- 1Department of Biotechnology, Jaypee Institute of Information Technology, Noida, Uttar Pradesh, India
- 2Department of Genetic Engineering, Computational Biology Lab, SRM Institute of Science and Technology, Chennai, India
- 3Biotechnology Divison, National Centre for Disease Control, New Delhi, India
- 4Department of Chemistry and Department of Carbon Materials, Chosun University, Gwangju, Republic of Korea
- 5Department of Biosciences, Jamia Millia University, New Delhi, India
Introduction: Kinesin family member 5A (KIF5A) is a motor neuron protein expressed in neurons and involved in anterograde transportation of organelles, proteins, and RNA. Variations in the KIF5A gene that interfere with axonal transport have emerged as a distinguishing feature in several neurodegenerative disorders, including hereditary spastic paraplegia (HSP10), Charcot-Marie-Tooth disease type 2 (CMT2), and Amyotrophic Lateral Sclerosis (ALS).
Methods: In this study, we implemented a computational structural and systems biology approach to uncover the role of KIF5A in ALS. Using the computational structural biology method, we explored the role of non-synonymous Single Nucleotide Polymorphism (nsSNPs) in KIF5A. Further, to identify the potential inhibitory molecule against the highly destabilizing structure variant, we docked 24 plant-derived phytochemicals involved in ALS.
Results: We found KIF5AS291F variant showed the most structure destabilizing behavior and the phytocompound “epigallocatechin gallate” showed the highest binding affinity (−9.0 Kcal/mol) as compared to wild KIF5A (−8.4 Kcal/mol). Further, with the systems biology approach, we constructed the KIF5A protein-protein interaction (PPI) network to identify the associated Kinesin Families (KIFs) proteins, modules, and their function. We also constructed a transcriptional and post-transcriptional regulatory network of KIF5A. With the network topological parameters of PPIN (Degree, Bottleneck, Closeness, and MNC) using CytoHubba and computational knock-out experiment using Network Analyzer, we found KIF1A, 5B, and 5C were the significant proteins. The functional modules were highly enriched with microtubule motor activity, chemical synaptic transmission in neurons, GTP binding, and GABA receptor activity. In regulatory network analysis, we found KIF5A post-transcriptionally down-regulated by miR-107 which is further transcriptionally up-regulated by four TFs (HIF1A, PPARA, SREBF1, and TP53) and down-regulated by three TFs (ZEB1, ZEB2, and LIN28A).
Discussion: We concluded our study by finding a crucial variant of KIF5A and its potential therapeutic target (epigallocatechin gallate) and KIF5A associated significant genes with important regulators which could decrypt the novel therapeutics in ALS and other neurodegenerative diseases.
Introduction
Kinesin molecular motor proteins are ATPase-dependent machinery that move along microtubule tracks to promote cargo transport within the cell. Motor proteins mainly consist of binding sites for cargo transport and interaction with tubulin subunits. Since its discovery, many members of the kinesin family (KIF) have been identified (Nakagawa et al., 1997; Miki et al., 2001), which is divided into 15 kinesin families, referred to as kinesin 1 to kinesin 14B (Hirokawa et al., 2009; Wang and Xu, 2015). Functional impairment, imaging techniques, and biochemical investigations have all revealed significant functions of kinesins in the process of cell division (Sekine et al., 1994; He et al., 2016), brain development (Hirokawa et al., 2010), and many neurological disorders such as Alzheimer’s disease (AD) (Kamal et al., 2001), amyotrophic lateral sclerosis (ALS) (Devon et al., 2006; Had et al., 2006), and hereditary spastic paraplegia (HSP10) (Reid et al., 2002). Multiple studies have indicated that kinesins play specific and crucial roles in synaptic transmission (Hirokawa et al., 2009; Chen et al., 2011; Kunz et al., 2013). Nonetheless, it remains uncertain whether only specific subsets or all members of kinesins are necessary for synaptic transmission, and the mechanisms through which KIFs govern excitatory synaptic transmission are yet to be fully understood.
KIF5A is a member of kinesin superfamily proteins. It is expressed in neuronal cells and plays an important role in axonal transport (Smith et al., 2006). KIF5A consists of a motor domain at N-terminal, a central coiled-coil stalk region, and a C-terminal cargo binding region (Smith et al., 2006). Studies have elucidated that mutation in N-terminal domains and cargo binding at the C-terminal region of KIF5A cause aggregation of abnormal proteins, metabolic dysfunction, cell-to-cell communication dysregulation, and alternation in genetic networks, leading to escalation of neuronal cell death (Valko and Ciesla, 2019). Interestingly, missense mutation in the N-terminal motor domain and the central stalk domain of KIF5A is known to be related to autosomal diseases including hereditary spastic paraplegia (Reid et al., 2002; Goizet et al., 2009; Morais et al., 2017) and Charcot–Marie–Tooth type 2 (CMT2) (Liu et al., 2014). Additionally, it has been found that splice site mutations in the C-terminal binding domain of KIF5A cause exon skipping, leading to the loss of RNA expression. Thus, it is suggested that haploinsufficiency is the most likely underlying molecular mechanism for the neurodegenerative disorder ALS (Brenner et al., 2018). It is the foremost neurodegenerative disease that arises in two forms, familial and sporadic ALS majorly persistent motor neuron disease, with an occurrence of 1–5 for every 100,000 individuals (Hardiman et al., 2017). Because of the absence of compelling treatment, ALS prompts death between 2 and 5 years after diagnosis, majorly because of the failure of respiratory tract function. However, most of the cases are sporadic (sALS) (Van Rhe et al., 2016), but only 5%–10% are associated with a genetic mutation that is inherited through the family (Chia et al., 2018). One of the recent studies has revealed that a non-synonymous SNP variant (rs113247976) in KIF5A, located in the C-terminal region [Pro986Leu] of the exon, plays a significant role in ALS (Brenner et al., 2018). Impairments in axonal transport and modifications in the cytoskeletal structure are prominent characteristics observed in ALS patients, primarily contributing to the degeneration of motor neuron pathways (Roy et al., 2010). Missense mutations within the C-terminal domain have been notably linked to disruptions in microtubule binding and ATP hydrolysis, resulting in the impairment of anterograde transport of cargo proteins within both dendritic and axonal regions (Ebbing et al., 2008). The primary cause of ALS is the loss of function resulting from a missense mutation in the C-terminal domain of KIF5A. Thus, there is an urgent need for preventive measures that can effectively restore or stabilize the loss of cytoskeleton function caused by mutations. This provides an opportunity for the development of drugs that could potentially address ALS. There are several non-phytochemical-based therapies used to treat neurodegenerative diseases and amyotrophic lateral sclerosis, and some of them had faced challenges or failures in clinical trials. Here, we present some current therapies and examples of drugs with their mechanisms of action and known challenges or failures: monoclonal antibodies targeting amyloid beta or tau (e.g., aducanumab for Alzheimer’s disease) aim to clear amyloid-beta plaques or tau aggregates (Zhang et al., 2023). Aducanumab’s FDA approval in 2021 was controversial and failed due to reasons of clinical efficacy and high cost. Glutamate modulators (e.g., memantine for Alzheimer’s disease) are NMDA receptor antagonists that regulate glutamate activity to protect neurons from excitotoxicity (Wang and Reddy, 2017). Memantine is used in Alzheimer’s treatment but may provide only modest benefits in some cases. Gene therapy (e.g., onasemnogene abeparvovec for spinal muscular atrophy) used to deliver a functional copy of the SMN1 gene to replace the defective gene in spinal muscular atrophy (SMA) has shown clinical benefits (Ogbonmide et al., 2023). Riluzole modulates glutamate activity and may slow the progression of ALS by reducing excitotoxicity (Jiang et al., 2022). Riluzole is an approved drug for ALS treatment and can extend survival by several months. Edaravone (Radicava) is an antioxidant that aims to reduce oxidative stress, which may contribute to motor neuron damage in ALS. While edaravone received FDA approval for ALS treatment, its clinical benefits are modest and primarily seen in specific patient subgroups (Jiang et al., 2022). Both riluzole and edaravone do not provide a permanent treatment to ALS patients (Jaiswal, 2019). Another antisense oligonucleotide therapy tofersen (formerly IONIS-SOD1Rx) to target SOD1 gene expression has been formulated. The drug showed promise in early-stage clinical trials, and further trials were underway to assess its efficacy (Miller et al., 2022). It is essential to recognize that neurodegenerative diseases and ALS are complex, heterogeneous conditions with multiple underlying mechanisms, and finding effective treatments remains a significant challenge. While some therapies have been successful in managing symptoms or slowing disease progression to some extent, there is still a need for more targeted and curative approaches. Ongoing research efforts continue to explore novel treatments and mechanisms to address these devastating diseases.
Here, we applied structural and systems biology approaches to identify novel molecules for drug design against ALS. Genetic variations play a crucial role in the process of evolution, as they are linked to the phenotypic effects that can shed light on various disorders (Venselaar et al., 2010). SNPs can damage the protein structure, stability, function, and activity (Wu and Jiang, 2013). Our study focused on identifying the plant-derived bioactive molecules involved in excitatory amino acid toxicity, neuroinflammation, calcium cytotoxicity, and oxidative stress (Mohi-ud-din et al., 2021) in ALS against highly damaging missense SNPs (Yue and Moult, 2006). The phytochemicals from plant sources, such as flavonoids, alkaloids, terpenes, and saponins, may still have the potential benefits that researchers are seeking, which is because of their distinctive chemical diversity (Solanki et al., 2015; Zeng et al., 2021; Shareena and Kumar). Some of these phytochemicals cannot be synthesized by currently known methods. Therefore, these natural substances are still unexplored as new therapeutic molecules for the treatment of ALS (Matheus et al., 2023). The systems biology approaches have played an important role in identifying novel disease network regulators in neurological diseases (Su et al., 2018). We constructed and analyzed the protein–protein interaction (PPI) network of KIF5A to identify the subnetworks involved in a particular function and identification of other KIF members that interacted with KIF5A using network analysis. Furthermore, we performed the GO enrichment analysis of subnetworks in terms of biological processes (BPs), molecular functions (MFs), and cellular components (CCs). Transcriptional and post-transcriptional regulation is an important mechanism for the cell to have a normal function. Notably, one gene may be regulated by multiple miRNAs and transcription factors (TFs), and each miRNA and TFs target more than one gene. The regulatory molecule transcription factors (transcription regulators: TFs) and microRNA (post-transcriptional regulators: miRNA) help elucidate the expression level of genes (Su et al., 2018). Computational predicted miRNA targets and transcription factors of KIF5A were extracted from databases to understand the regulatory mechanisms.
Methods
Data mining for SNPs
Retrieval of protein sequences and SNPs of KIF5A has been carried out from the dbSNP database build 155 on NCBI (https://www.ncbi.nlm.nih.gov/gene/3798) and ALS Variant Server (http://als.umassmed.edu/). Here, our search majorly focused on non-synonymous missense SNPs, which were subsequently subjected to analysis to determine their detrimental and disease-causing impacts on the KIF5A protein; the detailed schematic representation of the study is provided in Figure 1A. We used eight different sequences and structure-based tools SIFT (Ng and Henikoff, 2003), PolyPhen-2 (Adzhubei et al., 2013), SNAP2 (Bromberg and Rost, 2007), PhD-SNP (Capriotti and Fariselli, 2017), PMut (López-Ferrando et al., 2017), SNPs&GO (Capriotti et al., 2013), PANTHER (Tang and Thomas, 2016), and SuSPect (Yate et al., 2014) to analyze the functional effects of nsSNPs. Alongside common nsSNPs screened using eight different prediction programs, we incorporated an additional eight nsSNPs reported in the ALS Variant Database (http://als.umassmed.edu/). These eight nsSNPs were not presented in the NCBI dbSNP database build 155 during the screening of nsSNPs. Consequently, to comprehensively assess their structural impact and their influence on protein stability, we included them in our analysis (Figure 1A) (Nicolas et al., 2018; Bertolin et al., 2017).
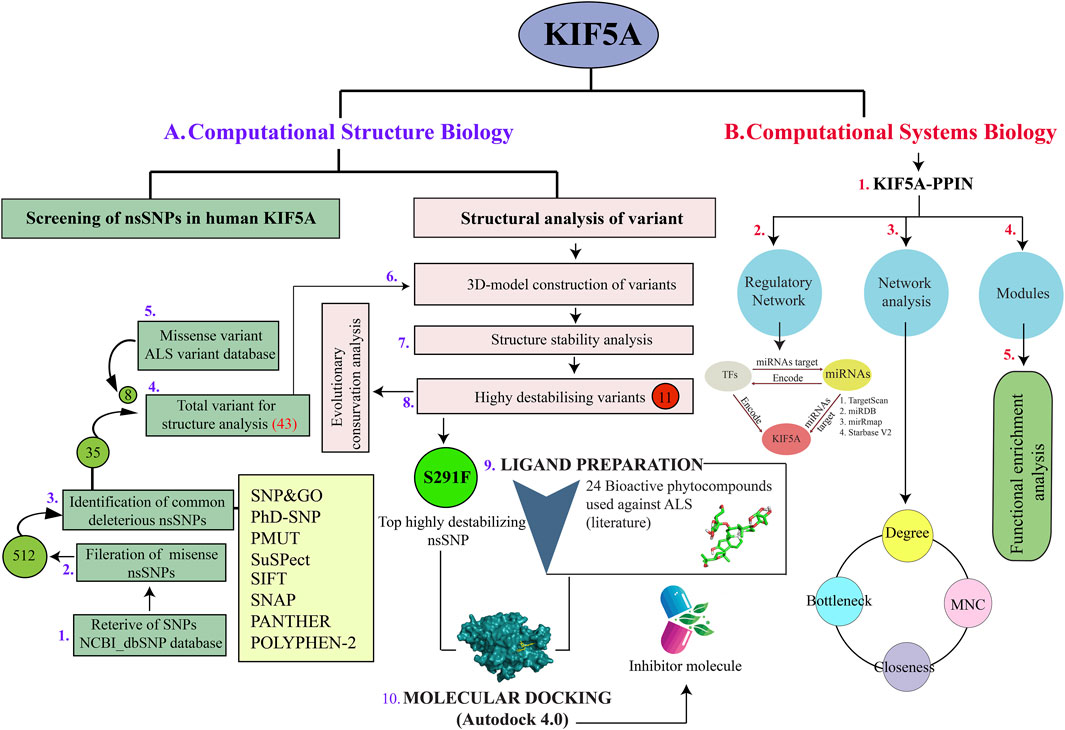
FIGURE 1. Implemented workflow used during the structure and systems biology approach in the KIF5A motor neuron protein. (A) Retrieval of SNPs using the dbSNP database used to filter missense SNPs (512), which was further used for identifying common deleterious nsSNPs (35) using eight different prediction tools. We also included eight nsSNPs associated with KIF5A, given in the ALS Variant database. Out of a total of 43 nsSNPs, we screened 11 destabilizing KIF5A variants, in which mutant S291F was the top destabilizing nsSNP. We prepared 24 bioactive compounds to screen the potential inhibitors of mutant S291F using molecular docking analysis. (B) Schematic analysis of PPIN of KIF5A included the regulatory network analysis using transcriptional and post-transcriptional molecules, transcription factors, and miRNAs. Identification of KIF5A associated functional modules; the KIF5A–PPIN network analysis to identify KIFs using the network parameters degree, bottleneck, MNC, and closeness.
3D model construction and validation
The three-dimensional structure of KIF5A was downloaded from UniProt (https://www.uniprot.org/uniprotkb/Q12840/entry). The wild-type structure of KIF5A was evaluated in PDBsum (http://www.ebi.ac.uk/thornton-srv/databases/pdbsum/Generate.html) that provides a visual representation of molecules, including the construction of structures like DNA, protein chains, ligands, and metal ions, along with a schematic depiction of their interactions (Yang et al., 2014). The Ramachandran plot assesses the dihedral angles of amino acid residues, and by analyzing their phi and psi dihedral angles, it identifies which residues are energetically permissible. This process helps establish the structural and functional characteristics of a protein (Gopalakrishnan et al., 2007; Ca et al., 2014). PDBsum offers a visual representation of a protein’s three-dimensional structure and provides information on angles, helices, motifs, beta-sheets, and strands. An ideal protein structure typically comprises over 90% of its residues within the favored region (Laskowski et al., 2018). PROCHECK evaluates the stereochemical characteristics of proteins by examining both the overall structural and residue-level geometry (https://www.ebi.ac.uk/thornton-srv/software/PROCHECK/) (Laskowski et al., 1993).
Stability change prediction of mutants
The structure of the mutants was generated using the WHAT IF server (https://swift.cmbi.umcn.nl/servers/html/index.html) (Vriend, 1990), and further energy minimization of mutants and native structure was performed using YASARA (http://www.yasara.org/minimizationserver.htm) (Krieger et al., 2009). The structural stability of the mutant for the wild-type was predicted utilizing FoldX (http://foldxsuite.crg.eu/) (Schymkowitz et al., 2005). It generates a quantitative estimation of significant interactions imparting to the stability of protein structure by utilizing its atomic description (Schymkowitz et al., 2005).
Domain prediction, evolutionary conservation, and mapping of highly deleterious nsSNPs
To predict the functional domain of KIF5A, we used the InterPro server (https://www.ebi.ac.uk/interpro/), version 95.0, accessed on 8 July 2023. InterPro provides the functional analysis of proteins by classifying them into families and predicting domains and important sites (Hunter et al., 2009). InterPro combines protein signatures from 13 member databases CATH-Gene3D, CDD, HAMAP, PANTHER, Pfam, PIRSF, PRINTS, PROSITE profiles, PROSITE patterns, SFLD, SMART, SUPERFAMILY, and TIGRFAMs (Hunter et al., 2009). The ConSurf web server was employed to compute the evolutionary conservation of amino acid positions (Ashkenazy et al., 2016) by assigning of a score between 1 (most variable position) and 9 (most conserved position) to each amino acid position. The protein sequence similarity searching was performed against UniRef90, in which CSI-BLAST (Context-Specific Iterated-Basic Local Alignment Search Tool), 3, and 0.0001 were set for the homolog search algorithm, number of iterations, and E-value cutoff, respectively. Furthermore, we mapped the predicted highly structure destabilizing nsSNPs against the functional domain of KIF5A to understand their functional impact (Figure 1A).
Selection of bioactive plant-derived compounds and molecular docking
Bioactive compounds derived from plants are being explored for their potential effectiveness against excitatory amino acid toxicity, neuroinflammation, calcium cytotoxicity, and oxidative stress in ALS as observed from the literature (Mohi-ud-din et al., 2021). These compounds were downloaded from ZINC and PubChem (https://pubchem.ncbi.nlm.nih.gov/databases) and were further pre-processed using AutoDock 4.0 (Eberhardt et al., 2021). To understand the mechanism of action of plant-derived bioactive compounds showing the highest binding affinity with the KIF5AS291F mutant, we performed a comparative docking study in the wild-type KIF5A kinesin motor domain and the mutant KIF5AS291F structure to calculate their binding affinity and interacting residues.
KIF5A–PPIN and its functional modules
A network theory-based approach is used to delineate connections within intricate systems and serves as an important organizing principle for cellular networks within the field of systems biology. This approach further facilitates the comprehension of how specific molecules contribute to various cellular processes. We constructed the PPIN of KIF5A using the STRING database (Szklarczyk et al., 2019) and identified the functional modules using “Molecular Complex Detection” (MCODE) v2.0.0 (Hogue and Groll, 2001). MCODE is a Cytoscape plugin that is designed to pinpoint nodes with high interconnections forming clusters that represent relatively stable, multi-protein complexes functioning as unified entities within the network. Modules control the network from dissortivity and also maintain the flow of information throughout the network (Lalwani et al., 2022; Nafis et al., 2014). The identified modules were functionally annotated in terms of BPs, MFs, and CCs using the Enrichr web-based tool to correlate the biological significance (Xie et al., 2021). We further analyze the PPIN of KIF5A using two local ranking methods degree (Pk) and maximum neighborhood component (MNC) and two global methods (bottleneck and closeness) with the help of the Cytoscape (v3.8.0) (Shannon et al., 2003) plugin CytoHubba (Chin et al., 2014). Using CytoHubba, we can identify the top 10 nodes which could play an important role in controlling the network and signal propagation (Chen et al., 2023; Takayama et al., 2023; Gu et al., 2023). We also performed the computational knockout experiments by eliminating the top significant node, resulting in network perturbations, a phenomenon known as the centrality–lethality rule (Jeong et al., 2001; Malik et al., 2019). Accumulating mutations in a gene can lead to the disruption or loss of expression of a particular protein, resulting in its impairment or absence within the cell. In general, this situation of re-examining the network parameter can be illustrated by eliminating the nodes that may have been modified from the network (Peng et al., 2015). Here, we computed the topological parameters such as degree distribution (Pk), betweenness centrality (BC), and neighborhood connectivity (NC) of the modified/reorganized network to assess the regulating capacities of nodes using Network Analyzer in Cytoscape (v3.8.0) (Shannon et al., 2003).
Tracing the key transcriptional and post-transcriptional regulators of KIF5A
To understand the post-transcriptional regulation of KIF5A, we identify the number of miRNA targets by using four different databases, TargetScanHuman release 8.0 (Agarwal et al., 2015), miRDB (Chen and Wang, 2020), miRmap (Vejnar and Zdobnov, 2012), and starBase v2.0 (Li et al., 2014). The TFs related to KIF5A were extracted using the ENCODE in-built option in NetworkAnalyst 3.0, a comprehensive gene expression profiling and network visual analytic tool (Zhou et al., 2019). To identify the key transcriptional and post-transcriptional regulators of KIF5A, we mapped the TFs and the common miRNA targets present in all four miRNA target prediction databases. We constructed and visualized the regulatory network of KIF5A–miRNAs–TFs using Cytoscape v3.8.0 (Shannon et al., 2003).
Results
Deleterious nsSNPs in KIF5A
The in silico screening of 511 nsSNPs was performed using sequence- and structure-based tools (Figure 1A). The eight screening programs SIFT, PolyPhen-2, SNAP2, PhD-SNP, PMut, SNPs&GO, PANTHER, and SuSPect identified 35 common nsSNPs as deleterious and could have a high chance of affecting the protein function (Table 1). We added eight more missense variants of exonic region SNPs of KIF5A (E669K, E758K, T858A, T947A, T887I, T976I, P897L, and P986L) retrieved from the ALS Variant Server to understand their deleterious impact on structure stability for further analysis.
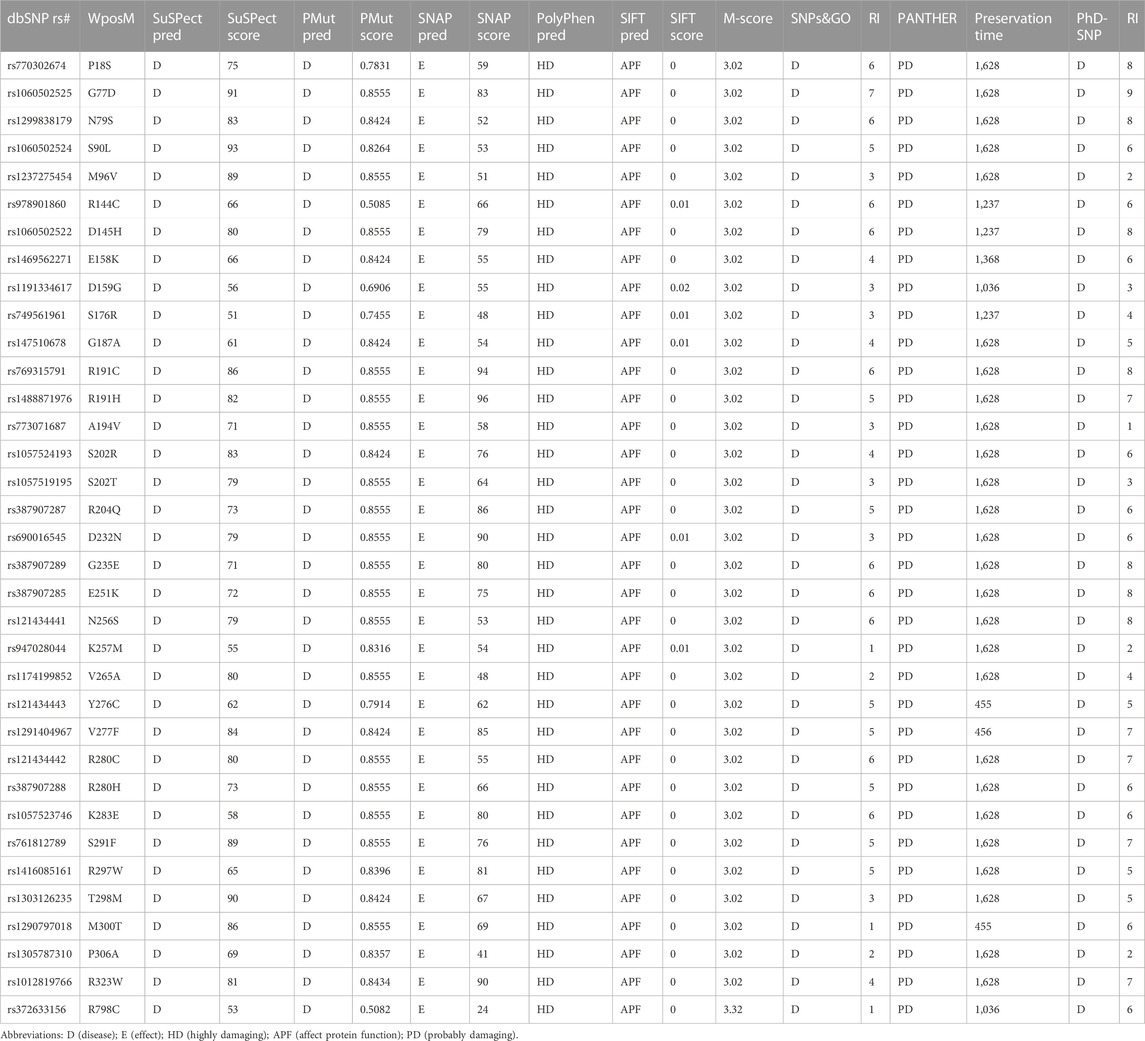
TABLE 1. List of 35 common nsSNPs identified as deleterious in eight different prediction tools having a high chance of affecting the protein function (KIF5A).
3D model of KIF5A, validation, and mutant stability comparison
The 3D structure for the KIF5A protein was downloaded using AlphaFold (ID: AF-Q12840) from the UniProt database. The downloaded model is considered a good model for structure analysis and contains a complete structure of 1–1,032 amino acid residues (Figure 2A). To validate the structure, the PDBsum and PROCHECK servers were employed, with the PROCHECK program generating a Ramachandran plot (RC plot) to assess the stereochemical characteristics of the protein structure and provide detailed residue-by-residue and overall geometry analysis. The predicted model’s reliability was confirmed, with 88.4% of residues located in the most favored region, 6.5% in the allowed region, and 2.6% in the generously allowed region, while only 2.5% of residues were situated in the disallowed region (Figure 2B). The structure stability analysis result by FoldX predicted that out of a total of 43 nsSNPs, S291F showed the highest structure-destabilizing behavior in terms of free energy (Figure 2C). The KIF5AS291F mutation was also presented in the kinesin motor domain (7–335 amino acids) of the KIF5A protein predicted by the InterPro tool (Figure 2D). To validate the structure stability differences, we checked the number of hydrogen bonds between wild KIF5A and variant KIF5AS291F. The wild-type KIF5ASER291 residue formed three hydrogen bonds with residues 81THR, 288LEU, 298THR, and ASN329, but in the case of mutant PHE291, only three hydrogen bonds were formed with 288LEU, 298THR, and ASN329, as shown in Figure 3A.
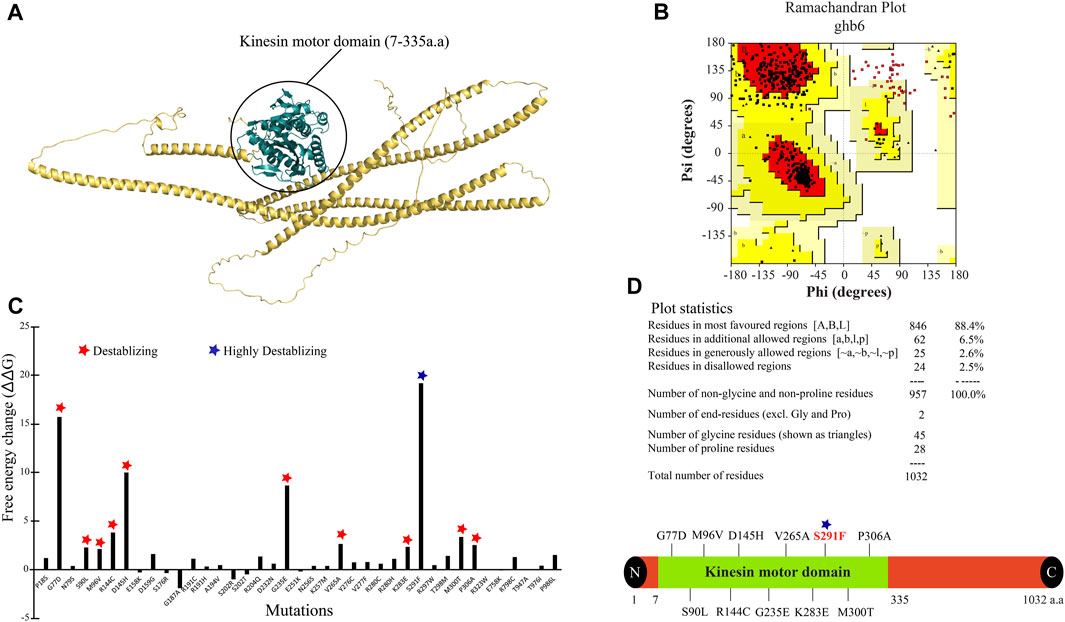
FIGURE 2. KIF5A protein structure, validation, mutant stability analysis, and their mapping. (A) The 3D structure of KIF5A protein having a sequence length of 1,032 showing the kinesin motor domain in deep teal color (7–335 amino acids). (B) The representation of the Ramachandran plot generated by PDBsum stated the information of amino acids in a 3D structure. (C) Highlighting the free energy differences between 43 mutants, in which “S291” showed the highest destabilizing nature. (D) Representation of destabilizing mutants in the kinesin motor domain; out of 12 destabilizing mutants, 11 were presented in the kinesin motor domain (7–335 amino acids), and the S291F mutant is shown in red text.
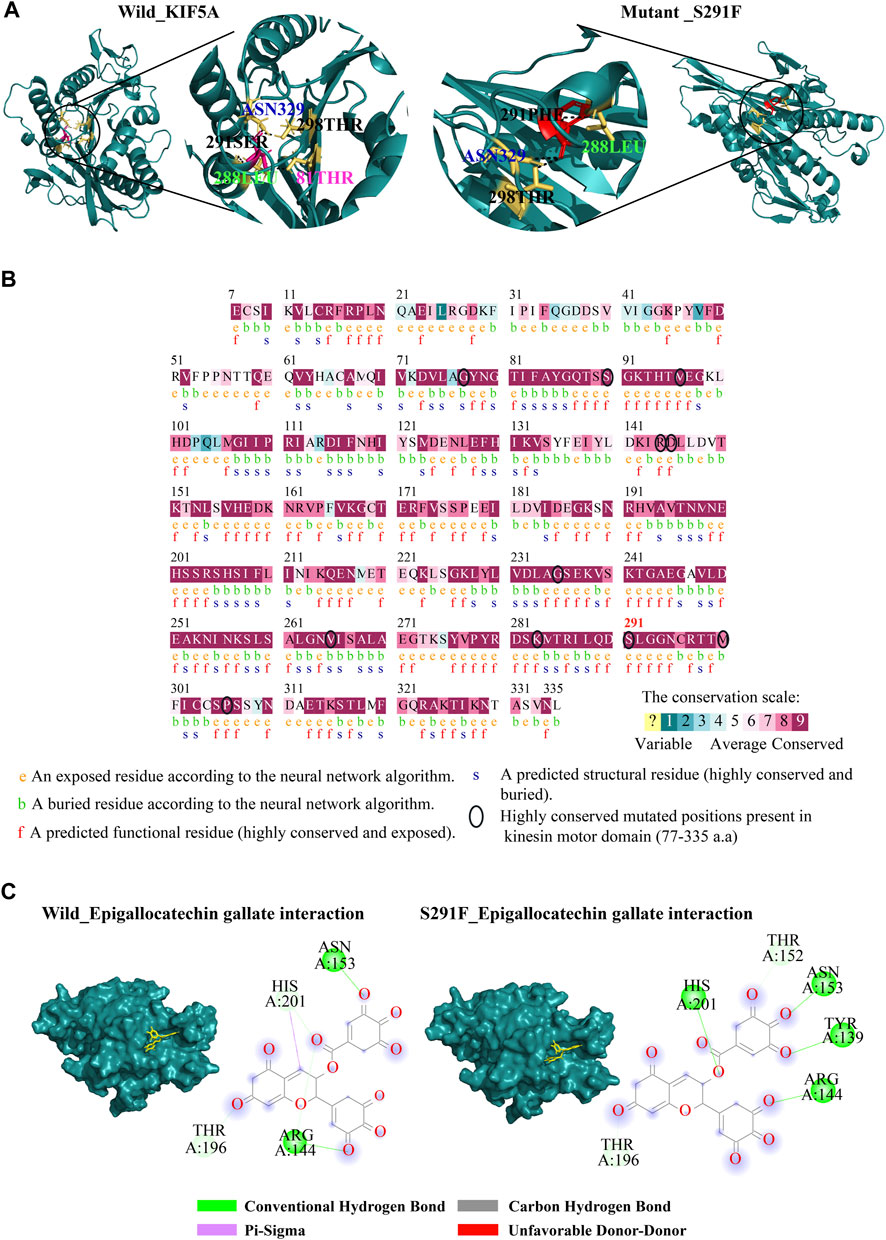
FIGURE 3. Structure analysis of wild-types and mutants. (A) Showing hydrogen bonds formed by wild-type and S291F mutant residues in the kinesin motor domain. The wild-type residue Ser291 (pink stick model) formed four H-bonds with THR81, LEU288, THR298, and ASN329, and the mutant residue PHE291 (red stick model) showed three H-bonds (LEU288, THR298, and ASN329). (B) Highlighting of evolutionary conservation analysis of the positions of mutant residues; the amino acid position is shown in the kinesin motor domain shown in the black circle. (C) Representation of the ligand interaction of epigallocatechin gallate phytocompounds with the kinesin motor domain of wild-type KIF5A and mutant S291F. Epigallocatechin gallate showed four H-bonds with the amino acid residues (TYR139, ARG144, ASN153, and HIS201) as compared to the wild-type ones.
Evolutionary conservation profile
The evolutionary conservation profile was identified for the amino acid position of highly destabilizing SNPs presented in the domain. The conservation scores calculated by this server range from 1 to 9 and discriminate between highly variable and highly conserved positions, respectively. The results included eight positions (77, 90, 96, 235, 265, 283, 291, and 306) with a score of 9 indicating that the pathogenic nsSNPs affect evolutionary conserved positions in the KIF5A protein as compared to the three positions (144, 145, and 300) with a score of 8 (Figure 3B).
Bioactive compounds and molecular docking
We fetched the 24 plant-derived bioactive compounds against ALS. These plant-derived bioactive compounds associated with excitatory amino acid toxicity (β-asarone, Huperzine A, catalpol, selaginellin, ferulic acid, and cryptotanshinone), neuroinflammation (celastrol, resveratrol, curcumin, isorhynchophylline, obovatol, paeonol, and wogonin), calcium cytotoxicity (paeoniflorin, ligustrazine, gastrodin, and muscone), and oxidative stress (madecassoside, ampelopsin, epigallocatechin gallate (EGCG), picroside II, morroniside, astragaloside IV, and diallyl trisulfide) (Table 2). The docking analysis results suggested that epigallocatechin gallate showed the highest binding affinity (−9.0 kcal/mol) toward the KIF5AS291F mutant present in the kinesin motor domain. We observed a comparative binding study with the wild-type KIF5A domain, in which EGCG showed less binding affinity (−8.4 kcal/mol), forming two hydrogen bonds with ARG144 and ASN153 position residues and four H-bonds with ARG144, ASN153, TYR139, and HIS201 residues in case of KIF5AS291F. Formation of a greater number of H-bonds between EGCG and KIF5AS291F provides more binding strength to the complex and makes it more stable. The interacting residues between EGCG with the wild-type and the KIF5AS291F kinesin motor domain are shown in Figure 3C. The binding affinity of other bioactive compounds is shown in Table 2.
KIF5A–PPIN and associated functional modules
In the constructed network, we considered the directly interacting proteins of KIF5A and within interactions between them. The PPIN of KIF5A was visualized using the Cytoscape tool; the PPIN consisted of 272 nodes and 4,353 edges (Figure 4A). Topological properties suggested that KIF5A–PPIN followed a scale-free hierarchical behavior means having clusters of nodes/module organization that could be controlled by the KIF5A protein. We found majorly KIF motor protein associations with each other in the top 10 nodes of parameters, such as degree (KIF5A, KIF5B, KIF5C, and KIF1A), bottleneck (KIF5A, KIF5B, KIF5C, KIF1A, and KIF13B), closeness (KIF5A, KIF5B, KIF5C, and KIF1A), MNC (KIF5A, KIF5B, KIF5C, and KIF1A), and MNC (KIF5A, KIF1A, KIF5B, and KIF5C), parameters calculated by the CytoHubba plugin in Cytoscape (Figure 4B). We found that three KIFs (KIF1A, -5B, and -5C) were the significant proteins common in all four parameters. In the knock-out experiment, while re-examining the network’s topological property change, we found a significant magnitude of changes in network metrics in particular, such as Pk, BC, and NC (Figures 5A–C), respectively. In the local ranking method (Pk and NC) and in the global ranking method (BC), three KIFs (KIF1A, -5B, and -5C) showed a significant change in properties. The significant change in BC could enhance local and global signal propagation throughout the network (Figure 5B). The degree distribution and neighborhood connectivity showed KIFs’ (KIF1A, -5B, and -5C) control over the regulation of PPIN, whereas the removal of other nodes showed a similar pattern of regulation (Figures 5A, C).
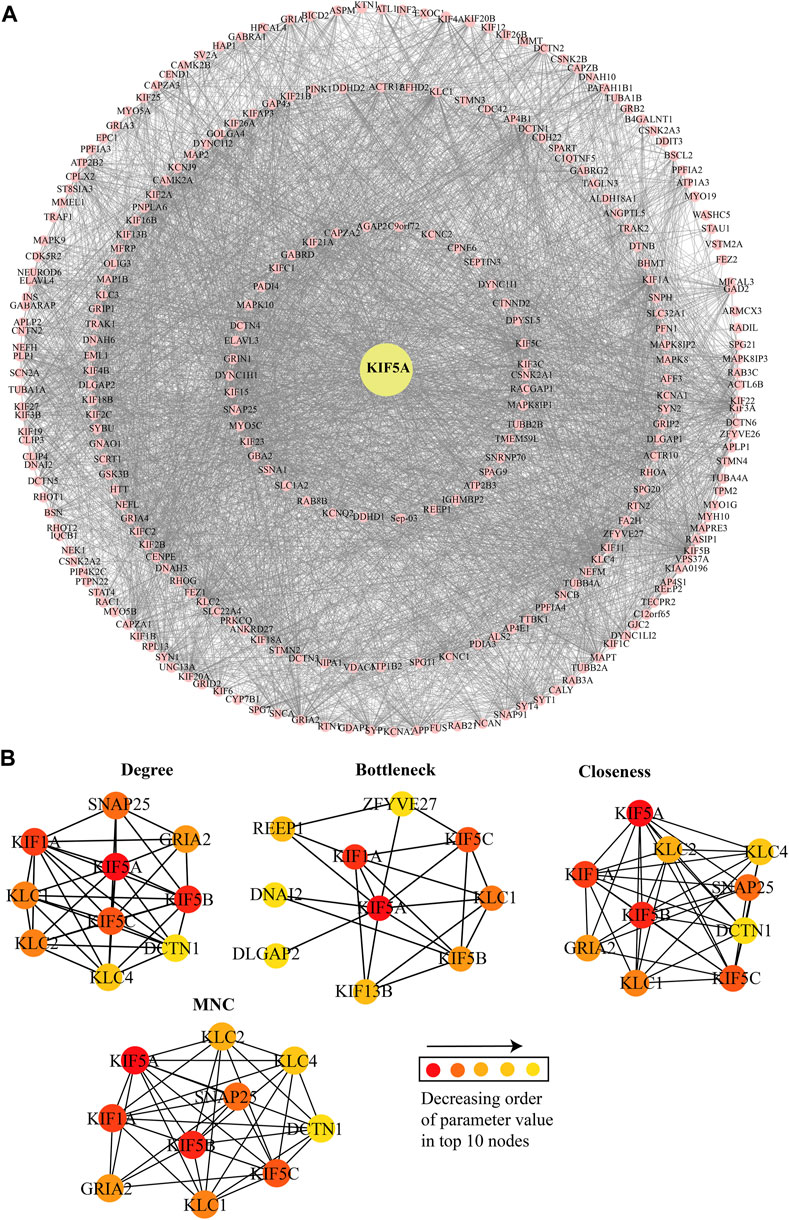
FIGURE 4. KIF5A protein–protein interaction network and its significant KIFs. (A) Protein–protein interaction (PPI) network of KIF5A (yellow-filled circle) with its associated proteins (pink-filled circle). KIF5A–PPIN consisted a total of 272 nodes and 4,353 edges. (B) Representation of top 10 nodes observed in five different network parameters; the color of the nodes in decreasing order of the value of each parameter.
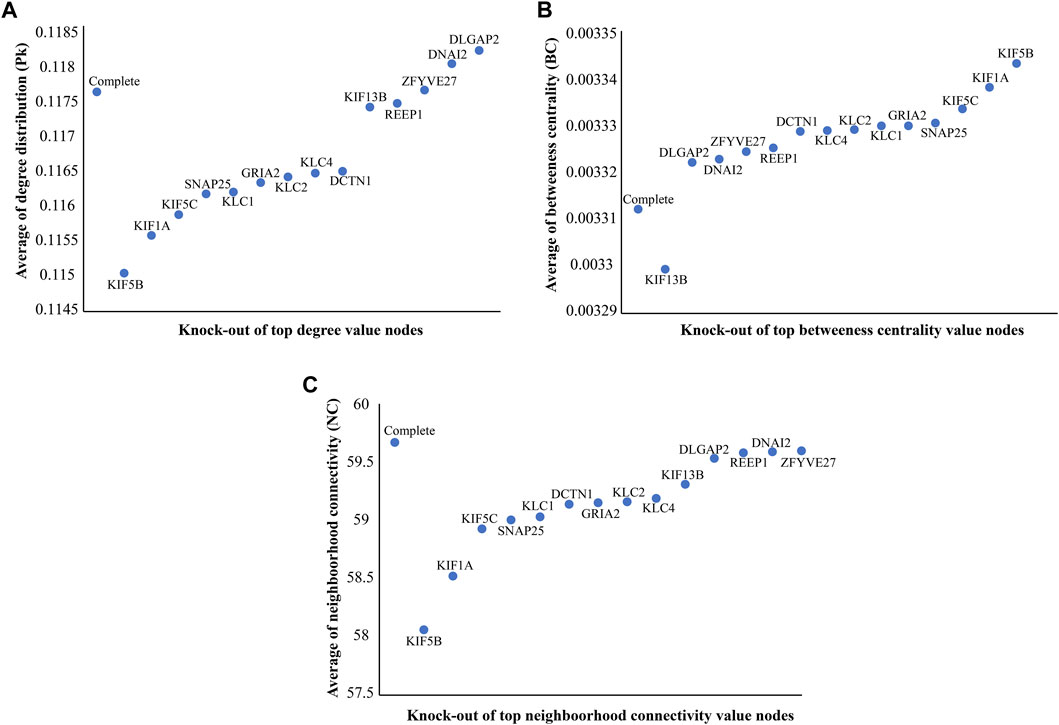
FIGURE 5. Knock-out study to identify significant nodes. (A) Differences of average degree distribution (Pk) values of top value degree nodes with the complete network. (B) Differences of average BC values of top (BC) value nodes with the complete network. (C) Differences of average neighborhood connectivity (NC) values of top (NC) value nodes with the complete network. We found KIFs (KIF1A, -5B, and -5C) allowed significant changes in Pk, BC, and NC, which could enhance local and global signal propagation throughout the network as compared to other nodes.
Furthermore, we considered the top five M-score modules in which the nodes were highly clustered (Figure 6). Module 1, 2, 3, 4, and 5 consisted of 49, 37, 53, 7, and 16 nodes with the corresponding M-scores of 41.70, 18, 17.346, 6.85, and 6, respectively. The identified top five modules may regulate the network to prevent dissortivity and simultaneously maintain the flow of information within the ALS network. We performed functional enrichment analysis of the selected five modules. Module-1 exhibited enrichment in proteins possessing microtubule motor activity and their association with biological processes related to the processing and presentation of exogenous peptide antigens via MHC class II, as well as mitotic spindle organization. These proteins were found to be mostly in microtubules and were cytoskeletal (Figure 7A). Module 2 was enriched in ligand-gated channel activity, glutamate receptor activity, and anterograde trans-synaptic and chemical synapse transmission. These proteins were found mainly in the neuron projection, axon, and dendrites (Figure 7B). Module 3 was associated to neuronic cells and is involved in tubulin binding and GABA-A receptor activity. These proteins were also involved in anterograde trans-synaptic and chemical synapse transmission (Figure 7C). Module-4 protein was associated with protein-arginine deiminase, regulation of intracellular signal transduction, and positive regulation of Toll-like receptor 9 signaling pathway and are mainly found in the cytoplasmic side of the plasma membrane and autophagosomes (Figure 7D). Module-5 proteins were mainly present in microtubules and were cytoskeletal, performing molecular functions such as GTP binding and GTPase activity peptidyl-threonine phosphorylation (Figure 7E).
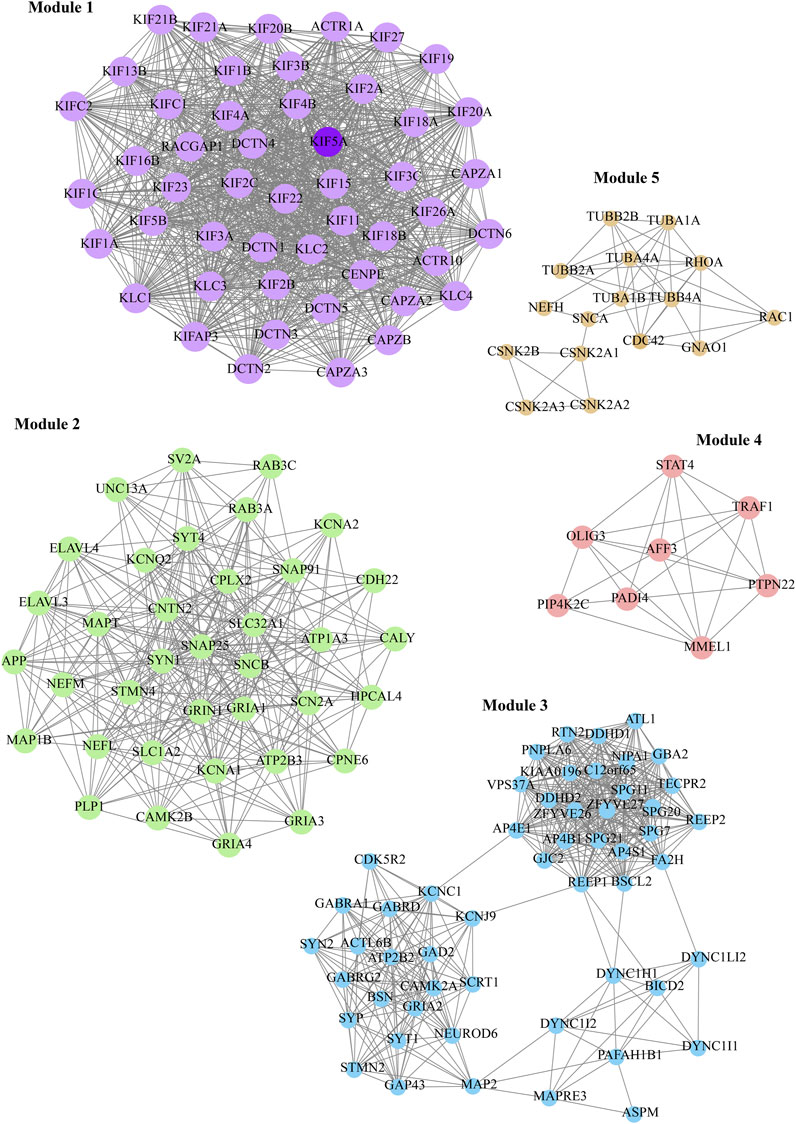
FIGURE 6. Representation of the top five functional modules presented in KIF5A–PPIN, shown in different colors. The KIF5A protein (dark purple color) is presented in the module.
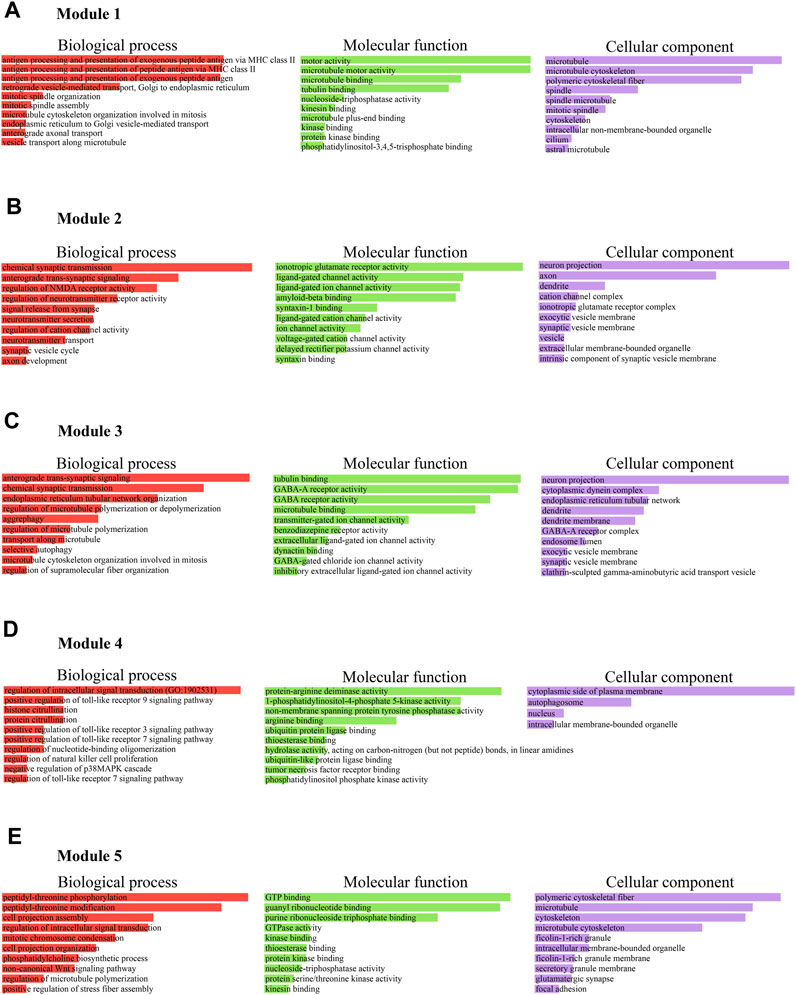
FIGURE 7. (A) Module 1, proteins were found to be mostly in microtubules and cytoskeletal involved in antigen processing, microtubule and motor neuron activities. (B) Module 2, was enriched in ligand-gated channel activity, glutamate receptor activity, and anterograde trans-synaptic and chemical synapse transmission in neuron. (C) Module 3 was associated to neuronic cells and is involved in tubulin binding and GABA-A receptor activity, anterograde trans-synaptic and chemical synapse transmission. (D) Module 4, protein was associated with protein-arginine deiminase, regulation of intracellular signal transduction, and positive regulation of Toll-like receptor 9 signaling pathway and presented in the cytoplasmic side of the plasma membrane and autophagosomes. (E) Module 5 proteins, were mainly present in microtubules and were cytoskeletal, performing molecular functions such as GTP binding and GTPase activity peptidyl-threonine phosphorylation.
Regulatory network of KIF5A
The miRNA-target prediction tools, miRDB v6.0, TargetScan v8.0, starBase v2, and miRmap, predicted 80, 821, 1,055, and 35 miRNA targets of KIF5A, respectively. All these four programs depicted 15 common miRNA (hsa-miR-107, hsa-miR-16-5p, hsa-miR-15a-5p, hsa-miR-17-5p, hsa-miR-20a-5p, hsa-miR-195-5p, hsa-miR-497-5p, hsa-miR-103a-3p, hsa-miR-15b-5p, hsa-miR-93-5p, hsa-miR-106b-5p, hsa-miR-20b-5p, hsa-miR-106a-5p, hsa-miR-503-5p, and hsa-miR-424-5p) targets of KIF5A (Figure 8A). The TF-target prediction tool, NetworkAnalyst tool, predicted 47 TFs regulating KIF5A. The common 15 miRNA–TF relationships predicted by the TransmiR database showed that hsa-miR-107 was upregulated by four TFs (HIF1A, PPARA, SREBF1, and TP53) and downregulated by three TFs (ZEB1, LIN28, and ZEB2) (Figure 8B).
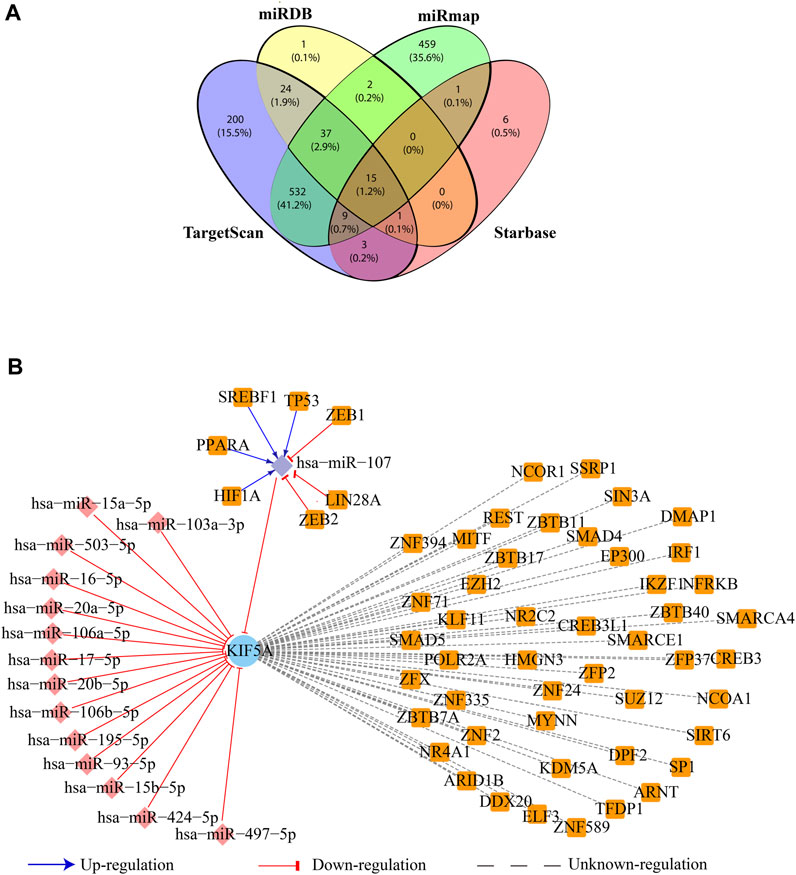
FIGURE 8. Regulatory network of the KIF5A protein. (A) Venn diagram representing the number of common miRNA targets of KIF5A in four databases. (B) Regulatory network of KIF5A with their associated miRNAs (diamond: pink) and transcription factors (square: orange); the mode of upregulation is shown with blue arrows and downregulation in a red T-shape.
Discussion
SNPs, or single-nucleotide polymorphisms, are a pivotal genetic factor contributing to ALS. Specifically, non-synonymous SNPs, which lead to alterations in a single-amino acid residue, play a significant role in ALS and various other neurological disorders. In this study, we employed both structure- and sequence-based computational tools to identify mutations impacting the structure and function of the KIF5A protein, potentially playing a critical role in disease onset. The mutation on the 291st position from the serine to phenylalanine residue was found to be highly destabilizing for the protein structure. The 291st residue exhibited a notably more evolutionary conservation score (Figure 3C), indicating that any mutation occurring at this specific position would likely disrupt the protein’s normal functionality. From this, interestingly, we found the S291F variant was present in a large globular N-terminal domain (kinesin motor domain) (Figure 2D) which may affect the associated protein functions and related pathways in motor neurons. A systems biology approach can be applied to anticipate regulators (such as genes, transcription factors, and microRNAs) relevant to ALS and assess their resilience in preserving self-organized behavior. This approach also helps uncover the complexities of signaling within fundamental cellular processes like cell survival and death, offering insights for developing strategies to understand motor neuron cell function. KIF5A functions as a motor protein responsible for the microtubule-based transport of intracellular organelles, RNA, and proteins (Hirokawa et al., 2010). KIF5A aids in RNA and its binding protein trafficking within the dendritic and axonal area. KIF5A plays a crucial role in facilitating the anterograde transport of mitochondria, aided by adapter proteins like TRAK1/2 and GABARAP. This function is vital for sustaining the capability and proper functions of neurons. Aberrant regulation of mitochondrial transport is linked to multiple neurodegenerative disorders (Sheng and Cai, 2012; Schwarz, 2013). ALS has recently been linked to a mutation in the cargo binding C-end domain region of KIF5A that affects exon-27 splicing (Nicolas et al., 2018). KIF5A plays a pivotal role in axonal transport, shedding light on the possibility that mutations in KIF5A can disrupt this process, thus contributing to the development of motor neuron degeneration (Hirokawa et al., 2010). A mutation in KIF5A could result in the accumulation of neurofilaments and hinder the transport of mitochondria, potentially serving as a hallmark of neurodegenerative diseases (Al-Chalabi et al., 1999; Palomo and Manfredi, 2015; Guo et al., 2017; Smith et al., 2017). Research conducted in zebrafish has revealed that a loss-of-function mutation in the C-terminal region leads to truncation and disrupts the proper axonal localization of mitochondria (Campbell et al., 2014). Within neuronal cells, reduced KIF5A expression and cargo binding lead to the buildup of amyloid precursor proteins and phosphorylated neurofilaments, serving as a distinctive hallmark of neurodegeneration (Hares et al., 2017).
Currently, only two FDA-approved drugs, namely, riluzole and edaravone, are available on the market for the treatment of ALS that provide limited benefits to the patients (Sadr et al., 2021). However, many studies have attempted to create an effective therapeutic target for ALS previously. Most drugs have completed preclinical animal trials, but human clinical trials have had underwhelming results. On the other hand, herbal remedies serve as an additional and alternate kind of treatment for ALS (Mohi-ud-din et al., 2021). The phytochemicals from plant sources, such as flavonoids, alkaloids, terpenes, and saponins, may still have the potential benefits that researchers are seeking because of their distinctive chemical diversity (Zeng et al., 2021; Shareena and Kumar, 2022 Solanki et al., 2015). Therefore, these natural substances are still unexplored as new therapeutic molecules for the treatment of ALS. For ALS, the phytochemicals isolated from medicinal herbs function in a variety of mechanisms, including as an antioxidant, an anti-inflammatory, and an anti-apoptotic agent. The demand for utilizing natural products in the treatment of ALS has grown due to their superior safety and effectiveness in comparison to conventional drugs, and their contribution has a viable alternative for treatment. In this study, the docking analysis showed EGCG bound with mutant S291F with the highest affinity (Table 2). The EGCG phytocompound present in green tea is a radical scavenger that mediates the antioxidant action in a number of neurodegenerative disorders, including ALS. An in vivo study reported that in the SOD1G93A transgenic mice model, epigallocatechin gallate significantly delays the disease onset by 1.4 weeks and prolongs the survival time by 1.8 weeks (Mandel et al., 2004). Epigallocatechin gallate prevented the oxidation stress-induced apoptosis of motor neurons by upregulating survival signals, including phosphatidylinositol 3-kinase, phospho-Akt, and phospho–glycogen synthase kinase-3 (pGSK-3) (Koh et al., 2004). EGCG had an inhibitory effect on GSK-3 activity, one of the important pathogenic mechanisms of an in vitro model of familial ALS (Koh et al., 2005). The presence of ROS and RNS will intensify protein misfolding. Experimental research has emphasized the potential of EGCG to function as an antioxidant, hindering the conversion of nitrate and peroxynitrite into NO. This action is believed to reduce ischemic neuronal harm and safeguard nerve cells (Nagai et al., 2002). Here, our finding suggested that out of the 24 phytocompounds, EGCG binding with KIF5AS291F could be a potential neuroprotective drug target for ALS disease.
The PPIN analysis revealed that KIF5A may be a crucial protein for ALS pathogenesis, controlling the function of the associated functional modules involved majorly in microtubule motor activities in the neuron. The network analysis approach resulted in a number of KIFs (KIF5A, KIF5B, KIF5C, KIF1A, KIF13B, KIF3C, KIF11, KIF18A, and KIF22) associated with KIF5A and was observed in the top 10 nodes for parameters such as degree, bottleneck, closeness, and MNC (Figure 4B). These KIFs are involved in excitatory synaptic transmissions, like KIF13B, and KIF11 regulates synaptic transmission through post-synaptic and/or pre-synaptic mechanisms (Swar et al., 2018). Three KIF5A, KIF5B, and KIF13B transporting cargos, two in microtubule rearrangements (KIF3C and KIF11), and KIF11, a mitotic KIF, are involved in spindle movement. Computational knockout experiment suggested that KIFs (KIF1A, -5B, and -5C) show a significant amount of changes in network properties, particularly in Pk, BC, and NC (Figure 5). These KIFs could enhance local and global signal propagation throughout the network (Figures 5A–C). One study reported that KIF5A conditional knockout mice significantly reduced the amplitude of mIPSCs (Nakajima et al., 2012). Furthermore, the regulatory network analysis showed that KIF5A gene expression can be regulated by both transcriptional and post-transcriptional factors (miRNAs). We found KIF5A appears to be predominantly directly or indirectly co-regulated by a number of TFs and miRNAs. Our regulatory network analysis has revealed that the expression of the KIF5A gene can be regulated at both the transcriptional and post-transcriptional levels, particularly by miRNAs. Our findings indicate that KIF5A is predominantly regulated, either directly or indirectly, by a range of transcription factors and miRNAs. Our analysis suggests that abnormal KIF5A gene expression due to mutations can be directly inhibited by 15 miRNAs (Figure 8B) and indirectly regulated by seven TFs (HIF1A, PPARA, SREBF1, TP53, ZEB1, LIN28, and ZEB2). Specifically, miR-107 directly inhibits the abnormal KIF5A expression, while TFs associated with both the upregulation (HIF1A, PPARA, SREBF1, and TP53) and downregulation (ZEB1, LIN28, and ZEB2) of miR-107 contribute to the indirect control of KIF5A expression.
Conclusion
Understanding the biological effects and mechanisms of action of the newly found phytochemicals can be accomplished by testing compounds using in vitro KIF5AS291F knockout model systems. Further validation of in vitro and in vivo conditions would help us in the identification of key immunological pathways and processes involved in the progression of ALS. In conclusion, the validation of the identified compound EGCG through the appropriate knockout model system (KIF5AS291F) may hold great promise for advancing our understanding of its potential therapeutic applications. As we move forward, it is essential to meticulously select model systems that closely mimic the specific conditions or disease. For instance, in the context of studying EGCG’s anti-cancer effects, the use of cancer cell lines with targeted gene knockouts related to cancer pathways can provide valuable insights (Almatrood et al., 2020). EGCG could serve as a “targeted ALS therapy,” which refers to a new class of medications developed to particularly block KIF5A and its mutations, thought to be essential for ALS development or progression. The generation of transgenic knock-out model organisms (KIF5AS291F) would provide an understanding, development, and pathogenesis of ALS, as well as for the evaluation of possible risk factors, preventive agents, and treatments. In future, experimental approaches may encompass a comprehensive strategy that includes control groups with wild-type and knock-out models, specifically targeting the KIF5A gene. Utilizing the computational analysis of gene knock-outs, we observed substantial alterations in network metrics, especially in degree distribution and betweenness centrality. This significant change in degree distribution and betweenness centrality could enhance local and global signal propagation throughout the network, followed mutually by KIF1A, -5B, and -5C. The association between KIF proteins and knock-out studies of such proteins would provide a basis for the identification of biomarkers that could be used as therapeutic interventions. Finally, this study may help us predict the key molecules (phytocompounds, miRNAs, and TFs), and the molecular interactions between them may provide an opportunity for understanding ALS pathology and therapeutic targets.
Data availability statement
The original contributions presented in the study are included in the article/Supplementary Material; further inquiries can be directed to the corresponding authors.
Author contributions
RK: Conceptualization, Data curation, Methodology, Software, Writing–original draft, Writing–review and editing. TM: Methodology, Visualization, Writing–review and editing. KP: Writing–review and Supervision. HS: Funding acquisition, Supervision, Visualization, Writing–review and editing. SH: Conceptualization, Investigation, Methodology, Supervision, Visualization, Writing–review and editing.
Funding
The author(s) declare that financial support was received for the research, authorship, and/or publication of this article. This research was supported (in part) by research funds from Chosun University, 2023.
Acknowledgments
RK (file no. 09/1132(0009)/2020-EMR-I) is financially supported by the Council of Scientific and Industrial Research (CSIR), India; SH sincerely thanks the Life Science Research Board (LSRB) and the Defence Research and Development Organization (DRDO), Ministry of Defence, New Delhi. MT and HS were provided financial support (in part) by research funds from Chosun University, 2023.
Conflict of interest
The authors declare that the research was conducted in the absence of any commercial or financial relationships that could be construed as a potential conflict of interest.
Publisher’s note
All claims expressed in this article are solely those of the authors and do not necessarily represent those of their affiliated organizations, or those of the publisher, the editors, and the reviewers. Any product that may be evaluated in this article, or claim that may be made by its manufacturer, is not guaranteed or endorsed by the publisher.
References
Adzhubei, I., Jordan, D. M., and Sunyaev, S. R. (2013). Predicting functional effect of human missense mutations using PolyPhen-2. Curr. Protoc. Hum. Genet. 7, Unit7.20. doi:10.1002/0471142905.hg0720s76
Agarwal, V., Bell, G. W., Nam, J. W., and Bartel, D. P. (2015). Predicting effective microRNA target sites in mammalian mRNAs. Elife 4, e05005–e05038. doi:10.7554/eLife.05005
Al-Chalabi, A., Andersen, P. M., Nilsson, P., Chioza, B., Andersson, J. L., Russ, C., et al. (1999). Deletions of the heavy neurofilament subunit tail in amyotrophic lateral sclerosis. Hum. Mol. Genet. 8, 157–164. doi:10.1093/hmg/8.2.157
Almatrood, S. A., Almatroudi, A., Khan, A. A., Alhumaydh, F. A., Alsahl, M. A., and Rahmani, A. H. (2020). Potential therapeutic targets of epigallocatechin gallate (EGCG), the most abundant catechin in green tea, and its role in the therapy of various types of cancer. Molecules 25, 3146. doi:10.3390/molecules25143146
Ashkenazy, H., Abadi, S., Martz, E., Chay, O., Mayrose, I., Pupko, T., et al. (2016). ConSurf 2016: an improved methodology to estimate and visualize evolutionary conservation in macromolecules. Nucleic Acids Res. 44, W344–W350. doi:10.1093/NAR/GKW408
Bertolin, C., Pensato, V., Castellotti, B., Corti, S., Cereda, C., Corrado, L., et al. (2017). HHS Public Access 48, 1037–1042. doi:10.1038/ng.3626.NEK1
Brenner, D., Yilmaz, R., Müller, K., Grehl, T., Petri, S., Meyer, T., et al. (2018). Hot-spot KIF5A mutations cause familial ALS. Brain 141, 688–697. doi:10.1093/brain/awx370
Bromberg, Y., and Rost, B. (2007). SNAP: predict effect of non-synonymous polymorphisms on function. Nucleic Acids Res. 35, 3823–3835. doi:10.1093/nar/gkm238
Campbell, P. D., Shen, K., Sapio, M. R., Glenn, T. D., Talbot, W. S., and Marlow, F. L. (2014). Unique function of kinesin Kif5A in localization of mitochondria in axons. J. Neurosci. 34, 14717–14732. doi:10.1523/JNEUROSCI.2770-14.2014
Capriotti, E., Calabrese, R., Fariselli, P., Martelli, P. L., Altman, R. B., and Casadio, R. (2013). WS-SNPs&GO: a web server for predicting the deleterious effect of human protein variants using functional annotation. BMC Genomics 14 (3), S6. doi:10.1186/1471-2164-14-s3-s6
Capriotti, E., and Fariselli, P. (2017). PhD-SNP g: a webserver and lightweight tool for scoring single nucleotide variants. Nucleic Acids Res. 45, W247–W252. doi:10.1093/nar/gkx369
Carrascoza, F., Zaric, S., and Silaghi-Dumitrescu, R. (2014). Computational study of protein secondary structure elements: ramachandran plots revisited. J. Mol. Graph Model 50, 125–133. doi:10.1016/j.jmgm.2014.04.001
Chen, S., Lv, J., Luo, Y., Chen, H., Ma, S., and Zhang, L. (2023). Bioinformatic analysis of key regulatory genes in adult asthma and prediction of potential drug candidates. Molecules 28, 4100. doi:10.3390/molecules28104100
Chen, X. ,., Reiter, P. L., and McRee, A.-L. (2011). HHS public access. Physiol. Behav. 176, 139–148. doi:10.1016/j.tins.2015.11.001.Roles
Chen, Y., and Wang, X. (2020). MiRDB: an online database for prediction of functional microRNA targets. Nucleic Acids Res. 48, D127–D131. doi:10.1093/nar/gkz757
Chia, R., Chiò, A., and Traynor, B. J. (2018). Novel genes associated with amyotrophic lateral sclerosis: diagnostic and clinical implications. Lancet Neurol. 17, 94–102. doi:10.1016/S1474-4422(17)30401-5
Chin, C. H., Chen, S. H., Wu, H. H., Ho, C. W., Ko, M. T., and Lin, C. Y. (2014). cytoHubba: identifying hub objects and sub-networks from complex interactome. BMC Syst. Biol. 8, S11. doi:10.1186/1752-0509-8-S4-S11
Devon, R. S., Orban, P. C., Gerrow, K., Barbieri, M. A., Schwab, C., Cao, L. P., et al. (2006). Als2-deficient mice exhibit disturbances in endosome trafficking associated with motor behavioral abnormalities. Proc. Natl. Acad. Sci. U. S. A. 103, 9595–9600. doi:10.1073/pnas.0510197103
Ebbing, B., Mann, K., Starosta, A., Jaud, J., Schöls, L., Schüle, R., et al. (2008). Effect of spastic paraplegia mutations in KIF5A kinesin on transport activity. Hum. Mol. Genet. 17, 1245–1252. doi:10.1093/hmg/ddn014
Eberhardt, J., Santos-Martins, D., Tillack, A. F., and Forli, S. (2021). AutoDock vina 1.2.0: new docking methods, expanded force field, and Python bindings. J. Chem. Inf. Model 61, 3891–3898. doi:10.1021/acs.jcim.1c00203
Goizet, C., Boukhris, A., Mundwiller, E., Tallaksen, C., Forlani, S., Toutain, A., et al. (2009). Complicated forms of autosomal dominant hereditary spastic paraplegia are frequent in SPG10. Hum. Mutat. 30, E376–E385. doi:10.1002/humu.20920
Gopalakrishnan, K., Sowmiya, G., Sheik, S. S., and Sekar, K. (2007). Ramachandran plot on the web (2.0). Protein Pept. Lett. 14, 669–671. doi:10.2174/092986607781483912
Gu, Y., Yu, W., Qi, M., Hu, J., Jin, Q., Wang, X., et al. (2023). Identification and validation of hub genes and pathways associated with mitochondrial dysfunction in hypertrophy of ligamentum flavum. Front. Genet. 14, 1117416–1117514. doi:10.3389/fgene.2023.1117416
Guo, W., Naujock, M., Fumagalli, L., Vandoorne, T., Baatsen, P., Boon, R., et al. (2017). HDAC6 inhibition reverses axonal transport defects in motor neurons derived from FUS-ALS patients. Nat. Commun. 8, 861. doi:10.1038/s41467-017-00911-y
Hadano, S., Benn, S. C., Kakuta, S., Otomo, A., Sudo, K., Kunita, R., et al. (2006). Mice deficient in the Rab5 guanine nucleotide exchange factor ALS2/alsin exhibit age-dependent neurological deficits and altered endosome trafficking. Hum. Mol. Genet. 15, 233–250. doi:10.1093/hmg/ddi440
Hardiman, O., Al-Chalabi, A., Chio, A., Corr, E. M., Logroscino, G., Robberecht, W., et al. (2017). Amyotrophic lateral sclerosis. Nat. Rev. Dis. Prim. 3, 17071. doi:10.1038/nrdp.2017.71
Hares, K., Redondo, J., Kemp, K., Rice, C., Scolding, N., and Wilkins, A. (2017). Axonal motor protein KIF5A and associated cargo deficits in multiple sclerosis lesional and normal-appearing white matter. Neuropathol. Appl. Neurobiol. 43, 227–241. doi:10.1111/nan.12305
He, J., Zhang, Z., Ouyang, M., Yang, F., Hao, H., Lamb, K. L., et al. (2016). PTEN regulates EG5 to control spindle architecture and chromosome congression during mitosis. Nat. Commun. 7, 12355–12413. doi:10.1038/ncomms12355
Hirokawa, N., Niwa, S., and Tanaka, Y. (2010). Molecular motors in neurons: transport mechanisms and roles in brain function, development, and disease. Neuron 68, 610–638. doi:10.1016/j.neuron.2010.09.039
Hirokawa, N., Noda, Y., Tanaka, Y., and Niwa, S. (2009). Kinesin superfamily motor proteins and intracellular transport. Nat. Rev. Mol. Cell Biol. 10, 682–696. doi:10.1038/nrm2774
Hogue, C. W., and Groll, M. (2001). An automated method for finding molecular complexes in large protein interaction networks. BMC Bioinforma. 29, 137–140. doi:10.1186/1471-2105-4-2
Hunter, S., Apweiler, R., Attwood, T. K., Bairoch, A., Bateman, A., Binns, D., et al. (2009). InterPro: the integrative protein signature database. Nucleic Acids Res. 37, 211–215. doi:10.1093/nar/gkn785
Jaiswal, M. K. (2019). Riluzole and edaravone: a tale of two amyotrophic lateral sclerosis drugs. Med. Res. Rev. 39, 733–748. doi:10.1002/med.21528
Jeong, H., Mason, S. P., Barabási, A. L., and Oltvai, Z. N. (2001). Lethality and centrality in protein networks. Nature 411, 41–42. doi:10.1038/35075138
Jiang, J. S., Wang, Y., and Deng, M. (2022). New developments and opportunities in drugs being trialed for amyotrophic lateral sclerosis from 2020 to 2022. Front. Pharmacol. 13, 1054006. doi:10.3389/fphar.2022.1054006
Kamal, A., Almenar-Queralt, A., LeBlanc, J. F., Roberts, E. A., and Goldstein, L. S. B. (2001). Kinesin-mediated axonal transport of a membrane compartment containing β-secretase and presenilin-1 requires APP. Nature 414, 643–648. doi:10.1038/414643a
Koh, S. H., Kwon, H., Kim, K. S., Kim, J., Kim, M. H., Yu, H. J., et al. (2004). Epigallocatechin gallate prevents oxidative-stress-induced death of mutant Cu/Zn-superoxide dismutase (G93A) motoneuron cells by alteration of cell survival and death signals. Toxicology 202, 213–225. doi:10.1016/j.tox.2004.05.008
Koh, S.-H., Lee, Y.-B., Kim, K. S., Kim, H.-J., Kim, M., Lee, Y. J., et al. Role of GSK-3beta activity in motor neuronal cell death induced by G93A or A4V mutant hSOD1 gene. Eur. J. Neurosci. 2005;22:301–309.doi:10.1111/j.1460-9568.2005.04191.x
Krieger, E., Joo, K., Lee, J., Lee, J., Raman, S., Thompson, J., et al. (2009). Improving physical realism, stereochemistry, and side-chain accuracy in homology modeling: four approaches that performed well in CASP8. Proteins Struct. Funct. Bioinforma. 77 9, 114–122. doi:10.1002/prot.22570
Kunz, P. A., Roberts, A. C., and Philpot, B. D. (2013). Presynaptic NMDA receptor mechanisms for enhancing spontaneous neurotransmitter release. J. Neurosci. 33, 7762–7769. doi:10.1523/JNEUROSCI.2482-12.2013
Lalwani, A. K., Krishnan, K., Bagabir, S. A., Alkhanani, M. F., Almalki, A. H., Haque, S., et al. (2022). Network theoretical approach to explore factors affecting signal propagation and stability in dementia’s protein-protein interaction network. Biomolecules 12, 451–524. doi:10.3390/biom12030451
Laskowski, R. A., Jabłońska, J., Pravda, L., Vařeková, R. S., and Thornton, J. M. (2018). PDBsum: structural summaries of PDB entries. Protein Sci. 27, 129–134. doi:10.1002/pro.3289
Laskowski, R. A., MacArthur, M. W., Moss, D. S., and Thornton, J. M. (1993). PROCHECK: a program to check the stereochemical quality of protein structures. J. Appl. Crystallogr. 26, 283–291. doi:10.1107/S0021889892009944
Li, J. H., Liu, S., Zhou, H., Qu, L. H., and Yang, J. H. (2014). StarBase v2.0: decoding miRNA-ceRNA, miRNA-ncRNA and protein-RNA interaction networks from large-scale CLIP-Seq data. Nucleic Acids Res. 42, 92–97. doi:10.1093/nar/gkt1248
Liu, Y. T., Laura, M., Hersheson, J., Horga, A., Jaunmuktane, Z., Brandner, S., et al. (2014). Extended phenotypic spectrum of KIF5A mutations: from spastic paraplegia to axonal neuropathy. Neurology 83, 612–619. doi:10.1212/WNL.0000000000000691
López-Ferrando, V., Gazzo, A., De La Cruz, X., Orozco, M., and Gelpí, J. L. (2017). PMut: a web-based tool for the annotation of pathological variants on proteins, 2017 update. Nucleic Acids Res. 45, W222–W228. doi:10.1093/nar/gkx313
Malik, M. Z., Chirom, K., Ali, S., Ishrat, R., Somvanshi, P., and Singh, R. K. B. (2019). Methodology of predicting novel key regulators in ovarian cancer network: a network theoretical approach. BMC Cancer 19, 1129. doi:10.1186/s12885-019-6309-6
Mandel, S., Weinreb, O., Amit, T., and Youdim, M. B. H. (2004). Cell signaling pathways in the neuroprotective actions of the green tea polyphenol (-)-epigallocatechin-3-gallate: implications for neurodegenerative diseases. J. Neurochem. 88, 1555–1569. doi:10.1046/j.1471-4159.2003.02291.x
Matheus, L., Oliveira, G. D., Barreto, R., Juciele, C., Ribeiro, V., Trias, E., et al. (2023). Impact of plant - derived compounds on amyotrophic lateral sclerosis. Neurotox. Res. 41, 288–309. doi:10.1007/s12640-022-00632-1
Miki, H., Setou, M., Kaneshiro, K., and Hirokawa, N. (2001). All kinesin superfamily protein, KIF, genes in mouse and human. Proc. Natl. Acad. Sci. U. S. A. 98, 7004–7011. doi:10.1073/pnas.111145398
Miller, T. M., Cudkowicz, M. E., Genge, A., Shaw, P. J., Sobue, G., Bucelli, R. C., et al. (2022). Trial of antisense oligonucleotide tofersen for SOD1 ALS. N. Engl. J. Med. 387, 1099–1110. doi:10.1056/nejmoa2204705
Mohi-ud-din, R., Mir, R. H., Shah, A. J., Sabreen, S., Wani, T. U., Masoodi, M. H., et al. (2021). Plant-derived natural compounds for the treatment of amyotrophic lateral sclerosis: an update. Curr. Neuropharmacol. 20, 179–193. doi:10.2174/1570159x19666210428120514
Morais, S., Raymond, L., Mairey, M., Coutinho, P., Brandão, E., Ribeiro, P., et al. (2017). Massive sequencing of 70 genes reveals a myriad of missing genes or mechanisms to be uncovered in hereditary spastic paraplegias. Eur. J. Hum. Genet. 25, 1217–1228. doi:10.1038/ejhg.2017.124
Nafis, S., Kalaiarasan, P., Singh, R. K. B., Husain, M., and Bamezai, R. N. K. (2014). Apoptosis regulatory protein-protein interaction demonstrates hierarchical scale-free fractal network. Brief. Bioinform 16, 675–699. doi:10.1093/bib/bbu036
Nagai, K., Jiang, M. H., Hada, J., Nagata, T., Yajima, Y., Yamamoto, S., et al. (2002). (−)-Epigallocatechin gallate protects against NO stress-induced neuronal damage after ischemia by acting as an anti-oxidant. Brain Res. 956, 319–322. doi:10.1016/S0006-8993(02)03564-3
Nakagawa, T., Tanaka, Y., Matsuoka, E., Kondo, S., Okada, Y., Noda, Y., et al. (1997). Identification and classification of 16 new kinesin superfamily (KIF) proteins in mouse genome. Proc. Natl. Acad. Sci. U. S. A. 94, 9654–9659. doi:10.1073/pnas.94.18.9654
Nakajima, K., Yin, X., Takei, Y., Seog, D. H., Homma, N., and Hirokawa, N. (2012). Molecular motor KIF5A is essential for GABAA receptor transport, and KIF5A deletion causes epilepsy. Neuron 76, 945–961. doi:10.1016/j.neuron.2012.10.012
Ng, P. C., and Henikoff, S. (2003). SIFT: predicting amino acid changes that affect protein function. function 31, 3812–3814. doi:10.1093/nar/gkg509
Nicolas, A., Kenna, K., Renton, A. E., Ticozzi, N., Faghri, F., Chia, R., et al. (2018). Genome-wide analyses identify KIF5A as a novel ALS gene. Neuron 97, 1268–1283.e6. doi:10.1016/j.neuron.2018.02.027
Ogbonmide, T., Rathore, R., Rangrej, S. B., Hutchinson, S., Lewis, M., Ojilere, S., et al. (2023). Gene therapy for spinal muscular atrophy (SMA): a review of current challenges and safety considerations for Onasemnogene Abeparvovec (zolgensma). Cureus 15, e36197–7. doi:10.7759/cureus.36197
Palomo, G. M., and Manfredi, G. (2015). Exploring new pathways of neurodegeneration in ALS: the role of mitochondria quality control. Brain Res. 1607, 36–46. doi:10.1016/j.brainres.2014.09.065
Peng, X., Wang, J., Wang, J., Wu, F. X., and Pan, Y. (2015). Rechecking the centrality-lethality rule in the scope of protein subcellular localization interaction networks. PLoS One 10, 01307433–e130822. doi:10.1371/journal.pone.0130743
Reid, E., Kloos, M., Ashley-Koch, A., Hughes, L., Bevan, S., Svenson, I. K., et al. (2002). A kinesin heavy chain (KIF5A) mutation in hereditary spastic paraplegia (SPG10). Am. J. Hum. Genet. 71, 1189–1194. doi:10.1086/344210
Roy, S., Lee, V. M. Y., and Trojanowski, J. Q. (2010). Axonal transport and neurodegenerative diseases. Encycl. Neurosci. 1762, 1199–1203. doi:10.1016/B978-008045046-9.00714-2
Sadr, A. S., Eslahchi, C., Ghassempour, A., and Kiaei, M. (2021). In silico studies reveal structural deviations of mutant profilin-1 and interaction with riluzole and edaravone in amyotrophic lateral sclerosis. Sci. Rep. 11, 6849–6914. doi:10.1038/s41598-021-86211-4
Schwarz, T. L. (2013). Mitochondrial trafficking in neurons. Cold Spring Harb. Perspect. Med. 5, a011304. doi:10.1101/cshperspect.a011304
Schymkowitz, J., Borg, J., Stricher, F., Nys, R., Rousseau, F., and Serrano, L. (2005). The FoldX web server: an online force field. Nucleic Acids Res. 33, W382–W388. doi:10.1093/nar/gki387
Sekine, Y., Okada, Y., Noda, Y., Kondo, S., Aizawa, H., Takemura, R., et al. (1994). A novel microtubule-based motor protein (KIF4) for organelle transports, whose expression is regulated developmentally. J. Cell Biol. 127, 187–201. doi:10.1083/jcb.127.1.187
Shannon, P., Markiel, A., Ozier, O., Baliga, N. S., Wang, J. T., Ramage, D., et al. (2003). Cytoscape: a software Environment for integrated models of biomolecular interaction networks. Genome Res. 13, 2498–2504. doi:10.1101/gr.1239303
Shareena, G., and Kumar, D. (2022). Biomedicine & Pharmacotherapy Traversing through half a century research timeline on Ginkgo biloba, in transforming a botanical rarity into an active. Funct. food Ingred. 153, 0–2.
Sheng, Z. H., and Cai, Q. (2012). Mitochondrial transport in neurons: impact on synaptic homeostasis and neurodegeneration. Nat. Rev. Neurosci. 13, 77–93. doi:10.1038/nrn3156
Smith, E. F., Shaw, P. J., and De Vos, K. J. (2017). The role of mitochondria in amyotrophic lateral sclerosis. Neurosci. Lett. 710, 132933. doi:10.1016/j.neulet.2017.06.052
Smith, M. J., Pozo, K., Brickley, K., and Stephenson, F. A. (2006). Mapping the GRIF-1 binding domain of the kinesin, KIF5C, substantiates a role for GRIF-1 as an adaptor protein in the anterograde trafficking of cargoes. J. Biol. Chem. 281, 27216–27228. doi:10.1074/jbc.M600522200
Solanki, I., Parihar, P., Mansuri, M. L., and Parihar, M. S. (2015). Flavonoid-based therapies in the early management of neurodegenerative diseases. Adv. Nutr. 6, 64–72. doi:10.3945/an.114.007500
Su, L. N., Song, X. Q., Xue, Z. X., Zheng, C. Q., Yin, H. F., and Wei, H. P. (2018). Network analysis of microRNAs, transcription factors, and target genes involved in axon regeneration. J. Zhejiang Univ. Sci. B 19, 293–304. doi:10.1631/jzus.B1700179
Swarnkar, S., Avchalumov, Y., Raveendra, B. L., Grinman, E., and Puthanveettil, S. V. (2018). Kinesin family of proteins Kif11 and Kif21B act as inhibitory constraints of excitatory synaptic transmission through distinct mechanisms. Sci. Rep. 8, 17419–17516. doi:10.1038/s41598-018-35634-7
Szklarczyk, D., Gable, A. L., Lyon, D., Junge, A., Wyder, S., Huerta-Cepas, J., et al. (2019). STRING v11: protein-protein association networks with increased coverage, supporting functional discovery in genome-wide experimental datasets. Nucleic Acids Res. 47, D607–D613. doi:10.1093/nar/gky1131
Takayama, Y., Akagi, Y., and Kida, Y. S. (2023). Deciphering the molecular mechanisms of autonomic nervous system neuron induction through integrative bioinformatics analysis. Int. J. Mol. Sci. 24, 9053. doi:10.3390/ijms24109053
Tang, H., and Thomas, P. D. (2016). PANTHER-PSEP: predicting disease-causing genetic variants using position-specific evolutionary preservation. Bioinformatics 32, 2230–2232. doi:10.1093/bioinformatics/btw222
Valko, K., and Ciesla, L. (2019). Amyotrophic lateral sclerosis. Prog. Med. Chem. 58, 63–117. doi:10.1016/bs.pmch.2018.12.001
Van Rheenen, W., Shatunov, A., Dekker, A. M., McLaughlin, R. L., Diekstra, F. P., Pulit, S. L., et al. (2016). Genome-wide association analyses identify new risk variants and the genetic architecture of amyotrophic lateral sclerosis. Nat. Genet. 48, 1043–1048. doi:10.1038/ng.3622
Vejnar, C. E., and Zdobnov, E. M. (2012). MiRmap: comprehensive prediction of microRNA target repression strength. Nucleic Acids Res. 40, 11673–11683. doi:10.1093/nar/gks901
Venselaar, H., te Beek, T. A. H., Kuipers, R. K. P., Hekkelman, M. L., and Vriend, G. (2010). Protein structure analysis of mutations causing inheritable diseases. An e-Science approach with life scientist friendly interfaces. BMC Bioinforma. 11, 548. doi:10.1186/1471-2105-11-548
Vriend, G. (1990). WHAT IF: a molecular modeling and drug design program. J. Mol. Graph 8 (29), 52–56. doi:10.1016/0263-7855(90)80070-v
Wang, N., and Xu, J. (2015). Functions of kinesin superfamily proteins in neuroreceptor trafficking. Biomed. Res. Int. 2015, 639301. doi:10.1155/2015/639301
Wang, R., and Reddy, H. (2017). Role of glutamate and NMDA receptors in Alzheimer's disease. J. Alzheimer’s Dis. 57, 1041–1048. doi:10.3233/JAD-160763
Wu, J., and Jiang, R. (2013). Prediction of deleterious nonsynonymous single-nucleotide polymorphism for human diseases. Sci. World Journa1 2013, 675851. doi:10.1155/2013/675851
Xie, Z., Bailey, A., Kuleshov, M. V., Clarke, D. J. B., Evangelista, J. E., Jenkins, S. L., et al. (2021). Gene set knowledge discovery with enrichr. Curr. Protoc. 1, e90. doi:10.1002/cpz1.90
Yang, J., Yan, R., Roy, A., Xu, D., Poisson, J., and Zhang, Y. (2014). The I-TASSER suite: protein structure and function prediction. Nat. Methods 12, 7–8. doi:10.1038/nmeth.3213
Yates, C. M., Filippis, I., Kelley, L. A., and Sternberg, M. J. E. (2014). SuSPect: enhanced prediction of single amino acid variant (SAV) phenotype using network features. J. Mol. Biol. 426, 2692–2701. doi:10.1016/j.jmb.2014.04.026
Yue, P., and Moult, J. (2006). Identification and analysis of deleterious human SNPs. J. Mol. Biol. 356, 1263–1274. doi:10.1016/j.jmb.2005.12.025
Zeng, P., Fang, M., Zhao, H., and Guo, J. (2021). A network pharmacology approach to uncover the key ingredients in Ginkgo Folium and their anti-Alzheimer ’ s disease mechanisms. Aging (Albany NY). 13, 18993–19012. doi:10.18632/aging.203348
Zhang, Y., Chen, H., Li, R., Sterling, K., and Song, W. (2023). Amyloid β-based therapy for Alzheimer’s disease: challenges, successes and future. Signal Transduct. Target Ther. 8, 248–326. doi:10.1038/s41392-023-01484-7
Keywords: neurodegenerative diseases, amyotrophic lateral sclerosis, kinesin family member 5A, nonsynonymous single-nucleotide polymorphisms, protein-protein interaction, phytochemicals, miRNA, transcription factor
Citation: Kumar R, Madhavan T, Ponnusamy K, Sohn H and Haider S (2023) Computational study of the motor neuron protein KIF5A to identify nsSNPs, bioactive compounds, and its key regulators. Front. Genet. 14:1282234. doi: 10.3389/fgene.2023.1282234
Received: 23 August 2023; Accepted: 24 October 2023;
Published: 10 November 2023.
Edited by:
Gurmeet Kaur, Corteva Agriscience LLC, United StatesReviewed by:
Maurice HT Ling, Temasek Polytechnic, SingaporeRonak Loonawat, Wuxi Advanced Therapeutics, Inc., United States
Copyright © 2023 Kumar, Madhavan, Ponnusamy, Sohn and Haider. This is an open-access article distributed under the terms of the Creative Commons Attribution License (CC BY). The use, distribution or reproduction in other forums is permitted, provided the original author(s) and the copyright owner(s) are credited and that the original publication in this journal is cited, in accordance with accepted academic practice. No use, distribution or reproduction is permitted which does not comply with these terms.
*Correspondence: Honglae Sohn, hsohn@chosun.ac.kr; Shazia Haider, shaider1@jmi.ac.in
†ORCID: Rupesh Kumar, orcid.org/0000-0002-1260-6216; Shazia Haider, orcid.org/0000-0002-1237-4400