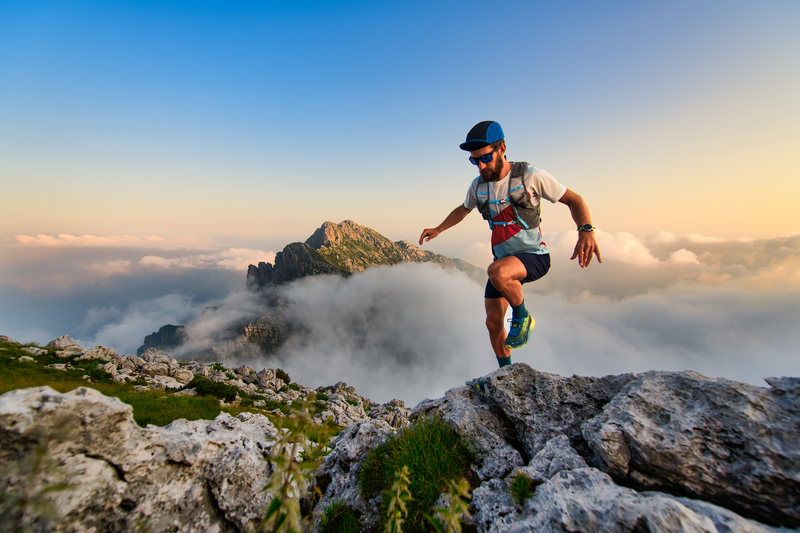
94% of researchers rate our articles as excellent or good
Learn more about the work of our research integrity team to safeguard the quality of each article we publish.
Find out more
EDITORIAL article
Front. Genet. , 26 July 2023
Sec. Computational Genomics
Volume 14 - 2023 | https://doi.org/10.3389/fgene.2023.1256025
This article is part of the Research Topic Network Bioscience Volume II View all 14 articles
Editorial on the Research Topic
Network bioscience Volume II
Network biology is based on the intuition that the quantitative modeling and algorithmic tools of network theory offer new possibilities to understand, model, and simulate the cell’s internal organization and evolution, fundamentally altering our view of cell biology. As network biology has been gaining ground and recognition in the last 20 years, the scope of its application, while still well grounded in molecular biology and genetics, has moved steadily from tackling fundamental biological questions towards translational medicine, including modeling of diseases and applications in drug design and drug action prediction.
This Research Topic Network Bioscience Vol II follows in the track of the first one Network Bioscience completed in 2019 (Antoniotti et al., 2019), and it aims at collecting cutting-edge research on the many guises of network bioscience.
The papers contained in the present Research Topic are examples of how network and graph analysis can be used to elucidate various aspects of biological systems from inferring missing annotations, handling heterogeneous data types, including the vast literature available online, understanding metabolic dynamics, phenotype-genotype linking, to relationships assessment among diverse omics data for drug design and drug repositioning, to a deeper understanding of modularity in gene networks.
Among the recent trends with a potential of high impact, a most notable one is the incorporation of causality considerations and concepts within the classical network models so to make better use of perturbation data that are currently not exploited to their full potential. In particular such hybrid causal network models help bridging the gap between descriptive and actionable network models, the former successfully describe biological systems as they are, the latter allows us to formulate questions and find answers within the vast scope of what-if, counterfactual, worlds.
The papers collected in this Research Topic are roughly grouped as follows:
• “Foundational” papers,
• Analysis of particular biomedical problems,
• Algorithms and Tools.
Five foundational papers in this collection tackle in innovative ways basic issues in the mathematical modeling of bio-networks, covering an overview of relevant modularity concepts, to incorporating causality and biologically plausible sparsity assumptions in gene regulatory networks (GRNs), as well as empirically-found motif sub-network distributions.
Alcalá-Corona et al. bridge a notable gap between the perspective on community detection and network modularity derived from statistical physics and network science on the one hand, and its adaptation and application in biological research, on the other hand.
Maheshwari et al. present a general biological network inference method that combines the discovery of a parsimonious network structure and the identification of Boolean functions that determine the dynamics of the system. The method uses a causal logic framework to assimilate indirect information obtained from perturbation experiments and infer relationships that have not yet been documented experimentally.
Seçilmiş et al. note that many gene regulatory networks (GRNs) use sparsity definitions that are independent of other relevant biological properties expected from GRNs. They thus provide a general approach for identifying GRN that are both biologically accurate and structurally sparse GRN, within the entire space of possible GRNs, by selection criteria based on Akaike and Bayesian Information Criterion (AIC and BIC) adapted to the task of GRN inference.
Zhivkoplias et al. developed a novel motif-based preferential attachment algorithm, FFLatt, that aims at constructing a gene-proteins gene-regulatory network (GRN) rich in feed-forward loop (FFL) which are network motifs known to be significantly enriched in experimentally validated GRN.
In cancer driver gene identification, it is often assumed that a driver mutation is less likely to occur in case of an earlier mutation that has common functionality in the same molecular pathway (mutual exclusivity—ME). Ahmed et al. note that the current mutual exclusivity tests lack a network-centric view and thus fail to model key aspects of the problem. Thus they propose a network-centric framework to evaluate the pairwise significance values found by statistical ME tests and correct potential biases.
Two articles apply network techniques in the area of optimization of antibody design for drug design and to challenging modeling of the immune system’s role for a specific relevant complex condition (human infertility).
In the context of SARS-CoV-2 studies, Gross and Sharan tackle a fundamental problem of growing importance for antibody design that is the identification of mutations of concern of the Spike protein with high escape probability.
Taraschi et al. use a network-based approach to explore the etiopathogenic mechanisms involved in human hypofertility and infertility, aiming at understanding the involvement of the immune system.
Several of the articles describe advances in designing and providing the scientific community with increasingly powerful tools and algorithms capable of making the best use of the vast amount of heterogeneous and often noisy and incomplete biological data available from online repositories.
Castresana-Aguirre et al. note that when analyzing the association between a gene set and a pathway an issue that is generally ignored is that gene sets often represent multiple pathways. They experimentally found that pre-clustering of genes can be beneficial in this association studies by increasing the sensitivity of pathway analysis methods and by providing deeper insights into biological mechanisms related to the phenotype under study.
Di Maria et al. introduce BioTAGME, a system for the inference of novel knowledge and new hypotheses from the current biomedical literature analysis by constructing an extensive Knowledge Graph modeling relations among biological terms and phrases extracted from titles and abstracts of papers available in PubMed.
Semantic knowledge graphs (KGs) are increasingly used to combine unstructured human-curated full-text literature data and structured gene expression data from biomedical databases. In this area, Gurbuz et al. here demonstrate how KGs can be used to find new indications for existing drug targets in order to accelerate the process of launching a new drug for a disease on the market.
Often newly sequenced prokaryotic genomes have poor initial gene functional annotation and missing metabolism pathway gene assignments. To counter these shortcomings, Lu et al. developed PPA-GCN, a prokaryotic pathways assignment framework based on graph convolutional network, to assist functional pathway assignments.
Galvão Ferrarini et al. describe a novel tool, Totoro, that aims at predicting the metabolic reactions that are most likely active during the transient states of a metabolic network as a result of network perturbation simulations.
Zhao et al. propose a computational method called LncPNet to predict potential lncRNA–protein interactions based on spatial embedding a lncRNA–protein heterogeneous network into a collection of low-dimensional latent representations.
All authors listed have made a substantial, direct, and intellectual contribution to the work and approved it for publication.
This work was partially supported by the CRUK/AIRC/FC-AECC Accelerator Award #22790, Single Cell Cancer Evolution in the Clinic, by the FAQC 2021 Competitive Funding Program of the Università degli Studi di Milano-Bicocca, Milan, Italy, and by the European Commission PPPA-AIPC-2021 project “Towards an UNIque approach for artificial intelligence data-driven solutions to fight Childhood cAncer FOR EUrope,” LC-01815952/101052609, UNICA4EU. This work has been partially supported with co-funding of the European Union—Next-Generation EU, in the context of the National Recovery and Resilience Plan, Investment 1.5 Ecosystems of Innovation, Project Tuscany Health Ecosystem (THE), CUP: B83C22003920001. This work has been partially supported by the National Cancer Institute Physical Sciences-Oncology Center Grant U54 CA193313-01.
The authors declare that the research was conducted in the absence of any commercial or financial relationships that could be construed as a potential conflict of interest.
All claims expressed in this article are solely those of the authors and do not necessarily represent those of their affiliated organizations, or those of the publisher, the editors and the reviewers. Any product that may be evaluated in this article, or claim that may be made by its manufacturer, is not guaranteed or endorsed by the publisher.
Keywords: network bioscience, gene regulatory networks, causal networks, knowledge graphs, metabolic graph models
Citation: Antoniotti M, Mishra B and Pellegrini M (2023) Editorial: Network bioscience Volume II. Front. Genet. 14:1256025. doi: 10.3389/fgene.2023.1256025
Received: 10 July 2023; Accepted: 17 July 2023;
Published: 26 July 2023.
Edited and reviewed by:
Richard D. Emes, Nottingham Trent University, United KingdomCopyright © 2023 Antoniotti, Mishra and Pellegrini. This is an open-access article distributed under the terms of the Creative Commons Attribution License (CC BY). The use, distribution or reproduction in other forums is permitted, provided the original author(s) and the copyright owner(s) are credited and that the original publication in this journal is cited, in accordance with accepted academic practice. No use, distribution or reproduction is permitted which does not comply with these terms.
*Correspondence: Marco Pellegrini, bWFyY28ucGVsbGVncmluaUBpaXQuY25yLml0
Disclaimer: All claims expressed in this article are solely those of the authors and do not necessarily represent those of their affiliated organizations, or those of the publisher, the editors and the reviewers. Any product that may be evaluated in this article or claim that may be made by its manufacturer is not guaranteed or endorsed by the publisher.
Research integrity at Frontiers
Learn more about the work of our research integrity team to safeguard the quality of each article we publish.