- Department of Spine Surgery, Xi’an Daxing Hospital, Yanan University, Xi’an, China
Objectives: Bone immune disorders are major contributors to osteoporosis development. This study aims to identify potential diagnostic markers and molecular targets for osteoporosis treatment from an immunological perspective.
Method: We downloaded dataset GSE56116 from the Gene Expression Omnibus database, and identified differentially expressed genes (DEGs) between normal and osteoporosis groups. Subsequently, differentially expressed immune-related genes (DEIRGs) were identified, and a functional enrichment analysis was performed. A protein-protein interaction network was also constructed based on data from STRING database to identify hub genes. Following external validation using an additional dataset (GSE35959), effective biomarkers were confirmed using RT-qPCR and immunohistochemical (IHC) staining. ROC curves were constructed to validate the diagnostic values of the identified biomarkers. Finally, a ceRNA and a transcription factor network was constructed, and a Gene Ontology and Kyoto Encyclopedia of Genes and Genomes enrichment analysis was performed to explore the biological functions of these diagnostic markers.
Results: In total, 307 and 31 DEGs and DEIRGs were identified, respectively. The enrichment analysis revealed that the DEIRGs are mainly associated with Gene Ontology terms of positive regulation of MAPK cascade, granulocyte chemotaxis, and cytokine receptor. protein–protein interaction network analysis revealed 10 hub genes: FGF8, KL, CCL3, FGF4, IL9, FGF9, BMP7, IL17RA, IL12RB2, CD40LG. The expression level of IL17RA was also found to be significantly high. RT-qPCR and immunohistochemical results showed that the expression of IL17RA was significantly higher in osteoporosis patients compared to the normal group, as evidenced by the area under the curve Area Under Curve of 0.802. Then, we constructed NEAT1-hsa-miR-128-3p-IL17RA, and SNHG1-hsa-miR-128-3p-IL17RA ceRNA networks in addition to ERF-IL17RA, IRF8-IL17RA, POLR2A-IL17RA and ERG-IL17RA transcriptional networks. Finally, functional enrichment analysis revealed that IL17RA was involved in the development and progression of osteoporosis by regulating local immune and inflammatory processes in bone tissue.
Conclusion: This study identifies the immune-related gene IL17RA as a diagnostic marker of osteoporosis from an immunological perspective, and provides insight into its biological function.
1 Introduction
Osteoporosis is the most common metabolic bone disease, characterized by reduced bone density and deterioration of bone tissue microarchitecture, increases bone fragility and the risk of fractures, leading to significant mortality (Chandra and Rajawat, 2021). Osteoporosis primarily affects postmenopausal women and men over the age of 50 (Borgström et al., 2020). The pathogenesis of osteoporosis exhibits noteworthy disparities between genders. In women, age-related bone loss and decreased estrogen secretion after menopause are the primary contributors to this condition (Shih et al., 2019). Estrogen plays a pivotal role in augmenting bone cell activity, inhibiting bone resorption, and averting calcium loss from bones. Moreover, estrogen restrains osteoclast formation and induces apoptosis in these cells, thereby curtailing bone resorption. In the absence of sufficient estrogen levels, osteoclast function heightens, leading to accelerated bone loss and the eventual onset of osteoporosis (Zhou et al., 2001). In men, the principal causes of osteoporosis include advancing age, prolonged glucocorticoid use, and declining testosterone levels (Vilaca et al., 2022). With age, inadequate testosterone levels impede the proliferation and differentiation of osteoblasts while intensifying osteoclast activity. Consequently, bone resorption escalates, resulting in subsequent loss of bone mass (Diab and Watts, 2021). It is evident that testosterone plays a pivotal role in the development of osteoporosis among elderly men.
Dual-energy x-ray absorptiometry (DXA) is considered the gold standard for diagnosing osteoporosis (Carey et al., 2022). Nonetheless, it has limitations when it comes to detecting early-stage bone loss. Early diagnosis and timely intervention are beneficial for preventing the development of osteoporosis (Sakka, 2022). In recent years, numerous studies have demonstrated that CUL1, PTEN, STAT1, MAPKAPK2, RARRES2, FLNA, STXBP2, miR-340-5p, and miR-506-3p could potentially serve as biomarkers for osteoporosis (Wang et al., 2022; Zhao Y. et al., 2022; Lu et al., 2023; Yuxuan et al., 2023). However, the majority of these molecular markers have not yet been validated using clinical samples. Thus, their potential for clinical applications remain limited. Therefore, there is still a need to find effective biomarkers for osteoporosis.
Osteoclasts, originating from hematopoietic cells of the myeloid lineage, play a crucial role in bone resorption (Thiolat et al., 2014). These cells undergo differentiation from osteoclast precursors when stimulated by M-CSF and RANKL (Zheng et al., 2014). Osteoblasts play a fundamental role in the synthesis of mineralized bone and are derived from a mesenchymal progenitor cell (Debnath et al., 2018). Multiple immune cells are involved in the regulation of osteoclast and osteoblast homeostasis. Th17 cells induce osteoclastogenesis by IL-17, Th1 cells activate osteoclast function through TNF-α (Adamopoulos and Bowman, 2008; Liu et al., 2011). Conversely, Sato et al. (2006) demonstrated that Th2 cells can impede osteoclast formation via IL-4. DCs enhance osteoclast activity by interacting with T cells through the RANK-RANKL signaling pathway (Santiago-Schwarz et al., 2001). Rivollier et al. (2004) revealed that DCs can transdifferentiate into osteoclasts in vitro in the presence of M-CSF and RANKL. Furthermore, B cells secrete RANKL, promoting osteoclast function (Kanematsu et al., 2000). Conversely, ILC2 cells suppress osteoclast formation through the release of IL-4 and IL-13 (Omata et al., 2020). Treg cells can inhibit monocyte differentiation into osteoclasts (Luo et al., 2011). Neutrophils hinder bone formation by affecting osteoblast function (Brunetti et al., 2013). M2 macrophages promote osteoblast differentiation (Vi et al., 2015). Conversely, M1 macrophages leads to bone resorption by increasing osteoclast activity and suppressing osteoblast-mediated bone formation (Bastian et al., 2011). In vitro studies have demonstrated the direct enhancement of osteoblast function by Treg cells (Lei et al., 2015). Additionally, B cells activate NF-κB signaling pathways to inhibit the differentiation of mesenchymal precursor cells into osteoblasts (Sun et al., 2018). Therefore, exploring the molecular mechanisms of osteoporosis from an immune perspective and developing new targets for immunotherapy is of great relevance for osteoporosis treatment.
Here, we performed a differential gene expression analysis on an osteoporosis microarray dataset downloaded from the Gene Expression Omnibus (GEO) database, and identified the intersection of differentially expressed genes (DEGs) with immune-related genes (IRGs) to determined differentially expressed immune-related genes (DEIRGs). Then, we constructed a protein–protein interaction (PPI) network to identify hub genes, and finally determined the immune-related gene IL17RA as a potential biomarker for osteoporosis after validating it in another dataset (GSE35959). RT-qPCR and immunohistochemical (IHC) staining were performed, and then ROC curves were constructed to verify its diagnostic value. In addition, we also explored the biological function of IL17RA by constructing ceRNA and transcription factor (TF) networks in addition to a Gene Ontology (GO) and Kyoto Encyclopedia of Genes and Genomes (KEGG) enrichment analysis to further elucidate the molecular mechanisms of osteoporosis in which IL17RA is involved.
2 Materials and methods
2.1 Microarray data
mRNA [GSE56116, https://www.ncbi.nlm.nih.gov/geo/query/acc.cgi?acc=GSE56116, GSE35959 (Benisch et al., 2012)], and miRNA microarray datasets [GSE201543 (Zhao S.-L. et al., 2022)] were downloaded from GEO (http://www.ncbi.nlm.nih.gov/geo/) by GEOquery package (Davis and Meltzer, 2007). GSE56116 was obtained from a GPL4133 Agilent-014850 Whole Human Genome Microarray 4 × 44 K G4112F. GSE35959 was obtained from a GPL570 (HG-U133_Plus_2) Affymetrix Human Genome U133 Plus 2.0 Array and GSE201543 was obtained from a GPL20712 Agilent-070156 Human miRNA (miRNA version). The probes were labeled with gene symbols, then multiple probes corresponding to the same gene were randomly selected to remove duplicates, and finally the gene expression matrix was obtained. GSE56116 contained 3 normal (healthy control) and 10 osteoporosis samples (4 kidney Yin deficiency, 3 kidney Yang deficiency, 3 non-kidney deficiency). GSE35959 contained 14 normal and 5 osteoporosis samples. GSE201543 contained 4 normal and 6 osteoporosis samples. GSE56116 (3 non-kidney deficiency osteoporosis samples and 3 normal samples), GSE35959, and GSE201543 were used as the training, validation, and miRNA validation datasets, respectively. Data of IRGs was downloaded from ImmPort database (https://www.immport.org/shared/), and finally 2,499 immune-related genes were obtained (Supplementary Table S1). The flow chart followed this study is shown in Figure 1.
2.2 Identification of DEIRGs
The data set was normalized using the normalizeBetweenArrays function of the limma package (Ritchie et al., 2015). The sample correction is visualized by box plots, and the clustering between sample groups was visualized using PCA plots. Screen for DEGs between patients and controls using the limma package, and p value of lower than 0.05 and [log2 fold change (FC)] equal to or higher than 1 were set as the threshold values for DEG identification. After that, the intersection of DEGs and IRGs was determined to obtain list of DEIRGs. The results were visualized using the ggplot2 (Wickham, 2009) and the VennDiagram packages (Chen and Boutros, 2011). The results of DEGs and DEIRGs were visualized using the ggplot2 package for volcano plots and the Complex Heatmap package (Gu et al., 2016) for heat maps.
2.3 GO and KEGG enrichment analyses of DEIRGs
GO and KEGG functional enrichment analysis of DEIRGs were conducted using the cluster Profiler package (Yu et al., 2012). The results were visualized using the ggplot2 package. The human genome was used as a background reference, and a p.adj of lower than 0.05 was set as cut-off.
2.4 Construction of PPI network and selection of hub genes
The PPI network of DEIRGs was constructed using the STRING database (https://string-db.org/) (Szklarczyk et al., 2019). Interaction scores higher than 0.4 were considered significant. The results were visualized using Cytoscape software (Version: 3.9.1). Top 10 genes were determined as hub genes using the cytoHubba plugin and on the maximum correlation criterion algorithm.
2.5 External validation of hub genes
To identify effective biomarkers of osteoporosis, differences in hub gene expression levels between the osteoporosis and the normal groups were validated using another dataset (GSE35959).
2.6 Construction of ceRNA network
MiRNAs were predicted using four different databases [miRDB (https://mirdb.org/mirdb/index.html), TargetScanHuman (https://www.targetscan.org/vert_80/), TarBase (https://dianalab.e-ce.uth.gr/html/diana/web/index.php?r=tarbasev8) and miRWalk (http://mirwalk.umm.uni-heidelberg.de/)]. Furthermore, lncRNA-miRNA relationships of predicted hub genes-associated miRNAs were obtained by overlapping results from starBase (http://starbase.sysu.edu.cn/) and DIANA-LncBase v3 (https://diana.e-ce.uth.gr/lncbasev3). The overlap was visualized by ggplot2 and VennDiagram packages. Finally, a competitive endogenous RNA (ceRNA) network regulating hub genes was constructed by using Cytoscape software (Version: 3.9.1).
2.7 Construction of TF network
Prediction of hub genes and their TFs were performed by TF-Marker (http://bio.liclab.net/TF-Marker/) and GRNdb (http://www.grndb.com/), the overlap was visualized by ggplot2 and VennDiagram packages. A TF network regulating hub genes was constructed by using igraph package (Csardi and Nepusz, 2006).
2.8 Study subjects
Peripheral blood samples were obtained from nine patients with osteoporosis and nine healthy adults who were hospitalized in the Department of Spine Surgery at Xi’an Daxing Hospital, affiliated with Yan’an University, and underwent BMD testing between January 2023 and April 2023 (Table 1). Those with a history of long-term use of drugs affecting bone metabolism, endocrine system disorders, spinal tumors or spinal tuberculosis were not included in this study. Bone tissue samples were obtained from twelve patients with osteoporotic compression fractures who were hospitalized for vertebroplasty surgery, with mild osteoporosis (−3. 5 < T-score ≤ −2. 5) and severe osteoporosis (T-score ≤ −3. 5), six in each group (Table 2). All patients underwent MRI and DXA of the spine, which confirmed the presence of fresh fractures and osteoporosis. Inclusion criteria were as follows: 1) age ≥50 years, BMD T-score ≤ −2.5; 2) vertebral fragility fracture, biopsy routinely performed during vertebroplasty. Exclusion criteria were as follows: previous long-term use of drugs affecting bone metabolism, presence of endocrine system diseases, spinal tumors and spinal tuberculosis. The diagnosis of osteoporosis was confirmed based on the classifcation criteria of the World Health Organization (WHO) based on T-score of BMD testing (Yoshimura et al., 2022): T-score ≥ −1.0 was considered normal bone mass, −2.5 < T-score < −1.0 was considered decreased bone mass, and T-score ≤ −2.5 was considered osteoporosis. All included subjects were informed of the medical record review and study design and signed consent documents before data collection. The Ethics Committee of the Xi’an Daxing Hospital, affiliated with Yan’an University approved and reviewed the study protocol.
2.9 Peripheral blood collection
Five milliliters peripheral blood samples were collected the morning following an overnight fast. The serum was obtained following centrifugation (3,000 r/min, 5 min) of blood samples and submitted for bone metabolism marker detection. Cell sediment was collected for RNA extraction. All samples were frozen at −80°C until analysis.
2.10 Bone tissue sample acquisition
The patient was placed in the prone position, and a 0.5–1 cm piece of cancellous bone tissue was drilled using a 14G bone biopsy ring perched on the fracture area within the vertebral body under local anesthesia via the arch root approach. The bone tissue was fixed in 10% neutral-buffered formalin for 1 week, followed by routine decalcification, dehydration, and paraffin embedding for subsequent studies.
2.11 BMD measurements
BMD measurements of the lumbar spine were performed using DXA (QDR X-Ray Bone Densitometer, Hologic, United States). All data were measured by the same group of imaging physicians in strict accordance with the specifications for measuring BMD by DXA.
2.12 RT-qPCR analysis
Total RNA was extracted using RNA Extraction Solution (G3013, Servicebio, Wuhan, China), and RNA concentration and purity were measured by Nanodrop 2000 spectrophotometer (Thermo Scientific, Waltham, United States). The RNA samples were reverse transcribed into cDNA using a reverse transcription kit (G3337, Servicebio, Wuhan, china), and the cDNA was used as a template to amplify the IL17RA gene. The reaction was performed via 40 amplification cycles using the following protocol: Denaturation at 95°C for 30 s, annealing at 60°C for 30 s, extension at 72°C for 60 s. Samples were analyzed in triplicate, the mRNA expression levels of IL17RA was calculated by the 2−ΔΔCT method, and GAPDH was used as internal reference. The sequences of the primers are listed in Table3.
2.13 HE and IHC staining
The wax blocks were placed in a paraffin slicer for continuous sectioning, with each section having 4 μm thickness. HE staining was performed using HE staining solution (G1003, Servicebio, Wuhan, China). For IHC, paraffin tissue sections were deparaffinized with xylene and rehydrated with an alcohol gradient and water. Sections were incubated with primary antibodies IL17RA (Catalogue number: DF3602, diluted 1:100, Affinity), at room temperature for 1 h and biotin-labelled secondary antibodies for 30 min, and then stained with DAB peroxidase substrate kit (G1212, Servicebio, Wuhan, China). Finally, washed with water and counterstained with haematoxylin. The results were observed and photographed by an microscope (Eclipse C1, Nikon, Japan).
2.14 Statistical analysis
All data processing and analysis were conducted using R software (version 4.2.1). RT-qPCR were repeated three times, and data were represented as the mean ± SD. Normality was tested using the Shapiro-Wilk normality test and chi-squaredness was tested using Levene’s test. Student’s t-test or Wilcoxon rank sum test was used to determine the significance of difference between two groups. Correlation coefficients were calculated using Spearman correlation analysis. ROCs were used to evaluate AUCs and predictive abilities. A p value lower than 0.05 was considered statistically significant.
3 Results
3.1 Identification of DEIRGs
The median, upper and lower quartiles, maximum and minimum values of each sample gene were significantly close to each other upon normalization of GSE56116 data (Supplementary Figure S1A, B). However, PCA revealed that the centers of the osteoporosis group were farther apart than those of the control group, indicating significant differences in gene expression between the two groups (Supplementary Figure S1C, D). Using a p value of lower than 0.05 and a [log2 fold change (FC)] equal to or higher than 1 as the threshold levels, we identified 307 DEGs, including 94 and 213 significantly up- and down-regulated genes, respectively (Supplementary Table S2). Figures 2A, B show results in the form of volcano plots and heat maps (Figures 2A, B). The intersection of DEGs and IRGs included 31 genes (DEIRGs) (Figure 2C; Supplementary Table S3), including 11 and 20 up- and down-regulated genes, respectively (Figure 2D).
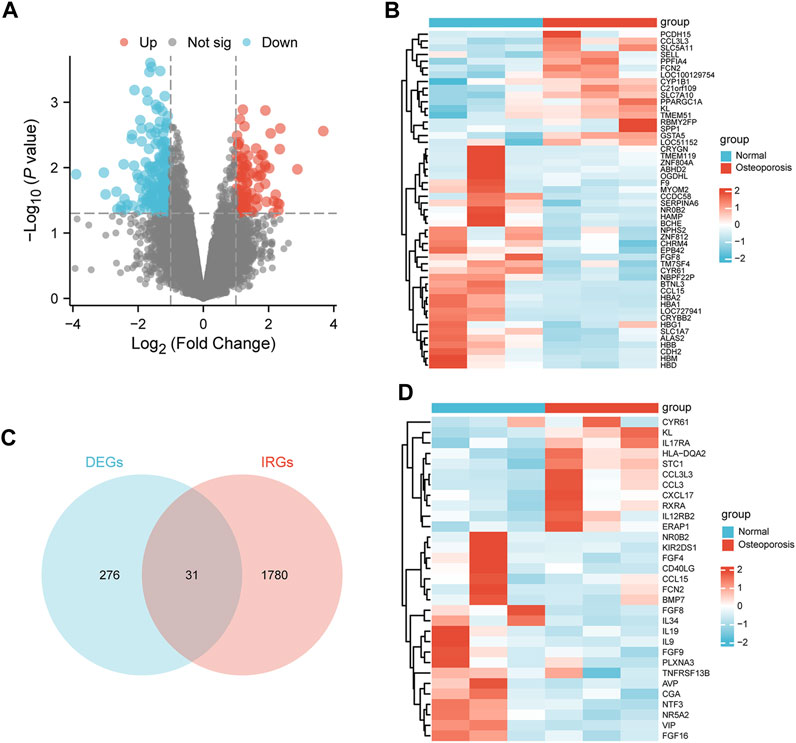
FIGURE 2. Identification of DEIRGs. (A) Volcano plot, (B) heatmap of DEGs between the osteoporosis and normal samples. (C) Venn diagram of overlapping genes between the DEGs and IRGs. (D) Heatmap of DEIRGs.
3.2 Functional enrichment analyses of DEIRGs
We performed GO and KEGG enrichment analysis to investigate the functions of DEIRGs. In the GO analysis, biological processes (BPs), cell components (CCs), and molecular functions (MFs) were distinguished. The BPs included regulation of chemotaxis, positive regulation of MAPK cascade, granulocyte chemotaxis, cell chemotaxis and granulocyte migration. CCs included clathrin−coated endocytic vesicle membrane, clathrin−coated endocytic vesicle, clathrin−coated vesicle membrane, serine−type peptidase complex and semaphorin receptor complex. Finally, MFs included signaling receptor activator activity, receptor ligand activity, growth factor activity, fibroblast growth factor receptor binding and growth factor receptor binding. KEGG analysis showed that DEIRGs were mainly associated with Cytokine−cytokine receptor interaction, Viral protein interaction with cytokine and cytokine receptor. Figures 3A–D show the top five enrichment items of BP, CC, MF in GO and KEGG analyses.
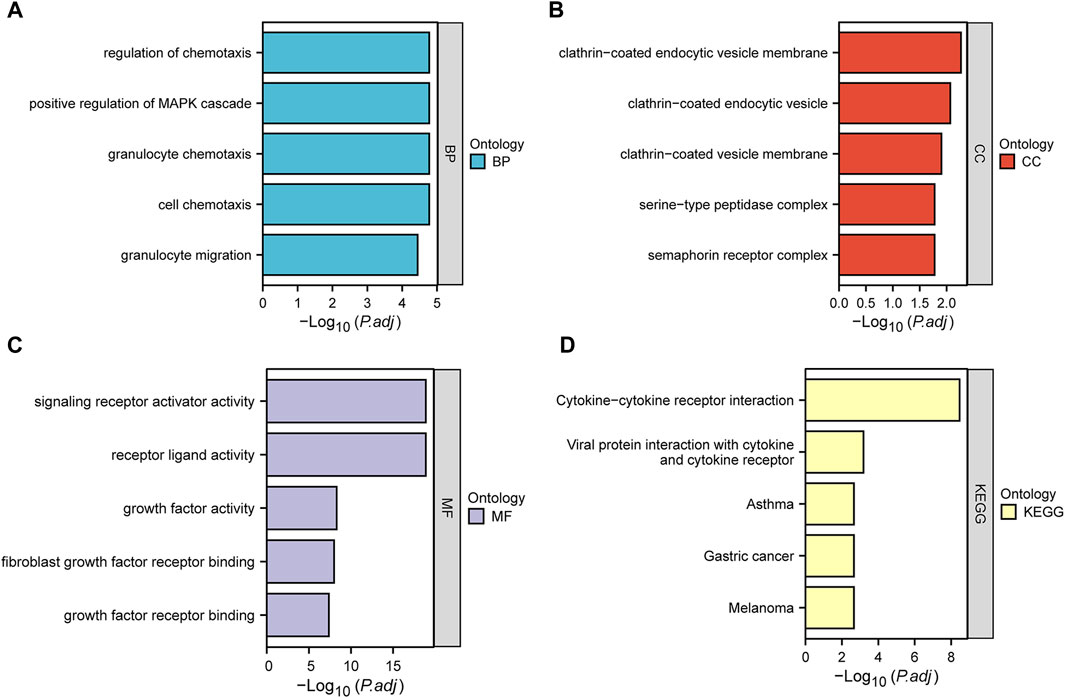
FIGURE 3. Bar plots of 31 DEIRGs-enriched GO terms and KEGG pathways. (A–D) represent BP, CC, MF, and KEGG, respectively.
3.3 Construction of the PPI network and identification of hub genes
The STRING database was used to construct a PPI network of 31 DEIRGs in order to investigate protein-protein interactions. A total of 30 nodes and 31 edges were identified in the PPI network (Figure 4A). The cytohubba plug-in of Cytoscape software was then used to select the top 10 hub genes based on their degree of connectivity (Figure 4B; Table 4).
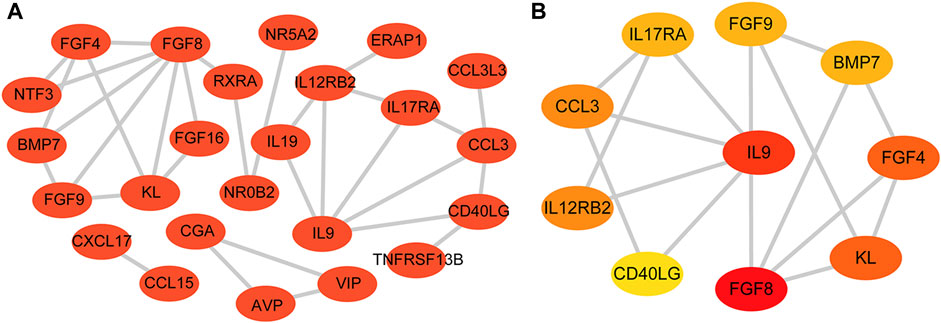
FIGURE 4. PPI network and hub genes. (A) PPI network constructed with the DEIRGs; (B) Top 10 hub genes.
3.4 Validation of diagnostic biomarkers
In the GSE35959 dataset, osteoporotic patients had significantly higher IL17RA expression levels than those of patients in the control group (p < 0.05) (Figure 5A; Supplementary Figure S2). To confirm the higher expression level of IL17RA in osteoporotic patients and its diagnostic performance, we validated this finding using clinical peripheral blood and bone tissue. RT-qPCR results showed that mRNA expression levels of IL17RA were significantly higher in peripheral blood of patients with osteoporosis compared to those of patients in the control group (p < 0.05) (Figure 5B). The IHC results showed that IL17RA expression was higher in the severe osteoporosis group compared to the mild osteoporosis group (Figure 5C). The horizontal and vertical coordinates of the ROC curve indicate sensitivity and specificity, respectively. A larger AUC indicates a more accurate diagnostic model. Accordingly, the AUC was 0.802 (Figure 5D), indicating significant differences between OP and control groups. Hence, IL17RA expression level could serve as a good diagnostic biomarker.
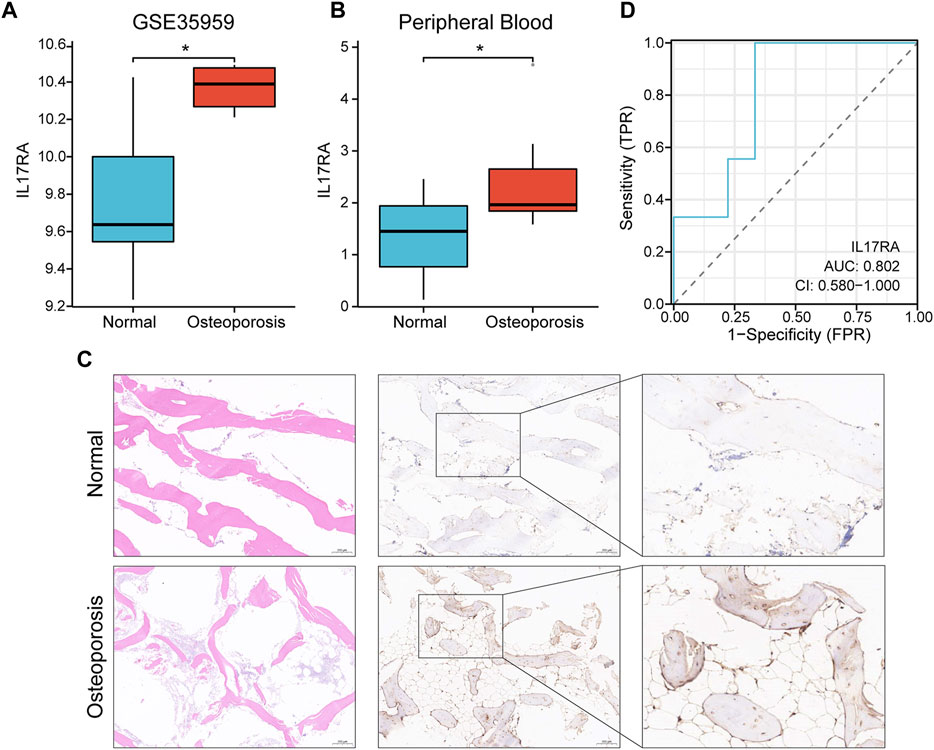
FIGURE 5. Verification of the diagnostic effectiveness of IL17RA. (A) IL17RA expression in the GSE35959 dataset. (B) IL17RA expression in peripheral blood samples. (C) IL17RA expression in bone tissue samples. (D) ROC curve.
3.5 Construction of ceRNA network
We analyzed upstream regulation of IL17RA, and screened for miRNAs or lncRNAs targeting IL17RA. We identified 142, 1,423, 13, and 2,113 miRNAs possibly targeting IL17RA from miRDB, TargetScanHuman, TarBase, and miRWalk databases, respectively (Figure 6A). Consequently, we determined hsa-miR-128-3p to be the most important miRNA regulator by comparing predictions based on each database. Complementary sequences between IL17RA and hsa-miR-128-3p are displayed in Figure 6B. We validated hsa-miR-128-3p expression in the GSE201543 dataset, and found that expression level of hsa-miR-128-3p was significantly low in osteoporotic patients (Figure 6C). Next, 30 lncRNAs that could bind to hsa-miR-128-3p were obtained from the overlapping results of DIANA-LncBase v3 and starBase databases (Figure 6D). A lncRNA-miRNA-mRNA network regulating IL17RA was constructed, in which lncRNAs competitively bind to miRNAs and attenuate the inhibition of IL17RA by miRNAs (Figure 6E). A review of the literature was used to determine these 30 lncRNAs. Our findings indicated that expression levels of NEAT1 and SNHG1 were significantly high in osteoporotic patients. Since a significantly high level of IL17RA expression and a significantly low level of hsa-miR-128-3p were found in osteoporotic patients, the interactions predicted by the above database (Figure 6F) further led us to hypothesize that NEAT1 and SNHG1 bind to hsa-miR-128-3p, and impair the inhibitory effect of hsa-miR-128-3p on IL17RA in osteoporosis (Figure 6G).
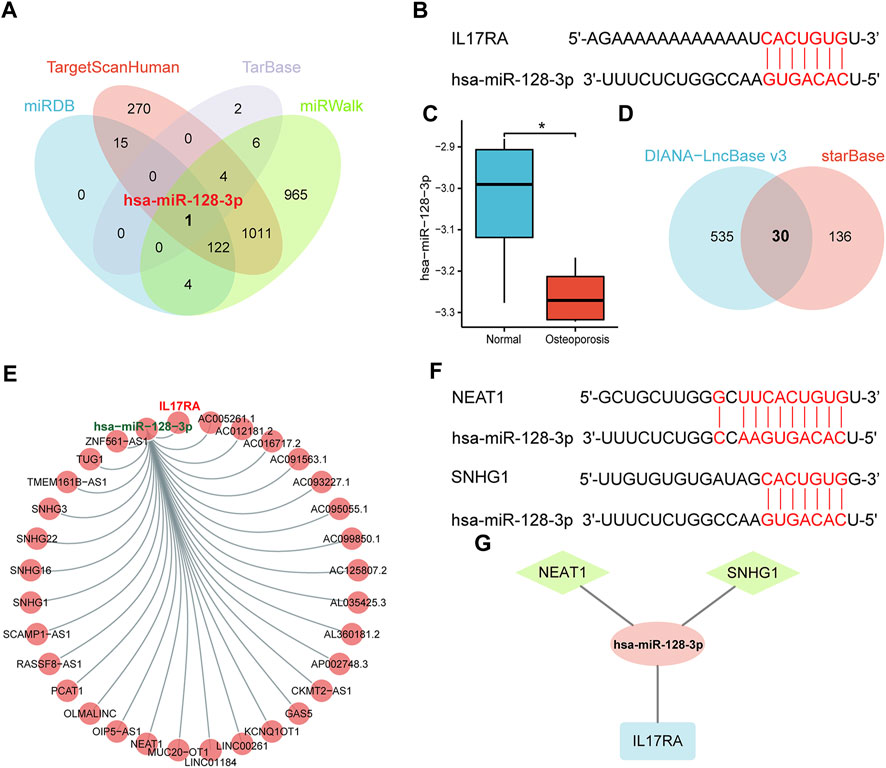
FIGURE 6. The ceRNA regulatory network of IL17RA. (A) Prediction of miRNAs targeting IL17RA using four different databases. (B) Predicted interaction between hsa-miR-128-3p and IL17RA. (C) Expression of hsa-miR-128-3p in the GSE201543 dataset. (D) Prediction of lncRNAs targeting hsa-miR-128-3p using two different databases. (E) lncRNA-miRNA-mRNA network of IL17RA. (F) Predicted interactions between NEAT1, SNHG1 and hsa-miR-128-3p. (G) A ceRNA network consisting of IL17RA, hsa-miR-128-3p, NEAT1 and SNHG1 in osteoporosis.
3.6 Transcriptome analysis
To better understand gene expression upstream of IL17RA, we performed a transcriptome analysis. First, we obtained 607 and 166 TFs regulating IL17RA from the TF-Marker and GRNdb databases, respectively. A total of 75 TFs were found in both databases (Figure 7A). Using these, an IL17RA transcriptional regulatory network was constructed (Figure 7B). We selected nine TFs that showed significant differential expression between the osteoporosis and normal groups (Figure 7C). Among these TFs, ERF (R = 0.661, p = 0.044), IRF8 (R = 0.709, p = 0.028), POLR2A (R = 0.867, p = 0.003) and ERG (R = −0.867, p = 0.003) were found to be correlated with IL17RA (Figure 7D; Supplementary Figure S3). Based on this, we constructed the osteoporosis ERF-IL17RA, IRF8-IL17RA, POLR2A-IL17RA and ERG-IL17RA transcriptional networks (Figure 7E).
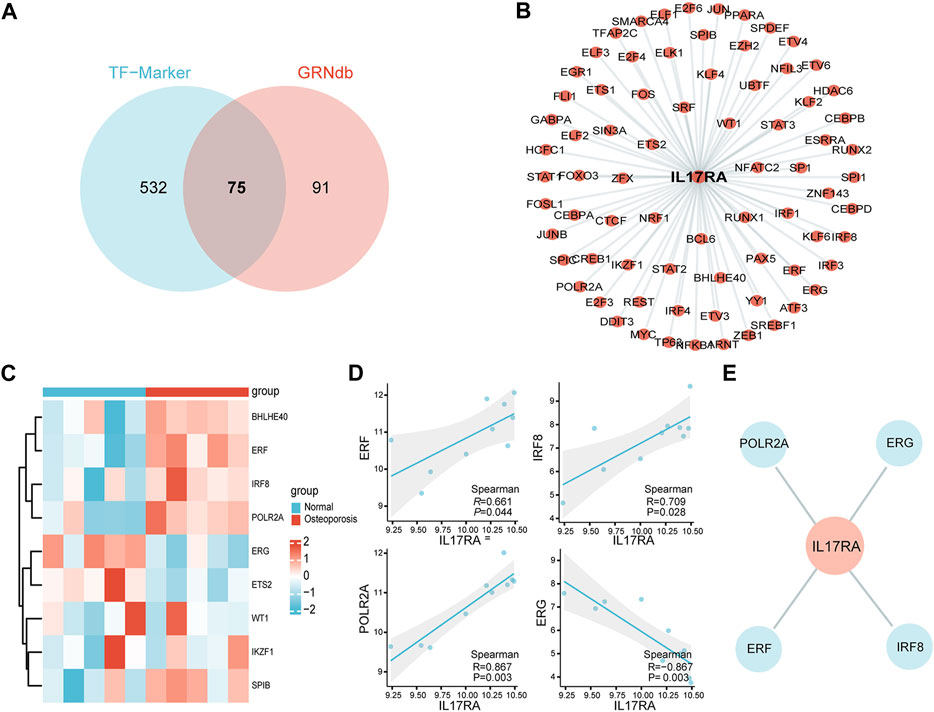
FIGURE 7. Transcriptional network of IL17RA. (A) Predicted TFs associated with IL17RA based on TF-Marker and GRNdb databases. (B) IL17RA transcriptional regulatory network. (C) Heat map of TFs expression between osteoporosis and normal groups. (D) Spearman correlation between ERF, IRF8, POLR2A and ERG and IL17RA. (E) Transcriptional network between ERF, IRF8, POLR2A, ERG and IL17RA in osteoporosis.
3.7 GO and KEGG pathway enrichment analysis of diagnostic biomarkers
To investigate the downstream regulatory roles of IL17RA, we used the STRING database to predict 10 IL17RA-interacting genes (using a confidence score of equal to or higher than 0.4), and constructed a PPI network using Cytoscape (Figure 8A). A total of five KEGG pathways were highlighted by KEGG analysis of IL17RA and IL17RA-interacting genes: IL−17 signaling pathway, Cytokine−cytokine receptor interaction, alcoholic liver disease, inflammatory bowel disease, and RIG−I−like receptor signaling pathway (Figure 8B). The GO enrichment analysis results indicated that cytokine receptor binding, cytokine activity, immune receptor activity, cytokine receptor activity, and thioesterase binding were the top 5 MF terms (Figure 8C). Cytokine−mediated signaling pathway, interleukin−17−mediated signaling pathway, cellular response to interleukin−17, and response to interleukin−17, positive regulation of interleukin−6 production were the top 5 BP terms (Figure 8D). Finally, plasma membrane signaling receptor complex, cytoplasmic side of membrane, cytoplasmic side of plasma membrane, CD40 receptor complex, and lipid droplet were the top 5 CC terms (Figure 8E).
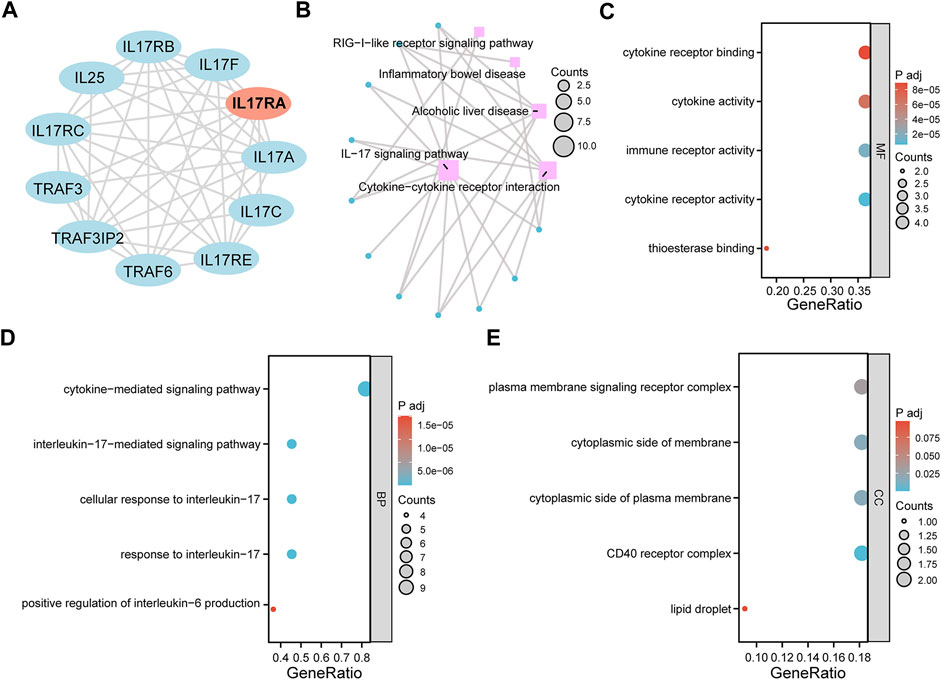
FIGURE 8. GO and KEGG pathway enrichment analysis. (A) An PPI network of IL17RA constructed with data from STRING. (B) KEGG enrichment analysis network diagram. (C) MF (D) BP (E) CC enrichment analysis bubble diagram.
3.8 Gene Set Enrichment Analysis (GSEA)
To investigate the functions of IL17RA in osteoporosis, we conducted Gene Set Enrichment Analysis (GSEA) by stratifying samples based on IL17RA expression. The results enriched several important pathways, including “INTERFERON_ALPHA_RESPONSE,” “INTERFERON_GAMMA_RESPONSE,” “IL6_JAK_STAT3_SIGNALING”, “INFLAMMATORY_RESPONSE”, “REACTIVE_OXYGEN_SPECIES_PATHWAY”, and “TNFA_SIGNALING_VIA_NFKB” (Supplementary Figure S4). These pathways play a critical role in immune response, pro-inflammatory reactions, cytokine signaling, and other vital biological processes. The identification and enrichment of these pathways shed light on the intricate connections between IL17RA and multiple molecular mechanisms involved in maintaining bone health and homeostasis.
4 Discussion
Osteoporosis is characterized by reduced bone strength and an increased risk of fracture. It is estimated that more than 200 million people worldwide suffer from osteoporosis, with 30%–50% of women experiencing fractures due to osteoporosis during their lifetime (Rachner et al., 2011). Since osteoporosis patients typically exhibit no obvious clinical symptoms before their first fracture, early diagnosis is crucial for timely intervention and pain relief. Hence, there is an urgent need for effective molecular diagnostic markers. Previous studies suggested that the immune system may play a significant role in osteoporosis development (Sapra et al., 2022; Wang et al., 2022), yet the specific immune targets and molecular mechanisms of osteoporosis remain unknown. Microarray technology has enabled the exploration of genetic alterations in osteoporosis, and has proven effective in identifying novel biomarkers for other diseases. In this study, we used bioinformatics methods to identify diagnostic markers for osteoporosis, and validated their diagnostic value using peripheral blood from osteoporosis patients.
An analysis of transcriptome data from peripheral blood samples of osteoporosis patients and healthy individuals yielded a total of 307 DEGs, including 94 up- and 213 down-regulated genes, respectively. The intersection of DEGs and IRGs yielded a total of 31 DEIRGs, including 11 and 20 up- and down-regulated genes, respectively. GO enrichment analysis of DEIRGs showed that the GO terms were associated with positive regulation of MAPK cascade, granulocyte chemotaxis, growth factor activity, and semaphorin receptor complex. KEGG analysis showed that DEIRGs were mainly associated with Cytokine−cytokine receptor interaction, Viral protein interaction with cytokine and cytokine receptor. These findings suggest that immunomodulation plays a significantly role in the development of osteoporosis. MAPK and innate immune signaling pathways are closely-linked through feedback regulation (Kitajima et al., 2018). Previous studies have reported that the MAPK signaling pathway is involved in the regulation of bone metabolism and osteoclast formation (Meng et al., 2021; Wang et al., 2021). Neutrophil chemokines stimulate the growth and development of osteoblasts and chondrocytes (Mori et al., 1997). Cytokine-cytokine receptor interaction, and viral protein interaction with cytokine suggests significant involvement of the immune system and inflammatory cytokines in the progression of osteoporosis. Inflammatory factors inhibit bone formation in part by suppressing osteoblast differentiation, which includes inhibition of Wnt signaling. In addition, they also promote bone resorption by inducing osteoclast differentiation and bone resorption functions, which in turn disrupt bone homeostasis and contribute to the progression of osteoporosis (Ivashkiv et al., 2011). Hence, dysregulation of the immune system can have a detrimental effect on bone integrity, leading to osteoporosis. Our results are consistent with previous findings.
The PPI network analysis revealed 10 key genes associated with osteoporosis: FGF8, KL, CCL3, FGF4, IL9, FGF9, BMP7, IL17RA, IL12RB2, CD40LG. Expression level of IL17RA was found to be significantly high in osteoporotic patients upon external dataset validation, suggesting that IL17RA may be an effective biomarker for osteoporosis. To further confirm the diagnostic performance of IL17RA, we verified IL17RA expression by RT-qPCR and IHC, and plotted ROC curves. RT-qPCR results showed that the mRNA expression level of IL17RA was significantly higher in peripheral blood of osteoporotic patients compared to that of the control group. IHC results were in line with RT-qPCR. The Area Under Curve (AUC) was 0.802, suggesting a high diagnostic value and potential of IL17RA as a diagnostic marker for osteoporosis.
The IL-17 family of inflammatory cytokines has gained attention as major contributors to bone formation and bone resorption. Most IL-17 cytokines act by signaling through the receptor complex of IL17RA. IL17RA signaling in osteoclast precursors were previously demonstrated to contribute to osteoclast formation and subsequent bone loss. Moreover, IL17RA deficiency increases bone mass by decreasing the abundance of osteoclast precursors (Roberts et al., 2022). In addition, IL17RA in osteoblasts/osteoclasts mediates parathyroid hormone-induced bone loss and enhances osteoblast RANKL production (Li et al., 2019). These studies are in line with our findings. However, Goswami et al. (2009) used the ovariectomy-induced osteoporosis (OVX) model in IL17RA (−/−) mice to assess the role of IL17A in estrogen deficiency-induced bone loss. The authors showed that IL17RA (−/−) mice were consistently more susceptible to OVX-induced bone loss than controls. IL17A inhibits bone resorption-related protease expression and osteoclast differentiation in RAW264.7 cells via IL17RA (Kitami et al., 2010). These findings suggests that IL17RA signaling plays an osteoprotective role in ovariectomy-induced bone loss. This also shows that the role of IL17RA in osteoporosis is still controversial, and an increased sample size is needed for an in-depth analysis.
The concept of CeRNA was introduced in 2011 (Salmena et al., 2011). In the ceRNA network, non-coding RNAs, such as lncRNAs or circRNAs, can compete to bind to miRNAs, and thereby weaken the repression of mRNAs by miRNAs. We identified hsa-miR-128-3p as a key regulatory miRNA for IL17RA in osteoporosis. Previous research has indicated that hsa-miR-128-3p can inhibit osteoblast differentiation of bone marrow mesenchymal stem cells by downregulating RUNX1, YWHAB and NTRK2 (Zhang W. et al., 2020). In addition, hsa-miR-128-3p promoted the proliferation, migration and osteoclast differentiation of RAW 264.7 cells and upregulated the osteoclastogenic markers c-Fos, NFATc1 and Ctsk (Zhang et al., 2022a). These findings suggests that hsa-miR-128-3p inhibits osteoblast differentiation and promotes osteoclast formation, which is inconsistent with our findings here. Further studies are needed to explain this paradox, and identify other mechanisms involving has-miR-128-3p in osteoporosis. We hypothesize that NEAT1 and SNHG1 target hsa-miR-128-3p. Studies have shown that NEAT1 promotes the proliferation and differentiation of osteoblasts and, regulates the development and progression of osteoporosis (Zhang Y. et al., 2020; Zhao X. et al., 2022). SNHG1 expression is up-regulated in OVX mice, which inhibits osteoblast differentiation and angiogenesis while promoting osteoclast formation, leading to osteoporosis (Yu et al., 2021; Yu et al., 2022). NEAT1 and SNHG1 are thus promising targets for the treatment of osteoporosis. The above-mentioned findings support the conclusions of our study. We constructed the NEAT1-hsa-miR-128-3p-IL17RA and SNHG1-hsa-miR-128-3p-IL17RA networks to provide a theoretical basis for understanding the molecular mechanisms of IL17RA involvement in osteoporosis.
We performed a transcriptional analysis as well. The ERF (ETS2 repressor factor) is located on Chromosome 19q13.2, and encodes a transcription factor bound directly by ERK1/2 to regulate the RAS-MEK-ERK signal transduction cascade (von Kriegsheim et al., 2009). A study found that reduced doses of ERF lead to complex cranial suture closure in humans and mice, and highlighted ERF as a novel regulator of osteogenic stimulation of RAS-ERK signaling (Sr et al., 2013). IRF8 inhibits osteoclastogenesis, and is involved in the development and progression of osteoporosis (Zhao et al., 2009; Jin et al., 2023). RNA polymerase II subunit A (POLR2A) encodes the largest catalytic subunit of the RNA polymerase II complex. Liu et al. (2021) showed that POLR2A blocks osteoclastic bone resorption and prevented osteoporosis by interacting with CREB1. ERG is closely associated with Ewing sarcoma (Dunn et al., 1994), cervical cancer (Zhang Z. et al., 2020) and prostate cancer (Dawoud et al., 2021). However, its role in bone metabolism remains unexplored. The TF network constructed here provides a clear direction to better understand the upstream transcriptional mechanism of IL17RA. To further investigate the downstream regulatory role of IL17RA, we also performed a functional enrichment analysis of IL17RA and its interacting genes. Accordingly, IL17RA may be involved in the development and progression of osteoporosis by regulating local immune and inflammatory processes in bone tissue.
There were also some limitations in this study. First, the sample size in the dataset selected for this study was small. Although we standardized the raw data, a larger sample size and a higher quality dataset are still needed to verify the reliability of the results. Secondly, although we validated the diagnostic value of IL17RA using patients’ peripheral blood samples and bone tissues, the sample size of this study was also limited, and the clinical translational value of IL17RA needs to be validated in a larger number of clinical osteoporosis samples. Finally, a more comprehensive study on molecular biological mechanisms involving IL17RA on both cellular and animal levels is needed.
In conclusion, we identified the immune-related gene IL17RA as a diagnostic marker of osteoporosis by elucidating its biological function within the immune system. Our findings may provide with a potential immune molecular target for the early diagnosis and treatment of osteoporosis.
Data availability statement
The datasets presented in this study can be found in online repositories. The names of the repository/repositories and accession number(s) can be found in the article/Supplementary Material.
Ethics statement
The studies involving human participants were reviewed and approved by Xi’an Daxing Hospital affiliated to Yanan University. The patients/participants provided their written informed consent to participate in this study.
Author contributions
Y-JD and BY contributed to the conception and design of the study. ZL, BW, and JL acquired the data. JM and Q-CL performed the data analysis. XX and XT wrote the first draft of the manuscript. YZ revised the manuscript critically. All authors contributed to the article and approved the submitted version.
Funding
This work was supported by the National Science Foundation of China (No. 82260443).
Acknowledgments
We would like to thank Editage (www.editage.cn) for English language editing.
Conflict of interest
The authors declare that the research was conducted in the absence of any commercial or financial relationships that could be construed as a potential conflict of interest.
Publisher’s note
All claims expressed in this article are solely those of the authors and do not necessarily represent those of their affiliated organizations, or those of the publisher, the editors and the reviewers. Any product that may be evaluated in this article, or claim that may be made by its manufacturer, is not guaranteed or endorsed by the publisher.
Supplementary material
The Supplementary Material for this article can be found online at: https://www.frontiersin.org/articles/10.3389/fgene.2023.1219894/full#supplementary-material
References
Adamopoulos, I. E., and Bowman, E. P. (2008). Immune regulation of bone loss by Th17 cells. Arthritis Res. Ther. 10, 225. doi:10.1186/ar2502
Bastian, O., Pillay, J., Alblas, J., Leenen, L., Koenderman, L., and Blokhuis, T. (2011). Systemic inflammation and fracture healing. J. Leukoc. Biol. 89, 669–673. doi:10.1189/jlb.0810446
Benisch, P., Schilling, T., Klein-Hitpass, L., Frey, S. P., Seefried, L., Raaijmakers, N., et al. (2012). The transcriptional profile of mesenchymal stem cell populations in primary osteoporosis is distinct and shows overexpression of osteogenic inhibitors. PLoS One 7, e45142. doi:10.1371/journal.pone.0045142
Borgström, F., Karlsson, L., Ortsäter, G., Norton, N., Halbout, P., Cooper, C., et al. (2020). Fragility fractures in europe: Burden, management and opportunities. Arch. Osteoporos. 15, 59. doi:10.1007/s11657-020-0706-y
Brunetti, G., Faienza, M. F., Piacente, L., Ventura, A., Oranger, A., Carbone, C., et al. (2013). High dickkopf-1 levels in sera and leukocytes from children with 21-hydroxylase deficiency on chronic glucocorticoid treatment. Am. J. Physiol. Endocrinol. Metab. 304, E546–E554. doi:10.1152/ajpendo.00535.2012
Carey, J. J., Chih-Hsing Wu, P., and Bergin, D. (2022). Risk assessment tools for osteoporosis and fractures in 2022. Best. Pract. Res. Clin. Rheumatol. 36, 101775. doi:10.1016/j.berh.2022.101775
Chandra, A., and Rajawat, J. (2021). Skeletal aging and osteoporosis: Mechanisms and therapeutics. Int. J. Mol. Sci. 22, 3553. doi:10.3390/ijms22073553
Chen, H., and Boutros, P. C. (2011). VennDiagram: A package for the generation of highly-customizable Venn and euler diagrams in R. BMC Bioinforma. 12, 35. doi:10.1186/1471-2105-12-35
Davis, S., and Meltzer, P. S. (2007). GEOquery: A bridge between the gene expression omnibus (GEO) and BioConductor. Bioinformatics 23, 1846–1847. doi:10.1093/bioinformatics/btm254
Dawoud, M. M., Aiad, H. A.-S., Bahbah, A. M. N. H., and Shaban, M. I. (2021). Comparative study of immunohistochemical expression of ERG and MAGI2 in prostatic carcinoma. Ann. Diagn Pathol. 52, 151727. doi:10.1016/j.anndiagpath.2021.151727
Debnath, S., Yallowitz, A. R., McCormick, J., Lalani, S., Zhang, T., Xu, R., et al. (2018). Discovery of a periosteal stem cell mediating intramembranous bone formation. Nature 562, 133–139. doi:10.1038/s41586-018-0554-8
Diab, D. L., and Watts, N. B. (2021). Updates on osteoporosis in men. Endocrinol. Metab. Clin. North Am. 50, 239–249. doi:10.1016/j.ecl.2021.03.001
Dunn, T., Praissman, L., Hagag, N., and Viola, M. V. (1994). ERG gene is translocated in an Ewing’s sarcoma cell line. Cancer Genet. Cytogenet 76, 19–22. doi:10.1016/0165-4608(94)90063-9
Goswami, J., Hernández-Santos, N., Zuniga, L. A., and Gaffen, S. L. (2009). A bone-protective role for IL-17 receptor signaling in ovariectomy-induced bone loss. Eur. J. Immunol. 39, 2831–2839. doi:10.1002/eji.200939670
Gu, Z., Eils, R., and Schlesner, M. (2016). Complex heatmaps reveal patterns and correlations in multidimensional genomic data. Bioinformatics 32, 2847–2849. doi:10.1093/bioinformatics/btw313
Ivashkiv, L. B., Zhao, B., Park-Min, K.-H., and Takami, M. (2011). Feedback inhibition of osteoclastogenesis during inflammation by IL-10, M-CSF receptor shedding, and induction of IRF8. Ann. N. Y. Acad. Sci. 1237, 88–94. doi:10.1111/j.1749-6632.2011.06217.x
Jin, W., Chen, F., Fang, Q., Mao, G., and Bao, Y. (2023). Oligosaccharides from Sargassum thunbergii inhibit osteoclast differentiation via regulation of IRF-8 signaling. Exp. Gerontol. 172, 112057. doi:10.1016/j.exger.2022.112057
Kanematsu, M., Sato, T., Takai, H., Watanabe, K., Ikeda, K., and Yamada, Y. (2000). Prostaglandin E2 induces expression of receptor activator of nuclear factor-kappa B ligand/osteoprotegrin ligand on pre-B cells: Implications for accelerated osteoclastogenesis in estrogen deficiency. J. Bone Min. Res. 15, 1321–1329. doi:10.1359/jbmr.2000.15.7.1321
Kitajima, S., Asahina, H., Chen, T., Guo, S., Quiceno, L. G., Cavanaugh, J. D., et al. (2018). Overcoming resistance to dual innate immune and MEK inhibition downstream of KRAS. Cancer Cell 34, 439–452. doi:10.1016/j.ccell.2018.08.009
Kitami, S., Tanaka, H., Kawato, T., Tanabe, N., Katono-Tani, T., Zhang, F., et al. (2010). IL-17A suppresses the expression of bone resorption-related proteinases and osteoclast differentiation via IL-17RA or IL-17RC receptors in RAW264.7 cells. Biochimie 92, 398–404. doi:10.1016/j.biochi.2009.12.011
Lei, H., Schmidt-Bleek, K., Dienelt, A., Reinke, P., and Volk, H.-D. (2015). Regulatory T cell-mediated anti-inflammatory effects promote successful tissue repair in both indirect and direct manners. Front. Pharmacol. 6, 184. doi:10.3389/fphar.2015.00184
Li, J.-Y., Yu, M., Tyagi, A. M., Vaccaro, C., Hsu, E., Adams, J., et al. (2019). IL-17 receptor signaling in osteoblasts/osteocytes mediates PTH-induced bone loss and enhances osteocytic RANKL production. J. Bone Min. Res. 34, 349–360. doi:10.1002/jbmr.3600
Liu, C., Han, Y., Zhao, X., Li, B., Xu, L., Li, D., et al. (2021). POLR2A blocks osteoclastic bone resorption and protects against osteoporosis by interacting with CREB1. J. Cell Physiol. 236, 5134–5146. doi:10.1002/jcp.30220
Liu, Y., Wang, L., Kikuiri, T., Akiyama, K., Chen, C., Xu, X., et al. (2011). Mesenchymal stem cell-based tissue regeneration is governed by recipient T lymphocytes via IFN-γ and TNF-α. Nat. Med. 17, 1594–1601. doi:10.1038/nm.2542
Lu, Z., Cao, H., and Hu, X. (2023). Circulating miR-340-5p and miR-506-3p as two osteo-miRNAs for predicting osteoporosis in a cohort of postmenopausal women. J. Environ. Public Health 2023, 7571696. doi:10.1155/2023/7571696
Luo, C. Y., Wang, L., Sun, C., and Li, D. J. (2011). Estrogen enhances the functions of CD4(+)CD25(+)Foxp3(+) regulatory T cells that suppress osteoclast differentiation and bone resorption in vitro. Cell Mol. Immunol. 8, 50–58. doi:10.1038/cmi.2010.54
Meng, J., Zhang, X., Guo, X., Cheng, W., Qi, X., Huang, J., et al. (2021). Briarane-type diterpenoids suppress osteoclastogenisis by regulation of Nrf2 and MAPK/NF-kB signaling pathway. Bioorg Chem. 112, 104976. doi:10.1016/j.bioorg.2021.104976
Mori, Y., Hiraki, Y., Shukunami, C., Kakudo, S., Shiokawa, M., Kagoshima, M., et al. (1997). Stimulation of osteoblast proliferation by the cartilage-derived growth promoting factors chondromodulin-I and -II. FEBS Lett. 406, 310–314. doi:10.1016/s0014-5793(97)00291-3
Omata, Y., Frech, M., Lucas, S., Primbs, T., Knipfer, L., Wirtz, S., et al. (2020). Type 2 innate lymphoid cells inhibit the differentiation of osteoclasts and protect from ovariectomy-induced bone loss. Bone 136, 115335. doi:10.1016/j.bone.2020.115335
Rachner, T. D., Khosla, S., and Hofbauer, L. C. (2011). Osteoporosis: Now and the future. Lancet 377, 1276–1287. doi:10.1016/S0140-6736(10)62349-5
Ritchie, M. E., Phipson, B., Wu, D., Hu, Y., Law, C. W., Shi, W., et al. (2015). Limma powers differential expression analyses for RNA-sequencing and microarray studies. Nucleic Acids Res. 43, e47. doi:10.1093/nar/gkv007
Rivollier, A., Mazzorana, M., Tebib, J., Piperno, M., Aitsiselmi, T., Rabourdin-Combe, C., et al. (2004). Immature dendritic cell transdifferentiation into osteoclasts: A novel pathway sustained by the rheumatoid arthritis microenvironment. Blood 104, 4029–4037. doi:10.1182/blood-2004-01-0041
Roberts, J. L., Mella-Velazquez, G., Dar, H. Y., Liu, G., and Drissi, H. (2022). Deletion of IL-17ra in osteoclast precursors increases bone mass by decreasing osteoclast precursor abundance. Bone 157, 116310. doi:10.1016/j.bone.2021.116310
Sakka, S. D. (2022). Osteoporosis in children and young adults. Best. Pract. Res. Clin. Rheumatol. 36, 101776. doi:10.1016/j.berh.2022.101776
Salmena, L., Poliseno, L., Tay, Y., Kats, L., and Pandolfi, P. P. (2011). A ceRNA hypothesis: The rosetta stone of a hidden RNA language? Cell 146, 353–358. doi:10.1016/j.cell.2011.07.014
Santiago-Schwarz, F., Anand, P., Liu, S., and Carsons, S. E. (2001). Dendritic cells (DCs) in rheumatoid arthritis (RA): Progenitor cells and soluble factors contained in RA synovial fluid yield a subset of myeloid DCs that preferentially activate Th1 inflammatory-type responses. J. Immunol. 167, 1758–1768. doi:10.4049/jimmunol.167.3.1758
Sapra, L., Shokeen, N., Porwal, K., Saini, C., Bhardwaj, A., Mathew, M., et al. (2022). Bifidobacterium longum Ameliorates Ovariectomy-induced bone loss via enhancing anti-osteoclastogenic and immunomodulatory potential of regulatory B cells (Bregs). Front. Immunol. 13, 875788. doi:10.3389/fimmu.2022.875788
Sato, K., Suematsu, A., Okamoto, K., Yamaguchi, A., Morishita, Y., Kadono, Y., et al. (2006). Th17 functions as an osteoclastogenic helper T cell subset that links T cell activation and bone destruction. J. Exp. Med. 203, 2673–2682. doi:10.1084/jem.20061775
Shih, Y.-R. V., Liu, M., Kwon, S. K., Iida, M., Gong, Y., Sangaj, N., et al. (2019). Dysregulation of ectonucleotidase-mediated extracellular adenosine during postmenopausal bone loss. Sci. Adv. 5, eaax1387. doi:10.1126/sciadv.aax1387
Sr, T., E, V., Sj, M., I, P., Al, F., Vp, S., et al. (2013). Reduced dosage of ERF causes complex craniosynostosis in humans and mice and links ERK1/2 signaling to regulation of osteogenesis. Nat. Genet. 45, 308–313. doi:10.1038/ng.2539
Sun, W., Meednu, N., Rosenberg, A., Rangel-Moreno, J., Wang, V., Glanzman, J., et al. (2018). B cells inhibit bone formation in rheumatoid arthritis by suppressing osteoblast differentiation. Nat. Commun. 9, 5127. doi:10.1038/s41467-018-07626-8
Szklarczyk, D., Gable, A. L., Lyon, D., Junge, A., Wyder, S., Huerta-Cepas, J., et al. (2019). STRING v11: Protein-protein association networks with increased coverage, supporting functional discovery in genome-wide experimental datasets. Nucleic Acids Res. 47, D607–D613. doi:10.1093/nar/gky1131
Thiolat, A., Semerano, L., Pers, Y. M., Biton, J., Lemeiter, D., Portales, P., et al. (2014). Interleukin-6 receptor blockade enhances CD39+ regulatory T cell development in rheumatoid arthritis and in experimental arthritis. Arthritis Rheumatol. 66, 273–283. doi:10.1002/art.38246
Vi, L., Baht, G. S., Whetstone, H., Ng, A., Wei, Q., Poon, R., et al. (2015). Macrophages promote osteoblastic differentiation in-vivo: Implications in fracture repair and bone homeostasis. J. Bone Min. Res. 30, 1090–1102. doi:10.1002/jbmr.2422
Vilaca, T., Eastell, R., and Schini, M. (2022). Osteoporosis in men. Lancet Diabetes Endocrinol. 10, 273–283. doi:10.1016/S2213-8587(22)00012-2
von Kriegsheim, A., Baiocchi, D., Birtwistle, M., Sumpton, D., Bienvenut, W., Morrice, N., et al. (2009). Cell fate decisions are specified by the dynamic ERK interactome. Nat. Cell Biol. 11, 1458–1464. doi:10.1038/ncb1994
Wang, G., Wang, F., Zhang, L., Yan, C., and Zhang, Y. (2021). miR-133a silencing rescues glucocorticoid-induced bone loss by regulating the MAPK/ERK signaling pathway. Stem Cell Res. Ther. 12, 215. doi:10.1186/s13287-021-02278-w
Wang, X., Zhiwei, P., Ting, H., Jirigala, A., Siqin, L., Wanxiong, H., et al. (2022). Prognostic analysis and validation of diagnostic marker genes in patients with osteoporosis. Front. Immunol. 13, 987937. doi:10.3389/fimmu.2022.987937
Wickham, H. (2009). ggplot2: Elegant graphics for data analysis. New York, NY: Springer New York. doi:10.1007/978-0-387-98141-3
Yoshimura, N., Iidaka, T., Horii, C., Muraki, S., Oka, H., Kawaguchi, H., et al. (2022). Trends in osteoporosis prevalence over a 10-year period in Japan: The ROAD study 2005-2015. J. Bone Min. Metab. 40, 829–838. doi:10.1007/s00774-022-01352-4
Yu, G., Wang, L.-G., Han, Y., and He, Q.-Y. (2012). clusterProfiler: an R package for comparing biological themes among gene clusters. OMICS 16, 284–287. doi:10.1089/omi.2011.0118
Yu, X., Rong, P.-Z., Song, M.-S., Shi, Z.-W., Feng, G., Chen, X.-J., et al. (2021). lncRNA SNHG1 induced by SP1 regulates bone remodeling and angiogenesis via sponging miR-181c-5p and modulating SFRP1/Wnt signaling pathway. Mol. Med. 27, 141. doi:10.1186/s10020-021-00392-2
Yu, X., Song, M.-S., Rong, P.-Z., Chen, X.-J., Shi, L., Wang, C.-H., et al. (2022). LncRNA SNHG1 modulates adipogenic differentiation of BMSCs by promoting DNMT1 mediated Opg hypermethylation via interacting with PTBP1. J. Cell Mol. Med. 26, 60–74. doi:10.1111/jcmm.16982
Yuxuan, D., Yunyun, W., Qing, S., and Yanxia, J. (2023). Identification of hub genes associated with osteoporosis development by comprehensive bioinformatics analysis. Front. Genet. 14, 1028681. doi:10.3389/fgene.2023.1028681
Zhang, H., Chen, L., Wang, Z., Sun, Z., Shan, Y., Li, Q., et al. (2022a). Long noncoding RNA KCNQ1OT1 inhibits osteoclast differentiation by regulating the miR-128-3p/NFAT5 axis. Aging (Albany NY) 14, 4486–4499. doi:10.18632/aging.204088
Zhang, W., Zhu, Y., Chen, J., Wang, J., Yao, C., and Chen, C. (2020a). Mechanisms of miR-128-3p in inhibiting osteoblast differentiation from bone marrow-derived mesenchymal stromal cells. Mol. Med. Rep. 22, 5041–5052. doi:10.3892/mmr.2020.11600
Zhang, Y., Chen, X.-F., Li, J., He, F., Li, X., and Guo, Y. (2020b). lncRNA Neat1 stimulates osteoclastogenesis via sponging miR-7. J. Bone Min. Res. 35, 1772–1781. doi:10.1002/jbmr.4039
Zhang, Z., Chen, F., Li, S., Guo, H., Xi, H., Deng, J., et al. (2020c). ERG the modulates Warburg effect and tumor progression in cervical cancer. Biochem. Biophys. Res. Commun. 522, 191–197. doi:10.1016/j.bbrc.2019.11.079
Zhao, B., Takami, M., Yamada, A., Wang, X., Koga, T., Hu, X., et al. (2009). Interferon regulatory factor-8 regulates bone metabolism by suppressing osteoclastogenesis. Nat. Med. 15, 1066–1071. doi:10.1038/nm.2007
Zhao, S.-L., Wen, Z.-X., Mo, X.-Y., Zhang, X.-Y., Li, H.-N., Cheung, W.-H., et al. (2022a). Bone-metabolism-related serum microRNAs to diagnose osteoporosis in middle-aged and elderly women. Diagn. (Basel) 12, 2872. doi:10.3390/diagnostics12112872
Zhao, X., Zhao, D., Geng, B., Yaobin, W., and Xia, Y. (2022b). A novel ceRNA regulatory network involving the long noncoding NEAT1, miRNA-466f-3p and its mRNA target in osteoblast autophagy and osteoporosis. J. Mol. Med. Berl. 100, 1629–1646. doi:10.1007/s00109-022-02255-7
Zhao, Y., Li, W., Zhang, K., Xu, M., Zou, Y., Qiu, X., et al. (2022c). Revealing oxidative stress-related genes in osteoporosis and advanced structural biological study for novel natural material discovery regarding MAPKAPK2. Front. Endocrinol. (Lausanne) 13, 1052721. doi:10.3389/fendo.2022.1052721
Zheng, T., Wang, X., and Yim, M. (2014). Miconazole inhibits receptor activator of nuclear factor-κB ligand-mediated osteoclast formation and function. Eur. J. Pharmacol. 737, 185–193. doi:10.1016/j.ejphar.2014.04.047
Zhou, S., Zilberman, Y., Wassermann, K., Bain, S. D., Sadovsky, Y., and Gazit, D. (2001). Estrogen modulates estrogen receptor alpha and beta expression, osteogenic activity, and apoptosis in mesenchymal stem cells (MSCs) of osteoporotic mice. J. Cell Biochem. Suppl. Suppl. 36, 144–155. doi:10.1002/jcb.1096
Keywords: IL17RA, osteoporosis, gene expression omnibus, DEIRGs, diagnostic markers
Citation: Deng Y-J, Li Z, Wang B, Li J, Ma J, Xue X, Tian X, Liu Q-C, Zhang Y and Yuan B (2023) Immune-related gene IL17RA as a diagnostic marker in osteoporosis. Front. Genet. 14:1219894. doi: 10.3389/fgene.2023.1219894
Received: 08 June 2023; Accepted: 26 July 2023;
Published: 04 August 2023.
Edited by:
Lei Chen, Shanghai Maritime University, ChinaReviewed by:
Rajeev Aurora, Saint Louis University, United StatesBaohong Liu, Chinese Academy of Agricultural Sciences, China
Copyright © 2023 Deng, Li, Wang, Li, Ma, Xue, Tian, Liu, Zhang and Yuan. This is an open-access article distributed under the terms of the Creative Commons Attribution License (CC BY). The use, distribution or reproduction in other forums is permitted, provided the original author(s) and the copyright owner(s) are credited and that the original publication in this journal is cited, in accordance with accepted academic practice. No use, distribution or reproduction is permitted which does not comply with these terms.
*Correspondence: Bin Yuan, eXVhbmJpbjgyMTBAMTYzLmNvbQ==