- 1The People’s Hospital of Danyang, Affiliated Danyang Hospital of Nantong University, Zhenjiang, China
- 2Department of Epidemiology, Center for Global Health, School of Public Health, Nanjing Medical University, Nanjing, China
- 3Department of Biostatistics, Center for Global Health, School of Public Health, Nanjing Medical University, Nanjing, China
Objective: We explore the candidate susceptibility genes for influenza A virus (IAV), measles, rubella, and mumps and their underlying biological mechanisms.
Methods: We downloaded the genome-wide association study summary data of four virus-specific immunoglobulin G (IgG) level data sets (anti-IAV IgG, anti-measles IgG, anti-rubella IgG, and anti-mumps virus IgG levels) and integrated them with reference models of three potential tissues from the Genotype-Tissue Expression (GTEx) project, namely, whole blood, lung, and transformed fibroblast cells, to identify genes whose expression is predicted to be associated with IAV, measles, mumps, and rubella.
Results: We identified 19 significant genes (ULK4, AC010132.11, SURF1, NIPAL2, TRAP1, TAF1C, AC000078.5, RP4-639F20.1, RMDN2, ATP1B3, SRSF12, RP11-477D19.2, TFB1M, XXyac-YX65C7_A.2, TAF1C, PCGF2, and BNIP1) associated with IAV at a Bonferroni-corrected threshold of p < 0.05; 14 significant genes (SOAT1, COLGALT2, AC021860.1, HCG11, METTL21B, MRPL10, GSTM4, PAQR6, RP11-617D20.1, SNX8, METTL21B, ANKRD27, CBWD2, and TSFM) associated with measles at a Bonferroni-corrected threshold of p < 0.05; 15 significant genes (MTOR, LAMC1, TRIM38, U91328.21, POLR2J, SCRN2, Smpd4, UBN1, CNTROB, SCRN2, HOXB-AS1, SLC14A1, AC007566.10, AC093668.2, and CPD) associated with mumps at a Bonferroni-corrected threshold of p < 0.05; and 13 significant genes (JAGN1, RRP12, RP11-452K12.7, CASP7, AP3S2, IL17RC, FAM86HP, AMACR, RRP12, PPP2R1B, C11orf1, DLAT, and TMEM117) associated with rubella at a Bonferroni-corrected threshold of p < 0.05.
Conclusions: We have identified several candidate genes for IAV, measles, mumps, and rubella in multiple tissues. Our research may further our understanding of the pathogenesis of infectious respiratory diseases.
Introduction
Although respiratory infections are largely preventable causes of illness and death, they remain the leading cause of death from infectious diseases worldwide, ranking fifth among all total causes of death (GBD 2015 LRI Collaborators, 2017). Influenza A virus (IAV), measles virus, rubella virus, and mumps virus infect a wide range of hosts, are highly transmissible and are prone to a mutation that can cause pandemics in a short period of time, placing an enormous burden and pressure on public health systems (Marshall and Plotkin, 2019; Harrington et al., 2021; Iacobucci, 2022; Raghunathan and Orenstein, 2022). The incidence of IAV, measles, mumps, and rubella has decreased drastically following the implementation of vaccination programs. However, the segmented genome of IAV allows for easy recombination and antigenic shift, resulting in novel antigens (Bouvier and Palese, 2008). Thus, the ability of IAV to rapidly evolve can result in highly pathogenic viral strains. Measles and rubella remain endemic in many countries, leading to the importation of cases and occasional local transmission within China, and the resurgence of mumps has recently been reported, with outbreaks still occurring and challenges remaining while controlling these diseases (Kauffmann et al., 2021). There is considerable variation in the severity of respiratory infections caused by viral infections. There are major determinants of this variability, such as intrinsic virus pathogenicity, acquired host factors (e.g., immunity and coexisting conditions), and innate host susceptibility. While viral genetic determinants of respiratory disease severity and host immunity have been well studied, host genetic determinants have been much less explored (Kennedy et al., 2014; Voigt et al., 2018; Sabikunnahar et al., 2022).
Genome-wide association studies (GWAS) are effective methods for understanding the genetic basis of many complex traits in common human diseases (Hayes, 2013). In particular, these have proven to be well-suited for identifying common single-nucleotide polymorphism (SNP) variants with moderate to large effects on phenotypes (Gorlova et al., 2022). However, the specific biological mechanisms and functional consequences of many of the genetic variants identified by the GWAS remain unclear, especially their role in disease severity; that is, GWAS methods may miss small effect–trait associations. Gene expression is an intermediate phenotype between genetic variation and an underlying disease predisposition trait (Albert and Kruglyak, 2015). Many genetic variants affect complex traits by regulating gene expressions. Unfortunately, large-scale expression–trait associations are hampered by sample availability and cost, as well as intrinsic factors and small effects. Therefore, to address these issues, transcriptome-wide association studies (TWASs) were developed, which integrate gene expression into large-scale GWAS (Derks and Gamazon, 2020). Through extensive simulation of existing GWAS data, TWASs have identified candidate genes associated with mental disorders (Thériault et al., 2018), calcified aortic stenosis (Liu et al., 2020), pancreatic cancer (Lin et al., 2017), and inflammatory biological age (Zhang et al., 2022). However, for many complex traits, biologically relevant tissues are unknown. Most existing studies identify gene–trait associations on the basis of a single tissue, whereby the significant gene effects that are identified get inflated. In addition, it has been shown that eQTLs with larger effects tend to regulate gene expressions in multiple tissues. The TWAS analysis of multiple tissues improves the accuracy of the results.
There have been some studies exploring the association of respiratory infectious diseases with some human tissues. Pulmonary inflammation and airway epithelial injury are hallmarks of human pulmonary infectious diseases. The association of HLA alleles with respiratory infectious diseases in lung cells has been confirmed by Zhang et al. (2022). Moreover, Xiao et al. (2023) found that interleukin-11 (IL-11) as a fibrotic factor may be associated with respiratory diseases leading to respiratory failure or even death. These potential connections deserve our attention.
In this study, we used TWASs to explore genes associated with IAV, measles, rubella, and mumps. Given that respiratory infectious diseases are associated with multiple tissues, we used eQTL reference panels for three tissues, namely, whole blood, lung, and transformed fibroblast cells, and pooled the GWAS data for IAV, measles, rubella, and mumps to identify tissue-specific susceptibility genes. This study lays the foundation for further understanding the pathogenesis of these four viruses.
Methods
GWAS data collection and processing
We collected four virus-specific immunoglobulin G (IgG) level data sets (anti-IAV IgG, anti-measles IgG, anti-rubella IgG, and anti-mumps virus IgG levels) from the NHGRI-EBI GWAS directory (https://www.ebi.ac.uk/gwas/downloads/summary-statistics). These data sets were originally obtained from 1,000 healthy individuals (MI), and their serum prevalence rates were 77.7%, 88.5%, 93.5%, and 91.2%, respectively, for these four viruses (Scepanovic et al., 2018). Considering that the main unit of anti-IAV IgG quantitative unit is S/CO (signal/cut-off ratio), the main unit of anti-measles and mumps virus IgG level is IA (index antibody), and the main unit of anti-rubella virus IgG is UI/mL. We also use SD as the change unit. Then, we screened the above data for single-nucleotide polymorphisms (SNPs) for further analysis. We retained the following SNPs: 1) autosomes (1–22), 2) minimum allele frequencies (MAF) greater than 0.001, and 3) more than 70% of observers having these specific SNPs (Yang and Zhou, 2020; Yang and Zhou, 2022). We also plotted Manhattan plots and q-q plots for the processed GWAS data sets for four traits to show the frequency and distribution of their genes (Figure 1; Supplementary Figure S1).
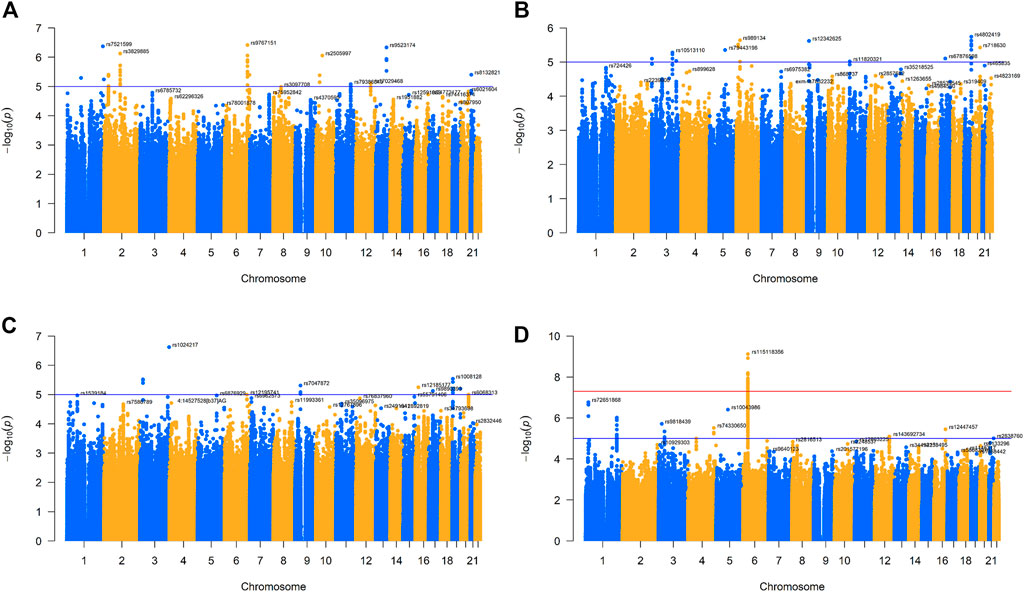
FIGURE 1. Manhattan plot for four GWAS of respiratory infectious diseases. (A). Manhattan plot of IAV. (B). Manhattan plot of measles. (C). Manhattan plot of mumps. (D). Manhattan plot of rubella.
Transcriptome-wide association analysis
We used FUSION to perform relevant TWAS tissue analysis using GWAS summary data formatted after the processing of whole blood, lung, and transformed fibroblast cells (Gusev et al., 2016). Specifically, FUSION uses the pre-calculated gene expression weight to perform summary statistics with respiratory infectious diseases GWAS and calculates the association between each gene and respiratory infectious diseases. Association statistics are defined as TWAS z-scores and estimated as follows:
The weight of Z and W plays a crucial role in the distribution of ZTWAS. In the formula, Z is the estimated value of the SNPs for the disease under study, wt is the weight, and s is the linkage disequilibrium between all SNPs. It is worth noting that ZTWAS is only well calibrated under the zero model of Z ∼ N (0, Σs,s) (mean 0, unit variance 0). The reference panel that we used came from the 1000 Genomes Project in Europe (Speed et al., 2020). We also downloaded from FUSION (http://gusevlab.org/projects/fusion/) pre-computed gene expression weights based on GTEx v7 feature weights and used these for the research of each tissue. Based on the highest cross-validation R2, FUSION selects the best expression prediction model among the top eQTL, the best linear predictor (BLUP) (Gusev et al., 2016), the Bayesian linear mixed model (BSLMM) (Zhou et al., 2013), the least absolute shrinkage and selection operator (LASSO) (Tibshirani, 1996; Veturi and Ritchie, 2018), and the elastic net (Hui and Hastie, 2005) to calculate the SNP expression weight within a 1 Mb window for a given gene. We also applied the Bonferroni correction to explain multiple hypotheses and set the p-value threshold to 0.01.
Results
Multi-tissue transcriptome-wide significant genes of IAV
We used FUSION to assess the relationship between the predictive gene expression and IAV. After Bonferroni correction, we found that 19 genes in the 3 tissues were significantly associated with IAV (Figure 2A; Table 1). Specifically, in the whole blood, we found ULK4 (p adjusted = 0.012), AC010132.11 (p adjusted = 0.022), and SURF1 (p adjusted = 0.030) to be IAV-related genes, while in the lung tissue, we found NIPAL2 (p adjusted = 0.019), TRAP1 (p adjusted = 0.042), TAF1C (p adjusted = 0.018), and AC000078.5 (p adjusted = 0.020) to be significantly associated with IAV. Other significant genes included RP4-639F20.1 (p adjusted = 0.041), RMDN2 (p adjusted = 0.009), ATP1B3 (p adjusted = 0.050), SRSF12 (p adjusted = 0.033), RP11-477D19.2 (p adjusted = 0.007), TFB1M (p adjusted = 0.015), XXyac-YX65C7_A.2 (p adjusted = 0.021), TAF1C (p adjusted = 0.030), and PCGF2 (p adjusted = 0.027) in the transformed fibroblast cells. However, we found that BNIP1 expressed in the whole blood, lung tissue, and transformed fibroblast cells was significantly associated with IAV.
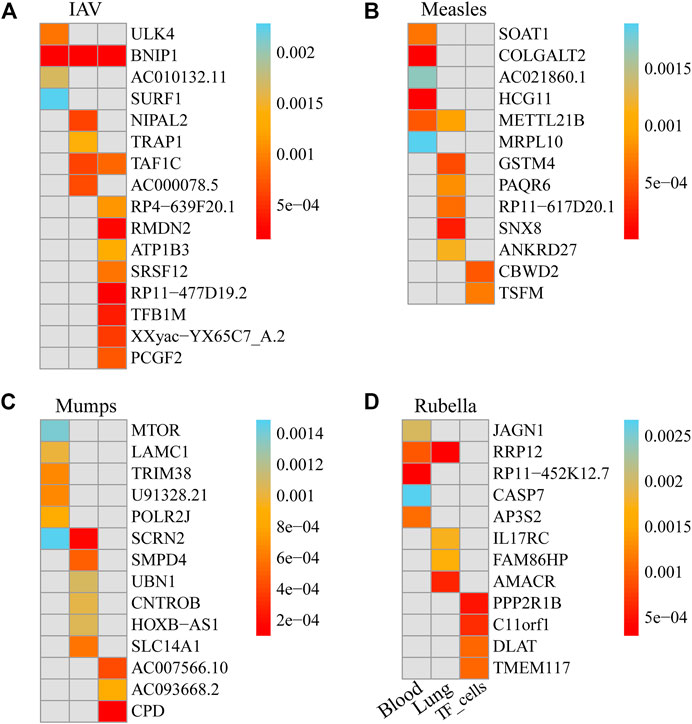
FIGURE 2. Genes significantly associated with the risk of four respiratory infectious diseases in each tissue. (A). Genes significantly associated with the risk of IAV in each tissue. (B). Genes significantly associated with the risk of measles in each tissue. (C). Genes significantly associated with the risk of mumps in each tissue. (D). Genes significantly associated with the risk of rubella in each tissue.
Multi-tissue transcriptome-wide significant genes of measles
We used FUSION to assess the relationship between predictive gene expression and measles. After Bonferroni correction, we found that 14 genes in the three tissues were significantly associated with measles (Figure 2B; Table 2). Specifically, in the whole blood, we found SOAT1 (p adjusted = 0.018), COLGALT2 (p adjusted = 0.000), AC021860.1 (p adjusted = 0.044), HCG11 (p adjusted = 0.000), METTL21B (p adjusted = 0.014), and MRPL10 (p adjusted = 0.049) as measles-related genes, while in the lung tissue, we found GSTM4 (p adjusted = 0.017), PAQR6 (p adjusted = 0.031), RP11-617D20.1 (p adjusted = 0.024), SNX8 (p adjusted = 0.009), METTL21B (p adjusted = 0.035), and ANKRD27 (p adjusted = 0.044) to be significantly associated with measles. Other significant genes included CBWD2 (p adjusted = 0.029) and TSFM (p adjusted = 0.041) in transformed fibroblast cells.
Multi-tissue transcriptome-wide significant genes of mumps
We used FUSION to assess the relationship between predictive gene expression and mumps. After Bonferroni correction, we found that 15 genes in the three tissues were significantly associated with mumps (Figure 2C; Table 3). In mumps, we found six significant genes, namely, MTOR (p adjusted = 0.029), LAMC1 (p adjusted = 0.021), TRIM38 (p adjusted = 0.014), U91328.21 (p adjusted = 0.014), POLR2J (p adjusted = 0.018), and SCRN2 (p adjusted = 0.031) in the whole blood; similarly, in the lung tissue, we found Smpd4 (p adjusted = 0.022), UBN1 (p adjusted = 0.049), CNTROB (p adjusted = 0.046), SCRN2 (p adjusted = 0.008), HOXB-AS1 (p adjusted = 0.048), and SLC14A1 (p adjusted = 0.027) as mumps-related genes, while in the transformed fibroblast cells, we found AC007566.10 (p adjusted = 0.023), AC093668.2 (p adjusted = 0.047), and CPD (p adjusted = 0.005) as significantly associated with mumps.
Multi-tissue transcriptome-wide significant genes of rubella
We used FUSION to assess the relationship between predictive gene expression and rubella. After Bonferroni correction, we found that 13 genes in the three tissues were significantly associated with rubella (Figure 2D; Table 4). In rubella, we found five significant genes, namely, JAGN1 (p adjusted = 0.036), RRP12 (p adjusted = 0.017), RP11-452K12.7 (p adjusted = 0.006), CASP7 (p adjusted = 0.048), and AP3S2 (p adjusted = 0.020) in the whole blood, while in the lung tissue, we found IL17RC (p adjusted = 0.035), FAM86HP (p adjusted = 0.034), AMACR (p adjusted = 0.012), and RRP12 (p adjusted = 0.006) to be significantly associated with rubella. Similarly, in transformed fibroblast cells, we found PPP2R1B (p adjusted = 0.022), C11orf1 (p adjusted = 0.029), DLAT (p adjusted = 0.045), and TMEM117 (p adjusted = 0.046) as rubella-related genes.
Discussion
In this study, we used tissue-specific TWAS to explore the association of genetically predicted gene expression with IAV, measles, rubella, and mumps and identified 19, 14, 15, and 13 genes in three tissues as potential IAV-, measles-, mumps-, and rubella- related genes, respectively. In IAV, including three in whole blood (ULK4, AC010132.11, and SURF1), four in the lung tissue (NIPAL2, TRAP1, TAF1C, and AC000078.5), nine in the transformed fibroblast cells (RP4-639F20.1, RMDN2, ATP1B3, SRSF12, RP11-477D19.2, TFB1M, XXyac-YX65C7_A.2, TAF1C, and PCGF2), and one expressed in three tissues as significantly associated with IAV (BNIP1). In measles, including six in whole blood (SOAT1, COLGALT2, AC021860.1, HCG11, METTL21B, and MRPL10), six in the lung tissue (GSTM4, PAQR6, RP11-617D20.1, SNX8, METTL21B, and ANKRD27), and two in the transformed fibroblast cells (CBWD2, and TSFM). In mumps, including six in whole blood (MTOR, LAMC1, TRIM38, U91328.21, POLR2J, and SCRN2), six in the lung tissue (Smpd4, UBN1, CNTROB, SCRN2, HOXB-AS1, and SLC14A1), and three in the transformed fibroblast cells (AC007566.10, AC093668.2, and CPD). In rubella, including five in whole blood (JAGN1, RRP12, RP11-452K12.7, CASP7, and AP3S2), four in the lung tissue (IL17RC, FAM86HP, AMACR, and RRP12), and four in the transformed fibroblast cells (PPP2R1B, C11orf1, DLAT, and TMEM117). The above 59 genes have never been clearly reported to be associated with IAV, measles, mumps, and rubella.
TNF receptor-associated protein-1 (TRAP1), a member of the mitochondria-specific Hsp90 family, is located in the mitochondrial matrix, mitochondrial endometrium, and the intermembrane space (Cechetto and Gupta, 2000; Pridgeon et al., 2007). However, among the few real TRAP1 clients described, two are subunits of the electron transport chain complex (ETC), a component of the complex II succinate dehydrogenase subunit A/B (SDHA/B) (Sanchez-Martin et al., 2020; Sanchez-Martin et al., 2021), and complex IV cytochromes c oxidase subunit 2 (COXII) (Xiang et al., 2016; Xiang et al., 2019). The II/SDH complex is a protein complex containing iron and sulfur groups whose function is to transfer electrons from succinate to the coenzyme Q10-ubiquinone (III complex) (Bezawork-Geleta et al., 2017). TRAP1 leaves the SDH in a partially unfolded state. Inhibition of TRAP1 can release active SDH and increase its activity (Agarwal et al., 2019; Hu et al., 2020). In addition, SDH activity (Masgras et al., 2017; Hu et al., 2020) and oxygen consumption (Masgras et al., 2017) were negatively correlated with TRAP1 expression, suggesting that TRAP1 promotes the Warburg effect (Guzzo et al., 2014). It should be noted that SDH also oxidizes succinic acid to fumarate, thus integrating the TCA cycle, suggesting a broad impact of TRAP1 on mitochondrial metabolism (Guzzo et al., 2014; Hsu et al., 2016). It can be assumed that the abnormal expression of the TRAP1 gene may contribute to the development of IAV by affecting mitochondrial metabolic processes.
GSTM4 belongs to the mu class of glutathione S-transferase (GST). Compared to other GSTMs (Comstock et al., 1993), GSTM4 exhibits greater consistency in amino acid sequence but has significant differences in physicochemical properties and tissue distribution. GSTM4 does not exhibit activity comparable to standard TPS substrates, and its specific substrate has not been identified. It is well known that the GST gene is highly polymorphic to adapt to the growing number of exotic compounds (Hayes and Strange, 2000). This polymorphism can alter individual susceptibility to the disease and response to therapeutic agents. For example, the T2517C polymorphism in GSTM4 has been shown to be associated with an increased risk of lung cancer (Liloglou et al., 2002). The mechanism underlying this association is not yet clear. Currently, there are few reports of the relationship between the GSTM4 gene and the disease. Efforts may be needed to verify its role in infectious respiratory diseases.
In addition to the aforementioned genes, we have identified other candidate genes (SOAT1, HCG11, PAQR6, and AP3S2) in different tissues, of which AP3S2 has been reported to be associated with the development of type 2 diabetes (Kazakova et al., 2017). Other genes have also been implicated in cancer. For example, SOAT1 has been linked to the development of stomach cancer (Zhu et al., 2021), and pancreatic cancer (Oni et al., 2020); HCG11 may influence the development of nasopharyngeal carcinoma (Zheng et al., 2022); the PAQR6 gene has also been associated with several cancers (Yang et al., 2021). Although there is little research on these genes and respiratory infectious diseases, the potential links between these genes deserve further attention in future studies.
In summary, our results list some genes for respiratory infections that have not been studied before. Based on the previous knowledge of these genes, this means that they may be involved in host infection through transcriptional processes of susceptible genes and RNA degradation processes. This study is helpful to deepen the understanding of the pathogenesis of respiratory infectious diseases.
Data availability statement
Publicly available data sets were analyzed in this study. These data can be found here: https://www.ebi.ac.uk/gwas/downloads/summary-statistics.
Author contributions
XZ, YZ, and LJ contributed equally to the conception, design, and execution of the study, as well as to the analysis and interpretation of data. XZ, YZ, LJ, XY, YZo, JT, JL, SY, RY, and PH drafted, revised, and approved the manuscript. RY and PH are the corresponding authors who took responsibility for the final submission. All authors agree to be accountable for all aspects of the work, ensuring the accuracy and integrity of the content.
Funding
This study was sponsored by the National Natural Science Foundation of China (82173585 and 82273741), the Natural Science Foundation of Jiangsu Province (BK20190106), and the Priority Academic Program Development of Jiangsu Higher Education Institutions (PAPD).
Acknowledgments
This study is a joint effort of many investigators and staff members, and their contributions are gratefully acknowledged.
Conflict of interest
The authors declare that the research was conducted in the absence of any commercial or financial relationships that could be construed as a potential conflict of interest.
Publisher’s note
All claims expressed in this article are solely those of the authors and do not necessarily represent those of their affiliated organizations, or those of the publisher, editors, and reviewers. Any product that may be evaluated in this article, or claim that may be made by its manufacturer, is not guaranteed or endorsed by the publisher.
Supplementary material
The Supplementary Material for this article can be found online at: https://www.frontiersin.org/articles/10.3389/fgene.2023.1164274/full#supplementary-material
References
Agarwal, E., Altman, B. J., Seo, J. H., Ghosh, J. C., Kossenkov, A. V., Tang, H. Y., et al. (2019). Myc-mediated transcriptional regulation of the mitochondrial chaperone TRAP1 controls primary and metastatic tumor growth. J. Biol. Chem. 294 (27), 10407–10414. doi:10.1074/jbc.AC119.008656
Albert, F. W., and Kruglyak, L. (2015). The role of regulatory variation in complex traits and disease. Nat. Rev. Genet. 16 (4), 197–212. doi:10.1038/nrg3891
Bezawork-Geleta, A., Rohlena, J., Dong, L., Pacak, K., and Neuzil, J. (2017). Mitochondrial complex II: At the crossroads. Trends Biochem Sci. 42 (4), 312–325. doi:10.1016/j.tibs.2017.01.003
Bouvier, N. M., and Palese, P. The biology of influenza viruses. Vaccine. 2008;26 (4):D49–D53. doi:10.1016/j.vaccine.2008.07.039
Cechetto, J. D., and Gupta, R. S. (2000). Immunoelectron microscopy provides evidence that tumor necrosis factor receptor-associated protein 1 (TRAP-1) is a mitochondrial protein which also localizes at specific extramitochondrial sites. Exp. Cell Res. 260 (1), 30–39. doi:10.1006/excr.2000.4983
Comstock, K. E., Johnson, K. J., Rifenbery, D., and Henner, W. D. (1993). Isolation and analysis of the gene and cDNA for a human Mu class glutathione S-transferase, GSTM4. J. Biol. Chem. 268 (23), 16958–16965. doi:10.1016/s0021-9258(19)85287-0
Derks, E. M., and Gamazon, E. R. (2020). Transcriptome-wide association analysis offers novel opportunities for clinical translation of genetic discoveries on mental disorders. World Psychiatry 19 (1), 113–114. doi:10.1002/wps.20702
GBD 2015 LRI Collaborators (2017). Estimates of the global, regional, and national morbidity, mortality, and aetiologies of lower respiratory tract infections in 195 countries: A systematic analysis for the global burden of disease study 2015. Lancet Infect. Dis. 17 (11), 1133–1161. doi:10.1016/S1473-3099(17)30396-1
Gorlova, O. Y., Xiao, X., Tsavachidis, S., Amos, C. I., and Gorlov, I. P. (2022). SNP characteristics and validation success in genome wide association studies. Hum. Genet. 141 (2), 229–238. doi:10.1007/s00439-021-02407-8
Gusev, A., Ko, A., Shi, H., Bhatia, G., Chung, W., Penninx, B. W. J. H., et al. (2016). Integrative approaches for large-scale transcriptome-wide association studies. Nat. Genet. 48 (3), 245–252. doi:10.1038/ng.3506
Guzzo, G., Sciacovelli, M., Bernardi, P., and Rasola, A. (2014). Inhibition of succinate dehydrogenase by the mitochondrial chaperone TRAP1 has antioxidant and anti-apoptotic effects on tumor cells. Oncotarget 5 (23), 11897–11908. doi:10.18632/oncotarget.2472
Harrington, W. N., Kackos, C. M., and Webby, R. J. (2021). The evolution and future of influenza pandemic preparedness. Exp. Mol. Med. 53 (5), 737–749. doi:10.1038/s12276-021-00603-0
Hayes, B. (2013). Overview of statistical methods for genome-wide association studies (GWAS). Methods Mol. Biol. 1019, 149–169. doi:10.1007/978-1-62703-447-0_6
Hayes, J. D., and Strange, R. C. (2000). Glutathione S-transferase polymorphisms and their biological consequences. Pharmacology 61 (3), 154–166. doi:10.1159/000028396
Hsu, C. C., Tseng, L. M., and Lee, H. C. (2016). Role of mitochondrial dysfunction in cancer progression. Exp. Biol. Med. (Maywood). 241 (12), 1281–1295. doi:10.1177/1535370216641787
Hu, S., Ferraro, M., Thomas, A. P., Chung, J. M., Yoon, N. G., Seol, J. H., et al. (2020). Dual binding to orthosteric and allosteric sites enhances the anticancer activity of a TRAP1-targeting drug. J. Med. Chem. 63 (6), 2930–2940. doi:10.1021/acs.jmedchem.9b01420
Hui, Z., and Hastie, T. (2005). Regularization and variable selection via the elastic net. J. R. Stat. Soc. 67 (5), 768.
Iacobucci, G. (2022). Measles is now "an imminent threat" globally, WHO and CDC warn. BMJ 379, o2844. doi:10.1136/bmj.o2844
Kauffmann, F., Heffernan, C., Meurice, F., Ota, M. O. C., Vetter, V., and Casabona, G. (2021). Measles, mumps, rubella prevention: How can we do better? Expert Rev. Vaccines 20 (7), 811–826. doi:10.1080/14760584.2021.1927722
Kazakova, E. V., Zghuang, T., Li, T., Fang, Q., Han, J., and Qiao, H. (2017). The Gas6 gene rs8191974 and Ap3s2 gene rs2028299 are associated with type 2 diabetes in the northern Chinese Han population. Acta Biochim. Pol. 64 (2), 227–231. doi:10.18388/abp.2016_1299
Kennedy, R. B., Ovsyannikova, I. G., Haralambieva, I. H., Lambert, N. D., Pankratz, V. S., and Poland, G. A. (2014). Genome-wide SNP associations with rubella-specific cytokine responses in measles-mumps-rubella vaccine recipients. Immunogenetics 66 (7-8), 493–499. doi:10.1007/s00251-014-0776-3
Liloglou, T., Walters, M., Maloney, P., Youngson, J., and Field, J. K. (2002). A T2517C polymorphism in the GSTM4 gene is associated with risk of developing lung cancer. Lung Cancer 37 (2), 143–146. doi:10.1016/s0169-5002(02)00078-8
Lin, H., Lunetta, K. L., Zhao, Q., Rong, J., Benjamin, E. J., Mendelson, M. M., et al. (2017). Transcriptome-wide association study of inflammatory biologic age. Aging (Albany NY) 9 (11), 2288–2301. doi:10.18632/aging.101321
Liu, D., Zhou, D., Sun, Y., Zhu, J., Ghoneim, D., Wu, C., et al. (2020). A transcriptome-wide association study identifies candidate susceptibility genes for pancreatic cancer risk. Cancer Res. 80 (20), 4346–4354. doi:10.1158/0008-5472.CAN-20-1353
Marshall, H. S., and Plotkin, S. The changing epidemiology of mumps in a high vaccination era. Lancet Infect. Dis. 2019;19(2):118–119. doi:10.1016/S1473-3099(18)30541-3
Masgras, I., Sanchez-Martin, C., Colombo, G., and Rasola, A. (2017). The chaperone TRAP1 as a modulator of the mitochondrial adaptations in cancer cells. Front. Oncol. 7, 58. . Published 2017 Mar 29. doi:10.3389/fonc.2017.00058
Oni, T. E., Biffi, G., Baker, L. A., Hao, Y., Tonelli, C., Somerville, T. D. D., et al. (2020). SOAT1 promotes mevalonate pathway dependency in pancreatic cancer. J. Exp. Med. 217 (9), e20192389. doi:10.1084/jem.20192389
Pridgeon, J. W., Olzmann, J. A., Chin, L. S., and Li, L. (2007). PINK1 protects against oxidative stress by phosphorylating mitochondrial chaperone TRAP1. PLoS Biol. 5 (7), e172. doi:10.1371/journal.pbio.0050172
Raghunathan, P. L., and Orenstein, W. (2022). Investing in global measles and rubella elimination is needed to avert deaths and advance health equity. Lancet Glob. Health 10 (10), e1363–e1364. doi:10.1016/S2214-109X(22)00388-6
Sabikunnahar, B., Lahue, K. G., Asarian, L., Fang, Q., McGill, M. M., Haynes, L., et al. (2022). Sex differences in susceptibility to influenza A virus infection depend on host genotype. PLoS One 17 (9), e0273050. Published 2022 Sep 16. doi:10.1371/journal.pone.0273050
Sanchez-Martin, C., Menon, D., Moroni, E., Ferraro, M., Masgras, I., Elsey, J., et al. (2021). Honokiol bis-dichloroacetate is a selective allosteric inhibitor of the mitochondrial chaperone TRAP1. Antioxid. Redox Signal 34 (7), 505–516. doi:10.1089/ars.2019.7972
Sanchez-Martin, C., Moroni, E., Ferraro, M., Laquatra, C., Cannino, G., Masgras, I., et al. (2020). Rational design of allosteric and selective inhibitors of the molecular chaperone TRAP1. Cell Rep. 31 (3), 107531. doi:10.1016/j.celrep.2020.107531
Scepanovic, P., Alanio, C., Hammer, C., Hodel, F., Bergstedt, J., Patin, E., et al. (2018). Human genetic variants and age are the strongest predictors of humoral immune responses to common pathogens and vaccines. Genome Med. 10 (1), 59. doi:10.1186/s13073-018-0568-8
Speed, D., Holmes, J., and Balding, D. J. (2020). Evaluating and improving heritability models using summary statistics. Nat. Genet. 52 (4), 458–462. doi:10.1038/s41588-020-0600-y
Thériault, S., Gaudreault, N., Lamontagne, M., Rosa, M., Boulanger, M. C., Messika-Zeitoun, D., et al. (2018). A transcriptome-wide association study identifies PALMD as a susceptibility gene for calcific aortic valve stenosis. Nat. Commun. 9 (1), 988. . Published 2018 Mar 7. doi:10.1038/s41467-018-03260-6
Tibshirani, R. (1996). Regression shrinkage and selection via the lasso. J. R. Stat. Soc. Ser. B 58 (1), 267–288. doi:10.1111/j.2517-6161.1996.tb02080.x
Veturi, Y., and Ritchie, M. D. (2018). How powerful are summary-based methods for identifying expression-trait associations under different genetic architectures? Pac Symp. Biocomput 23, 228–239.
Voigt, E. A., Haralambieva, I. H., Larrabee, B. L., Kennedy, R. B., Ovsyannikova, I. G., Schaid, D. J., et al. (2018). Polymorphisms in the wilms tumor gene are associated with interindividual variations in rubella virus-specific cellular immunity after measles-mumps-rubella II vaccination. J. Infect. Dis. 217 (4), 560–566. doi:10.1093/infdis/jix538
Xiang, F., Ma, S. Y., Lv, Y. L., Zhang, D. X., Song, H. P., and Huang, Y. S. (2019). Tumor necrosis factor receptor-associated protein 1 regulates hypoxia-induced apoptosis through a mitochondria-dependent pathway mediated by cytochrome c oxidase subunit II. Burns Trauma 7, 16. 16. Published 2019 May 23. doi:10.1186/s41038-019-0154-3
Xiang, F., Ma, S. Y., Zhang, D. X., Zhang, Q., and Huang, Y. S. (2016). Tumor necrosis factor receptor-associated protein 1 improves hypoxia-impaired energy production in cardiomyocytes through increasing activity of cytochrome c oxidase subunit II. Int. J. Biochem Cell Biol. 79, 239–248. doi:10.1016/j.biocel.2016.08.041
Xiao, R., Gu, L., Li, A. M., Gan, Y. L., Liao, J. X., et al. (2023). IL-11 drives the phenotypic transformation of tracheal epithelial cells and fibroblasts to enhance abnormal repair after tracheal injury. Biochim. Biophys. Acta Mol. Cell Res. 1870 (4), 119438. doi:10.1016/j.bbamcr.2023.119438
Yang, M., Li, J. C., Tao, C., Wu, S., Liu, B., Shu, Q., et al. (2021). PAQR6 upregulation is associated with AR signaling and unfavorite prognosis in prostate cancers. Biomolecules 11 (9), 1383. . Published 2021 Sep 18. doi:10.3390/biom11091383
Yang, S., and Zhou, X. (2020). Accurate and scalable construction of polygenic scores in large biobank data sets. Am. J. Hum. Genet. 106 (5), 679–693. doi:10.1016/j.ajhg.2020.03.013
Yang, S., and Zhou, X. (2022). PGS-Server: Accuracy, robustness and transferability of polygenic score methods for biobank scale studies. Brief. Bioinform 23 (2), bbac039. doi:10.1093/bib/bbac039
Zhang, Y., Sun, Y., Zhu, H., Hong, H., Jiang, J., Yao, P., et al. (2022). Allelic imbalance of HLA-B expression in human lung cells infected with coronavirus and other respiratory viruses. Eur. J. Hum. Genet. 30 (8), 922–929. doi:10.1038/s41431-022-01070-5
Zheng, J., Zhao, Z., Ren, H., Wang, Y., Meng, X., Zhang, W., et al. (2022). LncRNA HCG11 facilitates nasopharyngeal carcinoma progression through regulating miRNA-490-3p/MAP3K9 Axis. Front. Oncol. 12, 872033. Published 2022 Apr 7, doi:10.3389/fonc.2022.872033
Zhou, X., Carbonetto, P., and Stephens, M. (2013). Polygenic modeling with bayesian sparse linear mixed models. PLoS Genet. 9 (2), e1003264. doi:10.1371/journal.pgen.1003264
Keywords: transcriptome-wide association study, IAV, measles, mumps, rubella, multiple tissues, genome-wide association studies, gene expression
Citation: Zhu X, Zou Y, Jia L, Ye X, Zou Y, Tu J, Li J, Yu R, Yang S and Huang P (2023) Using multi-tissue transcriptome-wide association study to identify candidate susceptibility genes for respiratory infectious diseases. Front. Genet. 14:1164274. doi: 10.3389/fgene.2023.1164274
Received: 12 February 2023; Accepted: 03 March 2023;
Published: 20 March 2023.
Edited by:
Xianyong Yin, Department of Biostatistics, University of Michigan, Ann Arbor, United StatesReviewed by:
Xingjie Shi, East China Normal University, ChinaYue Fan, Xi’an Jiaotong University, China
Copyright © 2023 Zhu, Zou, Jia, Ye, Zou, Tu, Li, Yu, Yang and Huang. This is an open-access article distributed under the terms of the Creative Commons Attribution License (CC BY). The use, distribution or reproduction in other forums is permitted, provided the original author(s) and the copyright owner(s) are credited and that the original publication in this journal is cited, in accordance with accepted academic practice. No use, distribution or reproduction is permitted which does not comply with these terms.
*Correspondence: Rongbin Yu, rongbinyu@njmu.edu.cn; Peng Huang, huangpeng@njmu.edu.cn
†These authors have contributed equally to this work and share first authorship