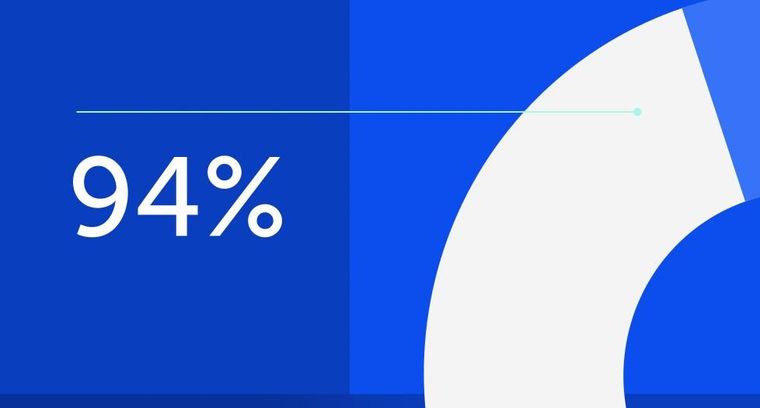
94% of researchers rate our articles as excellent or good
Learn more about the work of our research integrity team to safeguard the quality of each article we publish.
Find out more
EDITORIAL article
Front. Genet., 19 June 2023
Sec. Computational Genomics
Volume 14 - 2023 | https://doi.org/10.3389/fgene.2023.1233078
This article is part of the Research TopicMachine Learning Methods in Single-Cell Immune and Drug Response PredictionView all 6 articles
Editorial on the Research Topic
Machine learning methods in single-cell immune and drug response prediction
Machine learning methods play a crucial role in single-cell immune and drug response prediction. This field of research aims to understand the heterogeneity and complexity of the immune system at the single-cell level and how it interacts with drugs or therapies. By leveraging machine learning techniques, scientists can extract meaningful insights from high-dimensional and large-scale single-cell data, leading to improved understanding and prediction of immune responses to drugs.
Given that single-cell sequencing is suited to investigating cell-type-specific patterns of response to therapy, this Research Topic explores the potential for machine learning applied to characterize the single-cell transcriptomes of cancer and immune cells. We are pleased to accept the following five papers with the new sight of machine learning techniques selected from all submissions.
Machine learning methods facilitate the integration of single-cell data with other omics data types, such as bulk RNA-seq, proteomics, or epigenomics. To identify cell types in complex tissues and integrate reference-bulk RNA sequencing data and single-cell transcriptomes, Liu et al. present a linear fast semi-supervised clustering model. Integrative analysis can uncover multi-level relationships, identify regulatory mechanisms, and provide a more comprehensive view of immune responses and drug actions.
Two papers concentrate on cell type identification in this Research Topic. Cell-type identification involves the classification and annotation of individual cells into distinct cell types or cell states based on their gene expression profiles. It is crucial for understanding cellular heterogeneity, uncovering developmental processes, discovering novel cell types, linking cell types to functions, enabling comparative analyses, and integrating data across multiple modalities in scRNA-seq data analysis. Song et al. identified cell types for scRNA-seq based on a deep-learning transformer. Their innovative use of transformer annotation cells not only improves accuracy, but also forms the foundation for further downstream analyses and interpretation, ultimately leading to a deeper understanding of cellular biology, disease mechanisms, and therapeutic strategies. In addition, Li et al. employed a progressive clustering method to identify cell populations in scRNA-seq data, they described data with clustering trees and revealed the structure of both abundant cell populations and rare cell populations. Single-cell data can capture dynamic changes in cellular states during immune responses or drug treatments. Meanwhile, machine learning methods enable the characterization of developmental processes, lineage relationships, and how cells respond to perturbations, providing insights into immune system dynamics and drug responses. These models can then be used to guide drug development, personalize therapies, or uncover novel therapeutic targets.
Moreover, two papers accomplished classification tasks by traditional machine learning algorithms. For example, Xu et al. proposed a classification method for the characterization of chromatin accessibility patterns at single-cell resolution. Chromatin accessibility refers to the physical accessibility of DNA sequences within the chromatin structure. Changes in chromatin accessibility play a fundamental role in various biological processes, including development, differentiation, and response to environmental cues. Xu’s method combined Monte Carlo feature selection with incremental feature selection, yielding essential genes, classification rules, and an efficient random forest (RF) classifier. Besides, Li et al. constructed an optimal classifier with a decision tree and RF to study immune responses to COVID-19 vaccination strategies and analyze scRNA-seq data from multiple tissues. These methods provided insights into gene regulation, development, and disease mechanisms, and can aid in the development of novel therapeutic approaches.
In summary, machine learning methods enable the extraction of valuable insights from high-dimensional single-cell data, facilitating a deeper understanding of immune responses and the prediction of drug effects. Papers in this Research Topic have the potential to promote the development of precision medicine and advance our understanding of the immune system. Finally, we thank all efforts of the authors, reviewers, and staff at the Frontiers in Genetics editorial office.
QZ led the framed draft design, and RQ wrote the manuscript draft. All authors contributed to the article and approved the submitted version.
The work was supported by the National Natural Science Foundation of China (No. 62131004, No. 62201129); the Sichuan Provincial Science Fund for Distinguished Young Scholars (2021JDJQ0025); the Municipal Government of Quzhou under Grant Number 2021D004, 2022D023 and the Zhejiang Provincial Post-doctor Excellent Scientific Research Project Fund for ZJ2022038.
The authors declare that the research was conducted in the absence of any commercial or financial relationships that could be construed as a potential conflict of interest.
All claims expressed in this article are solely those of the authors and do not necessarily represent those of their affiliated organizations, or those of the publisher, the editors and the reviewers. Any product that may be evaluated in this article, or claim that may be made by its manufacturer, is not guaranteed or endorsed by the publisher.
Keywords: single-cell sequencing data, single-cell immune, drug response prediction, machine learning, feature selection
Citation: Qi R and Zou Q (2023) Editorial: Machine learning methods in single-cell immune and drug response prediction. Front. Genet. 14:1233078. doi: 10.3389/fgene.2023.1233078
Received: 01 June 2023; Accepted: 12 June 2023;
Published: 19 June 2023.
Edited and reviewed by:
Richard D. Emes, Nottingham Trent University, United KingdomCopyright © 2023 Qi and Zou. This is an open-access article distributed under the terms of the Creative Commons Attribution License (CC BY). The use, distribution or reproduction in other forums is permitted, provided the original author(s) and the copyright owner(s) are credited and that the original publication in this journal is cited, in accordance with accepted academic practice. No use, distribution or reproduction is permitted which does not comply with these terms.
*Correspondence: Quan Zou, em91cXVhbkBuY2xhYi5uZXQ=
Disclaimer: All claims expressed in this article are solely those of the authors and do not necessarily represent those of their affiliated organizations, or those of the publisher, the editors and the reviewers. Any product that may be evaluated in this article or claim that may be made by its manufacturer is not guaranteed or endorsed by the publisher.
Research integrity at Frontiers
Learn more about the work of our research integrity team to safeguard the quality of each article we publish.