- 1College of Life Sciences, Henan Agricultural University, Zhengzhou, China
- 2Henan Engineering Research Center for Osmanthus Germplasm Innovation and Resource Utilization, Henan Agricultural University, Zhengzhou, China
- 3College of Landscape Architecture and Art, Henan Agricultural University, Zhengzhou, China
- 4School of Pharmacy, Xi’an Jiaotong University, Xi’an, China
- 5Key Laboratory of Conservation Biology for Endangered Wildlife of the Ministry of Education, College of Life Sciences, Zhejiang University, Hangzhou, China
Understanding adaptive genetic variation of plant populations and their vulnerabilities to climate change are critical to preserve biodiversity and subsequent management interventions. To this end, landscape genomics may represent a cost-efficient approach for investigating molecular signatures underlying local adaptation. Tetrastigma hemsleyanum is, in its native habitat, a widespread perennial herb of warm-temperate evergreen forest in subtropical China. Its ecological and medicinal values constitute a significant revenue for local human populations and ecosystem. Using 30,252 single nucleotide polymorphisms (SNPs) derived from reduced-representation genome sequencing in 156 samples from 24 sites, we conducted a landscape genomics study of the T. hemsleyanum to elucidate its genomic variation across multiple climate gradients and genomic vulnerability to future climate change. Multivariate methods identified that climatic variation explained more genomic variation than that of geographical distance, which implied that local adaptation to heterogeneous environment might represent an important source of genomic variation. Among these climate variables, winter precipitation was the strongest predictor of the contemporary genetic structure. FST outlier tests and environment association analysis totally identified 275 candidate adaptive SNPs along the genetic and environmental gradients. SNP annotations of these putatively adaptive loci uncovered gene functions associated with modulating flowering time and regulating plant response to abiotic stresses, which have implications for breeding and other special agricultural aims on the basis of these selection signatures. Critically, modelling revealed that the high genomic vulnerability of our focal species via a mismatch between current and future genotype-environment relationships located in central-northern region of the T. hemsleyanum’s range, where populations require proactive management efforts such as assistant adaptation to cope with ongoing climate change. Taken together, our results provide robust evidence of local climate adaption for T. hemsleyanum and further deepen our understanding of adaptation basis of herbs in subtropical China.
1 Introduction
Local adaptation is ubiquitous in plants, which can result in the genetic divergence of populations cross the landscape (Sork et al., 2016; Cao et al., 2020; Shen et al., 2022). Climate is a major driver of such variation (Rehfeldt et al., 2014; Jia et al., 2020; Capblancq et al., 2022), whereas the primary climatic agents of selection and targets remain unknown for many species. The widely distributed species can span multiple climatic and topographic gradients whereby both adaptive and neutral processes can both affect their genome-wide variations (Savolainen et al., 2013; Wang et al., 2015; Nadeau et al., 2016). Disentangling the relative effects of natural selection and spatial isolation on genomic variation is important to quantify the contribution of adaptation in shaping the diversification and to unravel the specific climate selective agents underpinning its appearance (Gibson and Moyle, 2020). This, however, remains technically challenging mainly because selective climatic gradients and spatial variables are often confounding in natural populations (Wiens, 1989; Kissoudis et al., 2016; Nadeau et al., 2016). Advances in evolutionary and landscape genomics approaches and the increasing accessibility of large genomic and climate datasets enabled the characterization of the independent contributions of climate and space to explaining patterns of genetic variation (Feng and Du, 2022). These strategies serve as a complement to traditional approaches uncovering evidence for local adaptation (i.e., common garden experiments and/or reciprocal transplant), and aid efforts to identify agents of selection acting in natural populations and their possible genetic targets (Lasky et al., 2012; Lu et al., 2019; Capblancq et al., 2022).
Global climate change is a significant threat to biodiversity, which is impacting biosphere and altering ecosystem functions (Capblancq et al., 2020; Malhi et al., 2020). Numerous empirical studies have demonstrated that many plant species are already affected by rapid climate change and, as a result, displaying various responses, including shifting their ranges, loss of genetic diversity and fitness in nature populations as well as changes in population genetic composition (Exposito-Alonso et al., 2019; Exposito-Alonso et al., 2022; Sang et al., 2022). To date, predicting impacts of climate change on plant species traditionally depends on species distribution modelling (SDM) (Peterson et al., 2012; Dyderski et al., 2018; Thuiller et al., 2019). Although these methods can identify species vulnerable regions to future climate changes and forecast global patterns of extinction risk via integration of species occurrence records and fine-scale climate data, the SDM assumes that all individuals within a species have similar climate stress and ignores ecotypes and local adaptation (Smith et al., 2019; Capblancq et al., 2020; Aguirre-Liguori et al., 2021). Hence, it has been criticized for oversimplification and gradually replaced by methodologies that integrated local adaptation into projecting species responses to climate change (Fitzpatrick and Keller, 2015; Exposito-Alonso et al., 2018; Exposito-Alonso et al., 2019). In addition, the predominant methods utilized for detecting putatively adaptive signatures in natural systems are FST outlier analyses (OA) and environmental association analysis (EAA). OA studies screen SNPs with higher genetic divergence than expected among populations under a neutral model (Hohenlohe et al., 2010; Lotterhos and Whitlock, 2015), while EAA integrates genetic variation or allele frequencies and environmental variables and then detects adaptive signatures through identifying associations between them (Frichot and François, 2015; Rellstab et al., 2015). However, to identify loci putatively under selection is but one part of the question, additional efforts are needed to unravel how the selection stress acts on associated loci along the climatic gradients, and to understand functional significance of these loci. Keeping this in mind, we utilized integrative methods, including three OAs and one EAA, followed by SNP-specific generalized dissimilarity modelling (GDM) to determine changes of adaptive allelic frequencies throughout the species range (hereafter “allelic turnover”; Fitzpatrick and Keller, 2015), supported by gene annotation (see details in method sections). Once candidate adaptive loci have been identified, it is possible to assess genomic offset/genomic vulnerability that measures the change of the adaptive genetic composition in need to track the future climate shifts (Fitzpatrick and Keller, 2015; Bay et al., 2018; Rellstab et al., 2021). Furthermore, recent landmark studies have incorporated migration and dispersal into assessment of genomic vulnerability (Gougherty et al., 2021; Sang et al., 2022), which provide critical and promising information to guide adaptive management interventions for species keeping pace with future climate change, but have not been tested in many cases.
Here, we applied the above analytical methods to T. hemsleyanum (Vitaceae), one of the widespread components of China’s warm-temperate evergreen forest. This diploid, perennial herb is endemic to subtropical China (also occurs in southern Hainan and Taiwan Islands) with a distribution that spans 18° of longitude, 13° of latitude and 1,000 m of elevation (Figure 1), which makes it an ideal model species to investigate variation in local adaptation to climate. In previous studies, substantial phenotypic and genetic variations were observed within natural populations of this species. Population germplasms of different geographic origin vary substantially in morpho-agronomic traits (Zhu et al., 2015; Yang et al., 2019) as well as in phytochemicals and pharmacological activities (Jiang, 2015; Yin et al., 2021). Regarding the genetic structure, our previous study indicated that T. hemsleyanum populations were clustered into four genetically distinct groups (Wang et al., 2015), inhabiting different floristic regions of subtropical China that vary in climatic conditions (Wu and Wu, 1998; Wu et al., 2010). This result points to the potential importance of climatic difference among flora in shaping patterns of intraspecific genetic variation. However, the amount of genomic variation determined by climate factors, the key climatic drivers, and the possible genetic targets (SNPs and genes) remain unknown. Beyond that, T. hemsleyanum is also an endangered medicinal plant in the official protection list of crop germplasm resources (Hu et al., 2021). In recent decades, T. hemsleyanum experienced substantial natural population declines as a result of human over-exploitation coupled with its specific climatic requirement for growth (Wang et al., 2018; Guo et al., 2019), which will undoubtedly increase the risk of maladaptation of local populations in face of future climate changes of subtropical China. As forecasted by robust climate models, subtropical China will be subjected to a rise in temperature, an enhancement of spatial heterogeneity in precipitation and an enlargement of arid regions by the end of the century (TCNARCC, 2011; Zhao and Wu, 2014). However, the risks that this climate sensitive species may face from these future climate challenges and where it is most effective in achieving conservation goals are still unknown.
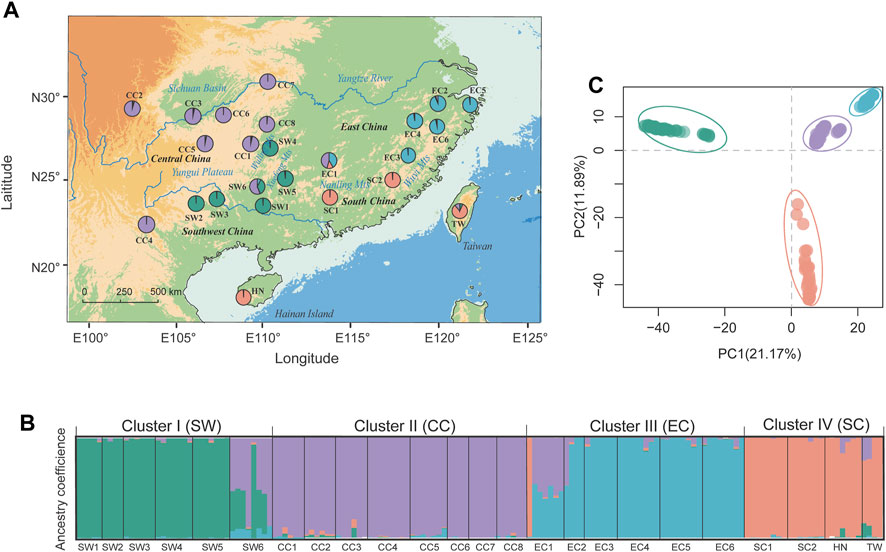
FIGURE 1. Genetic population structure of T. hemsleyanum as revealed by STRUCTURE (left panels) and DAPC analysis (top-right panel). (A) Geographic distribution of 24 populations with pie charts representing the ancestral components inferred by STRUCTURE analysis at K = 4. (B) Histogram of population assignment using STRUCTURE analysis with K = 4. The colored segment in each bar represents the individual ancestry coefficient of inferred clusters (or “gene pool”). (C) Population assignment results inferred from DAPC (using the R package adegenet).
Here, we sampled twenty-four populations of T. hemsleyanum across subtropical China and surveyed their genomic variation using specific-locus amplified fragment sequencing (SLAF, Sun et al., 2013). Our main objectives were to 1) evaluate the contributions of climate and geography to explaining patterns of genomic variation and characterize the key climate variables with the largest influence, 2) identify signatures of climate adaptation (i.e., via OA, EAA and annotation), and explore how locally divergent selection shaped patterns of allele frequencies changes of candidate adaptive SNP loci across species range, and 3) quantify and map which populations might be vulnerable to future climate change. Our study will facilitate a better understanding of the adaptation basis of China’s warm evergreen forest species and contribute to genetically informed measures for T. hemsleyanum resources management under shifting climates.
2 Materials and methods
2.1 Plant materials and genotyping
In this study, we collected fresh foliar samples of 252 georeferenced individuals at 24 independent natural sites (>50 km separation) (Supplementary Table S1; Figure 1). We chose 156 individuals for sequencing, including 132 individuals screened for variation previously using cpDNA and microsatellite loci (Wang et al., 2015), and 24 newly collected samples from Guangxi and Taiwan Island (Figure 1). Sampling locations were chosen to 1) cover entire species geographic and climatic distribution in mainland China and southern islands, including South/East China (SC1, SC2; EC1–EC6), Central China (CC1–CC8), Southwest China (SW1–SW6), Hainan (HN) and Taiwan (TW) Island; 2) represent major intraspecific phylogeographic clades identified in the previous study (Wang et al., 2015).
Total genomic DNA was isolated from the silica gel dried leaf material using Plantzol Reagent (Invitrogen). SLAF sequencing libraries were prepared using the HaeIII and RsaI restriction enzymes. The resulting 314 to 414 bp fragments (with barcodes and dual-index sequencing adaptors) were excised and purified with the Qiagen Gel Extraction Kit. After the library quality inspection, paired-end sequencing (the read lengths were 126 bp) was conducted on an Illumina HiSeq 2500 platform (Illumina, Inc., San Diego, CA, United States).
After trimming sequencing adapters, the raw reads were filtered for low-quality bases with a quality score less than Q20. Trimmed reads were demultiplexed using the Illumina bcl2fastq2 conversion software (Illumina, CA, USA). We aligned the reads to the T. voinierianum reference genome (Cai et al., 2021) using BWA-MEM (Li, 2013). T. voinierianum is a close relative to T. hemsleyanum with complete genome sequences available. SNP calling for each aligned sample were performed using GATK v.3.3 (DePristo et al., 2011) with HaplotypeCaller and GenotypeGVCFs tools. The SNPs identified by both methods were retained. Further SNP filters for subsequent analysis were applied with VCFtools v.0.1.14 (Danecek et al., 2011) and the “populations” program implemented in STACKS v.1.27 (Catchen et al., 2011) with the following criteria: a Phred pass score of 30, a mean maximum depth per locus of 100× across all sites (to avoid SNPs from over-represented organelle reads or falsely aligned paralogs), and a minimum read depth threshold of 6× for each sample. Furthermore, the minor allele frequency (MAF) per locus has to be greater than 5%, and only bi-allelic type of SNPs informative in at least 75% of the samples and 70% of the sites were retained. To reduce linkage, SNPs in strong linkage disequilibrium were removed using PLINK v.1.9 (Purcell et al., 2007), in a window size of 100 bp and a window step of 10 bp and a pairwise genotype correlation r2 > 0.5.
2.2 Environmental data
We retrieved 35 bioclimatic variables (see Supplementary Table S2) for each sampling site from the WorldClim v.1.4 (Hijmans et al., 2005) and one derived set ENVIREM (Title and Bemmels, 2018). WorldClim variables (BIO1–BIO19) are generated from interpolation of mean monthly weather station climate data from 1960 to 1990 (centered on ∼1975). ENVIREM includes an expanded set of 16 bioclimatic variables to complement the WorldClim dataset, most of which are relevant to the physiological and ecological processes of plants (Title and Bemmels, 2018). For the future predictions, this study used statistically downscaled, bias-corrected CMIP6 general circulation models (GCMs) with high resolution. To account for uncertainty in model projections, a composite average of six GCM models (MRI-ESM2-0, BCC-CSM2-MR, IPSL-CM6A-LR, CanESM5, CNRM-ESM2-1 and MIROC-ES2L) with low amount of interdependence (Brunner et al., 2020), was developed for the time period 2061–2080 (centered on 2070), and for two shared socioeconomic pathways (SSPs)—SSP370 and SSP580. The raster files for future data downloaded from WorldClim were further utilized to generate ENVIREM variables for all GCMs and the SSP scenario using online pipelines (http://envirem.github.io/ENVIREM_tutorial.html). All variables for downstream analysis in this study were obtained at 30-s resolution (∼1 km2), and were scaled, centered, log-transformed (if appropriate) and tested for normality. To reduce multicollinearity, climatic cells with sampling records from all 35 variables were checked for Spearman’s correlation coefficient in R. Ultimately, a subset of 11 variables (see Supplementary Table S2) that lacked strong correlation (|r| < 0.75) were considered for environmental association analysis.
2.3 Genetic diversity and population genetic structure
Population genetic diversity parameters, including expected heterozygosity (HE), percentage of polymorphic loci (P) and Wright’s inbreeding coefficient (FIS) per population were estimated using the “populations” module of STACKS v.1.27 (Catchen et al., 2011). We utilized an analysis of molecular variance (AMOVA) to evaluate the genetic divergence among populations and geographic regions in Arlequin v.3.5 (Excoffier and Lischer, 2010), and the significance of FST values were assessed with 1,000 permutations. We investigated population genetic structure using STRUCTURE v.2.3.4 (Pritchard et al., 2009). For each number of cluster (K) that varies from 2 to 10, we run 10 repetitions with a 10,000 burn-in steps of and 1,00,000 Markov chain Monte Carlo (MCMC) replications. The program was utilized applying independent allele frequencies and an admixture model. The most probable values of K were determined by ∆K method (Evanno et al., 2005) using HARVEST software (Earl and vonHoldt, 2012). In addition, we also performed a discriminant analysis of principal components (DAPC) to infer the number of genetic clusters in the R package adegenet (Jombart, 2008; Jombart and Ahmed, 2011).
2.4 Assessing the role of climate and geography
We estimated the amount of genomic variation attributable to climate and geography by two different variance partitioning approaches—redundancy analysis (RDA) and generalized dissimilarity modelling (GDM). All climate variables were scaled and centered to account for difference in magnitude when calculating environmental distance. To test for the presence of regional climatic differences, the difference of each climate variable among the four genetic clusters (identified in STRUCTURE) were assessed using analysis of variance (ANOVA), followed by post hoc Tukey’s test. As a form of constrained ordination, RDA is applicable to genomic data (Forester et al., 2018) and permits to evaluate the total explanatory power of climate and geographical variables for genotypes (Legendre and Legendre, 2012). Using partial RDA, it enables the estimation of SNP variation independently attributed to geography, climate and their colinear portion (climate + geography) (Gibson and Moyle, 2020; Chang et al., 2022). We utilized the R package VEGAN to perform RDAs using climate variables and spatial variables (distance-based Moran eigenvector maps, dbMEM) as independent predictors (Oksanen et al., 2019). We generated dbMEMs based on geographic coordinates of sites using the quickMEM function in the ADESPATIAL package detailed in Borcard et al. (2018). Forward selection implemented in this function was performed to select significant dbMEMs. These dbMEM were finally included as predictors in our RDA models, which generate canonical axes representing the spatially structured genetic variation across species’ distribution range. The bioclimate variables, retained by Pearson correlation analysis, were further tested by forward selection implemented in the R package ADESPATIAL to find variables predictive for partitioning. Forward selection was applied at the α = 0.05 level and a maximum global adjR2 threshold equal to the adjusted R2 of the RDA model including all initial variables (in this case adjR2 <0.318) with 10,000 permutations. In partial RDA, variance partitioning was carried out using population allele frequencies as the response variable in the two partial models that utilized the selected bioclimate variables as explanatory variables and the dbMEMs as conditioning variables and vice versa.
We additionally performed GDM to estimate the total and independent effects of environmental dissimilarity and geographic distance on genomic divergence. While GDM is in principle similar to RDA, it is based on a different statistical method, which employs non-linear regression to estimate the amount of population pairwise genetic distance attributed to pairwise differences of climate and dbMEM variables (Fitzpatrick and Keller, 2015). First, based on the same genomic data for variance partitioning in RDA, a population pairwise FST matrix was generated using the R package hierfstat (Goudet, 2005). Second, a site-by-environment predictor matrix was created including population IDs, geographic coordinates and the set of climate variables. Then, we fit GDM models in R package GDM using the matrix of genetic distance as the response variable and the climate variables as explanatory variables. We test the significance of each predictor variable by randomization tests (Shryock et al., 2021), and only included the predictor variables that significantly increase the explained deviance in the final fitted model (Manion et al., 2017). The relative importance of climatic predictors with regard to allelic turnover was estimated as the sum of I-spline basis functions (maximum height of each response curve; Fitzpatrick and Keller, 2015). To test the model robustness, we simulated 1,000 replicates, leaving out a random 10% of sites we sampled, and then performed GDMs the same before at each replicate (Murray et al., 2019).
2.5 Outlier and environmental association analysis
To identify genomic signatures of selection, we applied a combined analysis approach integrating three OAs (BAYESCAN, SELESTIM and BAYENV XT X) and EAA (BayPass). The three OAs we adopted are based on different demographic assumptions. BAYESCAN v. 2.1 (Foll and Gaggiotti, 2008) and SELESTIM v.1.1.4 (Vitalis et al., 2014) both used simple island model with migration. Specifically, BAYESCAN firstly generates population-specific and locus-specific FST coefficients while accounting for sample size variation, and then calculates the posterior probabilities of models with or without selection for each locus (Foll and Gaggiotti, 2008). In this study, three independent BAYESCAN runs were performed with prior odds of 10,000 following Lotterhos and Whitlock (2015), with 20 pilot runs of length 5,00,000, a thinning interval of 10, and a burn-in of 50,000. SNPs have consistent low false discovery rate (FDR < 0.05) in all three runs were considered as outliers. SELESTIM assumed that the study system followed a multinomial Dirichlet distribution of allele frequencies between populations as BAYESCAN, but relies on allelic frequencies instead of FST to identify loci under strong selective pressures (Hoban et al., 2016). This method uses Kullback-Leibler divergence (KLD) to estimate the distance of locus-specific coefficients of selection from the genome-wide effect of selection (Vitalis et al., 2014). The KLD was calibrated by simulated observed data (Vitalis et al., 2014). We carried 50 pilot runs with length of 5,000 to tune the model parameters, which is followed by 10,00,000 iterations with a burn-in of 1,00,000 and a thinning interval of 20. The threshold value of the empirical distribution of the KLD based on a pod analysis was set to 0.01.
To account for more complex demographic histories, we performed a third outliers test using BAYENV XT X (Günther and Coop, 2013). This method uses a population covariance matrix to accounts for neutral genetic structure. The matrix was created using SNPs not significant in other OAs. To minimize the stochasticity in null model estimation, the matrix was generated from the mean covariance matrix across three replicated runs produced from 5,00,000 iterations each. We then perform three runs for 5,00,000 iterations with the covariance matrix, and calculate XT X across replicates for each SNP. SNPs with top 5% of ranked XT X values were deemed to be outliers.
To complement the OA method, we used BayPass v2.1 (Gautier, 2015) to detect signals of selection based on associations between allele frequencies and climate variables, while accounting for hierarchical structure of populations. BayPass is an elaboration on the model of BayEnv2 and is wrapped under the script Baypass_workflow.R implemented in pyRona v.0.3.7 (Pina-Martins et al., 2017). Prior to runs, a covariance matrix was generated with the full SNP data set using the similar algorithm implemented in BayEnv2. Baypass was run under a standard covariance model (STD) with default parameter settings. Significant associations were defined as having an eBPis greater than 3 and a Bayes Factor greater than 15 dB, which are thresholds consistent with other studies (Ahrens et al., 2019; Seabra et al., 2021).
2.6 Landscape modelling
We test for spatially explicit selection processes for independent candidate SNPs using the package GDM in R (Ferrier et al., 2007; Manion et al., 2017). We adopted a “single-SNP” approach following Dudaniec et al. (2018) and modelled each candidate SNP independently, regardless of genomic contexts. The complete set of candidate loci identified by OA (by at least two methods) and EAA methods were used for GDM since each of the method adopt a statistic approach that is uniquely valid to reveal signatures of selection, and there is only minor overlap in the number and identity of retained SNPs across approaches.
Given the strong interspecific genetic differentiation and significant correlation between genetic structure and environmental gradients in T. hemsleyanum (see results), false positives were expected for the OA and EAA methods even with a correction for population structure. As a result, we conducted two additional steps to control for false positives by GDM. Firstly, we randomly choose 200 SNPs from the full set of 30,252 SNPs, and incorporate the random sample as a “reference” in the GDM to investigate if explanatory power of a candidate SNP is higher than that of the reference group. Secondly, we integrated geographic distance in the GDMs to reveal if spatial isolation better explained the observed partial allelic turnover across climatic gradients. This acts as a second screening for loci that predominantly respond to neutral genetic processes (i.e., isolation by distance). Overall, we tried to reduce false-positive rates, 1) by comparing the response of SNP-specific partial allelic turnover to climate variables with the response to geographic distance and 2) by comparing the response of each candidate SNP with reference SNP group to test whether a given locus explained a higher deviance within the GDM than a random sample of genetic variation.
We created the population pairwise FST matrices using the package HIERFSTAT in R (Goudet, 2005) and applied GDM to each of the candidate SNPs identified by EAA and OA. To evaluate the role of a specific SNP in selection processes, we ranked the SNP-specific allelic turnover functions in relation to each climate variable in two different ways as described in Dudaniec et al. (2018). Within each SNP, the ranking per variable is based on the partial allelic turnover magnitude relative to the other variables included in the GDM model. Across SNPs, the ranking per SNP is based on the explained deviance ranked relative to full candidate data set. After filtering of SNPs that 1) had the highest magnitude of partial allelic turnovers related to one of the climate variables rather than geographic distance and 2) explained higher model deviance than the reference SNP group (which had an explanatory power of 10.05%), 275 of 497 previous identified SNPs were retained.
2.7 Genomic contexts of candidate SNPs
The positions of candidate SNPs accompanying coding genes were identified based on gene models on the T. voinierianum genome (Cai et al., 2021) using BEDTools (Quinlan and Hall, 2010) and SnpEff (Cingolani et al., 2012). The gene models were predicted based on the T. voinierianum transcriptome (Matasci et al., 2014) and Vitis vinifera proteomes as described in Cai et al. (2021). The genes that are 1,000 bp upstream or downstream from the candidate SNPs were also presented (if any) to catch possible regulatory sites located in intergenic regions (Harris and Nielsen, 2014; Ahrens et al., 2019).
2.8 Genomic offset under future climates
For this threatened and endangered species, we are particularly interested in identifying spatial regions or populations at highest risk of future maladaptation, and where migration will be most effective to maintain the current status of adaptation. To do so, we extended the GDMs to estimate genomic offset, also called genomic vulnerability (Bay et al., 2018), which represents the disruptive effect of future climate change on contemporary genotype-climate associations (Rellstab, 2021). In this study, we followed a novel developed method in Gougherty et al. (2021) that incorporate migration into predict genomic models and used GDMs to calculated three metrics of genomic offsets that termed local, forward and reverse offsets. We fit GDMs to FST of the 275 retained candidate SNPs under putative selection. The fitted models were predicted to climate in 2070 and two shared socioeconomic pathways (SSPs)—SSP370 and SSP580. As described in Fitzpatrick and Keller (2015), local (classic) offset was calculated by estimating in situ allele frequencies shift at the robust set of climate-adaptive loci that a resident population required to response to local climate changes in 2070 (assuming no migration). Forward and reverse offsets were recently developed by Gougherty et al. (2021). For forward offset estimates, we firstly calculated the genomic offset of each current grid cell within the extant range of T. hemsleyanum to all future grid cells within China. Then we identified minimum offset among genomic offsets estimated across all future grid cells (termed “forward offset”), which assumes unconstrained dispersal of T. hemsleyanum populations to any location within China. High values of forward offset indicate a low adaptation capacity of the population to all future climates of China and a high chance of extinction in current genotype–climate relationships across the landscape. Besides, the distance and initial bearing that populations would migrate/disperse to the grid cell that minimized forward offset were estimated in R package geosphere (Hijmans et al., 2017) using the distGeo and bearing functions, respectively. Reverse offset is predicted by identifying the minimum offset among populations under current climate and “hypothetical” populations in 2017 and, both within the current range of this species. High reverse offset indicates the future novelty of genotype-climate relationships, since such relationships are not existed at any location of current landscape. The Spearman’s correlations between population structure, genomic offsets and climate variable shifts were quantified in R. To visualize genomic vulnerability to climate change, we presented an RGB image using ArcGIS 10.1 to simultaneously map all three genomic offset measures as red, green and blue bands, respectively, in geographic space for the year 2070. Prior to plotting, the values of each color band were rescaled to their quantiles (analogous to a histogram equalization) to illustrate the full range of each band.
3 Results
3.1 SLAF-seq and SNP calling
In this study, a total of c. 850 million reads was generated from all individuals with an average Q30 of 95.69% and a GC content of approximately 41.33% (Supplementary Table S3). The average number of reads per sample is 5.45 × 106 (minimum: 2.02 × 106; maximum: 13.17 × 106). High-quality SLAF tags (68,13,573 in total) were identified throughout the genome with an average of 12.05-fold sequencing depth (Supplementary Table S3). Subsequently, a total of 1,28,15,312 SNPs in 16,86,020 polymorphic SLAF tags were obtained. Of the 156 samples, 78.2% had a mean depth greater than 10×. After filtering for read depth and missing data (as described in the Methods), we retained a total of 30,252 high quality SNPs across samples.
3.2 Genetic diversity and population structure
Based on the high-quality SNP data, the genetic diversity of 24 populations in T. hemsleyanum were calculated and summarized in Supplementary Table S1. Nei’s genetic diversity (HE) was similar across populations (mean HE = 0.364, SD = 0.025) and varied from 0.045 (SW1) to 0.125 (EC2). The FIS values ranged from −0.044 (EC6) to 0.098 (EC1) with a mean value of 0.038. Significant pairwise genetic differentiation was detected for all population pairs (mean FST = 0.289, p < .001), ranging from 0.079 (between CC3 and CC6) to 0.455 (between EC2 and SW1) (Supplementary Table S4). Bayesian clustering implemented in STRUCTURE supported an optimal clustering at K = 4 (Supplementary Figure S1). The geographical assignment pattern of the individuals in the four clusters were almost concordant with to the geographical regions of Southwest [“SW” (green; pops SW1–5)], Central [“CC” (purple; CC1–8)], South [“SC” (tangerine; SC1–2, and adjacent island populations HN, TW) and East China [“EC” (light blue; EC1–5)] (Figures 1A, B). We observed high probabilities of ancestry to a given cluster for all populations, except that two populations (i.e., EC1, SW6) exhibited homogeneous levels of admixture. DAPC revealed a grouping pattern in concordance with that observed from STRUCTURE analysis, and the first two PCs explained 21.17% and 11.89% of the genetic variation, respectively (Figure 1C). AMOVA indicated that 37.01% of the genomic variation was distributed among regional groups (SW, CC, SC and EC) (FCT = 0.37, p < 0.001), while 39.25% of the variation occurred within populations of T. hemsleyanum (Supplementary Table S5).
3.3 Impacts of climate and spatial variables on genome-wide population differentiation
3.3.1 RDA analysis
We performed RDA using partially constrained ordination (without accounting for spatial structure), whereby ten climate variables were identified as significant predictive of genetic variation among populations (Table 1), with one factor (PETS: Monthly variability in potential evapotranspiration) excluded. The most predictive variables include BIO15 (Precipitation Seasonality), GDD0 (degree days above 0°C) and PETWeQ (Mean monthly PET of wettest quarter). In Partial redundancy analysis (pRDA), four dbMEM were retained as sufficient to explain the geographic structure among populations (Supplementary Table S6; Supplementary Figure S2). Among these four axes, dbMEM2 and dbMEM4 described broad-scale spatial structure, whereas dbMEM5 and dbMEM6 described fine-scale structure (Supplementary Table S6; Supplementary Figure S2). dbMEM2 contributed most variation of any single spatial variable (6.19%; Supplementary Table S6). Cumulatively more variation was explained by broad-scale spatial variables (8.30%) than fine-scale ones (5.95%). Forward selection constrained on dbMEMs retained ten climate variables (Table 1; Figure 2), which is predictive while accounting for spatial effect. The strongest predictor contributing to the total variation was BIO19 (Precipitation of Coldest Quarter; 11.72%), followed by PETWeQ (3.29%) and GDD0 (3.27%), while AIT (Aridity Index Thornthwaite) was the weakest predictor (Table 1; Figure 2).
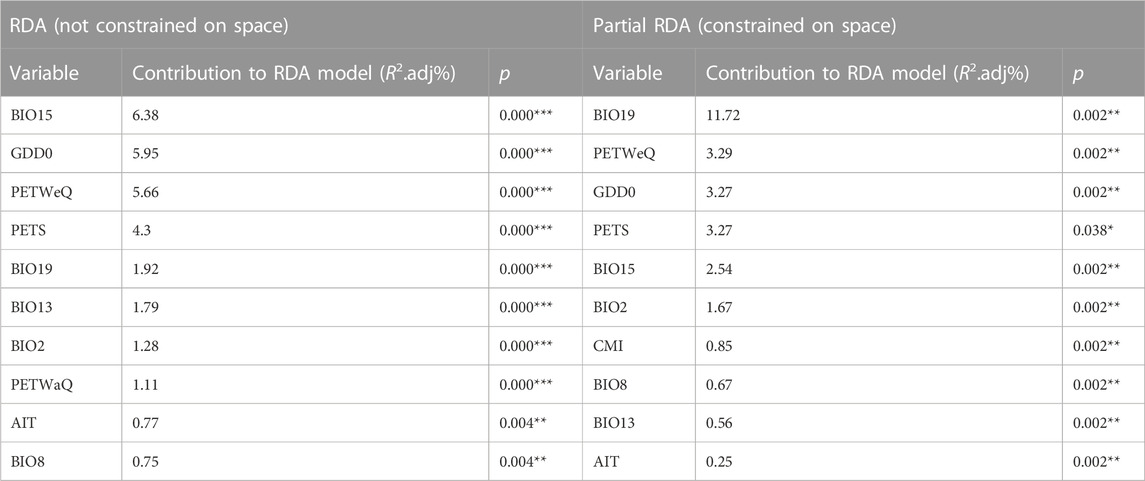
TABLE 1. Climate variables retained by forward selection and their contribution to genomic SNP variation using RDAs. The climate variable definitions are in Supplementary Material.
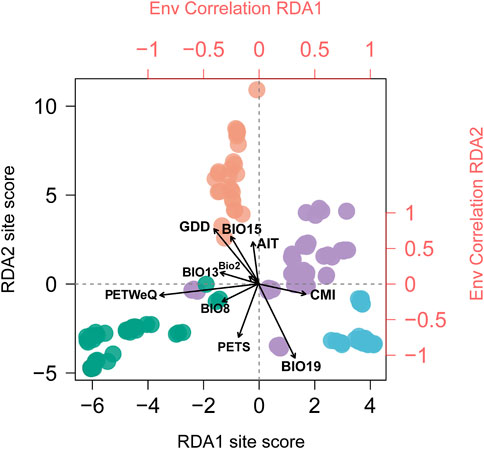
FIGURE 2. Biplot of Redundancy analysis conditioned on geography. Individuals are colored in corresponding with genetic clusters inferred by STRUCTURE analysis (see Figure 1). Top and right axes in red displayed the correlation of each climate variable (see Supplementary Table S2 for variable abbreviations) with RDA1 and RDA2 axes, respectively. Black vectors represent climate variables.
Using RDA, the climate (ten variables identified by forward selection) and geography both explained significant proportions of genetic variation (11.9%–31.8%, p < 0.001; “combined fractions” in Table 2). To further decompose their contribution, we performed partial RDA, which revealed that climate and geography jointly explained 36.4% of the inter-population genetic variation (“total explained,” Table 2). A minority of this (6.4%) was attributed to the collinear portion of climate and geography (Figure 3), that could represent the effects of clinical climate factors. Considering their independent effects, climate alone explained a substantially larger fraction of genetic variation than the geography alone (27.5% vs. 2.5%; Figure 3).
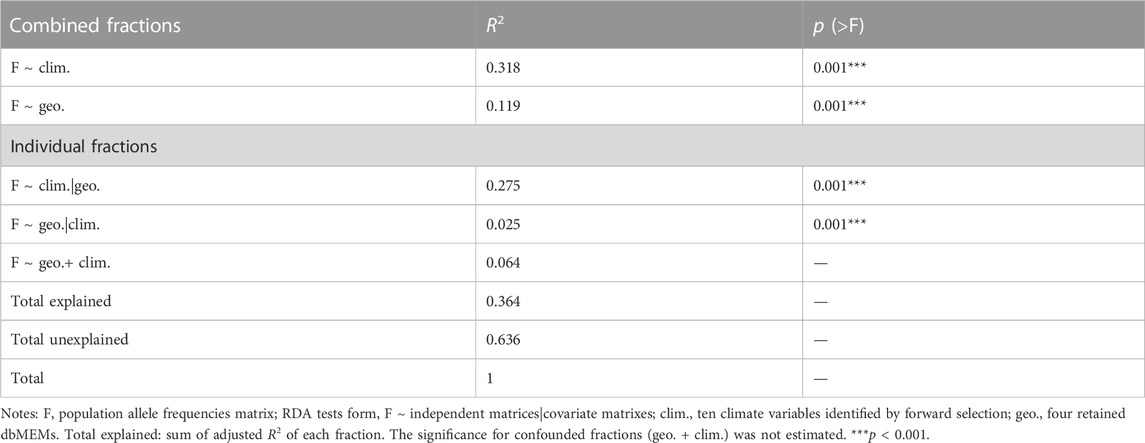
TABLE 2. RDAs to partition genomic variation among T. hemsleyanum populations into climate, geography and their combined fractions.
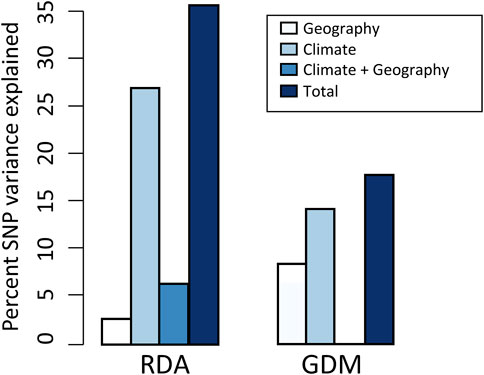
FIGURE 3. Results of variance partitioning estimated by RDA and GDM models. The total in legend means the genetic variation that climate and geography jointly explained, and the climate + geography means the collinear part of genetic variation. Note that GDM cannot estimate the contribution of climate + geography.
The correlations of climatic predictors with each RDA axes indicated that their contribution to population genetic variation varies geographically across the distribution range and therefore the climate variables acting as leading force in driving divergent selection regarding the geographic clusters being compared. Populations from South China (cluster IV) are exposed to greater growing degree days in comparison to populations from rest of the range; eastern coastal populations (cluster III) experience more winter precipitation; and southwestern populations (cluster I) experience highly potential evapotranspiration in warm and rainy seasons (Supplementary Figure S3). Moreover, we detected significant difference among the four genetic clusters that may contribute to divergent selection (p < 0.0001 for all tests; Supplementary Figure S3), indicating that these regional clusters tend to occupy habitats characterized by more or less unique climate features.
3.3.2 GDM analysis
The full GDM (climate + geography) model explained 17.6% of the deviance in spatial patterns of genetic variation among populations (p < 0.001) (Figure 3; Supplementary Table S7). The models accounting for individual effect of geography and climate explained 8.1% and 14.3% of the deviance, respectively (Figure 3). As with RDA, this finding suggests that a larger proportion of variation is attributable to climate differentiation than to geographic isolation. In climate GDM model, the sums of I-spine basis functions (Supplementary Figure S4) indicated that BIO15 (Precipitation Seasonality) had the greatest magnitude in partial allelic turnover response (importance weight = 0.330), followed by BIO19 (Precipitation of Coldest Quarter; importance weight = 0.298). It is worth noticing that these two variables were consistently identified as significant predictors contributing to SNP variation in both GDM and RDA analysis (see Supplementary Tables S8, S9 for variable contributions in GDM models).
3.4 Identification of candidate adaptive SNP
By OA, a total of 107 SNPs was detected in at least two of the three tests (Figure 4; Supplementary Table S10). Specifically, BayeScan (see Supplementary Figure S5), BayENV XT X and Selestim identified 55, 303, and 343 putatively adaptive SNPs, respectively. 20 SNPs were identified in all three tests and 87 in two tests, with the rest SNPs exclusively to BayeScan (9), BayENV XT X (204) and Selestim (254) (Figure 4). Using EAA analysis (Baypass), 402 SNPs were identified with significant associations with climate variables, and 14 of these SNPs were related to multiple climate variables (Supplementary Table S11). Collectively, a total of 497 SNPs was identified to be outliers by multiple OA methods and/or to be associated with at least one of the eleven climate variables tested, representing 1.7% of the SNP dataset. Of these candidate SNPs, only 12 SNPs were shared between OA and EAA approaches (Supplementary Tables S10, S11).
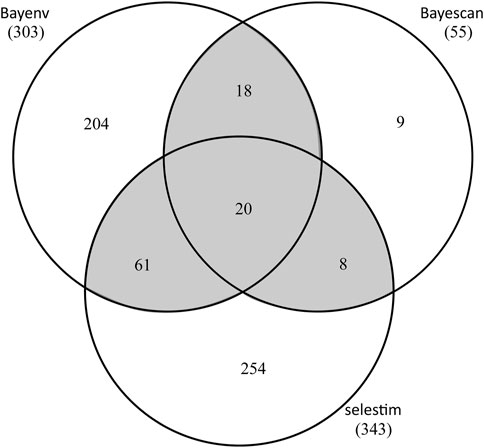
FIGURE 4. Number of T. hemsleyanum SNPs detected in three FST outlier tests. Shaded overlapping regions (outliers in >2 tests) represent SNPs deemed as outliers in this study.
GDMs were independently applied to the 497 candidate SNPs to quantify partial allelic turnovers through climatic gradients (Supplementary Table S12). For each variable, we presented GDM results for the top 150 SNPs with the greatest magnitude of partial allelic turnovers in Supplementary Figure S6, which revealed varying gradients and strengths of selection acting across a range of loci. For example, the SNPs associated with geography (Supplementary Figure S6A), BIO19 (Supplementary Figure S6F) and PET seasonality (Supplementary Figure S6J) mostly appeared to reach the highest allelic turnover magnitudes at positions where the greatest change occurs along environmental gradients, while SNPs associated with BIO15 mostly ceased allelic turnover beyond a precipitation seasonality cut-off of 58 (Supplementary Figure S6). On average, the magnitude in partial allelic turnovers per climate variable for the 497 SNPs were highest in relation to BIO19 (0.147), followed by BIO13 (0.141) and PET seasonality (0.120) (Supplementary Table S12). BIO13 (Supplementary Figure S6D) and GDD0 (Supplementary Figure S6I) drove the most variable magnitudes of partial allelic turnover, both of which had distinct thresholds of turnover recognizable for each related locus.
Among the 497 previous identified candidate SNPs, 6 SNPs (1.2%) showed non-significant allelic turnover response to any of the variables, and were not interpreted further. 50 of the 497 SNPs (10.1%) had less magnitude in partial allelic turnover associated with climate variables than with geographic distance (and considered to be possible false positives). The “reference SNP group” explained 10.5% of the deviance in GDM model, and therefore the SNPs with explanatory power less than 10.5% were also excluded. Finally, we retained 275 SNPs for further functional annotation.
3.5 Annotation
Of the 275 putative adaptive SNPs we identified, 219 occurred in SLAF scaffolds that aligned to contigs of T. voinierianum genome assembly, and reside in different genomic regions including coding, intron and intergenic regions. No candidate SNPs were found within regulatory regions. Of the 219 SNPs, 92 SNPs were predicted to fall within genes. 31 (33.7%) out of the genic SNPs were exonic variants, including two identified in OA, 28 in EAA test (associated with climate variables) and one in both tests (Table 3). The 31 SNPs uncovered gene functions associated with abiotic stimuli response, cell cycle progression, flora reproductive development and terpenoid synthesis. Full annotation results of all candidate SNPs are given in Supplementary Table S13.
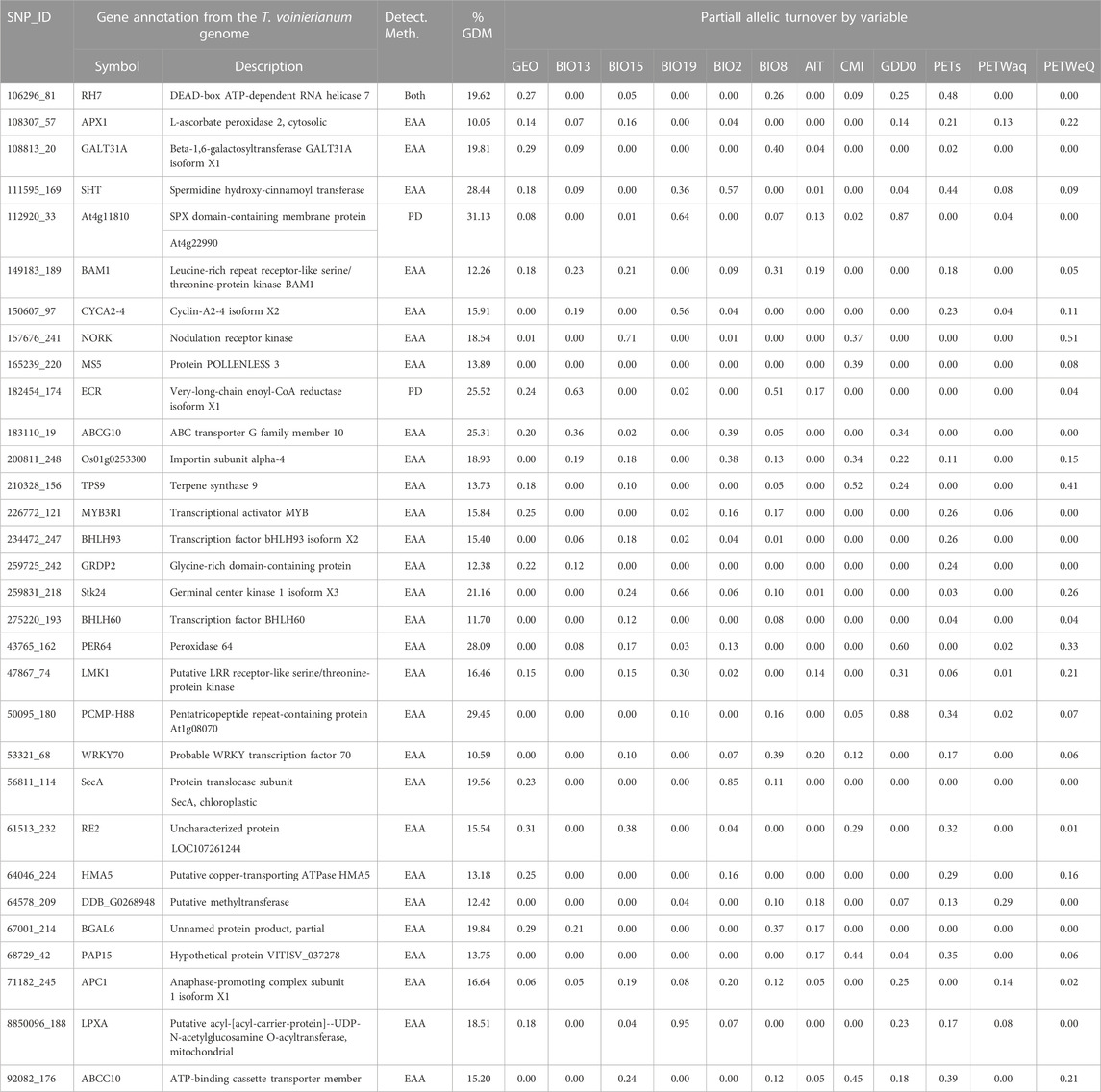
TABLE 3. Gene annotation and associated variables for potentially adaptive SNPs. SNP IDs, gene function on the T. voinierianum genome are shown. The detection method (PD/EA tests) and partial allelic turnover by variables predicted by GDM are listed for each annotated locus. The SNP presented had 1) higher allelic turnover responses associated with climate variables relative to geographic distance, and 2) a higher explained deviance (%) in GDMs than the reference SNP group. The climate variable definitions are in Supplementary Material.
3.6 Genomic offset and migration to climate change
The GDM model based on 275 potentially adaptive loci explained 25.91% of the deviance. BIO19 was the most important predictor for the observed adaptive genetic variation (Supplementary Figure S7). Under a scenario of future climate change, although we found differing patterns of the local, forward and reverse offsets across the distribution range of T. hemsleyanum, some general patterns can be observed. The three genomic offsets were predicted be lowest for populations in the southern range margin of the mainland (Figure 5; Supplementary Figure S7). By contrast, the central-north part of the range, especially along eastern Yungui Plateau and Xuefeng Mts, have relatively high levels of genomic offsets (Figure 5; Supplementary Figure S7), indicative of relatively high future disruption of genotype-climate relationships in populations occupied current locations, and such effects of climate change cannot be mitigated by movement towards more suitable locations. Local and forward offsets were most strongly associated with shifts in BIO19, PET seasonality and arid index (Supplementary Figure S9). Besides, there is no obvious relationships between local/forward offset and population structure, except for a significant negative relationship existed between local offset and the estimated ancestry coefficients for the “South” cluster (Supplementary Figure S9).
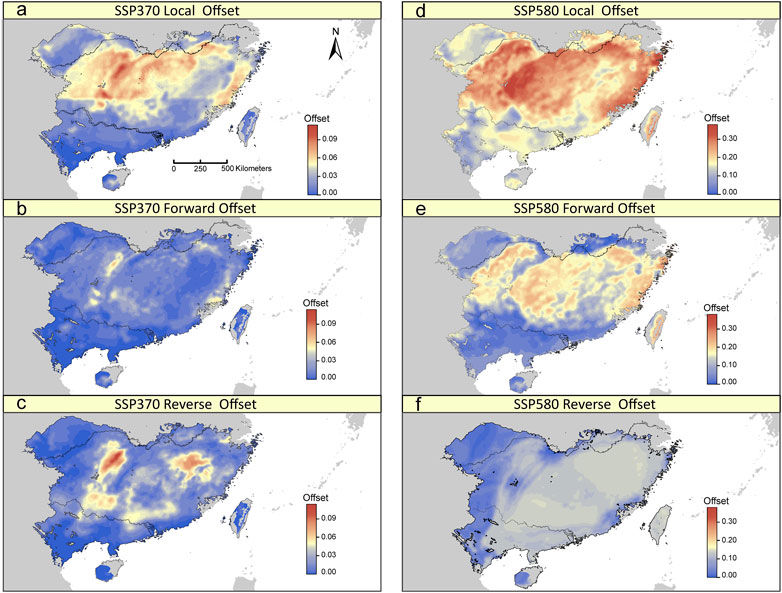
FIGURE 5. Spatial distribution of local, forward, and reverse genomic offsets estimated from GDM under SSP370 (A–C) and SSP580 (D–F) in 2070.
For the set of adaptive loci, few (<1%) locations had the distance to locations that minimized forward offset (Dmin) equals to zero. The longest Dmin was predicted in central-north part of the range, whereas the shortest Dmin mainly occurred in the southern and northwestern range edge (Figure 6A). Furthermore, the initial direction that populations would adhere to move to locations that minimize maladaptation risks varied throughout the range. The GDMs predicted an overall northward trajectory for most (71.14%) locations within the range, but substantial variations were observed beyond the core of the range, especially along the northwestern range edge, where populations showed eastward, westward, or even southward trajectories (Figure 6B).
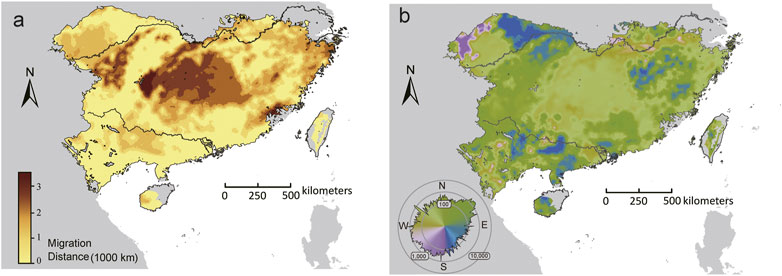
FIGURE 6. The initial bearing (A) and migrate distance (B) to locations that can minimize forward offset in the future climate scenarios (2070, SSP370). The polar histogram in (B) indicated the log10 number of cells within each bearing bin.
4 Discussion
This study demonstrated a broad correlation between genetic and regional climatic difference in this species, and identified climate as a predominant force in driving current patterns of genetic structure. To trace footprints of climate-related adaptation, we adopted a landscape genomics framework to analyze population genomic data, from which candidate SNPs were discovered by OA and EAA methods, then further screened by SNP-specific GDM modelling and functional annotation. Based on the adaptive genomic variants, this study, for the first time, incorporated migration and dispersal into vulnerability assessment of this widespread species in subtropical China. We investigated most vulnerable populations that require proactive management efforts and candidate adaptive genes for breeding and other special agricultural aims. The results highlighted the role of heterogeneous climate conditions of subtropical China in shaping genomic structure and driving local adaptation among populations, facilitating future T. hemsleyanum conservation managements.
4.1 Regional climatic differences contribute to genomic divergence
In this study, we applied RDA and GDM to quantify the contributions of spatial isolation and climate variables to patterns of genomic variation, and obtained concordant results. Both methods identified a larger proportion of SNP variation attributed to climate than to geography (Figure 3), indicating that the assessed climate variables are more influential in driving the pattern of genetic variation in T. hemsleyanum, which has been frequently reported in plant species (Shryock et al., 2017; Vidaller et al., 2020; Feliciano et al., 2022). When we isolate the effect of climate variables, geography alone explained a rather small portion (RDA: 2.5%; GDM: 8.1%; Figure 3), which potentially reflect the absence of unmeasured spatially structured climate variables (Feliciano et al., 2022). Furthermore, our RDA and regional climatic differences revealed that climate predictors acting as key selective agents vary across specific geographic clusters (Figure 2; Supplementary Figure S3).
Unlike GDM analysis, RDA was able to quantify the contribution of the colinear fraction of climate and geography. This fraction, termed as induced spatial dependence (ISD), could represent impact of climatic gradients that are highly related to space (Borcard et al., 2011; Mikulyuk et al., 2011; Ma et al., 2020). While clinal gradients (e.g., latitudinal temperature gradients) are treated as key adaptive evolutionary forces in plant species (Adrion et al., 2015; Kooyers et al., 2015; Gibson and Moyle, 2020), the estimated influence of ISD on genomic variation of T. hemsleyanum was low (6.4%; Figure 3; Table 2). This eliminated some of the confounding effect of demography that could impact the inference of local adaptation.
Apart from quantifying the relative effects of climate and space, we also found specific climate factors that uniquely contributed to genomic variation. In subtropical areas, the survival and distribution of plants has been reported to be constrained by insufficient precipitation (Allan et al., 2010; Aguirre-Gutiérrez et al., 2019), and across the range of T. hemsleyanum, BIO19 and PETWeQ represented the most important predictors of genome-wide SNP variation when taking the effects of spatial isolation into account, which suggested that populations are responding to selective forces related to drought. This result agrees with the general consensus that T. hemsleyanum is best suited to moist hillsides or valleys and is a drought-sensitive species (Du et al., 2015; Song et al., 2017), and reinforces previous findings of distribution models that identified water availability as a major determinant of contemporary distribution range of this species (Wang et al., 2022). Beyond that, GDD0 was by far the most important variable in the temperature regime associated with genetic variation (Table 1), and its corresponding range was from 51,930 days degrees at Xingshan (CC7) in Central China to 1,03,932 days degrees at Janfengling (HN) in South China (Supplementary Table S1). The large variations in temperature conditions during growing seasons reflected differential heat requirement of T. hemsleyanum across regions, and this seemed to be a strong abiotic selective agent, especially between populations in southern and central regions. Indeed, local adaptation of populations for phenological traits in relation to accumulated heat conditions has been described in several species with wide distributions (Khoufi et al., 2013; Olson et al., 2013). As a result, we presume that similar pattern may also be found in T. hemsleyanum populations along the GDD gradient. Such hypothesis is testable in the future, with common garden experiments to assess phenological variation across natural populations that are predicted to differ at specific SNPs associated with growing degree days. This may also aid in the selection of populations genetically adapted in their phenology to face less GDDs and better adapted to cold stress.
Although in our study climatic selection processes appeared to be the most important in driving genomic variation, a large portion of the variation remains unexplained in RDA (63.6%) and GDM (77.6%) analysis, which accords with the other genetic (Jia et al., 2020; Boulanger et al., 2021) and community ecological studies (Ma et al., 2019) using similar approaches. This probably results from several factors not fully addressed in this study. Firstly, despite that many climate predictor variables were considered in this study, other ecological forces may also play a role; these may include biotic interactions, and abiotic factors that were unmeasured or occurred at relatively small scales (Geue et al., 2016; Gibson and Moyle, 2020). Secondly, the remaining variation can be attributed to balancing selection or neutral and/or stochastic process that maintain local allelic diversity, which may weaken the predictive power of the models. Thirdly, the four dbMEM variables generated here may not fully represent geographic heterogeneity present in subtropical China. Lastly, RDA analysis, which model linear associations between geography/climate and SNP loci, cannot fully capture non-linear statistical relationships. As a result, we also applied GDM to the SNP data and detected the non-linear SNP-climate relationships arise across the range of T. hemsleyanum; this would be critical to understand the process of local adaptation in the context of multivariate climates.
4.2 Detection of candidate SNPs by multiple methods
Combining population differentiation with EAA methods is a desirable way to identify potentially adaptive SNPs and reduce the rate of false positives (Martins et al., 2018; Lu et al., 2019). One notable aspect of these two methods employed in this study is the minimal overlap of SNPs we identified. Specifically, only 12 SNPs deemed as FST outliers were detected in BayPass, and between 2.70% and 9.67% of the genotype-environment associations were overlapped among two or more variables (Supplementary Tables S10, S11). The low levels of congruence are not surprising since the two methods captures different selection signatures (Eckert et al., 2010; Hancock et al., 2010; Martins et al., 2018; Lu et al., 2019). OA are generally more sensitive to detect strong signatures of divergent selection acting directly on new mutations, yet it is impossible to determine specific environment forces (de Villemereuil et al., 2014). EAA tests, on the other hand, performs better in identifying polygenic or weak selection signatures (Narum and Hess, 2011; de Villemereuil et al., 2014; Frichot and François, 2015) and are nowadays widely applied to explore adaptive loci with subtle variation across landscape (Jones et al., 2013; Martins et al., 2018). Concordantly, previous studies comparing OA and EAA have found little overlap in the significant SNPs between the approaches (Dudaniec et al., 2018; Lu et al., 2019). A caveat on using EAA is that a locus may not be significantly associated with environmental factors when it is advantageous across a range of environment conditions at the same time (Frichot et al., 2013). The GDM analysis included in our approach is complementary to EAA, since it is capable to simultaneously characterize relative allelic responses across predictor variables.
In forest species, climate adaptation is likely driven by polygenic alleles with small effects (Savolainen et al., 2013; Sang et al., 2022). In T. hemsleyanum, we surmise that a number of SNPs identified here are genetic variants of small effects according to previous reviews and case studies (Mackay et al., 2009; Rockman, 2012; Savolainen et al., 2013; Barghi et al., 2020). Indeed, climate explains a small to moderate portion of allelic turnover for majority of the SNPs detected by multiple models (Supplementary Figure S6; Supplementary Table S12), and the defined climate points of allelic turnover varies across adaptive variants (Supplementary Figure S6), suggesting that climate is affecting each SNP differently. This finding may reflect additive genetic variation related to many genes or genomic regions and multilocus patterns of adaptation (Shaw and Etterson, 2012). Furthermore, for the annotated candidates (listed in Table 3), especially for that highly supported by GDM, the observed FST changes (0.27–0.50) were not biased towards greater values (Supplementary Table S12), which indicated that a higher probability to identify a SNP under selection does not correlate with a greater shift of FST values across climatic gradients. Overall, these observations indicate that climate adaptation in T. hemsleyanum is polygenic and potentially related to both small- and large-effect genetic variants. Future experiments that investigate gene interactions may help to elaborate the polygenic basis of local adaptation (He et al., 2016; De Kort et al., 2022).
Detecting signals of local adaptation is complicated by the issue of disentangling geographically structured variation from adaptive variation (Hoban et al., 2016), which is especially relevant when environmental gradients are highly correlated with neutral structure (Lotterhos and Whitlock, 2015). Given the strong population differentiation and broad correlations between genetic clusters and floristic divisions (Supplementary Figure S10) in this species, we adopted multiple filtering and correcting steps in genome scans to better control for false positives. First, we selected only putatively adaptive SNPs found by two or three outlier tests (only diversifying selection) and excluded SNPs in relation to geography in EAA. Next, considering the small overlap of loci detected by OA and EAA methods, we used two subsequent screening approaches in GDM to control for false positives by 1) excluding loci that had an explanatory power not exceeding the “reference” SNP group and 2) excluding loci with highest partial allelic turnover response to geography. Finally, we focus our interpretation on SNPs located in stimuli response or other ecologically relevant genes, since they offer a better opportunity to elucidate gene function and climatic forces driving the current patterns of adaptive variation. Future whole genome sequencing of T. hemsleyanum individuals may facilitate more comprehensive investigation of genetic targets of selection and mining of adaptive loci valuable for breeding purposes.
4.3 Key genes with a local climate adaptation signature
We retained 275 SNPs with significant signatures of selection, some of which were located in exonic regions of genes associated with plant adaptation to abiotic environment (Table 3; Supplementary Figure S13). Of foremost interest are those genes that act as key regulators of abiotic stress tolerance. For example, one SNP 106296_81 (identified by both OA and EAA methods) resides in the RH7 gene encoding a DEAD box helicase (Table 3). DEAD box helicases serve as important molecular tools in developing stress tolerant plants (Nidumukkala et al., 2019). In Arabidopsis thaliana RH7 allelic mutants, plants showed developmental defects and high sensitivity to cold stress (Huang et al., 2016; Liu et al., 2016). We also found one SNP (108307_57) located in the APX1, which plays a vital role in adaptation of plants to a combination of drought and heat stress (Zandalinas et al., 2018). Not surprising that both EAA and GDM identified this SNP to be significantly associated with potential evapotranspiration (PET) (Table 3; Supplementary Figure S11), which is a climatic measure integrating temperature and humidity to reflect water availability (Rehana and Monish, 2021). We also identified one candidate SNP linked to a pentatricopeptide repeat (PPR) gene. In cotton, a single recessive mutation of a PPR gene reduces the heat accumulation (Kim et al., 2021). In this study, the SNP annotated to the PPR gene showed the highest magnitude of partial allelic turnover in response to GDD (0.88) and served as top-ranked SNPs for growing degree days (9) (Table 3; Supplementary Figure S12), suggesting that this PPR gene is among good candidates for further functional studies.
Beyond that, a role for gene regulation variation, not merely functional variation, for climate adaptation was indicated by the detection of genes encoding several families (bHLH, MYB, WRKY, etc.) of transcription factors (TFs), for example, bHCH93, identified as a key player in regulating flowering (Wang et al., 2017) and abiotic stress responses (Bhaskarla et al., 2020; Samarina et al., 2020; Wang et al., 2021a), and MYB3R1 acting as a “master switch” in a variety of stress tolerance (Dai et al., 2007). The TF families and members we found here mostly match results in previous studies, such as bHLH transcription factors identified as outliers in Corchorus olitorius (Sarkar et al., 2019) and Pinus taeda (Lu et al., 2019), and MYB transcription factors that identified by both OA and EAA methods in Brachypodium distachyon (Dell’Acqua et al., 2014).
Furthermore, we note two candidate genes encoding A-type cyclin (CYCA) and anaphase-promoting complex (APC), both of which control cell cycle progression. Recent studies have begun to address the important role of cell cycle regulator, especially cyclins (such as CYCA2;4 we identified here) and cyclin-dependent kinases (CDKs), in stimulus response of plants (Komaki and Sugimoto, 2012). Arabidopsis thaliana and maize were reported of cell cycle arrest and cell proliferation reduction in response to salt and drought stresses due to the disruption of cell cycle regulators (Kamal et al., 2021).
It is noteworthy that several genes involved in flora reproductive development and flowering time were also identified (Table 3). GRDP2 encodes a novel glycine-rich domain protein that modulates flowering time and ovule development (Ortega-Amaro et al., 2015; Czolpinska and Rurek, 2018; Wang et al., 2021b), and was previously identified to be involved in environmental response in Arabidopsis (Mangeon et al., 2010; Ortega-Amaro et al., 2015). BAM1 has been shown to regulate anther development in Arabidopsis (Hord et al., 2006), and MS5 is known to be essential for male fertility in Arabidopsis and Brassica species (Glover et al., 1998; Zeng et al., 2021). The initiation of reproduction is an important transition of life cycle (Franks and Hoffmann, 2012). In plants, due to their sedentary life-style, the exact timing of flowering has strong impacts on reproductive success and, thus fitness (Anderson et al., 2011). As outlined in previous studies, selection can optimize flowering time to track suitable climatic conditions, thus contributing to plant adaptation to climate change (Sandring and Ågren, 2009; Keller et al., 2012). In this study, we found that in this perennial herb, the candidate genes associated with reproductive development and flowering were mainly significantly related to precipitation and aridity index (Table 3; Supplementary Figure S11).
A few candidate genes were ambiguous in how they are associated with climate. For example, an LRR (Leucine-rich repeat) receptor-like serine/threonine-protein kinase gene, associated with precipitation, appears to be involved in plant-pathogen interaction and developmental control (Afzal et al., 2008). Precipitation and moisture availability have a positive impact on the dispersal and infection success of phytopathogens (Swinfield et al., 2012; Milici et al., 2020), and pathogen recognition could be vital for plants in a mesic climate (Ahrens et al., 2019).
Overall, the results indicate that adaptation to climate is polygenic, potentially involving multiple adaptive mechanisms (Jordan et al., 2017; Dudaniec et al., 2018; Ahrens et al., 2019). Although the genes we identified only form a part of the broader adaptive evolutionary processes, we can take advantage of these patterns as a proxy to generate spatial patterns of adaptive variation and improve future management strategies. Future studies, based on high-quality genomic sequencing, knockout mutations and common garden experiments, are in need to validate the identified candidate gene regions in this study (Rellstab et al., 2015).
4.4 Population-level risk of future climate change
The influence of future shifts in climate across species’ distribution range is mediated by the collective potential of adapted populations to climate and/or migration in response. But few studies integrated intra-specific adaptation and migration when predicting how species responses to climate change (but see Sang et al., 2022). In this study, we followed a novel approach presented in Gougherty et al. (2021) to assess the contribution of in situ adaptation versus migration by simultaneously calculating three metrics of genomic offset. Overall, it is predicted that the genomic offsets are highest in central-north part of the species’ range, suggesting that there are no extant populations throughout the distribution range preadapted to the future climate in this area. Moreover, the effect of local climate shifts in this area cannot be mitigated by movement of populations to more suitable climate conditions, since the distances to locations that can minimize future maladaptation were predicted to exceed 3,000 km (largest within the range we assessed) that realistically is not reachable given the seed and pollen dispersal limitations. On the other hand, the genomic offset (vulnerability) of T. hemsleyanum was generally low in the southern (trailing) edge, which is in contrast with some theoretical work (Hampe and Petit, 2005). These patterns partially reflect the fact that the influence of temperature is secondary to that of precipitation/moisture condition in promoting the adaptive differentiation of loci we investigated, since precipitation in the coldest quarter is the most important climate variable in our GDM models (Supplementary Figure S7). The dominant effect of winter precipitation probably results from the involvement of a portion of candidate SNPs in drought response and/or phenological events in cool season. Future projection of precipitation predicted that the most notable change (a deceasing trend) of winter precipitation within our sampling area will occur in the middle reaches of the Yangtze river basin, especially along the mid-altitude mountainous region (Bucchignani et al., 2014; Bao et al., 2015) that largely corresponded to the central-north part of the species’ range. In addition to the winter precipitation decrease, the rising winter temperature will lead to increasing evapotranspiration, and exacerbate soil moisture losses. As predicted by high-resolution climate simulations (Yin et al., 2015; Ma et al., 2019), mutual reinforcement of these effects would induce a shift towards greater aridity in this humid region (aridity index increase ∼20% in 2070–2099) that populations may have not undergone in the recent past, thus rendering high adaptive offsets in central-north part of the range. Perhaps as a consequence of this, we observed significant associations of local and forward offsets not only with shifts in BIO19 and PETs (two most predictive variables in GDM), but with shifts in aridity index (Supplementary Figure S9). Besides, a general lack of correlation was observed between local/forward offset and the underlying pattern of population structure. This finding suggested that the genomic offsets in our focal species mainly reflect the influence of climate adaptation instead of the expected shifts in neutral variation.
Besides, our results further revealed substantial difference in the direction of migration that populations would follow to mitigate maladaptation to future climate challenges, and such pattern has been reported by several recent studies (Shaw, 2018; Gougherty et al., 2021). While most locations in the contemporary species range showed an overall northward shift, GDM indicated diverse population-level trajectories especially along the northwestern range edge (Figure 6B). Future climate projections by multiple general circulation models consistently discovered that subtropical China may experience an enhancement of spatial heterogeneity in precipitation and an expansion of arid regions (TCNARCC, 2011; Zhao and Wu, 2014). Since precipitation and PET related factors were the most significant predictors for GDMs, we propose that future shifts in precipitation regimes and moisture availability may account for some of the non-northward dispersal of T. hemsleyanum in our predictions.
Local populations vulnerable to future climate may occupy substantial unique adaptive genetic resources, thus special conservation and management efforts could be sensible as an insurance against such future genetic losses. For example, in central-north part of the range where T. hemsleyanum populations demonstrated maladaptation, we propose assisted gene flow strategy that involves the translocation of genotypes preadapted to future climate scenarios, particularly introducing from the moist and warm regions in southern margin of the mainland China. The genome-informed assisted gene flow can be beneficial for threatened species, as it may increase adaptive potential and alleviate inbreeding depression by introducing and increasing the frequency of adaptive alleles (Browne et al., 2019).
The reliability of genomic offsets as metrics of climate maladaptation was confirmed by recent works using data from population trend surveys and common garden experiments (Bay et al., 2018; Fitzpatrick et al., 2021). But it should be noted that the assumptions of future maladaptation made here is based on genomic SNP data, which does not account for alternatives (beyond allelic changes) for continued adaptation provided by epigenetic and expression changes, and phenotypic plasticity (Kenkel and Matz, 2016; Gao et al., 2022). Furthermore, we recognize that although we adopted GDMs to predict the genomic vulnerability to climate change, the genomic complexity of polygenic climate adaptation, for example, genetic redundancy and pleiotropy, have not been accounted here. It would be valuable to integrate quantitative genetics and systems biology methods to validate the current genotype–environment interactions and improve the prediction of species’ response to future climate challenges.
Data availability statement
The datasets presented in this study can be found in online repositories. The names of the repository/repositories and accession number(s) can be found below: https://www.ncbi.nlm.nih.gov/sra/, PRJNA922859.
Author contributions
YW acquired funding. LF and PG designed the conceptual approach. YZ carried out the field work, and LZ performed the molecular laboratory work. WM analyzed the genomic data. ML generated and analyzed climate data. YW wrote the manuscript, with major contributions from CF and LF. All authors read and approved the final version of the manuscript.
Funding
This research was supported by the National Natural Science Foundation of China (Nos. 32271550, 31700193), Henan Province Postdoctoral Research Grant (No. 201901020), Scientific and Technological Project of Henan Province (No. 202102110004) and Young Elite Scientists Program by Henan Agricultural University (30500580).
Acknowledgments
The authors are grateful to Yuanmiao Fu, Pan Li, Li Zheng, and Shuting Yang for collecting plant materials, to Rebecca Jordan, Wen Yao, and Nan Lin for advice on data analysis, and to Prof. Fude Shang for great support with laboratory work.
Conflict of interest
The authors declare that the research was conducted in the absence of any commercial or financial relationships that could be construed as a potential conflict of interest.
Publisher’s note
All claims expressed in this article are solely those of the authors and do not necessarily represent those of their affiliated organizations, or those of the publisher, the editors and the reviewers. Any product that may be evaluated in this article, or claim that may be made by its manufacturer, is not guaranteed or endorsed by the publisher.
Supplementary material
The Supplementary Material for this article can be found online at: https://www.frontiersin.org/articles/10.3389/fgene.2023.1150704/full#supplementary-material
References
Adrion, J. R., Hahn, M. W., and Cooper, B. S. (2015). Revisiting classic clines in Drosophila melanogaster in the age of genomics. Trends Genet. 31, 434–444. doi:10.1016/j.tig.2015.05.006
Afzal, A. J., Wood, A. J., and Lightfoot, D. A. (2008). Plant receptor-like serine threonine kinases: Roles in signaling and plant defense. Mol. Plant-Microbe Interact. 21, 507–517. doi:10.1094/MPMI-21-5-0507
Aguirre-Gutiérrez, J., Oliveras, I., Rifai, S., Fauset, S., Adu-Bredu, S., Affum-Baffoe, K., et al. (2019). Drier tropical forests are susceptible to functional changes in response to a long-term drought. Ecol. Lett. 22, 855–865. doi:10.1111/ele.13243
Aguirre-Liguori, J. A., Ramírez-Barahona, S., and Gaut, B. S. (2021). The evolutionary genomics of species’ responses to climate change. Nat. Ecol. Evol. 5, 1350–1360. doi:10.1038/s41559-021-01526-9
Ahrens, C. W., Byrne, M., and Rymer, P. D. (2019). Standing genomic variation within coding and regulatory regions contributes to the adaptive capacity to climate in a foundation tree species. Mol. Ecol. 28, 2502–2516. doi:10.1111/mec.15092
Allan, R. P., Soden, B. J., John, V. O., Ingram, W., and Good, P. (2010). Current changes in tropical precipitation. Environ. Res. Lett. 5, 025205. doi:10.1088/1748–9326/5/2/025205
Anderson, J. T., Willis, J. H., and Mitchell-Olds, T. (2011). Evolutionary genetics of plant adaptation. Trends Genet. 27, 258–266. doi:10.1016/j.tig.2011.04.001
Bao, J., Feng, J., and Wang, Y. (2015). Dynamical downscaling simulation and future projection of precipitation over China. J. Geophys. Res. Atmos. 120, 8227–8243. doi:10.1002/2015JD023275
Barghi, N., Hermisson, J., and Schlötterer, C. (2020). Polygenic adaptation: A unifying framework to understand positive selection. Nat. Rev. Genet. 21, 769–781. doi:10.1038/s41576-020-0250-z
Bay, R. A., Harrigan, R. J., Underwood, V. L., Gibbs, H. L., Smith, T. B., and Ruegg, K. (2018). Genomic signals of selection predict climate-driven population declines in a migratory bird. Science 359, 83–86. doi:10.1126/science.aan4380
Bhaskarla, V., Zinta, G., Ford, R., Jain, M., Varshney, R. K., and Mantri, N. (2020). Comparative root transcriptomics provide insights into drought adaptation strategies in chickpea (Cicer arietinum L.). Int. J. Mol. Sci. 21, 1781. doi:10.3390/ijms21051781
Borcard, D., Gillet, F., and Legendre, P. (2011). “Eigenvector-based spatial variables and spatial modelling,” in Numerical ecology with R. Editors D. Borcard, F. Gillet, and P. Legendre (New York: Springer), 243–285.
Borcard, D., Gillet, F., and Legendre, P. (2018). “Spatial analysis of ecological data,” in Numerical ecology with R. Editors D. Borcard, F. Gillet, and P. Legendre (Cham: Springer), 299–367.
Boulanger, E., Loiseau, N., Valentini, A., Arnal, V., Boissery, P., Dejean, T., et al. (2021). Environmental DNA metabarcoding reveals and unpacks a biodiversity conservation paradox in Mediterranean marine reserves. Proc. R. Soc. Lond. B Biol. Sci. 288, 20210112. doi:10.1098/rspb.2021.0112
Browne, L., Wright, J. W., Fitz-Gibbon, S., Gugger, P. F., and Sork, V. L. (2019). Adaptational lag to temperature in valley oak (Quercus lobata) can be mitigated by genome-informed assisted gene flow. Proc. Natl. Acad. Sci. USA. 116, 25179–25185. doi:10.1073/pnas.1908771116
Brunner, M. I., Melsen, L. A., Newman, A. J., Wood, A. W., and Clark, M. P. (2020). Future streamflow regime changes in the United States: Assessment using functional classification. Hydrol. Earth Syst. Sci. 24, 3951–3966. doi:10.5194/hess-24-3951-2020
Bucchignani, E., Montesarchio, M., Cattaneo, L., Manzi, M. P., and Mercogliano, P. (2014). Regional climate modeling over China with COSMO-CLM: Performance assessment and climate projections. J. Geophys. Res. Atmos. 119, 12,151–12,170. doi:10.1002/2014JD022219
Cai, L., Arnold, B. J., Xi, Z., Khost, D. E., Patel, N., Hartmann, C. B., et al. (2021). Deeply altered genome architecture in the endoparasitic flowering plant Sapria himalayana griff. (Rafflesiaceae). Curr. Biol. 31, 1002–1011. e9. doi:10.1016/j.cub.2020.12.045
Cao, Y. N., Zhu, S. S., Chen, J., Comes, H. P., Wang, I. J., Chen, L. Y., et al. (2020). Genomic insights into historical population dynamics, local adaptation, and climate change vulnerability of the East Asian Tertiary relict Euptelea (Eupteleaceae). Evol. Appl. 13, 2038–2055. doi:10.1111/eva.12960
Capblancq, T., Afitzpatrick, M., Abay, R., Aexposito-Alonso, M., and Akeller, S. (2020). Genomic prediction of (Mal)Adaptation across current and future climatic landscapes. Annu. Rev. Ecol. Evol. S. 51 (1), 245–269. doi:10.1146/annurev-ecolsys-020720-042553
Capblancq, T., Lachmuth, S., Fitzpatrick, M. C., and Keller, S. R. (2022). From common gardens to candidate genes: Exploring local adaptation to climate in red spruce. New Phytol. 121, 1590–1605. doi:10.1111/nph.18465
Catchen, J. M., Amores, A., Hohenlohe, P., Cresko, W., and Postlethwait, J. H. (2011). Stacks: Building and genotyping loci de novo from short-read sequences. G3-Genes Genom Genet. 1, 171–182. doi:10.1534/g3.111.000240
Chang, C. W., Fridman, E., Mascher, M., Himmelbach, A., and Schmid, K. (2022). Physical geography, isolation by distance and environmental variables shape genomic variation of wild barley (Hordeum vulgare L. ssp. spontaneum) in the Southern Levant. Heredity 128, 107–119. doi:10.1038/s41437-021-00494-x
Cingolani, P., Platts, A., Wang, L. L., Coon, M., Nguyen, T., Wang, L., et al. (2012). A program for annotating and predicting the effects of single nucleotide polymorphisms, SnpEff: SNPs in the genome of Drosophila melanogaster strain w1118; iso-2; iso-3. Fly 6, 80–92. doi:10.4161/fly.19695
Czolpinska, M., and Rurek, M. (2018). Plant glycine-rich proteins in stress response: An emerging, still prospective story. Front. Plant Sci. 9, 302. doi:10.3389/fpls.2018.00302
Dai, X., Xu, Y., Ma, Q., Xu, W., Wang, T., Xue, Y., et al. (2007). Overexpression of an R1R2R3 MYB gene, OsMYB3R-2, increases tolerance to freezing, drought, and salt stress in transgenic Arabidopsis. Plant Physiol. 143, 1739–1751. doi:10.1104/pp.106.094532
Danecek, P., Auton, A., Abecasis, G., Albers, C. A., Banks, E., DePristo, M. A., et al. (2011). The variant call format and VCFtools. Bioinformatics 27, 2156–2158. doi:10.1093/bioinformatics/btr330
De Kort, H., Toivainen, T., Van Nieuwerburgh, F., Andrés, J., Hytönen, T. P., and Honnay, O. (2022). Signatures of polygenic adaptation align with genome-wide methylation patterns in wild strawberry plants. New Phytol. 235, 1501–1514. doi:10.1111/nph.18225
De Villemereuil, P., Frichot, É., Bazin, É., François, O., and Gaggiotti, O. E. (2014). Genome scan methods against more complex models: When and how much should we trust them? Mol. Ecol. 23, 2006–2019. doi:10.1111/mec.12705
Dell’Acqua, M., Zuccolo, A., Tuna, M., Gianfranceschi, L., and Pè, M. E. (2014). Targeting environmental adaptation in the monocot model Brachypodium distachyon: A multi-faceted approach. BMC Genomics 15, 801. doi:10.1186/1471-2164-15-801
DePristo, M. A., Banks, E., Poplin, R., Garimella, K. V., Maguire, J. R., Hartl, C., et al. (2011). A framework for variation discovery and genotyping using next-generation DNA sequencing data. Nat. Genet. 43, 491–498. doi:10.1038/ng.806
Du, S., Xiang, T., Song, Y., Huang, L., Sun, Y., and Han, Y. (2015). Transgenic hairy roots of Tetrastigma hemsleyanum: Induction, propagation, genetic characteristics and medicinal components. Plant Plant Cell. tiss.org. 122, 373–382. doi:10.1007/s11240-015-0775-6
Dudaniec, R. Y., Yong, C. J., Lancaster, L. T., Svensson, E. I., and Hansson, B. (2018). Signatures of local adaptation along environmental gradients in a range-expanding damselfly (Ischnura elegans). Mol. Ecol. 27, 2576–2593. doi:10.1111/mec.14709
Dyderski, M. K., Paź, S., Frelich, L. E., and Jagodziński, A. M. (2018). How much does climate change threaten European forest tree species distributions? Glob. Change Biol. 24, 1150–1163. doi:10.1111/gcb.13925
Earl, D. A., and vonHoldt, B. M. (2012). Structure harvester: A website and program for visualizing STRUCTURE output and implementing the Evanno method. Conserv. Genet. Resour. 4, 359–361. doi:10.1007/s12686-011-9548-7
Eckert, A. J., Bower, A. D., GonzÁLez-MartÍNez, S. C., Wegrzyn, J. L., Coop, G., and Neale, D. B. (2010). Back to nature: Ecological genomics of loblolly pine (Pinus taeda, pinaceae). Mol. Ecol. 19, 3789–3805. doi:10.1111/j.1365-294X.2010.04698.x
Evanno, G., Regnaut, S., and Goudet, J. (2005). Detecting the number of clusters of individuals using the software structure: A simulation study. Mol. Ecol. 14, 2611–2620. doi:10.1111/j.1365-294X.2005.02553.x
Excoffier, L., and Lischer, H. E. L. (2010). Arlequin suite ver 3.5: A new series of programs to perform population genetics analyses under linux and windows. Mol. Ecol. Resour. 10, 564–567. doi:10.1111/j.1755-0998.2010.02847.x
Exposito-Alonso, M., Booker, T. R., Czech, L., Gillespie, L., Hateley, S., Kyriazis, C. C., et al. (2022). Genetic diversity loss in the Anthropocene. Science 377 (6613), 1431–1435. doi:10.1126/science.abn5642
Exposito-Alonso, M., Exposito-Alonso, M., Gómez Rodríguez, R., Barragán, C., Capovilla, G., Chae, E., et al. (2019). Natural selection on the Arabidopsis thaliana genome in present and future climates. Nature 573, 126–129. doi:10.1038/s41586-019-1520-9
Exposito-Alonso, M., Vasseur, F., Ding, W., Wang, G., Burbano, H. A., and Weigel, D. (2018). Genomic basis and evolutionary potential for extreme drought adaptation in Arabidopsis thaliana. Nat. Ecol. Evol. 2, 352–358. doi:10.1038/s41559-017-0423-0
Feliciano, D. C., De Godoy, S. M., Da Silva, J. F. M., Góes, B. D., Ferraz, J. R., De Oliveira Santos, P., et al. (2022). Landscape genetics reveal low diversity and adaptive divergence in Portulaca hatschbachii (portulacaceae): An endangered species endemic to rocky outcrops of the atlantic forest. Bot. J. Linn. Soc. 200, 116–141. doi:10.1093/botlinnean/boac006
Feng, L., and Du, F. K. (2022). Landscape genomics in tree conservation under a changing environment. Front. Plant Sci. 13, 822217. doi:10.3389/fpls.2022.822217
Ferrier, S., Manion, G., Elith, J., and Richardson, K. (2007). Using generalized dissimilarity modelling to analyse and predict patterns of beta diversity in regional biodiversity assessment. Divers. Distrib. 13, 252–264. doi:10.1111/j.1472-4642.2007.00341.x
Fitzpatrick, M. C., Chhatre, V. E., Soolanayakanahally, R. Y., and Keller, S. R. (2021). Experimental support for genomic prediction of climate maladaptation using the machine learning approach Gradient Forests. Mol. Ecol. Resour. 21, 2749–2765. doi:10.1111/1755-0998.13374
Fitzpatrick, M. C., and Keller, S. R. (2015). Ecological genomics meets community-level modelling of biodiversity: Mapping the genomic landscape of current and future environmental adaptation. Ecol. Lett. 18, 1–16. doi:10.1111/ele.12376
Foll, M., and Gaggiotti, O. (2008). A genome-scan method to identify selected loci appropriate for both dominant and codominant markers: A bayesian perspective. Genetics 180, 977–993. doi:10.1534/genetics.108.092221
Forester, B. R., Lasky, J. R., Wagner, H. H., and Urban, D. L. (2018). Comparing methods for detecting multilocus adaptation with multivariate genotype–environment associations. Mol. Ecol. 27, 2215–2233. doi:10.1111/mec.14584
Franks, S., and Hoffmann, A. (2012). Genetics of climate change adaptation. Annu. Rev. Genet. 46, 185–208. doi:10.1146/annurev-genet-110711-155511
Frichot, E., and François, O. (2015). Lea: An R package for landscape and ecological association studies. Methods Ecol. Evol. 6, 925–929. doi:10.1111/2041-210X.12382
Frichot, E., Schoville, S. D., Bouchard, G., and François, O. (2013). Testing for associations between loci and environmental gradients using latent factor mixed models. Mol. Biol. Evol. 30, 1687–1699. doi:10.1093/molbev/mst063
Gao, Y., Chen, Y., Li, S., Huang, X., Hu, J., Bock, D. G., et al. (2022). Complementary genomic and epigenomic adaptation to environmental heterogeneity. Mol. Ecol. 31, 3598–3612. doi:10.1111/mec.16500
Gautier, M. (2015). Genome-wide scan for adaptive divergence and association with population-specific covariates. Genetics 201, 1555–1579. doi:10.1534/genetics.115.181453
Geue, J. C., Vágási, C. I., Schweizer, M., Pap, P. L., and Thomassen, H. A. (2016). Environmental selection is a main driver of divergence in house sparrows (Passer domesticus) in Romania and Bulgaria. Ecol. Evol. 6, 7954–7964. doi:10.1002/ece3.2509
Gibson, M. J. S., and Moyle, L. C. (2020). Regional differences in the abiotic environment contribute to genomic divergence within a wild tomato species. Mol. Ecol. 29, 2204–2217. doi:10.1111/mec.15477
Glover, J., Grelon, M., Craig, S., Chaudhury, A., and Dennis, E. (1998). Cloning and characterization of MS5 from Arabidopsis: A gene critical in male meiosis. Plant J. 15, 345–356. doi:10.1046/j.1365-313X.1998.00216.x
Goudet, J. (2005). Hierfstat, a package for r to compute and test hierarchical F-statistics. Mol. Ecol. Notes 5, 184–186. doi:10.1111/j.1471-8286.2004.00828.x
Gougherty, A. V., Keller, S. R., and Fitzpatrick, M. C. (2021). Maladaptation, migration and extirpation fuel climate change risk in a forest tree species. Nat. Clim. Change 11, 166–171. doi:10.1038/s41558-020-00968-6
Günther, T., and Coop, G. (2013). Robust identification of local adaptation from allele frequencies. Genetics 195, 205–220. doi:10.1534/genetics.113.152462
Guo, W., Yang, Z., Hou, Z., Hou, Z., Qi, Z., and Sun, Y. (2019). A comprehensive review of a Chinese folk herbal species Tetrastigmae hemsleyanum with multiplicity of pharmacological effects. Chin. Trad. Med. J. 1, 1–19.
Hampe, A., and Petit, R. J. (2005). Conserving biodiversity under climate change: The rear edge matters. Ecol. Lett. 8, 461–467. doi:10.1111/j.1461-0248.2005.00739.x
Hancock, A. M., Alkorta-Aranburu, G., Witonsky, D. B., and Di Rienzo, A. (2010). Adaptations to new environments in humans: The role of subtle allele frequency shifts. Philos. Trans. R. Soc. Lond B Biol. Sci. 365, 2459–2468. doi:10.1098/rstb.2010.0032
Harris, K., and Nielsen, R. (2014). Error-prone polymerase activity causes multinucleotide mutations in humans. Genome Res. 24, 1445–1454. doi:10.1101/gr.170696.113
He, F., Arce, A. L., Schmitz, G., Koornneef, M., Novikova, P., Beyer, A., et al. (2016). The footprint of polygenic adaptation on stress-responsive cis-regulatory divergence in the Arabidopsis genus. Mol. Biol. Evol. 33, 2088–2101. doi:10.1093/molbev/msw096
Hijmans, R. J., Cameron, S. E., Parra, J. L., Jones, P. G., and Jarvis, A. (2005). Very high resolution interpolated climate surfaces for global land areas. Int. J. Climatol. 25, 1965–1978. doi:10.1002/joc.1276
Hijmans, R. J., Williams, E., Vennes, C., and Hijmans, M. R. J. (2017). Package ‘geosphere’. Spherical trigonometry. Version 1.5-18.
Hoban, S., Kelley, J. L., Lotterhos, K. E., Antolin, M. F., Bradburd, G., Lowry, D. B., et al. (2016). Finding the genomic basis of local adaptation: Pitfalls, practical solutions, and future directions. Am. Nat. 188, 379–397. doi:10.1086/688018
Hohenlohe, P. A., Phillips, P. C., and Cresko, W. A. (2010). Using population genomics to detect selection in natural populations: Key concepts and methodological considerations. Int. J. Philos. Stud. 171, 1059–1071. doi:10.1086/656306
Hord, C. L. H., Chen, C., DeYoung, B. J., Clark, S. E., and Ma, H. (2006). The BAM1/BAM2 receptor-like kinases are important regulators of Arabidopsis early anther development. Plant Cell. 18, 1667–1680. doi:10.1105/tpc.105.036871
Hu, W., Zheng, Y., Xia, P., and Liang, Z. (2021). The research progresses and future prospects of Tetrastigma hemsleyanum diels et gilg: A valuable Chinese herbal medicine. J. Ethnopharmacol. 271, 113836. doi:10.1016/j.jep.2021.113836
Huang, C.-K., Shen, Y.-L., Huang, L.-F., Wu, S.-J., Yeh, C.-H., and Lu, C.-A. (2016). The DEAD-box RNA helicase AtRH7/PRH75 participates in pre-rRNA processing, plant development and cold tolerance in Arabidopsis. Plant Cell. Physiol. 57, 174–191. doi:10.1093/pcp/pcv188
Jia, K. H., Zhao, W., Maier, P. A., Hu, X. G., Jin, Y., Zhou, S. S., et al. (2020). Landscape genomics predicts climate change-related genetic offset for the widespread Platycladus orientalis (Cupressaceae). Evol. Appl. 13, 665–676. doi:10.1111/eva.12891
Jiang, W. (2015). “Studies on germplasm evaluation and quality controltechnology of Tetrastigma hemsleyanum,”. dissertation/doctoral thesis (Zhejiang, China: Zhejiang University).
Jombart, T. (2008). adegenet: a R package for the multivariate analysis of genetic markers. Bioinformatics 24, 1403–1405. doi:10.1093/bioinformatics/btn129
Jombart, T., and Ahmed, I. (2011). Adegenet 1.3-1: new tools for the analysis of genome-wide SNP data. Bioinformatics 27, 3070–3071. doi:10.1093/bioinformatics/btr521
Jones, M. R., Forester, B. R., Teufel, A. I., Adams, R. V., Anstett, D. N., Goodrich, B. A., et al. (2013). Integrating landscape genomics and spatially explicit approaches to detect loci under selection in clinal populations. Evolution 67, 3455–3468. doi:10.1111/evo.12237
Jordan, R., Hoffmann, A. A., Dillon, S. K., and Prober, S. M. (2017). Evidence of genomic adaptation to climate in Eucalyptus microcarpa: Implications for adaptive potential to projected climate change. Mol. Ecol. 26, 6002–6020. doi:10.1111/mec.14341
Kamal, K. Y., Khodaeiaminjan, M., Yahya, G., El-Tantawy, A. A., Abdel El-Moneim, D., El-Esawi, M. A., et al. (2021). Modulation of cell cycle progression and chromatin dynamic as tolerance mechanisms to salinity and drought stress in maize. Physiol. Plant. 172, 684–695. doi:10.1111/ppl.13260
Keller, S. R., Levsen, N., Olson, M. S., and Tiffin, P. (2012). Local adaptation in the flowering-time gene network of balsam poplar, Populus balsamifera L. Mol. Biol. Evol. 29, 3143–3152. doi:10.1093/molbev/mss121
Kenkel, C. D., and Matz, M. V. (2016). Gene expression plasticity as a mechanism of coral adaptation to a variable environment. Nat. Ecol. Evol. 1, 0014. doi:10.1038/s41559-016-0014
Khoufi, S., Khamassi, K., Teixeira da Silva, J. A., Aoun, N., Rezgui, S., and Ben Jeddi, F. (2013). Assessment of diversity of phenologically and morphologically related traits among adapted populations of sunflower (Helianthus annuus L.). Helia 36, 29–40. doi:10.2298/hel1358029k
Kim, H. J., Kato, N., Ndathe, R., Thyssen, G. N., Jones, D. C., and Ratnayaka, H. H. (2021). Evidence for thermosensitivity of the cotton (Gossypium hirsutum L.) immature fiber (im) mutant via hypersensitive stomatal activity. PLoS One 16, e0259562. doi:10.1371/journal.pone.0259562
Kissoudis, C., Sunarti, S., van de Wiel, C., Visser, R. G., van der Linden, C. G., and Bai, Y. (2016). Responses to combined abiotic and biotic stress in tomato are governed by stress intensity and resistance mechanism. J. Exp. Bot. 67, 5119–5132. doi:10.1093/jxb/erw285
Komaki, S., and Sugimoto, K. (2012). Control of the plant cell cycle by developmental and environmental cues. Plant Cell. Physiol. 53, 953–964. doi:10.1093/pcp/pcs070
Kooyers, N. J., Greenlee, A. B., Colicchio, J. M., Oh, M., and Blackman, B. K. (2015). Replicate altitudinal clines reveal that evolutionary flexibility underlies adaptation to drought stress in annual Mimulus guttatus. New Phytol. 206, 152–165. doi:10.1111/nph.13153
Lasky, J. R., Des Marais, D. L., McKay, J. K., Richards, J. H., Juenger, T. E., and Keitt, T. H. (2012). Characterizing genomic variation of Arabidopsis thaliana: The roles of geography and climate. Mol. Ecol. 21, 5512–5529. doi:10.1111/j.1365-294X.2012.05709.x
Li, H. (2013). Aligning sequence reads, clone sequences and assembly contigs with BWA-MEM. arXiv preprint arXiv:1303.3997.
Liu, Y., Tabata, D., and Imai, R. (2016). A cold-inducible DEAD-box RNA helicase from Arabidopsis thaliana regulates plant growth and development under low temperature. PLoS One 11, e0154040. doi:10.1371/journal.pone.0154040
Lotterhos, K. E., and Whitlock, M. C. (2015). The relative power of genome scans to detect local adaptation depends on sampling design and statistical method. Mol. Ecol. 24, 1031–1046. doi:10.1111/mec.13100
Lu, M., Loopstra, C. A., and Krutovsky, K. V. (2019). Detecting the genetic basis of local adaptation in loblolly pine (Pinus taeda L.) using whole exome-wide genotyping and an integrative landscape genomics analysis approach. Ecol. Evol. 9, 6798–6809. doi:10.1002/ece3.5225
Ma, D., Deng, H., Yin, Y., Wu, S., and Zheng, D. (2019). Sensitivity of arid/humid patterns in China to future climate change under a high-emissions scenario. J. Geog. Sci. 29, 29–48. doi:10.1007/s11442-019-1582-5
Ma, J.-Z., Chen, X., Mallik, A., Bu, Z.-J., Zhang, M.-M., Wang, S.-Z., et al. (2020). Environmental together with interspecific interactions determine bryophyte distribution in a protected mire of northeast China. Front. Earth Sci. 8, 32. doi:10.3389/feart.2020.00032
Mackay, T. F. C., Stone, E. A., and Ayroles, J. F. (2009). The genetics of quantitative traits: Challenges and prospects. Nat. Rev. Genet. 10, 565–577. doi:10.1038/nrg2612
Malhi, Y., Franklin, J., Seddon, N., Solan, M., Turner, M. G., Field, C. B., et al. (2020). Climate change and ecosystems: Threats, opportunities and solutions. Philos. Trans. R. Soc. B 375(1794), 20190104. doi:10.1098/rstb.2019.0104
Mangeon, A., Junqueira, R. M., and Sachetto-Martins, G. (2010). Functional diversity of the plant glycine-rich proteins superfamily. Plant Signal. Behav. 5, 99–104. doi:10.4161/psb.5.2.10336
Manion, G., Lisk, M., Ferrier, S., Lugilde, K. M., Fitzpatrick, M. C., Fitzpatrick, M. M. C., et al. (2017). Package ‘gdm’. A toolkit with functions to fit, plot, and summarize generalized dissimilarity models: CRAN repository.
Martins, K., Gugger, P. F., Llanderal-Mendoza, J., González-Rodríguez, A., Fitz-Gibbon, S. T., Zhao, J.-L., et al. (2018). Landscape genomics provides evidence of climate-associated genetic variation in Mexican populations of Quercus rugosa. Evol. Appl. 11, 1842–1858. doi:10.1111/eva.12684
Matasci, N., Hung, L.-H., Yan, Z., Carpenter, E. J., Wickett, N. J., Mirarab, S., et al. (2014). Data access for the 1,000 Plants (1KP) project. GigaScience 3, 17. doi:10.1186/2047-217X-3-17
Mikulyuk, A., Sharma, S., Van Egeren, S., Erdmann, E., Nault, M. E., and Hauxwell, J. (2011). The relative role of environmental, spatial, and land-use patterns in explaining aquatic macrophyte community composition. Can. J. Fish. Aquat.Sci. 68, 1778–1789. doi:10.1139/f2011-095
Milici, V. R., Dalui, D., Mickley, J. G., and Bagchi, R. (2020). Responses of plant–pathogen interactions to precipitation: Implications for tropical tree richness in a changing world. J. Ecol. 108, 1800–1809. doi:10.1111/1365-2745.13373
Murray, K. D., Janes, J. K., Jones, A., Bothwell, H. M., Andrew, R. L., and Borevitz, J. O. (2019). Landscape drivers of genomic diversity and divergence in woodland Eucalyptus. Mol. Ecol. 28, 5232–5247. doi:10.1111/mec.15287
Nadeau, S., Meirmans, P. G., Aitken, S. N., Ritland, K., and Isabel, N. (2016). The challenge of separating signatures of local adaptation from those of isolation by distance and colonization history: The case of two white pines. Ecol. Evol. 6, 8649–8664. doi:10.1002/ece3.2550
Narum, S. R., and Hess, J. E. (2011). Comparison of FST outlier tests for SNP loci under selection. Mol. Ecol. Resour. 11, 184–194. doi:10.1111/j.1755-0998.2011.02987.x
Nidumukkala, S., Tayi, L., Chittela, R. K., Vudem, D. R., and Khareedu, V. R. (2019). DEAD box helicases as promising molecular tools for engineering abiotic stress tolerance in plants. Crit. Rev. Biotechnol. 39, 395–407. doi:10.1080/07388551.2019.1566204
Oksanen, J., Blanchet, F., Friendly, M., Kindt, R., Legendre, P., McGlinn, D., et al. (2019). Vegan: Community ecology package (version 2.5-6). The comprehensive R archive network.
Olson, M. S., Levsen, N., Soolanayakanahally, R. Y., Guy, R. D., Schroeder, W. R., Keller, S. R., et al. (2013). The adaptive potential of Populus balsamifera L. to phenology requirements in a warmer global climate. Mol. Ecol. 22, 1214–1230. doi:10.1111/mec.12067
Ortega-Amaro, M. A., Rodríguez-Hernández, A. A., Rodríguez-Kessler, M., Hernández-Lucero, E., Rosales-Mendoza, S., Ibáñez-Salazar, A., et al. (2015). Overexpression of AtGRDP2, a novel glycine-rich domain protein, accelerates plant growth and improves stress tolerance. Front. Plant Sci. 5, 782. doi:10.3389/fpls.2014.00782
Peterson, A. T., Soberón, J., Pearson, R. G., Anderson, R. P., Martínez-Meyer, E., Nakamura, M., et al. (2012). Ecological niches and geographic distributions (MPB-49). Princeton: Princeton University Press.
Pina-Martins, F., Silva, D. N., Fino, J., and Paulo, O. S. (2017). Structure_threader: An improved method for automation and parallelization of programs structure, fastStructure and MavericK on multicore CPU systems. Mol. Ecol. Resour. 17, e268–e274. doi:10.1111/1755-0998.12702
Pritchard, J. K., Wen, X., and Falush, D. (2009). Documentation for structure software: Version 2.3. Waltham: Thermo Fisher scientific. Available at: http://pritch.bsd.uchicago.edu/structure.html.
Purcell, S., Neale, B., Todd-Brown, K., Thomas, L., Ferreira, M. A. R., Bender, D., et al. (2007). Plink: A tool set for whole-genome association and population-based linkage analyses. Am. J. Hum. Genet. 81, 559–575. doi:10.1086/519795
Quinlan, A. R., and Hall, I. M. (2010). BEDTools: A flexible suite of utilities for comparing genomic features. Bioinformatics 26, 841–842. doi:10.1093/bioinformatics/btq033
Rehana, S., and Monish, N. T. (2021). Impact of potential and actual evapotranspiration on drought phenomena over water and energy-limited regions. Theor. Appl. Climatol. 144, 215–238. doi:10.1007/s00704-021-03521-3
Rehfeldt, G. E., Leites, L. P., Bradley St Clair, J., Jaquish, B. C., Sáenz-Romero, C., López-Upton, J., et al. (2014). Comparative genetic responses to climate in the varieties of Pinus ponderosa and Pseudotsuga menziesii: Clines in growth potential. For. Ecol. Manage. 324, 138–146. doi:10.1016/j.foreco.2014.02.041
Rellstab, C., Dauphin, B., and Exposito-Alonso, M. (2021). Prospects and limitations of genomic offset in conservation management. Evol. Appl. 14 (5), 1202–1212. doi:10.1111/eva.13205
Rellstab, C. (2021). Genomics helps to predict maladaptation to climate change. Nat. Clim. Change 11, 85–86. doi:10.1038/s41558-020-00964-w
Rellstab, C., Gugerli, F., Eckert, A. J., Hancock, A. M., and Holderegger, R. (2015). A practical guide to environmental association analysis in landscape genomics. Mol. Ecol. 24, 4348–4370. doi:10.1111/mec.13322
Rockman, M. V. (2012). The QTN program and the alleles that matter for evolution: All that's gold does not glitter. Evolution 66, 1–17. doi:10.1111/j.1558-5646.2011.01486.x
Samarina, L. S., Bobrovskikh, A. V., Doroshkov, A. V., Malyukova, L. S., Matskiv, A. O., Rakhmangulov, R. S., et al. (2020). Comparative expression analysis of stress-inducible candidate genes in response to cold and drought in tea plant [Camellia sinensis (L.) kuntze]. Front. Genet. 11, 611283. doi:10.3389/fgene.2020.611283
Sandring, S., and Ågren, J. (2009). Pollinator-mediated selection on floral display and flowering time in the perennial herb Arabidopsis lyrata. Evolution 63, 1292–1300. doi:10.1111/j.1558-5646.2009.00624.x
Sang, Y., Long, Z., Dan, X., Feng, J., Shi, T., Jia, C., et al. (2022). Genomic insights into local adaptation and future climate-induced vulnerability of a keystone forest tree in East Asia. Nat. Commun. 13, 6541. doi:10.1038/s41467-022-34206-8
Sarkar, D., Kundu, A., Das, D., Chakraborty, A., Mandal, N. A., Satya, P., et al. (2019). Resolving population structure and genetic differentiation associated with RAD-SNP loci under selection in tossa jute (Corchorus olitorius L.) Mol. Genet. Genomics 294, 479–492. doi:10.1007/s00438-018-1526-2
Savolainen, O., Lascoux, M., and Merilä, J. (2013). Ecological genomics of local adaptation. Nat. Rev. Genet. 14, 807–820. doi:10.1038/nrg3522
Seabra, S. G., Rodrigues, A. S. B., Silva, S. E., Neto, A. C., Pina-Martins, F., Marabuto, E., et al. (2021). Population structure, adaptation and divergence of the meadow spittlebug, Philaenus spumarius (Hemiptera, Aphrophoridae), revealed by genomic and morphological data. PeerJ 9, e11425. doi:10.7717/peerj.11425
Shaw, R. G., and Etterson, J. R. (2012). Rapid climate change and the rate of adaptation: Insight from experimental quantitative genetics. New Phytol. 195, 752–765. doi:10.1111/j.1469-8137.2012.04230.x
Shaw, R. G. (2018). From the past to the future: Considering the value and limits of evolutionary prediction. Am. Nat.t 193, 1–10. doi:10.1086/700565
Shen, Y., Xia, H., Tu, Z., Zong, Y., Yang, L., and Li, H. (2022). Genetic divergence and local adaptation of Liriodendron driven by heterogeneous environments. Mol. Ecol. 31, 916–933. doi:10.1111/mec.16271
Shryock, D. F., Havrilla, C. A., DeFalco, L. A., Esque, T. C., Custer, N. A., and Wood, T. E. (2017). Landscape genetic approaches to guide native plant restoration in the Mojave Desert. Ecol. Appl. 27, 429–445. doi:10.1002/eap.1447
Shryock, D. F., Washburn, L. K., DeFalco, L. A., and Esque, T. C. (2021). Harnessing landscape genomics to identify future climate resilient genotypes in a desert annual. Mol. Ecol. 30, 698–717. doi:10.1111/mec.15672
Smith, A. B., Godsoe, W., Rodríguez-Sánchez, F., Wang, H.-H., and Warren, D. (2019). Niche estimation above and below the species level. Trends Ecol. Evol. 34 (3), 260–273. doi:10.1016/j.tree.2018.10.012
Song, Y., Wu, P., Li, Y., Tong, X., Zheng, Y., Chen, Z., et al. (2017). Effect of endophytic fungi on the host plant growth, expression of expansin gene and flavonoid content in Tetrastigma hemsleyanum Diels & Gilg ex Diels. Plant Soil 417, 393–402. doi:10.1007/s11104-017-3266-1
Sork, V. L., Squire, K., Gugger, P. F., Steele, S. E., Levy, E. D., and Eckert, A. J. (2016). Landscape genomic analysis of candidate genes for climate adaptation in a California endemic oak, Quercus lobata. Am. J. Bot. 103, 33–46. doi:10.3732/ajb.1500162
Sun, X., Liu, D., Zhang, X., Li, W., Liu, H., Hong, W., et al. (2013). SLAF-Seq: An efficient method of large-scale de novo SNP discovery and genotyping using high-throughput sequencing. PLoS One 8, e58700. doi:10.1371/journal.pone.0058700
Swinfield, T., Lewis, O. T., Bagchi, R., and Freckleton, R. P. (2012). Consequences of changing rainfall for fungal pathogen-induced mortality in tropical tree seedlings. Ecol. Evol. 2, 1408–1413. doi:10.1002/ece3.252
TCNARCC (2011). China’s national assessment report on climate change(the second time). Beijing: Science Press.
Thuiller, W., Guéguen, M., Renaud, J., Karger, D. N., and Zimmermann, N. E. (2019). Uncertainty in ensembles of global biodiversity scenarios. Nat. Commun. 10, 1446. doi:10.1038/s41467-019-09519-w
Title, P. O., and Bemmels, J. B. (2018). Envirem: An expanded set of bioclimatic and topographic variables increases flexibility and improves performance of ecological niche modeling. Ecography 41, 291–307. doi:10.1111/ecog.02880
Vidaller, C., Baumel, A., Juin, M., Dutoit, T., and Bischoff, A. (2020). Comparison of neutral and adaptive differentiation in the Mediterranean grass Brachypodium retusum. Bot. J. Linn. Soc. 192, 536–549. doi:10.1093/botlinnean/boz089
Vitalis, R., Gautier, M., Dawson, K. J., and Beaumont, M. A. (2014). Detecting and measuring selection from gene frequency data. Genetics 196, 799–817. doi:10.1534/genetics.113.152991
Wang, J., Li, Z., Lei, M., Fu, Y., Zhao, J., Ao, M., et al. (2017). Integrated DNA methylome and transcriptome analysis reveals the ethylene-induced flowering pathway genes in pineapple. Sci. Rep. 7, 17167. doi:10.1038/s41598-017-17460-5
Wang, J., Qian, Q., Zhang, F., Jia, X., and He, J. (2022). The possible future changes in potential suitable habitats of Tetrastigma hemsleyanum (Vitaceae) in China predicted by an ensemble model. Glob. Ecol. Conserv. 35, e02083. doi:10.1016/j.gecco.2022.e02083
Wang, L., Liu, Y., Aslam, M., Jakada, B. H., Qin, Y., and Cai, H. (2021b). The glycine-rich domain protein GRDP2 regulates ovule development via the auxin pathway in Arabidopsis. Front. Plant Sci. 12, 698487. doi:10.3389/fpls.2021.698487
Wang, Y. H., Jiang, W. M., Comes, H. P., Hu, F. S., Qiu, Y. X., and Fu, C. X. (2015). Molecular phylogeography and ecological niche modelling of a widespread herbaceous climber, Tetrastigma hemsleyanum (vitaceae): Insights into plio–pleistocene range dynamics of evergreen forest in subtropical China. New Phytol. 206, 852–867. doi:10.1111/nph.13261
Wang, Y., Jiang, W., Ye, W., Fu, C., Gitzendanner, M. A., Soltis, P. S., et al. (2018). Evolutionary insights from comparative transcriptome and transcriptome-wide coalescence analyses in Tetrastigma hemsleyanum. BMC Plant Biol. 18, 208–215. doi:10.1186/s12870-018-1429-8
Wang, Y., Wang, S., Tian, Y., Wang, Q., Chen, S., Li, H., et al. (2021a). Functional characterization of a sugar beet BvbHLH93 transcription factor in salt stress tolerance. Int. J. Mol. Sci. 22, 3669. doi:10.3390/ijms22073669
Wu, Z., Sun, H., Zhou, Z., Li, D., and Peng, H. (2010). Floristics of seed plants from China. Beijing: Science Press.
Wu, Z., and Wu, S. (1998). “A proposal for a new floristic kingdom (realm): The E. Asiatic kingdom, its delineation and characteristics,” in Floristic characteristics and diversity of East Asian plants. Editors A. L. Zhang, and S. G. Wu (Beijing: China Higher Education Press/Springer), 3–42.
Yang, X., Yang, Z., Cheng, X., and Tan, M. (2019). Analysis on phenotypic diversity of Tetrastigma hemsleyanum from different provenances (In Chinese). J. Res. Environ. 28, 78–80. doi:10.1111/tan.13528
Yin, S., Cui, H., Zhang, L., Yan, J., Qian, L., and Ruan, S. (2021). Transcriptome and metabolome integrated analysis of two ecotypes of Tetrastigma hemsleyanum reveals candidate genes involved in chlorogenic acid accumulation. Plants 10, 1288. doi:10.3390/plants10071288
Yin, Y., Ma, D., Wu, S., and Pan, T. (2015). Projections of aridity and its regional variability over China in the mid-21st century. Int. J. Climatol. 35, 4387–4398. doi:10.1002/joc.4295
Zandalinas, S. I., Mittler, R., Balfagón, D., Arbona, V., and Gómez-Cadenas, A. (2018). Plant adaptations to the combination of drought and high temperatures. Physiol. Plant. 162, 2–12. doi:10.1111/ppl.12540
Zeng, X., Li, H., Li, K., Yuan, R., Zhao, S., Li, J., et al. (2021). Evolution of the Brassicaceae-specific MS5-Like family and neofunctionalization of the novel MALE STERILITY 5 gene essential for male fertility in Brassica napus. New Phytol. 229, 2339–2356. doi:10.1111/nph.17053
Zhao, D., and Wu, S. (2014). Vulnerability of natural ecosystem in China under regional climate scenarios: An analysis based on eco-geographical regions. J. Geog. Sci. 24, 237–248. doi:10.1007/s11442-014-1085-3
Keywords: candidate genes, climate change, genomic variation, genomic vulnerability, local adaptation, T. hemsleyanum
Citation: Wang Y, Zhang L, Zhou Y, Ma W, Li M, Guo P, Feng L and Fu C (2023) Using landscape genomics to assess local adaptation and genomic vulnerability of a perennial herb Tetrastigma hemsleyanum (Vitaceae) in subtropical China. Front. Genet. 14:1150704. doi: 10.3389/fgene.2023.1150704
Received: 24 January 2023; Accepted: 04 April 2023;
Published: 18 April 2023.
Edited by:
Alison G. Nazareno, Federal University of Minas Gerais, BrazilReviewed by:
Yong Li, Inner Mongolia Normal University, ChinaOlivier Francois, Grenoble Institute of Technology, France
Copyright © 2023 Wang, Zhang, Zhou, Ma, Li, Guo, Feng and Fu. This is an open-access article distributed under the terms of the Creative Commons Attribution License (CC BY). The use, distribution or reproduction in other forums is permitted, provided the original author(s) and the copyright owner(s) are credited and that the original publication in this journal is cited, in accordance with accepted academic practice. No use, distribution or reproduction is permitted which does not comply with these terms.
*Correspondence: Peng Guo, NjI1MzczMEAxNjMuY29t; Li Feng, bGlmZW5nMDA3QHhqdHUuZWR1LmNu