- Centre for Omics Sciences, IRCCS San Raffaele Scientific Institute, Milan, Italy
In the last years, liquid biopsy gained increasing clinical relevance for detecting and monitoring several cancer types, being minimally invasive, highly informative and replicable over time. This revolutionary approach can be complementary and may, in the future, replace tissue biopsy, which is still considered the gold standard for cancer diagnosis. “Classical” tissue biopsy is invasive, often cannot provide sufficient bioptic material for advanced screening, and can provide isolated information about disease evolution and heterogeneity. Recent literature highlighted how liquid biopsy is informative of proteomic, genomic, epigenetic, and metabolic alterations. These biomarkers can be detected and investigated using single-omic and, recently, in combination through multi-omic approaches. This review will provide an overview of the most suitable techniques to thoroughly characterize tumor biomarkers and their potential clinical applications, highlighting the importance of an integrated multi-omic, multi-analyte approach. Personalized medical investigations will soon allow patients to receive predictable prognostic evaluations, early disease diagnosis, and subsequent ad hoc treatments.
Introduction
In the last decades, the old “one-size-fits-all” approach in cancer treatment has been replaced by a personalized model in which therapeutic strategy is based on biological features of the patient’s disease (Gambardella et al., 2020; Kulavi et al., 2021). This approach, known as personalized medicine, aims to identify patients who will respond to specific therapies by reducing the risk of adverse effects as well as improving the sustainability of healthcare systems (Biankin et al., 2015; Jameson and Longo, 2015; Hyman et al., 2017; Yates et al., 2018). During the selection of “the right treatment for the right person,” the identification of a new generation of biomarkers, that guide all aspects of cancer patient care, represents the most urgent challenge today (Sawyers, 2008). Tissue-based biomarkers are currently used for tumor diagnosis and therapy response prediction; however, they do not allow treatment real-time monitoring and early identification of resistance mechanisms. In some cases, tissue biopsy is invasive, expensive and time consuming, but above all, it is totally inappropriate in capturing tumor heterogeneity. Indeed, we can identify only a fraction of tumor heterogeneity since serial sampling is not clinically practical and is affected by the patient’s health status.
To overcome these limitations, a non-invasive sampling approach known as liquid biopsy (LB) was developed (Lone et al., 2022). Based on the study of circulating biomarkers in biological fluids, it aims to circumvent the temporal and spatial heterogeneity of the tumor by providing valuable information on the onset and progression of the disease over time (Siravegna et al., 2017; Ignatiadis et al., 2021). Besides blood, different biofluids such as urine (Nuzzo et al., 2020; Xu et al., 2020), stool (Diehl et al., 2008a), saliva/sputum (Wu et al., 2019), pleural effusions (Li et al., 2019a), and cerebrospinal fluid (CSF) (Miller et al., 2019) can be used to isolate circulating tumor components for clinical applications (Figure 1). Biologically, elements that can be analyzed using LB are classified into two main categories: targets with cellular or subcellular structures such as circulating tumor cells (CTCs) (Zhang et al., 2015; Jordan et al., 2016; Guo et al., 2018; Zhou et al., 2019), extracellular vesicles (EVs) (McKiernan et al., 2016; Mannavola et al., 2019; Sun et al., 2020) and tumor-educated platelets (TEPs) (Best et al., 2015); and molecules without cellular structures such as cell-free (cf) nucleic acids (cfDNA and cfRNA) (Diehl et al., 2008b; Comino-Mendez and Turner, 2017; Urabe et al., 2019; Quirico and Orso, 2020; Lo et al., 2021; Wada et al., 2021; Joosse and Pantel, 2022) proteins (Martinez-Garcia et al., 2017; Signore et al., 2021), metabolites (Lee et al., 2020; Maslov et al., 2022) and lipids (Saito et al., 2021; Wolrab et al., 2022). Over the past twoades, thanks to the great advancement in sequencing technologies, a more genomic approach has been used in clinical oncology. This brought an improvement in personalized medicine in terms of prevention and treatment, providing a wide range of information on the mutational and molecular structure of many tumors (Jamal-Hanjani et al., 2016; Scherer et al., 2016; Thompson et al., 2016). The analysis of each component provides useful information on cancer diagnosis, prognosis and treatment (Lone et al., 2022). In the clinical setting, the detection of CTC above the cut-off value has a negative prognostic value in metastatic patients (Cristofanilli et al., 2004; Cohen et al., 2008) as well as the identification of a specific mutation on the ctDNA can direct therapeutic treatment or indicate drug resistance (Garlan et al., 2017). However, the exclusive use of a single omic approach does not have the power to establish all causal relationships between molecular alterations and phenotypic manifestations.
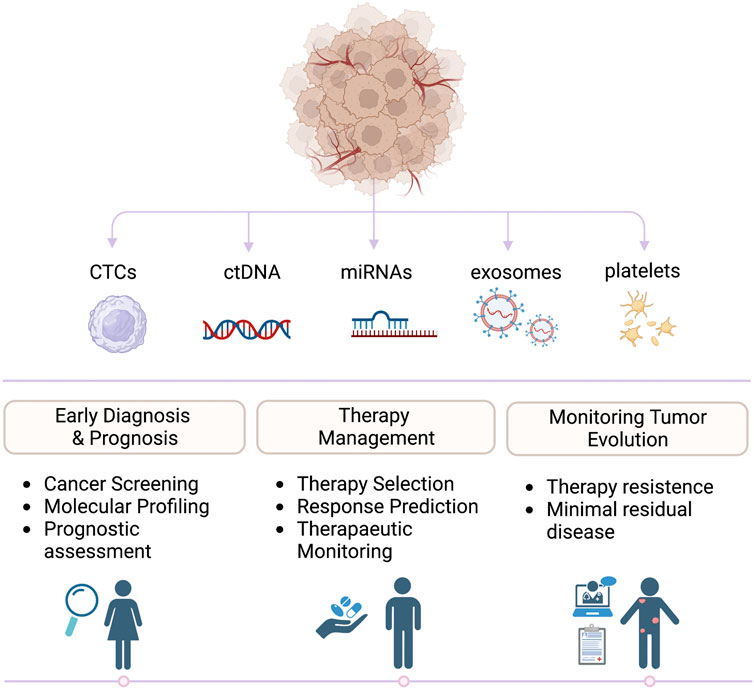
FIGURE 1. Clinical applications of liquid biopsies. Biomarkers released by primary tumors and metastasis can be detected in liquid biopsies and analyzed to guide prognosis, diagnosis, and treatments in oncologic patients.
To better understand the mechanisms underlying different phenotypes during malignant transformation, investigating different omics, e.g., transcriptomics, epigenomics, proteomics and metabolomics, became necessary. Although LB represents a promising tool to monitor the dynamic evolution of cancer in a non-invasive way, its integration into clinical practice is hampered by the lack of reproducibility due to the absence of standardization across workflows (Salvianti et al., 2020). The main goal is to develop unique procedures for detection and analysis of liquid components that are reproducible and have a high degree of sensitivity and specificity.
In this review we are going to explore how the main single omics are interrogated in LB, discussing multiple types of cancers and clinical applications. At the same time, we will explain how multidimensional analysis of different liquid components and integration of omics will lead to new biomarkers discovery for cancer management, and identification of therapeutic targets linked to cancer-specific molecular pathways.
Liquid biopsy elements
Circulating tumor cells
Described for the first time in 1869 by Ashworth, CTCs are tumor cells that detach from the main tumor masses and travel through the circulatory systems (Pantel and Speicher, 2016). It took researchers more than a century to understand that they represent the seed for metastasis (Nguyen et al., 2009; Eslami-S et al., 2022). Epithelial to mesenchymal transition (EMT) properties and stemness features allow their dissemination in distant organs (Ye and Weinberg, 2015; Fares et al., 2020). CTCs preserve tumor heterogeneity and mimic cancer properties: for this reason, they can be used as clinical biomarkers for disease screening, dynamic monitoring and prognosis prediction (Pantel et al., 2013; Jamal-Hanjani et al., 2015; Keller and Pantel, 2019).
CTCs are related to tumor stage (Ankeny et al., 2016; Cristofanilli et al., 2019) but their clinical utility in cancer screening and/or early detection is still under debate. CTCs prognostic value has been amply proven, and their enumeration in some metastatic tumors became an independent prognostic factor (Cristofanilli et al., 2004; Cohen et al., 2008). In addition, both the presence and the size of CTC clusters can be associated with a worse clinical outcome than single CTCs (Chang et al., 2016; Paoletti et al., 2019; Lim et al., 2021).
CTCs molecular phenotype has also a strong prognostic value: for instance patients with CTC expressing mesenchymal markers (Armstrong et al., 2011), stem markers (Lecharpentier et al., 2011) or antigens such as PD-L1 (Kong et al., 2021), HER2 (Müller et al., 2021), CD47 (Agelaki et al., 2019) undergo reduced Progression Free Survival (PFS) and Overall Survival (OS). Also CD44 and CD77 have been lately proposed as prognostic markers of brain metastases (Loreth et al., 2021).
To predict and monitor therapeutic responses, CTCs can be used in combination with serum biomarkers and imaging, as demonstrated by several clinical studies. For example, patients with Castration-Resistant Prostate Cancer (CRPC) expressing androgen receptor 7 splice variant (AR-V7) protein on CTCs had a better survival rate after taxane chemotherapy (Graf et al., 2020). Expression of PDL1 on CTC seems to be a promising predictive biomarker of treatment response when using immunotherapy in Non-Small Cell Lung Cancer (NSCLC) (Kloten et al., 2019). CTC genomic aberrations and surface protein alterations can be used for monitoring tumor resistance to therapeutic regimen in breast (Gasch et al., 2016; Jordan et al., 2016), lung (Maheswaran et al., 2008; Sundaresan et al., 2016; Chang et al., 2021) and prostate cancer (Darshan et al., 2011; de Bono et al., 2021).
CTCs are difficult to detect due to their low amount and the paucity of standardized detection strategies (Table 1). To sort out this issue, usage of CTC-derived xenograft (CDX) models and CTC-derived ex vivo cultures have been suggested: these models represent an opportunity to identify drug susceptibility changes in patients as their tumors evolve (Hodgkinson et al., 2014; Lee et al., 2015; Suvilesh et al., 2022), as well as to understand the role of CTCs in metastatic process and metastases organotropism (Klotz et al., 2020; Bowley and Marchetti, 2022).
Circulating tumor DNA
Since its observation in human plasma in the late 1940s (Mandel and Metais, 1948), cfDNA has received enormous attention as a noninvasive disease biomarker. Unlike cfDNA, circulating tumor DNA (ctDNA) is present in very low concentrations, ranging from ≥ 5%–10% in late stage, to ≤ 0.01%–0.1% in early stage cancers (Bettegowda et al., 2014) and has a greater degree of fragmentation. Several studies demonstrate how the length of the fragments can be linked to the mechanism of release into the circulation. Indeed, while short fragments (<200 bp) are released during apoptosis, large fragments (>200 bp) originate during necrosis (Jiang and Lo, 2016) or from viable cancer cells of primary tumors and/or metastases.
By using ctDNA we can detect specific cancer-related alterations, including point mutations, copy number variations (CNVs), and methylation changes that provide valuable insight into disease status. There is an open debate in the scientific community about the use of ctDNA for early cancer detection (Fiala and Diamandis, 2018). Grail is a company developing an Artificial Intelligence (AI)-aided early cancer detection test based on cfDNA analysis. In colon cancer (CC), the absence of ctDNA after surgery has been shown to be associated with a better prognosis and a low chance of recurrence (Schraa et al., 2022). Tracking alterations in ctDNA also allow us to detect minimal residual disease and predict recurrence several months in advance, as seen in patients with colon (Schraa et al., 2022), breast (Coombes et al., 2019) lung (Otsubo et al., 2019), ovarian (Lin et al., 2019) and prostate (Wyatt et al., 2016) cancer.
Over the last decade, the Food and Drug Administration (FDA) has approved a series of LB tests based on ctDNA. The Cobas EGFR Mutation Test v2 (Roche, Basel, Switzerland) is a diagnostic test for EGFR tyrosine kinase inhibitor therapies in NSCLC, while Guardant360 CDx (Guardant Health, Lansdale, PA, USA) and Liquid CDx (Foundation Medicine, Cambridge, MA, USA), analyzing the complete tumor genomic profile, help clinicians to understand responsiveness to checkpoint inhibitors and targeted therapy. In the near future, it will be necessary to validate non-invasive methods to detect limited amounts of ctDNA to guide personalized therapeuticisions.
Exosome
Exosome-based oncology research has recently achieved impressive results by offering potential tools for the clinical management of the disease. Initially considered a cellular waste disposal system, they are important players in intercellular communication (Maia et al., 2018).
They are small extracellular vesicles released from every type of cell which circulate stably in most biological fluids such as blood, urine, milk, saliva. They carry nucleic acids, proteins and lipids and can reach very distant cells influencing their biological functions (Zhang et al., 2019).
In cancer exosomes are involved in remodeling of the tumor microenvironment (Huang et al., 2022a), formation of pre-metastatic niches (Costa-Silva et al., 2015) and immune-escape (Chen et al., 2018).
In liquid biopsy, for their unique features, exosomes have complementary and potentially broader applications than CTCs and ctDNA. Melo et al. (2015) found high levels of GPC1+-circulating exosomes in Pancreatic Ductal Adenocarcinoma (PDAC) patients compared to healthy controls, suggesting a strong correlation between GPC-1 exosome and cancer. Other recent studies suggested the prognostic role of circulating exosomal microRNA (miRNA). In CRPC patients, high levels of miR-1290 and -375 were significantly associated with poor OS in the follow-up cohort (Huang et al., 2015a). Besides early diagnosis and prognosis, exosomes also play a potential role in treatment response assessment. In metastatic melanoma patients, the increase in circulating exosomal PD-L1 during the early stages of pembrolizumab treatment might reflect the presence of successful antitumor immunity elicited by anti-PD-1 therapy (Chen et al., 2018). Del Re and collaborators (Del Re et al., 2017) proved that the presence of AR-V7 in exosomal RNA was associated with shorter OS and resistance to hormone therapy in CRPC patients. Despite the tangible therapeutic potential of exosomes, the lack of standardized protocols and universally accepted markers for quality control limits their use in the preclinical setting.
During exosome analysis, the real challenge to overcome is discriminating tumor exosomes from non-tumor extracellular vesicles still. A possible solution could be using highly specific analytical techniques such as mass spectrometry (MS), Flow Cytometry (FC) and High Throughput Sequencing (HTS). Big data analysis would then be capable of distinguishing tumor exosomes from the physiological material (Nguyen et al., 2019).
microRNA
To date, the majority of circulating nucleic acid studies in oncology concern ctDNA. However the spotlight has turned on cfRNA which has promised to improve cancer diagnosis and treatment (Anfossi et al., 2018). Like exosomes, cfRNA can derive from cancerous cells, but also from non-tumor components such as stroma and the immune system. The analysis of cfRNA dynamically reflects the changes that occur in the tumor microenvironment, revealing important intercellular signaling that can be exploited in clinical practice (Anfossi et al., 2018). In the field of cfRNA biomarkers, most studies have focused on miRNAs for their greater stability in biological fluids (Glinge et al., 2017). miRNAs are a family of small non-coding RNAs that regulate a wide array of biological processes including carcinogenesis (Lu et al., 2005). They circulate in the blood carried by exosomes, apoptotic bodies or protein-miRNA complexes, functioning as either oncogenes or tumor suppressors depending on the conditions (Bartel, 2004).
In the last years, several studies demonstrated that miRNA expression is dysregulated in cancer, and its signatures could be used for diagnosis, prognosis and therapeutic management of cancer (Bartel, 2004; Reda El Sayed et al., 2021). Recently, more miRNAs have been investigated such as early diagnostic and prognosis markers for lung cancer often associated with biomarkers such as CEA and Cyfra 21-1 (Liu et al., 2021a). miRNAs may also be used to evaluate therapeutic outcomes. An example the chemotherapy increases the serologic concentration of miRNAs in colon cancer patients (Hansen et al., 2015). However, what emerges from all the studies is a high variability as more miRNAs can be associated with the same tumor with a different sensitivity and specificity. This can be partly explained by the differences in the samples tested, in terms of ethnicity of the courts, the use of endogenous controls, the biofluid analyzed, but above all on the detection method used. Consequently, standardization of procedures is needed to take full advantage of miRNAs as cancer biomarkers (Cabús et al., 2022).
Tumor-educated platelets
The interaction between tumors and platelets was first observed in 1868 when Trousseau noted that spontaneous coagulation was common in cancer patients. A decade later, Billroth described that these clots contained cancer cells and for this reason could be agents of metastasis (Billroth, 1871). Today we know that platelets, besides carrying out protein synthesis in the absence of a nucleus, continuously exchange nucleic acids and circulating proteins with the tumor and its microenvironment (In ’t Veld and Wurdinger, 2019). TEPs are the result of this exchange which can be achieved by the direct recruitment of tumor components or indirectly by post-transcriptional splicing (Roweth and Battinelli, 2021). Thus far, the preliminary data strongly suggest that TEP-derived mRNA onco-signatures may be harnessed for cancer diagnostics, with many potential applications (Best et al., 2018). Best et al. compared TEPs derived from healthy donors and both treated and untreated patients with early, localized, or advanced metastatic cancer. They reported that molecular interrogation of blood platelet mRNA can offer valuable diagnostics information for all cancer patients analyzed—spanning six different tumor types. Platelets may be employable as an all-in-one biosource to broadly search molecular traces of cancer and provide a strong indication on tumor type and molecular subclass (Best et al., 2015). Their involvement in metastasis generation has been recently studied: TEPs seem to contribute to the survival of CTCs by protecting them from immune attacks and shear stress, promoting CTC intravenous extravasation (Liu et al., 2021b; Pereira-Veiga et al., 2022).
All in all, several liquid biopsy markers have been characterized for their clinical relevance in informing about disease biology, treatment response and progression. Each of those makers has specific strengths and limitations, and it is apparent that their combined use could be instrumental in obtaining a comprehensive description of tumor features.
For example, CTCs are informative of the tumor phenotype and genotype, representing a source of information about tumor heterogeneity and presence of molecular targets for therapy.
CTC analysis anyway poses some limitations mostly related to their difficulty to be isolated due to their limiting number in the bloodstream. On the contrary, ctDNA is informative of tumor genotype, and also of its epigenetic profile (e.g., fragmentation profile, methylation status) and is therefore useful to assess mutational profile, clonality, and tissue-of-origin. Anyway, some limits in sensitivity and specificity due to presence of other cfDNA sources and limited ctDNA quantities are still present.
Relatively new markers such as exosomes and platelets are a great promise for liquid biopsy as they hold information about relevant tumor biomolecules (nucleic acids, proteins) instrumental to retrieve information about tumor biology and microenvironment. Their combined analysis with more established markers such as CTC and ctDNA can help in obtaining a more comprehensive picture of tumor of origin, with clinical impact in diagnosis and monitoring.
Genomics
Genomic analysis
Tumor genetic profile is assessed in the clinical routine by molecular characterization of biopsy specimens. Due to the invasive nature of the procedure, limitations in longitudinal monitoring and intrinsic sampling biases, LB represents a valuable alternative tool to investigate the genomic landscape of tumors during time (Crowley et al., 2013). The two main sources of DNA to be evaluated in LB are ctDNA and CTCs. They both give a snapshot of the tumor mutational profile at a defined moment of the disease history. Specifically, CTCs and ctDNA can inform about point mutations, CNVs and genomic rearrangement (Tan et al., 2016). The degree of fragmentation of ctDNA and the scarce amount of genomic material contained in CTCs are factors influencing the method of choice for the downstream genomic analysis, in order to avoid bias-related amplification and to retain biological information.
Available techniques to assess the genomic profile of ctDNA and CTCs can be divided into targeted and whole-genome, targeted approaches analyze the mutational profile of a subset of genes that have been linked to the pathogenesis of disease and include clinically actionable genes of interest. Such analysis can be performed using droplet digital PCR (ddPCR) (Hindson et al., 2013) or Next Generation Sequencing (NGS)-based approaches based on amplicon sequencing such as in Tagged-Amplicon Deep Sequencing (TAm-Seq) (Forshew et al., 2012), Next Generation-Targeted Amplicon Sequencing (NG-TAS) (Gao et al., 2019) or hybridization-based capturing as in Cancer Personalized Profiling by Deep Sequencing (CAPP-Seq) (Table 2). Several commercially available panels are focused on specific gene sets and can be either specific for a tumor type or pan-cancer, covering up to hundreds of regions. A more comprehensive targeted analysis can be focused on the entire set of expressed genes by Whole-Exome Sequencing (WES).
DDPCR is particularly indicated for rare mutation detection or to perform CNVs analysis (Li et al., 2019b). It is highly sensitive for detecting and quantifying low levels of ctDNA (Hindson et al., 2013) even when starting from a very limited amount of input DNA or searching for residual disease. A derivation of ddPRC, Beads, Emulsion, Amplification, Magnetics (BEAMing) (Diehl et al., 2008b) exploits emulsion PCR combined with flow cytometry to identify and quantify specific somatic mutations present in cfDNA. Basically, target DNA regions are amplified by specific primers, then encapsulated in droplets where each single fragment gets amplified by primers covalently attached to a magnetic bead. These DNA-coated beads are purified and labeled with fluorescent probes (one for mutant, the other one for wild-type) and finally analyzed by flow cytometry (Vessies et al., 2020).
As for the NGS-based approaches, CAPP-Seq was first time reported to study ctDNA in NSCLC by Newman et al., proving high sensitivity and subsequently applied to other cancer types (Newman et al., 2014; Nikanjam et al., 2022). This approach exploits bioinformatically designed biotinylated oligonucleotides called “selectors” to specifically target exons of recurrent mutated driver genes of specific tumors. The method shows the advantage of analyzing many regions at the same time, compared to ddPCR. CAPP-seq can not only be applied to study point mutations, but also CNVs while presenting limitations in fusion detections (Newman et al., 2014; Nikanjam et al., 2022).
TAm-Seq allows the amplification of specific regions of interest through the generation of short and overlapping fragments of about 150–200 bases, then indexed singularly and deep sequenced. This technique is particularly indicated for the detection of cancer mutations with an allele frequency up to 2%. Due to the short length of amplicons generated, the method is suitable for the analysis of highly fragmented ctDNA (Forshew et al., 2012; Li et al., 2019b; Nikanjam et al., 2022). The latest version of TAm-seq, Enhanced TAm-Seq (Gale et al., 2018; Xiao et al., 2023) leverages on multiplexed PCR and reduced the detection limit to 0.02% Allele Frequency (AF) with high per-base specificity (99.9%) using as low as 6.6 ng input cfDNA. Whole genome sequencing (WGS) approaches provide a more comprehensive view of genome aberration than targeted or WES since it includes all intronic sequences, including non-coding variations. This increased resolution over the genome requires a great amount of sequencing, making WGS the most expensive of all the described approaches. The advantage of targeted approaches is indeed the great depth of information for a fraction of the cost, which is relevant in clinical diagnosis setting.
For CTCs analysis, due to the low input single cell DNA amount, whole genome amplification (WGA) is necessary to achieve enough genomic material suitable for library preparation and sequencing. The main WGA techniques utilized are: Degenerate Oligonucleotide-Primed (DOP-PCR) (Telenius et al., 1992), Multiple Displacement Amplification (MDA) (Dean et al., 2001), Multiple Annealing and Looping Based Amplification Cycles (MALBAC) (Zong et al., 2012) (Table 2). Those techniques differ in the approach used for amplification and therefore have specific advantages and limitations. The DOP-PCR method consists of a two-step exponential amplification with random primers; this approach suffers from low uniformity of genome coverage and amplification. This limit is overcome in MDA and MALBAC (Huang et al., 2015b). MDA exploits high-fidelity polymerase Φ29 to perform displacement amplification with hexamer random primers (Spits et al., 2006) and performs better in terms of false positive rate and chimera rate compared to MALBAC and DOP-PCR, thus being the preferable method for structural variation detection (Huang et al., 2015b). On the contrary, MALBAC uses quasi-random primers to perform quasi-linear amplification, thus avoiding amplification related bias, reducing allele dropout and increasing coverage uniformity (Huang et al., 2015b; Zhou et al., 2020). For this reason, MALBAC is the better method to perform CNV analysis (Huang et al., 2015b). After WGA, libraries are often sequenced at low coverage (low pass; e.g., from 0,1X coverage) to profile CNVs at a fraction of the price for high coverage. To perform mutational analysis and complex structural variant analysis a higher coverage is needed.
Depending on the type of genomic alteration to be analyzed, e.g., CNVs, Insertion–deletion (indel) or single nucleotide variants (SNV), different metrics are required. For example, CNVs analysis requires high coverage uniformity, while for indels and SNV detection, low rate of amplification errors and allele dropout are particularly critical (Lu et al., 2020).
Clinical applications
Until now, most clinically validated LB tests are based on the assessment of actionable genomic alterations with the aim of supporting decisions about targeted cancer therapy and monitoring treatment response (Ou et al., 2018). However, with the advancement of sequencing platforms that enable more sensitive detection of different classes of mutations, genomic approaches on circulating biomarkers are expanding the range of potential applications in cancer management.
Currently, the most promising application of LB is cancer screening and early detection. Compared to metastatic setting, cancer in early stage releases a low amount of circulating tumor material and thus their detection requires highly sensitive and specific techniques. Moreover, recent reports of somatic mutations accumulating both in solid tissues and in the hematopoietic system as a function of age are reported (Genovese et al., 2014; Alexandrov et al., 2015). In this regard, the company GRAIL, Inc., (https://grail.com) is developing a ctDNA-based multi-cancer screening test using advanced NGS approaches and Machine Learning (ML) (Aravanis et al., 2017). Its first multi-center clinical study, the Circulating Cell-free Genome Atlas (CCGA) will analyze samples from 10,000 participants between cancer and in healthy donors with the aim of identifying a specific genomic signature that distinguishes healthy from sick at an earlier stage of disease. From earliest data, SNV and somatic copy number alteration (SCNA) showed statistically worse sensitivity than WG methylation (Jamshidi et al., 2022). On the contrary, in another study, Manier et al. performed Low-pass WGS (LP-WGS) to study SCNAs in CTCs and ctDNA to uncover the genomic profile of multiple myeloma patients in early stages of disease. Combining the analysis of both analytes, they obtained a higher fraction of patients providing different yet complementary information regarding clonal heterogeneity (Manier et al., 2018).
Different studies exploit genomic approaches for the prognostic validation of circulating biomarkers. A large meta-study suggests that the presence of ctDNA KRAS mutations was associated with shorter OS in NSCLC (Zhang et al., 2019\). In another study, a high number of CTCs (≥20 CTC/10 mL of blood) with mesenchymal phenotype, identified with V600E mutation in BRAF, were correlated with a poor prognosis of the melanoma patients (Tucci et al., 2020). To date, the real strength of LB-based patient management is the ability to track tumor evolution for predicting and monitoring treatment response and resistance mechanisms. Recently, it has been demonstrated that the evaluation of the genomic profile of advanced BC in cfDNA can identify subclonal resistance mutations not appreciable on by single site metastatic tumor biopsies. The cfDNA analyzed with a clinical panel of 74 tumor-associated genes revealed diverse subclonal resistance mutations in specific breast subtype such as HER2 mutations in HER2 + disease, PIK3CA mutations in HR + disease or mutual exclusivity of ESR1 mutations and MAPK pathway alterations in HR + HER2 − BC subtype (Turner et al., 2020; Kingston et al., 2021). With the advent of immunotherapy, many studies have attempted to establish a reliable predictor of response to immune checkpoint blockade. In addition to PD-L1, Tumor mutational burden (TMB) of tumor tissue has been shown to correlate with response to immune checkpoint therapy (Goodman et al., 2017; Klempner et al., 2020; Li et al., 2021). However, some patients lack a high-quality tissue biopsy suitable for biomarker analyses.
To address these challenges, Foundation Medicine has developed a new test based on circulating TMB which has already demonstrated clinical validity. Data published in Nature Medicine demonstrated that the blood-TMB (bTMB) test could predict response to atezolizumab in patients with previously treated NSCLC. Furthermore, the results show that bTMB may be an independent predictor of response compared to PD-L1 expression, as assessed by immunohistochemistry in patients who also had a tissue biopsy available (Gandara et al., 2018). In other work, Georgiadis et al. developed an approach for detection of Microsatellite Instability (MSI) and TMB in the cfDNA of late stage-cancer patients treated with PD-1 blockade. Patients with MSI and TMB-Hight tumors had improved PFS and OS (Georgiadis et al., 2019).
The use of genomic approach on ctDNA to identify resistance alterations to treatment still represents the only validated application in clinical practice. The ctDNA analysis of T790M-mutant NSCLC patients, resistant to first line EGFR Tyrosine Kinase Inhibitor (TKI) therapy, revealed different patterns of genetic alterations in patients with innate versus acquired resistance to Osimertinib (Kato et al., 2021). BRCA reversion mutations detected in cfDNA of platinum-resistant high-grade ovarian carcinoma (OC) patients were associated with decreased clinical benefit from enzyme poly ADP ribose polymerase (PARP) inhibitor rucaparib. Besides, ctDNA analysis identified multiple BRCA reversion mutations, indicating the ability to capture multiclonal heterogeneity of this tumor (Georgiadis et al., 2019). Several key studies have further highlighted the transformative impact of LB for Minimal Residual Disease (MRD) detection, illustrating the future clinical potential and real-world impact (Tie et al., 2016; Schøler et al., 2017; Coombes et al., 2019). A recent paper by Parikh et al. evaluated the feasibility of MRD detection with a plasma ctDNA assay in CC patients with stage I–IV undergoing curative-intent surgery (Parikh et al., 2021). Of 70 patients with eligible plasma for testing, 17 of 70 patients (24%) were ctDNA positive after completion of therapy and 15 of these patients recurred while standard serum carcinoembryonic antigen levels were not predictive of any recurrence.
Transcriptomics
Transcriptomic analysis
Transcriptome analysis (both coding and non-coding RNAs) in LB represent a valuable source of biomarker for precocious identification, stratification and prediction of tumor outcomes (Cuzick et al., 2011). In particular, miRNA, other than their biological role as post-transcriptional regulators, have been found to be dysregulated in several cancer types (Lu et al., 2005; Fernandez-Mercado et al., 2015). miRNAs are protectively released by tumor mass in association with RNA-binding proteins or included in microvesicles and are involved in oncogenesis and tumor progression through their ability to regulate expression of specific genes (Pinzani et al., 2021). Their increased stability and resistance to degradation, compared to mRNA, allow easier analysis of miRNA in body fluids (Mitchell et al., 2008). The analysis of mRNA from plasma is particularly challenging due to its limited stability and quantity. Extracellular mRNA is indeed highly fragmented by ribonucleases. This is reflected in few transcriptome-wide studies (Li et al., 2022) and more targeted approaches intended to capture selected miRNA or mRNAs (Qu et al., 2017). Reverse transcription associated with PCR (e.g.,.ddPCR or qPCR) is actually the method of choice to interrogate circulating tumor RNAs (Pinzani et al., 2021; ruyenaere et al., 2021). For instance, the application is suitable for the analysis of specific cancer mutated genes such as PD-L1 whose expression is evaluated in many tumor types (Ishiba et al., 2018; Pinzani et al., 2021).
A more comprehensive view of circulating and CTC RNAs is provided by NGS-based approaches.
Indeed, it is possible to profile mRNAs using methods that enrich poly(A) RNAs, or selectively reverse transcribe them. In case of non-coding RNAs, sequencing libraries can be obtained from total RNA as well as after enrichment for short transcripts.
To profile the reduced quantity of RNA present in CTCs, several single cell analysis methods have been developed, e.g., Smart-Seq (Picelli et al., 2013), CEL-seq (Hashimshony et al., 2012) and STRT-Seq (Islam et al., 2011) (Table 3). The Smart-seq technology is considered the gold standard for single cell RNA-seq thanks to its higher sensitivity, accuracy and lower costs. In comparison with CEL-seq technology, Smart-seq shows higher sensitivity for the detection of higher number of genes per cell and lower dropout rate (Ziegenhain et al., 2017).
Clinical applications
Over time, it became clear that direct analysis of circulating RNAs could help better understand the evolutionary dynamics of cancer, by providing useful insights for developing personalized approaches for tumor diagnosis and therapy (Supplitt et al., 2021). Several studies demonstrate that miRNAs alone or in combination with other biomarkers improve the diagnostic and prognostic power of different tumors (Chan et al., 2013; Hou et al., 2016; Roman-Canal et al., 2019; Zhang et al., 2022). An interesting study performed cell-free RNA and exosome-RNA analysis on 44 early-stage PDAC patients, identifying 13 upregulated miRNA in PDAC patients compared to healthy controls. A combinatorial analysis of cell-free and exosomal miRNA identifies a particular signature able to detect patients at an early stage of the disease, thus evidencing the diagnostic power of the test (Nakamura et al., 2022).
Furthermore, Sabato and colleagues recently identified specific upregulated and downregulated circulating plasma EV linked to microRNA expression in metastatic melanoma patients compared to healthy donors. They bioinformatically identified 4 pEV-microRNAs able to distinguish metastatic patients from healthy controls with a high diagnostic potential (Sabato et al., 2022). In addition to early diagnosis and prognosis, miRNAs have also been tested for predictive ability to patients’ therapeutic response and cancer resistance in a broad category of tumor types (Hon et al., 2018; Niwa et al., 2019; Tian et al., 2019). In a study of 43 BC patients, the authors identified a clear association of four pEV miRNA with a pathological response to neoadjuvant therapy while no correlation between the miRNA transcriptional profile performed on plasma compared to tissue biopsy one (Baldasici et al., 2022). In a recent study, exosomal miRNAs were shown to participate in osimertinib resistance through abnormal activation of the RAS-MAPK and PI3K pathways. Particularly the expression of miR-184 and miR-3913-5p in the peripheral blood of NSCLC patients could be used as biomarkers to indicate osimertinib resistance (Giallombardo et al., 2016). RNA-seq of single prostate CTCs from patients resistant to Androgen Receptor (AR) inhibitor showed activation of noncanonical Wnt signaling and low glucocorticoid receptor expression compared with untreated cases (Miyamoto et al., 2015). Furthermore, the transcriptomic profile of CTCs was analyzed to identify a specific subpopulation involved in the metastatic spread and organotropism of different cancer types (Giuliano et al., 2014; Castro-Giner and Aceto, 2020; Schuster et al., 2021; Yu et al., 2021).
It has been shown that CTC-derived cell cultures and xenograft models could more accurately define cell clones with an initial metastatic long-term potential (Vishnoi et al., 2019; Brungs et al., 2020; Felici et al., 2022). Analyzing CTCs isolated from breast patients, Boral et al. identified a specific signature associated with brain metastases (Boral et al., 2017). An interesting work studied the spatiotemporal transcriptional dynamics of CTCs during hematogenous dissemination in patients affected by hepatocellular carcinoma. The authors identified specific CTC phenotypes in different vascular compartments involved in liver tumor dissemination. They observed an overexpression of chemokine CCL5 involved in CTC immune escape and metastatic seeding mediated by recruitment of regulatory T cells (Tregs). These findings open the way to directly block CTC dissemination through the inhibition of CCL5, with the possibility to extend the target to other driver genes involved in immune evasion (Sun et al., 2021).
Elsewhere, CTCs isolated from metastatic gastric cancer (GC) patients presented upregulation of genes involved in platelet adhesion. The transcriptomic profile of CTCs demonstrated the important contribution of platelets in EMT progression and acquisition of chemoresistance (Negishi et al., 2022). Taken together, these results represent an important step in developing effective strategies against CTCs to prevent cancer dissemination and immune evasion.
Epigenomics
Epigenomic analysis
Epigenetic alterations include DNA methylation, histone modification and chromatin accessibility to transcription factors. By determining gene expression patterns, those modifications shape cellular phenotype. Alterations resulting in an overly permissive or overly restrictive epigenetic regulation can lead to the generation of malignant subclones responsible for tumor progression and therapeutic resistance (Ponnusamy et al., 2019; Fujimura et al., 2020; Chen and Yan, 2021). For this reason, epigenetic alterations have been proposed as potential diagnostic, prognostic and predictive biomarkers in cancer management (Heyn and Esteller, 2012). Detection of epigenetic modifications of cfDNA and CTC using LB can reveal the epigenetic aberration of cancer.
DNA methylation is the most studied epigenetic modification. Silencing of tumor suppressor genes by extensive promoter hypermethylation has been observed in several cancers (Greger et al., 1989; Herman and Baylin, 2003; Chen et al., 2014). DNA methylation analysis can be performed using three experimental approaches: bisulfite conversion, digestion with methylation-sensitive restriction enzymes and affinity enrichment (Table 4) (Galardi et al., 2020).
The bisulfite conversion is the gold standard in 5-metil-cytosine (5 mC) detection and it’s based on chemical modification of unmethylated cytosines. Comparison of bisulfite-converted and reference unconverted sequences allows the identification of methylated cytosine with a single base resolution. Bisulfite conversion can be exploited for targeted analysis, when followed by Methylation-Specific PCR (MSP) or genome-wide characterization by means of Whole-Genome Bisulfite Sequencing (WGBS-seq), Reduced-Representation Bisulfite Sequencing (RRBS-seq) (Meissner et al., 2005; Gu et al., 2011) methylation arrays and Methylated CpG Tandems Amplification and Sequencing (MCTA-seq). Although WGBS offers a comprehensive profiling of GpC methylation status at a single base resolution, it is not applicable to large cohort analysis due to high costs of whole genome sequencing. RRBS instead profiles approx. 4 million of cytosines in CpG dense regions for a fraction of WGBS cost (Gu et al., 2011). Human methylation arrays such as Infinium MethylationEPIC BeadChip Kit (Illumina) instead probes 850,000 functionally relevant methylation sites at single-nucleotide resolution but requires a quite high input material (200–300 ng), thus reducing applicability to liquid biopsy studies. To overcome this limitation, pooling approaches to methylation array strategy (Gallego-Fabrega et al., 2015) have been applied to profile cfDNA of CC patients (Gallardo-Gómez et al., 2018). To apply bisulfite analysis on CTCs, single cell-RRBS (Guo et al., 2015), PCR-based and target bisulfite sequencing methods can be exploited.
As a general limitation associated with use of bisulfite is the damaging effect of chemical treatment on DNA, which is associated with material loss and requirement of moderately high starting material (Grunau et al., 2001), bisulfite-free methods for epigenomic profiling have been recently developed. For example, Methylation Restriction Enzymes method (MREs) uses methylation sensitive restriction enzymes that recognize and cut only unmethylated DNA. Quantitative analysis of methylation status after digestion can be performed using various technologies based on real-time PCR (Hashimoto et al., 2007; Pulverer et al., 2021), sequencing (Oda et al., 2009) and microarray (Hatt et al., 2015).
Other bisulfite-free assays are based on affinity enrichment either using anti-methylcytosine antibodies (meDIP-seq) (Taiwo et al., 2012) and low input, cfDNA compatible cfMeDIP-seq (Shen et al., 2019) or Methyl-Binding Domain (MBD) of methyl-CpG binding proteins to capture the methylated genomic regions (Serre et al., 2010) optimized for cfDNA (Huang et al., 2022b).
In addition to 5mC, 5-hydroxymethylcytosine (5hmC) has recently proven to be a regulatory modification associated with transcriptional activation (Song et al., 2011) and several studies identified 5hmC as putative marker in cancer (Vasanthakumar and Godley, 2015) detectable in ctDNA samples (Song et al., 2017). Standard bisulfite methods cannot discriminate between cytosine modifications, and enrichment methods often require high input material. A recently developed technology called Enzymatic Methyl-seq (EM-seq) allows the identification of both 5mC and 5hmC starting from picograms of material exploiting two sequential enzymatic reactions (Vaisvila et al., 2021). Another innovative method for studying genome-wide 5hmC is 5hmC-Seal (Song et al., 2011) which uses a selective chemical labeling on low levels of DNA with high sensitivity.
Finally, a recently developed technological approach for detecting cancer-specific methylation and cancer-associated fragmentation signatures, without disrupting bisulfite conversion, is native Nanopore sequencing (Katsman et al., 2022; Lau et al., 2022). In this assay, PCR-free libraries are read at a single molecule level by passing through nanopores generating an alteration of electric signal which is recorded by the sequencer. This alteration is specific to the DNA modification and is different between methylated and unmethylated CpGs. By abolishing PCR, the biases in molecule quantification are strongly reduced. With this approach it is possible to cover many informative cfDNA CpG sites, even with a shallow coverage, still obtaining significant discrimination on aberrant methylation state (Lau et al., 2022) and retain cell-of-origin information (Katsman et al., 2022).
Aside from DNA methylation, chromatin accessibility and histone modifications are becoming increasingly studied in parallel with the development of new technologies for their detection in the context of liquid biopsy. Open chromatin regions undergo higher fragmentation than compacted portions which are protected by nucleosomes. Those accessible regions are associated with transcription and have been associated with a typical fragmentation pattern related to the tissue-of-origin (Snyder et al., 2016; Moss et al., 2018).
As reference chromatin accessibility profiles for many cancer types are now available (Corces et al., 2018), computational identification of key disease features to be targeted in liquid biopsies is possible, allowing computational imputation of cfDNA tissue derivation (Cristiano et al., 2019; Sun et al., 2019). That information can be integrated in panel design to prioritize coverage of marker regions with highest detection probability (Taklifi et al., 2022).
It has been observed that cancer-derived cfDNAbe more variable in length than cfDNA from non-cancer cells, due to the altered chromatin accessibility and associated nucleosome positioning. This feature of cfDNA has been exploited in the combined detection of fragmentation patterns and genetic markers resulting in improved sensitivity in cfDNA analysis (Peneder et al., 2021). This approach can additionally benefit from enrichment in short (50–150 bp) plasma derived fragments in combination with LP-WGS (Mouliere et al., 2018).
Cell-free chromatin in plasma can be further analyzed to detect nucleosomes and associated Post-Translational Modifications (PTM). Immunoprecipitation of cell-free fragments followed by low-depth NGS sequencing (cfChIP-seq) has been shown to inform about cell type and program-specific expression patterns (Table 4) (Sadeh et al., 2021). In CC patients, plasma detected levels of H3K9me3 and LINE, detected by immunoprecipitation followed by sequencing, were found to be significatively associated with disease (Gezer et al., 2013). Finally, a database of nucleosome positioning in vivo and of cell-free DNA nucleosomes (NucPosDB) has been recently released (Shtumpf et al., 2022). It has been built from published in vivo nucleosome positioning datasets together with datasets of sequenced cfDNA. This type of data represents an invaluable resource for model training especially for association of cfDNA nucleosomes to the tissue and cell of origin, and to set comparison between different conditions.
Clinical application
Alteration of epigenetic markers in liquid biopsy has shown to be clinically meaningful although it is still affected by limited availability of standardized tests (Palanca-Ballester et al., 2021).
Most promising diagnostic applications are based on cfDNA methylation analysis, especially with custom panels, which better balance sensitivity and test cost. The PanSeer assay was developed for interrogation of cancer-specific DNA methylation signatures from peripheral blood (Chen et al., 2020). This assay targets more than 10,000 CpG sites across the genome, panel is tissue-of-origin (TOO) independent and was able to detect cancer-related aberration up to 5 years before in asymptomatic patients. Similarly, a 100,000 regions classifier based on methylation status was developed by the TCGA consortium to provide for cancer detection and TOO identification (Liu et al., 2020) in >50 cancer types. This assay has been validated on a case-control cohort and showed a >99% specificity which increases with cancer stage, and a TOO detection accurate in 90% of cases. Furthermore, recent studies have shown that the cfDNA hydroxymethylation pattern is associated with cancer type and can change in a stage-dependent manner (Song et al., 2017).
Beside those pan-cancer panels, several tumor-specific methylation patterns have been identified such as in BC in which the analysis of cfDNA methylation signature improves early detection of BC compared to mammography (Zhang et al., 2021). Whereas in glioma, the mere identification of the methylation status of the MGMT promoter in CSF rather than plasma provides a promising clinical tool for early diagnosis (Wang et al., 2015). Such targeted approach benefit from improved sensitivity when integrated with other cfDNA parameters such as fragment size analysis (Shin et al., 2022) and/or cfDNA plasma concentration to differentially methylated regions analysis (Panagopoulou et al., 2019; Bagley et al., 2021). A very recent study proposed a hydroxymethylation classifier for early stages CC in cfDNA plasma which can be expanded to DNA fragment size and abundance to increase sensitivity (Walker et al., 2022). Integration of haplotype blocks methylation analysis represents an add-on to single CpG loci analysis. Those are genomic segments of strictly related CpG sites whose methylation status is informative of tumor load and tissue-of-origin mapping (Guo et al., 2017). A haplotype load metric has been correlated with tumor load and tissue-of-origin mapping in cfDNA of lung or CC patients (Guo et al., 2017) thus representing a promising analysis tool for deconvolution and disease identification.
Epigenetic markers monitoring has also proven useful for outcome prediction and longitudinal monitoring of minimal residual disease in different cancer types. Interrogation of a panel of ctDNA methylation markers in post-surgery colon cancer patients has been shown to be predictive of recurrence with good accuracy (Jin et al., 2021). Similarly, methylation levels of specific marker USP44 has been associated with disease-free survival in prostate adenocarcinoma patients (Londra et al., 2021). Interestingly, WGBS approach has been used to assess the methylation profile of single CTCs and CTC clusters in BC patients, revealing specific hypomethylation of proliferation and stemness regulators binding sites only in CTC clusters. Moreover, PFS analysis showed that those regions hypomethylated in CTC clusters are associated with poor prognosis (Gkountela et al., 2019).
Methylation markers are not only significant for prognosis, but also for predicting therapeutic response. Indeed, in patients with NSCLC, increased RARB2 methylation in cfDNA after chemotherapy and tumor resection was associated with disease recurrence at 9 months (Ponomaryova et al., 2013) as well as decreased levels of plasma SHOX2 methylation was associated with response to platinum-based chemo/radiotherapy (Schmidt et al., 2015). In metastatic CRPC, methylation changes in specific genes were predictive of response to AR treatment (Peter et al., 2020; Peter et al., 2022). In early stage NSCLC patients, Markou et al. recently showed correlation between relapse incidence and promoter methylation status of at least one of a five selected genes panel (APC, RASSFIA1, FOXA1, SLFN11, SHOX2) in CTC or plasma-cfDNA (Markou et al., 2022). In the analyzed cohort there was no correlation between CTCs and primary tumor methylation profile.
Paired analysis of another nine-gene promoter methylation panel in ctDNA and CTC of a different NSCLC cohort, analyzed before osimertinib treatment and during progression, revealed inconsistency between CTC and ctDNA profiles. However, in both cases Progression Disease (PD) was associated with increased methylation levels with respect to baseline samples. Kaplan-Meier analysis revealed correlation between earlier PD and methylation status of at least one panel gene (Ntzifa et al., 2021). As those data were obtained on small CTC bulks by real-time methylation specific PCR assays, this can suggest the relevance of performing additional single cell analysis to deconvolve potential heterogeneity.
Proteomics
Proteomic analysis
Proteomic analysis of LB represents a minimally invasive and repeatable way to accomplish a broad range of milestones such as: identification of targets to direct new treatments, development and validation of biomarkers to allow early detection of diseases, design of proteomic signatures as predictive models for cancer diagnosis and prognosis (Kim et al., 2016). Using the appropriate technique to investigate proteomic signatures is becoming as important as studying more than one specimen from the same patient, to achieve a deeper level of molecular complexity.
A traditional method to validate protein biomarkers is using enzyme linked immunosorbent assays (ELISA), but this approach is time consuming, expensive and depends on the availability of existent antibodies pairs for every target protein. A method which does not rely on existing validated antibodies is MS (Kim et al., 2016). To analyze complex protein mixtures ensuring high sensitivity, MS is often coupled with gas or liquid chromatography, capillary electrophoresis, or Ultra-Performance Liquid Chromatography (UPLC). Based on the identification of the ratio of mass to charge (m/z) of a molecule and/or its fragments, the initial chromatographic step increases the isobaric species’ resolution, gaining a better detection of less abundant proteins. However, the use of chromatography increases the processing times and therefore limits the number of samples to be tested daily (Ardrey, 2003). When applicable, biofluids could be injected directly in the mass spectrometer: this method, called flow-injection MS, even if characterized by reduced sensitivity, could be advantageous for initial screenings aimed at having a general picture of the total protein amount of the sample (Sarvin et al., 2020).
A complementary analysis of both plasma and urine from the same patient allows a thorough examination since urine is a filtrate product of blood (Chinello et al., 2019). As a recent work demonstrates, label-free liquid chromatography−tandem mass spectrometry (LC−MS/MS) approach, through which liquid chromatography was coupled to tandem MS to fragment selected peptides, was used to compare soluble protein signatures of urine and plasma from patients affected by renal cell carcinoma. Some differentially expressed proteins were shared between the two biofluids such as immunoglobulin, complement factors, activators of the complement cascade, modulators of the acute response, innate immune system, and platelet degranulation. However, urine and blood carried specific biofluid functional signatures (Chinello et al., 2019).
Although extremely informative, MS results are often validated using more conventional techniques such as ELISA and Western Blotting (WB), which can be still useful to identify a few target proteins in low abundance, but without giving information about the protein expression levels (Lequin, 2005; Kurien and Scofield, 2015). Nevertheless, even these techniques are evolving into more precise and accurate methods to quantify circulating proteins and interrogate proteomic signatures at a single-cell level: multiplex ELISA and single-cell (sc)-WB (scWB) can serve as a model (Velez et al., 2021).
Differently to standard ELISA, multiplex ELISA can detect and process up to 1,000 human proteins, allowing us to develop a multiplex bioassay and add more candidate proteins into a panel of interest (Song et al., 2019). However the performance of an antibody-based approach might be affected by several variables such as abundance of the protein of interest, affinity and specificity of the capture antibody (Fu et al., 2010).
For phenotyping characterization of CTCs, scWB can be used. Among the novel single-cell immunoassays, scWB can potentially allow multiplex detection of surface, intracellular and intranuclear proteins simultaneously. Into ad hoc microwells all steps of WB are performed, minimizing cell loss and maximizing protein concentration (Sinkala et al., 2017). The initial electrophoretic separation reduces the antibody cross-reactivity and increases assay specificity, while antigen immobilization on the detection membrane is associated with molecular size standards. Despite the enormous potential of this method, initial steps of CTCs isolation, enrichment and transfer into micro wells can be challenging and opportune instruments are required (Abdulla et al., 2022).
It has been a few years already that single-cell proteome analysis is performed using mass cytometry (MC), which comes from the fusion of two technologies, FC and MS. MC allows in-depth analysis of homogenous cell populations, providing measurement of over 40 parameters at single cell resolution (Spitzer and Nolan, 2016) from a limited sample volume. Both surface and intracellular proteins can be targeted using FC features and antibodies against selected targets. Then, after cell nebulization and ion cloud filtration using a quadrupole, droplets can enter into the mass cytometer and enriched heavy metal reporter ions are finally quantified by time-of-flight. On this basis, a single-cell measurement can be achieved, even for rare populations, enabling the analysis of heterogeneous complex cellular systems (Frei et al., 2016). However, despite the enormous potential of this technique, this kind of analysis does not allow live cell recovery, and measurement of low expressed molecular features may fail due to the paucity of available sensitive fluorophores and the background noise.
Noteworthy, a technology that could be used for rapidly investigating surface protein expression in CTCs is the DEPArray system (Menarini Silicon Biosystems, S.p.A., Italy). Already known for its ability to isolate single, viable rare cells using dielectrophoretic principles combined with an image-based selection, DEPArray technology has gradually gained clinical relevance during the lastade (Bulfoni et al., 2016; Boral et al., 2017).
Clinical application
It has been decades that the diagnostic, prognostic and treatment monitoring value of serum and urine tumor markers have been proved. Serum PSA levels for diagnosing prostate cancer in men, cancer antigen 15-3 (CA15-3) for breast cancer and cancer antigen 19-9 (CA19-9) for pancreatic cancer are just a few examples of how medicine has applied soluble protein dosage to diagnose cancer to date (De Angelis et al., 2007; Sturgeon et al., 2009). However, due to their reduced sensitivity and lack of cancer specificity, tissue biopsy is regularly performed to confirm the definitive diagnosis, avoiding incorrect interpretations associated with benign conditions.
Plasma and urine have been the most characterized biological source of data by far, however some biomarkers are massively diluted and alternative more concentrated clinical samples have been investigated recently. Among the broad set of biological fluids considered valuable sources for biomarker discovery, nipple aspirate fluid (NAF) is gaining an emerging role for BC screening in young women at high risk. Indeed, not only mammography has low specificity in differentiating between benign and malignant growth, but also in this scenario this test lacks accuracy due to young breast density. Sadr-ul Shaheed and collaborators (Shaheed et al., 2017) investigated the protein-rich composition of NAF using MS techniques identifying 332 new biomarkers unique to NAF. Using 2D-LC/MS, NAF proteome from BC patients and healthy volunteers was analyzed to prove the potential diagnostic value of the above cited procedure. NAF proteome already has the potential to give us plenty of data about breast health, especially for its highly rich composition of biological materials (Chan et al., 2016; Do Canto et al., 2016; Shidfar et al., 2016).
Similarly, Gabriel Vales et al. have recently validated 20 proteins using quantitative multiplex ELISA array starting from vitreous samples while looking for uveal melanoma biomarkers associated with metastatic risk (Velez et al., 2021). In this study, for the first time in vitreous, this technique was used to investigate such a large set of proteins, also confirming previous gene expression analysis. Sampling and analyzing circulating tumor markers in these fluids in a minimally invasive way is essential when tumors are critically located.
If soluble proteins’ dosage is effortless because they are easily accessible, we cannot state the same for surface, intracellular and particles’ proteins. Over the past decade, EV and particles EV (EVP), which include small exosomes, large exosomes and exomeres (Zhang and Lyden, 2019) have gained increasing importance in cancer detection from LB and beyond, since they reflect the systemic effects of cancer. Recently, the prognostic and functional importance of tumor-derived exosome’s proteins has been proved in tumor progression, immune regulation and therapy guidance (Costa-Silva et al., 2015; Hoshino et al., 2015; Chen et al., 2017; Rodrigues et al., 2019).
A recent multicentric study has elegantly investigated the proteomic profile of EVPs in 426 human samples from tissues, plasma and other bodily fluids, related to adult (pancreatic, lung, breast, and colorectal carcinomas and melanoma) and pediatric cancers (neuroblastoma and osteosarcoma) (Hoshino et al., 2020). Using MS combined with ML approach, they identified pan-EVP markers, demonstrating that tumor-associated EVP proteins are reliable biomarkers for early-stage cancer detection and determination of uncertain primary tumor types, reaching 95% sensitivity and 90% specificity.
As for many exploratory studies, Hoshino’s research group interrogated publicly available protein databases to find proteome quantitative data and then make a list of tumor specific EVP proteins of interest (Omenn et al., 2005; De Angelis et al., 2007; Mathivanan and Simpson, 2009; Kalra et al., 2012; Hoshino et al., 2015; Chan et al., 2016; Do Canto et al., 2016; Shidfar et al., 2016; Chen et al., 2017; Shaheed et al., 2017; Rodrigues et al., 2019; Zhang and Lyden, 2019; Hoshino et al., 2020). After having selected conventional and newly identified markers, they employed a targeted MS-approach, using a designed time-scheduled parallel reaction monitoring (PRM) method, to quantify tissue-specific tumor-derived EVP proteins in patients. PRM is particularly suitable for quantifying tens to hundreds of targeted proteins in complex matrices with attomole-level limit of detection. Tumor-associated EVP protein profiles could serve as a LB tool to detect cancer and discriminate among heterogeneous cancer types, as also confirmed by EVP profiles of tissue biopsies (i.e., lymph nodes).
Similarly, Yunee Kim and his collaborators used targeted proteomics combined with computational biology to define proteomic signatures for prostate cancer from urines collected by men with extra prostatic and organ-confined prostate cancer, in a 74-patient cohort. Since protein signatures, rather than individual soluble proteins, allow us to accurately discriminate patient groups, they tried to distinguish pT2 stage from pT3 stage tumors, before radical prostatectomy, for potentially modifying and personalizing patient treatment (Kim et al., 2016). Selected Reaction Monitoring Mass Spectrometry (SRM-MS) allows targeted quantification of a large number of proteins in a selective and sensitive way (Kim et al., 2016). A ready-to-use prognostic signature could help in the clinical decisions-making process leading to appropriate treatments, improving survival. If to date CTCs enumeration alone has been a new method to diagnose cancer, monitoring surface and intracellular protein expression in CTCs, together with transcriptomic and genomic analyses, is going to be the next step to enhance prognostic decisions, classify patients in low and high-risk groups, and better guide treatments. scWB and MC could both satisfy this clinical need (Stelzer et al., 2021). Indeed, E. Sinkala and his collaborators, within a pilot study, used scWB to investigate the expression of eight surface and intracellular proteins in CTCs from metastatic BC patients, to assess individual response to therapy (Sinkala et al., 2017). Since therapies that target proteins are increasingly rising, monitoring protein expression in CTCs isolated from peripheral peripheral blood may guide therapeutic selection in the near future.
Recently, FC-based technologies have been used and adapted to detect multiple markers at a single cell level too. In this regard, CTCs from osteosarcoma patient blood samples were characterized by Shulin Li’s research group a couple of years ago (Batth et al., 2020). After having isolated CTCs for their positivity to vimentin and negativity to CD45, a multiplex labeling using antibodies conjugated to metal ions revealed the presence of 18 different markers simultaneously. After protein quantification, they used a tailored bioinformatic analysis to obtain unique patient-related protein signatures containing information about active signaling pathways, which could help us to predict future tumor behavior and guide treatment choice.
Metabolomics
Metabolomic analysis
Since the discovery of the famous Warburg effect (Warburg et al., 1927), several studies have shown that there is a broad spectrum of bioenergetic and metabolic phenotypes supporting cell proliferation, metastasis and resistance to cancer therapies. Oncometabolites are small endogenous and exogenous molecules present in tissues and biofluids, accumulated by altered metabolic pathways during malignant transformation (Khatami et al., 2019). They originate in the tumor microenvironment to create the optimal growing conditions for the tumor (Elia and Haigis, 2021; Hofer et al., 2021).
Metabolomics focuses on the profiling of small intracellular or free metabolites (≤1,500 Daltons) in bodily fluids including blood, urine, CSF and saliva (Han et al., 2021). Metabolites of interest can be detected using Nuclear Magnetic Resonance (NMR) (mostly proton NMR, H-NMR) and MS, in association with different separation methods (Fiehn, 2016; Kang et al., 2018; Lane et al., 2019; Sinclair and Dudley, 2019). Based on chemical properties of specific atoms in a molecule, NMR can be used with biological samples without prior processing, and the unaltered starting material can be re-used for additional investigations. However, compared to MS, a lower number of metabolites, with lower sensitivity (micromolar vs. nanomolar) can be identified using NMR, and for this reason MS still represents a gold standard.
Clinical application
Metabolomics is mainly used for early cancer detection and biomarkers discovery (Schmidt et al., 2021). Uchiyama et al. showed that benzoic acid has a high diagnostic capacity in CRC and identified a correlation between CRC stages and upregulation/downregulation of different serum metabolites (Uchiyama et al., 2017). Another study based on proton NMR revealed that fecal metabolomic fingerprinting can be used as an early diagnostic tool in CC patients (Uchiyama et al., 2017). In a wide-scale metabolic investigation of plasma samples from OC patients, Ke et al. demonstrated that metabolic signatures can facilitate early diagnosis of OC, helping us to discriminate early from late stages (Ke et al., 2015).
Interesting results have been obtained from studies of metabolomic composition of urine(u)-EV of prostate cancer patients (Clos-Garcia et al., 2018). According to Puhka et al., patients before prostatectomy presented a different uEV metabolome content compared to those after prostatectomy and healthy controls (Puhka et al., 2017). A different study reported that approximately 76 metabolites were differentially expressed between patients with prostate cancer and patients with benign prostatic hyperplasia (BPH) some of which were among the metabolic alterations reported in PCa (Xu et al., 2021).
Increased evidence has demonstrated the influence of microbiota in human malignancies including cancer (ich-Poore et al., 2021). Microbiome metabolites can influence the tumor microenvironment by regulating different aspects of carcinogenesis including proliferation, angiogenesis, inflammation and metastasis (Rossi et al., 2020). The connection between serum metabolome and the intestinal microbiome in patients with lung cancer at different stages was recently investigated. As the disease progressed, the L-valine and Lachnospiraceae_UCG006reased suggesting L-valine is a potential marker for lung cancer diagnosis (Chen et al., 2022). A different research group identified specific microbiome-associated metabolites in CRC patients analyzing their fecal microbiome (Yang et al., 2019). They found proteobacteria, fusobacteria, high concentrations of polyamines (cadaverine and putrescine), amino acids (Pro, Glu) and urea in patients compared to healthy volunteers in which, on the contrary, sugars and fatty acids (Yang et al., 2019) were abundant.
In conclusion, implementation of analytical techniques and validation of algorithms for analyzing metabolomics data are still needed to let metabolomics take its rightful place among precision oncology omics.
Bioinformatics tools for liquid biopsy
The reduced amount of target molecules in LB affects the capability to detect low-frequency genomic variations. To increase detection sensitivity, a panel of genes rather than the whole genome can be sequenced numerous times, even though this leads to higher false positivity risk. To solve this issue, advanced bioinformatic tools or machine learning algorithms are needed to reduce false positive results and eliminate background noise.
Tools such as the popular IchorCNA (Adalsteinsson et al., 2017), have been designed to estimate a low coverage plasma sample tumor fraction in an ultra LP-WGS scenario (0.1X) and help the operator decides whether enough material is available for a WES comprehensive clonal analysis. Following, in a recent paper, Zviran et al. (2020) stick to WGS and proposed replacing depth of sequencing with breath for sensitive detection of low-burden cancer, by increasing the number of detectable sites (SNVs) performing WGS at an average 35X. The WGS approach enabling effective integration across orthogonal data dimensions such as SNV and CNV allows clinical application to a wide range of tumor types that have either high mutation load or aneuploidy (Taylor et al., 2018). Unfortunately, if the technique provides sensitive detection of ctDNA it does also provide limited confidence in the sensitivity to detect any individual site so, target sequencing remains the election choice to identify driver mutational events.
Similar approaches can be used to detect CTC genome alterations. Once CTC are isolated from biological fluids their DNA is amplified and sequenced by LP or ultra LP-WGS. At a coverage of 1X or 0.1X heterogeneity that might arise due to small genomic aberrations such as SNVs and short indels will be missed. Several computational tools are available for CNV analysis using bulk sequencing data including CNVkit (Talevich et al., 2016), ControlFreec (Boeva et al., 2012), ASCAT (Van Loo et al., 2010) and Sequenza (Favero et al., 2015), just to name some, and many groups rely on these for the analysis of single cell data (Liu et al., 2019a; Pailler et al., 2019; Oulhen et al., 2021). Among the few open-source tools specifically designed for CNV calling in single cells we can list the cloud-based Ginkgo (Garvin et al., 2015) which is developed for LP-WGS data, making the CNV analysis procedure user-friendly, including for those with limited bioinformatics experience.
When the goal is to analyze large datasets, more computationally efficient strategies are required. AneuFinder (Bakker et al., 2016) and SCOPE (Wang et al., 2020) are two R-Bioconductor packages developed to explore tumor single cell data to identify evidence for copy number variations in WGS samples while among the python packages we can highlight SCNV (Wang et al., 2019), baseqCNV (Fu et al., 2019), SCCNV (Dong et al., 2020), SCICoNE (Kuipers et al., 2020) and CHISEL (Zaccaria and Raphael, 2021). If genomic alterations’ analysis is influenced by the scarcity of the starting material, investigations on RNA must be performed also taking into account its instability in biological fluids (Cheng et al., 2019; Vaisvila et al., 2021). Therefore, even if the bioinformatics pipelines currently used for CTC derived bulk and scRNAseq sequencing data are the same used for other types of transcriptomic data (Satija et al., 2015; Wolf et al., 2018) analyses of these samples require additional quality evaluation and preprocessing.
Recent research suggests that cfDNA fragmentation patterns can provide additional information beyond the genetic analysis of somatic mutations and copy-number abnormalities. Indeed, DNA fragmentation from dying tumor cells seems not randomly distributed but also determined by the DNA sequence: it appears to reflect the chromatin structure and epigenetic states of the cells, from which DNA fragments derive (Snyder et al., 2016; Cristiano et al., 2019; Sun et al., 2019). Peneder et al. (2021), in their inspiring paper, identified a specific epigenetic signature among fragmentation patterns in tumor DNA isolated from the blood of patients with Ewing Sarcoma. The authors introduced a new algorithm for detecting ctDNA based on cancer-specific chromatin signatures and combined several fragmentation-based metrics into an integrated machine-learning classifier that exploits widespread epigenetic deregulation and is tailored to cancers with few genetic lesions such as pediatric tumors.
Among the positive outcomes of multi-omic investigations there is the construction of useful databases, built to help researchers delineate which proteins are expressed in specific tissues in physiological and pathological conditions. Examples are computational resources such as the Human Protein Atlas (https://www.proteinatlas.org): a repository of information about mRNA and protein expression across several healthy tissues and cancers, and the Genotype-Tissue Expression (GTEx) project (https://gtexportal.org).
Several ML frameworks have been developed to characterize tumor biomarkers in an unbiased, automated and reproducible manner (Svensson et al., 2015; Ko et al., 2018), mostly purely based on extracted-features traditional methods. Recently, Zeune et al. (2020) showed how with a more complex, “black box” approach combining autoencoding convolutional neural networks (CNN) with advanced visualization techniques they were able to segregate 164 metastatic BC patients based on favorable and unfavorable prognosis starting from CellSearch imaging data, proving the deep learning method was at least as good as manual CTC count in cell classification. Automatic, objective cell classification with a CNN-based image processing path was followed by several other groups (He et al., 2020; Guo et al., 2022) highlighting the importance for research centers, and hospitals to work with instruments producing accessible, good quality and properly formatted CTC imaging data for reliable ML-based predictions.
Challenges and perspectives
Although there is great potential in LB’s biomarkers, some biological and technical issues must be solved before entering into medical practice. Several completed and ongoing are going to establish whether these biomarkers can be fully adopted in clinicalisions (Table 5). However, it is not yet clear whether liquid biomarkers can capture the entire tumor heterogeneity at the time of analysis, especially in metastatic patients in which distinguishing the contribution of each site is currently not feasible. Detection and characterization of circulating tumor components, especially in the early setting, presents many difficulties due to their low amounts in biological fluids. These unresolved aspectsgenerate inconclusive and conflicting data with a high rate of false positives (overdiagnosis) and/or false negatives (underdiagnosis).
One possible solution that has been recently proposed is the use of a multi-omic, multi-analyte LB which could offer a high-resolution snapshot of cancer complexity (Figure 2). Indeed, the identification of a single biomarker able to reconcile biological and technical needs represents a lost cause. Clinical sensitivity and specificity of CTC and ctDNA tests can be improved when coupled with protein-based markers. First evidence is the prospective study of Imperiale et al. which compares a multitarget stool DNA test with a fecal immunochemical test (FIT) to predict the risk of developing CC. The stool test combines identification of KRAS mutation and aberrant NDRG4 and BMP3 methylation with fecal hemoglobin dosage. DNA test demonstrated to have higher sensitivity than FIT assay for both advanced precancerous lesions and colorectal cancer, although with lower specificity (Imperiale et al., 2014).
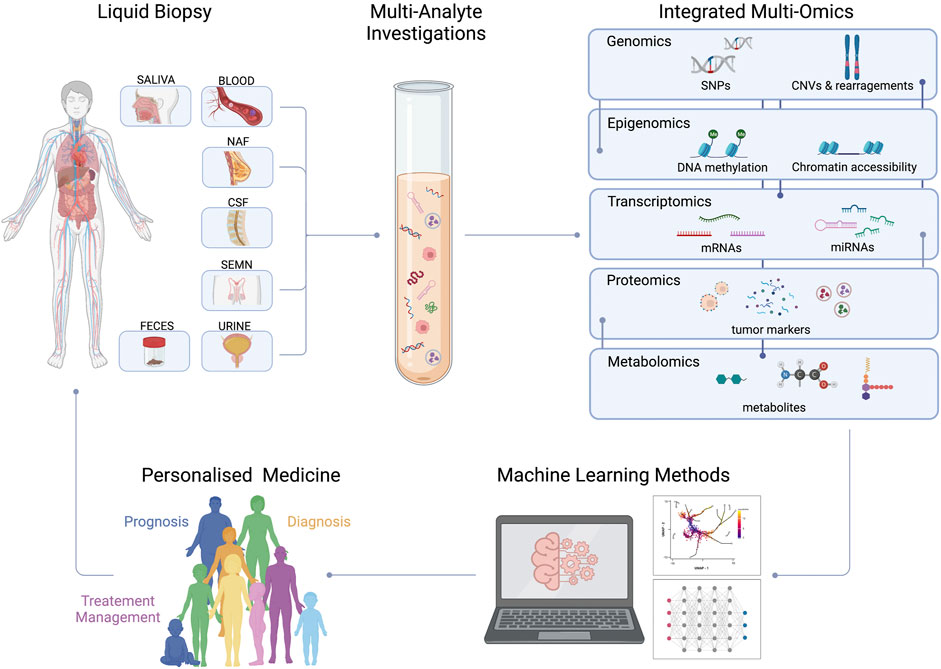
FIGURE 2. Integrated multi-omic approach in liquid biopsy. An integrated multi-omic approach is going to be used investigating single or multiple analytes from different fluids of the same patient. Artificial intelligence will be educated using the enormous amount of information that has been created. From the resulting predictive models can be originated personalized medical decisions (NAF, Nipple Aspirate Fluid; CSF,Cerebrospinal Fluid; SEMN, Seminal Fluid; CNV, Copy Number Variations; SNPs, Single Nucleotide Polymorphisms).
Cohen et al. developed a multi-analyte blood test, called CancerSEEK that simultaneously evaluates eight protein biomarkers and tumor-specific mutations in circulating DNA of common human cancer types (Cohen et al., 2018). The assay had a sensitivity of 69%–98% for five cancers for which no screening tests are available for high-risk individuals while specificity was greater than 99% with only 7 of 812 healthy controls testing positive. Moreover, without any clinical information on patients, the test identified the single anatomic site in a median of 63% of the patients (Cohen et al., 2018). Interesting preliminary data were obtained with a multianalyte panel based on analysis of EV RNA, cfDNA and dosage of CA19-9 protein in PDAC patients (Yang et al., 2020). Combining different blood-based biomarkers, authors distinguished patients with PDAC patients from healthy controls with 92% accuracy, 95% specificity and 88% sensitivity. This model could improve detection of occult metastases not visible with conventional imaging at baseline and only discovered intraoperatively or after 4 months of baseline blood draw (Yang et al., 2020).
On the one hand, multi omic analysis seeks to fullyipher the complexity of cancer heterogeneity, in contrast it generates a huge amount of data. Omic data can “apparently” be very different from each other as numerous biological and non-biological variables can influence their production. The choice of patients, the type of protocol adopted to perform the analysis, or the personal experience of the operator can greatly affect the final data. Over the years different repositories like TCGA (https://portal.gdc.cancer.gov/) and ICGA (https://dcc.icgc.org/) have been created to collect all omics data in an orderly manner. Since there is an urgent need to connect multi-level information concerning molecular signatures and the phenotypic manifestation of different cancer types, AI and ML approaches have been proposed to transform big-sized complex data into evidence-based medicalisions. Although there is growing evidence demonstrating the potential of ML to improve the performance of various LB tests, their integration into the clinical workflow represents the main challenge of the upcoming years (Macaulay et al., 2015).
Author contributions
GDS and VR collected the related literature and wrote the article. SEF and AM contributed to the original writing. DL critically revised the article. CF and FG conceptualized and supervised the manuscript writing. All authors went through the final manuscript draft and approved it.
Acknowledgments
The Authors are grateful to Prof. Giovanni Tonon for critical reading and helpful comments during the manuscript revision. Figures 1, 2 were created with BioRender.com.
Conflict of interest
The authors declare that the research was conducted in the absence of any commercial or financial relationships that could be construed as a potential conflict of interest.
Publisher’s note
All claims expressed in this article are solely those of the authors and do not necessarily represent those of their affiliated organizations, or those of the publisher, the editors and the reviewers. Any product that may be evaluated in this article, or claim that may be made by its manufacturer, is not guaranteed or endorsed by the publisher.
References
Abdulla, A., Zhang, T., Li, S., Guo, W., Warden, A. R., Xin, Y., et al. (2022). Integrated microfluidic single-cell immunoblotting chip enables high-throughput isolation, enrichment and direct protein analysis of circulating tumor cells. Microsyst. Nanoeng. 8, 13. doi:10.1038/s41378-021-00342-2
Adalsteinsson, V. A., Ha, G., Freeman, S. S., Choudhury, A. D., Stover, D. G., Parsons, H. A., et al. (2017). Scalable whole-exome sequencing of cell-free DNA reveals high concordance with metastatic tumors. Nat. Commun. 8 (1), 1324. doi:10.1038/s41467-017-00965-y
Agelaki, S., Papadaki, M. A., Tsoulfas, P. G., Aggouraki, D., Monastirioti, A. A., Merodoulaki, K. A., et al. (2019). Role of the expression of PD-L1 and CD47 on circulating tumor cells (CTCs) in the prediction of outcome in metastatic breast cancer (mBC) patients. JCO 37 (15), e14045. doi:10.1200/jco.2019.37.15_suppl.e14045
Alexandrov, L. B., Jones, P. H., Wedge, D. C., Sale, J. E., Campbell, P. J., Nik-Zainal, S., et al. (2015). Clock-like mutational processes in human somatic cells. Nat. Genet. 47 (12), 1402–1407. doi:10.1038/ng.3441
Anfossi, S., Babayan, A., Pantel, K., and Calin, G. A. (2018). Clinical utility of circulating non-coding RNAs - an update. Nat. Rev. Clin. Oncol. 15 (9), 541–563. doi:10.1038/s41571-018-0035-x
Ankeny, J. S., Court, C. M., Hou, S., Li, Q., Song, M., Wu, D., et al. (2016). Circulating tumour cells as a biomarker for diagnosis and staging in pancreatic cancer. Br. J. Cancer 114 (12), 1367–1375. doi:10.1038/bjc.2016.121
Aravanis, A. M., Lee, M., and Klausner, R. D. (2017). Next-generation sequencing of circulating tumor DNA for early cancer detection. Cell 168 (4), 571–574. doi:10.1016/j.cell.2017.01.030
Ardrey, R. E. (2003). Liquid chromatography – mass spectrometry: An introduction. Chichester, UK: John Wiley & Sons, Ltd.
Armstrong, A. J., Marengo, M. S., Oltean, S., Kemeny, G., Bitting, R. L., Turnbull, J. D., et al. (2011). Circulating tumor cells from patients with advanced prostate and breast cancer display both epithelial and mesenchymal markers. Mol. Cancer Res. 9 (8), 997–1007. doi:10.1158/1541-7786.MCR-10-0490
Bagley, S. J., Till, J., Abdalla, A., Sangha, H. K., Yee, S. S., Freedman, J., et al. (2021). Association of plasma cell-free DNA with survival in patients with IDH wild-type glioblastoma. Neurooncol Adv. 3 (1), vdab011. doi:10.1093/noajnl/vdab011
Bakker, B., Taudt, A., Belderbos, M. E., Porubsky, D., Spierings, D. C. J., de Jong, T. V., et al. (2016). Single-cell sequencing reveals karyotype heterogeneity in murine and human malignancies. Genome Biol. 17 (1), 115. doi:10.1186/s13059-016-0971-7
Baldasici, O., Balacescu, L., Cruceriu, D., Roman, A., Lisencu, C., Fetica, B., et al. (2022). Circulating small EVs miRNAs as predictors of pathological response to neo-adjuvant therapy in breast cancer patients. Int. J. Mol. Sci. 23 (20), 12625. doi:10.3390/ijms232012625
Bartel, D. P. (2004). MicroRNAs: Genomics, biogenesis, mechanism, and function. Cell 116 (2), 281–297. doi:10.1016/s0092-8674(04)00045-5
Batth, I. S., Meng, Q., Wang, Q., Torres, K. E., Burks, J., Wang, J., et al. (2020). Rare osteosarcoma cell subpopulation protein array and profiling using imaging mass cytometry and bioinformatics analysis. BMC Cancer 20 (1), 715. doi:10.1186/s12885-020-07203-7
Begum, S., Brait, M., Dasgupta, S., Ostrow, K. L., Zahurak, M., Carvalho, A. L., et al. (2011). An epigenetic marker panel for detection of lung cancer using cell-free serum DNA. Clin. Cancer Res. 17 (13), 4494–4503. doi:10.1158/1078-0432.CCR-10-3436
Best, M. G., Sol, N., Kooi, I., Tannous, J., Westerman, B. A., Rustenburg, F., et al. (2015). RNA-seq of tumor-educated platelets enables blood-based pan-cancer, multiclass, and molecular pathway cancer diagnostics. Cancer Cell 28 (5), 666–676. doi:10.1016/j.ccell.2015.09.018
Best, M. G., Wesseling, P., and Wurdinger, T. (2018). Tumor-educated platelets as a noninvasive biomarker source for cancer detection and progression monitoring. Cancer Res. 78 (13), 3407–3412. doi:10.1158/0008-5472.CAN-18-0887
Bettegowda, C., Sausen, M., Leary, R. J., Kinde, I., Wang, Y., Agrawal, N., et al. (2014). Detection of circulating tumor DNA in early- and late-stage human malignancies. Sci. Transl. Med. 6 (224), 224ra24. doi:10.1126/scitranslmed.3007094
Biankin, A. V., Piantadosi, S., and Hollingsworth, S. J. (2015). Patient-centric trials for therapeutic development in precision oncology. Nature 526 (7573), 361–370. doi:10.1038/nature15819
Billroth, T. (1871). General surgical pathology and therapeutics in fifty lectures. Am. J. Med. Sci. 62 (123), 219–225. doi:10.1097/00000441-187107000-00030
Boeva, V., Popova, T., Bleakley, K., Chiche, P., Cappo, J., Schleiermacher, G., et al. (2012). Control-FREEC: A tool for assessing copy number and allelic content using next-generation sequencing data. Bioinformatics 28 (3), 423–425. doi:10.1093/bioinformatics/btr670
Boral, D., Vishnoi, M., Liu, H. N., Yin, W., Sprouse, M. L., Scamardo, A., et al. (2017). Molecular characterization of breast cancer CTCs associated with brain metastasis. Nat. Commun. 8 (1), 196. doi:10.1038/s41467-017-00196-1
Bowley, T., and Marchetti, D. (2022). Abstract 5120:deciphering melanoma CTC signatures leading to immune escape and brain metastasis: The first MRI CTC xenograft model. Cancer Res. 82 (12), 5120. doi:10.1158/1538-7445.am2022-5120
Brady, L., Hayes, B., Sheill, G., Baird, A-M., Guinan, E., Stanfill, B., et al. (2020). Platelet cloaking of circulating tumour cells in patients with metastatic prostate cancer: Results from ExPeCT, a randomised controlled trial. PLoS ONE 15 (12), e0243928. doi:10.1371/journal.pone.0243928
Brungs, D., Minaei, E., Piper, A-K., Perry, J., Splitt, A., Carolan, M., et al. (2020). Establishment of novel long-term cultures from EpCAM positive and negative circulating tumour cells from patients with metastatic gastroesophageal cancer. Sci. Rep. 10 (1), 539. doi:10.1038/s41598-019-57164-6
Bulfoni, M., Turetta, M., Del Ben, F., Di Loreto, C., Beltrami, A. P., and Cesselli, D. (2016). Dissecting the heterogeneity of circulating tumor cells in metastatic breast cancer: Going far beyond the needle in the haystack. Int. J. Mol. Sci. 17 (10), 1775. doi:10.3390/ijms17101775
Cabús, L., Lagarde, J., Curado, J., Lizano, E., and Pérez-Boza, J. (2022). Current challenges and best practices for cell-free long RNA biomarker discovery. Biomark. Res. 10 (1), 62. doi:10.1186/s40364-022-00409-w
Castro-Giner, F., and Aceto, N. (2020). Tracking cancer progression: From circulating tumor cells to metastasis. Genome Med. 12 (1), 31. doi:10.1186/s13073-020-00728-3
Chan, A. A., Bashir, M., Rivas, M. N., Duvall, K., Sieling, P. A., Pieber, T. R., et al. (2016). Characterization of the microbiome of nipple aspirate fluid of breast cancer survivors. Sci. Rep. 6, 28061. doi:10.1038/srep28061
Chan, M., Liaw, C. S., Ji, S. M., Tan, H. H., Wong, C. Y., Thike, A. A., et al. (2013). Identification of circulating microRNA signatures for breast cancer detection. Clin. Cancer Res. 19 (16), 4477–4487. doi:10.1158/1078-0432.CCR-12-3401
Chang, M-C., Chang, Y-T., Chen, J-Y., Jeng, Y-M., Yang, C-Y., Tien, Y-W., et al. (2016). Clinical significance of circulating tumor microemboli as a prognostic marker in patients with pancreatic ductal adenocarcinoma. Clin. Chem. 62 (3), 505–513. doi:10.1373/clinchem.2015.248260
Chang, Y., Wang, Y., Li, B., Lu, X., Wang, R., Li, H., et al. (2021). Whole-Exome sequencing on circulating tumor cells explores platinum-drug resistance mutations in advanced non-small cell lung cancer. Front. Genet. 12, 722078. doi:10.3389/fgene.2021.722078
Chen, G., Huang, A. C., Zhang, W., Zhang, G., Wu, M., Xu, W., et al. (2018). Exosomal PD-L1 contributes to immunosuppression and is associated with anti-PD-1 response. Nature 560 (7718), 382–386. doi:10.1038/s41586-018-0392-8
Chen, H-Y., Zhang, W-L., Zhang, L., Yang, P., Li, F., Yang, Z-R., et al. (2021). 5-Hydroxymethylcytosine profiles of cfDNA are highly predictive of R-CHOP treatment response in diffuse large B cell lymphoma patients. Clin. Epigenetics 13 (1), 33. doi:10.1186/s13148-020-00973-8
Chen, I-H., Xue, L., Hsu, C-C., Paez, J. S. P., Pan, L., Andaluz, H., et al. (2017). Phosphoproteins in extracellular vesicles as candidate markers for breast cancer. Proc. Natl. Acad. Sci. U. S. A. 114 (12), 3175–3180. doi:10.1073/pnas.1618088114
Chen, J. F., and Yan, Q. (2021). The roles of epigenetics in cancer progression and metastasis. Biochem. J. 478 (17), 3373–3393. doi:10.1042/BCJ20210084
Chen, S., Gui, R., Zhou, X-H., Zhang, J-H., Jiang, H-Y., Liu, H-T., et al. (2022). Combined microbiome and metabolome analysis reveals a novel interplay between intestinal flora and serum metabolites in lung cancer. Front. Cell Infect. Microbiol. 12, 885093. doi:10.3389/fcimb.2022.885093
Chen, X., Gole, J., Gore, A., He, Q., Lu, M., Min, J., et al. (2020). Non-invasive early detection of cancer four years before conventional diagnosis using a blood test. Nat. Commun. 11 (1), 3475. doi:10.1038/s41467-020-17316-z
Chen, Z-Y., Zhang, J-L., Yao, H-X., Wang, P-Y., Zhu, J., Wang, W., et al. (2014). Aberrant methylation of the SPARC gene promoter and its clinical implication in gastric cancer. Sci. Rep. 4, 7035. doi:10.1038/srep07035
Cheng, Y-H., Chen, Y-C., Lin, E., Brien, R., Jung, S., Chen, Y-T., et al. (2019). Hydro-Seq enables contamination-free high-throughput single-cell RNA-sequencing for circulating tumor cells. Nat. Commun. 10 (1), 2163. doi:10.1038/s41467-019-10122-2
Chinello, C., Stella, M., Piga, I., Smith, A. J., Bovo, G., Varallo, M., et al. (2019). Proteomics of liquid biopsies: Depicting RCC infiltration into the renal vein by MS analysis of urine and plasma. J. Proteomics 191, 29–37. doi:10.1016/j.jprot.2018.04.029
Chiu, B. C-H., Zhang, Z., You, Q., Zeng, C., Stepniak, E., Bracci, P. M., et al. (2019). Prognostic implications of 5-hydroxymethylcytosines from circulating cell-free DNA in diffuse large B-cell lymphoma. Blood Adv. 3 (19), 2790–2799. doi:10.1182/bloodadvances.2019000175
Clos-Garcia, M., Loizaga-Iriarte, A., Zuñiga-Garcia, P., Sánchez-Mosquera, P., Rosa Cortazar, A., González, E., et al. (2018). Metabolic alterations in urine extracellular vesicles are associated to prostate cancer pathogenesis and progression. J. Extracell. Vesicles 7 (1), 1470442. doi:10.1080/20013078.2018.1470442
Cohen, J. D., Li, L., Wang, Y., Thoburn, C., Afsari, B., Danilova, L., et al. (2018). Detection and localization of surgically resectable cancers with a multi-analyte blood test. Science 359 (6378), 926–930. doi:10.1126/science.aar3247
Cohen, S. J., Punt, C. J. A., Iannotti, N., Saidman, B. H., Sabbath, K. D., Gabrail, N. Y., et al. (2008). Relationship of circulating tumor cells to tumor response, progression-free survival, and overall survival in patients with metastatic colorectal cancer. J. Clin. Oncol. 26 (19), 3213–3221. doi:10.1200/JCO.2007.15.8923
Comino-Mendez, I., and Turner, N. (2017). Predicting relapse with circulating tumor DNA analysis in lung cancer. Cancer Discov. 7 (12), 1368–1370. doi:10.1158/2159-8290.CD-17-1086
Coombes, R. C., Page, K., Salari, R., Hastings, R. K., Armstrong, A., Ahmed, S., et al. (2019). Personalized detection of circulating tumor DNA antedates breast cancer metastatic recurrence. Clin. Cancer Res. 25 (14), 4255–4263. doi:10.1158/1078-0432.CCR-18-3663
Corces, M. R., Granja, J. M., Shams, S., Louie, B. H., Seoane, J. A., Zhou, W., et al. (2018). The chromatin accessibility landscape of primary human cancers. Science 362 (6413), eaav1898. doi:10.1126/science.aav1898
Costa-Silva, B., Aiello, N. M., Ocean, A. J., Singh, S., Zhang, H., Thakur, B. K., et al. (2015). Pancreatic cancer exosomes initiate pre-metastatic niche formation in the liver. Nat. Cell Biol. 17 (6), 816–826. doi:10.1038/ncb3169
Cristiano, S., Leal, A., Phallen, J., Fiksel, J., Adleff, V., Bruhm, D. C., et al. (2019). Genome-wide cell-free DNA fragmentation in patients with cancer. Nature 570 (7761), 385–389. doi:10.1038/s41586-019-1272-6
Cristofanilli, M., Budd, G. T., Ellis, M. J., Stopeck, A., Matera, J., Miller, M. C., et al. (2004). Circulating tumor cells, disease progression, and survival in metastatic breast cancer. N. Engl. J. Med. 351 (8), 781–791. doi:10.1056/nejmoa040766
Cristofanilli, M., Pierga, J-Y., Reuben, J., Rademaker, A., Davis, A. A., Peeters, D. J., et al. (2019). The clinical use of circulating tumor cells (CTCs) enumeration for staging of metastatic breast cancer (MBC): International expert consensus paper. Crit. Rev. Oncol. Hematol. 134, 39–45. doi:10.1016/j.critrevonc.2018.12.004
Crowley, E., Di Nicolantonio, F., Loupakis, F., and Bardelli, A. (2013). Liquid biopsy: Monitoring cancer-genetics in the blood. Nat. Rev. Clin. Oncol. 10 (8), 472–484. doi:10.1038/nrclinonc.2013.110
Cuzick, J., Swanson, G. P., Fisher, G., Brothman, A. R., Berney, D. M., Reid, J. E., et al. (2011). Prognostic value of an RNA expression signature derived from cell cycle proliferation genes in patients with prostate cancer: A retrospective study. Lancet Oncol. 12 (3), 245–255. doi:10.1016/S1470-2045(10)70295-3
Darshan, M. S., Loftus, M. S., Thadani-Mulero, M., Levy, B. P., Escuin, D., Zhou, X. K., et al. (2011). Taxane-induced blockade to nuclear accumulation of the androgen receptor predicts clinical responses in metastatic prostate cancer. Cancer Res. 71 (18), 6019–6029. doi:10.1158/0008-5472.CAN-11-1417
De Angelis, G., Rittenhouse, H. G., Mikolajczyk, S. D., Blair Shamel, L., and Semjonow, A. (2007). Twenty years of PSA: From prostate antigen to tumor marker. Rev. Urol. 9 (3), 113–123.
de Bono, J. S., Pantel, K., Efstathiou, E., Sternberg, C. N., Gauna, D. C., Fizazi, K., et al. (2021). 614P Circulating tumor cell (CTC) morphologic sub-types present prior to treatment in the CARD trial identify therapy resistance. Ann. Oncol. 32, S653–S654. doi:10.1016/j.annonc.2021.08.1127
Dean, F. B., Nelson, J. R., Giesler, T. L., and Lasken, R. S. (2001). Rapid amplification of plasmid and phage DNA using Phi 29 DNA polymerase and multiply-primed rolling circle amplification. Genome Res. 11 (6), 1095–1099. doi:10.1101/gr.180501
Del Re, M., Biasco, E., Crucitta, S., Derosa, L., Rofi, E., Orlandini, C., et al. (2017). The detection of androgen receptor splice variant 7 in plasma-derived exosomal RNA strongly predicts resistance to hormonal therapy in metastatic prostate cancer patients. Eur. Urol. 71 (4), 680–687. doi:10.1016/j.eururo.2016.08.012
Diehl, F., Li, M., He, Y., Kinzler, K. W., Vogelstein, B., and Dressman, D. (2006). BEAMing: Single-molecule PCR on microparticles in water-in-oil emulsions. Nat. Methods 3 (7), 551–559. doi:10.1038/nmeth898
Diehl, F., Schmidt, K., Choti, M. A., Romans, K., Goodman, S., Li, M., et al. (2008). Circulating mutant DNA to assess tumor dynamics. Nat. Med. 14 (9), 985–990. doi:10.1038/nm.1789
Diehl, F., Schmidt, K., Durkee, K. H., Moore, K. J., Goodman, S. N., Shuber, A. P., et al. (2008). Analysis of mutations in DNA isolated from plasma and stool of colorectal cancer patients. Gastroenterology 135 (2), 489–498. doi:10.1053/j.gastro.2008.05.039
Do Canto, L. M., Marian, C., Willey, S., Sidawy, M., Da Cunha, P. A., Rone, J. D., et al. (2016). MicroRNA analysis of breast ductal fluid in breast cancer patients. Int. J. Oncol. 48 (5), 2071–2078. doi:10.3892/ijo.2016.3435
Dong, Y., Wang, Z., and Shi, Q. (2020). Liquid biopsy based single-cell transcriptome profiling characterizes heterogeneity of disseminated tumor cells from lung adenocarcinoma. Proteomics 20 (13), e1900224. doi:10.1002/pmic.201900224
Elia, I., and Haigis, M. C. (2021). Metabolites and the tumour microenvironment: From cellular mechanisms to systemic metabolism. Nat. Metab. 3 (1), 21–32. doi:10.1038/s42255-020-00317-z
Eslami-S, Z., Cortés-Hernández, L. E., Thomas, F., Pantel, K., and Alix-Panabières, C. (2022). Functional analysis of circulating tumour cells: The KEY to understand the biology of the metastatic cascade. Br. J. Cancer 127 (5), 800–810. doi:10.1038/s41416-022-01819-1
Fares, J., Fares, M. Y., Khachfe, H. H., Salhab, H. A., and Fares, Y. (2020). Molecular principles of metastasis: A hallmark of cancer revisited. Signal Transduct. Target Ther. 5 (1), 28. doi:10.1038/s41392-020-0134-x
Favero, F., Joshi, T., Marquard, A. M., Birkbak, N. J., Krzystanek, M., Li, Q., et al. (2015). Sequenza: Allele-specific copy number and mutation profiles from tumor sequencing data. Ann. Oncol. 26 (1), 64–70. doi:10.1093/annonc/mdu479
Felici, C., Mannavola, F., Stucci, L. S., Duda, L., Cafforio, P., Porta, C., et al. (2022). Circulating tumor cells from melanoma patients show phenotypic plasticity and metastatic potential in xenograft NOD.CB17 mice. BMC Cancer 22 (1), 754. doi:10.1186/s12885-022-09829-1
Fernandez-Mercado, M., Manterola, L., Larrea, E., Goicoechea, I., Arestin, M., Armesto, M., et al. (2015). The circulating transcriptome as a source of non-invasive cancer biomarkers: Concepts and controversies of non-coding and coding RNA in body fluids. J. Cell Mol. Med. 19 (10), 2307–2323. doi:10.1111/jcmm.12625
Fiala, C., and Diamandis, E. P. (2018). Utility of circulating tumor DNA in cancer diagnostics with emphasis on early detection. BMC Med. 16 (1), 166. doi:10.1186/s12916-018-1157-9
Fiehn, O. (2016). Metabolomics by gas chromatography-mass spectrometry: Combined targeted and untargeted profiling. Curr. Protoc. Mol. Biol. 114, 30.4.1. doi:10.1002/0471142727.mb3004s114
Forshew, T., Murtaza, M., Parkinson, C., Gale, D., Tsui, D. W. Y., Kaper, F., et al. (2012). Noninvasive identification and monitoring of cancer mutations by targeted deep sequencing of plasma DNA. Sci. Transl. Med. 4 (136), 136ra68. doi:10.1126/scitranslmed.3003726
Frei, A. P., Bava, F-A., Zunder, E. R., Hsieh, E. W. Y., Chen, S-Y., Nolan, G. P., et al. (2016). Highly multiplexed simultaneous detection of RNAs and proteins in single cells. Nat. Methods 13 (3), 269–275. doi:10.1038/nmeth.3742
Fu, Q., Zhu, J., and Van Eyk, J. E. (2010). Comparison of multiplex immunoassay platforms. Clin. Chem. 56 (2), 314–318. doi:10.1373/clinchem.2009.135087
Fu, Y., Zhang, F., Zhang, X., Yin, J., Du, M., Jiang, M., et al. (2019). High-throughput single-cell whole-genome amplification through centrifugal emulsification and eMDA. Commun. Biol. 2, 147. doi:10.1038/s42003-019-0401-y
Fujimura, A., Pei, H., Zhang, H., Sladitschek, H. L., and Chang, L. (2020). Editorial: The role of epigenetic modifications in cancer progression. Front. Oncol. 10, 617178. doi:10.3389/fonc.2020.617178
Galardi, F., Luca, F. D., Romagnoli, D., Biagioni, C., Moretti, E., Biganzoli, L., et al. (2020). Cell-free DNA-methylation-based methods and applications in oncology. Biomolecules 10 (12), 1677. doi:10.3390/biom10121677
Gale, D., Lawson, A. R. J., Howarth, K., Madi, M., Durham, B., Smalley, S., et al. (2018). Development of a highly sensitive liquid biopsy platform to detect clinically-relevant cancer mutations at low allele fractions in cell-free DNA. PLoS ONE 13 (3), e0194630. doi:10.1371/journal.pone.0194630
Gallardo-Gómez, M., Moran, S., Páez de la Cadena, M., Martínez-Zorzano, V. S., Rodríguez-Berrocal, F. J., Rodríguez-Girondo, M., et al. (2018). A new approach to epigenome-wide discovery of non-invasive methylation biomarkers for colorectal cancer screening in circulating cell-free DNA using pooled samples. Clin. Epigenetics 10, 53. doi:10.1186/s13148-018-0487-y
Gallego-Fabrega, C., Carrera, C., Muiño, E., Montaner, J., Krupinski, J., Fernandez-Cadenas, I., et al. (2015). DNA methylation levels are highly correlated between pooled samples and averaged values when analysed using the Infinium HumanMethylation450 BeadChip array. Clin. Epigenetics 7 (1), 78. doi:10.1186/s13148-015-0097-x
Gambardella, V., Tarazona, N., Cejalvo, J. M., Lombardi, P., Huerta, M., Roselló, S., et al. (2020). Personalized medicine: Recent progress in cancer therapy. Cancers (Basel) 12 (4), 1009. doi:10.3390/cancers12041009
Gandara, D. R., Paul, S. M., Kowanetz, M., Schleifman, E., Zou, W., Li, Y., et al. (2018). Blood-based tumor mutational burden as a predictor of clinical benefit in non-small-cell lung cancer patients treated with atezolizumab. Nat. Med. 24 (9), 1441–1448. doi:10.1038/s41591-018-0134-3
Gao, M., Callari, M., Beddowes, E., Sammut, S-J., Grzelak, M., Biggs, H., et al. (2019). Next generation-targeted amplicon sequencing (NG-TAS): An optimised protocol and computational pipeline for cost-effective profiling of circulating tumour DNA. Genome Med. 11 (1), 1. doi:10.1186/s13073-018-0611-9
García-Foncillas, J., Tabernero, J., Élez, E., Aranda, E., Benavides, M., Camps, C., et al. (2018). Prospective multicenter real-world RAS mutation comparison between OncoBEAM-based liquid biopsy and tissue analysis in metastatic colorectal cancer. Br. J. Cancer 119 (12), 1464–1470. doi:10.1038/s41416-018-0293-5
Garlan, F., Laurent-Puig, P., Sefrioui, D., Siauve, N., Didelot, A., Sarafan-Vasseur, N., et al. (2017). Early evaluation of circulating tumor DNA as marker of therapeutic efficacy in metastatic colorectal cancer patients (PLACOL study). Clin. Cancer Res. 23 (18), 5416–5425. doi:10.1158/1078-0432.CCR-16-3155
Garvin, T., Aboukhalil, R., Kendall, J., Baslan, T., Atwal, G. S., Hicks, J., et al. (2015). Interactive analysis and assessment of single-cell copy-number variations. Nat. Methods 12 (11), 1058–1060. doi:10.1038/nmeth.3578
Gasch, C., Oldopp, T., Mauermann, O., Gorges, T. M., Andreas, A., Coith, C., et al. (2016). Frequent detection of PIK3CA mutations in single circulating tumor cells of patients suffering from HER2-negative metastatic breast cancer. Mol. Oncol. 10 (8), 1330–1343. doi:10.1016/j.molonc.2016.07.005
Genovese, G., Kähler, A. K., Handsaker, R. E., Lindberg, J., Rose, S. A., Bakhoum, S. F., et al. (2014). Clonal hematopoiesis and blood-cancer risk inferred from blood DNA sequence. N. Engl. J. Med. 371 (26), 2477–2487. doi:10.1056/NEJMoa1409405
Georgiadis, A., Durham, J. N., Keefer, L. A., Bartlett, B. R., Zielonka, M., Murphy, D., et al. (2019). Noninvasive detection of microsatellite instability and high tumor mutation burden in cancer patients treated with PD-1 blockade. Clin. Cancer Res. 25 (23), 7024–7034. doi:10.1158/1078-0432.CCR-19-1372
Gezer, U., Ustek, D., Yörüker, E. E., Cakiris, A., Abaci, N., Leszinski, G., et al. (2013). Characterization of H3K9me3-and H4K20me3-associated circulating nucleosomal DNA by high-throughput sequencing in colorectal cancer. Tumour Biol. 34 (1), 329–336. doi:10.1007/s13277-012-0554-5
Giallombardo, M., Jorge Chacartegui, J., Reclusa, P., Van Meerbeeck, J. P., Alessandro, R., Peeters, M., et al. (2016). Follow up analysis by exosomal miRNAs in EGFR mutated non-small cell lung cancer (NSCLC) patients during osimertinib (AZD9291) treatment: A potential prognostic biomarker tool. JCO 34 (15), e23035. doi:10.1200/jco.2016.34.15_suppl.e23035
Giuliano, M., Giordano, A., Jackson, S., De Giorgi, U., Mego, M., Cohen, E. N., et al. (2014). Circulating tumor cells as early predictors of metastatic spread in breast cancer patients with limited metastatic dissemination. Breast Cancer Res. 16 (5), 440. doi:10.1186/s13058-014-0440-8
Gkountela, S., Castro-Giner, F., Szczerba, B. M., Vetter, M., Landin, J., Scherrer, R., et al. (2019). Circulating tumor cell clustering shapes DNA methylation to enable metastasis seeding. Cell 176 (1–2), 98–112.e14. doi:10.1016/j.cell.2018.11.046
Glinge, C., Clauss, S., Boddum, K., Jabbari, R., Jabbari, J., Risgaard, B., et al. (2017). Stability of circulating blood-based MicroRNAs - pre-analytic methodological considerations. PLoS ONE 12 (2), e0167969. doi:10.1371/journal.pone.0167969
Goodman, A. M., Kato, S., Bazhenova, L., Patel, S. P., Frampton, G. M., Miller, V., et al. (2017). Tumor mutational burden as an independent predictor of response to immunotherapy in diverse cancers. Mol. Cancer Ther. 16 (11), 2598–2608. doi:10.1158/1535-7163.MCT-17-0386
Gordevičius, J., Kriščiūnas, A., Groot, D. E., Yip, S. M., Susic, M., Kwan, A., et al. (2018). Cell-free DNA modification dynamics in abiraterone acetate-treated prostate cancer patients. Clin. Cancer Res. 24 (14), 3317–3324. doi:10.1158/1078-0432.CCR-18-0101
Graf, R. P., Hullings, M., Barnett, E. S., Carbone, E., Dittamore, R., and Scher, H. I. (2020). Clinical utility of the nuclear-localized AR-V7 biomarker in circulating tumor cells in improving physician treatment choice in castration-resistant prostate cancer. Eur. Urol. 77 (2), 170–177. doi:10.1016/j.eururo.2019.08.020
Greger, V., Passarge, E., Höpping, W., Messmer, E., and Horsthemke, B. (1989). Epigenetic changescontribute to the formation and spontaneous regression of retinoblastoma. Hum. Genet. 83 (2), 155–158. doi:10.1007/BF00286709
Grunau, C., Clark, S. J., and Rosenthal, A. (2001). Bisulfite genomic sequencing: Systematic investigation of critical experimental parameters. Nucleic Acids Res. 29 (13), E65–5. doi:10.1093/nar/29.13.e65
Gu, H., Smith, Z. D., Bock, C., Boyle, P., Gnirke, A., and Meissner, A. (2011). Preparation of reduced representation bisulfite sequencing libraries for genome-scale DNA methylation profiling. Nat. Protoc. 6 (4), 468–481. doi:10.1038/nprot.2010.190
Guo, H., Zhu, P., Guo, F., Li, X., Wu, X., Fan, X., et al. (2015). Profiling DNA methylome landscapes of mammalian cells with single-cell reduced-representation bisulfite sequencing. Nat. Protoc. 10 (5), 645–659. doi:10.1038/nprot.2015.039
Guo, P., Zheng, H., Li, Y., Li, Y., Xiao, Y., Zheng, J., et al. (2023). Hepatocellular carcinoma detection via targeted enzymatic methyl sequencing of plasma cell-free DNA. Clin. Epigenetics 15 (1), 2. doi:10.1186/s13148-022-01420-6
Guo, S., Diep, D., Plongthongkum, N., Fung, H-L., Zhang, K., and Zhang, K. (2017). Identification of methylation haplotype blocks aids inonvolution of heterogeneous tissue samples and tumor tissue-of-origin mapping from plasma DNA. Nat. Genet. 49 (4), 635–642. doi:10.1038/ng.3805
Guo, W., Sun, Y-F., Shen, M-N., Ma, X-L., Wu, J., Zhang, C-Y., et al. (2018). Circulating tumor cells with stem-like phenotypes for diagnosis, prognosis, and therapeutic response evaluation in hepatocellular carcinoma. Clin. Cancer Res. 24 (9), 2203–2213. doi:10.1158/1078-0432.CCR-17-1753
Guo, Z., Lin, X., Hui, Y., Wang, J., Zhang, Q., and Kong, F. (2022). Circulating tumor cell identification based on deep learning. Front. Oncol. 12, 843879. doi:10.3389/fonc.2022.843879
Hai, L., Li, L., Liu, Z., Tong, Z., and Sun, Y. (2022). Whole-genome circulating tumor DNA methylation landscape reveals sensitive biomarkers of breast cancer. MedComm 3 (3), e134. doi:10.1002/mco2.134
Han, J., Li, Q., Chen, Y., and Yang, Y. (2021). Recent metabolomics analysis in tumor metabolism reprogramming. Front. Mol. Biosci. 8, 763902. doi:10.3389/fmolb.2021.763902
Hansen, T. F., Carlsen, A. L., Heegaard, N. H. H., Sørensen, F. B., and Jakobsen, A. (2015). Changes in circulating microRNA-126 during treatment with chemotherapy and bevacizumab predicts treatment response in patients with metastatic colorectal cancer. Br. J. Cancer 112 (4), 624–629. doi:10.1038/bjc.2014.652
Hashimoto, K., Kokubun, S., Itoi, E., and Roach, H. I. (2007). Improved quantification of DNA methylation using methylation-sensitive restriction enzymes and real-time PCR. Epigenetics 2 (2), 86–91. doi:10.4161/epi.2.2.4203
Hashimshony, T., Senderovich, N., Avital, G., Klochendler, A., de Leeuw, Y., Anavy, L., et al. (2016). CEL-Seq2: Sensitive highly-multiplexed single-cell RNA-seq. Genome Biol. 17, 77. doi:10.1186/s13059-016-0938-8
Hashimshony, T., Wagner, F., Sher, N., and Yanai, I. (2012). CEL-seq: Single-cell RNA-seq by multiplexed linear amplification. Cell Rep. 2 (3), 666–673. doi:10.1016/j.celrep.2012.08.003
Hatt, L., Aagaard, M. M., Graakjaer, J., Bach, C., Sommer, S., Agerholm, I. E., et al. (2015). Microarray-based analysis of methylation status of CpGs in placental DNA and maternal blood DNA--potential new epigenetic biomarkers for cell free fetal DNA-based diagnosis. PLoS ONE 10 (7), e0128918. doi:10.1371/journal.pone.0128918
He, B., Lu, Q., Lang, J., Yu, H., Peng, C., Bing, P., et al. (2020). A new method for CTC images recognition based on machine learning. Front. Bioeng. Biotechnol. 8, 897. doi:10.3389/fbioe.2020.00897
Herman, J. G., and Baylin, S. B. (2003). Gene silencing in cancer in association with promoter hypermethylation. N. Engl. J. Med. 349 (21), 2042–2054. doi:10.1056/nejmra023075
Heyn, H., and Esteller, M. (2012). DNA methylation profiling in the clinic: Applications and challenges. Nat. Rev. Genet. 13 (10), 679–692. doi:10.1038/nrg3270
Hindson, C. M., Chevillet, J. R., Briggs, H. A., Gallichotte, E. N., Ruf, I. K., Hindson, B. J., et al. (2013). Absolute quantification by droplet digital PCR versus analog real-time PCR. Nat. Methods 10 (10), 1003–1005. doi:10.1038/nmeth.2633
Hodgkinson, C. L., Morrow, C. J., Li, Y., Metcalf, R. L., Rothwell, D. G., Trapani, F., et al. (2014). Tumorigenicity and genetic profiling of circulating tumor cells in small-cell lung cancer. Nat. Med. 20 (8), 897–903. doi:10.1038/nm.3600
Hofer, F., Di Sario, G., Musiu, C., Sartoris, S., De Sanctis, F., and Ugel, S. (2021). A complex metabolic network confers immunosuppressive functions to myeloid-derived suppressor cells (MDSCs) within the tumour microenvironment. Cells 10 (10), 2700. doi:10.3390/cells10102700
Hon, K. W., Abu, N., Ab Mutalib, N-S., and Jamal, R. (2018). miRNAs and lncRNAs as predictive biomarkers of response to FOLFOX therapy in colorectal cancer. Front. Pharmacol. 9, 846. doi:10.3389/fphar.2018.00846
Hoshino, A., Costa-Silva, B., Shen, T-L., Rodrigues, G., Hashimoto, A., Tesic Mark, M., et al. (2015). Tumour exosome integrins determine organotropic metastasis. Nature 527 (7578), 329–335. doi:10.1038/nature15756
Hoshino, A., Kim, H. S., Bojmar, L., Gyan, K. E., Cioffi, M., Hernandez, J., et al. (2020). Extracellular vesicle and particle biomarkers define multiple human cancers. Cell 182 (4), 1044–1061.e18. doi:10.1016/j.cell.2020.07.009
Hou, J., Meng, F., Chan, L. W. C., Cho, W. C. S., and Wong, S. C. C. (2016). Circulating plasma micrornas as diagnostic markers for NSCLC. Front. Genet. 7, 193. doi:10.3389/fgene.2016.00193
Huang, J., Soupir, A. C., Schlick, B. D., Teng, M., Sahin, I. H., Permuth, J. B., et al. (2021). Cancer detection and classification by CpG island hypermethylation signatures in plasma cell-free DNA. Cancers (Basel) 13 (22), 5611. doi:10.3390/cancers13225611
Huang, J., Soupir, A. C., and Wang, L. (2022). Cell-free DNA methylome profiling by MBD-seq with ultra-low input. Epigenetics 17 (3), 239–252. doi:10.1080/15592294.2021.1896984
Huang, L., Ma, F., Chapman, A., Lu, S., and Xie, X. S. (2015). Single-cell whole-genome amplification and sequencing: Methodology and applications. Annu. Rev. Genomics Hum. Genet. 16, 79–102. doi:10.1146/annurev-genom-090413-025352
Huang, X., Yuan, T., Liang, M., Du, M., Xia, S., Dittmar, R., et al. (2015). Exosomal miR-1290 and miR-375 as prognostic markers in castration-resistant prostate cancer. Eur. Urol. 67 (1), 33–41. doi:10.1016/j.eururo.2014.07.035
Huang, Y., Kanada, M., Ye, J., Deng, Y., He, Q., Lei, Z., et al. (2022). Exosome-mediated remodeling of the tumor microenvironment: From local to distant intercellular communication. Cancer Lett. 543, 215796. doi:10.1016/j.canlet.2022.215796
Hulbert, A., Jusue-Torres, I., Stark, A., Chen, C., Rodgers, K., Lee, B., et al. (2017). Early detection of lung cancer using DNA promoter hypermethylation in plasma and sputum. Clin. Cancer Res. 23 (8), 1998–2005. doi:10.1158/1078-0432.CCR-16-1371
Hyman, D. M., Taylor, B. S., and Baselga, J. (2017). Implementing genome-driven oncology. Cell 168 (4), 584–599. doi:10.1016/j.cell.2016.12.015
ich-Poore, G. D., Zitvogel, L., Straussman, R., Hasty, J., Wargo, J. A., and Knight, R. (2021). The microbiome and human cancer. Science 371 (6536), 371. doi:10.1126/science.abc4552
Ignatiadis, M., Sledge, G. W., and Jeffrey, S. S. (2021). Liquid biopsy enters the clinic - implementation issues and future challenges. Nat. Rev. Clin. Oncol. 18 (5), 297–312. doi:10.1038/s41571-020-00457-x
Imperiale, T. F., Ransohoff, D. F., Itzkowitz, S. H., Levin, T. R., Lavin, P., Lidgard, G. P., et al. (2014). Multitarget stool DNA testing for colorectal-cancer screening. N. Engl. J. Med. 370 (14), 1287–1297. doi:10.1056/nejmoa1311194
In ’t Veld, S. G. J. G., and Wurdinger, T. (2019). Tumor-educated platelets. Blood 133 (22), 2359–2364. doi:10.1182/blood-2018-12-852830
Ishiba, T., Hoffmann, A-C., Usher, J., Elshimali, Y., Sturdevant, T., Dang, M., et al. (2018). Frequencies and expression levels of programmed death ligand 1 (PD-L1) in circulating tumor RNA (ctRNA) in various cancer types. Biochem. Biophys. Res. Commun. 500 (3), 621–625. doi:10.1016/j.bbrc.2018.04.120
Islam, S., Kjällquist, U., Moliner, A., Zajac, P., Fan, J-B., Lönnerberg, P., et al. (2011). Characterization of the single-cell transcriptional landscape by highly multiplex RNA-seq. Genome Res. 21 (7), 1160–1167. doi:10.1101/gr.110882.110
Jamal-Hanjani, M., Quezada, S. A., Larkin, J., and Swanton, C. (2015). Translational implications of tumor heterogeneity. Clin. Cancer Res. 21 (6), 1258–1266. doi:10.1158/1078-0432.ccr-14-1429
Jamal-Hanjani, M., Wilson, G. A., Horswell, S., Mitter, R., Sakarya, O., Constantin, T., et al. (2016). Detection of ubiquitous and heterogeneous mutations in cell-free DNA from patients with early-stage non-small-cell lung cancer. Ann. Oncol. 27 (5), 862–867. doi:10.1093/annonc/mdw037
Jameson, J. L., and Longo, D. L. (2015). Precision medicine--personalized, problematic, and promising. N. Engl. J. Med. 372 (23), 2229–2234. doi:10.1056/NEJMsb1503104
Jamshidi, A., Liu, M. C., Klein, E. A., Venn, O., Hubbell, E., Beausang, J. F., et al. (2022). Evaluation of cell-free DNA approaches for multi-cancer early detection. Cancer Cell 40 (12), 1537–1549.e12. doi:10.1016/j.ccell.2022.10.022
Jiang, P., and Lo, Y. M. D. (2016). The long and short of circulating cell-free DNA and the ins and outs of molecular diagnostics. Trends Genet. 32 (6), 360–371. doi:10.1016/j.tig.2016.03.009
Jin, S., Zhu, D., Shao, F., Chen, S., Guo, Y., Li, K., et al. (2021). Efficient detection and post-surgical monitoring of colon cancer with a multi-marker DNA methylation liquid biopsy. Proc. Natl. Acad. Sci. U. S. A. 118 (5), e2017421118. doi:10.1073/pnas.2017421118
Joosse, S. A., and Pantel, K. (2022). Circulating DNA and liquid biopsies in the management of patients with cancer. Cancer Res. 82 (12), 2213–2215. doi:10.1158/0008-5472.CAN-22-1405
Jordan, N. V., Bardia, A., Wittner, B. S., Benes, C., Ligorio, M., Zheng, Y., et al. (2016). HER2 expression identifies dynamic functional states within circulating breast cancer cells. Nature 537 (7618), 102–106. doi:10.1038/nature19328
Kalra, H., Simpson, R. J., Ji, H., Aikawa, E., Altevogt, P., Askenase, P., et al. (2012). Vesiclepedia: A compendium for extracellular vesicles with continuous community annotation. PLoS Biol. 10 (12), e1001450. doi:10.1371/journal.pbio.1001450
Kang, Y. P., Ward, N. P., and DeNicola, G. M. (2018). Recent advances in cancer metabolism: A technological perspective. Exp. Mol. Med. 50 (4), 1–16. doi:10.1038/s12276-018-0027-z
Kato, R., Hayashi, H., Sakai, K., Suzuki, S., Haratani, K., Takahama, T., et al. (2021). CAPP-seq analysis of circulating tumor DNA from patients with EGFR T790M-positive lung cancer after osimertinib. Int. J. Clin. Oncol. 26 (9), 1628–1639. doi:10.1007/s10147-021-01947-3
Katsman, E., Orlanski, S., Martignano, F., Fox-Fisher, I., Shemer, R., Dor, Y., et al. (2022). Detecting cell-of-origin and cancer-specific methylation features of cell-free DNA from Nanopore sequencing. Genome Biol. 23 (1), 158. doi:10.1186/s13059-022-02710-1
Ke, C., Hou, Y., Zhang, H., Fan, L., Ge, T., Guo, B., et al. (2015). Large-scale profiling of metabolic dysregulation in ovarian cancer. Int. J. Cancer 136 (3), 516–526. doi:10.1002/ijc.29010
Keller, L., and Pantel, K. (2019). Unravelling tumour heterogeneity by single-cell profiling of circulating tumour cells. Nat. Rev. Cancer 19 (10), 553–567. doi:10.1038/s41568-019-0180-2
Khatami, F., Aghamir, S. M. K., and Tavangar, S. M. (2019). Oncometabolites: A new insight for oncology. Mol. Genet. Genomic Med. 7 (9), e873. doi:10.1002/mgg3.873
Kim, Y., Jeon, J., Mejia, S., Yao, C. Q., Ignatchenko, V., Nyalwidhe, J. O., et al. (2016). Targeted proteomics identifies liquid-biopsy signatures for extracapsular prostate cancer. Nat. Commun. 7, 11906. doi:10.1038/ncomms11906
Kingston, B., Cutts, R. J., Bye, H., Beaney, M., Walsh-Crestani, G., Hrebien, S., et al. (2021). Genomic profile of advanced breast cancer in circulating tumour DNA. Nat. Commun. 12 (1), 2423. doi:10.1038/s41467-021-22605-2
Klempner, S. J., Fabrizio, D., Bane, S., Reinhart, M., Peoples, T., Ali, S. M., et al. (2020). Tumor mutational burden as a predictive biomarker for response to immune checkpoint inhibitors: A review of current evidence. Oncologist 25 (1), e147–e159. doi:10.1634/theoncologist.2019-0244
Kloten, V., Lampignano, R., Krahn, T., and Schlange, T. (2019). Circulating tumor cell PD-L1 expression as biomarker for therapeutic efficacy of immune checkpoint inhibition in NSCLC. Cells 8 (8), 809. doi:10.3390/cells8080809
Klotz, R., Thomas, A., Teng, T., Han, S. M., Iriondo, O., Li, L., et al. (2020). Circulating tumor cells exhibit metastatic tropism and reveal brain metastasis drivers. Cancer Discov. 10 (1), 86–103. doi:10.1158/2159-8290.CD-19-0384
Ko, J., Baldassano, S. N., Loh, P-L., Kording, K., Litt, B., and Issadore, D. (2018). Machine learning to detect signatures of disease in liquid biopsies - a user’s guide. Lab. Chip 18 (3), 395–405. doi:10.1039/c7lc00955k
Kong, D., Zhang, W., Yang, Z., Li, G., Cheng, S., Zhang, K., et al. (2021). Correlation between PD-L1 expression on CTCs and prognosis of patients with cancer: A systematic review and meta-analysis. Oncoimmunology 10 (1), 1938476. doi:10.1080/2162402x.2021.1938476
Kuipers, J., Tuncel, M. A., Ferreira, P., Jahn, K., and Beerenwinkel, N. (2020). Single-cell copy number calling and event history reconstruction. BioRxiv.
Kulavi, S., Ghosh, C., Saha, M., and Chatterjee, S. (2021). One size does not fit all: An overview of personalized treatment in cancer. JPRI, 87–103. doi:10.9734/jpri/2021/v33i28a31513
Kurien, B. T., and Scofield, R. H. (2015). Western blotting: An introduction. Methods Mol. Biol. 1312, 17–30. doi:10.1007/978-1-4939-2694-7_5
Lane, A. N., Higashi, R. M., and Fan, T. W-M. (2019). NMR and MS-based stable isotope-resolved metabolomics and applications in cancer metabolism. Trends Anal. Chem. 120, 115322. doi:10.1016/j.trac.2018.11.020
Lau, B. T., Almeda, A., Schauer, M., McNamara, M., Bai, X., Meng, Q., et al. (2022). Single molecule methylation profiles of cell-free DNA in cancer with nanopore sequencing. BioRxiv.
Lecharpentier, A., Vielh, P., Perez-Moreno, P., Planchard, D., Soria, J. C., and Farace, F. (2011). Detection of circulating tumour cells with a hybrid (epithelial/mesenchymal) phenotype in patients with metastatic non-small cell lung cancer. Br. J. Cancer 105 (9), 1338–1341. doi:10.1038/bjc.2011.405
Lee, B., Mahmud, I., Marchica, J., Dereziński, P., Qi, F., Wang, F., et al. (2020). Integrated RNA and metabolite profiling of urine liquid biopsies for prostate cancer biomarker discovery. Sci. Rep. 10 (1), 3716. doi:10.1038/s41598-020-60616-z
Lee, H. W., Lee, J-I., Lee, S. J., Cho, H. J., Song, H. J., Jeong, D. E., et al. (2015). Patient-derived xenografts from non-small cell lung cancer brain metastases are valuable translational platforms for the development of personalized targeted therapy. Clin. Cancer Res. 21 (5), 1172–1182. doi:10.1158/1078-0432.CCR-14-1589
Lequin, R. M. (2005). Enzyme immunoassay (EIA)/enzyme-linked immunosorbent assay (ELISA). Clin. Chem. 51 (12), 2415–2418. doi:10.1373/clinchem.2005.051532
Li, H., Jing, C., Wu, J., Ni, J., Sha, H., Xu, X., et al. (2019). Circulating tumor DNA detection: A potential tool for colorectal cancer management. Oncol. Lett. 17 (2), 1409–1416. doi:10.3892/ol.2018.9794
Li, H., Li, M., Guo, H., Lin, G., Huang, Q., and Qiu, M. (2022). Integrative analyses of circulating mRNA and lncRNA expression profile in plasma of lung cancer patients. Front. Oncol. 12, 843054. doi:10.3389/fonc.2022.843054
Li, Y., Ma, Y., Wu, Z., Zeng, F., Song, B., Zhang, Y., et al. (2021). Tumor mutational burden predicting the efficacy of immune checkpoint inhibitors in colorectal cancer: A systematic review and meta-analysis. Front. Immunol. 12, 751407. doi:10.3389/fimmu.2021.751407
Li, Z., Wang, Z., Tang, Y., Lu, X., Chen, J., Dong, Y., et al. (2019). Liquid biopsy-based single-cell metabolic phenotyping of lung cancer patients for informative diagnostics. Nat. Commun. 10 (1), 3856. doi:10.1038/s41467-019-11808-3
Lim, M., Park, S., Jeong, H-O., Park, S. H., Kumar, S., Jang, A., et al. (2021). Circulating tumor cell clusters are cloaked with platelets and correlate with poor prognosis in unresectable pancreatic cancer. Cancers (Basel) 13 (21), 5272. doi:10.3390/cancers13215272
Lin, K. K., Harrell, M. I., Oza, A. M., Oaknin, A., Ray-Coquard, I., Tinker, A. V., et al. (2019). BRCA reversion mutations in circulating tumor DNA predict primary and acquired resistance to the PARP inhibitor rucaparib in high-grade ovarian carcinoma. Cancer Discov. 9 (2), 210–219. doi:10.1158/2159-8290.CD-18-0715
Liu, F., Li, T., Hu, P., and Dai, L. (2021). Upregulation of serum miR-629 predicts poor prognosis for non-small-cell lung cancer. Dis. Markers 2021, 8819934. doi:10.1155/2021/8819934
Liu, M. C., Oxnard, G. R., Klein, E. A., Swanton, C., and Seiden, M. V.CCGA Consortium (2020). Sensitive and specific multi-cancer detection and localization using methylation signatures in cell-free DNA. Ann. Oncol. 31 (6), 745–759. doi:10.1016/j.annonc.2020.02.011
Liu, X., Li, J., Cadilha, B. L., Markota, A., Voigt, C., Huang, Z., et al. (2019). Epithelial-type systemic breast carcinoma cells with a restricted mesenchymal transition are a major source of metastasis. Sci. Adv. 5 (6), eaav4275. doi:10.1126/sciadv.aav4275
Liu, X., Taftaf, R., Kawaguchi, M., Chang, Y-F., Chen, W., Entenberg, D., et al. (2019). Homophilic CD44 interactions mediate tumor cell aggregation and polyclonal metastasis in patient-derived breast cancer models. Cancer Discov. 9 (1), 96–113. doi:10.1158/2159-8290.CD-18-0065
Liu, Y., Zhang, Y., Ding, Y., and Zhuang, R. (2021). Platelet-mediated tumor metastasis mechanism and the role of cell adhesion molecules. Crit. Rev. Oncol. Hematol. 167, 103502. doi:10.1016/j.critrevonc.2021.103502
Lo, Y. M. D., Han, D. S. C., Jiang, P., and Chiu, R. W. K. (2021). Epigenetics, fragmentomics, and topology of cell-free DNA in liquid biopsies. Science 372 (6538), eaaw3616. doi:10.1126/science.aaw3616
Londra, D., Mastoraki, S., Bournakis, E., Zavridou, M., Thanos, A., Rampias, T., et al. (2021). USP44 promoter methylation in plasma cell-free DNA in prostate cancer. Cancers (Basel) 13 (18), 4607. doi:10.3390/cancers13184607
Lone, S. N., Nisar, S., Masoodi, T., Singh, M., Rizwan, A., Hashem, S., et al. (2022). Liquid biopsy: A step closer to transform diagnosis, prognosis and future of cancer treatments. Mol. Cancer 21 (1), 79. doi:10.1186/s12943-022-01543-7
Loreth, D., Schuette, M., Zinke, J., Mohme, M., Piffko, A., Schneegans, S., et al. (2021). CD74 and CD44 expression on CTCs in cancer patients with brain metastasis. Int. J. Mol. Sci. 22 (13), 6993. doi:10.3390/ijms22136993
Lu, J., Getz, G., Miska, E. A., Alvarez-Saavedra, E., Lamb, J., Peck, D., et al. (2005). MicroRNA expression profiles classify human cancers. Nature 435 (7043), 834–838. doi:10.1038/nature03702
Lu, S., Chang, C-J., Guan, Y., Szafer-Glusman, E., Punnoose, E., Do, A., et al. (2020). Genomic analysis of circulating tumor cells at the single-cell level. J. Mol. Diagn 22 (6), 770–781. doi:10.1016/j.jmoldx.2020.02.013
Macaulay, I. C., Haerty, W., Kumar, P., Li, Y. I., Hu, T. X., Teng, M. J., et al. (2015). G&T-seq: Parallel sequencing of single-cell genomes and transcriptomes. Nat. Methods 12 (6), 519–522. doi:10.1038/nmeth.3370
Maheswaran, S., Sequist, L. V., Nagrath, S., Ulkus, L., Brannigan, B., Collura, C. V., et al. (2008). Detection of mutations in EGFR in circulating lung-cancer cells. N. Engl. J. Med. 359 (4), 366–377. doi:10.1056/NEJMoa0800668
Maia, J., Caja, S., Strano Moraes, M. C., Couto, N., and Costa-Silva, B. (2018). Exosome-based cell-cell communication in the tumor microenvironment. Front. Cell Dev. Biol. 6, 18. doi:10.3389/fcell.2018.00018
Mandel, P., and Metais, P. (1948). Les acides nucléiques du plasma sanguin chez l’Homme. C R. Seances Soc. Biol. Fil. 142 (3–4), 241–243.
Manier, S., Park, J., Capelletti, M., Bustoros, M., Freeman, S. S., Ha, G., et al. (2018). Whole-exome sequencing of cell-free DNA and circulating tumor cells in multiple myeloma. Nat. Commun. 9 (1), 1691. doi:10.1038/s41467-018-04001-5
Mannavola, F., Salerno, T., Passarelli, A., Tucci, M., Internò, V., and Silvestris, F. (2019). Revisiting the role of exosomes in colorectal cancer: Where are we now? Front. Oncol. 9, 521. doi:10.3389/fonc.2019.00521
Månsson, C. T., Vad-Nielsen, J., Meldgaard, P., Nielsen, A. L., and Sorensen, B. S. (2021). EGFR transcription in non-small-cell lung cancer tumours can be revealed in ctDNA by cell-free chromatin immunoprecipitation (cfChIP). Mol. Oncol. 15 (11), 2868–2876. doi:10.1002/1878-0261.13093
Markou, Α., Londra, D., Tserpeli, V., Kollias, Ι., Tsaroucha, E., Vamvakaris, I., et al. (2022). DNA methylation analysis of tumor suppressor genes in liquid biopsy components of early stage NSCLC: A promising tool for early detection. Clin. Epigenetics 14 (1), 61. doi:10.1186/s13148-022-01283-x
Martinez-Garcia, E., Lesur, A., Devis, L., Cabrera, S., Matias-Guiu, X., Hirschfeld, M., et al. (2017). Targeted proteomics identifies proteomic signatures in liquid biopsies of the endometrium to diagnose endometrial cancer and assist in the prediction of the optimal surgical treatment. Clin. Cancer Res. 23 (21), 6458–6467. doi:10.1158/1078-0432.CCR-17-0474
Maslov, D. L., Trifonova, O. P., Lichtenberg, S., Balashova, E. E., Mamedli, Z. Z., Alferov, A. A., et al. (2022). Blood plasma metabolome profiling at different stages of renal cell carcinoma. Cancers (Basel) 15 (1), 140. doi:10.3390/cancers15010140
Mathivanan, S., and Simpson, R. J. (2009). ExoCarta: A compendium of exosomal proteins and RNA. Proteomics 9 (21), 4997–5000. doi:10.1002/pmic.200900351
McKiernan, J., Donovan, M. J., O’Neill, V., Bentink, S., Noerholm, M., Belzer, S., et al. (2016). A novel urine exosome gene expression assay to predict high-grade prostate cancer at initial biopsy. JAMA Oncol. 2 (7), 882–889. doi:10.1001/jamaoncol.2016.0097
Meissner, A., Gnirke, A., Bell, G. W., Ramsahoye, B., Lander, E. S., and Jaenisch, R. (2005). Reduced representation bisulfite sequencing for comparative high-resolution DNA methylation analysis. Nucleic Acids Res. 33 (18), 5868–5877. doi:10.1093/nar/gki901
Melo, S. A., Luecke, L. B., Kahlert, C., Fernandez, A. F., Gammon, S. T., Kaye, J., et al. (2015). Glypican-1 identifies cancer exosomes and detects early pancreatic cancer. Nature 523 (7559), 177–182. doi:10.1038/nature14581
Miller, A. M., Shah, R. H., Pentsova, E. I., Pourmaleki, M., Briggs, S., Distefano, N., et al. (2019). Tracking tumour evolution in glioma through liquid biopsies of cerebrospinal fluid. Nature 565 (7741), 654–658. doi:10.1038/s41586-019-0882-3
Mitchell, P. S., Parkin, R. K., Kroh, E. M., Fritz, B. R., Wyman, S. K., Pogosova-Agadjanyan, E. L., et al. (2008). Circulating microRNAs as stable blood-based markers for cancer detection. Proc. Natl. Acad. Sci. U. S. A. 105 (30), 10513–10518. doi:10.1073/pnas.0804549105
Miyamoto, D. T., Zheng, Y., Wittner, B. S., Lee, R. J., Zhu, H., Broderick, K. T., et al. (2015). RNA-Seq of single prostate CTCs implicates noncanonical Wnt signaling in antiandrogen resistance. Science 349 (6254), 1351–1356. doi:10.1126/science.aab0917
Moss, J., Magenheim, J., Neiman, D., Zemmour, H., Loyfer, N., Korach, A., et al. (2018). Comprehensive human cell-type methylation atlas reveals origins of circulating cell-free DNA in health and disease. Nat. Commun. 9 (1), 5068. doi:10.1038/s41467-018-07466-6
Mouliere, F., Chandrananda, D., Piskorz, A. M., Moore, E. K., Morris, J., Ahlborn, L. B., et al. (2018). Enhanced detection of circulating tumor DNA by fragment size analysis. Sci. Transl. Med. 10 (466), eaat4921. doi:10.1126/scitranslmed.aat4921
Müller, V., Banys-Paluchowski, M., Friedl, T. W. P., Fasching, P. A., Schneeweiss, A., Hartkopf, A., et al. (2021). Prognostic relevance of the HER2 status of circulating tumor cells in metastatic breast cancer patients screened for participation in the DETECT study program. ESMO Open 6 (6), 100299. doi:10.1016/j.esmoop.2021.100299
Nakamura, K., Zhu, Z., Roy, S., Jun, E., Han, H., Munoz, R. M., et al. (2022). An exosome-based transcriptomic signature for noninvasive, early detection of patients with pancreatic ductal adenocarcinoma: A multicenter cohort study. Gastroenterology 163 (5), 1252–1266.e2. doi:10.1053/j.gastro.2022.06.090
Negishi, R., Yamakawa, H., Kobayashi, T., Horikawa, M., Shimoyama, T., Koizumi, F., et al. (2022). Transcriptomic profiling of single circulating tumor cells provides insight into human metastatic gastric cancer. Commun. Biol. 5 (1), 20. doi:10.1038/s42003-021-02937-x
Newman, A. M., Bratman, S. V., To, J., Wynne, J. F., Eclov, N. C. W., Modlin, L. A., et al. (2014). An ultrasensitive method for quantitating circulating tumor DNA with broad patient coverage. Nat. Med. 20 (5), 548–554. doi:10.1038/nm.3519
Nguyen, D. X., Bos, P. D., and Massagué, J. (2009). Metastasis: From dissemination to organ-specific colonization. Nat. Rev. Cancer 9 (4), 274–284. doi:10.1038/nrc2622
Nguyen, H-Q., Lee, D., Kim, Y., Paek, M., Kim, M., Jang, K-S., et al. (2019). Platelet factor 4 as a novel exosome marker in MALDI-MS analysis of exosomes from human serum. Anal. Chem. 91 (20), 13297–13305. doi:10.1021/acs.analchem.9b04198
Nikanjam, M., Kato, S., and Kurzrock, R. (2022). Liquid biopsy: Current technology and clinical applications. J. Hematol. Oncol. 15 (1), 131. doi:10.1186/s13045-022-01351-y
Niwa, Y., Yamada, S., Sonohara, F., Kurimoto, K., Hayashi, M., Tashiro, M., et al. (2019). Identification of a serum-based miRNA signature for response of esophageal squamous cell carcinoma to neoadjuvant chemotherapy. J. Transl. Med. 17 (1), 1. doi:10.1186/s12967-018-1762-6
Ntzifa, A., Londra, D., Rampias, T., Kotsakis, A., Georgoulias, V., and Lianidou, E. (2021). DNA methylation analysis in plasma cell-free DNA and paired CTCs of NSCLC patients before and after osimertinib treatment. Cancers (Basel) 13 (23), 5974. doi:10.3390/cancers13235974
Nuzzo, P. V., Berchuck, J. E., Korthauer, K., Spisak, S., Nassar, A. H., Abou Alaiwi, S., et al. (2020). Detection of renal cell carcinoma using plasma and urine cell-free DNA methylomes. Nat. Med. 26 (7), 1041–1043. doi:10.1038/s41591-020-0933-1
Oda, M., Glass, J. L., Thompson, R. F., Mo, Y., Olivier, E. N., Figueroa, M. E., et al. (2009). High-resolution genome-wide cytosine methylation profiling with simultaneous copy number analysis and optimization for limited cell numbers. Nucleic Acids Res. 37 (12), 3829–3839. doi:10.1093/nar/gkp260
Omenn, G. S., States, D. J., Adamski, M., Blackwell, T. W., Menon, R., Hermjakob, H., et al. (2005). Overview of the HUPO plasma proteome project: Results from the pilot phase with 35 collaborating laboratories and multiple analytical groups, generating a core dataset of 3020 proteins and a publicly-available database. Proteomics 5 (13), 3226–3245. doi:10.1002/pmic.200500358
Otsubo, K., Sakai, K., Takeshita, M., Harada, D., Azuma, K., Ota, K., et al. (2019). Genetic profiling of non-small cell lung cancer at development of resistance to first- or second-generation EGFR-TKIs by CAPP-seq analysis of circulating tumor DNA. Oncologist 24 (8), 1022–1026. doi:10.1634/theoncologist.2019-0101
Ou, S-H. I., Nagasaka, M., and Zhu, V. W. (2018). Liquid biopsy to identify actionable genomic alterations. Am. Soc. Clin. Oncol. Educ. Book 38, 978–997. doi:10.1200/EDBK_199765
Oulhen, M., Pawlikowska, P., Tayoun, T., Garonzi, M., Buson, G., Forcato, C., et al. (2021). Circulating tumor cell copy-number heterogeneity in ALK-rearranged non-small-cell lung cancer resistant to ALK inhibitors. NPJ Precis. Oncol. 5 (1), 67. doi:10.1038/s41698-021-00203-1
Pailler, E., Faugeroux, V., Oulhen, M., Mezquita, L., Laporte, M., Honoré, A., et al. (2019). Acquired resistance mutations to ALK inhibitors identified by single circulating tumor cell sequencing in ALK-rearranged non-small-cell lung cancer. Clin. Cancer Res. 25 (22), 6671–6682. doi:10.1158/1078-0432.CCR-19-1176
Palanca-Ballester, C., Rodriguez-Casanova, A., Torres, S., Calabuig-Fariñas, S., Exposito, F., Serrano, D., et al. (2021). Cancer epigenetic biomarkers in liquid biopsy for high incidence malignancies. Cancers (Basel) 13 (12), 3016. doi:10.3390/cancers13123016
Panagopoulou, M., Karaglani, M., Balgkouranidou, I., Biziota, E., Koukaki, T., Karamitrousis, E., et al. (2019). Circulating cell-free DNA in breast cancer: Size profiling, levels, and methylation patterns lead to prognostic and predictive classifiers. Oncogene 38 (18), 3387–3401. doi:10.1038/s41388-018-0660-y
Pantel, K., Diaz, L. A., and Polyak, K. (2013). Tracking tumor resistance using “liquid biopsies”. Nat. Med. 19 (6), 676–677. doi:10.1038/nm.3233
Pantel, K., and Speicher, M. R. (2016). The biology of circulating tumor cells. Oncogene 35 (10), 1216–1224. doi:10.1038/onc.2015.192
Paoletti, C., Miao, J., Dolce, E. M., Darga, E. P., Repollet, M. I., Doyle, G. V., et al. (2019). Circulating tumor cell clusters in patients with metastatic breast cancer: A SWOG S0500 translational medicine study. Clin. Cancer Res. 25 (20), 6089–6097. doi:10.1158/1078-0432.CCR-19-0208
Parikh, A. R., Van Seventer, E. E., Siravegna, G., Hartwig, A. V., Jaimovich, A., He, Y., et al. (2021). Minimal residual disease detection using a plasma-only circulating tumor DNA assay in patients with colorectal cancer. Clin. Cancer Res. 27 (20), 5586–5594. doi:10.1158/1078-0432.CCR-21-0410
Peeters, D. J. E., van Dam, P. J., Van den Eynden, G. G. M., Rutten, A., Wuyts, H., Pouillon, L., et al. (2014). Detection and prognostic significance of circulating tumour cells in patients with metastatic breast cancer according to immunohistochemical subtypes. Br. J. Cancer 110 (2), 375–383. doi:10.1038/bjc.2013.743
Peneder, P., Stütz, A. M., Surdez, D., Krumbholz, M., Semper, S., Chicard, M., et al. (2021). Multimodal analysis of cell-free DNA whole-genome sequencing for pediatric cancers with low mutational burden. Nat. Commun. 12 (1), 3230. doi:10.1038/s41467-021-23445-w
Pereira-Veiga, T., Schneegans, S., Pantel, K., and Wikman, H. (2022). Circulating tumor cell-blood cell crosstalk: Biology and clinical relevance. Cell Rep. 40 (9), 111298. doi:10.1016/j.celrep.2022.111298
Peter, M. R., Bilenky, M., Isserlin, R., Bader, G. D., Shen, S. Y., De Carvalho, D. D., et al. (2020). Dynamics of the cell-free DNA methylome of metastatic castration resistant prostate cancer during androgen-targeting treatment. BioRxiv.
Peter, M. R., Bilenky, M., Shi, Y., Pu, J., Kamdar, S., Hansen, A. R., et al. (2022). A novel methylated cell-free DNA marker panel to monitor treatment response in metastatic prostate cancer. Epigenomics 14 (13), 811–822. doi:10.2217/epi-2022-0103
Picelli, S., Björklund, Å. K., Faridani, O. R., Sagasser, S., Winberg, G., and Sandberg, R. (2013). Smart-seq2 for sensitive full-length transcriptome profiling in single cells. Nat. Methods 10 (11), 1096–1098. doi:10.1038/nmeth.2639
Pinzani, P., D’Argenio, V., Del Re, M., Pellegrini, C., Cucchiara, F., Salvianti, F., et al. (2021). Updates on liquid biopsy: Current trends and future perspectives for clinical application in solid tumors. Clin. Chem. Lab. Med. 59 (7), 1181–1200. doi:10.1515/cclm-2020-1685
Ponnusamy, L., Mahalingaiah, P. K. S., Chang, Y-W., and Singh, K. P. (2019). Role of cellular reprogramming and epigenetic dysregulation in acquired chemoresistance in breast cancer. Cancer Drug Resist 2 (2), 297–312. doi:10.20517/cdr.2018.11
Ponomaryova, A. A., Rykova, E. Y., Cherdyntseva, N. V., Skvortsova, T. E., Dobrodeev, A. Y., Zav’yalov, A. A., et al. (2013). Potentialities of aberrantly methylated circulating DNA for diagnostics and post-treatment follow-up of lung cancer patients. Lung Cancer 81 (3), 397–403. doi:10.1016/j.lungcan.2013.05.016
Puhka, M., Takatalo, M., Nordberg, M-E., Valkonen, S., Nandania, J., Aatonen, M., et al. (2017). Metabolomic profiling of extracellular vesicles and alternative normalization methods reveal enriched metabolites and strategies to study prostate cancer-related changes. Theranostics 7 (16), 3824–3841. doi:10.7150/thno.19890
Pulverer, W., Kruusmaa, K., Schönthaler, S., Huber, J., Bitenc, M., Bachleitner-Hofmann, T., et al. (2021). Multiplexed DNA methylation analysis in colorectal cancer using liquid biopsy and its diagnostic and predictive value. Curr. Issues Mol. Biol. 43 (3), 1419–1435. doi:10.3390/cimb43030100
Qu, F., Xie, W., Nakabayashi, M., Zhang, H., Jeong, S. H., Wang, X., et al. (2017). Association of AR-V7 and prostate-specific antigen RNA levels in blood with efficacy of abiraterone acetate and enzalutamide treatment in men with prostate cancer. Clin. Cancer Res. 23 (3), 726–734. doi:10.1158/1078-0432.CCR-16-1070
Quirico, L., and Orso, F. (2020). The power of microRNAs as diagnostic and prognostic biomarkers in liquid biopsies. Cancer Drug Resist 21, 117. doi:10.20517/cdr.2019.103
Reda El Sayed, S., Cristante, J., Guyon, L., Denis, J., Chabre, O., and Cherradi, N. (2021). Microrna therapeutics in cancer: Current advances and challenges. Cancers (Basel) 13 (11), 2680. doi:10.3390/cancers13112680
Rodrigues, G., Hoshino, A., Kenific, C. M., Matei, I. R., Steiner, L., Freitas, D., et al. (2019). Tumour exosomal CEMIP protein promotes cancer cell colonization in brain metastasis. Nat. Cell Biol. 21 (11), 1403–1412. doi:10.1038/s41556-019-0404-4
Roman-Canal, B., Tarragona, J., Moiola, C. P., Gatius, S., Bonnin, S., Ruiz-Miró, M., et al. (2019). EV-associated miRNAs from peritoneal lavage as potential diagnostic biomarkers in colorectal cancer. J. Transl. Med. 17 (1), 208. doi:10.1186/s12967-019-1954-8
Rossi, T., Vergara, D., Fanini, F., Maffia, M., Bravaccini, S., and Pirini, F. (2020). Microbiota-derived metabolites in tumor progression and metastasis. Int. J. Mol. Sci. 21 (16), 5786. doi:10.3390/ijms21165786
Roweth, H. G., and Battinelli, E. M. (2021). Lessons to learn from tumor-educated platelets. Blood 137 (23), 3174–3180. doi:10.1182/blood.2019003976
Ruano, A. P. C., Gadelha Guimarães, A. P., Braun, A. C., Flores, B. C. T. C. P., Tariki, M. S., Abdallah, E. A., et al. (2022). Fusion cell markers in circulating tumor cells from patients with high-grade ovarian serous carcinoma. Int. J. Mol. Sci. 23 (23), 14687. doi:10.3390/ijms232314687
ruyenaere, P., Offner, F., and Vandesompele, J. (2021). Circulating RNA biomarkers in diffuse large B-cell lymphoma: A systematic review. Exp. Hematol. Oncol. 10 (1), 13. doi:10.1186/s40164-021-00208-3
Sabato, C., Noviello, T. M. R., Covre, A., Coral, S., Caruso, F. P., Besharat, Z. M., et al. (2022). A novel microRNA signature for the detection of melanoma by liquid biopsy. J. Transl. Med. 20 (1), 469. doi:10.1186/s12967-022-03668-1
Sadeh, R., Sharkia, I., Fialkoff, G., Rahat, A., Gutin, J., Chappleboim, A., et al. (2021). ChIP-seq of plasma cell-free nucleosomes identifies gene expression programs of the cells of origin. Nat. Biotechnol. 39 (5), 586–598. doi:10.1038/s41587-020-00775-6
Saito, R., Yoshimura, K., Shoda, K., Furuya, S., Akaike, H., Kawaguchi, Y., et al. (2021). Diagnostic significance of plasma lipid markers and machine learning-based algorithm for gastric cancer. Oncol. Lett. 21 (5), 405. doi:10.3892/ol.2021.12666
Salvianti, F., Gelmini, S., Costanza, F., Mancini, I., Sonnati, G., Simi, L., et al. (2020). The pre-analytical phase of the liquid biopsy. N. Biotechnol. 55, 19–29. doi:10.1016/j.nbt.2019.09.006
Sarvin, B., Lagziel, S., Sarvin, N., Mukha, D., Kumar, P., Aizenshtein, E., et al. (2020). Fast and sensitive flow-injection mass spectrometry metabolomics by analyzing sample-specific ion distributions. Nat. Commun. 11 (1), 3186. doi:10.1038/s41467-020-17026-6
Satija, R., Farrell, J. A., Gennert, D., Schier, A. F., and Regev, A. (2015). Spatial reconstruction of single-cell gene expression data. Nat. Biotechnol. 33 (5), 495–502. doi:10.1038/nbt.3192
Sawyers, C. L. (2008). The cancer biomarker problem. Nature 452 (7187), 548–552. doi:10.1038/nature06913
Scherer, F., Kurtz, D. M., Newman, A. M., Stehr, H., Craig, A. F. M., Esfahani, M. S., et al. (2016). Distinct biological subtypes and patterns of genome evolution in lymphoma revealed by circulating tumor DNA. Sci. Transl. Med. 8 (364), 364ra155. doi:10.1126/scitranslmed.aai8545
Schmidt, B., Beyer, J., Dietrich, D., Bork, I., Liebenberg, V., and Fleischhacker, M. (2015). Quantification of cell-free mSHOX2 Plasma DNA for therapy monitoring in advanced stage non-small cell (NSCLC) and small-cell lung cancer (SCLC) patients. PLoS ONE 10 (2), e0118195. doi:10.1371/journal.pone.0118195
Schmidt, D. R., Patel, R., Kirsch, D. G., Lewis, C. A., Vander Heiden, M. G., and Locasale, J. W. (2021). Metabolomics in cancer research and emerging applications in clinical oncology. CA Cancer J. Clin. 71 (4), 333–358. doi:10.3322/caac.21670
Schøler, L. V., Reinert, T., Ørntoft, M. W., Kassentoft, C. G., Árnadóttir, S. S., Vang, S., et al. (2017). Clinical implications of monitoring circulating tumor DNA in patients with colorectal cancer. Clin. Cancer Res. 23 (18), 5437–5445. doi:10.1158/1078-0432.CCR-17-0510
Schraa, S. J., van Rooijen, K. L., Koopman, M., Vink, G. R., and Fijneman, R. J. A. (2022). Cell-free circulating (tumor) DNA before surgery as a prognostic factor in non-metastatic colorectal cancer: A systematic review. Cancers (Basel) 14 (9), 2218. doi:10.3390/cancers14092218
Schuster, E., Taftaf, R., Reduzzi, C., Albert, M. K., Romero-Calvo, I., and Liu, H. (2021). Better together: Circulating tumor cell clustering in metastatic cancer. Trends Cancer 7 (11), 1020–1032. doi:10.1016/j.trecan.2021.07.001
Serre, D., Lee, B. H., and Ting, A. H. (2010). MBD-isolated Genome Sequencing provides a high-throughput and comprehensive survey of DNA methylation in the human genome. Nucleic Acids Res. 38 (2), 391–399. doi:10.1093/nar/gkp992
Shaheed, S-U., Tait, C., Kyriacou, K., Mullarkey, J., Burrill, W., Patterson, L. H., et al. (2017). Nipple aspirate fluid-A liquid biopsy for diagnosing breast health. Proteomics Clin. Appl. 11 (9–10), 1700015. doi:10.1002/prca.201700015
Shen, S. Y., Burgener, J. M., Bratman, S. V., and De Carvalho, D. D. (2019). Preparation of cfMeDIP-seq libraries for methylome profiling of plasma cell-free DNA. Nat. Protoc. 14 (10), 2749–2780. doi:10.1038/s41596-019-0202-2
Shidfar, A., Fatokun, T., Ivancic, D., Chatterton, R. T., Khan, S. A., and Wang, J. (2016). Protein biomarkers for breast cancer risk are specifically correlated with local steroid hormones in nipple aspirate fluid. Horm. Cancer 7 (4), 252–259. doi:10.1007/s12672-016-0264-3
Shin, S., Jo, S-S., Charton, C., Kim, S., Lim, H., Kim, S., et al. (2022). Integrative analysis of plasma cell-free DNA fragmentation and methylation patterns for colorectal cancer detection. JCO 40 (16), e15022. doi:10.1200/jco.2022.40.16_suppl.e15022
Shtumpf, M., Piroeva, K. V., Agrawal, S. P., Jacob, D. R., and Teif, V. B. (2022). NucPosDB: A database of nucleosome positioning in vivo and nucleosomics of cell-free DNA. Chromosoma 131 (1–2), 19–28. doi:10.1007/s00412-021-00766-9
Signore, M., Alfonsi, R., Federici, G., Nanni, S., Addario, A., Bertuccini, L., et al. (2021). Diagnostic and prognostic potential of the proteomic profiling of serum-derived extracellular vesicles in prostate cancer. Cell Death Dis. 12 (7), 636. doi:10.1038/s41419-021-03909-z
Sinclair, K., and Dudley, E. (2019). Metabolomics and biomarker discovery. Adv. Exp. Med. Biol. 1140, 613–633. doi:10.1007/978-3-030-15950-4_37
Sinkala, E., Sollier-Christen, E., Renier, C., Rosàs-Canyelles, E., Che, J., Heirich, K., et al. (2017). Profiling protein expression in circulating tumour cells using microfluidic Western blotting. Nat. Commun. 8, 14622. doi:10.1038/ncomms14622
Siravegna, G., Marsoni, S., Siena, S., and Bardelli, A. (2017). Integrating liquid biopsies into the management of cancer. Nat. Rev. Clin. Oncol. 14 (9), 531–548. doi:10.1038/nrclinonc.2017.14
Snyder, M. W., Kircher, M., Hill, A. J., Daza, R. M., and Shendure, J. (2016). Cell-free DNA comprises an in vivo nucleosome footprint that informs its tissues-of-origin. Cell 164 (1–2), 57–68. doi:10.1016/j.cell.2015.11.050
Song, C-X., Szulwach, K. E., Fu, Y., Dai, Q., Yi, C., Li, X., et al. (2011). Selective chemical labeling reveals the genome-wide distribution of 5-hydroxymethylcytosine. Nat. Biotechnol. 29 (1), 68–72. doi:10.1038/nbt.1732
Song, C-X., Yin, S., Ma, L., Wheeler, A., Chen, Y., Zhang, Y., et al. (2017). 5-Hydroxymethylcytosine signatures in cell-free DNA provide information about tumor types and stages. Cell Res. 27 (10), 1231–1242. doi:10.1038/cr.2017.106
Song, J., Merbs, S. L., Sokoll, L. J., Chan, D. W., and Zhang, Z. (2019). A multiplex immunoassay of serum biomarkers for the detection of uveal melanoma. Clin. Proteomics 16, 10. doi:10.1186/s12014-019-9230-8
Spits, C., Le Caignec, C., De Rycke, M., Van Haute, L., Van Steirteghem, A., Liebaers, I., et al. (2006). Whole-genome multiple displacement amplification from single cells. Nat. Protoc. 1 (4), 1965–1970. doi:10.1038/nprot.2006.326
Spitzer, M. H., and Nolan, G. P. (2016). Mass cytometry: Single cells, many features. Cell 165 (4), 780–791. doi:10.1016/j.cell.2016.04.019
Stelzer, I. A., Ghaemi, M. S., Han, X., Ando, K., Hédou, J. J., Feyaerts, D., et al. (2021). Integrated trajectories of the maternal metabolome, proteome, and immunome predict labor onset. Sci. Transl. Med. 13 (592), eabd9898. doi:10.1126/scitranslmed.abd9898
Sturgeon, C. M., Lai, L. C., and Duffy, M. J. (2009). Serum tumour markers: How to order and interpret them. BMJ 339, b3527. doi:10.1136/bmj.b3527
Sun, K., Jiang, P., Cheng, S. H., Cheng, T. H. T., Wong, J., Wong, V. W. S., et al. (2019). Orientation-aware plasma cell-free DNA fragmentation analysis in open chromatin regions informs tissue of origin. Genome Res. 29 (3), 418–427. doi:10.1101/gr.242719.118
Sun, N., Lee, Y-T., Zhang, R. Y., Kao, R., Teng, P-C., Yang, Y., et al. (2020). Purification of HCC-specific extracellular vesicles on nanosubstrates for early HCC detection by digital scoring. Nat. Commun. 11 (1), 4489. doi:10.1038/s41467-020-18311-0
Sun, Y-F., Wu, L., Liu, S-P., Jiang, M-M., Hu, B., Zhou, K-Q., et al. (2021). Dissecting spatial heterogeneity and the immune-evasion mechanism of CTCs by single-cell RNA-seq in hepatocellular carcinoma. Nat. Commun. 12 (1), 4091. doi:10.1038/s41467-021-24386-0
Sundaresan, T. K., Sequist, L. V., Heymach, J. V., Riely, G. J., Jänne, P. A., Koch, W. H., et al. (2016). Detection of T790M, the acquired resistance EGFR mutation, by tumor biopsy versus noninvasive blood-based analyses. Clin. Cancer Res. 22 (5), 1103–1110. doi:10.1158/1078-0432.CCR-15-1031
Supplitt, S., Karpinski, P., Sasiadek, M., and Laczmanska, I. (2021). Current achievements and applications of transcriptomics in personalized cancer medicine. Int. J. Mol. Sci. 22 (3), 1422. doi:10.3390/ijms22031422
Suvilesh, K. N., Nussbaum, Y. I., Radhakrishnan, V., Manjunath, Y., Avella, D. M., Staveley-O’Carroll, K. F., et al. (2022). Tumorigenic circulating tumor cells from xenograft mouse models of non-metastatic NSCLC patients reveal distinct single cell heterogeneity and drug responses. Mol. Cancer 21 (1), 73. doi:10.1186/s12943-022-01553-5
Svensson, C-M., Hübler, R., and Figge, M. T. (2015). Automated classification of circulating tumor cells and the impact of interobsever variability on classifier training and performance. J. Immunol. Res. 2015, 573165. doi:10.1155/2015/573165
Taiwo, O., Wilson, G. A., Morris, T., Seisenberger, S., Reik, W., Pearce, D., et al. (2012). Methylome analysis using MeDIP-seq with low DNA concentrations. Nat. Protoc. 7 (4), 617–636. doi:10.1038/nprot.2012.012
Taklifi, P., Palizban, F., and Mehrmohamadi, M. (2022). Integrating chromatin accessibility states in the design of targeted sequencing panels for liquid biopsy. Sci. Rep. 12 (1), 10447. doi:10.1038/s41598-022-14675-z
Talevich, E., Shain, A. H., Botton, T., and Bastian, B. C. (2016). CNVkit: Genome-Wide copy number detection and visualization from targeted DNA sequencing. PLoS Comput. Biol. 12 (4), e1004873. doi:10.1371/journal.pcbi.1004873
Tan, C. R. C., Zhou, L., and El-Deiry, W. S. (2016). Circulating tumor cells versus circulating tumor DNA in colorectal cancer: Pros and cons. Curr. Colorectal Cancer Rep. 12 (3), 151–161. doi:10.1007/s11888-016-0320-y
Taylor, A. M., Shih, J., Ha, G., Gao, G. F., Zhang, X., Berger, A. C., et al. (2018). Genomic and functional approaches to understanding cancer aneuploidy. Cancer Cell 33 (4), 676–689.e3. doi:10.1016/j.ccell.2018.03.007
Telenius, H., Carter, N. P., Bebb, C. E., Nordenskjöld, M., Ponder, B. A., and Tunnacliffe, A. (1992). Degenerate oligonucleotide-primed PCR: General amplification of target DNA by a single degenerate primer. Genomics 13 (3), 718–725. doi:10.1016/0888-7543(92)90147-k
Thompson, J. C., Yee, S. S., Troxel, A. B., Savitch, S. L., Fan, R., Balli, D., et al. (2016). Detection of therapeutically targetable driver and resistance mutations in lung cancer patients by next-generation sequencing of cell-free circulating tumor DNA. Clin. Cancer Res. 22 (23), 5772–5782. doi:10.1158/1078-0432.CCR-16-1231
Tian, X-P., Huang, W-J., Huang, H-Q., Liu, Y-H., Wang, L., Zhang, X., et al. (2019). Prognostic and predictive value of a microRNA signature in adults with T-cell lymphoblastic lymphoma. Leukemia 33 (10), 2454–2465. doi:10.1038/s41375-019-0466-0
Tie, J., Wang, Y., Tomasetti, C., Li, L., Springer, S., Kinde, I., et al. (2016). Circulating tumor DNA analysis detects minimal residual disease and predicts recurrence in patients with stage II colon cancer. Sci. Transl. Med. 8 (346), 346ra92. doi:10.1126/scitranslmed.aaf6219
Tucci, M., D’Oronzo, S., Mannavola, F., Felici, C., Lovero, D., Cafforio, P., et al. (2020). Dual-procedural aration of CTCs in cutaneous melanoma provides useful information for both molecular diagnosis and prognosis. Ther. Adv. Med. Oncol. 12, 1758835920905415. doi:10.1177/1758835920905415
Turner, N. C., Kingston, B., Kilburn, L. S., Kernaghan, S., Wardley, A. M., Macpherson, I. R., et al. (2020). Circulating tumour DNA analysis to direct therapy in advanced breast cancer (plasmaMATCH): A multicentre, multicohort, phase 2a, platform trial. Lancet Oncol. 21 (10), 1296–1308. doi:10.1016/S1470-2045(20)30444-7
Uchiyama, K., Yagi, N., Mizushima, K., Higashimura, Y., Hirai, Y., Okayama, T., et al. (2017). Serum metabolomics analysis for early detection of colorectal cancer. J. Gastroenterol. 52 (6), 677–694. doi:10.1007/s00535-016-1261-6
Urabe, F., Matsuzaki, J., Yamamoto, Y., Kimura, T., Hara, T., Ichikawa, M., et al. (2019). Large-scale circulating microRNA profiling for the liquid biopsy of prostate cancer. Clin. Cancer Res. 25 (10), 3016–3025. doi:10.1158/1078-0432.CCR-18-2849
Vaisvila, R., Ponnaluri, V. K. C., Sun, Z., Langhorst, B. W., Saleh, L., Guan, S., et al. (2021). Enzymatic methyl sequencing detects DNA methylation at single-base resolution from picograms of DNA. Genome Res. 31 (7), 1280–1289. doi:10.1101/gr.266551.120
Van Loo, P., Nordgard, S. H., Lingjærde, O. C., Russnes, H. G., Rye, I. H., Sun, W., et al. (2010). Allele-specific copy number analysis of tumors. Proc. Natl. Acad. Sci. U. S. A. 107 (39), 16910–16915. doi:10.1073/pnas.1009843107
Van Paemel, R., De Koker, A., Vandeputte, C., van Zogchel, L., Lammens, T., Laureys, G., et al. (2021). Minimally invasive classification of paediatric solid tumours using reduced representation bisulphite sequencing of cell-free DNA: A proof-of-principle study. Epigenetics 16 (2), 196–208. doi:10.1080/15592294.2020.1790950
Vasanthakumar, A., and Godley, L. A. (2015). 5-hydroxymethylcytosine in cancer: Significance in diagnosis and therapy. Cancer Genet. 208 (5), 167–177. doi:10.1016/j.cancergen.2015.02.009
Velez, G., Nguyen, H. V., Chemudupati, T., Ludwig, C. A., Toral, M., Reddy, S., et al. (2021). Liquid biopsy proteomics of uveal melanoma reveals biomarkers associated with metastatic risk. Mol. Cancer 20 (1), 39. doi:10.1186/s12943-021-01336-4
Vessies, D. C. L., Greuter, M. J. E., van Rooijen, K. L., Linders, T. C., Lanfermeijer, M., Ramkisoensing, K. L., et al. (2020). Performance of four platforms for KRAS mutation detection in plasma cell-free DNA: ddPCR, idylla, COBAS z480 and BEAMing. Sci. Rep. 10 (1), 8122. doi:10.1038/s41598-020-64822-7
Vishnoi, M., Liu, N. H., Yin, W., Boral, D., Scamardo, A., Hong, D., et al. (2019). The identification of a TNBC liver metastasis gene signature by sequential CTC-xenograft modeling. Mol. Oncol. 13 (9), 1913–1926. doi:10.1002/1878-0261.12533
Vrba, L., Oshiro, M. M., Kim, S. S., Garland, L. L., Placencia, C., Mahadevan, D., et al. (2020). DNA methylation biomarkers discovered in silico detect cancer in liquid biopsies from non-small cell lung cancer patients. Epigenetics 15 (4), 419–430. doi:10.1080/15592294.2019.1695333
Wada, Y., Shimada, M., Murano, T., Takamaru, H., Morine, Y., Ikemoto, T., et al. (2021). A liquid biopsy assay for noninvasive identification of lymph node metastases in T1 colorectal cancer. Gastroenterology 161 (1), 151–162.e1. doi:10.1053/j.gastro.2021.03.062
Walker, N. J., Rashid, M., Yu, S., Bignell, H., Lumby, C. K., Livi, C. M., et al. (2022). Hydroxymethylation profile of cell-free DNA is a biomarker for early colorectal cancer. Sci. Rep. 12 (1), 16566. doi:10.1038/s41598-022-20975-1
Wang, R., Lin, D-Y., and Jiang, Y. (2020). Scope: A normalization and copy-number estimation method for single-cell DNA sequencing. Cell Syst. 10 (5), 445–452.e6. doi:10.1016/j.cels.2020.03.005
Wang, Y., Li, L., Cohen, J. D., Kinde, I., Ptak, J., Popoli, M., et al. (2019). Prognostic potential of circulating tumor DNA measurement in postoperative surveillance of nonmetastatic colorectal cancer. JAMA Oncol. 5 (8), 1118–1123. doi:10.1001/jamaoncol.2019.0512
Wang, Z., Jiang, W., Wang, Y., Guo, Y., Cong, Z., Du, F., et al. (2015). MGMT promoter methylation in serum and cerebrospinal fluid as a tumor-specific biomarker of glioma. Biomed. Rep. 3 (4), 543–548. doi:10.3892/br.2015.462
Warburg, O., Wind, F., and Negelein, E. (1927). The metabolism of tumors in the body. J. Gen. Physiol. 8 (6), 519–530. doi:10.1085/jgp.8.6.519
Wolf, F. A., Angerer, P., and Theis, F. J. (2018). Scanpy: Large-scale single-cell gene expression data analysis. Genome Biol. 19 (1), 15. doi:10.1186/s13059-017-1382-0
Wolrab, D., Jirásko, R., Cífková, E., Höring, M., Mei, D., Chocholoušková, M., et al. (2022). Lipidomic profiling of human serum enables detection of pancreatic cancer. Nat. Commun. 13 (1), 124. doi:10.1038/s41467-021-27765-9
Wu, L., Zheng, K., Yan, C., Pan, X., Liu, Y., Liu, J., et al. (2019). Genome-wide study of salivary microRNAs as potential noninvasive biomarkers for detection of nasopharyngeal carcinoma. BMC Cancer 19 (1), 843. doi:10.1186/s12885-019-6037-y
Wyatt, A. W., Azad, A. A., Volik, S. V., Annala, M., Beja, K., McConeghy, B., et al. (2016). Genomic alterations in cell-free DNA and enzalutamide resistance in castration-resistant prostate cancer. JAMA Oncol. 2 (12), 1598–1606. doi:10.1001/jamaoncol.2016.0494
Xiao, Y-L., Liu, S., Ge, R., Wu, Y., He, C., Chen, M., et al. (2023). Transcriptome-wide profiling and quantification of N6-methyladenosine by enzyme-assisted adenosine deamination. Nat. Biotechnol. doi:10.1038/s41587-022-01587-6
Xu, B., Chen, Y., Chen, X., Gan, L., Zhang, Y., Feng, J., et al. (2021). Metabolomics profiling discriminates prostate cancer from benign prostatic hyperplasia within the prostate-specific antigen gray zone. Front. Oncol. 11, 730638. doi:10.3389/fonc.2021.730638
Xu, W., Lu, J., Zhao, Q., Wu, J., Sun, J., Han, B., et al. (2019). Genome-wide plasma cell-free DNA methylation profiling identifies potential biomarkers for lung cancer. Dis. Markers 2019, 4108474. doi:10.1155/2019/4108474
Xu, Y., Ma, X., Ai, X., Gao, J., Liang, Y., Zhang, Q., et al. (2020). A urine-based liquid biopsy method for detection of upper tract urinary carcinoma. Front. Oncol. 10, 597486. doi:10.3389/fonc.2020.597486
Yang, Y., Misra, B. B., Liang, L., Bi, D., Weng, W., Wu, W., et al. (2019). Integrated microbiome and metabolome analysis reveals a novel interplay between commensal bacteria and metabolites in colorectal cancer. Theranostics 9 (14), 4101–4114. doi:10.7150/thno.35186
Yang, Z., LaRiviere, M. J., Ko, J., Till, J. E., Christensen, T., Yee, S. S., et al. (2020). A multianalyte panel consisting of extracellular vesicle miRNAs and mRNAs, cfDNA, and CA19-9 shows utility for diagnosis and staging of pancreatic ductal adenocarcinoma. Clin. Cancer Res. 26 (13), 3248–3258. doi:10.1158/1078-0432.CCR-19-3313
Yates, L. R., Seoane, J., Le Tourneau, C., Siu, L. L., Marais, R., Michiels, S., et al. (2018). The European society for medical oncology (ESMO) precision medicine glossary. Ann. Oncol. 29 (1), 30–35. doi:10.1093/annonc/mdx707
Ye, X., and Weinberg, R. A. (2015). Epithelial-mesenchymal plasticity: A central regulator of cancer progression. Trends Cell Biol. 25 (11), 675–686. doi:10.1016/j.tcb.2015.07.012
Yu, T., Wang, C., Xie, M., Zhu, C., Shu, Y., Tang, J., et al. (2021). Heterogeneity of CTC contributes to the organotropism of breast cancer. Biomed. Pharmacother. 137, 111314. doi:10.1016/j.biopha.2021.111314
Zaccaria, S., and Raphael, B. J. (2021). Characterizing allele- and haplotype-specific copy numbers in single cells with CHISEL. Nat. Biotechnol. 39 (2), 207–214. doi:10.1038/s41587-020-0661-6
Zavridou, M., Mastoraki, S., Strati, A., Koutsodontis, G., Klinakis, A., Psyrri, A., et al. (2020). Direct comparison of size-dependent versus EpCAM-dependent CTC enrichment at the gene expression and DNA methylation level in head and neck squamous cell carcinoma. Sci. Rep. 10 (1), 6551. doi:10.1038/s41598-020-63055-y
Zeune, L. L., Boink, Y. E., van Dalum, G., Nanou, A., de Wit, S., Andree, K. C., et al. (2020). Deep learning of circulating tumour cells. Nat. Mach. Intell. 2, 124–133. doi:10.1038/s42256-020-0153-x
Zhang, C., Sun, C., Zhao, Y., Wang, Q., Guo, J., Ye, B., et al. (2022). Overview of MicroRNAs as diagnostic and prognostic biomarkers for high-incidence cancers in 2021. Int. J. Mol. Sci. 23 (19), 11389. doi:10.3390/ijms231911389
Zhang, H., Chen, J., Liu, T., Dang, J., and Li, G. (2019). First-line treatments in EGFR-mutated advanced non-small cell lung cancer: A network meta-analysis. PLoS ONE 14 (10), e0223530. doi:10.1371/journal.pone.0223530
Zhang, H., and Lyden, D. (2019). Asymmetric-flow field-flow fractionation technology for exomere and small extracellular vesicle aration and characterization. Nat. Protoc. 14 (4), 1027–1053. doi:10.1038/s41596-019-0126-x
Zhang, X., Zhao, D., Yin, Y., Yang, T., You, Z., Li, D., et al. (2021). Circulating cell-free DNA-based methylation patterns for breast cancer diagnosis. NPJ Breast Cancer 7 (1), 106. doi:10.1038/s41523-021-00316-7
Zhang, Y., Liu, Y., Liu, H., and Tang, W. H. (2019). Exosomes: Biogenesis, biologic function and clinical potential. Cell Biosci. 9, 19. doi:10.1186/s13578-019-0282-2
Zhang, Z., Ramnath, N., and Nagrath, S. (2015). Current status of ctcs as liquid biopsy in lung cancer and future directions. Front. Oncol. 5, 209. doi:10.3389/fonc.2015.00209
Zhao, L., Wu, X., Zheng, J., and Dong, D. (2021). DNA methylome profiling of circulating tumor cells in lung cancer at single base-pair resolution. Oncogene 40 (10), 1884–1895. doi:10.1038/s41388-021-01657-0
Zhou, Q., Geng, Q., Wang, L., Huang, J., Liao, M., Li, Y., et al. (2019). Value of folate receptor-positive circulating tumour cells in the clinical management of indeterminate lung nodules: A non-invasive biomarker for predicting malignancy and tumour invasiveness. EBioMedicine 41, 236–243. doi:10.1016/j.ebiom.2019.02.028
Zhou, X., Xu, Y., Zhu, L., Su, Z., Han, X., Zhang, Z., et al. (2020). Comparison of multiple displacement amplification (MDA) and multiple annealing and looping-based amplification Cycles (MALBAC) in limited DNA sequencing based on tube and droplet. Micromachines (Basel) 11 (7), 645. doi:10.3390/mi11070645
Ziegenhain, C., Vieth, B., Parekh, S., Reinius, B., Guillaumet-Adkins, A., Smets, M., et al. (2017). Comparative analysis of single-cell RNA sequencing methods. Mol. Cell 65 (4), 631–643.e4. doi:10.1016/j.molcel.2017.01.023
Zong, C., Lu, S., Chapman, A. R., and Xie, X. S. (2012). Genome-wide detection of single-nucleotide and copy-number variations of a single human cell. Science 338 (6114), 1622–1626. doi:10.1126/science.1229164
Keywords: personalized medicine, tumor biomarker, circulating tumor cell (CTC), circulating tumor DNA (ctDNA), multi-omics, exosome, miRNA, liquid biopsy
Citation: Di Sario G, Rossella V, Famulari ES, Maurizio A, Lazarevic D, Giannese F and Felici C (2023) Enhancing clinical potential of liquid biopsy through a multi-omic approach: A systematic review. Front. Genet. 14:1152470. doi: 10.3389/fgene.2023.1152470
Received: 27 January 2023; Accepted: 20 March 2023;
Published: 03 April 2023.
Edited by:
Elisa Frullanti, University of Siena, ItalyReviewed by:
Areti Strati, National and Kapodistrian University of Athens, GreeceGianluca Santamaria, Technical University of Munich, Germany
Maria Palmieri, University of Siena, Italy
Viola Bianca Serio, University of Siena, Siena, Italy in collaboration with reviewer MP
Copyright © 2023 Di Sario, Rossella, Famulari, Maurizio, Lazarevic, Giannese and Felici. This is an open-access article distributed under the terms of the Creative Commons Attribution License (CC BY). The use, distribution or reproduction in other forums is permitted, provided the original author(s) and the copyright owner(s) are credited and that the original publication in this journal is cited, in accordance with accepted academic practice. No use, distribution or reproduction is permitted which does not comply with these terms.
*Correspondence: Claudia Felici, felici.claudia@hsr.it
†These authors have contributed equally to this work and share first authorship
‡These authors have contributed equally to this work and share last authorship