribute to the emerging field of immunometabolism in which therapy against HIV-1 infection is being evaluated. Published transcriptomic evidence supports the role of the glycolysis/gluconeogenesis pathways in the regulation of susceptibility to HIV, and that cumulative effects of genetic modifiers in glycolysis/gluconeogenesis pathways may potentially have effects on the expression and clinical variability of HIV-1. Identified genes and pathways provide novel avenues for other interventions, with the potential for informing the design of new therapeutics.
Introduction
The study of human genomes has revolutionized our understanding of human biology, population diversity, and disease susceptibility (Collins and Fink, 1995; International Human Genome Sequencing Consortium, 2004). Despite Africa being the cradle of humanity (Berger et al., 2015; Hublin et al., 2017), the vast majority of genetic studies have been conducted on people of European ancestry, while only 2% have been carried out on populations of African descent (Beltrame et al., 2016; McGuire et al., 2020; Wonkam and Adeyemo, 2023). Moreover, the human reference genome does not entirely capture variants found in African genomes (Sherman et al., 2019). This underrepresentation has led to a significant disparity in the understanding of the genetic architecture of African populations, potentially biasing our understanding of the genetic etiology of diseases and limiting therapeutic development (Sirugo et al., 2019). Studying the human genomes of Africans is imperative to confront this disparity. By studying genetic variation in African populations, we can gain insights into population history, disease susceptibility, and drug response, which ultimately will lead to better diagnoses, treatments, and a better overall healthcare system for people of African ancestry (Bentley et al., 2017; Wonkam et al., 2022).
There is a disproportionate burden of infectious diseases in Africa and HIV is one of the most prevalent in the region (Nkengasong and Tessema, 2020; Niohuru, 2023). Southern and eastern Africa carry the highest prevalence of human immunodeficiency virus (HIV) infection globally. Botswana is the third most affected country in Southern Africa, followed by eSwatini and South Africa which are in first and second positions, respectively (UNAIDS, 2019). The country is affected predominantly by HIV-1C. The HIV epidemic became severe in Botswana by the late 1990s at a prevalence of 30%–40% in pregnant women (Essex, 1999), reducing to the current 20.8% (Statistics Botswana, 2022) due to the rapid scale-up of anti-HIV drugs that has led to a sharp decline in morbidity and mortality (Farahani et al., 2014; Escudero et al., 2019; Statistics Botswana, 2022). Nonetheless, Botswana remains one of the most affected countries globally due to the high baseline HIV prevalence.
Here, we investigate genetic mutation burden and assess population genetic diversity in an HIV-1 cohort from Botswana.
Leveraging whole genome sequencing (WGS) of Botswana and existing genome databases, this work aimed to 1) unravel genetic variation and substructure within an HIV-1 cohort of Botswana, 2) assess diversity between the Botswana population and other global populations, and 3) study variation in HIV-1 specific host genes. Prioritizing variants in medical genetics entails distinguishing background benign variants from pathogenic variants that can lead to disease phenotypes (Conrad et al., 2010; Torkamani et al., 2011). Therefore, we perform in silico functional annotation using many tools and aggregate the classifications to predict pathogenicity of variants (Bope et al., 2019; Chimusa et al., 2022). Notably, our identified deleterious and loss-of-function variants are enriched in pathways associated with relevant pathophysiological mechanisms, including some that are already therapeutic targets. This study fills an important gap in knowledge by using a WGS approach focusing on deleterious variants important in HIV-1 status.
Materials and methods
Ethical approval
This study is part of a bigger protocol titled “Host Genetics of HIV-1 Subtype C Infection, Progression and Treatment in Africa/GWAS on determinants of HIV-1 Subtype C Infection” conducted by Botswana Harvard AIDS Institute Partnership. Ethics approval was obtained according to The Declaration of Helsinki. All participants provided written informed consent. Institutional Review Board (IRB) approval was obtained for these samples from Botswana Ministry of Health and Wellness—Health Research Development Committee (HRDC) & Harvard School of Public Health IRB (reference number: HPDME 13/18/1) and the University of Cape Town—Human Research Ethics Committee (HREC reference number: 316/2019).
Selection of study participants and data acquisition
Botswana population
This is a retrospective study that used samples from previous studies conducted at Botswana Harvard AIDS Institute Partnership between 2001 and 2007. Of the 390 participants, 265 were HIV-1 positive and 125 were HIV-1 negative (Supplementary Table S1A). The participants were recruited from four locations within the southern region of Botswana (Mochudi, Molepolole, Lobatse and Gaborone; Supplementary Figure S1). The HIV-1 positive participants were previously part of the Mashi study (Shapiro et al., 2006; Thior et al., 2006), while HIV-1 negative participants were previously part of the Tshedimoso study (Novitsky et al., 2008).
DNA was extracted from buffy coat using Qiagen DNA isolation kit following manufacturer’s instructions. DNA concentration was quantified using Nanodrop® 1000 (Thermo Scientific, United States). Whole genome sequences of 394 Botswana nationals were generated using paired end libraries on Illumina HiSeq 2000 sequencer at BGI (Cambridge, MA, US).
Quality assessment was performed on paired-end WGS (minimum of 30X depth) in FASTQ format (Cock et al., 2010) using FastQC (Van Der Auwera et al., 2014). Low-quality sequence bases and adapters were trimmed using Trimmomatic with default parameters (Bolger et al., 2014). The sequencing reads were aligned to the GRCh38 human reference genome using Burrows-Wheeler Aligner (BWA-MEM) (Li et al., 2008; Li and Durbin, 2009) and post-alignment quality control including adding of read groups, marking duplicates, fix mating and recalibration of base quality scores was performed using Picard tools, SAMtools (Li, 2011) and Genome Analysis Toolkit (GATK) (McKenna et al., 2010). Four samples (HIV-1 positive females) were excluded due to poor quality of sequences, the remaining dataset had 390 individuals. We have run FastQC on all final BAM files prior the variant calling, then we aggregated the results from FastQC into a single report by using MultiQC (Ewels et al., 2016). All the remaining sequences passed quality control.
We performed population joint calling (Nielsen et al., 2011; Pfeifer, 2017) using two different population joint calling methods to leverage the quality and accuracy of our results: GATK’s HaplotypeCaller (McKenna et al., 2010; DePristo et al., 2011) and BCFtools (Li, 2011). The variant call format (VCF) dataset was filtered using VCFTOOLS (Danecek et al., 2011), GATK’s Variant Quality Score Recalibration and BCFtools. The specific filtering parameters employed for both call-sets have been detailed in Supplementary Material. Downstream analyses were performed with GATK call-set and BCFtools call-set used as a validation set.
1000 genomes project and African genome variation project data
We assembled a total of 4,932 samples from 1000 Genomes Project (The 1000 Genomes Project Consortium et al., 2010; The 1000 Genomes Project Consortium et al., 2012) and the African Genome Variation Project (AGVP) (Gurdasani et al., 2015). We have detailed the integration of these data in our previous work (Chimusa et al., 2022). Based on the initial sample description (population or country labels), we used the ethnolinguistic information (Gudykunst and Schmidt, 1987; Michalopoulos, 2012) to categorize the obtained data per ethnic group and define 20 global ethnolinguistic groups as described in Supplementary Table S2. The populations are African-American, African-Caribbean, Afro-Asiatic, Afro-Asiatic Cushitic, Afro-Asiatic Omotic, Afro-Asiatic Semitic, Latin American, Khoe-San, Niger-Congo Bantu Center, Niger-Congo Bantu South, Niger-Congo Volta, Niger-Congo West, European North, European South, United States European, European Center, East Asian, South Asian, United Kingdom South Asian and United States Indian.
Assessment of population structure and admixture
We merged the 4,932 samples from 1000 Genomes Project and AGVP with our 390 Botswana samples resulting in a final total of 5,322 samples using PLINK (Purcell et al., 2007) as per our previous approach (Choudhury et al., 2017; Choudhury et al., 2020). We merged datasets based on common SNPs from autosomal chromosomes using the most-parsimonious alleles from the human genome reference (GRCh38), carried out quality control and pruning of the merged dataset (Choudhury et al., 2017; Choudhury et al., 2020; Chimusa et al., 2022). Unaligned alleles were solved by strand flipping with 1000 Genomes alleles as a reference. Variants were pruned to remove those with minor allele frequency <5%, >2% missingness, those that deviated from Hardy-Weinberg Equilibrium (HWE p > 1.0 × 10−5), and those in linkage disequilibrium (LD) r2 > 0.85 within 1000 kb window size, incrementing with 50 bases step (--indep-pairwise 1000 50 0.15). Pairwise allele sharing (identity-by-descent, IBD) was determined using pi_hat threshold of 0.2 (--genome --min 0.2). This resulted in 258,773 variants retained for assessing population diversity.
To assess structure between the population of Botswana and the 20 ethnolinguistic populations, PCA implemented in the EIGENSTRAT/smartpca programme of the EIGENSOFT package (Patterson et al., 2006; Price et al., 2006) was applied to the merged dataset. We also evaluated the extent of substructure within the Botswana population. Population structure and admixture were visualized by PCA plots generated using Genesis software (Buchmann and Hazelhurst, 2015) and R (R Core Team, 2022). The ADMIXTURE (Alexander et al., 2009) algorithm was used to estimate the ancestry proportions of the Botswana HIV-positive and -negative groups. The accurate admixture cluster was identified from model inference with lowest cross-validation (CV) error and the genome-wide admixture proportion estimations of that model inference were used as accurate genetic ancestry contribution (Supplementary Figure S2). From these, and also basing on the population history of Southern Africa (Thami and Chimusa, 2019), we chose the best 3 proxy ancestral populations that had the highest genome-wide ancestry proportions from admixture analysis: Niger-Congo, Khoe-San and European.
Genetic distance (FST) and inbreeding analysis
Pairwise genetic distance was estimated between the Botswana population and the 20 global ethnic populations using the Weir and Cockerham’s FST (Weir and Cockerham, 1984) in PLINK. A heatmap and hierarchical clustering of the genetic distances was generated using the ComplexHeatmap package (Gu et al., 2016) in R (R Core Team, 2022). We used PLINK to calculate homozygosity by keeping some of the default parameters while adjusting the window length and number of heterozygous SNVs allowed in the window (--homozyg-kb 150 and --homozyg-window-het 3). We visualized and compared the median lengths and segments of the runs of homozygosity (ROH) between the Botswana individuals and other world ethnic groups using Mann-Whitney U test using R (R Core Team, 2022) and ggplot2 (Wickham, 2016).
Variants annotation and mutation prioritization
Gene-based annotation for each population VCF file to determine whether the variants putatively cause protein coding changes was performed using ANNOVAR (Wang et al., 2010), with loss-of-function validations done through snpEFF version 4.3T (Cingolani et al., 2012). We used ANNOVAR “2016Dec18” setting, where the population frequency, pathogenicity for each variant was obtained from 1000 Genomes exome (The 1000 Genomes Project Consortium et al., 2015), Exome Aggregation Consortium (Karczewski et al., 2017) (ExAC), targeted exon datasets and COSMIC (Forbes et al., 2015). Gene functions were obtained from RefGene (O’Leary et al., 2016) and different functional predictions were obtained from ANNOVAR’s library. A total of 14 predictions that included 7 functional prediction scores (SIFT (Ng and Henikoff, 2003; Sim et al., 2012), LRT (Chun and Fay, 2009), MutationTaster (Schwarz et al., 2014), MutationAssessor (Reva et al., 2011), FATHMM (Shihab et al., 2013; Shihab et al., 2014), Polyphen2 HVAR (Adzhubei et al., 2010), Polyphen2 HDIV (Adzhubei et al., 2010)), 3 ensemble scores (RadialSVM (Kircher et al., 2014), LR (Kircher et al., 2014), CADD (Kircher et al., 2014; Rentzsch et al., 2019)), and 4 conservation scores (GERP++ (Cooper et al., 2005), PhyloP-placental (Garber et al., 2009), PhyloP-vertebrate (Garber et al., 2009) and SiPhy (Garber et al., 2009)). From each resulting functional annotated dataset, we independently filtered for predicted functional status (of which each predicted functional status is of “deleterious” (D), “probably damaging” (D), “disease_causing_automatic” (A) or “disease_causing” (D)) from SIFT, LRT, MutationTaster, MutationAssessor, FATHMM, RadialSVM, LR, CADD, GERP++, Polyphen2 HVAR, Polyphen2 HDIV, PhyloP-placental, PhyloP-vertebrate and SiPhy.
As for our previous work (Chimusa et al., 2022), we prioritized the variants by retaining a variant only if it had at least 10 predicted functional status “D” or “A” out of 14 (Wonkam et al., 2020; Chimusa et al., 2022). We classified the most deleterious variants as those that were assigned “D” by FATHMM (Shihab et al., 2013; Dong et al., 2014; Shihab et al., 2014), a disease-specific weighting scheme, which uses a Hidden Markov Models prediction algorithm capable of discriminating between disease-causing mutations and neutral polymorphisms. FATHMM has been found to have the most discriminative power among other individual in silico mutation prediction tools (Dong et al., 2014). We identified additional deleterious variants within the prioritized genes with snpEFF loss-of-function (LOF) module (Cingolani et al., 2012).
Distribution of minor allele frequency and gene-specific in SNP frequencies
The distribution of the minor allele frequency of variants within HIV-1 specific host genes across the 20 global populations (Supplementary Table S2) was investigated. To this end, the proportion of minor alleles were categorized into 6 bins (0-0.05, 0.05-0.1, 0.1-0.2, 0.2-0.3, 0.3-0.4, 0.4-0.5) with respect to each group. The minor allele frequency (MAF) per SNP for each category was computed using PLINK software (Purcell et al., 2007). Furthermore, the aggregated SNP frequency in each gene was computed considering SNPs upstream and downstream of the gene region that are in close proximity and possibly in LD (Chimusa et al., 2016; Chimusa et al., 2022). We obtained a list of 730 HIV associated genes from GWAS Catalog (www.ebi.ac.uk/gwas/), literature and gene-diseases database such DisGeNET (disgenet.org). We also leveraged the dbSNP151 database (https://www.ncbi.nlm.nih.gov/snp/ (Sherry et al., 2001)) to extract SNVs associated with these genes in the Botswana dataset (Supplementary Table S3).
Pathways enrichment analysis and gene-gene interactions
The GeneMANIA (Warde-Farley et al., 2010) tool was used to analyse how the genes harbouring the most deleterious variants (in the Botswana population) interact in a biological network. This allowed us to obtain an enrichment of related genes within the obtained sub-network with potentially affected biological pathways, processes, and molecular functions. Gene-set enrichment analysis was performed using Enrichr package (Chen et al., 2013; Kuleshov et al., 2016) in R (R Core Team, 2022).
Results
Assessment of population structure and admixture
Population structure was assessed within the population of Botswana, and between the Botswana population and other global populations using 258,773 shared bi-allelic variants. The Botswana population formed a cluster with other African populations of the Niger-Congo ethnolinguistic phylum, away from the other ethnicities (Figure 1A). In addition, the results from the pairwise genetic distance (FST) (Figure 1B) accentuates what was observed in assessment of global population structure with PCA. The heatmap and hierarchical clustering shows two distinct clusters separating into the Eurasian and African clades. A sub-clade that branches into the Niger-Congo populations and the Khoe-San population was observed. An inner sub-clade that separates Southern Bantu-speakers (including the Botswana population) from other Niger-Congo population is also observed. We also assessed the genetic relationship between the Botswana population, other Niger-Congo populations and the Khoe-San. We see in Figure 1B that the Botswana population and the Niger-Congo Bantu South (Zulu people of South Africa) formed a separate cluster from other Niger-Congo populations. The Botswana population showed a closer affinity with the Niger-Congo Bantu South population. This is expected as a close affinity of the Sotho with the Niger-Congo Bantu South has previously been reported (Choudhury et al., 2017) and our sampling sites are populated with Setswana speaking ethnic groups. These groups are members of the Sotho-Tswana clan of Southern Africa that includes the Sotho (of Lesotho and South Africa) and Batswana (of Botswana and South Africa) (Batibo, 1999; Berman, 2017). Within population substructure was not observed in the Botswana population. The plot of the first 2 PCs show a homogeneous mix of individuals from the HIV positive and the HIV negative groups with 3 outliers (Figure 3A).
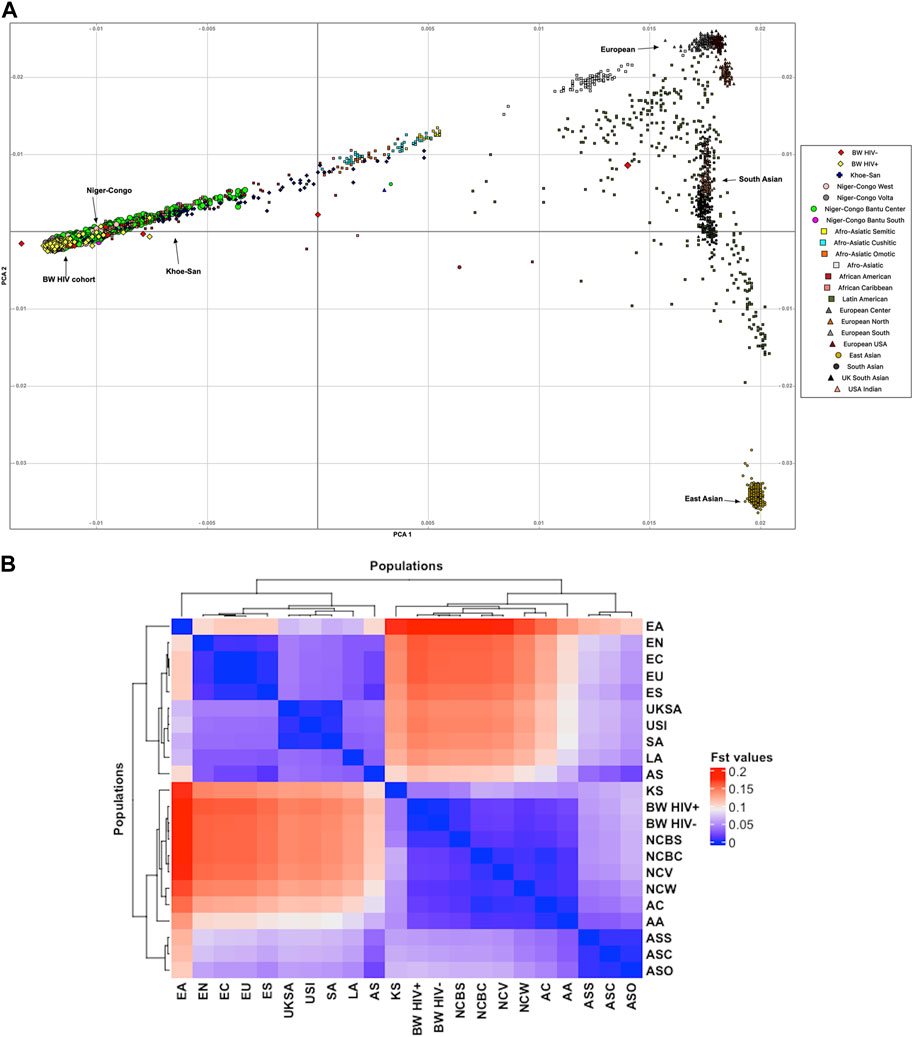
FIGURE 1. Botswana population structure in relation to the global population structure. (A) PCA showing genetic relationship between the Botswana and global populations. (B) Pairwise genetic distance between the Botswana population and 20 global ethnic groups. This is a heatmap and dendrogram of FST values showing pairwise genetic divergence between populations. The blue shade represents similarity while the red shade represents divergence between the populations. The populations are AA, African-American; AC, African-Caribbean; AS, Afro-Asiatic; ASC, Afro-Asiatic Cushitic; ASO, Afro-Asiatic Omotic; ASS, Afro-Asiatic Semitic; LA, Latin American; KS, Khoe-San; BW HIV+, Botswana HIV-1 positive; BW HIV-, Botswana HIV-1 negative; NCBC, Niger-Congo Bantu Center; NCBS, Niger-Congo Bantu South; NCV, Niger-Congo Volta; NCW, Niger-Congo West; EN, European North; ES, European South; EU, United States European; EC, European center; EA, East Asian; SA, South Asian; UKSA, United Kingdom South Asian and USI, United States Indian.
Furthermore, our results showed diversity in the runs of homozygosity (ROH) segments among African populations, and between the African populations and non-African populations (Figure 2; Supplementary Table S4). Generally, the Niger-Congo populations (including the Botswana cohort) had lower ROH lengths and less abundant ROH segments than the European, Asian, Indian, Latin-American and Khoe-San populations (Figure 2). For instance, we observe a lower median ROH length (p-value <2.2 × 10−16) in Niger-Congo (35.446 Mb; IQR: 27.704, 43.267) populations than in the European populations (121.306 Mb; IQR: 109.443, 138.028).
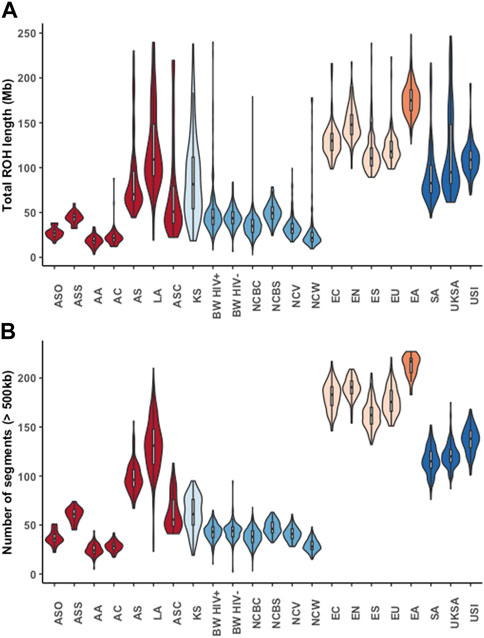
FIGURE 2. The lengths and number of runs of homozygosity (ROH) segments across different global ethnic groups. Violin plots showing the median lengths (in Mb) and number of ROH segments. The colours represent different super-groups: Mixed populations (African-American (AA), African-Caribbean (AC), Afro-Asiatic (AS), Afro-Asiatic Cushitic (ASC), Afro-Asiatic Omotic (ASO), Afro-Asiatic Semitic (ASS) and Latin American (LA)) in dark-red, Khoe-San (KS) in light blue, Niger-Congo (Botswana HIV-1 positive (BW HIV+), Botswana HIV-1 negative (BW HIV-), Niger-Congo Bantu Center (NCBC), Niger-Congo Bantu South (NCBS), Niger-Congo Volta (NCV) and Niger-Congo West (NCW)) in blue, Europeans [European North (EN), European South (ES), United States European (EU), European center (EC)] in light orange, East Asian (EA) in orange and South Asians [South Asian, (SA), United Kingdom South Asian (UKSA) and United States Indian (USI)] in orange.
Given the results in Figure 1A, we performed admixture analysis to estimate the individual fraction of genetic ancestry. The optimal admixture model (see Materials and Methods) was the one that showed stability (K = 3) in estimation of ancestry proportions and with the lowest cross-validation (CV) value (Figure 3B). This estimated number K = 3 is consistent with the number of source populations from literature that contributed to the admixture of East and Southern African populations (Tishkoff et al., 2009; Pickrell et al., 2012; Chimusa et al., 2013a; Pickrell et al., 2014; Gurdasani et al., 2015; Busby et al., 2016; Choudhury et al., 2017; Retshabile et al., 2018; Choudhury et al., 2020). The Botswana population assessed in this study shows elevated components shared with the following ancestry proportions: Niger-Congo (65.9%), Khoe-San (32.9%) and Eurasian (1.1%) (Figure 3B).
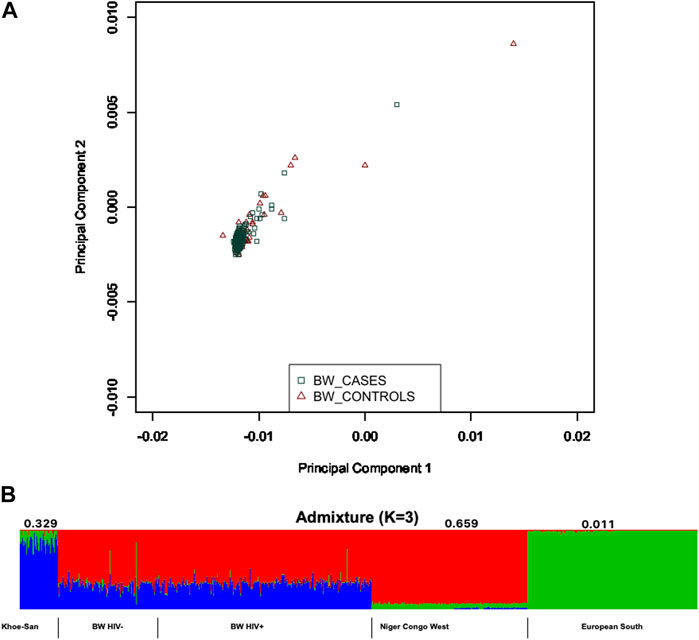
FIGURE 3. Within population diversity of the Botswana population. (A) A depiction of population substructure of Botswana from PCA showing genetic relationship between HIV positive in green and HIV negative in brown. (B) Genome-wide admixture proportions of Botswana. Khoe-San, Niger-Congo and European populations were used as proxy ancestral populations that may have potentially contributed to the genetic architecture of Botswana.
Characterization of variants and variants effect in the Botswana population
We provide a broad survey of polymorphisms in whole genome sequences of 390 unrelated HIV positive and negative individuals from Botswana. A total of 265 HIV-1 positive and 125 HIV-1 negative individuals passed WGS analysis quality control. The demographics of the study population are presented in Supplementary Table S1A (Materials and Methods). We identified 27.7 million variants from the 390 individuals of Botswana. Of these 27.7 million variations, we found 25.1 million SNVs and 2.6 million indels (Supplementary Table S1B); 2,789,599 (10.08%) of these variations were novel, i.e., not found in dbSNP151, 1000 Genomes Project (1KGP), African Genome Variation Project (AGVP) and Genome Aggregation Database (gnomAD) (Karczewski et al., 2020) (Figure 4A). The average transition-transversion (Ti/Tv) ratio was 2.1, which is within the expected Ti/Tv ratio for whole genomes.
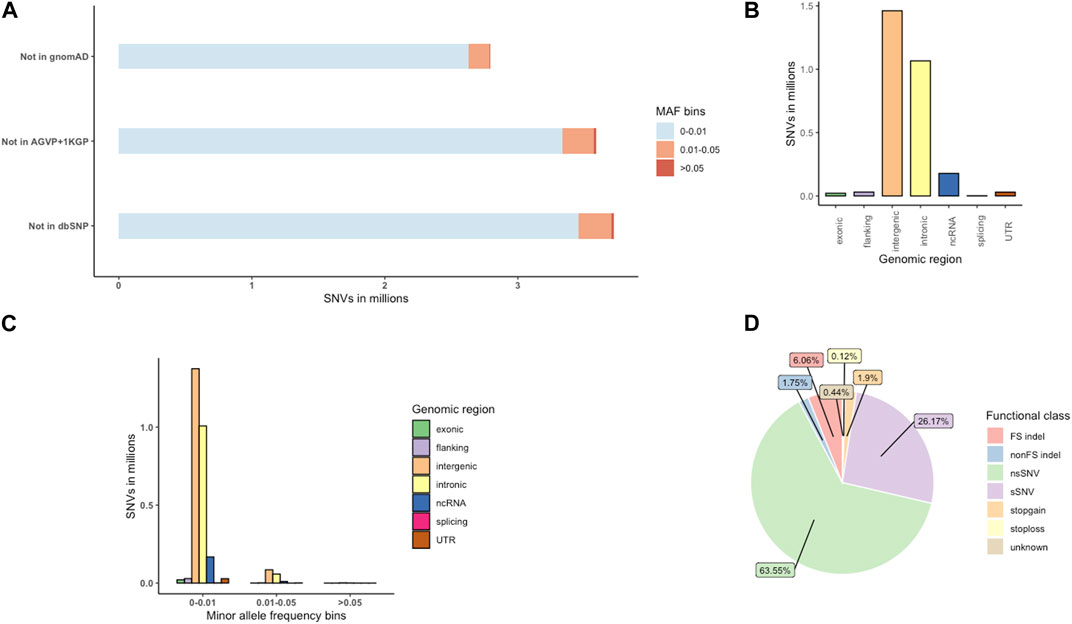
FIGURE 4. The distribution of novel variants in the Botswana population genomes. (A) Novel variants, absent from dbSNP151, the African Genome Variation Project (AGVP), the 1000 Genomes Project (1KGP) and gnomAD. (B) Genome-wide distribution of novel variant effects by functional elements. (C) Distribution of novel functional elements across MAF bins (D) Distribution of exonic variants by functional elements. FS, frameshift; sSNV (synonymous SNVs); nsSNV (non-synonymous SNVs).
The novel variants were classified into which genomic position the variants occur, and what consequence they have on the transcript or encode gene. Positional annotations show whether the variant overlaps the following regions: coding (exonic), intron (intronic), intergenic region, 1-kb region upstream or downstream of transcription start site (upstream, downstream), a transcript without coding annotation (ncRNA), 5′untranslated region or 3′untranslated regions (5′UTR,3′UTR). Exonic variants are further classified into functional consequences: synonymous (does not cause an amino-acid change), nonsynonymous (causes an amino-acid change), frameshift (changes the open reading frame of the coding sequence), stopgain (introduces a stop codon at the variant site), stoploss (removes a stop codon at the variant site) and variants of unknown function (Wang et al., 2010; Wang, 2023).
Intergenic variants were observed at the highest frequency (1,461,193; 5.28%), followed by intronic (1,066,166; 3.85%) and ncRNA (178,178; 0.64%) variants (Figure 4B; Supplementary Table S1B). Most of the novel (2,786,546; 99.89%) variants were singletons, rare (MAF <0.01) and low frequency variants (MAF 0.01-0.05) (Supplementary Table S5; Figures 4A, C). Potentially protein altering variants (nonsynonymous SNVs (nsSNV), stop gain, stop loss variants, frameshift (FS indel) and non-frameshift (nonFS) indels), synonymous (sSNV) and variants of unknown consequence formed 73.39% (15,899), 26.17% (5,670) and 0.44% (Chan et al., 2019) of the exonic variants respectively (Figure 4D; Supplementary Table S1B).
Variant prioritization and prediction of mutation burden
Potentially pathogenic SNVs were identified by selecting variant predictions of deleteriousness (Supplementary Table S6) from at least 10 out of 14 predictive tools using ANNOVAR (Wang et al., 2010) (Materials and Methods). We identified deleterious variants in a list of 24 genes that are all known HIV-1 specific genes (TTLL10, ACTRT2, ENO1, CYP4A22, PM20D1, HOXD12, DNAH7, PDHA2, LRBA, DCHS2, VCAN, ADGRV1, MRPS18A, ABCB5, AKR1B10, ADAM7, OR51A4, OR2D2, KRT76, OR6S1, ATP8B4, ABCC12, OR3A1, PHF20) (Supplementary Table S6). We trimmed this list of these genes by further classifying variants as “damaging” by FATHHM (Shihab et al., 2013; Shihab et al., 2014). This resulted in 5 most deleterious mutations within the ACTRT2, HOXD12, ABCB5, ATP8B4 and ABCC12 genes (Table 1).
Distribution of MAF in known HIV-1 specific host genes
We used variants extracted from the prioritized list of the 24 genes that harboured deleterious variants. We observed variation in the distribution of MAF at rare and common variants between Botswana HIV-positive and -negative group, and the rest of 20 ethnolinguistic groups, except Niger-Congo Bantu that has similar pattern of the distribution of MAF with Botswana HIV -positive and -negative group (Figure 5A). Botswana HIV-1 cohort and Niger-Congo populations have low proportion of rare and low frequency variants (between 0% and 5%) and relatively high proportion of common variants (greater than 5%–30%). This is not surprising as African population have the highest genetic diversity. The results might imply that the multiple common variants affect genetic predisposition or resistance to HIV-1 among Africans (Thami and Chimusa, 2019). We observe variation in the aggregated SNP frequency in known HIV-1 specific host genes between African ethnolinguistic groups and those out of Africa (See Materials and Methods). Importantly, variation in the aggregated SNP frequency is observed in the CYP4A22, AKR1B10, HOXD12, OR6S1, MRPS18A, ORS1A4 and ACTRT2 genes (Figure 5B; Supplementary Table S8) between Botswana HIV-positive and -negative population, suggesting that these genes may harbour differing effects among Botswana HIV-positive and -negative population.
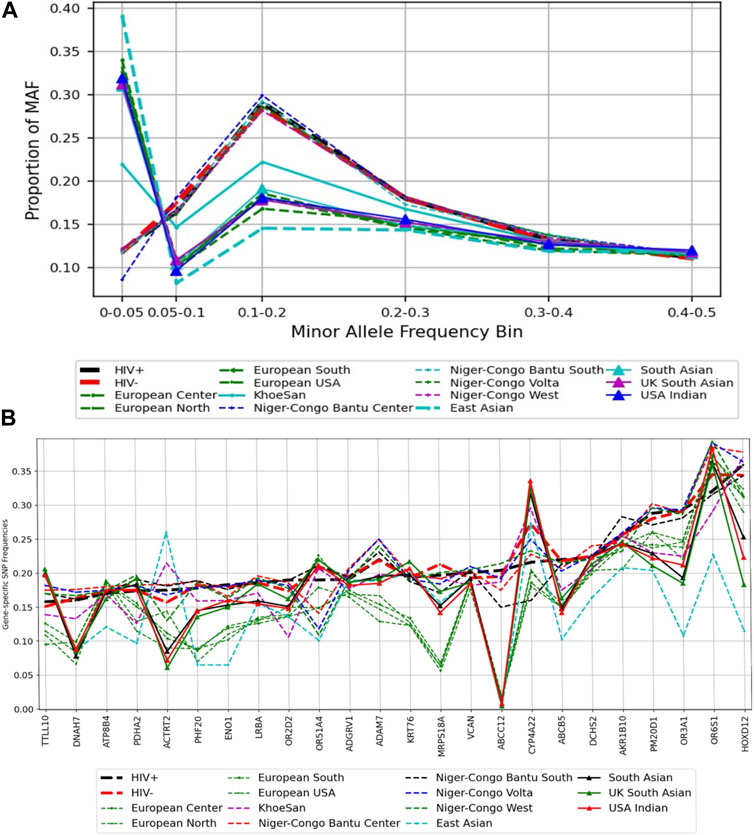
FIGURE 5. A comparison of minor alleles frequencies across global populations from known HIV-1 associated host genes. (A) Distribution of variants across MAF bins in global populations. (B) Gene-specific SNPs Frequencies: the distribution of the minor allele frequency at the gene level.
Pathways enrichment analysis and gene-gene interactions in the Botswana population
The 24 genes (Table 1; Supplementary Tables S6, S7) harbouring potentially pathogenic variants were subjected to enrichment analysis using GeneMANIA (Warde-Farley et al., 2010) and Enrichr (Kuleshov et al., 2016) bioinformatics tools to identify biological processes and pathways putatively perturbed (Figure 3; Table 2). To successfully enrich for biological processes and pathways, the identified genes were used to find 20 more related genes that are co-expressed and predicted to physically interact with the identified genes (Figure 6).
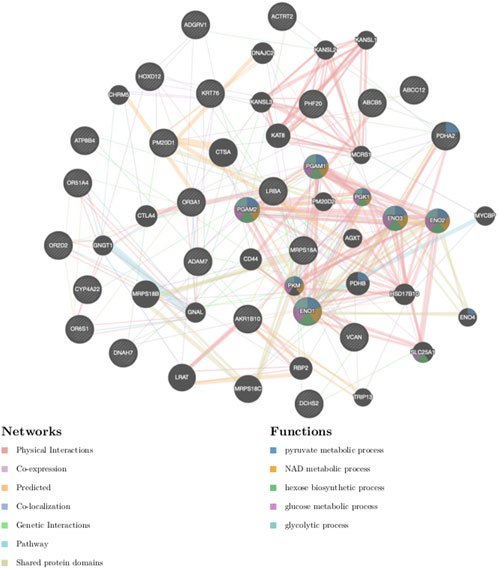
FIGURE 6. Gene-gene interaction network of genes harbouring the most deleterious variants. The different colours of branches represent how the genes are related; pink: physical interactions, purple: co-expression, orange: predicted, navy blue: co-localization, blue: pathway, green: genetic interactions, yellow: shared protein domains. Black and stripped nodes: genes provided as input into GeneMANIA. Black only nodes: genes predicted by GeneMANIA to interact with the input list. Connecting lines represent interactions.
The products of the identified genes were predicted to perform the following biological processes: gluconeogenesis, hexose and acyl-COA biosynthesis (Table 2). These gene products are localized within the oxoglutarate dehydrogenase complex and the mitochondria. The predicted molecular functions of these gene products were catalysis of peptidase, hydro-lyase, alcohol dehydrogenase and ATPase activities. The identified genes were found to be associated with Pyruvate dehydrogenase complex deficiency (PDCD). One of the identified genes, tumor susceptibility 101 (TSG101), was also found to be associated with human immunodeficiency virus 1 (HIV-1), albeit not statistically significant (Table 2). The affected pathways (Table 2) included the glycolysis and gluconeogenesis (p < 2.84e-6), Citrate cycle (TCA cycle) (p < 5.57e-9), HIF-1 signalling pathway (p < 2.08e-9), Hereditary leiomyomatosis and renal cell carcinoma pathways (p < 1.10e-5). Importantly, published transcriptomic evidence (Akusjärvi et al., 2022) provides functional support for the role of the identified pathways, including the Glycolysis, Gluconeogenesis and HIF-1 signalling pathways, regulating susceptibility to HIV-1 infection. This suggests that some of the identified mutant genes may act together in these biological pathways to have a cumulative effect on HIV-1 expression.
Discussion
Our study assessed genetic diversity and mutation bias in an HIV-1 cohort from Botswana using a whole-genome sequencing approach. We also studied diversity between the Botswana population and 20 global ethnolinguistic groups. The PCA plots revealed that the Botswana HIV-positive and -negative population is overall largely homogenous (Figure 1A). Although the Botswana HIV-1 positives and negatives almost completely overlap, there is a considerable spread in the data points (Figure 1A; Figure 3A). The spread is even more than that of European samples combined, this signifying a higher genetic diversity in the Botswana study population and African populations generally. The study participants were recruited from three districts in the southern part (Southern, Kweneng and South-East) of Botswana. Although the sampling sites do not span the whole of Botswana, the current study helps us to better understand genetic variation in the southern part of Botswana. Effective sampling that includes populations from different regions is needed to capture all the variation in the Botswana population and for better generalizability of findings.
Major events such as the “Bantu expansion” and Eurasian migration into Southern Africa have shaped the genetic landscape of the region. These events have led to varying degrees of admixture of the migrant groups and indigenous population (Tishkoff et al., 2009; Chimusa et al., 2013b; Petersen et al., 2013; Pickrell et al., 2014; Gurdasani et al., 2015; Choudhury et al., 2017; Montinaro et al., 2017; Thami and Chimusa, 2019). These previous findings are congruent with the current study that reports elevated components shared with Niger-Congo (65.9%), Khoe-San (32.9%) and European (1.1%) populations (Figure 3B).
We found no evidence of consanguinity in the Botswana HIV-1 cohort as defined by less abundant segments and lower lengths of ROH in comparison to non-African populations and the Khoe-San (Figure 2). This finding is supported by the previous observation of no extended ROH lengths in a Botswana HIV positive cohort (Retshabile et al., 2018). Among the Niger-Congo populations, the median ROH length in the Botswana HIV-1 cohort and the Niger-Congo Bantu South were significantly higher (p = 2.2e-16) than of the Niger-Congo Bantu, Niger-Congo West and the Niger-Congo Volta (Figure 2). These results are consistent with what was observed by Choudhury et. al., who observed that the Niger-Congo Bantu population of Southern Africa had the highest lengths of ROH compared to Niger-Congo populations of East, Central West and West Africa (Choudhury et al., 2017). Overall, the results of Figure 2 provide an interesting consequence and affirmation of the Out of Africa (OoA) founder event. The dispersal of a smaller group from Africa into Eurasian regions made decreased genetic variation and inbreeding likely, hence the longer runs of identical haplotypes in Eurasian populations.
It was not surprising to observe a considerable proportion of the Khoe-San ancestry as Botswana is one of the countries with the largest number of the Khoe-San. The Khoe-San are known to be the indigenous people of Southern Africa (Schlebusch et al., 2020). Moreover a recent study postulated that modern humans come from a Khoe-San woman who inhabited the prehistoric wetlands (Makgadikgadi-Okavango) in the northern part of Botswana (Chan et al., 2019). Although there is controversy around this recent finding, the Chan et al. study may support our previous hypothesis of that the Northern San originated in Botswana as evidenced by the rock paintings at Tsodilo Hills in North-western Botswana (Thami and Chimusa, 2019). Overtime the Khoe-San are expected to have mingled and interbred with the Niger-Congo people of Botswana. Hence, by showing shared genetic components with the Khoe-San, this work shows the pivotal role played by genetics in the reconstruction of population histories. A limitation of this study is that the Botswana population had no ethnolinguistic meta-data, as such ethnicity inferences cannot be drawn from this study. Nevertheless, population structure could still be assessed as the PCA is an unsupervised machine learning method and therefore can still give meaningful results.
For the analysis of Botswana samples only, we identified 27.7 million variants from 30X depth whole genomes of 390 individuals of Botswana. A critical and convenient QC metric to measure the quality and accuracy of genomic variation data is the Ti/Tv ratio (DePristo et al., 2011). The average Ti/Tv ratio of this set of variants was 2.1. This Ti/Tv ratio is within the expected range for human whole genome data which is ∼2.0–2.1, meaning that the data has a very low frequency of false positive variant sites (DePristo et al., 2011; Carson et al., 2014). As observed previously (Choudhury et al., 2017), intergenic variants had the highest frequency, followed by intronic variants and non-coding RNA (ncRNA) variants. Ten percent (2,789,599) of the discovered SNVs were novel. This number of previously uncaptured genetic variation highlights a potential of identifying population-specific variations through WGS. WGS also offers an opportunity to identify intronic variants and variants within non-coding regions. To this effect 1,066,166 intronic and 178,178 (ncRNA) novel variants were identified.
Recent human population expansion has resulted in a skewness towards excessive rare variants. This means that rare variants constitute a large part of the human genomic variations (Nagasaki et al., 2015; Keinan and Clark, 2012; Johnston et al., 2015; Hernandez et al., 2019; Epi25 Collaborative, 2019). Hence it is not surprising that 2,786,546 (99.89%) of the novel variants identified in the current study were very rare (Supplementary Table S5; Figures 4A, C). According to sequence ontology classifications, 73.39% of the exonic variants were potentially protein altering (Figure 4D; Supplementary Table S1B). Protein altering variants cause a change in the amino acid leading to a change in the protein sequence, an abnormal truncation or elongation of the protein, all leading to a change in the conformation and function of the encoded protein (Pagel et al., 2017). These nonsynonymous mutations have a potential to disturb normal biological processes and cause disease.
The product of ACTRT2 gene may be involved cytoskeletal organization (GeneCards, 2020). The rs3795263 variant was previously identified as harmful and associated with a severe form of tick-borne encephalitis virus infection (Ignatieva et al., 2019). The HOXD12 gene belongs to the homeobox (HOX) family of genes that encode transcription factors involved in regulation of embryonic development (Lappin et al., 2006; GeneCards, 2020). The exact role of HOXD12 is unknown (GeneCards, 2020). The HOX genes have been implicated in maintenance and control of HIV-1 latency through epigenetic regulation (Khan et al., 2018).
The ABCB5 gene belongs to the ATP-binding cassette (ABC) family that encodes proteins responsible for transmembrane transport of molecules including drugs such as doxorubicin (GeneCards, 2020). ABCB5 is thought to also mediate chemoresistance of doxorubicin in malignant melanoma, (Whirl-Carrillo et al., 2012). The ATP8B4 gene encodes an ATPase protein that is responsible for phospholipid translocation in the cell membrane (GeneCards, 2020). The ABCC12 gene also encodes an ABC protein responsible for transmembrane transport of molecules. Some members of the ABC family regulate the efflux of HIV-1 antiretrovirals from intracellular compartments (Eilers et al., 2008; Salvaggio et al., 2017). Biological pathways potentially affected by the products of these putatively deleterious genes and their interactome are discussed in subsequent paragraphs.
The minor allele frequencies of the HOXD12 rs200302685, ABCB5 rs111647033, ATP8B4 rs77004004 and ABCC12 rs113496237 variants in the Botswana data were generally higher when comparing to the gnomAD and the 1000 Genomes Project data. While the MAF for the ACTRT2 rs3795263 variant was lower than in the gnomAD and the 1000 Genomes Project data. This highlights that MAFs do vary per ethnicity which could affect the risk of disease differently between populations (Table 1).
The pyruvate dehydrogenase (PDH), enolase (ENO) and aldo-keto reductase (AKR1) genes (Figure 6; Table 2) were significantly associated with glycolysis and gluconeogenesis (1.34e-3). Both glycolysis and gluconeogenesis are glucose metabolism pathways; glycolysis is the catabolism of glucose (or glycogen) into pyruvate, while gluconeogenesis is the anabolism of pyruvate (from mainly proteins) into glucose (Yang and Brunengraber, 2000; Bonora et al., 2012). Identification of ACTRT2, HOXD12, ABCB5, ATP8B4, ABCC12 genes (Table 1) involved in Krebs cycle, renal carcinoma, Hypoxia-inducible factor 1 (HIF-1) signalling, RNA degradation, Histidine metabolism and folate biosynthesis pathways (Figure 6) requires further study to explore their roles in modifying the HIV-1 phenotype.
Pyruvate produced from glycolysis is a substrate for TCA where acetyl-CoA, a precursor of cholesterol, is produced (Berg et al., 2002). Cholesterol is required for plasma membrane formation and integrity. Furthermore cholesterol is required for viral fusion to the host’s cell membrane for entry and virus release following assembly and maturation (egress) (Coomer et al., 2020). Oxidation of cholesterol to 25-hydroxycholesterol can block HIV-1 cell entry (Liu et al., 2013). In addition, HIV-1 infection upregulates glycolysis to meet the demands of viral replication and CD4+ T-cells with higher glycolysis rate are more susceptible to HIV-1 infection (Hegedus et al., 2014; Palmer et al., 2016; Valle-Casuso et al., 2019). Our results are timely and can contribute to the emerging field of immunometabolism in which therapy against HIV-1 infection is being evaluated through reduction of glycolysis and inhibition of cholesterol (Liu et al., 2013; Valle-Casuso et al., 2019; Taylor and Palmer, 2020; Shytaj et al., 2021).
The association of AKR1 genes (Figure 6) with alcohol dehydrogenase (NADP+) activity and folate biosynthesis (Table 2) could be explained by that the alcohol dehydrogenases catalyse the reduction of NADP + to NADPH (Penning, 2015). This reaction also takes place within glycolysis, gluconeogenesis and pentose phosphate pathways (Berg et al., 2002; Bonora et al., 2012). Furthermore, there is also evidence of NADPH being produced from folate metabolism (Fan et al., 2014). The human polynucleotide phosphorylase (hPNPaseold-35) is an evolutionary conserved RNA-degradation enzyme that has homologues in organisms such as Escherichia coli and yeast (Leszczyniecka et al., 2002; Das et al., 2011). In E. coli PNPase forms part of the degradosome with enolase and a helicase (Wilusz and Wilusz, 2008). This link between enolase and the evolutionary conserved PNPase may explain the association of the ENO genes with RNA degradation (Table 2). The degradation of HIV-1 mRNA in HIV-1 infected cells is important in suppressing HIV-1 replication (Hillebrand et al., 2019). Moreover, ENO-1 has been shown to prevent HIV-1 reverse transcription and ultimately decrease HIV-1 infectivity (Kishimoto et al., 2017), suggesting its translation into clinical practice. To the best of our knowledge, this is the largest study to use deep sequencing in efforts to delineate a complete genome map of the human population of Botswana and evaluate the burden of human genomic mutations in Botswana. To this effect we identified single nucleotide variants which could potentially disrupt the function of 24 genes, the most deleterious (damaging) variants being ACTRT2 rs3795263, HOXD12 rs200302685, ABCB5 rs111647033, ATP8B4 rs77004004 and ABCC12 rs113496237 (Table 1). These variants had never been identified to be associated with HIV-1. The strength of the study is the use of several different but complementary analytical approaches to identify novel variants that are potential modifiers of HIV.
Rare and low-frequency variants constituted the bulk of novel variants that were identified in this study. This was made possible by the unique potential of deep sequencing that offers an opportunity to discover rare variants. This is important because unlike Mendelian conditions, complex traits are influenced by many small-effect variants from different genetic loci, a concept known as polygenicity (Visscher et al., 2012). The cumulative effect of rare variants plays an important role in the expression of complex traits such as HIV-1. Glycolysis, Gluconeogenesis and HIF-1 signaling, TCA and hexo-pentose pathways emerged to be the most affected by the putatively deleterious variants. These are critical physiological pathways responsible for energy production, amino-acid biosynthesis, immunity, and tumorigenesis among other roles.
Nonetheless, the study has some limitations. However, it should be noted that our focus is on deleterious variants and variation among HIV-1 positive and negative individuals and not on the genetic susceptibility or association studies of HIV-1 in Botswana as previously reported (Xie et al., 2017; Shevchenko et al., 2021). Also, the sample size is relatively modest and larger sample sizes would probably yield more findings. Nonetheless, the total number of whole genomes sequenced in the study represents one of the largest cohorts in Africa to date.
In summary, we reported a WGS study as part of ongoing and recent study (Shevchenko et al., 2021) on clinical phenotypes of HIV-1 in Africa. Notably, we generated a catalogue of candidate modifier genes and their associated pathways that clustered in pathophysiological pathways important in HIV-1 and with implications for therapeutic intervention. This study fills a critical gap in knowledge by using a WGS approach focusing on deleterious variants identified in HIV-1 positive and negative individuals, in contrast to most other studies that used a GWAS approach. This study thus makes significant contributions to present knowledge of the natural history and clinical heterogeneity in HIV-1 populations, with the potential for informing the design of new therapeutics.
Data availability statement
The WGS data used in this study is available through requests at the Botswana Harvard AIDS Institute Partnership, Institutional Data Access/Ethics Committee (info@bhp.org.bw, Ref. HREC 316/2019). All metadata, scripts, software information including additional resources used in the analyses and/or analysed to produce the results during the current study are all available in the GitHub project https://github.com/pthami07/botswana-hiv-host-genes.
Ethics statement
The studies involving humans were approved by the Institutional Review Board (IRB) from the Botswana Ministry of Health and Wellness—Health Research Development Committee (HRDC) and Harvard School of Public Health IRB (reference number: HPDME 13/18/1) and the University of Cape Town—Human Research Ethics Committee (HREC reference number: 316/2019). The studies were conducted in accordance with the local legislation and institutional requirements. The participants provided their written informed consent to participate in this study.
Author contributions
PT: Conceptualization, Data curation, Formal Analysis, Funding acquisition, Investigation, Methodology, Validation, Visualization, Writing–original draft, Writing–review and editing, Project administration. WC: Writing–review and editing, Data curation. CD: Writing–review and editing. SO’B: Resources, Writing–review and editing. ME: Resources, Writing–review and editing. SG: Funding acquisition, Resources, Supervision, Writing–review and editing. EC: Funding acquisition, Resources, Supervision, Writing–review and editing, Conceptualization, Methodology, Validation, Visualization.
Funding
The author(s) declare financial support was received for the research, authorship, and/or publication of this article. We are grateful to the sub-Saharan African Network for TB/HIV Research Excellence (SANTHE), a DELTAS Africa Initiative (grant # DEL-15-00) for funding this work. The DELTAS Africa Initiative is an independent funding scheme of the African Academy of Sciences (AAS)’s Alliance for Accelerating Excellence in Science in Africa (AESA) and supported by the New Partnership for Africa’s Development Planning and Coordinating Agency (NEPAD Agency) with funding from the Wellcome Trust (grant # 107752/Z/15/Z) and the UK government. This work was also supported through the Academy of Medical Sciences Professorship (Grant # APR9\1024). The views expressed in this publication are those of the authors and not necessarily those of AAS, NEPAD Agency, Wellcome Trust, or the UK government.
Acknowledgments
We thank the participants, investigators, and key personnel of the “Host Genetics of HIV-1C Infection, Progression, and Treatment in Africa/GWAS on Determinants of HIV-1C Infection” study at Botswana Harvard AIDS Institute Partnership. All computational analysis was performed through the Centre of High-Performance Computing clusters (Cape Town, South Africa).
Conflict of interest
The authors declare that the research was conducted in the absence of any commercial or financial relationships that could be construed as a potential conflict of interest.
Publisher’s note
All claims expressed in this article are solely those of the authors and do not necessarily represent those of their affiliated organizations, or those of the publisher, the editors and the reviewers. Any product that may be evaluated in this article, or claim that may be made by its manufacturer, is not guaranteed or endorsed by the publisher.
Supplementary material
The Supplementary Material for this article can be found online at: https://www.frontiersin.org/articles/10.3389/fgene.2023.1290624/full#supplementary-material
References
Adzhubei, I. A., Schmidt, S., Peshkin, L., Ramensky, V. E., Gerasimova, A., Bork, P., et al. (2010). A method and server for predicting damaging missense mutations. Nat. Methods 7 (4), 248–249. doi:10.1038/nmeth0410-248
Akusjärvi, S. S., Ambikan, A. T., Krishnan, S., Gupta, S., Sperk, M., Végvári, A., et al. (2022). Integrative proteo-transcriptomic and immunophenotyping signatures of HIV-1 elite control phenotype: a cross-talk between glycolysis and HIF signaling. iScience 25 (1), 103607. doi:10.1016/j.isci.2021.103607
Alexander, D. H., Novembre, J., and Lange, K. (2009). Fast model-based estimation of ancestry in unrelated individuals. Genome Res. 19 (9), 1655–1664. doi:10.1101/gr.094052.109
Batibo, H. M. (1999). A lexicostatistical survey of the Setswana dialects spoken in Botswana. South Afr. J. Afr. Lang. 19 (1), 2–11. doi:10.1080/02572117.1999.10587376
Beltrame, M. H., Rubel, M. A., and Tishkoff, S. A. (2016). Inferences of African evolutionary history from genomic data. Curr. Opin. Genet. Dev. 41, 159–166. doi:10.1016/j.gde.2016.10.002
Bentley, A. R., Callier, S., and Rotimi, C. N. (2017). Diversity and inclusion in genomic research: why the uneven progress? J. Community Genet. 8 (4), 255–266. doi:10.1007/s12687-017-0316-6
Berg, J., Tymoczko, J., and Stryer, L. (2002a). “Amino acids are made from intermediates of the citric acid cycle and other major pathways,” in Biochemistry (New York: W. H. Freeman). Available from: https://www.ncbi.nlm.nih.gov/books/NBK22459/.
Berg, J. M., Tymoczko, J. L., and Stryer, L. (2002b). Cholesterol is synthesized from acetyl coenzyme A in three stages. Biochemistry.
Berger, L. R., Hawks, J., de Ruiter, D. J., Churchill, S. E., Schmid, P., Delezene, L. K., et al. (2015). Homo naledi, a new species of the genus Homo from the Dinaledi Chamber, South Africa. Elife 4, e09560. doi:10.7554/eLife.09560
Berman, S. K. (2017). A Bible translation inspired look at the history and ethnography of the Batswana. Skriflig 51, 1–6. doi:10.4102/ids.v51i1.2153
Bolger, A. M., Lohse, M., and Usadel, B. (2014). Trimmomatic: a flexible trimmer for Illumina sequence data. Bioinformatics 30 (15), 2114–2120. doi:10.1093/bioinformatics/btu170
Bonora, M., Patergnani, S., Rimessi, A., De Marchi, E., Suski, J. M., Bononi, A., et al. (2012). ATP synthesis and storage. Purinergic Signal 8 (3), 343–357. doi:10.1007/s11302-012-9305-8
Bope, C. D., Chimusa, E. R., Nembaware, V., Mazandu, G. K., de Vries, J., and Wonkam, A. (2019). Dissecting in silico mutation prediction of variants in african genomes: challenges and perspectives. Front. Genet. 10, 601. doi:10.3389/fgene.2019.00601
Buchmann, R., and Hazelhurst, S. (2015). The ‘Genesis’ manual. Available from: http://www.bioinf.wits.ac.za/software/genesis/Genesis.pdf (Accessed November 28, 2018).
Busby, G. B., Band, G., Si Le, Q., Jallow, M., Bougama, E., Mangano, V. D., et al. (2016). Admixture into and within sub-saharan Africa. Elife 5, e15266. doi:10.7554/eLife.15266
Carson, A. R., Smith, E. N., Matsui, H., Brækkan, S. K., Jepsen, K., Hansen, J.-B., et al. (2014). Effective filtering strategies to improve data quality from population-based whole exome sequencing studies. BMC Bioinforma. 15 (1), 125. doi:10.1186/1471-2105-15-125
Chan, E. K. F., Timmermann, A., Baldi, B. F., Moore, A. E., Lyons, R. J., Lee, S.-S., et al. (2019). Human origins in a southern African palaeo-wetland and first migrations. Nature 575 (7781), 185–189. doi:10.1038/s41586-019-1714-1
Chatr-aryamontri, A., Ceol, A., Peluso, D., Nardozza, A., Panni, S., Sacco, F., et al. (2009). VirusMINT: a viral protein interaction database. Nucleic Acids Res. 37 (Database issue), D669–D673. doi:10.1093/nar/gkn739
Chen, E. Y., Tan, C. M., Kou, Y., Duan, Q., Wang, Z., Meirelles, G. V., et al. (2013). Enrichr: interactive and collaborative HTML5 gene list enrichment analysis tool. BMC Bioinforma. 14 (1), 128. doi:10.1186/1471-2105-14-128
Chimusa, E. R., Alosaimi, S., and Bope, C. D. (2022). Dissecting generalizability and actionability of disease-associated genes from 20 worldwide ethnolinguistic cultural groups. Front. Genet. 13, 835713. doi:10.3389/fgene.2022.835713
Chimusa, E. R., Daya, M., Moller, M., Ramesar, R., Henn, B. M., van Helden, P. D., et al. (2013b). Determining ancestry proportions in complex admixture scenarios in South Africa using a novel proxy ancestry selection method. PLoS One 8 (9), e73971. doi:10.1371/journal.pone.0073971
Chimusa, E. R., Mbiyavanga, M., Mazandu, G. K., and Mulder, N. J. (2016). ancGWAS: a post genome-wide association study method for interaction, pathway and ancestry analysis in homogeneous and admixed populations. Bioinformatics 32 (4), 549–556. doi:10.1093/bioinformatics/btv619
Chimusa, E. R., Zaitlen, N., Daya, M., Moller, M., van Helden, P. D., Mulder, N. J., et al. (2013a). Genome-wide association study of ancestry-specific TB risk in the South African Coloured population. Hum. Mol. Genet. 23 (3), 796–809. doi:10.1093/hmg/ddt462
Choudhury, A., Aron, S., Botigué, L. R., Sengupta, D., Botha, G., Bensellak, T., et al. (2020). High-depth African genomes inform human migration and health. Nature 586 (7831), 741–748. doi:10.1038/s41586-020-2859-7
Choudhury, A., Ramsay, M., Hazelhurst, S., Aron, S., Bardien, S., Botha, G., et al. (2017). Whole-genome sequencing for an enhanced understanding of genetic variation among South Africans. Nat. Commun. 8 (1), 2062. doi:10.1038/s41467-017-00663-9
Chun, S., and Fay, J. C. (2009). Identification of deleterious mutations within three human genomes. Genome Res. 19 (9), 1553–1561. doi:10.1101/gr.092619.109
Cingolani, P., Platts, A., Wang, L. L., Coon, M., Nguyen, T., Wang, L., et al. (2012). A program for annotating and predicting the effects of single nucleotide polymorphisms, SnpEff: SNPs in the genome of Drosophila melanogaster strain w1118; iso-2; iso-3. Fly. (Austin) 6 (2), 80–92. doi:10.4161/fly.19695
Cock, P. J. A., Fields, C. J., Goto, N., Heuer, M. L., and Rice, P. M. (2010). The Sanger FASTQ file format for sequences with quality scores, and the Solexa/Illumina FASTQ variants. Nucleic Acids Res. 38 (6), 1767–1771. doi:10.1093/nar/gkp1137
Collins, F. S., and Fink, L. (1995). The human genome project. Alcohol Health Res. World 19 (3), 190–195.
Conrad, D. F., Pinto, D., Redon, R., Feuk, L., Gokcumen, O., Zhang, Y., et al. (2010). Origins and functional impact of copy number variation in the human genome. Nature 464 (7289), 704–712. doi:10.1038/nature08516
Coomer, C. A., Carlon-Andres, I., Iliopoulou, M., Dustin, M. L., Compeer, E. B., Compton, A. A., et al. (2020). Single-cell glycolytic activity regulates membrane tension and HIV-1 fusion. PLoS Pathog. 16 (2), e1008359. doi:10.1371/journal.ppat.1008359
Cooper, G. M., Stone, E. A., Asimenos, G., Program, N. C. S., Green, E. D., Batzoglou, S., et al. (2005). Distribution and intensity of constraint in mammalian genomic sequence. Genome Res. 15 (7), 901–913. doi:10.1101/gr.3577405
Danecek, P., Auton, A., Abecasis, G., Albers, C. A., Banks, E., DePristo, M. A., et al. (2011). The variant call format and VCFtools. Bioinformatics 27 (15), 2156–2158. doi:10.1093/bioinformatics/btr330
Das, S. K., Bhutia, S. K., Sokhi, U. K., Dash, R., Azab, B., Sarkar, D., et al. (2011). Human polynucleotide phosphorylase (hPNPaseold-35): an evolutionary conserved gene with an expanding repertoire of RNA degradation functions. Oncogene 30 (15), 1733–1743. doi:10.1038/onc.2010.572
DePristo, M. A., Banks, E., Poplin, R., Garimella, K. V., Maguire, J. R., Hartl, C., et al. (2011). A framework for variation discovery and genotyping using next-generation DNA sequencing data. Nat. Genet. 43 (5), 491–498. doi:10.1038/ng.806
Dong, C., Wei, P., Jian, X., Gibbs, R., Boerwinkle, E., Wang, K., et al. (2014). Comparison and integration of deleteriousness prediction methods for nonsynonymous SNVs in whole exome sequencing studies. Hum. Mol. Genet. 24 (8), 2125–2137. doi:10.1093/hmg/ddu733
Eilers, M., Roy, U., and Mondal, D. (2008). MRP (ABCC) transporters-mediated efflux of anti-HIV drugs, saquinavir and zidovudine, from human endothelial cells. Exp. Biol. Med. 233 (9), 1149–1160. doi:10.3181/0802-RM-59
Epi25 Collaborative (2019). Ultra-rare genetic variation in the epilepsies: a whole-exome sequencing study of 17,606 individuals. Am. J. Hum. Genet. 105 (2), 267–282. doi:10.1016/j.ajhg.2019.05.020
Escudero, D. J., Marukutira, T., McCormick, A., Makhema, J., and Seage, GRIII (2019). Botswana should consider expansion of free antiretroviral therapy to immigrants. J. Int. AIDS Soc. 22 (6), e25328. doi:10.1002/jia2.25328
Essex, M. (1999). Human immunodeficiency viruses in the developing world. Adv. Virus Res. 53, 71–88. doi:10.1016/s0065-3527(08)60343-7
Ewels, P., Magnusson, M., Lundin, S., and Käller, M. (2016). MultiQC: summarize analysis results for multiple tools and samples in a single report. Bioinformatics 32 (19), 3047–3048. doi:10.1093/bioinformatics/btw354
Fan, J., Ye, J., Kamphorst, J. J., Shlomi, T., Thompson, C. B., and Rabinowitz, J. D. (2014). Quantitative flux analysis reveals folate-dependent NADPH production. Nature 510 (7504), 298–302. doi:10.1038/nature13236
Farahani, M., Vable, A., Lebelonyane, R., Seipone, K., Anderson, M., Avalos, A., et al. (2014). Outcomes of the Botswana national HIV/AIDS treatment programme from 2002 to 2010: a longitudinal analysis. Lancet Glob. Heal 2 (1), e44–e50. doi:10.1016/S2214-109X(13)70149-9
Forbes, S. A., Beare, D., Gunasekaran, P., Leung, K., Bindal, N., Boutselakis, H., et al. (2015). COSMIC: exploring the world’s knowledge of somatic mutations in human cancer. Nucleic Acids Res. 43 (Database issue), D805–D811. doi:10.1093/nar/gku1075
Garber, M., Guttman, M., Clamp, M., Zody, M. C., Friedman, N., and Xie, X. (2009). Identifying novel constrained elements by exploiting biased substitution patterns. Bioinformatics 25 (12), i54–i62. doi:10.1093/bioinformatics/btp190
GeneCards (2020). GeneCards - human gene database. Available from: https://www.genecards.org/ (Accessed August 3, 2020).
Gu, Z., Eils, R., and Schlesner, M. (2016). Complex heatmaps reveal patterns and correlations in multidimensional genomic data. Bioinformatics 32, 2847–2849. doi:10.1093/bioinformatics/btw313
Gudykunst, W. B., and Schmidt, K. L. (1987). Language and ethnic identity: an overview and prologue. J. Lang. Soc. Psychol. 6 (3–4), 157–170. doi:10.1177/0261927x8763001
Gurdasani, D., Carstensen, T., Tekola-Ayele, F., Pagani, L., Tachmazidou, I., Hatzikotoulas, K., et al. (2015). The african genome variation project shapes medical genetics in Africa. Nature 517 (7534), 327–332. doi:10.1038/nature13997
Harris, M. A., Clark, J., Ireland, A., Lomax, J., Ashburner, M., Foulger, R., et al. (2004). The Gene Ontology (GO) database and informatics resource. Nucleic Acids Res. 32, D258–D261. doi:10.1093/nar/gkh036
Hegedus, A., Kavanagh Williamson, M., and Huthoff, H. (2014). HIV-1 pathogenicity and virion production are dependent on the metabolic phenotype of activated CD4+ T cells. Retrovirology 11 (1), 98. doi:10.1186/s12977-014-0098-4
Hernandez, R. D., Uricchio, L. H., Hartman, K., Ye, C., Dahl, A., and Zaitlen, N. (2019). Ultrarare variants drive substantial cis heritability of human gene expression. Nat. Genet. 51 (9), 1349–1355. doi:10.1038/s41588-019-0487-7
Hillebrand, F., Ostermann, P. N., Müller, L., Degrandi, D., Erkelenz, S., Widera, M., et al. (2019). Gymnotic delivery of LNA mixmers targeting viral SREs induces HIV-1 mRNA degradation. Int. J. Mol. Sci. 20 (5), 1088. doi:10.3390/ijms20051088
Hublin, J.-J., Ben-Ncer, A., Bailey, S. E., Freidline, S. E., Neubauer, S., Skinner, M. M., et al. (2017). New fossils from Jebel Irhoud, Morocco and the pan-African origin of Homo sapiens. Nature 546 (7657), 289–292. doi:10.1038/nature22336
Ignatieva, E. V., Yurchenko, A. A., Voevoda, M. I., and Yudin, N. S. (2019). Exome-wide search and functional annotation of genes associated in patients with severe tick-borne encephalitis in a Russian population. BMC Med. Genomics 12 (Suppl. 3), 61. doi:10.1186/s12920-019-0503-x
International Human Genome Sequencing Consortium (2004). Finishing the euchromatic sequence of the human genome. Nature 431 (7011), 931–945. doi:10.1038/nature03001
Johnston, H. R., Hu, Y., and Cutler, D. J. (2015). Population genetics identifies challenges in analyzing rare variants. Genet. Epidemiol. 39 (3), 145–148. doi:10.1002/gepi.21881
Kanehisa, M., and Goto, S. (2000). KEGG: kyoto encyclopedia of genes and genomes. Nucleic Acids Res. 28 (1), 27–30. doi:10.1093/nar/28.1.27
Karczewski, K. J., Francioli, L. C., Tiao, G., Cummings, B. B., Alföldi, J., Wang, Q., et al. (2020). The mutational constraint spectrum quantified from variation in 141,456 humans. Nature 581 (7809), 434–443. doi:10.1038/s41586-020-2308-7
Karczewski, K. J., Weisburd, B., Thomas, B., Solomonson, M., Ruderfer, D. M., Kavanagh, D., et al. (2017). The ExAC browser: displaying reference data information from over 60 000 exomes. Nucleic Acids Res. 45 (D1), D840–D845. doi:10.1093/nar/gkw971
Keinan, A., and Clark, A. G. (2012). Recent explosive human population growth has resulted in an excess of rare genetic variants. Science 336 (6082), 740–743. doi:10.1126/science.1217283
Khan, S., Iqbal, M., Tariq, M., Baig, S. M., and Abbas, W. (2018). Epigenetic regulation of HIV-1 latency: focus on polycomb group (PcG) proteins. Clin. Epigenetics 10 (1), 14. doi:10.1186/s13148-018-0441-z
Kircher, M., Witten, D. M., Jain, P., O’Roak, B. J., Cooper, G. M., and Shendure, J. (2014). A general framework for estimating the relative pathogenicity of human genetic variants. Nat. Genet. 46 (3), 310–315. doi:10.1038/ng.2892
Kishimoto, N., Iga, N., Yamamoto, K., Takamune, N., and Misumi, S. (2017). Virion-incorporated alpha-enolase suppresses the early stage of HIV-1 reverse transcription. Biochem. Biophys. Res. Commun. 484 (2), 278–284. doi:10.1016/j.bbrc.2017.01.096
Kuleshov, M. V., Jones, M. R., Rouillard, A. D., Fernandez, N. F., Duan, Q., Wang, Z., et al. (2016). Enrichr: a comprehensive gene set enrichment analysis web server 2016 update. Nucleic Acids Res. 44 (W1), W90–W97. doi:10.1093/nar/gkw377
Landrum, M. J., Lee, J. M., Riley, G. R., Jang, W., Rubinstein, W. S., Church, D. M., et al. (2013). ClinVar: public archive of relationships among sequence variation and human phenotype. Nucleic Acids Res. 42, D980–D985. doi:10.1093/nar/gkt1113
Lappin, T. R. J., Grier, D. G., Thompson, A., and Halliday, H. L. (2006). HOX genes: seductive science, mysterious mechanisms. Ulst. Med. J. 75 (1), 23–31.
Leszczyniecka, M., Kang, D., Sarkar, D., Su, Z., Holmes, M., Valerie, K., et al. (2002). Identification and cloning of human polynucleotide phosphorylase, hPNPase old-35, in the context of terminal differentiation and cellular senescence. Proc. Natl. Acad. Sci. 99 (26), 16636–16641. doi:10.1073/pnas.252643699
Li, H. (2011). A statistical framework for SNP calling, mutation discovery, association mapping and population genetical parameter estimation from sequencing data. Bioinformatics 27 (21), 2987–2993. doi:10.1093/bioinformatics/btr509
Li, H., and Durbin, R. (2009). Fast and accurate short read alignment with Burrows-Wheeler transform. Bioinformatics 25 (14), 1754–1760. doi:10.1093/bioinformatics/btp324
Li, H., Ruan, J., Durbin, R., Li, H., Ruan, J., and Durbin, R. (2008). Mapping short DNA sequencing reads and calling variants using mapping quality scores. Genome Res. 18, 1851–1858. doi:10.1101/gr.078212.108
Liu, S.-Y., Aliyari, R., Chikere, K., Li, G., Marsden, M. D., Smith, J. K., et al. (2013). Interferon-inducible cholesterol-25-hydroxylase broadly inhibits viral entry by production of 25-hydroxycholesterol. Immunity 38 (1), 92–105. doi:10.1016/j.immuni.2012.11.005
McGuire, A. L., Gabriel, S., Tishkoff, S. A., Wonkam, A., Chakravarti, A., Furlong, E. E. M., et al. (2020). The road ahead in genetics and genomics. Nat. Rev. Genet. 21 (10), 581–596. doi:10.1038/s41576-020-0272-6
McKenna, A., Hanna, M., Banks, E., Sivachenko, A., Cibulskis, K., Kernytsky, A., et al. (2010). The Genome Analysis Toolkit: a MapReduce framework for analyzing next-generation DNA sequencing data. Genome Res. Sep. 20 (9), 1297–1303. doi:10.1101/gr.107524.110
McKusick, V. A. (1998). Mendelian inheritance in man: a catalog of human genes and genetic disorders. Nucleic Acids Res. 1, 1.
Mi, H., Huang, X., Muruganujan, A., Tang, H., Mills, C., Kang, D., et al. (2017). PANTHER version 11: expanded annotation data from Gene Ontology and Reactome pathways, and data analysis tool enhancements. Nucleic Acids Res. 45 (D1), D183–D189. doi:10.1093/nar/gkw1138
Michalopoulos, S. (2012). The origins of ethnolinguistic diversity. Am. Econ. Rev. 102 (4), 1508–1539. doi:10.1257/aer.102.4.1508
Montinaro, F., Busby, G. B. J., Gonzalez-Santos, M., Oosthuitzen, O., Oosthuitzen, E., Anagnostou, P., et al. (2017). Complex ancient genetic structure and cultural transitions in southern african populations. Genetics 205 (1), 303–316. doi:10.1534/genetics.116.189209
Nagasaki, M., Yasuda, J., Katsuoka, F., Nariai, N., Kojima, K., Kawai, Y., et al. (2015). Rare variant discovery by deep whole-genome sequencing of 1,070 Japanese individuals. Nat. Commun. 6, 8018–8113. doi:10.1038/ncomms9018
Ng, P. C., and Henikoff, S. (2003). SIFT: predicting amino acid changes that affect protein function. Nucleic Acids Res. 31 (13), 3812–3814. doi:10.1093/nar/gkg509
Nielsen, R., Paul, J. S., Albrechtsen, A., and Song, Y. S. (2011). Genotype and SNP calling from next-generation sequencing data. Nat. Rev. Genet. 12 (6), 443–451. doi:10.1038/nrg2986
Niohuru, I. (2023). “Disease burden and mortality,” in Healthcare and disease burden in Africa: the impact of socioeconomic factors on public health (Cham: Springer International Publishing), 35–85. doi:10.1007/978-3-031-19719-2_3
Nkengasong, J. N., and Tessema, S. K. (2020). Africa needs a new public health order to tackle infectious disease threats. Cell. 183 (2), 296–300. doi:10.1016/j.cell.2020.09.041
Novitsky, V., Woldegabriel, E., Wester, C., McDonald, E., Rossenkhan, R., Ketunuti, M., et al. (2008). Identification of primary HIV-1C infection in Botswana. AIDS Care 20 (7), 806–811. doi:10.1080/09540120701694055
O'Leary, N. A., Wright, M. W., Brister, J. R., Ciufo, S., Haddad, D., McVeigh, R., et al. (2016). Reference sequence (RefSeq) database at NCBI: current status, taxonomic expansion, and functional annotation. Nucleic Acids Res. 44 (D1), D733–D745. doi:10.1093/nar/gkv1189
Pagel, K. A., Pejaver, V., Lin, G. N., Nam, H.-J., Mort, M., Cooper, D. N., et al. (2017). When loss-of-function is loss of function: assessing mutational signatures and impact of loss-of-function genetic variants. Bioinformatics 33 (14), i389–i398. doi:10.1093/bioinformatics/btx272
Palmer, C. S., Henstridge, D. C., Yu, D., Singh, A., Balderson, B., Duette, G., et al. (2016). Emerging role and characterization of immunometabolism: relevance to HIV pathogenesis, serious non-AIDS events, and a cure. J. Immunol. 196 (11), 4437–4444. doi:10.4049/jimmunol.1600120
Patterson, N., Price, A. L., and Reich, D. (2006). Population structure and eigenanalysis. PLoS Genet. 2 (12), e190. doi:10.1371/journal.pgen.0020190
Penning, T. M. (2015). The aldo-keto reductases (AKRs): overview. Chem. Biol. Interact. 234, 236–246. doi:10.1016/j.cbi.2014.09.024
Petersen, D. C., Libiger, O., Tindall, E. A., Hardie, R.-A., Hannick, L. I., Glashoff, R. H., et al. (2013). Complex patterns of genomic admixture within southern Africa. PLoS Genet. 9 (3), e1003309. doi:10.1371/journal.pgen.1003309
Pfeifer, S. P. (2017). From next-generation resequencing reads to a high-quality variant data set. Hered. (Edinb) 118 (2), 111–124. doi:10.1038/hdy.2016.102
Pickrell, J. K., Patterson, N., Barbieri, C., Berthold, F., Gerlach, L., Güldemann, T., et al. (2012). The genetic prehistory of southern Africa. Nat. Commun. 3, 1143. doi:10.1038/ncomms2140
Pickrell, J. K., Patterson, N., Loh, P.-R., Lipson, M., Berger, B., Stoneking, M., et al. (2014). Ancient west Eurasian ancestry in southern and eastern Africa. Proc. Natl. Acad. Sci. U. S. A. 111 (7), 2632–2637. doi:10.1073/pnas.1313787111
Price, A. L., Patterson, N. J., Plenge, R. M., Weinblatt, M. E., Shadick, N. A., and Reich, D. (2006). Principal components analysis corrects for stratification in genome-wide association studies. Nat. Genet. 38 (8), 904–909. doi:10.1038/ng1847
Purcell, S., Neale, B., Todd-Brown, K., Thomas, L., Ferreira, M. A. R., Bender, D., et al. (2007). PLINK: a tool set for whole-genome association and population-based linkage analyses. Am. J. Hum. Genet. 81 (3), 559–575. doi:10.1086/519795
R Core Team (2022). R: a language and environment for statistical computing. Vienna, Austria: Environment for Statistical Computing. Available from: https://www.r-project.org/.
Rentzsch, P., Witten, D., Cooper, G. M., Shendure, J., and Kircher, M. (2019). CADD: predicting the deleteriousness of variants throughout the human genome. Nucleic Acids Res. 47 (D1), D886–D894. doi:10.1093/nar/gky1016
Retshabile, G., Mlotshwa, B. C., Williams, L., Mwesigwa, S., Mboowa, G., Huang, Z., et al. (2018). Whole-exome sequencing reveals uncaptured variation and distinct ancestry in the southern african population of Botswana. Am. J. Hum. Genet. 102 (5), 731–743. doi:10.1016/j.ajhg.2018.03.010
Reva, B., Antipin, Y., and Sander, C. (2011). Predicting the functional impact of protein mutations: application to cancer genomics. Nucleic Acids Res. 39 (17), e118. doi:10.1093/nar/gkr407
Richard, A., and Becker, O. S. (2018). Enhancements by thomas P minka ARWR, deckmyn. A. Maps: draw geographical maps. Available from: https://cran.r-project.org/package=maps.
Salvaggio, S. E., Giacomelli, A., Falvella, F. S., Oreni, M. L., Meraviglia, P., Atzori, C., et al. (2017). Clinical and genetic factors associated with kidney tubular dysfunction in a real-life single centre cohort of HIV-positive patients. BMC Infect. Dis. 17 (1), 396. doi:10.1186/s12879-017-2497-3
Schlebusch, C. M., Sjödin, P., Breton, G., Günther, T., Naidoo, T., Hollfelder, N., et al. (2020). Khoe-san genomes reveal unique variation and confirm the deepest population divergence in Homo sapiens. Mol. Biol. Evol. 37 (10), 2944–2954. doi:10.1093/molbev/msaa140
Schwarz, J. M., Cooper, D. N., Schuelke, M., and Seelow, D. (2014). MutationTaster2: mutation prediction for the deep-sequencing age. Nat. methods 11, 361–362. doi:10.1038/nmeth.2890
Shapiro, R. L., Thior, I., Gilbert, P. B., Lockman, S., Wester, C., Smeaton, L. M., et al. (2006). Maternal single-dose nevirapine versus placebo as part of an antiretroviral strategy to prevent mother-to-child HIV transmission in Botswana. Aids 20 (9), 1281–1288. doi:10.1097/01.aids.0000232236.26630.35
Sherman, R. M., Forman, J., Antonescu, V., Puiu, D., Daya, M., Rafaels, N., et al. (2019). Assembly of a pan-genome from deep sequencing of 910 humans of African descent. Nat. Genet. 51 (1), 30–35. doi:10.1038/s41588-018-0273-y
Sherry, S. T., Ward, M. H., Kholodov, M., Baker, J., Phan, L., Smigielski, E. M., et al. (2001). dbSNP: the NCBI database of genetic variation. Nucleic acids Res. 29, 308–311. doi:10.1093/nar/29.1.308
Shevchenko, A. K., Zhernakova, D. V., Malov, S. V., Komissarov, A., Kolchanova, S. M., Tamazian, G., et al. (2021). Genome-wide association study reveals genetic variants associated with HIV-1C infection in a Botswana study population. Proc. Natl. Acad. Sci. 118 (47), e2107830118. doi:10.1073/pnas.2107830118
Shihab, H. A., Gough, J., Cooper, D. N., Stenson, P. D., Barker, G. L. A., Edwards, K. J., et al. (2013). Predicting the functional, molecular, and phenotypic consequences of amino acid substitutions using hidden Markov models. Hum. Mutat. 34 (1), 57–65. doi:10.1002/humu.22225
Shihab, H. A., Gough, J., Mort, M., Cooper, D. N., Day, I. N. M., and Gaunt, T. R. (2014). Ranking non-synonymous single nucleotide polymorphisms based on disease concepts. Hum. Genomics 8 (1), 11. doi:10.1186/1479-7364-8-11
Shytaj, I. L., Procopio, F. A., Tarek, M., Carlon-Andres, I., Tang, H.-Y., Goldman, A. R., et al. (2021). Glycolysis downregulation is a hallmark of HIV-1 latency and sensitizes infected cells to oxidative stress. EMBO Mol. Med. 13 (8), e13901. doi:10.15252/emmm.202013901
Sim, N.-L., Kumar, P., Hu, J., Henikoff, S., Schneider, G., and Ng, P. C. (2012). SIFT web server: predicting effects of amino acid substitutions on proteins. Nucleic Acids Res. 40 (W1), W452–W457. doi:10.1093/nar/gks539
Sirugo, G., Williams, S. M., and Tishkoff, S. A. (2019). The missing diversity in human genetic studies. Cell. 177, 26–31. doi:10.1016/j.cell.2019.02.048
Statistics Botswana (2022). BAIS V summary report. Available from: https://www.statsbots.org.bw/sites/default/files/BAISVPreliminaryReport.pdf (Accessed October 14, 2022).
Slenter, D. N., Kutmon, M., Hanspers, K., Riutta, A., Windsor, J., Nunes, N., et al. (2018). WikiPathways: a multifaceted pathway database bridging metabolomics to other omics research. Nucleic Acids Res. 46 (D1), D661–D667. doi:10.1093/nar/gkx1064
Svitin, A., Malov, S., Cherkasov, N., Geerts, P., Rotkevich, M., Dobrynin, P., et al. (2014). GWATCH: a web platform for automated gene association discovery analysis. Gigascience 3 (1), 18–10. doi:10.1186/2047-217X-3-18
Taylor, H. E., and Palmer, C. S. (2020). CD4 T cell metabolism is a major contributor of HIV infectivity and reservoir persistence. Immunometabolism 2 (1), e200005. doi:10.20900/immunometab20200005
Thami, P. K., and Chimusa, E. R. (2019). Population structure and implications on the genetic architecture of HIV-1 phenotypes within southern Africa. Front. Genet. 10, 905. doi:10.3389/fgene.2019.00905
The 1000 Genomes Project Consortium Abecasis, G. R., Altshuler, D., Auton, A., Brooks, L. D., Durbin, R. M., et al. (2010). A map of human genome variation from population-scale sequencing. Nature 467 (7319), 1061–1073. doi:10.1038/nature09534
The 1000 Genomes Project Consortium Abecasis, G. R., Auton, A., Brooks, L. D., DePristo, M. A., Durbin, R. M., et al. (2012). An integrated map of genetic variation from 1,092 human genomes. Nature 491, 56–65. doi:10.1038/nature11632
The 1000 Genomes Project Consortium Auton, A., Abecasis, G. R., Altshuler, D. M., Durbin, R. M., Abecasis, G. R., et al. (2015). A global reference for human genetic variation. Nature 526 (7571), 68–74. doi:10.1038/nature15393
Thior, I., Lockman, S., Smeaton, L. M., Shapiro, R. L., Wester, C., Heymann, S. J., et al. (2006). Breastfeeding plus infant zidovudine prophylaxis for 6 months vs formula feeding plus infant zidovudine for 1 month to reduce mother-to-child HIV transmission in Botswana: a randomized trial: the Mashi Study. Jama 296 (7), 794–805. doi:10.1001/jama.296.7.794
Tishkoff, S. A., Reed, F. A., Friedlaender, F. R., Ehret, C., Ranciaro, A., Froment, A., et al. (2009). The genetic structure and history of Africans and African Americans. Science 324 (5930), 1035–1044. doi:10.1126/science.1172257
Torkamani, A., Scott-Van Zeeland, A. A., Topol, E. J., and Schork, N. J. (2011). Annotating individual human genomes. Genomics 98 (4), 233–241. doi:10.1016/j.ygeno.2011.07.006
UNAIDS (2019). UNAIDS data 2019. Available from: https://www.unaids.org/en/regionscountries/countries/botswana (Accessed March 6, 2020).
Valle-Casuso, J. C., Angin, M., Volant, S., Passaes, C., Monceaux, V., Mikhailova, A., et al. (2019). Cellular metabolism is a major determinant of HIV-1 reservoir seeding in CD4+ T cells and offers an opportunity to tackle infection. Cell. Metab. 29 (3), 611–626. doi:10.1016/j.cmet.2018.11.015
Van Der Auwera, G. A., Carneiro, M. O., Hartl, C., Poplin, R., Levy-moonshine, A., Jordan, T., et al. (2014). From FastQ data to high confidence variant calls: the Genome Analysis Toolkit best practices pipeline. Curr. Protoc. Bioinforma. 11 (1110). doi:10.1002/0471250953.bi1110s43
Visscher, P. M., Brown, M. A., McCarthy, M. I., and Yang, J. (2012). Five years of GWAS discovery. Am. J. Hum. Genet. 90 (1), 7–24. doi:10.1016/j.ajhg.2011.11.029
Wang, K. (2023). ANNOVAR documentation: user guide. Available from: https://annovar.openbioinformatics.org/en/latest/user-guide/gene/ (Accessed October 21, 2023).
Wang, K., Li, M., and Hakonarson, H. (2010). ANNOVAR: functional annotation of genetic variants from high-throughput sequencing data. Nucleic Acids Res. 38 (16), e164. doi:10.1093/nar/gkq603
Warde-Farley, D., Donaldson, S. L., Comes, O., Zuberi, K., Badrawi, R., Chao, P., et al. (2010). The GeneMANIA prediction server: biological network integration for gene prioritization and predicting gene function. Nucleic Acids Res. 38, W214–W220. doi:10.1093/nar/gkq537
Weir, B. S., and Cockerham, C. C. (1984). ESTIMATING F-STATISTICS FOR THE ANALYSIS OF POPULATION STRUCTURE. Evolution 38 (6), 1358–1370. doi:10.1111/j.1558-5646.1984.tb05657.x
Whirl-Carrillo, M., McDonagh, E. M., Hebert, J. M., Gong, L., Sangkuhl, K., Thorn, C. F., et al. (2012). Pharmacogenomics knowledge for personalized medicine. Clin. Pharmacol. Ther. 92 (4), 414–417. doi:10.1038/clpt.2012.96
Wickham, H. (2016). ggplot2: elegant graphics for data analysis. New York: Springer-Verlag. Available from: https://ggplot2.tidyverse.org.
Wilusz, C. J., and Wilusz, J. (2008). New ways to meet your (3′) end—oligouridylation as a step on the path to destruction. Genes. Dev. 22 (1), 1–7. doi:10.1101/gad.1634508
Wonkam, A., and Adeyemo, A. (2023). Leveraging our common African origins to understand human evolution and health. Cell. genomics 3, 100278. doi:10.1016/j.xgen.2023.100278
Wonkam, A., Chimusa, E. R., Mnika, K., Pule, G. D., Ngo, B. V. J., Mulder, N., et al. (2020). Genetic modifiers of long-term survival in sickle cell anemia. Clin. Transl. Med. 10 (4), e152. doi:10.1002/ctm2.152
Wonkam, A., Munung, N. S., Dandara, C., Esoh, K. K., Hanchard, N. A., and Landoure, G. (2022). Five priorities of african genomics research: the next frontier. Annu. Rev. Genomics Hum. Genet. 23 (1), 499–521. doi:10.1146/annurev-genom-111521-102452
Xie, W., Agniel, D., Shevchenko, A., Malov, S. V., Svitin, A., Cherkasov, N., et al. (2017). Genome-wide analyses reveal gene influence on HIV disease progression and HIV-1C acquisition in southern Africa. AIDS Res. Hum. Retroviruses 33 (6), 597–609. doi:10.1089/AID.2016.0017
Keywords: genome variation, population genetics, human immunodeficiency virus (HIV-1), genomics, functional prediction, Botswana, Africa
Citation: Thami PK, Choga WT, Dandara C, O’Brien SJ, Essex M, Gaseitsiwe S and Chimusa ER (2023) Whole genome sequencing reveals population diversity and variation in HIV-1 specific host genes. Front. Genet. 14:1290624. doi: 10.3389/fgene.2023.1290624
Received: 07 September 2023; Accepted: 20 November 2023;
Published: 20 December 2023.
Edited by:
Francesco Montinaro, University of Tartu, EstoniaReviewed by:
Ryan Daniels, University of the Western Cape, South AfricaJonathon Mohl, The University of Texas at El Paso, United States
Copyright © 2023 Thami, Choga, Dandara, O’Brien, Essex, Gaseitsiwe and Chimusa. This is an open-access article distributed under the terms of the Creative Commons Attribution License (CC BY). The use, distribution or reproduction in other forums is permitted, provided the original author(s) and the copyright owner(s) are credited and that the original publication in this journal is cited, in accordance with accepted academic practice. No use, distribution or reproduction is permitted which does not comply with these terms.
*Correspondence: Emile R. Chimusa, emile.chimusa@.northumbria.ac.uk; Prisca K. Thami, p.thami@imperial.ac.uk
†Present address: Prisca K. Thami, Imperial College London, National Heart and Lung Institute, London, United Kingdom, Medical Research Council Laboratory of Medical Sciences, Imperial College London, London, United Kingdom