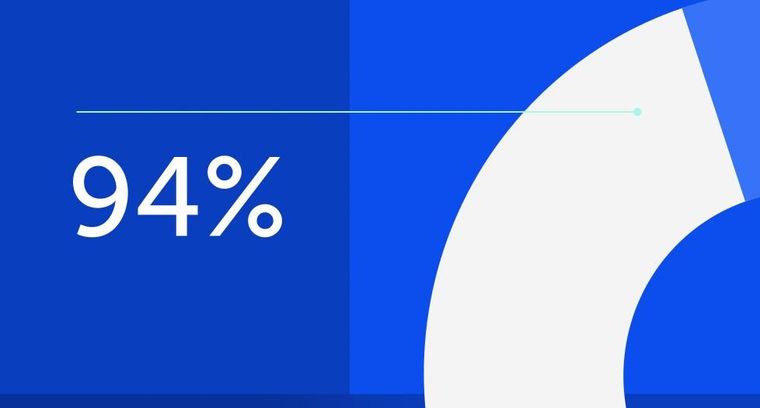
94% of researchers rate our articles as excellent or good
Learn more about the work of our research integrity team to safeguard the quality of each article we publish.
Find out more
ORIGINAL RESEARCH article
Front. Genet., 14 June 2023
Sec. Human and Medical Genomics
Volume 14 - 2023 | https://doi.org/10.3389/fgene.2023.1175864
Objective: DNA methylation plays a potential role in the pathogenesis of Alzheimer’s disease (AD). However, little is known about the global changes of blood leukocyte DNA methylome profiles from Chinese patients with mild cognitive impairment (MCI) and with AD, or the specific DNA methylation-based signatures associated with MCI and AD. In this study, we sought to dissect the characteristics of blood DNA methylome profiles in MCI- and AD-affected Chinese patients with the aim of identifying novel DNA methylation biomarkers for AD.
Methods: In this study, we profiled the DNA methylome of peripheral blood leukocytes from 20 MCI- and 20 AD-affected Chinese patients and 20 cognitively healthy controls (CHCs) with the Infinium Methylation EPIC BeadChip array.
Results: We identified significant alterations of the methylome profiles in MCI and AD blood leukocytes. A total of 2,582 and 20,829 CpG sites were significantly and differentially methylated in AD and MCI compared with CHCs (adjusted p < 0.05), respectively. Furthermore, 441 differentially methylated positions (DMPs), aligning to 213 unique genes, were overlapped by the three comparative groups of AD versus CHCs, MCI versus CHCs, and AD versus MCI, of which 6 and 5 DMPs were continuously hypermethylated and hypomethylated in MCI and AD relative to CHCs (adjusted p < 0.05), respectively, such as FLNC cg20186636 and AFAP1 cg06758191. The DMPs with an area under the curve >0.900, such as cg18771300, showed high potency for predicting MCI and AD. In addition, gene ontology and pathway enrichment results showed that these overlapping genes were mainly involved in neurotransmitter transport, GABAergic synaptic transmission, signal release from synapse, neurotransmitter secretion, and the regulation of neurotransmitter levels. Furthermore, tissue expression enrichment analysis revealed a subset of potentially cerebral cortex-enriched genes associated with MCI and AD, including SYT7, SYN3, and KCNT1.
Conclusion: This study revealed a number of potential biomarkers for MCI and AD, also highlighted the presence of epigenetically dysregulated gene networks that may engage in the underlying pathological events resulting in the onset of cognitive impairment and AD progression. Collectively, this study provides prospective cues for developing therapeutic strategies to improve cognitive impairment and AD course.
Dementia is a common syndrome characterized by deterioration in cognitive function, in which memory, language, thinking, comprehension, as well as judgement and learning capacity are often affected. According to the latest data of dementia epidemiology released by World Health Organization (https://www.who.int/news-room/fact-sheets/detail/dementia). Currently there are approximately 55 million people affected by dementia worldwide, especially in low- and middle-income countries. Moreover, almost 10 million new cases are diagnosed every year, this number is estimated to rise to 66 million and 131 million by 2030 and 2050 (Livingston et al., 2017), respectively. As a public health priority, dementia has prompted the World Health Assembly to endorse the Global Action Plan on The Public Health Response to Dementia 2017–2025, in May 2017. In China, approximately 15.07 million individuals aged 60 years and older are affected by dementia currently, of which 9.83 million are AD cases, 3.92 million are vascular dementia cases and 1.32 million are other types of dementia (Ren et al., 2022). Furthermore, approximately 38.77 million individuals are affected by mild cognitive impairment (MCI) among Chinese populations over 60 years of age (Jia et al., 2020a). In response, the Chinese government has launched a battery of plans including ‘Healthy China Action’ Plan of 2019–2030 and related policies of the 13th Five-Year Plan, to better manage dementia and related disorders (Jia et al., 2020b; Ren et al., 2022). There are many different forms of dementia, such as Alzheimer’s disease (AD), dementia with Lewy bodies, frontotemporal dementia and vascular dementia (Oh and Rabins, 2019). Late-onset AD, also known as the most common contributor of dementia (Van Cauwenberghe et al., 2016; Garcia-Blanco et al., 2017), is characterized by deposition of β-amyloid peptides and accumulation of intracellular neurofibrillary tangles (Morgan et al., 2019; van der Kant et al., 2020), which is followed by neuron death and a permanent loss of cognitive function (Wang et al., 2013; Minter et al., 2016; Lane et al., 2018). MCI, particularly amnestic MCI, often considered as an early phase of AD, is characterized by subtle impairment of memory and other cognitive functions (Petersen, 2004; Gauthier et al., 2006; Jicha et al., 2006), even though these symptoms have no obvious effects on daily living.
The pathological factors for AD are complex and heterogeneous. The incidence of AD increases with age. In addition to age, the most common cause of AD, genetic mutations are also risk factors known to confer AD susceptibility. Numerous genome-wide association studies (GWAS) on familial or sporadic AD have highlighted the hereditary and genetic predisposition of AD. For instance, mutations in amyloid precursor protein (APP) (Chartier-Harlin et al., 1991; Goate et al., 1991; Murrell et al., 1991), presenilin 1 (PSEN1) (Mullan et al., 1992; Schellenberg et al., 1992; St George-Hyslop et al., 1992; Van Broeckhoven et al., 1992; Sherrington et al., 1995), and PSEN2 genes (Levy-Lahad et al., 1995a; Levy-Lahad et al., 1995b; Rogaev et al., 1995) were the earliest identified genetic pathogenic factors causing familial AD. Subsequently, apolipoprotein E (APOE) gene ε4 and ε2 haplotypes were found associated with the risk of AD (Pericak-Vance et al., 1991; Corder et al., 1993; Strittmatter et al., 1993; Corder et al., 1994). Over the past decades, abundant GWAS works have identified that additional common or rare variants in multiple genes, such as CLU, PICALM, CR1, MS4A4E/MS4A6A, CD2AP, CD33, EPHA1, ABCA7, SORL1, CASS4, CELF1, DSG2, FERMT2, HLA-DRB1/HLA-DRB5, INPP5D, MEF2C, NME8, PTK2B, SLC24A4, RIN3, and ZCWPW1 (Rogaeva et al., 2007; Lee et al., 2008; Harold et al., 2009; Lambert et al., 2009; Jun et al., 2010; Seshadri et al., 2010; Hollingworth et al., 2011; Naj et al., 2011; Reitz et al., 2011; Lambert et al., 2013), were genetically associated with AD. These AD susceptibility genes are involved in multiple pathways including synaptic cell endocytosis and functionality, hippocampal synapse function, amyloid pathway, Tau pathology, immunoinflammatory response, lipid metabolism and transport, cell migration, axonal transport and cytoskeletal function.
However, genetic studies on identical twins revealed incomplete concordance, thus implying that in addition to genetics, environmental and related factors also influence AD pathophysiology (Breitner et al., 1995; Raiha et al., 1996; Gatz et al., 1997; Pedersen et al., 2004). Moreover, genetic variants account only for approximately 5% of all AD patients, suggesting the possibility of epigenetic variations involved in the pathology of AD (Prasad and Jho, 2019). An increasing number of studies have confirmed the role of epigenetic factors in contributing to the etiopathology and progression of AD. One such pattern is DNA methylation, which reversibly regulates gene expression and interferes with the course of disease (Holliday and Pugh, 1975; Moore et al., 2013). DNA methylation has been successfully used as a biomarker for the diagnosis of other diseases, such as cardiopathy and cancer (Meder et al., 2017; Pan et al., 2018). Currently, many epigenome-wide association studies (EWAS) have investigated the differences of DNA methylome profiles in postmortem human brain tissue biospecimens between patients with AD and matched controls, which have identified several differentially methylated loci, such as ANK1, BIN1, RPL13, CDH23 and RHBDF2, associated with cognitive decline and AD dementia progression (Bakulski et al., 2012; De Jager et al., 2014; Lord and Cruchaga, 2014; Lunnon et al., 2014; Watson et al., 2016; Ellison et al., 2017; Mano et al., 2017; Zhao et al., 2017; Gasparoni et al., 2018; Hernandez et al., 2018; Smith et al., 2018; Altuna et al., 2019; Fetahu et al., 2019; Lardenoije et al., 2019; Smith et al., 2019; Brokaw et al., 2020; Smith et al., 2021). Furthermore, additional genome-wide DNA methylation studies of peripheral blood in AD/dementia have also been performed and have revealed a number of AD-linked loci annotated to NCAPH2, LMF2, B3GALT4 and ZADH2 (Lunnon et al., 2014; Di Francesco et al., 2015; Kobayashi et al., 2016; Shinagawa et al., 2016; Madrid et al., 2018; Lardenoije et al., 2019; Vasanthakumar et al., 2020; Perez et al., 2022). Nevertheless, latent DNA methylation variation events occurs prior to detectable pathological hallmarks and visible clinical symptoms (Fransquet et al., 2020). To better understand the underlying changes of DNA methylation profiles in the early stages of AD, it is necessary to include MCI samples to identify possible signatures that can predict progression from a cognitively normal status to MCI, as well as from MCI to AD.
Numerous genome-wide methylation profiling studies of patients with AD from Caucasian populations have been performed over the past decades. However, little is known about the characteristics of DNA methylome profiles in white blood cells from MCI- and AD-affected Chinese patients, as well as the potential DNA methylation-based signatures associated with cognitive decline and AD trajectory. In this study, we revealed significant alterations in the DNA methylome profiles of blood leukocytes from Chinese patients with MCI and AD, in which several signature genes harboring differentially methylated positions might play a role in cognitive function recession and AD pathology. Furthermore, these findings indicated the presence of epigenetically dysregulated gene networks that might facilitate the development from cognitively normal state to MCI, and the subsequent transition from MCI to AD, in an integrally coordinated DNA methylation-dependent manner.
The protocols of this study were reviewed and approved by the Ethics Committee of The Second People’s Hospital of Lishui (Zhejiang, China) before recruiting subjects (approval number: 20171116–1). Prior to participant enrollment, informed written consent was acquired from each subject or legal guardian of patient. The diagnostic criteria of the National Institute of Neurological and Communicative Disorders and Stroke-AD and Related Disorders Association (NINCDS-ADRDA) (McKhann et al., 1984) and the fourth edition of the Diagnostic and Statistical Manual of Mental Disorders (DSM-IV) was used for AD diagnosis. 20 patients with AD and 20 MCI subjects were recruited from Lishui City in Zhejiang province (China), from January 2019 to December 2019. In addition, 20 cognitively healthy subjects were enrolled as controls (Table 1). The brain of each AD patient was scanned by computed tomography (CT) and magnetic resonance imaging (MRI), and all AD-affected patients were diagnosed with brain atrophy. Individuals in the MCI group were recruited from the Department of Memory in The Second People’s Hospital of Lishui. MCI subjects were scored as 1.0 or 0.5 by the memory category of the Clinical Dementia Rating Scale (CDR) (Morris, 1993) or 0.5 by total CDR. All subjects from the MCI group showed memory problems without substantial impairment in diurnal living based on Petersen’s criteria of MCI (Petersen et al., 1999).
The Chinese versions of Mini-Mental State Examination (MMSE), Screening Scale for Mild Cognitive Impairment (SMCI) and Alzheimer’s Disease-8 (AD8) were used to score the cognitive and functional status of all subjects. Demographic data and detailed clinical information for all participants are shown in Table 1. The exclusion criteria were as follows: early-onset AD or familial AD dementia; another type of neurodegenerative or mental disorders such as Parkinson’s disease, depression and schizophrenia; Diagnosed cancer/tumor such as gastric carcinoma and breast cancer; other autoimmune or inflammatory diseases such as inflammatory bowel condition; active infectious diseases included bacterial, fungal, or viral infections; obesity (body mass index ≥30.0); smoking and drinking.
Genomic DNA was extracted from peripheral white blood cells of 20 patients with AD, 20 patients with MCI and 20 healthy controls using Blood DNA Extraction Kit (QIAGEN) according to the manufacturer’s protocol, which was followed by bisulfite conversion using the EZ DNA Methylation-Direct Kit (Zymo Research, Orange, CA) and processed according to Illumina protocols. Subsequently, converted DNA was scanned with the Infinium MethylationEPIC array BeadChips (850 K chip) (Illumina Inc., California, United States). All 60 samples were processed together to minimize batch effects.
An overview of the methodological flow in this study is shown in the Supplementary Figure S1. Data analysis of raw intensity (.idat file) was performed in R software using the ChAMP pipeline (Morris et al., 2014; Tian et al., 2017) from Bioconductor with default settings. The quality control (QC) analysis started by screening samples. Samples of technical replication and from smokers, as well as samples with a p-value >0.01 in at least 5% probes, were removed from further analysis. BeadArray Controls Reporter software (https://support.illumina.com/downloads/beadarray-controls-reporter-installer.html) was used to perform experimental quality control, and no one sample with low experimental quality was excluded. Gender status was examined by adopting the “minfi” R package (Teschendorff et al., 2009). Background correction and dye-bias normalization were conducted by using the ChAMP.norm function. The initial step involved removal of low-quality probes. Probes with a detection p-value >0.01 in more than 5% samples, or with bead count <3 in at least 5% of samples, were removed. This step led to the removal of 3,973 and 7,642 probes with poor quality, respectively. Then probes related to SNPs (n = 96,381) or probes aligned to multiple locations (n = 11) were removed, as well as probes for non CpG sites (n = 2,972). Furthermore, probes on X and Y chromosomes (n = 16,697) were removed to avoid any deviation caused by gender differences. The methylation ratios of a certain CpG site were represented by beta values ranging from 0 to 1.0. The beta mixture quartile (BMIQ) method implemented in the ChAMP R package was used to adjust beta values. Significant variation (p-value <0.05) arising from the slide variable were completely removed after running the ComBat program. Following the standard procedures QC procedures described above, 722,324 probes across 60 samples, including 20 patients with AD, 20 MCI subjects and 20 unaffected controls, were available for subsequent analysis.
Cell percentage differences between heterogeneous samples, such as blood cells, between patients and unaffected controls needed to be examined and controlled in DNA methylation analysis. Cell type heterogeneity was adjusted by using the ChAMP.refbase function.
The champ. SVD () function (Teschendorff et al., 2009) was used to investigate the effects of age and sex, and the champ. runCombat () method was used to adjust age and sex confounders. An adjusted beta value matrix was used to identify differentially methylated positions (DMPs) by using the ChAMP.DMP function. DMPs were determined by comparing the beta values per single nucleotide at each cytosine ‘CpG’ locus between MCI subjects or patients with AD and cognitively healthy controls, as well as patients with AD and MCI subjects. p values were adjusted by the Benjamini–Hochberg (BH) procedure. Probes with an adjusted P (Padj) value <0.05 were considered significant. Bonferroni correction was used to perform multiple testing with a p-value <6.68 × 10−8 (corresponding to Padj value <0.05) as the significance threshold. Epigenome-wide association studies (EWAS) were performed to identify MCI- and AD-associated DMPs after regressing age and gender. EWAS was carried out by a logistic regression model implemented in GLINT (Rahmani et al., 2017).
Differentially methylated regions (DMRs) combine methylation information from multiple neighboring CpG sites, were identified by using the function ChAMP.DMR with the Lasso method (Butcher and Beck, 2015). For each DMR, a minimum number of three consecutive CpG sites and the distance between two adjacent DMPs less than 1,000 bp were required to constitute an individual DMR. Regions with a Padj value <0.05 corrected by BH method were considered significant. All DMRs were annotated by using ChAMP.import function.
Unsupervised principal component analysis (PCA) was performed by using prcomp function in R. Supervised analysis such as partial least squares-discriminant analysis (PLS-DA) was performed by the R package mixOmics (Rohart et al., 2017). A Manhattan plot was created using the R package qqman. Gene Ontology (GO) and Kyoto Encyclopedia of Genes and Genomes (KEGG) enrichment analysis was performed by using R package clusterProfiler (Yu et al., 2012). Volcano plots, bar plots, pie charts and violin plots were created by the R package ggplot2. Protein-protein interaction (PPI) analysis was performed by online tool STRING (www.string-db.org/) (Szklarczyk et al., 2021).
Ten samples from each group were selected randomly as the training data set. The rest of samples were combined as the validation data set. The R function glm was used to create linear models. Receiver operating characteristic (ROC) curve analysis was performed using pROC in R package (Robin et al., 2011), and ggplot2 was used to plot ROC curves to identify the performance of models.
Tissue enrichment analysis was performed by online TissueEnrich tools (https://tissueenrich.gdcb.iastate.edu/) (Jain and Tuteja, 2019). Human protein atlas database was used as data set (Yu N. Y. et al., 2015). Transcription factor motif enrichment analysis was performed with the AME online tool (https://meme-suite.org/meme/tools/ame) (McLeay and Bailey, 2010; Bailey et al., 2015). The 100 bp upstream and downstream sequences of target probe were used as input. The HOCOMOCO Human (v11 CORE) was used as motif database.
Bonferroni correction was used to perform multiple testing adjustment in EWAS. Benjamini–Hochberg approach was used for correction to obtain Padj value. Independent t-tests, Mann-Whitney U-tests, and White’s non-parametric t-tests were used to analyze continuous variables. Statistical analysis was performed with SPSS V19.0 software (Chicago, IL, United States). Statistical significance was tested using two-sided approach, and only a p-value <0.05 or a corrected Padj value <0.05 was considered statistically significant.
The demographic information and clinical characteristics of all subjects are shown in Table 1. In total, 20 AD-affected patients, 20 subjects with MCI and 20 cognitively healthy controls (CHCs) were enrolled. There were significant differences in age range and gender ratio between the three groups (p < 0.05); these differences were controlled well in this study. The results of singular value decomposition analysis suggested that age had no effect on the principal component (PC)-1∼8, and that sex had only a mild effect on the PC-7 (Supplementary Figure S2). In contrast, the sample group had a major effect on PC-1, and PC-3∼6, and slide had a significant effect on PC-1 and PC-5 (Supplementary Figure S2), thus implying that AD and MCI disease status and batch, but not age and sex, had dominant effects on our data. Despite this, we adjusted the age and sex along with slide confounders to exclude their effects. Participants who smoked, drank alcohol or were obese, as well as subjects diagnosed with a concurrent mental illness, cancer, autoimmune and infectious diseases, were excluded from subsequent analysis. No significant differences were observed in the number of subjects with chronic diseases such as hypertension, diabetes mellitus and coronary heart disease (p > 0.05). The MMSE and SMCI scores for patients with AD and MCI were significantly lower than that of CHCs (p < 0.05) while the AD8 score was significantly higher in patients with MCI and AD than in CHCs (p < 0.05), thus suggesting impaired cognitive function and severe dementia status in the MCI and AD patients. Moreover, brain computed tomography results showed that all AD patients exhibited typical AD symptoms, such as obvious atrophy of the temporal lobe and the cerebral gyrus, dilated temporal horn, deepened sulcus, and reduced transverse diameter of the hippocampus (Figures 1A–C), thus certifying that all of the patients recruited were definitely clinically diagnosed with AD.
FIGURE 1. Representative brain CT images of AD patients. (A) Red asterisk indicates obvious atrophy of temporal lobe and dilated temporal horn in the bilateral brain. (B) Significantly atrophied cerebral gyrus, yellow asterisk denotes deepened sulcus. (C) Red arrow represents distinctly reduced transverse diameter of hippocampus in the bilateral brain.
To identify the overall changes in DNA methylation levels of peripheral blood leukocytes in Chinese patients with MCI and AD, we extracted genomic DNA from the blood leukocytes of 20 patients with MCI, 20 patients with AD and 20 CHC samples. These samples were analyzed with Infinium Methylation EPIC array BeadChips which features probes for more than 850,000 CpGs per sample. The results of both unsupervised PCA and supervised analysis such as PLS-DA, showed a good separation of AD and MCI samples from CHCs (Supplementary Figures S3A, B), thus suggesting a significant change in the methylome profiles of peripheral leukocytes from Chinese patients with AD and MCI.
A total of 722,324 CpG sites passed standard quality control procedures; of these, 184,316 and 381,573 probes had a Padj value <0.05 (Bonferroni correction) for AD versus CHCs and MCI versus CHCs (Supplementary Figures S4A, B), respectively, thus highlighting distinct differences in global DNA methylation profiles between AD patients or MCI subjects and CHCs. Furthermore, 265,194 probes passed Bonferroni adjustment for AD versus MCI (Padj < 0.05) (Supplementary Figure S4C). When |Delta beta| thresholds were considered by volcano plots, compared to CHCs, 1,400 significantly hypermethylated and 1,182 significantly hypomethylated DMPs were identified in AD samples (Padj < 0.05, |Delta beta| > 0.1) (Figure 2A; Supplementary Table S1). In addition, 8,484 significantly hypermethylated and 12,345 significantly hypomethylated DMPs were identified in MCI versus CHCs (Padj < 0.05, |Delta beta| > 0.1) (Figure 2B; Supplementary Table S2). Moreover, 7,145 significantly hypermethylated and 4,489 significantly hypomethylated DMPs were identified in AD versus MCI (Padj < 0.05, |Delta beta| > 0.1) (Figure 2C; Supplementary Table S3). When the |Delta beta| cutoff was set at 0.2, 272 significantly hypermethylated and 179 significantly hypomethylated DMPs were observed in AD versus CHCs (Supplementary Figure S5A; Supplementary Table S4). In addition, 1,100 significantly hypermethylated and 1,327 significantly hypomethylated DMPs were identified in MCI versus CHCs (Supplementary Figure S5B; Supplementary Table S5). Furthermore, 574 significantly hypermethylated and 439 significantly hypomethylated DMPs were identified in AD versus MCI (Supplementary Figure S5C; Supplementary Table S6). In addition, significant DMRs were identified by combining signals from nearby CpG positions in the three comparative groups. Compared with CHCs, 142 and 3,831 significant DMRs were identified in AD and MCI samples (Supplementary Tables S7, S8), overlapping with 119 and 2,716 unique genes, respectively; Furthermore, 1,131 significant DMRs were identified in AD patients when compared to MCI subjects, overlapping with 911 unique genes (Supplementary Table S9). Furthermore, 45 unique genes (1.23%) were shared by the three comparative groups.
FIGURE 2. Significant differential methylated positions between AD, MCI and cognitively healthy controls. (A) Differentially methylated positions between AD and cognitively healthy controls (CHCs), Padj value <0.05, |Delta beta| cutoff >0.1. 1400 hypermethylated and 1182 hypomethylated positions. (B) Differentially methylated positions between MCI and CHCs, Padj value <0.05, |Delta beta| cutoff >0.1. 8,484 hypermethylated and 12,345 hypomethylated positions. (C) Differentially methylated positions between AD and MCI, Padj value <0.05, |Delta beta| cutoff >0.1. 7,145 hypermethylated and 4,489 hypomethylated positions.
Functional genomic regions of the significant DMPs are shown in Supplementary Figure S6. In AD versus CHCs, the majority of hypermethylated and hypomethylated DMPs were located in gene bodies and regulatory regions, including body, first Exon, 3′ UTR, 5′ UTR, TSS200, and TSS1500, while relatively fewer DMPs were aligned to other regions (Supplementary Figures S6A, B). A similar distribution pattern was also observed in MCI versus CHCs and AD versus MCI (Supplementary Figures S6C–F). Furthermore, the majority of hypermethylated DMPs were scattered in open sea areas (located >4.0 kb from a CpG island), while the minority of hypermethylated DMPs were found near or within CpG islands in AD versus CHCs (Figure 3A). However, an opposite pattern was shown with hypomethylated DMPs from AD versus CHCs (Figure 3B). Unlike AD versus CHCs, an opposite pattern was seen in the hypermethylated and hypomethylated DMPs from MCI versus CHCs (Figures 3C, D). Furthermore, most hypermethylated and hypomethylated DMPs were scattered in open sea areas, whereas fewer DMPs were near or within CpG islands in the AD versus MCI (Figures 3E, F). Ternary plots also showed the same results (Supplementary Figure S7).
FIGURE 3. Pie chart indicating the location of DMPs relative to CpG islands. (A, B) The percentage of various CpG island locations harboring significant (Padj value <0.05) hypermethylated and hypomethylated positions between the AD and CHCs. (C, D) The percentage of various CpG island locations harboring significant (Padj value <0.05) hypermethylated and hypomethylated positions between the MCI and CHCs. (E, F) The percentage of various CpG island locations harboring significant (Padj value <0.05) hypermethylated and hypomethylated positions between the AD and MCI groups. Domains are labeled with different colors. N_Shelf, 2–4 kb upstream of CpG island; N_Shore, 0–2 kb upstream of CpG island; OpenSea, >4 kb from a CpG island; S_Shelf, 2–4 kb downstream of CpG island; S_Shore, 0–2 kb downstream of CpG island.
To identify potential DMPs biomarkers related to cognitive impairment and AD, we mainly focused on the common DMPs between the three comparative groups of AD versus CHCs, MCI versus CHCs, and AD versus MCI. A total of 441 common DMPs aligning to 213 unique genes were identified (Figure 4; Supplementary Figures S8A, B; Supplementary Table S10). Of these common DMPs, 6 CpG sites were continuously and significantly hypermethylated (i.e., DNA methylation level: AD > MCI > CHCs) in MCI and AD samples compared to CHCs (Padj < 0.05), including RHOJ cg18771300, RHOJ cg07157030, RHOJ cg07189587, PARK2 cg09656629, cg22100363, and FLNC cg20186636 (Figures 5A–F), while 5 CpG sites were continuously and significantly hypomethylated (i.e., DNA methylation level CHCs > MCI > AD) in MCI and AD groups relative to CHCs (Padj < 0.05), such as cg24361198, ANKH cg02821156, cg15970769, cg22721608, and AFAP1 cg06758191 (Figures 5G–K). To further exclude the effects of age and sex confounders, the analysis of age and sex adjustment was performed. The results showed that the correction of age and sex had no dramatic influences on the identified significant DMPs, such as FLNC cg20186636 (Supplementary Figure S9). Pyrosequencing results further demonstrated that cg07157030 and cg18771300 were significantly and increasingly methylated in independent samples of MCI and AD when compared to that of CHCs (Padj < 0.05) (Figure 6).
FIGURE 4. Heatmap of the common 441 DMPs. Total of 441 significant DMPs (Padj value <0.05) were overlapped by the three comparative groups, i.e., AD versus CHCs, MCI versus CHCs, and AD versus MCI. Red and green denote upregulated and downregulated, respectively. AD group: AD 01–20, MCI group: MCI 01–20, CHCs group: Con 01–20. The heatmap was plotted using the Pheatmap package in R (v.3.3.2).
FIGURE 5. Violin plots of continuously hyper- and hypomethylated CpG sites in MCI and AD. (A–F) 6 continuously hypermethylated CpGs in MCI and AD compared to CHCs. (G–K) 5 continuously hypomethylated CpGs in MCI and AD relative to CHCs. Patients with AD and MCI, and CHCs are labeled with red, purple, and green, respectively. The Y-axis represents the beta value of each CpG site. BH procedure was used for correction to obtain Padj value. *: Padj < 0.05, **: Padj < 0.01, ***: Padj < 0.001.
FIGURE 6. cg07157030 and cg18771300 were increasingly methylated in MCI and AD. The two DMPs of cg07157030 and cg18771300 were validated by pyrosequencing in independent samples of MCI, AD and CHCs. The BH procedure was used for correction to obtain a Padj value. *: Padj < 0.05, ***: Padj < 0.001.
The results of ROC analysis showed that several DMPs had an area under the curve (AUC) > 0.900 in predicting MCI event (Figure 7A), for instance, cg18771300 (AUC = 0.988, confidence interval:0.955–1.000, specificity = 100.00%, sensitivity = 95.00%), cg20186636 (AUC = 0.985, confidence interval:0.950–1.000, specificity = 95.00%, sensitivity = 95.00%), cg22721608 (AUC = 0.970, confidence interval:0.910–1.000, specificity = 100.00%, sensitivity = 90.00%), cg07157030 (AUC = 0.970, confidence interval:0.915–1.000, specificity = 100.00%, sensitivity = 85.00%), cg07189587 (AUC = 0.965, confidence interval:0.900–1.000, specificity = 100.00%, sensitivity = 85.00%) and cg15970769 (AUC = 0.943, confidence interval:0.838–1.000, specificity = 95.00%, sensitivity = 95.00%) (Supplementary Table S11). In addition, several combinations of two DMPs had an AUC >0.9000 in predicting MCI and AD conversion, such as cg15970769 and cg18771300 (AUC = 0.990, confidence interval:0.940–1.000, specificity = 90.00%, sensitivity = 100.00%), cg09656629 and cg07189587 (AUC = 0.950, confidence interval:0.820–1.000, specificity = 100.00%, sensitivity = 90.00%), cg02821156 and cg18771300 (AUC = 0.938, confidence interval:0.792–1.000, specificity = 100.00%, sensitivity = 83.33%), cg15970769 and cg24361198 (AUC = 0.929, confidence interval:0.798–1.000, specificity = 88.89%, sensitivity = 81.82%), cg02821156 and cg07157030 (AUC = 0.929, confidence interval:0.778–1.000, specificity = 90.91%, sensitivity = 88.89%), as well as cg15970769 and cg09656629 (AUC = 0.919, confidence interval:0.758–1.000, specificity = 77.78%, sensitivity = 100.00%) (Figure 7B). A single DMP had reduced potential for predicting the transition of MCI to AD (Supplementary Figure S10). In addition, another combination of DMPs also showed potential ability for predicting MCI and AD events (Supplementary Table S11). Collectively, these data suggested that these combinations of significant DMPs have the potential to act as biomarkers for the prediction of AD onset and progression.
FIGURE 7. ROC analysis of several CpG position for predicting MCI and AD events. (A) Receiver operating characteristic (ROC) curves analysis of 6 DMPs including cg15970769, cg22721608, cg18771300, cg07157030, cg07189587, and cg20186636 in predicting the transition of CHCs to MCI. (B) ROC analysis of several combinations of two CpG sites, such as cg15970769 and cg24361198, cg15970769 and cg18771300, cg15970769 and cg09656629, cg02821156 and cg07157030, cg02821156 and cg18771300, and cg09656629 and cg07189587, in predicting MCI and AD conversion. ROC analysis was performed using pROC in the R package.
To further investigate the function of epigenetically dysregulated genes harboring the common 441 DMPs, we performed GO and KEGG pathway enrichment analysis, as well as PPI and tissue expression enrichment analyses. GO annotation results showed that these overlapping genes were mainly enriched in multiple biological processes of neural activities, such as neurotransmitter transport, GABAergic synaptic transmission, signal release from synapse, neurotransmitter secretion, and regulation of neurotransmitter levels (Figure 8A). Furthermore, the proteins encoded by these epigenetically dysregulated genes were primarily localized in synaptic membrane, synaptic vesicle membrane, and transport vesicle membrane (Figure 8B). The main function of these common genes was aspartic type/metallo-endopeptidase activity (Figure 8C). In addition, these epigenetically dysregulated genes involved in multiple pathways, including neuroactive ligand-receptor interaction, MAPK signaling, and cell adhesion (Figure 8D). Moreover, TissueEnrich tools analysis showed identified 36 tissue-specific overlapping genes, which encoded proteins that were enriched significantly in the cerebral cortex (Fold change = 2.58, -Log10Padj = 5.42), such as SYT7, SYN3, and KCNT1 (Figures 8E, F; Supplementary Table S12). The results of PPI analysis revealed that the proteins encoded by these common genes constitute complex and tight networks (Supplementary Figure S11). Taken together, these epigenetically dysregulated genes might play an important role in cognitive impairment and AD etiology.
FIGURE 8. Functional enrichment analysis of epigenetically dysregulated genes harboring the common 441 DMPs. (A–C) Gene ontology enrichment analysis results of the 213 unique genes harboring the common 441 DMPs. (A–C) represents enrichment analysis of biological process, cellular component and molecular function, respectively. (D) KEGG pathway enrichment analysis results. (E, F) Tissue-specific expression enrichment analysis of the overlapping 213 genes, which was performed by using online TissueEnrich tools.
It was well known that differential methylation can occur at specific sites (Hannon et al., 2018). To explore the underlying transcription factor binding motif around the common 441 DMPs, we performed enrichment analysis of the transcription factor binding motif. All of the significantly enriched transcription factors and their binding motifs are shown in Supplementary Figure S12. As expected, some identified transcriptional factors recognize and bind to purine- or GC-rich motifs among the common 213 genes. For instance, KLF12, KLF10, as well as SP2 and SP4 bind to GC-rich motifs, while ETV1 and ETV6 recognize purine-rich sequences (Supplementary Figure S12).
Epigenetic mechanisms such as DNA methylation, play an important role in the etiopathology of neurodegenerative disorders including AD, dementia with Lewy bodies, vascular dementia, Parkinson’s disease and AD-like conditions (Fransquet et al., 2018; Fransquet and Ryan, 2019; Li et al., 2019). Previous study suggested that various neurodegenerative diseases shared similar aberrant DNA methylation pattern in certain gene set (Sanchez-Mut et al., 2016). However, objective and reliable biomarkers for the early diagnosis of AD dementia are still absent (Zetterberg and Burnham, 2019), which would impede the decisions about effective prevention and timely interventions before the appearance of clinical symptoms (Huang et al., 2020).
There were some studies suggested DNA methylation levels reduced in certain brain region from patients with AD or suffering aging compared to controls (Wang et al., 2008; Mastroeni et al., 2009; Mastroeni et al., 2010; Hernandez et al., 2011; Mastroeni et al., 2011; Chouliaras et al., 2013), whereas other studies indicated the level of DNA methylation was increased in AD patients relative to healthy controls (Bollati et al., 2011; Coppieters et al., 2014; Di Francesco et al., 2015). DNA methylation within the brain plays a critical role in memory (Miller and Sweatt, 2007; Miller et al., 2010). Also, DNA methylation has been suggested play an indispensable role in the etiopathology of AD (Mastroeni et al., 2010; Tong et al., 2015). Aberrant epigenetic changes in CpG islands may enhance the pathology of late-onset AD (Wang et al., 2008). A number of studies indicated that amyloidogenic pathway-involved genes, such as amyloid precursor protein (APP) (West et al., 1995; Tohgi et al., 1999; Wang et al., 2008; Barrachina and Ferrer, 2009; Hou et al., 2013; Iwata et al., 2014), β-secretase 1 (BACE1) (Do Carmo et al., 2016; Li et al., 2019), presenilin 1 (PSEN1) (Fuso et al., 2005; Fuso et al., 2008), sortilin-related receptor 1 (SORL1) (Scherzer et al., 2004; Yu L. et al., 2015), and neprilysin (NEP) (Chen et al., 2009; Nagata et al., 2018), were differentially methylated in AD patients or in animal model of AD compared to that of controls, even though current therapeutic strategies targeting Aβ are not satisfactory in AD treatment (Panza et al., 2019). Interestingly, one recent study revealed that aberrant autolysosome acidification-induced autophagy barrier, but not amyloidogenic pathway, was likely to be the most fundamental causal factor for AD (Lee et al., 2022), which might provide us with a more promising therapeutic strategy against AD onset and progression. Furthermore, a previous study showed that abnormal methylation in circadian genes such as CRY1 and PER1 leads to dementia symptoms (Liu et al., 2008). Moreover, one recent epigenome-wide association study identified that HOXB6 gene was robustly hypermethylated in MCI and AD blood samples compared with healthy controls (Roubroeks et al., 2020), suggesting HOXB6 gene hypermethylation signature may be potential biomarker for the diagnosis of MCI and AD.
In this study, we assessed the global changes of leukocyte DNA methylation in MCI- and AD-affected Chinese patients compared to that of cognitively healthy controls and reported multiple potential DNA methylation-based signatures associated with cognitive decline and AD, including CpG positions harbored in RHOJ, PARK2, FLNC, ANKH, and AFAP1 genes. A previous study suggested that the Ras homolog (Rho) kinase pathway was changed in leukocytes and the brains of subjects with Huntington’s Disease (Narayanan et al., 2016). Ras homolog gene family member A (RhoA) involved in vascular dementia and serves as potential targets of new drugs for vascular dementia treatment (Wang et al., 2018). Furthermore, a role of aberrant RhoA signaling involved in multiple neurodegenerative disease such as AD, Parkinson’s disease, and Huntington’s disease (Schmidt et al., 2022). Fasudil, the first clinically administered inhibitor of Ras homolog-associated kinase, and is currently used as a therapeutic target for neurodegenerative disorders (Wang et al., 2022), suggesting that drugs targeting Rho/Rho-associated kinases have the potential to alleviate neurodegenerative conditions such as AD. In this study, we found that the methylation level of three significant CpG sites, including cg18771300, cg07189587 and cg07157030 within Ras homology family member J (RHOJ) gene, was significantly elevated in MCI and AD samples compared with cognitively healthy controls (Padj < 0.05). These findings proposed a potential role of RHOJ in epigenetic regulation of cognitive impairment and AD etiopathology. The three remarkedly hypermethylated CpG sites, includes cg18771300, cg07189587 and cg07157030, may be able to serve as reliable biomarkers to predict AD onset and progression. Furthermore, RHOJ might have the potency to be developed as a potential therapeutic target against AD progression in the future.
It is well known that cognitive decline and AD dementia are highly complex conditions, which is caused by both genetic and environmental factors (Kivipelto et al., 2018; Lourida et al., 2019). Apart from ethnic background, environmental factors such as geographical conditions, lifestyle and dietary habits, can also elicit epigenetic alterations like DNA methylation changes that have been manifested to be related with dementia both in peripheral blood and in brain (Fransquet et al., 2018). Therefore, the epigenome landscape of AD-affected Chinese patients is likely to differ from that of Caucasian population with AD. Indeed, our study found that the DNA methylome of peripheral blood cells from Chinese patients with AD was significantly different from those of AD-affected Caucasian patients. Previous studies have highlighted the association of ANK1 and BIN1 methylation changes with AD dementia neuropathology in a Caucasian population (De Jager et al., 2014; Lunnon et al., 2014; Chibnik et al., 2015; Watson et al., 2016; Salcedo-Tacuma et al., 2019; Semick et al., 2019). In particular, altered DNA methylation has been shown to regulate the expression of BIN1 (Wechsler-Reya et al., 1997), and several studies have suggested that BIN1 expression was changed in the AD brain (Chapuis et al., 2013; Glennon et al., 2013; De Rossi et al., 2016). Another study on Chinese patients with AD suggested that UQCRC1 was hypermethylated in AD-affected patients relative to healthy controls and found that UQCRC1 hypermethylation was notably associated with the expression levels of CTSB, CTSD, DDT and NRD1 (Ma et al., 2016). In the current study, we identified the most significant DMPs (Padj < 0.05), including cg18771300, cg07157030, cg07189587, cg09656629, cg20186636, cg02821156, and cg06758191, in Chinese patients with AD relative to cognitively healthy controls, which aligned to RHOJ, PARK2, FLNC, ANKH, and AFAP1 genes (Figure 5), respectively. These significant DMPs was continuously hypermethylated or hypomethylated in MCI and AD compared with cognitively healthy controls, implying that these DNA methylation-based signatures have the potential as a biomarker for MCI and AD diagnosis. The inconsistent results from different AD methylome studies probably due to multiple possible factors, for example, distinct ethic background (Caucasian or Chinese population), the type of tissue collected (postmortem human brain tissue or blood cell), sampling time point during disease course (early or late stage), different sample sizes, as well as various environmental factors.
However, our current study had several limitations. First, the sample size of patients with MCI and AD, as well as non-dementia controls, was relatively small, which might give rise to inaccurate results. Given that the small sample size would limit the power to detect differentially methylated sites and dysregulated genes (Zhang et al., 2020), we now need to perform a validation assay on an independent sample cohort in our further studies. Second, the age range and sex ratio of all participants recruited in this study were very different when compared between the three comparative groups, thus creating more difficulty when analyzing the methylome data. To further exclude the effects of age and sex, we adjusted age and sex confounders when identifying DMPs, even though the results of singular value decomposition analysis suggested that age had no effect on the principal component (PC)-1∼8, and sex had only a mild effect on the PC-7 (Supplementary Figure S2). Unlike age and sex confounders, the sample group had a major effect on PC-1, and PC-3∼6; moreover, slide confounders had a major effect on PC-1 and PC-5 (Supplementary Figure S2), thus implying AD and MCI disease status and batch, but not age and gender, have dominant effects on the results in our current study. Indeed, further analysis showed that the adjustment of age and sex had no dramatic influence on the significant DMP and gene signatures we identified, such as FLNC cg20186636. However, the standard correction procedure might not completely eliminate the effects of age and sex. Thus, the identified DMP signatures and related genes need to be further verified in expanded subject populations in our future studies. Third, our results indicated that the characteristics of blood leukocyte DNA methylation was significantly changed within a subset of genes, which may be enriched in the cerebral cortex (Figures 8E, F). Although communications existed between the brain tissue and peripheral blood, particularly in the status of disease; however, not all alterations of MCI- and AD-associated DNA methylation found in the blood cells may occurred in the brain tissue and functionally participated in AD pathology. Hence, postmortem human brain tissue biospecimens need to be investigated to dissect the underlying signature genes involved in AD-related processes that occur in the brain. Fourth, our study only investigated the cross-sectional cohorts of MCI and AD, it is essential to conduct long-term clinical follow-up study to observe the conversion of MCI to AD, as well as identify the preclinical changes of MCI and AD subjects. This could lead us to reveal the bona fide DNA methylation-based signatures associated with the course of AD, which might serve as a biomarker for early diagnosis and therapeutic targets of AD. Fifth, it is well known that the amount of specific blood cell types is mildly altered in AD and MCI (Lunnon et al., 2012). Even though the proportions of different blood cells have been controlled in this study, single types of blood cell or a single-cell DNA methylome strategy should be more suitable for identifying MCI- and AD-associated signatures (Karemaker and Vermeulen, 2018). Sixth, our present study has identified a number of potential DNA methylation-based biosignatures of MCI and AD, but the exact role of these signature genes in cognitive function impairment and AD etiopathology is not clear. In the future, the AD relevance of these DNA methylation biomarkers should be investigated in parallel with functional studies of novel cognitive decline-associated genes should be carried out in vitro and in animal models to provide stronger evidence to support differential DNA methylation modulation of AD pathogenesis. This could enhance a deeper understanding of epigenetic and environmental stimuli of cognitive deterioration and AD pathology.
In summary, our study suggested significant differences in the global methylome profiles in the genomes of blood leukocytes between Chinese patients with MCI/AD and cognitively healthy controls. We report multiple differentially methylated CpG positions related to AD, of which epigenetically dysregulated signature genes such as RHOJ were highlighted. Given that the pathophysiology of AD dementia initiates many years, even decades, before overt clinical symptoms (Sperling et al., 2011). Hence, the identification of a reliable biomarker is crucial for timely and effective interventional strategies. The findings of current study might contribute to determine novel potential blood DNA methylation-based biomarkers for the diagnosis of AD onset and progression in Chinese populations.
The datasets presented in this study can be found in online repositories. The names of the repository/repositories and accession number(s) can be found in the article/Supplementary Material. The raw DNA methylome data used in this study is publicly available on the Gene Expression Omnibus database with the accession number GSE208623. Further inquiries can be directed to the corresponding authors.
The studies involving human participants were reviewed and approved by The Ethics Committee of the Second People’s Hospital of Lishui. The patients/participants provided their written informed consent to participate in this study.
SW: conceptualisation, study design, funding acquisition, sample collection; FY: literature search, study design, investigation, data collection, data analysis, figures, data interpretation, writing-original draft, and project administration; SC: data analysis, data curation, methodology, formal analysis, and visualization; BW: literature search, data analysis, methodology; WW: literature search, data analysis, methodology; HL: literature search, data curation, samples collection; LY: supervision, and writing-review and editing; LH: supervision, and writing-review and editing; XL: conceptualisation, supervision, and writing-review and editing; LS: literature search, conceptualisation, funding acquisition, supervision, and writing-review and editing; SQ: conceptualisation, funding acquisition, project administration, supervision, and writing-review and editing. All authors contributed to the article and approved the submitted version.
This study was funded by grants from the S&T Major Project of Lishui City (grant numbers: 2017ZDYF04, 2017YSKZ-01) and the Postdoctoral Fund of Affiliated Hospital of Zunyi Medical University (grant number: 283767).
We thank all of the recruited subjects, including patients with mild cognitive impairment/AD dementia and cognitively healthy volunteers, for participating in this study.
The authors declare that the research was conducted in the absence of any commercial or financial relationships that could be construed as a potential conflict of interest.
All claims expressed in this article are solely those of the authors and do not necessarily represent those of their affiliated organizations, or those of the publisher, the editors and the reviewers. Any product that may be evaluated in this article, or claim that may be made by its manufacturer, is not guaranteed or endorsed by the publisher.
The Supplementary Material for this article can be found online at: https://www.frontiersin.org/articles/10.3389/fgene.2023.1175864/full#supplementary-material
SUPPLEMENTARY FIGURE S1 | An overview of the methodological flow.
SUPPLEMENTARY FIGURE S2 | Singular value decomposition analysis of the effects of confounders. The effects of all confounders, including age, sex, sample group, sample well, slide, and array, were investigated by performing singular value decomposition (SVD) analysis via champ. SVD() function.
SUPPLEMENTARY FIGURE S3 | PCA and PLS-DA models for separating Alzheimer’s disease, mild cognitive impairment, and cognitively healthy controls. (A) PCA plot. For X variable dataset, model interpretability R2X = 0.223. (B) PLS-DA plot. The respective model interpretability for X and Y variable dataset was R2X = 0.340 and R2Y = 0.613, model predictability Q2 = 1.000. AD: Alzheimer’s disease; MCI: mild cognitive impairment; CHCs: cognitively healthy controls.
SUPPLEMENTARY FIGURE S4 | Manhattan plot showing the top hits for AD and MCI group. (A) AD versus CHCs group, (B) MCI versus CHCs group, (C) AD versus MCI group. Manhattan plot of all probes across the whole genome illustrating P values (Y-axis, -log10 scale) against genomic location (X-axis). Chromosomes are distinguished by different colors. The red horizontal solid line represents –log10(6.68×10-8) (corresponding to the Bonferroni adjusted P value = 0.05).
SUPPLEMENTARY FIGURE S5 | Volcano plot of significant differential methylated positions between AD, MCI and CHCs. (A) Differentially methylated positions between AD and CHCs, Padj value < 0.05, |Delta beta| cutoff > 0.2. 272 hypermethylated and 179 hypomethylated positions. (B) Differentially methylated positions between MCI and CHCs, Padj value < 0.05, |Delta beta| cutoff > 0.2. 1100 hypermethylated and 1327 hypomethylated positions. (C) Differentially methylated positions between AD and MCI, Padj value < 0.05, |Delta beta| cutoff > 0.2. 574 hypermethylated and 439 hypomethylated positions.
SUPPLEMENTARY FIGURE S6 | Bar plots demonstrating the functional genomic regions distribution patterns of differentially methylated positions. (A) and (B) represents the number of various genomic regions harboring significant (Padj value < 0.05) hypermethylated and hypomethylated positions from the comparative group of AD versus CHCs, respectively. (C) and (D) represents the number of various genomic regions harboring significant (Padj value < 0.05) hypermethylated and hypomethylated positions from the comparative group of MCI versus CHCs, respectively. (E) and (F) represents the number of various genomic regions harboring significant (Padj value < 0.05) hypermethylated and hypomethylated positions from the comparative group of AD versus MCI, respectively. Colors represent different regions. TSS1500 and TSS200 means the upstream 1500 and 200 base-pairs of the transcription start site (TSS), respectively; 5´UTR means the region at the 5’ end of a mature transcript preceding the initiation codon that is not translated into protein; 3´UTR means the region at the 3’ end of a mature transcript preceding the stop codon that is not translated into protein; 1stExon means the first exon of certain gene; Body means the sequence from the initiation codon to the stop codon.
SUPPLEMENTARY FIGURE S7 | Ternary plots demonstrating the genomic distribution pattern of significant CpG sites. (A) and (B) shows the genomic distribution pattern of significantly (Padj < 0.05) hypermethylated and hypomethylated CpG sites of the three comparative groups of AD versus CHCs, MCI versus CHCs, and AD versus MCI, respectively.
SUPPLEMENTARY FIGURE S8 | The common 441 differentially methylated positions overlapped by the three comparative groups. (A) Total of 441 differentially methylated positions were shared by the comparative groups of AD versus CHCs, MCI versus CHCs, and AD versus MCI. (B) The common 441 DMPs were aligned to 213 unique genes.
SUPPLEMENTARY FIGURE S9 | Violin plots of differentially methylated CpG positions compared between AD, MCI, and CHCs group after age and sex adjustment. (A) FLNC cg20186636. (B) PARK2 cg09656629. (C-E) RHOJ cg18771300, cg07189587, and cg07157030. (F) cg22721608. (G) cg24361198. (H) AFAP1 cg06758191. (I) ANKH cg02821156, and (J) cg15970769. Patients with AD and MCI, and CHCs are labeled with red, purple, and green, respectively. The Y-axis represents the beta value of each CpG site. BH procedure was used for correction to obtain Padj value. *: Padj < 0.05, **: Padj < 0.01, ***: Padj < 0.001.
SUPPLEMENTARY FIGURE S10 | ROC analysis of several CpG positions for predicting AD event. Receiver operating characteristic (ROC) curves analysis of 7 DMPs including cg15970769, cg24361198, cg18771300, cg02821156, cg07157030, cg09656629, and cg07189587 in predicting the transition of MCI to AD. ROC analysis was performed using pROC in the R package.
SUPPLEMENTARY FIGURE S11 | Protein-protein interaction analysis of all proteins encoded by the common 213 genes. Protein-protein interaction (PPI) analysis was performed on all proteins encoded by the overlapping 213 genes between AD versus CHCs, MCI versus CHCs, and AD versus MCI. PPI analysis was carried out by using online tool STRING.
SUPPLEMENTARY FIGURE S12 | Transcription factor binding motif enrichment analysis of the overlapping 441 DMPs. Transcription factor motif enrichment analysis of the 441 differentially methylated positions (+-100 bp) in 213 genes. +-100bp sequences around target CpG were acquired by using hg19(GRch37).
Altuna, M., Urdanoz-Casado, A., Sanchez-Ruiz de Gordoa, J., Zelaya, M. V., Labarga, A., Lepesant, J. M. J., et al. (2019). DNA methylation signature of human hippocampus in Alzheimer's disease is linked to neurogenesis. Clin. Epigenetics 11 (1), 91. doi:10.1186/s13148-019-0672-7
Bailey, T. L., Johnson, J., Grant, C. E., and Noble, W. S. (2015). The MEME suite. Nucleic acids Res. 43 (W1), W39–W49. doi:10.1093/nar/gkv416
Bakulski, K. M., Dolinoy, D. C., Sartor, M. A., Paulson, H. L., Konen, J. R., Lieberman, A. P., et al. (2012). Genome-wide DNA methylation differences between late-onset Alzheimer's disease and cognitively normal controls in human frontal cortex. J. Alzheimer's Dis. JAD 29 (3), 571–588. doi:10.3233/JAD-2012-111223
Barrachina, M., and Ferrer, I. (2009). DNA methylation of Alzheimer disease and tauopathy-related genes in postmortem brain. J. Neuropathol. Exp. Neurol. 68 (8), 880–891. doi:10.1097/NEN.0b013e3181af2e46
Bollati, V., Galimberti, D., Pergoli, L., Dalla Valle, E., Barretta, F., Cortini, F., et al. (2011). DNA methylation in repetitive elements and Alzheimer disease. Brain, Behav. Immun. 25 (6), 1078–1083. doi:10.1016/j.bbi.2011.01.017
Breitner, J. C., Welsh, K. A., Gau, B. A., McDonald, W. M., Steffens, D. C., Saunders, A. M., et al. (1995). Alzheimer's disease in the national academy of sciences-national research council registry of aging twin veterans. III. Detection of cases, longitudinal results, and observations on twin concordance. Archives neurology 52 (8), 763–771. doi:10.1001/archneur.1995.00540320035011
Brokaw, D. L., Piras, I. S., Mastroeni, D., Weisenberger, D. J., Nolz, J., Delvaux, E., et al. (2020). Cell death and survival pathways in alzheimer's disease: An integrative hypothesis testing approach utilizing -omic data sets. Neurobiol. aging 95, 15–25. doi:10.1016/j.neurobiolaging.2020.06.022
Butcher, L. M., and Beck, S. (2015). Probe Lasso: A novel method to rope in differentially methylated regions with 450K DNA methylation data. Methods 72, 21–28. doi:10.1016/j.ymeth.2014.10.036
Chapuis, J., Hansmannel, F., Gistelinck, M., Mounier, A., Van Cauwenberghe, C., Kolen, K. V., et al. (2013). Increased expression of BIN1 mediates Alzheimer genetic risk by modulating tau pathology. Mol. psychiatry 18 (11), 1225–1234. doi:10.1038/mp.2013.1
Chartier-Harlin, M. C., Crawford, F., Houlden, H., Warren, A., Hughes, D., Fidani, L., et al. (1991). Early-onset Alzheimer's disease caused by mutations at codon 717 of the beta-amyloid precursor protein gene. Nature 353 (6347), 844–846. doi:10.1038/353844a0
Chen, K. L., Wang, S. S., Yang, Y. Y., Yuan, R. Y., Chen, R. M., and Hu, C. J. (2009). The epigenetic effects of amyloid-beta(1-40) on global DNA and neprilysin genes in murine cerebral endothelial cells. Biochem. biophysical Res. Commun. 378 (1), 57–61. doi:10.1016/j.bbrc.2008.10.173
Chibnik, L. B., Yu, L., Eaton, M. L., Srivastava, G., Schneider, J. A., Kellis, M., et al. (2015). Alzheimer's loci: Epigenetic associations and interaction with genetic factors. Ann. Clin. Transl. Neurol. 2 (6), 636–647. doi:10.1002/acn3.201
Chouliaras, L., Mastroeni, D., Delvaux, E., Grover, A., Kenis, G., Hof, P. R., et al. (2013). Consistent decrease in global DNA methylation and hydroxymethylation in the hippocampus of Alzheimer's disease patients. Neurobiol. aging 34 (9), 2091–2099. doi:10.1016/j.neurobiolaging.2013.02.021
Coppieters, N., Dieriks, B. V., Lill, C., Faull, R. L., Curtis, M. A., and Dragunow, M. (2014). Global changes in DNA methylation and hydroxymethylation in Alzheimer's disease human brain. Neurobiol. aging 35 (6), 1334–1344. doi:10.1016/j.neurobiolaging.2013.11.031
Corder, E. H., Saunders, A. M., Strittmatter, W. J., Schmechel, D. E., Gaskell, P. C., Small, G. W., et al. (1993). Gene dose of apolipoprotein E type 4 allele and the risk of Alzheimer's disease in late onset families. Science 261 (5123), 921–923. doi:10.1126/science.8346443
Corder, E. H., Saunders, A. M., Risch, N. J., Strittmatter, W. J., Schmechel, D. E., Gaskell, P. C., et al. (1994). Protective effect of apolipoprotein E type 2 allele for late onset Alzheimer disease. Nat. Genet. 7 (2), 180–184. doi:10.1038/ng0694-180
De Jager, P. L., Srivastava, G., Lunnon, K., Burgess, J., Schalkwyk, L. C., Yu, L., et al. (2014). Alzheimer's disease: Early alterations in brain DNA methylation at ANK1, BIN1, RHBDF2 and other loci. Nat. Neurosci. 17 (9), 1156–1163. doi:10.1038/nn.3786
De Rossi, P., Buggia-Prevot, V., Clayton, B. L., Vasquez, J. B., van Sanford, C., Andrew, R. J., et al. (2016). Predominant expression of Alzheimer's disease-associated BIN1 in mature oligodendrocytes and localization to white matter tracts. Mol. Neurodegener. 11 (1), 59. doi:10.1186/s13024-016-0124-1
Di Francesco, A., Arosio, B., Falconi, A., Micioni Di Bonaventura, M. V., Karimi, M., Mari, D., et al. (2015). Global changes in DNA methylation in Alzheimer's disease peripheral blood mononuclear cells. Brain, Behav. Immun. 45, 139–144. doi:10.1016/j.bbi.2014.11.002
Do Carmo, S., Hanzel, C. E., Jacobs, M. L., Machnes, Z., Iulita, M. F., Yang, J., et al. (2016). Rescue of early bace-1 and global DNA demethylation by S-adenosylmethionine reduces amyloid pathology and improves cognition in an alzheimer's model. Sci. Rep. 6, 34051. doi:10.1038/srep34051
Ellison, E. M., Bradley-Whitman, M. A., and Lovell, M. A. (2017). Single-base resolution mapping of 5-hydroxymethylcytosine modifications in Hippocampus of alzheimer's disease subjects. J. Mol. Neurosci. 63 (2), 185–197. doi:10.1007/s12031-017-0969-y
Fetahu, I. S., Ma, D., Rabidou, K., Argueta, C., Smith, M., Liu, H., et al. (2019). Epigenetic signatures of methylated DNA cytosine in Alzheimer's disease. Sci. Adv. 5 (8), eaaw2880. doi:10.1126/sciadv.aaw2880
Fransquet, P. D., and Ryan, J. (2019). The current status of blood epigenetic biomarkers for dementia. Crit. Rev. Clin. Lab. Sci. 56 (7), 435–457. doi:10.1080/10408363.2019.1639129
Fransquet, P. D., Lacaze, P., Saffery, R., McNeil, J., Woods, R., and Ryan, J. (2018). Blood DNA methylation as a potential biomarker of dementia: A systematic review. Alzheimer's dementia J. Alzheimer's Assoc. 14 (1), 81–103. doi:10.1016/j.jalz.2017.10.002
Fransquet, P. D., Lacaze, P., Saffery, R., Phung, J., Parker, E., Shah, R., et al. (2020). Blood DNA methylation signatures to detect dementia prior to overt clinical symptoms. Alzheimers Dement. (Amst) 12 (1), e12056. doi:10.1002/dad2.12056
Fuso, A., Seminara, L., Cavallaro, R. A., D'Anselmi, F., and Scarpa, S. (2005). S-adenosylmethionine/homocysteine cycle alterations modify DNA methylation status with consequent deregulation of PS1 and BACE and beta-amyloid production. Mol. Cell. Neurosci. 28 (1), 195–204. doi:10.1016/j.mcn.2004.09.007
Fuso, A., Nicolia, V., Cavallaro, R. A., Ricceri, L., D'Anselmi, F., Coluccia, P., et al. (2008). B-vitamin deprivation induces hyperhomocysteinemia and brain S-adenosylhomocysteine, depletes brain S-adenosylmethionine, and enhances PS1 and BACE expression and amyloid-beta deposition in mice. Mol. Cell. Neurosci. 37 (4), 731–746. doi:10.1016/j.mcn.2007.12.018
Garcia-Blanco, A., Baquero, M., Vento, M., Gil, E., Bataller, L., and Chafer-Pericas, C. (2017). Potential oxidative stress biomarkers of mild cognitive impairment due to Alzheimer disease. J. Neurol. Sci. 373, 295–302. doi:10.1016/j.jns.2017.01.020
Gasparoni, G., Bultmann, S., Lutsik, P., Kraus, T. F. J., Sordon, S., Vlcek, J., et al. (2018). DNA methylation analysis on purified neurons and glia dissects age and Alzheimer's disease-specific changes in the human cortex. Epigenetics Chromatin 11 (1), 41. doi:10.1186/s13072-018-0211-3
Gatz, M., Pedersen, N. L., Berg, S., Johansson, B., Johansson, K., Mortimer, J. A., et al. (1997). Heritability for alzheimer's disease: The study of dementia in Swedish twins. journals gerontology. Ser. A, Biol. Sci. Med. Sci. 52 (2), M117–M125. doi:10.1093/gerona/52a.2.m117
Gauthier, S., Reisberg, B., Zaudig, M., Petersen, R. C., Ritchie, K., Broich, K., et al. (2006). Expert Conference on mild cognitive. Mild cognitive impairment. Lancet 367 (9518), 1262–1270. doi:10.1016/S0140-6736(06)68542-5
Glennon, E. B., Whitehouse, I. J., Miners, J. S., Kehoe, P. G., Love, S., Kellett, K. A., et al. (2013). BIN1 is decreased in sporadic but not familial Alzheimer's disease or in aging. PloS one 8 (10), e78806. doi:10.1371/journal.pone.0078806
Goate, A., Chartier-Harlin, M. C., Mullan, M., Brown, J., Crawford, F., Fidani, L., et al. (1991). Segregation of a missense mutation in the amyloid precursor protein gene with familial Alzheimer's disease. Nature 349 (6311), 704–706. doi:10.1038/349704a0
Hannon, E., Knox, O., Sugden, K., Burrage, J., Wong, C. C. Y., Belsky, D. W., et al. (2018). Characterizing genetic and environmental influences on variable DNA methylation using monozygotic and dizygotic twins. PLoS Genet. 14 (8), e1007544. doi:10.1371/journal.pgen.1007544
Harold, D., Abraham, R., Hollingworth, P., Sims, R., Gerrish, A., Hamshere, M. L., et al. (2009). Genome-wide association study identifies variants at CLU and PICALM associated with Alzheimer's disease. Nat. Genet. 41 (10), 1088–1093. doi:10.1038/ng.440
Hernandez, D. G., Nalls, M. A., Gibbs, J. R., Arepalli, S., van der Brug, M., Chong, S., et al. (2011). Distinct DNA methylation changes highly correlated with chronological age in the human brain. Hum. Mol. Genet. 20 (6), 1164–1172. doi:10.1093/hmg/ddq561
Hernandez, H. G., Sandoval-Hernandez, A. G., Garrido-Gil, P., Labandeira-Garcia, J. L., Zelaya, M. V., Bayon, G. F., et al. (2018). Alzheimer's disease DNA methylome of pyramidal layers in frontal cortex: Laser-assisted microdissection study. Epigenomics 10 (11), 1365–1382. doi:10.2217/epi-2017-0160
Holliday, R., and Pugh, J. E. (1975). DNA modification mechanisms and gene activity during development. Science 187 (4173), 226–232.
Hollingworth, P., Harold, D., Sims, R., Gerrish, A., Lambert, J. C., Carrasquillo, M. M., et al. (2011). Common variants at ABCA7, MS4A6A/MS4A4E, EPHA1, CD33 and CD2AP are associated with Alzheimer's disease. Nat. Genet. 43 (5), 429–435. doi:10.1038/ng.803
Hou, Y., Chen, H., He, Q., Jiang, W., Luo, T., Duan, J., et al. (2013). Changes in methylation patterns of multiple genes from peripheral blood leucocytes of Alzheimer's disease patients. Acta Neuropsychiatr. 25 (2), 66–76. doi:10.1111/j.1601-5215.2012.00662.x
Huang, L. K., Chao, S. P., and Hu, C. J. (2020). Clinical trials of new drugs for Alzheimer disease. J. Biomed. Sci. 27 (1), 18. doi:10.1186/s12929-019-0609-7
Iwata, A., Nagata, K., Hatsuta, H., Takuma, H., Bundo, M., Iwamoto, K., et al. (2014). Altered CpG methylation in sporadic Alzheimer's disease is associated with APP and MAPT dysregulation. Hum. Mol. Genet. 23 (3), 648–656. doi:10.1093/hmg/ddt451
Jain, A., and Tuteja, G. (2019). TissueEnrich: Tissue-specific gene enrichment analysis. Bioinformatics 35 (11), 1966–1967. doi:10.1093/bioinformatics/bty890
Jia, L., Du, Y., Chu, L., Zhang, Z., Li, F., Lyu, D., et al. (2020a). Prevalence, risk factors, and management of dementia and mild cognitive impairment in adults aged 60 years or older in China: A cross-sectional study. Lancet Public Health 5 (12), e661–e671. doi:10.1016/S2468-2667(20)30185-7
Jia, L., Quan, M., Fu, Y., Zhao, T., Li, Y., Wei, C., et al. (2020b). For the Project of Dementia Situation in. Dementia in China: epidemiology, clinical management, and research advances. Lancet. Neurology 19 (1), 81–92. doi:10.1016/S1474-4422(19)30290-X
Jicha, G. A., Parisi, J. E., Dickson, D. W., Johnson, K., Cha, R., Ivnik, R. J., et al. (2006). Neuropathologic outcome of mild cognitive impairment following progression to clinical dementia. Archives neurology 63 (5), 674–681. doi:10.1001/archneur.63.5.674
Jun, G., Naj, A. C., Beecham, G. W., Wang, L. S., Buros, J., Gallins, P. J., et al. (2010). Meta-analysis confirms CR1, CLU, and PICALM as alzheimer disease risk loci and reveals interactions with APOE genotypes. Archives neurology 67 (12), 1473–1484. doi:10.1001/archneurol.2010.201
Karemaker, I. D., and Vermeulen, M. (2018). Single-cell DNA methylation profiling: Technologies and biological applications. Trends Biotechnol. 36 (9), 952–965. doi:10.1016/j.tibtech.2018.04.002
Kivipelto, M., Mangialasche, F., and Ngandu, T. (2018). Lifestyle interventions to prevent cognitive impairment, dementia and Alzheimer disease. Nat. Rev. Neurol. 14 (11), 653–666. doi:10.1038/s41582-018-0070-3
Kobayashi, N., Shinagawa, S., Nagata, T., Shimada, K., Shibata, N., Ohnuma, T., et al. (2016). Development of biomarkers based on DNA methylation in the NCAPH2/LMF2 promoter region for diagnosis of alzheimer's disease and amnesic mild cognitive impairment. PloS one 11 (1), e0146449. doi:10.1371/journal.pone.0146449
Lambert, J. C., Heath, S., Even, G., Campion, D., Sleegers, K., Hiltunen, M., et al. (2009). Genome-wide association study identifies variants at CLU and CR1 associated with Alzheimer's disease. Nat. Genet. 41 (10), 1094–1099. doi:10.1038/ng.439
Lambert, J. C., Ibrahim-Verbaas, C. A., Harold, D., Naj, A. C., Sims, R., Bellenguez, C., et al. (2013). Meta-analysis of 74,046 individuals identifies 11 new susceptibility loci for Alzheimer's disease. Nat. Genet. 45 (12), 1452–1458. doi:10.1038/ng.2802
Lane, C. A., Hardy, J., and Schott, J. M. (2018). Alzheimer's disease. Eur. J. Neurol. 25 (1), 59–70. doi:10.1111/ene.13439
Lardenoije, R., Roubroeks, J. A. Y., Pishva, E., Leber, M., Wagner, H., Iatrou, A., et al. (2019). Alzheimer's disease-associated (hydroxy)methylomic changes in the brain and blood. Clin. Epigenetics 11 (1), 164. doi:10.1186/s13148-019-0755-5
Lee, J. H., Cheng, R., Honig, L. S., Vonsattel, J. P., Clark, L., and Mayeux, R. (2008). Association between genetic variants in SORL1 and autopsy-confirmed Alzheimer disease. Neurology 70 (11), 887–889. doi:10.1212/01.wnl.0000280581.39755.89
Lee, J. H., Yang, D. S., Goulbourne, C. N., Im, E., Stavrides, P., Pensalfini, A., et al. (2022). Faulty autolysosome acidification in Alzheimer's disease mouse models induces autophagic build-up of Abeta in neurons, yielding senile plaques. Nat. Neurosci. 25 (6), 688–701. doi:10.1038/s41593-022-01084-8
Levy-Lahad, E., Wijsman, E. M., Nemens, E., Anderson, L., Goddard, K. A., Weber, J. L., et al. (1995a). A familial Alzheimer's disease locus on chromosome 1. Science 269 (5226), 970–973. doi:10.1126/science.7638621
Levy-Lahad, E., Wasco, W., Poorkaj, P., Romano, D. M., Oshima, J., Pettingell, W. H., et al. (1995b). Candidate gene for the chromosome 1 familial Alzheimer's disease locus. Science 269 (5226), 973–977. doi:10.1126/science.7638622
Li, P., Marshall, L., Oh, G., Jakubowski, J. L., Groot, D., He, Y., et al. (2019). Epigenetic dysregulation of enhancers in neurons is associated with Alzheimer's disease pathology and cognitive symptoms. Nat. Commun. 10 (1), 2246. doi:10.1038/s41467-019-10101-7
Liu, H. C., Hu, C. J., Tang, Y. C., and Chang, J. G. (2008). A pilot study for circadian gene disturbance in dementia patients. Neurosci. Lett. 435 (3), 229–233. doi:10.1016/j.neulet.2008.02.041
Livingston, G., Sommerlad, A., Orgeta, V., Costafreda, S. G., Huntley, J., Ames, D., et al. (2017). Dementia prevention, intervention, and care. Lancet 390 (10113), 2673–2734. doi:10.1016/S0140-6736(17)31363-6
Lord, J., and Cruchaga, C. (2014). The epigenetic landscape of Alzheimer's disease. Nat. Neurosci. 17 (9), 1138–1140. doi:10.1038/nn.3792
Lourida, I., Hannon, E., Littlejohns, T. J., Langa, K. M., Hypponen, E., Kuzma, E., et al. (2019). Association of lifestyle and genetic risk with incidence of dementia. Jama 322 (5), 430–437. doi:10.1001/jama.2019.9879
Lunnon, K., Ibrahim, Z., Proitsi, P., Lourdusamy, A., Newhouse, S., Sattlecker, M., et al. (2012). Mitochondrial dysfunction and immune activation are detectable in early Alzheimer's disease blood. J. Alzheimer's Dis. JAD 30 (3), 685–710. doi:10.3233/JAD-2012-111592
Lunnon, K., Smith, R., Hannon, E., De Jager, P. L., Srivastava, G., Volta, M., et al. (2014). Methylomic profiling implicates cortical deregulation of ANK1 in Alzheimer's disease. Nat. Neurosci. 17 (9), 1164–1170. doi:10.1038/nn.3782
Ma, S. L., Tang, N. L., and Lam, L. C. (2016). Association of gene expression and methylation of UQCRC1 to the predisposition of Alzheimer's disease in a Chinese population. J. psychiatric Res. 76, 143–147. doi:10.1016/j.jpsychires.2016.02.010
Madrid, A., Hogan, K. J., Papale, L. A., Clark, L. R., Asthana, S., Johnson, S. C., et al. (2018). DNA hypomethylation in blood links B3GALT4 and ZADH2 to alzheimer's disease. J. Alzheimer's Dis. JAD 66 (3), 927–934. doi:10.3233/JAD-180592
Mano, T., Nagata, K., Nonaka, T., Tarutani, A., Imamura, T., Hashimoto, T., et al. (2017). Neuron-specific methylome analysis reveals epigenetic regulation and tau-related dysfunction of BRCA1 in Alzheimer's disease. Proc. Natl. Acad. Sci. U. S. A. 114 (45), E9645–E9654. doi:10.1073/pnas.1707151114
Mastroeni, D., McKee, A., Grover, A., Rogers, J., and Coleman, P. D. (2009). Epigenetic differences in cortical neurons from a pair of monozygotic twins discordant for Alzheimer's disease. PloS one 4 (8), e6617. doi:10.1371/journal.pone.0006617
Mastroeni, D., Grover, A., Delvaux, E., Whiteside, C., Coleman, P. D., and Rogers, J. (2010). Epigenetic changes in alzheimer's disease: Decrements in DNA methylation. Neurobiol. aging 31 (12), 2025–2037. doi:10.1016/j.neurobiolaging.2008.12.005
Mastroeni, D., Grover, A., Delvaux, E., Whiteside, C., Coleman, P. D., and Rogers, J. (2011). Epigenetic mechanisms in Alzheimer's disease. Neurobiol. aging 32 (7), 1161–1180. doi:10.1016/j.neurobiolaging.2010.08.017
McKhann, G., Drachman, D., Folstein, M., Katzman, R., Price, D., and Stadlan, E. M. (1984). Clinical diagnosis of alzheimer's disease: Report of the NINCDS-ADRDA work group under the auspices of department of health and human services task force on alzheimer's disease. Neurology 34 (7), 939–944. doi:10.1212/wnl.34.7.939
McLeay, R. C., and Bailey, T. L. (2010). Motif enrichment analysis: A unified framework and an evaluation on ChIP data. BMC Bioinforma. 11, 165. doi:10.1186/1471-2105-11-165
Meder, B., Haas, J., Sedaghat-Hamedani, F., Kayvanpour, E., Frese, K., Lai, A., et al. (2017). Epigenome-wide association study identifies cardiac gene patterning and a novel class of biomarkers for heart failure. Circulation 136 (16), 1528–1544. doi:10.1161/CIRCULATIONAHA.117.027355
Miller, C. A., and Sweatt, J. D. (2007). Covalent modification of DNA regulates memory formation. Neuron 53 (6), 857–869. doi:10.1016/j.neuron.2007.02.022
Miller, C. A., Gavin, C. F., White, J. A., Parrish, R. R., Honasoge, A., Yancey, C. R., et al. (2010). Cortical DNA methylation maintains remote memory. Nat. Neurosci. 13 (6), 664–666. doi:10.1038/nn.2560
Minter, M. R., Taylor, J. M., and Crack, P. J. (2016). The contribution of neuroinflammation to amyloid toxicity in Alzheimer's disease. J. Neurochem. 136 (3), 457–474. doi:10.1111/jnc.13411
Moore, L. D., Le, T., and Fan, G. (2013). DNA methylation and its basic function. Neuropsychopharmacol. official Publ. Am. Coll. Neuropsychopharmacol. 38 (1), 23–38. doi:10.1038/npp.2012.112
Morgan, A. R., Touchard, S., Leckey, C., O'Hagan, C., Nevado-Holgado, A. J., Consortium, N., et al. (2019). Inflammatory biomarkers in Alzheimer's disease plasma. Alzheimer's dementia J. Alzheimer's Assoc. 15 (6), 776–787. doi:10.1016/j.jalz.2019.03.007
Morris, T. J., Butcher, L. M., Feber, A., Teschendorff, A. E., Chakravarthy, A. R., Wojdacz, T. K., et al. (2014). 450k chip analysis methylation pipeline. Bioinformatics 30 (3), 428–430. doi:10.1093/bioinformatics/btt684
Morris, J. C. (1993). The clinical dementia rating (CDR): Current version and scoring rules. Neurology 43 (11), 2412–2414. doi:10.1212/wnl.43.11.2412-a
Mullan, M., Houlden, H., Windelspecht, M., Fidani, L., Lombardi, C., Diaz, P., et al. (1992). A locus for familial early-onset Alzheimer's disease on the long arm of chromosome 14, proximal to the alpha 1-antichymotrypsin gene. Nat. Genet. 2 (4), 340–342. doi:10.1038/ng1292-340
Murrell, J., Farlow, M., Ghetti, B., and Benson, M. D. (1991). A mutation in the amyloid precursor protein associated with hereditary Alzheimer's disease. Science 254 (5028), 97–99. doi:10.1126/science.1925564
Nagata, K., Mano, T., Murayama, S., Saido, T. C., and Iwata, A. (2018). DNA methylation level of the neprilysin promoter in Alzheimer's disease brains. Neurosci. Lett. 6, 708–713. doi:10.1016/j.neulet.2018.01.003
Naj, A. C., Jun, G., Beecham, G. W., Wang, L. S., Vardarajan, B. N., Buros, J., et al. (2011). Common variants at MS4A4/MS4A6E, CD2AP, CD33 and EPHA1 are associated with late-onset Alzheimer's disease. Nat. Genet. 43 (5), 436–441. doi:10.1038/ng.801
Narayanan, K. L., Chopra, V., Rosas, H. D., Malarick, K., and Hersch, S. (2016). Rho kinase pathway alterations in the brain and leukocytes in huntington's disease. Mol. Neurobiol. 53 (4), 2132–2140. doi:10.1007/s12035-015-9147-9
Oh, E. S., and Rabins, P. V. (2019). Dementia. Ann. Intern Med. 171 (5), ITC33–ITC48. doi:10.7326/AITC201909030
Pan, Y., Liu, G., Zhou, F., Su, B., and Li, Y. (2018). DNA methylation profiles in cancer diagnosis and therapeutics. Clin. Exp. Med. 18 (1), 1–14. doi:10.1007/s10238-017-0467-0
Panza, F., Lozupone, M., Logroscino, G., and Imbimbo, B. P. (2019). A critical appraisal of amyloid-beta-targeting therapies for Alzheimer disease. Nat. Rev. Neurol. 15 (2), 73–88. doi:10.1038/s41582-018-0116-6
Pedersen, N. L., Gatz, M., Berg, S., and Johansson, B. (2004). How heritable is alzheimer's disease late in life? Findings from Swedish twins. Ann. neurology 55 (2), 180–185. doi:10.1002/ana.10999
Perez, R. F., Alba-Linares, J. J., Tejedor, J. R., Fernandez, A. F., Calero, M., Roman-Dominguez, A., et al. (2022). Blood DNA methylation patterns in older adults with evolving dementia. journals gerontology. Ser. A, Biol. Sci. Med. Sci. 77, 1743. doi:10.1093/gerona/glac068
Pericak-Vance, M. A., Bebout, J. L., Gaskell, P. C., Yamaoka, L. H., Hung, W. Y., Alberts, M. J., et al. (1991). Linkage studies in familial alzheimer disease: Evidence for chromosome 19 linkage. Am. J. Hum. Genet. 48 (6), 1034–1050.
Petersen, R. C., Smith, G. E., Waring, S. C., Ivnik, R. J., Tangalos, E. G., and Kokmen, E. (1999). Mild cognitive impairment: Clinical characterization and outcome. Archives neurology 56 (3), 303–308. doi:10.1001/archneur.56.3.303
Petersen, R. C. (2004). Mild cognitive impairment as a diagnostic entity. J. Intern Med. 256 (3), 183–194. doi:10.1111/j.1365-2796.2004.01388.x
Prasad, R., and Jho, E. H. (2019). A concise review of human brain methylome during aging and neurodegenerative diseases. BMB Rep. 52 (10), 577–588.
Rahmani, E., Yedidim, R., Shenhav, L., Schweiger, R., Weissbrod, O., Zaitlen, N., et al. (2017). Glint: A user-friendly toolset for the analysis of high-throughput DNA-methylation array data. Bioinformatics 33 (12), 1870–1872. doi:10.1093/bioinformatics/btx059
Raiha, I., Kaprio, J., Koskenvuo, M., Rajala, T., and Sourander, L. (1996). Alzheimer's disease in Finnish twins. Lancet 347 (9001), 573–578. doi:10.1016/s0140-6736(96)91272-6
Reitz, C., Cheng, R., Rogaeva, E., Lee, J. H., Tokuhiro, S., Zou, F., et al. (2011). Meta-analysis of the association between variants in SORL1 and Alzheimer disease. Archives neurology 68 (1), 99–106. doi:10.1001/archneurol.2010.346
Ren, R., Qi, J., Lin, S., Liu, X., Yin, P., Wang, Z., et al. (2022). The China alzheimer report 2022. Gen. Psychiatr. 35 (1), e100751. doi:10.1136/gpsych-2022-100751
Robin, X., Turck, N., Hainard, A., Tiberti, N., Lisacek, F., Sanchez, J. C., et al. (2011). pROC: an open-source package for R and S+ to analyze and compare ROC curves. BMC Bioinforma. 12, 77. doi:10.1186/1471-2105-12-77
Rogaev, E. I., Sherrington, R., Rogaeva, E. A., Levesque, G., Ikeda, M., Liang, Y., et al. (1995). Familial Alzheimer's disease in kindreds with missense mutations in a gene on chromosome 1 related to the Alzheimer's disease type 3 gene. Nature 376 (6543), 775–778. doi:10.1038/376775a0
Rogaeva, E., Meng, Y., Lee, J. H., Gu, Y., Kawarai, T., Zou, F., et al. (2007). The neuronal sortilin-related receptor SORL1 is genetically associated with Alzheimer disease. Nat. Genet. 39 (2), 168–177. doi:10.1038/ng1943
Rohart, F., Gautier, B., Singh, A., and mixOmics, K. A. Le Cao. (2017). An R package for 'omics feature selection and multiple data integration. PLoS Comput. Biol. 13 (11), e1005752. doi:10.1371/journal.pcbi.1005752
Roubroeks, J. A. Y., Smith, A. R., Smith, R. G., Pishva, E., Ibrahim, Z., Sattlecker, M., et al. (2020). An epigenome-wide association study of Alzheimer's disease blood highlights robust DNA hypermethylation in the HOXB6 gene. Neurobiol. aging 95, 26–45. doi:10.1016/j.neurobiolaging.2020.06.023
Salcedo-Tacuma, D., Melgarejo, J. D., Mahecha, M. F., Ortega-Rojas, J., Arboleda-Bustos, C. E., Pardo-Turriago, R., et al. (2019). Differential methylation levels in CpGs of the BIN1 gene in individuals with alzheimer disease. Alzheimer Dis. Assoc. Disord. 33 (4), 321–326. doi:10.1097/WAD.0000000000000329
Sanchez-Mut, J. V., Heyn, H., Vidal, E., Moran, S., Sayols, S., Delgado-Morales, R., et al. (2016). Human DNA methylomes of neurodegenerative diseases show common epigenomic patterns. Transl. psychiatry 6, e718. doi:10.1038/tp.2015.214
Schellenberg, G. D., Bird, T. D., Wijsman, E. M., Orr, H. T., Anderson, L., Nemens, E., et al. (1992). Genetic linkage evidence for a familial Alzheimer's disease locus on chromosome 14. Science 258 (5082), 668–671. doi:10.1126/science.1411576
Scherzer, C. R., Offe, K., Gearing, M., Rees, H. D., Fang, G., Heilman, C. J., et al. (2004). Loss of apolipoprotein E receptor LR11 in Alzheimer disease. Archives neurology 61 (8), 1200–1205. doi:10.1001/archneur.61.8.1200
Schmidt, S. I., Blaabjerg, M., Freude, K., and Meyer, M. (2022). RhoA signaling in neurodegenerative diseases. Cells 11 (9). doi:10.3390/cells11091520
Semick, S. A., Bharadwaj, R. A., Collado-Torres, L., Tao, R., Shin, J. H., Deep-Soboslay, A., et al. (2019). Integrated DNA methylation and gene expression profiling across multiple brain regions implicate novel genes in Alzheimer's disease. Acta neuropathol. 137 (4), 557–569. doi:10.1007/s00401-019-01966-5
Seshadri, S., Fitzpatrick, A. L., Ikram, M. A., DeStefano, A. L., Gudnason, V., Boada, M., et al. (2010). Genome-wide analysis of genetic loci associated with Alzheimer disease. Jama 303 (18), 1832–1840. doi:10.1001/jama.2010.574
Sherrington, R., Rogaev, E. I., Liang, Y., Rogaeva, E. A., Levesque, G., Ikeda, M., et al. (1995). Cloning of a gene bearing missense mutations in early-onset familial Alzheimer's disease. Nature 375 (6534), 754–760. doi:10.1038/375754a0
Shinagawa, S., Kobayashi, N., Nagata, T., Kusaka, A., Yamada, H., Kondo, K., et al. (2016). DNA methylation in the NCAPH2/LMF2 promoter region is associated with hippocampal atrophy in Alzheimer's disease and amnesic mild cognitive impairment patients. Neurosci. Lett. 629, 33–37. doi:10.1016/j.neulet.2016.06.055
Smith, R. G., Hannon, E., De Jager, P. L., Chibnik, L., Lott, S. J., Condliffe, D., et al. (2018). Elevated DNA methylation across a 48-kb region spanning the HOXA gene cluster is associated with Alzheimer's disease neuropathology. Alzheimer's dementia J. Alzheimer's Assoc. 14 (12), 1580–1588. doi:10.1016/j.jalz.2018.01.017
Smith, A. R., Smith, R. G., Pishva, E., Hannon, E., Roubroeks, J. A. Y., Burrage, J., et al. (2019). Parallel profiling of DNA methylation and hydroxymethylation highlights neuropathology-associated epigenetic variation in Alzheimer's disease. Clin. Epigenetics 11 (1), 52. doi:10.1186/s13148-019-0636-y
Smith, R. G., Pishva, E., Shireby, G., Smith, A. R., Roubroeks, J. A. Y., Hannon, E., et al. (2021). A meta-analysis of epigenome-wide association studies in Alzheimer's disease highlights novel differentially methylated loci across cortex. Nat. Commun. 12 (1), 3517. doi:10.1038/s41467-021-23243-4
Sperling, R. A., Aisen, P. S., Beckett, L. A., Bennett, D. A., Craft, S., Fagan, A. M., et al. (2011). Toward defining the preclinical stages of alzheimer's disease: Recommendations from the national Institute on aging-alzheimer's association workgroups on diagnostic guidelines for alzheimer's disease. Alzheimer's dementia J. Alzheimer's Assoc. 7 (3), 280–292. doi:10.1016/j.jalz.2011.03.003
St George-Hyslop, P., Haines, J., Rogaev, E., Mortilla, M., Vaula, G., Pericak-Vance, M., et al. (1992). Genetic evidence for a novel familial Alzheimer's disease locus on chromosome 14. Nat. Genet. 2 (4), 330–334. doi:10.1038/ng1292-330
Strittmatter, W. J., Saunders, A. M., Schmechel, D., Pericak-Vance, M., Enghild, J., Salvesen, G. S., et al. (1993). high-avidity binding to beta-amyloid and increased frequency of type 4 allele in late-onset familial Alzheimer disease. Proc. Natl. Acad. Sci. U. S. A. 90 (5), 1977–1981. doi:10.1073/pnas.90.5.1977
Szklarczyk, D., Gable, A. L., Nastou, K. C., Lyon, D., Kirsch, R., Pyysalo, S., et al. (2021). The STRING database in 2021: Customizable protein-protein networks, and functional characterization of user-uploaded gene/measurement sets. Nucleic acids Res. 49 (D1), D605–D612. doi:10.1093/nar/gkaa1074
Teschendorff, A. E., Menon, U., Gentry-Maharaj, A., Ramus, S. J., Gayther, S. A., Apostolidou, S., et al. (2009). An epigenetic signature in peripheral blood predicts active ovarian cancer. PloS one 4 (12), e8274. doi:10.1371/journal.pone.0008274
Tian, Y., Morris, T. J., Webster, A. P., Yang, Z., Beck, S., Feber, A., et al. (2017). ChAMP: Updated methylation analysis pipeline for Illumina BeadChips. Bioinformatics 33 (24), 3982–3984. doi:10.1093/bioinformatics/btx513
Tohgi, H., Utsugisawa, K., Nagane, Y., Yoshimura, M., Genda, Y., and Ukitsu, M. (1999). Reduction with age in methylcytosine in the promoter region -224 approximately -101 of the amyloid precursor protein gene in autopsy human cortex. Brain Res. Mol. Brain Res. 70 (2), 288–292. doi:10.1016/s0169-328x(99)00163-1
Tong, Z., Han, C., Qiang, M., Wang, W., Lv, J., Zhang, S., et al. (2015). Age-related formaldehyde interferes with DNA methyltransferase function, causing memory loss in Alzheimer's disease. Neurobiol. aging 36 (1), 100–110. doi:10.1016/j.neurobiolaging.2014.07.018
Van Broeckhoven, C., Backhovens, H., Cruts, M., De Winter, G., Bruyland, M., Cras, P., et al. (1992). Mapping of a gene predisposing to early-onset Alzheimer's disease to chromosome 14q24.3. Nat. Genet. 2 (4), 335–339. doi:10.1038/ng1292-335
Van Cauwenberghe, C., Van Broeckhoven, C., and Sleegers, K. (2016). The genetic landscape of alzheimer disease: Clinical implications and perspectives. Genet. Med. official J. Am. Coll. Med. Genet. 18 (5), 421–430. doi:10.1038/gim.2015.117
van der Kant, R., Goldstein, L. S. B., and Ossenkoppele, R. (2020). Amyloid-beta-independent regulators of tau pathology in Alzheimer disease. Nat. Rev. Neurosci. 21 (1), 21–35. doi:10.1038/s41583-019-0240-3
Vasanthakumar, A., Davis, J. W., Idler, K., Waring, J. F., Asque, E., Riley-Gillis, B., et al. (2020). Harnessing peripheral DNA methylation differences in the Alzheimer's Disease Neuroimaging Initiative (ADNI) to reveal novel biomarkers of disease. Clin. Epigenetics 12 (1), 84. doi:10.1186/s13148-020-00864-y
Wang, S. C., Oelze, B., and Schumacher, A. (2008). Age-specific epigenetic drift in late-onset Alzheimer's disease. PloS one 3 (7), e2698. doi:10.1371/journal.pone.0002698
Wang, J., Yu, J. T., Tan, M. S., Jiang, T., and Tan, L. (2013). Epigenetic mechanisms in alzheimer's disease: Implications for pathogenesis and therapy. Ageing Res. Rev. 12 (4), 1024–1041. doi:10.1016/j.arr.2013.05.003
Wang, F., Cao, Y., Ma, L., Pei, H., Rausch, W. D., and Li, H. (2018). Dysfunction of cerebrovascular endothelial cells: Prelude to vascular dementia. Front. aging Neurosci. 10, 376. doi:10.3389/fnagi.2018.00376
Wang, Q., Song, L. J., Ding, Z. B., Chai, Z., Yu, J. Z., Xiao, B. G., et al. (2022). Advantages of Rho-associated kinases and their inhibitor fasudil for the treatment of neurodegenerative diseases. Neural Regen. Res. 17 (12), 2623–2631. doi:10.4103/1673-5374.335827
Watson, C. T., Roussos, P., Garg, P., Ho, D. J., Azam, N., Katsel, P. L., et al. (2016). Genome-wide DNA methylation profiling in the superior temporal gyrus reveals epigenetic signatures associated with Alzheimer's disease. Genome Med. 8 (1), 5. doi:10.1186/s13073-015-0258-8
Wechsler-Reya, R., Sakamuro, D., Zhang, J., Duhadaway, J., and Prendergast, G. C. (1997). Structural analysis of the human BIN1 gene. Evidence for tissue-specific transcriptional regulation and alternate RNA splicing. J. Biol. Chem. 272 (50), 31453–31458. doi:10.1074/jbc.272.50.31453
West, R. L., Lee, J. M., and Maroun, L. E. (1995). Hypomethylation of the amyloid precursor protein gene in the brain of an Alzheimer's disease patient. J. Mol. Neurosci. 6 (2), 141–146. doi:10.1007/BF02736773
Yu, G., Wang, L. G., Han, Y., and He, Q. Y. (2012). clusterProfiler: an R package for comparing biological themes among gene clusters. Omics a J. Integr. Biol. 16 (5), 284–287. doi:10.1089/omi.2011.0118
Yu, N. Y., Hallstrom, B. M., Fagerberg, L., Ponten, F., Kawaji, H., Carninci, P., et al. (2015a). Complementing tissue characterization by integrating transcriptome profiling from the Human Protein Atlas and from the FANTOM5 consortium. Nucleic acids Res. 43 (14), 6787–6798. doi:10.1093/nar/gkv608
Yu, L., Chibnik, L. B., Srivastava, G. P., Pochet, N., Yang, J., Xu, J., et al. (2015b). Association of Brain DNA methylation in SORL1, ABCA7, HLA-DRB5, SLC24A4, and BIN1 with pathological diagnosis of Alzheimer disease. JAMA neurol. 72 (1), 15–24. doi:10.1001/jamaneurol.2014.3049
Zetterberg, H., and Burnham, S. C. (2019). Blood-based molecular biomarkers for Alzheimer's disease. Mol. Brain 12 (1), 26. doi:10.1186/s13041-019-0448-1
Zhang, L., Silva, T. C., Young, J. I., Gomez, L., Schmidt, M. A., Hamilton-Nelson, K. L., et al. (2020). Epigenome-wide meta-analysis of DNA methylation differences in prefrontal cortex implicates the immune processes in Alzheimer's disease. Nat. Commun. 11 (1), 6114. doi:10.1038/s41467-020-19791-w
Zhao, J., Zhu, Y., Yang, J., Li, L., Wu, H., De Jager, P. L., et al. (2017). A genome-wide profiling of brain DNA hydroxymethylation in Alzheimer's disease. Alzheimer's dementia J. Alzheimer's Assoc. 13 (6), 674–688. doi:10.1016/j.jalz.2016.10.004
Keywords: mild cognitive impairment, Alzheimer’s disease, blood DNA methylome profile, differentially methylated CpG sites, potential biomarker
Citation: Wu S, Yang F, Chao S, Wang B, Wang W, Li H, Yu L, He L, Li X, Sun L and Qin S (2023) Altered DNA methylome profiles of blood leukocytes in Chinese patients with mild cognitive impairment and Alzheimer’s disease. Front. Genet. 14:1175864. doi: 10.3389/fgene.2023.1175864
Received: 28 February 2023; Accepted: 01 June 2023;
Published: 14 June 2023.
Edited by:
Alberto Montesanto, University of Calabria, ItalyReviewed by:
Haoxiang Cheng, Icahn School of Medicine at Mount Sinai, United StatesCopyright © 2023 Wu, Yang, Chao, Wang, Wang, Li, Yu, He, Li, Sun and Qin. This is an open-access article distributed under the terms of the Creative Commons Attribution License (CC BY). The use, distribution or reproduction in other forums is permitted, provided the original author(s) and the copyright owner(s) are credited and that the original publication in this journal is cited, in accordance with accepted academic practice. No use, distribution or reproduction is permitted which does not comply with these terms.
*Correspondence: Shengying Qin, Y2hpbnNpckBzanR1LmVkdS5jbg==; Liya Sun, c3VubGl5YV9zanR1QDE2My5jb20=; Xingwang Li, eHdsaUBzanR1LmVkdS5jbg==
†These authors have contributed equally to this work
Disclaimer: All claims expressed in this article are solely those of the authors and do not necessarily represent those of their affiliated organizations, or those of the publisher, the editors and the reviewers. Any product that may be evaluated in this article or claim that may be made by its manufacturer is not guaranteed or endorsed by the publisher.
Research integrity at Frontiers
Learn more about the work of our research integrity team to safeguard the quality of each article we publish.