- 1Department of Ophthalmology and Visual Sciences, The Ohio State University, Columbus, OH, United States
- 2Department of Biomedical Informatics, The Ohio State University, Columbus, OH, United States
- 3Division of Human Genetics, The Ohio State University, Columbus, OH, United States
- 4Division of Research, Kaiser Permanente Northern California, Oakland, CA, United States
Intraocular pressure (IOP) is the only modifiable risk factor for glaucoma, the leading cause of irreversible blindness worldwide. In this review, we summarize the findings of genome-wide association studies (GWASs) of IOP published in the past 10 years and prior to December 2022. Over 190 genetic loci and candidate genes associated with IOP have been uncovered through GWASs, although most of these studies were conducted in subjects of European and Asian ancestries. We also discuss how these common variants have been used to derive polygenic risk scores for predicting IOP and glaucoma, and to infer causal relationship with other traits and conditions through Mendelian randomization. Additionally, we summarize the findings from a recent large-scale exome-wide association study (ExWAS) that identified rare variants associated with IOP in 40 novel genes, six of which are drug targets for clinical treatment or are being evaluated in clinical trials. Finally, we discuss the need for future genetic studies of IOP to include individuals from understudied populations, including Latinos and Africans, in order to fully characterize the genetic architecture of IOP.
Introduction
The first genome-wide association study (GWAS) of intraocular pressure (IOP) was published in 2012 (van Koolwijk et al., 2012). Since then, numerous common genetic variants associated with IOP have been discovered (Hysi et al., 2014; Springelkamp et al., 2014; Choquet et al., 2017; Springelkamp et al., 2017; Gao et al., 2018; Khawaja et al., 2018; MacGregor et al., 2018). Recently, a study using whole-exome sequencing data on large-scale biobanks has also led to significant new gene discoveries in the genetic architecture of IOP, demonstrating the important contribution of rare variants to this glaucoma endophenotype (Gao et al., 2022). On the tenth anniversary of the first IOP GWAS, it is time to reflect on the progress that has been made in this field and to consider the future direction of the genetics of IOP.
IOP is the amount of fluid pressure in the eye which is mainly determined by the balance between aqueous humor production and drainage (Civan and Macknight, 2004; Machiele et al., 2022). Elevated IOP is a major risk factor for primary open-angle glaucoma (POAG), the most common form of glaucoma that affects around 90% of glaucoma patients (Lang, 2007). Currently, IOP is the only modifiable risk factor for glaucoma, and lowering IOP helps to prevent the onset and delay the progression of POAG (Collaborative Normal-Tension Glaucoma Study Group, 1998; Heijl et al., 2002; Kass et al., 2002). IOP can be influenced by many factors, such as time of the day (Qassim et al., 2020a), measurement techniques, such as the Goldmann applanation tonometer and the ocular response analyzer (Martinez-de-la-Casa et al., 2006), age and ethnic background (Klein et al., 1992; Wu and Leske, 1997; Baek et al., 2015), and genetics. Studies have found that the heritability of IOP ranges from 0.35 to 0.67, depending on the study design (Klein et al., 2004; Chang et al., 2005; van Koolwijk et al., 2007; Carbonaro et al., 2009; Zheng et al., 2009; Sanfilippo et al., 2010; Asefa et al., 2019). Identifying genetic factors that contribute to IOP aid in uncovering the biological mechanisms regulating this trait (Ojha et al., 2013; Xu et al., 2021), which provides new management avenues for IOP and POAG.
GWASs have identified over 190 genetic loci associated with IOP (Choquet et al., 2017; Gao et al., 2018; Khawaja et al., 2018; MacGregor et al., 2018), demonstrating the contribution of common genetic variants to this trait. Additionally, these studies have shown that there is a strong bivariate genetic correlation between IOP and POAG ranging from 0.49 to 0.71 (Aschard et al., 2017; MacGregor et al., 2018) and a significant polygenic overlap between these two traits (Hysi et al., 2014; Khawaja et al., 2018). While numerous loci have been associated with IOP, these common variants typically have small effect sizes, contrary to those seen in rare variants, which can have large effect sizes (Gao et al., 2018; Gao et al., 2022). The role of rare genetic variants in IOP was recently reported in a large-scale exome-wide association study (ExWAS) that identified 40 novel genes associated with IOP (Gao et al., 2022).
Over the last 10 years, GWASs and ExWAS have provided strong evidence that IOP is a polygenic trait and a powerful endophenotype for POAG. These studies have also revealed valuable biological insights, pleiotropic effects, and potential drug targets associated with IOP genetic loci. The results of these studies have been applied to the development of polygenic risk scores (PRSs), which could potentially be used to stratify and screen for POAG risk in a population using IOP information (Khawaja et al., 2018; MacGregor et al., 2018; Gao et al., 2019; Qassim et al., 2020b). Moreover, the results of these studies have been applied to the development of genetic instruments that may be used in Mendelian randomization (MR) studies to better understand the nature of the relationships between eye traits and conditions (Han et al., 2020; Hysi et al., 2020; Choquet et al., 2022). In this review, we summarize these findings and discuss the potential future of GWASs in the study of IOP.
GWAS and ExWAS
GWASs have revolutionized the field of complex diseases and traits genetics over the past 17 years (Visscher et al., 2017; Tam et al., 2019). They involve examining the association between diseases or traits and hundreds of thousands (Klein et al., 2005) to millions of densely spaced single nucleotide polymorphisms (SNPs) (Gao and Edwards, 2011). These studies do not require any prior biological knowledge and are therefore an agnostic method for identifying the genetic effects of complex human diseases and traits. They are based on the assumption that densely genotyped common variants (minor allele frequency [MAF]
Each individual GWAS can have a limited sample size, which affects its statistical power. Additionally, genetic association signals that are identified need to be independently replicated in order to be considered reliable. To overcome these limitations, researchers often use genome-wide association meta-analysis (Willer et al., 2010; McGuire et al., 2021), which combines the results of multiple GWAS studies, and genotype imputation (Li and Abecasis, 2006; Marchini et al., 2007), which can infer ungenotyped variants from known data. Together, these methods can provide a more comprehensive and robust analysis of the genetic basis of a particular trait or disease.
More recently, other genome-wide studies, including whole-exome and whole-genome sequencing studies have been conducted in parallel of GWASs to assess rare variants associations and their roles in the genetic causes of diseases/traits. ExWAS employs whole-exome sequencing data that focuses on specific parts of the genome that encodes proteins, called exons, and allows for changes within such regions to be identified and analyzed. To address the relatively low statistical power issues in rare-variant analysis, researchers have designed many collapsing or gene-based methods (Li and Leal, 2008; Madsen and Browning, 2009; Wu et al., 2010; Wu et al., 2011; Lee et al., 2012a; Lee et al., 2012b; Zhou et al., 2018; Zhou et al., 2020).
Study inclusion criteria
To identify previously published IOP GWAS and ExWAS papers, we queried two websites: GWAS Catalog and PubMed, using the keywords “intraocular pressure” and “exome intraocular pressure,” respectively. We found 28 studies in the GWAS Catalog and three in PubMed. We then manually curated the search results to focus on studies with IOP as the main phenotype. Finally, we excluded studies that did not report novel significant IOP loci. As a result, Table 1 includes the 14 studies (GWAS and ExWAS) that were selected based on these two criteria: 1) IOP as the target phenotype of GWAS analyses; and 2) reported novel findings being genome-wide or exome-wide significant, either single variant (p < 5.0 × 10−8) or gene-based (p < 2.5 × 10−6), over the past 10 years. One study (Ozel et al., 2014) that reported borderline genome-wide significance (p = 8 × 10−8) was included in Table 1 as well. These criteria excluded two IOP studies (Chen et al., 2015; Chakraborty et al., 2021) that reported suggestively significant findings (P ∼ 5.0 × 10−5 or P ∼ 5.0 × 10−6).
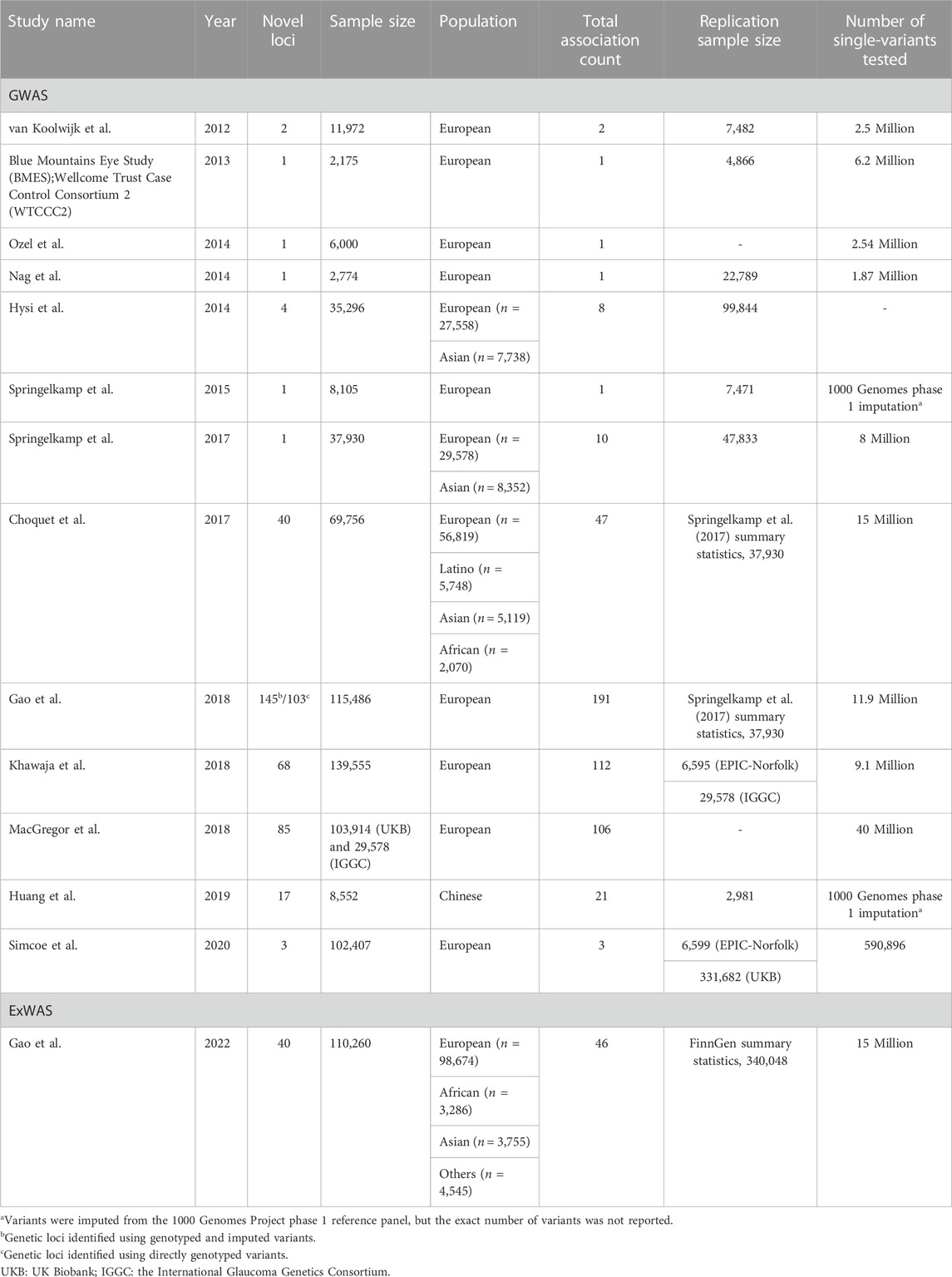
TABLE 1. Genome-wide and exome-wide association studies of intraocular pressure in the last 10 years.
GWAS of IOP
van Koolwijk et al. (2012) reported the first GWAS on IOP in 2012. They used 11,972 participants from four cohorts in The Netherlands, conducted linear regression analysis in each cohort, and then performed meta-analyses. They further carried out replication using cohorts from UK, Australia, Canada, and The Wellcome Trust Case Control Consortium 2 (WTCCC2)/Blue Mountains Eye Study (BMES). Variants rs11656696 at GAS7 and rs7555523 at TMCO1 were significantly associated at the genome-wide level with IOP and were also associated with POAG. After 2012, multiple groups continued to rely on meta-analysis of GWASs as their primary method for identifying genetic loci related to IOP.
Researchers from The Blue Mountains Eye Study and The Wellcome Trust Case Control Consortium 2 (2013) identified rs59072263, a common variant between GLCCI1 and ICA1 at 7q21, which had a combined p = 1.10
In 2018, with the advent of the biobank era, three groups reported genetic loci for IOP using the large UK Biobank prospective cohort dataset (Allen et al., 2014; Sudlow et al., 2015). Gao et al. (2018) described a GWAS of IOP using 115,486 European UKB participants and identified 103 and 145 novel loci using directly genotyped SNPs and an imputed genetic dataset, respectively. In addition to uncovering common variants, Gao et al. (2018) also reported low-frequency variant (MAF in the range of 0.005–0.01) associations with IOP, including rs28991009 in ANGPTL7. rs28991009 was subsequently studied in another report by Tanigawa et al. (2020). Khawaja et al. (2018) performed a meta-analysis of 139,555 European participants from UKB (n = 103,382), the International Glaucoma Genetics Consortium (IGGC) (n = 29,578) and EPIC-Norforlk (n = 6,595), and identified 68 novel genomic loci associated with IOP. MacGregor et al. (2018) identified 85 novel loci for IOP using a combined analysis of 133,492 participants from UKB (n = 103,914) and results from IGCC (n = 29,578). The large number of novel IOP loci identified by independent groups clearly demonstrated the power of the large-scale UKB dataset.
In the 2 years following 2018, two studies reported common-variant associations for IOP. Huang et al. (2019) reported 17 newly identified loci for IOP from a GWAS of 8,552 Chinese participants. In contrast to previous studies that explored autosomal SNPs, Simcoe et al. (2020) performed association analyses across the X chromosome using 102,407 participants from UKB and identified three loci, located within or near MXRA5 (rs2107482), GPM6B (rs66819623), and NDP/EFHC2 (rs12558081), associated with IOP.
Figure 1 shows the relationship between the number of novel IOP loci identified and the sample sizes used in 13 previously published GWASs and one ExWAS (described in more details in the next section) in the last 10 years. With larger sample sizes, typically more novel loci are identified. For instance, for a cohort size of about 12,000–35,000 European individuals, only one to four novel IOP loci were identified from GWASs. When the sample size increased to around 110,000 European individuals, more than 100 novel loci were identified, which represent a significant increase in the number of identified loci compared to studies with less than 40,000 individuals. However, for not well studied populations, such as East Asian, even less than 9,000 individuals generated 17 novel loci. This may indicate some genetic differences of IOP among different ethnic groups. For the three reports in 2018, i.e., Gao et al. (2018), Khawaja et al. (2018), MacGregor et al. (2018), different numbers of novel IOP loci were identified among different research groups, though there was a large overlap of the UKB sample used, possibly due to different analytic approaches, including MAF cutoff, phenotype definition, and number of principal components of genetic ancestry adjusted for.
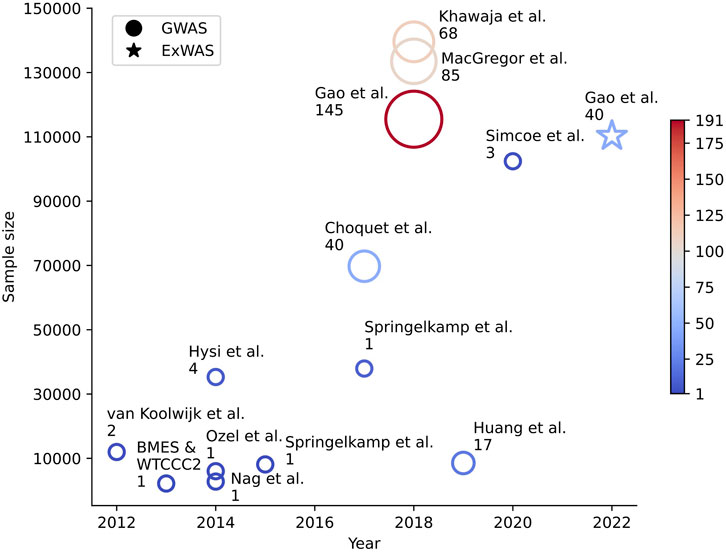
FIGURE 1. Number of novel intraocular pressure loci and study sample size in the last 10 years. Genetic association studies for IOP published in the past 10 years are represented by either (a) a circle for GWAS or (b) a star for ExWAS. The x-axis shows the year the study was published, and the y-axis shows the sample size of the study. The color and size of each plotted icon are proportional to the total number of loci and the number of novel loci discoveries that were reported in the corresponding study, respectively.
ExWAS of IOP
ExWAS is similar to, but different from, a common-variant GWAS. It focuses on coding regions and a different set of genetic variants, specifically rare ones typically with MAF less than 0.01, which represent a new avenue for IOP genetics research. In a very recent study, Gao et al. (2022) reported the largest rare-variant study of IOP to date using whole-exome sequences of 110,260 UKB participants. In addition to confirming known IOP genes, Gao’s group identified 40 novel genes harboring rare variants associated with IOP, including BOD1L1, ACAD10, HLA-B, ADRB1, PTPRB, RPL26, RPL10A, EGLN2, and MTOR. This study demonstrated the power of including and aggregating rare variants in gene discovery. About half of the identified IOP genes were also found to be associated with glaucoma phenotypes in UKB and the FinnGen cohort, a large biobank study focused on the population of Finland (Kurki et al., 2022). Most of the novel rare variants associated with IOP in Gao et al. (2022)’s study showed large effect sizes, which is consistent with the pattern that rare variants can show much larger effect sizes than common variants observed in many other studies (Gorlov et al., 2011; Zuk et al., 2014; Forgetta et al., 2020; Van Hout et al., 2020).
Biological insights
The above GWAS and ExWAS studies of IOP provided invaluable biological insights into both IOP and POAG. For example, van Koolwijk et al. (2012) found that GAS7 and TMCO1 are highly expressed in glaucoma-related ocular tissues, such as ciliary body, trabecular meshwork, lamina cribrosa, optic nerve, and retina. Hysi et al. (2014) found that FNDC3B and ABCA1 also showed association with POAG and both genes were expressed in most ocular tissues. In Choquet et al. (2017), Choquet et al. (2018), functional studies support IOP-related influences of FMNL2 and LMX1B, with certain LMX1B mutations causing high IOP and glaucoma resembling POAG in mice. In Gao et al. (2018), the top five Reactome pathways associated with IOP included the olfactory signaling pathway, defective B3GALTL causing Peters-plus syndrome, O-glycosylation of TSR domain-containing proteins, ABC transports in lipid homeostasis, and extracellular matrix organization. The loci reported by Khawaja et al. (2018) suggest that angiopoietin-receptor tyrosine kinase signaling, lipid metabolism, mitochondrial function, and developmental processes play a significant role in the risk of elevated IOP. Additionally, 14 of these associations were significantly associated with POAG after correction for multiple comparisons. MacGregor et al. (2018) studied the expression of genes at the newly identified IOP loci that were also associated with glaucoma in various human ocular tissues, including the corneal epithelium, corneal stroma, corneal endothelium, trabecular meshwork, ciliary body pigmented epithelium, neurosensory retina, optic nerve head, and optic nerve. They found that the expression of their newly associated genes was more enriched in the trabecular meshwork than other ocular tissues. MacGregor et al. further used FANTOM5 Cap Analysis of Gene Expression data and found evidence of correlation between enhancers with associated SNPs and the promoters of nine genes, including PTPN1, BCLAF1, and GAS7, in stromal and eye tissues. Nair et al. (2021) showed that mice deficient in GLIS1 developed chronically elevated IOP and GLIS1 impacts the expression of several other IOP and glaucoma-related genes, including MYOC and CYP1B1.
Numerous IOP genes also showed apparent pleiotropic nature (Choquet et al., 2017; Gao et al., 2018). Pleiotropy is the phenomenon in which a single gene or genetic variant has multiple effects on different traits (Stearns, 2010; Solovieff et al., 2013). Pleiotropy is important because it helps explain how a single genetic change can have multiple effects on an organism. It also helps to explain why certain traits and conditions may be inherited together, even if they seem unrelated. In addition to glaucoma risk, Choquet et al. (2017) reported that several their own IOP loci are also associated with cup area, central corneal thickness, and Axenfeld-Rieger syndrome. Gao et al. (2018) studied the pleiotropic effects of 671 variants (from directly genotyped variants found in 149 unique loci) using the GWAS catalog. Many neurological disorders associated with eye diseases were directly linked to the included SNPs, such as primary open-angle, primary angle closure, and high-pressure glaucoma, as well as age-related macular degeneration. In addition, ocular parameters such as central corneal thickness, axial length, optic cup area, and iris characteristics were mapped. The SNPs were also matched to digestive and immune disorders, cancer, and cardiovascular and hematological measurements, including blood pressure, body mass index, and type 2 diabetes. Pleiotropy undoubtedly plays an important role in furthering our understanding of human biology and disease (Gao and Huang, 2019), including IOP and glaucoma. Studying the pleiotropic nature of human traits can provide new insights into disease prevention and treatment (Gao, 2020), e.g., drugs that have been approved for the treatment of one disease could be repurposed for the management of IOP based on information about pleiotropy. This may lead to the discovery of novel uses for existing drugs.
Drug targets
One of the goals of GWAS/ExWAS is to facilitate drug target discoveries, which has been the endeavor of many pharmaceutical companies. Drug candidates that have genetics support are twice as likely to be successful as those without genetics support (Nelson et al., 2015). Focused analyses of CAV1/CAV2 revealed their association with IOP and replicated the previously reported associations with POAG in both effect size and direction (Ozel et al., 2014). Knockout mice exhibit elevated IOP and decreased outflow facility, demonstrating the direct role for CAV1 in IOP homeostasis (Elliott et al., 2014). The extracellular matrix (ECM) was also shown to be associated with IOP (Choquet et al., 2017; Gao et al., 2018; MacGregor et al., 2018). ECM plays an important role in regulating the outflow of aqueous humor and may be a promising target for new therapies, such as those targeting the rho kinase, nitric oxide, adenosine A1, prostaglandin EP4, and potassium channel pathways involved in the conventional outflow of aqueous humor (Prasanna et al., 2016). Manipulating the ECM in the aqueous outflow pathway impacts IOP in genetic knockouts (Vranka et al., 2015). Furthermore, ANGPTL7 was shown to modulate the trabecular meshwork’s ECM and the response of this tissue to steroids (Comes et al., 2011) and may serve as a good candidate for glaucoma therapy (Borrás, 2017). Six genes, namely, ADRB1, PTPRB, RPL26, RPL10A, EGLN2, and MTOR, out of Gao et al. (2022)’s gene-based investigation have existing therapeutic molecular targets. The most notable one, ADRB1, is the target of cardiovascular and glaucoma drugs, including the broad class of glaucoma drugs targeting the beta-adrenergic receptor antagonists, or beta-blockers, known to lower IOP. The other five genes are targets in many clinical trials involving razuprotafib (targeting PTPRB), ataluren, ELX-02, MT-3724 (targeting RPL26 and RPL10A), roxadustat, daprodustat, vadadustat (targeting EGLN2), and perhexiline (targeting MTOR), providing candidates for drug repurposing for possible glaucoma treatment.
Applications of GWAS/ExWAS results
In addition to the biological insights that we can gain from all these GWAS and ExWAS studies, there are two other major categories of applications, i.e., PRS and MR, utilizing the summary statistics from GWAS/ExWAS studies to make powerful predictions, e.g., to stratify individuals into high and low risk groups, and to infer possible causal effects.
Polygenic risk scores
Similarly to the three IOP GWASs reported in 2018, studies by Khawaja et al. (2018), MacGregor et al. (2018), and Gao and Fan (2018) also explored the use of IOP PRS or SNPs to predict glaucoma. Both Khawaja et al. (2018), and MacGregor et al. (2018) used p < 5
Mendelian randomization
GWAS results and PRSs are also used in other types of studies, such as MR studies, which observe the causal effects of an exposure to a specific external factor or a disease outcome based on variation in the population genome. They play an important role in our understanding of how external factors influence the development of a disease in an individual based on their specific genetic makeup. Kim et al. (2021) carried out MR analyses using the UKB dataset and assessed whether genetic loci linked to coffee consumption were associated with IOP. By using a PRS combining 111 IOP genetic variants, they were able to observe the interactions between the cohort’s genomes and diets for over 121,000 individuals. They observed that coffee, tea, and caffeine consumption were weakly associated with lower IOP, and that these exposures had no association with glaucoma. However, the association between caffeine intake and IOP was modified by an IOP PRS, such that higher caffeine intake was positively associated with both IOP and glaucoma prevalence, but only among individuals with the highest genetic susceptibility to elevated IOP. Hysi et al. (2020) used variants of IOP as instruments and explored the relationship between refractive error and IOP. They found that IOP predicts a decrease in the spherical equivalent of diopters (more myopic). Choquet et al. (2022) conducted a two-sample MR study to evaluate the nature of the relationship between myopic refractive error and POAG and performed a multivariable MR analysis to adjust for the potential effect of IOP. Han et al. (2020) used a two-sample MR and found evidence of a potential causal inference for the associations of myopia and IOP with retinal detachment.
Genetic association studies of IOP in underrepresented populations
Genetic association studies of IOP in underrepresented populations are rather scarce. Here, underrepresented populations are defined as subgroups that have low representation relative to their numbers in the general population based on the definition described by the National Center for Advancing Translational Sciences of NIH (https://toolkit.ncats.nih.gov/glossary/underrepresented-population/). As such, the following groups are considered underrepresented: African Americans, Hispanics or Latinos, American Indians Alaskan Natives, and Native Hawaiians and other Pacific Islanders. Instead of carrying out ancestry-specific studies, most previous association studies embedded underrepresented populations in studies with a large number of European individuals (Choquet et al., 2017; Gao et al., 2022), possibly due to the smaller sample size of each individual underrepresented cohort. Choquet et al. (2017) used a meta-analysis approach to include underrepresented population samples to increase the overall sample size in their common variant association with IOP analysis. Gao et al. (2022) used a pan-ancestry approach by pooling all samples together and applying mixed-effect models that accounted for both principal components of genetic ancestry and genetic subpopulations in their rare variant analysis. After IOP genetic loci were identified in the overall combined multiethnic meta-analysis or sample, the loci were then explored in individual ethnicity groups (Choquet et al., 2017; Gao et al., 2022). To the best of our knowledge, only one study examined the association between IOP and genetic ancestry in a standalone Latino cohort (Nannini et al., 2016). Using linear regression analyses, Nannini et al. (2016) found that African ancestry was significantly associated with higher IOP in Latinos. After accounting for age, sex, body mass index, systolic blood pressure, central corneal thickness, and type 2 diabetes, this association remained significant. They also found that the association between African ancestry and IOP was modified by a significant interaction with hypertension, such that hypertensive individuals experienced a greater increase in IOP with increasing African ancestry. This study demonstrated for the first time that African ancestry and its interaction with hypertension are associated with higher IOP in Latinos.
Discussion
Since the first success of GWAS in human genetics (Klein et al., 2005), GWASs have become a widely used tool in genetic epidemiology (Gao and Edwards, 2011). These studies have led to the identification of many genetic variants that are associated with a variety of human diseases and traits, including IOP and glaucoma. The use of GWASs has greatly enhanced our ability to search for genetic contributions to complex traits. Due to the need for high statistical power, researchers often employ meta-analysis to combine the results of multiple GWAS studies. The availability of biobank datasets, especially UKB with half a million participants, also propelled genetic studies to another level of discoveries in both common and rare variants. Through ExWASs, large effect-size rare variants for IOP have begun to be unveiled. Such sequencing approaches will become more prevalent in the research world in the years to come. Not only have we seen a much deeper level of biological insights, including pleiotropy, but also have researchers used IOP PRS to help predict glaucoma and genetic instruments for IOP in MR studies for inferring causal relationships with other eye conditions.
Despite all the discoveries that have been made, the majority of the GWASs of IOP were done in individuals of European (95.6%) and Asian (3.1%) descents. Some efforts have been made to include individuals of non-European descent, such as the studies conducted by Choquet et al. (2017); Gao et al. (2022). However, the underrepresented population specific information is still largely unknown. Genetic discoveries in standalone underrepresented population are still in scarcity, with only one Latino-specific genetic association study published so far. Presently, PRSs work mostly in European individuals since most GWASs were done in European samples and do not transfer well to other underrepresented populations. PRS widespread use can create health disparities if this continues (Martin et al., 2019). It would be interesting to compare the discovery of genetic variants associated with a particular trait or disease in a sample of European individuals to that of a sample of individuals of African descent or Latino individuals, with the same sample size, for example, of 100,000 individuals. This comparison could provide insight into potential differences and similarities in the genetic basis of the trait or disease between these populations, which can have implications for the development and application of genetic testing and personalized medicine. Equity, diversity, and inclusion are critically important in all aspects of our life, including genetic research. Efforts to diversity are being made to address the significant imbalance in this field (Denny and Collins, 2021). The All of Us research program, a part of the National Institutes of Health, is working to build an inclusive, diverse database by inviting individuals from all backgrounds to participate (The All of Us Research Program Investigators, 2019). They have over 550,000 enrolled participants, with over 387,000 having completed the first steps to integrate the research program. Over 50% of their participants are from an ethnic minority, and over 80% correspond to a group underrepresented in research.
At the start of a new decade of IOP GWASs, what can we expect to see next? It is possible that GWASs by genotyping arrays will be replaced by GWASs by sequencing as the cost of sequencing continue to decrease. The use of whole-genome sequencing in GWASs is almost certain to yield unexpected discoveries, similar to what agnostic GWASs have already shown. Additionally, advancements in artificial intelligence may transform how we analyze and understand human genetics (Gao et al., 2020). These genetic discoveries are likely to be applied to individual patients of all backgrounds to aid in prevention, diagnosis and treatment.
Author contributions
XG conceived, planned and oversaw the present study. XG and MC wrote the draft of the manuscript and edited it to its final version. HC provided critical feedback on the manuscript and helped in editing it to its final version. AA participated in the overall draft and organization of the manuscript.
Conflict of interest
The authors declare that the research was conducted in the absence of any commercial or financial relationships that could be construed as a potential conflict of interest.
Publisher’s note
All claims expressed in this article are solely those of the authors and do not necessarily represent those of their affiliated organizations, or those of the publisher, the editors and the reviewers. Any product that may be evaluated in this article, or claim that may be made by its manufacturer, is not guaranteed or endorsed by the publisher.
References
Allen, N. E., Sudlow, C., Peakman, T., and Collins, R. (2014). UK biobank data: Come and get it. Sci. Transl. Med. 6, 224ed4. doi:10.1126/scitranslmed.3008601
Aschard, H., Kang, J. H., Iglesias, A. I., Hysi, P., Cooke Bailey, J. N., Khawaja, A. P., et al. (2017). Genetic correlations between intraocular pressure, blood pressure and primary open-angle glaucoma: A multi-cohort analysis. Eur. J. Hum. Genet. 25, 1261–1267. doi:10.1038/ejhg.2017.136
Asefa, N. G., Neustaeter, A., Jansonius, N. M., and Snieder, H. (2019). Heritability of glaucoma and glaucoma-related endophenotypes: Systematic review and meta-analysis. Surv. Ophthalmol. 64, 835–851. doi:10.1016/j.survophthal.2019.06.002
Baek, S. U., Kee, C., and Suh, W. (2015). Longitudinal analysis of age-related changes in intraocular pressure in South Korea. Eye (Lond) 29, 625–629. doi:10.1038/eye.2015.11
Borrás, T. (2017). The pathway from genes to gene therapy in glaucoma: A review of possibilities for using genes as glaucoma drugs. Asia Pac J. Ophthalmol. (Phila) 6, 80–93. doi:10.22608/APO.2016126
Carbonaro, F., Andrew, T., Mackey, D. A., Young, T. L., Spector, T. D., and Hammond, C. J. (2009). Repeated measures of intraocular pressure result in higher heritability and greater power in genetic linkage studies. Invest. Ophthalmol. Vis. Sci. 50, 5115–5119. doi:10.1167/iovs.09-3577
Chakraborty, S., Sharma, A., Bagchi, I., Pal, S., Bhattacharyya, C., Gupta, V., et al. (2021). A genomewide association study on individuals with occludable angles identifies potential risk loci for intraocular pressure. J. Genet. 100, 69. doi:10.1007/s12041-021-01321-2
Chang, T. C., Congdon, N. G., Wojciechowski, R., Munoz, B., Gilbert, D., Chen, P., et al. (2005). Determinants and heritability of intraocular pressure and cup-to-disc ratio in a defined older population. Ophthalmology 112, 1186–1191. doi:10.1016/j.ophtha.2005.03.006
Chen, F., Klein, A. P., Klein, B. E. K., Lee, K. E., Truitt, B., Klein, R., et al. (2015). Exome array analysis identifies CAV1/CAV2 as a susceptibility locus for intraocular pressure. Investigative Ophthalmol. Vis. Sci. 56, 544–551. doi:10.1167/iovs.14-15204
Choquet, H., Khawaja, A. P., Jiang, C., Yin, J., Melles, R. B., Glymour, M. M., et al. (2022). Association between myopic refractive error and primary open-angle glaucoma: A 2-sample mendelian randomization study. JAMA Ophthalmol. 140, 864–871. doi:10.1001/jamaophthalmol.2022.2762
Choquet, H., Paylakhi, S., Kneeland, S. C., Thai, K. K., Hoffmann, T. J., Yin, J., et al. (2018). A multiethnic genome-wide association study of primary open-angle glaucoma identifies novel risk loci. Nat. Commun. 9, 2278. doi:10.1038/s41467-018-04555-4
Choquet, H., Thai, K. K., Yin, J., Hoffmann, T. J., Kvale, M. N., Banda, Y., et al. (2017). A large multi-ethnic genome-wide association study identifies novel genetic loci for intraocular pressure. Nat. Commun. 8, 2108. doi:10.1038/s41467-017-01913-6
Civan, M. M., and Macknight, A. D. (2004). The ins and outs of aqueous humour secretion. Exp. Eye Res. 78, 625–631. doi:10.1016/j.exer.2003.09.021
Collaborative Normal-Tension Glaucoma Study Group (1998). Comparison of glaucomatous progression between untreated patients with normal-tension glaucoma and patients with therapeutically reduced intraocular pressures. Collaborative Normal-Tension Glaucoma Study Group. Am. J. Ophthalmol. 126, 487–497.
Comes, N., Buie, L. K., and Borrás, T. (2011). Evidence for a role of angiopoietin-like 7 (ANGPTL7) in extracellular matrix formation of the human trabecular meshwork: Implications for glaucoma. Genes. cells. 16, 243–259. doi:10.1111/j.1365-2443.2010.01483.x
Denny, J. C., and Collins, F. S. (2021). Precision medicine in 2030-seven ways to transform healthcare. Cell. 184, 1415–1419. doi:10.1016/j.cell.2021.01.015
The All Of Us Research Program Investigators Denny, J. C., Rutter, J. L., Goldstein, D. B., Philippakis, A., Smoller, J. W., Jenkins, G., et al. (2019). The “all of us” research program. N. Engl. J. Med. 381, 668–676. doi:10.1056/NEJMsr1809937
Elliott, M. H., Gu, X., Ashpole, N. E., Griffith, G. L., Boyce, T. M., Tanito, M., et al. (2014). Role of caveolin-1 in intraocular pressure and conventional outflow regulation. Investigative Ophthalmol. Vis. Sci. 55, 2888.
Forgetta, V., Manousaki, D., Istomine, R., Ross, S., Tessier, M. C., Marchand, L., et al. (2020). Rare genetic variants of large effect influence risk of type 1 diabetes. Diabetes 69, 784–795. doi:10.2337/db19-0831
Gao, X., and Edwards, T. L. (2011). Genome-wide association studies: Where we are heading? World J. Med. Genet. 1, 23–35. doi:10.5496/wjmg.v1.i1.23
Gao, X. R., Cebulla, C. M., and Ohr, M. P. (2020). “Chapter 19 - Advancing to precision medicine through big data and artificial intelligence,” in Genetics and genomics of eye disease. Editor X. R. GAO (Cambridge: Academic Press).
Gao, X. R. (2020). “Chapter 18 - pleiotropy in eye disease and related traits,” in Genetics and genomics of eye disease. Editor X. R. GAO (Cambridge: Academic Press).
Gao, X. R., Chiariglione, M., and Arch, A. J. (2022). Whole-exome sequencing study identifies rare variants and genes associated with intraocular pressure and glaucoma. Nat. Commun. 13, 7376. doi:10.1038/s41467-022-35188-3
Gao, X. R., and Fan, F. (2018). Polygenic risk score is associated with intraocular pressure and improves glaucoma prediction in the UK Biobank cohort. Investigative Ophthalmol. Vis. Sci. 59, 779.
Gao, X. R., Huang, H., and Kim, H. (2019). Polygenic risk score is associated with intraocular pressure and improves glaucoma prediction in the UK biobank cohort. Transl. Vis. Sci. Technol. 8, 10. doi:10.1167/tvst.8.2.10
Gao, X. R., Huang, H., Nannini, D. R., Fan, F., and Kim, H. (2018). Genome-wide association analyses identify new loci influencing intraocular pressure. Hum. Mol. Genet. 27, 2205–2213. doi:10.1093/hmg/ddy111
Gao, X. R., and Huang, H. (2019). PleioNet: A web-based visualization tool for exploring pleiotropy across complex traits. Bioinformatics 35, 4179–4180. doi:10.1093/bioinformatics/btz179
Gorlov, I. P., Gorlova, O. Y., Frazier, M. L., Spitz, M. R., and Amos, C. I. (2011). Evolutionary evidence of the effect of rare variants on disease etiology. Clin. Genet. 79, 199–206. doi:10.1111/j.1399-0004.2010.01535.x
Han, X., Ong, J. S., An, J., Craig, J. E., Gharahkhani, P., Hewitt, A. W., et al. (2020). Association of myopia and intraocular pressure with retinal detachment in European descent participants of the UK biobank cohort: A mendelian randomization study. JAMA Ophthalmol. 138, 671–678. doi:10.1001/jamaophthalmol.2020.1231
Heijl, A., Leske, M. C., Bengtsson, B., Hyman, L., Bengtsson, B., Hussein, M., et al. (2002). Reduction of intraocular pressure and glaucoma progression: Results from the early manifest glaucoma trial. Arch. Ophthalmol. 120, 1268–1279. doi:10.1001/archopht.120.10.1268
Huang, L., Chen, Y., Lin, Y., Tam, P. O. S., Cheng, Y., Shi, Y., et al. (2019). Genome-wide analysis identified 17 new loci influencing intraocular pressure in Chinese population. Sci. China Life Sci. 62, 153–164. doi:10.1007/s11427-018-9430-2
Hysi, P. G., Cheng, C. Y., Springelkamp, H., Macgregor, S., Bailey, J. N., Wojciechowski, R., et al. (2014). Genome-wide analysis of multi-ancestry cohorts identifies new loci influencing intraocular pressure and susceptibility to glaucoma. Nat. Genet. 46, 1126–1130. doi:10.1038/ng.3087
Hysi, P. G., Choquet, H., Khawaja, A. P., Wojciechowski, R., Tedja, M. S., Yin, J., et al. (2020). Meta-analysis of 542,934 subjects of European ancestry identifies new genes and mechanisms predisposing to refractive error and myopia. Nat. Genet. 52, 401–407. doi:10.1038/s41588-020-0599-0
Kass, M. A., Heuer, D. K., Higginbotham, E. J., Johnson, C. A., Keltner, J. L., Miller, J. P., et al. (2002). The ocular hypertension treatment study: A randomized trial determines that topical ocular hypotensive medication delays or prevents the onset of primary open-angle glaucoma. Arch. Ophthalmol. 120, 701–713. ; discussion 829-30. doi:10.1001/archopht.120.6.701
Khawaja, A. P., Cooke Bailey, J. N., Wareham, N. J., Scott, R. A., Simcoe, M., Igo, R. P., et al. (2018). Genome-wide analyses identify 68 new loci associated with intraocular pressure and improve risk prediction for primary open-angle glaucoma. Nat. Genet. 50, 778–782. doi:10.1038/s41588-018-0126-8
Kim, J., Aschard, H., Kang, J. H., Lentjes, M. A. H., Do, R., Wiggs, J. L., et al. (2021). Intraocular pressure, glaucoma, and dietary caffeine consumption: A gene-diet interaction study from the UK biobank. Ophthalmology 128, 866–876. doi:10.1016/j.ophtha.2020.12.009
Klein, B. E., Klein, R., and Lee, K. E. (2004). Heritability of risk factors for primary open-angle glaucoma: The beaver dam eye study. Invest. Ophthalmol. Vis. Sci. 45, 59–62. doi:10.1167/iovs.03-0516
Klein, B. E., Klein, R., and Linton, K. L. (1992). Intraocular pressure in an American community. The beaver dam eye study. Invest. Ophthalmol. Vis. Sci. 33, 2224–2228.
Klein, R. J., Zeiss, C., Chew, E. Y., Tsai, J. Y., Sackler, R. S., Haynes, C., et al. (2005). Complement factor H polymorphism in age-related macular degeneration. Science 308, 385–389. doi:10.1126/science.1109557
Kurki, M. I., Karjalainen, J., Palta, P., Sipilä, T. P., Kristiansson, K., Donner, K., et al. (2022). FinnGen: Unique genetic insights from combining isolated population and national health register data. medRxiv, 2022.03.03.22271360.
Lee, S., Emond, M. J., Bamshad, M. J., Barnes, K. C., Rieder, M. J., Nickerson, D. A., et al. (2012a). Optimal unified approach for rare-variant association testing with application to small-sample case-control whole-exome sequencing studies. Am. J. Hum. Genet. 91, 224–237. doi:10.1016/j.ajhg.2012.06.007
Lee, S., Wu, M. C., and Lin, X. (2012b). Optimal tests for rare variant effects in sequencing association studies. Biostatistics 13, 762–775. doi:10.1093/biostatistics/kxs014
Li, B., and Leal, S. M. (2008). Methods for detecting associations with rare variants for common diseases: Application to analysis of sequence data. Am. J. Hum. Genet. 83, 311–321. doi:10.1016/j.ajhg.2008.06.024
Li, Y., and Abecasis, G. R. (2006). Mach 1.0: Rapid haplotype reconstruction and missing genotype inference. Am. J. Hum. Genet. S79.
Macarthur, J., Bowler, E., Cerezo, M., Gil, L., Hall, P., Hastings, E., et al. (2017). The new NHGRI-EBI Catalog of published genome-wide association studies (GWAS Catalog). Nucleic Acids Res. 45, D896–D901. doi:10.1093/nar/gkw1133
Macgregor, S., Ong, J. S., An, J., Han, X., Zhou, T., Siggs, O. M., et al. (2018). Genome-wide association study of intraocular pressure uncovers new pathways to glaucoma. Nat. Genet. 50, 1067–1071. doi:10.1038/s41588-018-0176-y
Machiele, R., Motlagh, M., and Patel, B. C. (2022). Intraocular pressure. Treasure Island (FL): StatPearls.
Madsen, B. E., and Browning, S. R. (2009). A groupwise association test for rare mutations using a weighted sum statistic. PLoS Genet. 5, e1000384. doi:10.1371/journal.pgen.1000384
Marchini, J., Howie, B., Myers, S., Mcvean, G., and Donnelly, P. (2007). A new multipoint method for genome-wide association studies by imputation of genotypes. Nat. Genet. 39, 906–913. doi:10.1038/ng2088
Martin, A. R., Kanai, M., Kamatani, Y., Okada, Y., Neale, B. M., and Daly, M. J. (2019). Clinical use of current polygenic risk scores may exacerbate health disparities. Nat. Genet. 51, 584–591. doi:10.1038/s41588-019-0379-x
Martinez-De-La-Casa, J. M., Garcia-Feijoo, J., Fernandez-Vidal, A., Mendez-Hernandez, C., and Garcia-Sanchez, J. (2006). Ocular response analyzer versus Goldmann applanation tonometry for intraocular pressure measurements. Invest. Ophthalmol. Vis. Sci. 47, 4410–4414. doi:10.1167/iovs.06-0158
Mcguire, D., Jiang, Y., Liu, M., Weissenkampen, J. D., Eckert, S., Yang, L., et al. (2021). Model-based assessment of replicability for genome-wide association meta-analysis. Nat. Commun. 12, 1964. doi:10.1038/s41467-021-21226-z
Nag, A., Venturini, C., Small, K. S., Young, T. L., Viswanathan, A. C., Mackey, D. A., et al. (2014). A genome-wide association study of intra-ocular pressure suggests a novel association in the gene FAM125B in the TwinsUK cohort. Hum. Mol. Genet. 23, 3343–3348. doi:10.1093/hmg/ddu050
Nair, K. S., Srivastava, C., Brown, R. V., Koli, S., Choquet, H., Kang, H. S., et al. (2021). GLIS1 regulates trabecular meshwork function and intraocular pressure and is associated with glaucoma in humans. Nat. Commun. 12, 4877. doi:10.1038/s41467-021-25181-7
Nannini, D., Torres, M., Chen, Y. D., Taylor, K. D., Rotter, J. I., Varma, R., et al. (2016). African ancestry is associated with higher intraocular pressure in Latinos. Ophthalmology 123, 102–108. doi:10.1016/j.ophtha.2015.08.042
Nelson, M. R., Tipney, H., Painter, J. L., Shen, J., Nicoletti, P., Shen, Y., et al. (2015). The support of human genetic evidence for approved drug indications. Nat. Genet. 47, 856–860. doi:10.1038/ng.3314
Ojha, P., Wiggs, J. L., and Pasquale, L. R. (2013). The genetics of intraocular pressure. Semin. Ophthalmol. 28, 301–305. doi:10.3109/08820538.2013.825291
Ozel, A. B., Moroi, S. E., Reed, D. M., Nika, M., Schmidt, C. M., Akbari, S., et al. (2014). Genome-wide association study and meta-analysis of intraocular pressure. Hum. Genet. 133, 41–57. doi:10.1007/s00439-013-1349-5
Pe'er, I., Yelensky, R., Altshuler, D., and Daly, M. J. (2008). Estimation of the multiple testing burden for genomewide association studies of nearly all common variants. Genet. Epidemiol. 32, 381–385. doi:10.1002/gepi.20303
Prasanna, G., Li, B., Mogi, M., and Rice, D. S. (2016). Pharmacology of novel intraocular pressure-lowering targets that enhance conventional outflow facility: Pitfalls, promises and what lies ahead? Eur. J. Pharmacol. 787, 47–56. doi:10.1016/j.ejphar.2016.03.003
Qassim, A., Mullany, S., Awadalla, M. S., Hassall, M. M., Nguyen, T., Marshall, H., et al. (2020a). A polygenic risk score predicts intraocular pressure readings outside office hours and early morning spikes as measured by home tonometry. Ophthalmol. Glaucoma 4, 411–420. doi:10.1016/j.ogla.2020.12.002
Qassim, A., Souzeau, E., Siggs, O. M., Hassall, M. M., Han, X., Griffiths, H. L., et al. (2020b). An intraocular pressure polygenic risk score stratifies multiple primary open-angle glaucoma parameters including treatment intensity. Ophthalmology 127, 901–907. doi:10.1016/j.ophtha.2019.12.025
Risch, N., and Merikangas, K. (1996). The future of genetic studies of complex human diseases. Science 273, 1516–1517. doi:10.1126/science.273.5281.1516
Sanfilippo, P. G., Hewitt, A. W., Hammond, C. J., and Mackey, D. A. (2010). The heritability of ocular traits. Surv. Ophthalmol. 55, 561–583. doi:10.1016/j.survophthal.2010.07.003
Simcoe, M. J., Khawaja, A. P., Mahroo, O. A., Hammond, C. J., Hysi, P. G., Eye, U. K. B., et al. (2020). The role of chromosome X in intraocular pressure variation and sex-specific effects. Invest. Ophthalmol. Vis. Sci. 61, 20. doi:10.1167/iovs.61.11.20
Solovieff, N., Cotsapas, C., Lee, P. H., Purcell, S. M., and Smoller, J. W. (2013). Pleiotropy in complex traits: Challenges and strategies. Nat. Rev. Genet. 14, 483–495. doi:10.1038/nrg3461
Springelkamp, H., Hohn, R., Mishra, A., Hysi, P. G., Khor, C. C., Loomis, S. J., et al. (2014). Meta-analysis of genome-wide association studies identifies novel loci that influence cupping and the glaucomatous process. Nat. Commun. 5, 4883. doi:10.1038/ncomms5883
Springelkamp, H., Iglesias, A. I., Cuellar-Partida, G., Amin, N., Burdon, K. P., Van Leeuwen, E. M., et al. (2015). ARHGEF12 influences the risk of glaucoma by increasing intraocular pressure. Hum. Mol. Genet. 24, 2689–2699. doi:10.1093/hmg/ddv027
Springelkamp, H., Iglesias, A. I., Mishra, A., Hohn, R., Wojciechowski, R., Khawaja, A. P., et al. (2017). New insights into the genetics of primary open-angle glaucoma based on meta-analyses of intraocular pressure and optic disc characteristics. Hum. Mol. Genet. 26, 438–453. doi:10.1093/hmg/ddw399
Stearns, F. W. (2010). One hundred years of pleiotropy: A retrospective. Genetics 186, 767–773. doi:10.1534/genetics.110.122549
Sudlow, C., Gallacher, J., Allen, N., Beral, V., Burton, P., Danesh, J., et al. (2015). UK biobank: An open access resource for identifying the causes of a wide range of complex diseases of middle and old age. Plos Med. 12, e1001779. doi:10.1371/journal.pmed.1001779
Tam, V., Patel, N., Turcotte, M., Bossé, Y., Paré, G., and Meyre, D. (2019). Benefits and limitations of genome-wide association studies. Nat. Rev. Genet. 20, 467–484. doi:10.1038/s41576-019-0127-1
Tanigawa, Y., Wainberg, M., Karjalainen, J., Kiiskinen, T., Venkataraman, G., Lemmela, S., et al. (2020). Rare protein-altering variants in ANGPTL7 lower intraocular pressure and protect against glaucoma. PLoS Genet. 16, e1008682. doi:10.1371/journal.pgen.1008682
The Blue Mountains Eye Study and The Wellcome Trust Case Control Consortium 2 (2013). Genome-wide association study of intraocular pressure identifies the GLCCI1/ICA1 region as a glaucoma susceptibility locus. Hum. Mol. Genet. 22, 4653–4660. doi:10.1093/hmg/ddt293
Van Hout, C. V., Tachmazidou, I., Backman, J. D., Hoffman, J. D., Liu, D., Pandey, A. K., et al. (2020). Exome sequencing and characterization of 49,960 individuals in the UK Biobank. Nature 586, 749–756. doi:10.1038/s41586-020-2853-0
Van Koolwijk, L. M., Despriet, D. D., Van Duijn, C. M., Pardo Cortes, L. M., Vingerling, J. R., Aulchenko, Y. S., et al. (2007). Genetic contributions to glaucoma: Heritability of intraocular pressure, retinal nerve fiber layer thickness, and optic disc morphology. Invest. Ophthalmol. Vis. Sci. 48, 3669–3676. doi:10.1167/iovs.06-1519
Van Koolwijk, L. M., Ramdas, W. D., Ikram, M. K., Jansonius, N. M., Pasutto, F., Hysi, P. G., et al. (2012). Common genetic determinants of intraocular pressure and primary open-angle glaucoma. PLoS Genet. 8, e1002611. doi:10.1371/journal.pgen.1002611
Visscher, P. M., Wray, N. R., Zhang, Q., Sklar, P., Mccarthy, M. I., Brown, M. A., et al. (2017). 10 Years of GWAS discovery: Biology, function, and translation. Am. J. Hum. Genet. 101, 5–22. doi:10.1016/j.ajhg.2017.06.005
Vranka, J. A., Kelley, M. J., Acott, T. S., and Keller, K. E. (2015). Extracellular matrix in the trabecular meshwork: Intraocular pressure regulation and dysregulation in glaucoma. Exp. Eye Res. 133, 112–125. doi:10.1016/j.exer.2014.07.014
Welter, D., Macarthur, J., Morales, J., Burdett, T., Hall, P., Junkins, H., et al. (2014). The NHGRI GWAS Catalog, a curated resource of SNP-trait associations. Nucleic Acids Res. 42, D1001–D1006. doi:10.1093/nar/gkt1229
Willer, C. J., Li, Y., and Abecasis, G. R. (2010). Metal: Fast and efficient meta-analysis of genomewide association scans. Bioinformatics 26, 2190–2191. doi:10.1093/bioinformatics/btq340
Wu, M. C., Kraft, P., Epstein, M. P., Taylor, D. M., Chanock, S. J., Hunter, D. J., et al. (2010). Powerful SNP-set analysis for case-control genome-wide association studies. Am. J. Hum. Genet. 86, 929–942. doi:10.1016/j.ajhg.2010.05.002
Wu, M. C., Lee, S., Cai, T., Li, Y., Boehnke, M., and Lin, X. (2011). Rare-variant association testing for sequencing data with the sequence kernel association test. Am. J. Hum. Genet. 89, 82–93. doi:10.1016/j.ajhg.2011.05.029
Wu, S. Y., and Leske, M. C. (1997). Associations with intraocular pressure in the Barbados eye study. Arch. Ophthalmol. 115, 1572–1576. doi:10.1001/archopht.1997.01100160742012
Xu, Z., Hysi, P., and Khawaja, A. P. (2021). Genetic determinants of intraocular pressure. Annu. Rev. Vis. Sci. 7, 727–746. doi:10.1146/annurev-vision-031021-095225
Zheng, Y., Xiang, F., Huang, W., Huang, G., Yin, Q., and He, M. (2009). Distribution and heritability of intraocular pressure in Chinese children: The guangzhou twin eye study. Invest. Ophthalmol. Vis. Sci. 50, 2040–2043. doi:10.1167/iovs.08-3082
Zhou, W., Nielsen, J. B., Fritsche, L. G., Dey, R., Gabrielsen, M. E., Wolford, B. N., et al. (2018). Efficiently controlling for case-control imbalance and sample relatedness in large-scale genetic association studies. Nat. Genet. 50, 1335–1341. doi:10.1038/s41588-018-0184-y
Zhou, W., Zhao, Z., Nielsen, J. B., Fritsche, L. G., Lefaive, J., Gagliano Taliun, S. A., et al. (2020). Scalable generalized linear mixed model for region-based association tests in large biobanks and cohorts. Nat. Genet. 52, 634–639. doi:10.1038/s41588-020-0621-6
Keywords: intraocular pressure, GWAS, ExWAS, polygenic risk score, Mendelian randomization, underrepresented population
Citation: Gao XR, Chiariglione M, Choquet H and Arch AJ (2023) 10 Years of GWAS in intraocular pressure. Front. Genet. 14:1130106. doi: 10.3389/fgene.2023.1130106
Received: 22 December 2022; Accepted: 05 April 2023;
Published: 12 April 2023.
Edited by:
Jordi Pérez-Tur, Spanish National Research Council (CSIC), SpainReviewed by:
Yeunjoo E. Song, Case Western Reserve University, United StatesAndrew DeWan, Yale University, United States
Copyright © 2023 Gao, Chiariglione, Choquet and Arch. This is an open-access article distributed under the terms of the Creative Commons Attribution License (CC BY). The use, distribution or reproduction in other forums is permitted, provided the original author(s) and the copyright owner(s) are credited and that the original publication in this journal is cited, in accordance with accepted academic practice. No use, distribution or reproduction is permitted which does not comply with these terms.
*Correspondence: Xiaoyi Raymond Gao, cmF5bW9uZC5nYW9Ab3N1bWMuZWR1