- 1Department of Gastrointestinal Colorectal Surgery, China-Japan Union Hospital of Jilin University, Changchun, China
- 2Department of Breast Surgery, China-Japan Union Hospital of Jilin University, Changchun, China
- 3Department of Orthopedics, China-Japan Union Hospital of Jilin University, Changchun, China
This study systematically and comprehensively analyzed the characteristics of matrix metalloproteinases (MMPs) in gastric cancer (GC) and revealed the relationship between MMPs and prognoses, clinicopathological features, tumor microenvironment, gene mutations, and drug therapy response in patients with GC. Based on the mRNA expression profiles of 45 MMP-related genes in GC, we established a model that classified GC patients into three groups based on cluster analysis of the mRNA expression profiles. The 3 groups of GC patients showed significantly different prognoses as well as tumor microenvironmental characteristics. Next, we used Boruta’s algorithm and PCA method to establish an MMP scoring system and found that lower MMP scores were associated with better prognoses, lower clinical stages, better immune cell infiltration, lower degrees of immune dysfunction and rejection, and more genetic mutations. Whereas a high MMP score was the opposite. These observations were further validated with data from other datasets, showing the robustness of our MMP scoring system. Overall, MMP could be involved in the tumor microenvironment (TME), clinical features, and prognosis of GC. An in-depth study of MMP patterns can better understand the indispensable role of MMP in the development of GC and reasonably assess the survival prognosis, clinicopathological features, and drug efficacy of different patients, thus providing clinicians with a broader vision of GC progression and treatment.
Introduction
Due to the improvement of general hygiene and wide applications of H. pylori quadruple therapy and gastroscopy, the incidence and mortality of gastric cancer (GC) have declined significantly worldwide in recent years (Choi et al., 2018). However, it is still a challenging disease in many countries and regions. The incidence of GC ranks third after lung and liver cancers, with a mortality rate of 8.2% (Bray et al., 2018). Moreover, the age of onset for GC has been decreasing in the last decades (Zhou et al., 2016). The development of GC is a complex process involving numerous factors (such as genetic and environmental factors) and steps. Genetic factors are supposed to be the most important factor for GC development. For instance, in a Swedish study that included 23,386 twins, it was found that if one of the twins was diagnosed with GC, the other was five times more likely to develop GC (Ekstrom et al., 2000), suggesting the importance of genetic factors in the development of GC. Previous studies have identified many susceptibility genes associated with GC. Cho et al. found that high mRNA expression of CTNNBA1, TOP2A, LZTR1, EXOSC3, LBA1, and CCL5 were closely associated with worse prognoses of GC (Cho et al., 2011). Kang et al. found that mRNA expression of ESRRG was significantly reduced in GC and negatively correlated with the stage and prognosis of GC (Kang et al., 2018). However, the prediction accuracy based on these genes is not satisfied. There are some targeted therapies, such as trastuzumab for HER-2, bevacizumab for VEGF-A (Wadhwa et al., 2013), and cetuximab for EGFR, that are currently widely used. However, only 5%–10% of GC patients are HER-2 positive, half of the GC patients have mutant KRAS genes (Albertini et al., 2017) and not all patients respond well to bevacizumab. All these indicate that more genes that can better reflect the severity and evaluate the prognosis of GC need to be identified (Wu et al., 2014; Ciliberto et al., 2015).
MMPs, belonging to the family of Ca2+ and Zn2+ dependent protein hydrolases, are synthesized and secreted by a variety of cells and can degrade components in the extracellular matrix (ECM). MMPs play important roles in cell proliferation, differentiation, migration, and apoptosis. There are 26 members in the MMP family, which can be further divided into five subgroups (Scherer et al., 2008). MMP2 and MMP9 are rich in invasive pseudopods and therefore have a strong catabolic effect on ECM both in vitro and in vivo (Murphy and Courtneidge, 2011). During tumor development, MMP2 and MMP9 may be involved in the regulation of tumor angiogenesis by undermining immunity, activating the TGF-β signaling, and releasing VEGF and bFGF (Solov'Eva et al., 2014), thus promoting the rapid growth and distant metastasis of tumor cells. Therefore, MMP may act as a bond between tumor cells and the tumor microenvironment of GC. In a previous meta-analysis, it was found that high expression of MMP2 was significantly associated with a worse prognosis of GC (Wang et al., 2014a). In another large-scale study, strong associations were observed between the positive expression of MT1-MMP, and peritoneal metastasis, lymph node metastasis, and worse prognosis of GC (Mimori et al., 2008). These previous studies indicate that MMPs might be important diagnostic and prognostic markers for GC. Therefore, analysis of MMP-related genes might reveal insights for the diagnosis and prognosis prediction of GC.
Thus, in this study, we established a molecular model that can classify GC patients into 3 patterns based on the mRNA expression of MMPs, which showed different prognostic and tumor microenvironmental features. Furthermore, based on this molecular model, the establishment of an MMP scoring system might be valuable for early diagnosis, prognosis prediction, and treatment of patients with GC.
Results
Establishment of a model based on the expression profiles of MMP-related genes
In this study, mRNA expression data of 45 MMP-related genes in GC were selected and analyzed. To maximize the classification of models with different characteristics, we applied an unsupervised cluster analysis method. The consensus cluster analysis revealed the optimal K = 3 (Figures 1A–C). As a result, the 411 GC patients were divided into 3 groups, i.e., Cluster (Group) 1, Cluster (Group) 2, and Cluster (Group) 3 (C1 = 113, C2 = 135, C3 = 163). The distribution of the 3 groups based on mRNA expression downscaled and validated by the PCA algorithm is shown in Figure 1D.
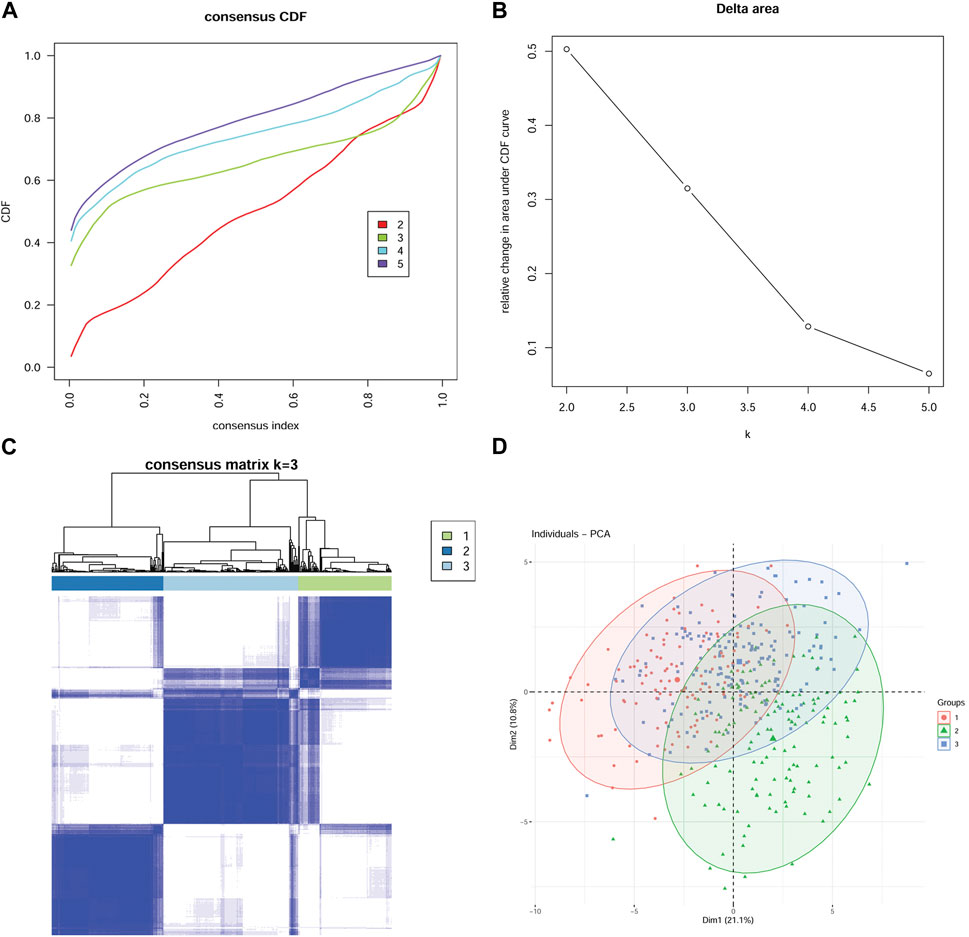
FIGURE 1. Establishment of a model based on expression profiles of 45 MMP-related genes Consensus CDF Plot (A): A plot of the cumulative distribution function for different values of K, used to determine the value of K at which the CDF reaches its approximate maximum. Thus the smaller the slope of the curve, the better the K value. Delta Area Plot (B) demonstrates the relative change in the area under the CDF curve when K is compared to K-1. The smaller the relative change, the higher the confidence level of the clustering. Therefore, after combining the Consensus CDF Plot and Delta Area Plot, K = 3 is the optimal number of clusters. Consensus Matrix Plot (C) verifies the high clustering confidence at K = 3. The higher the clustering confidence and differentiation, the higher the degree of color aggregation of different matrices in the matrix plot and the more obvious the degree of discrepancy between matrices. (D) The principal component analysis (PCA) algorithm confirmed the validity and reasonableness of the classification.
The three MMP- related groups are different in prognosis and tumor microenvironmental characteristics
Aiming at directly comparing the expression levels of the 45 MMP-related genes in the three groups, a heat map is drawn and the results are shown in Figure 2A. To compare the prognosis of patients in different model groups, we used Cox regression to analyze the survival time of patients in these three groups and found that there were significant differences in overall survival (OS) (p = 0.075; Figure 2B), progression-free survival (PFS) (p = 0.0015; Figure 2C), and disease-specific survival (DSS) (p = 0.0043; Figure 2D) among the three groups of patients. More specifically, patients in group C1 have better prognoses than patients in groups C2 and C3. However, disease-free survival (DFS) was not significantly different between these three groups (p = 0.18; Figure 2E). MSI scores are criteria that reflect the instability level of the microsatellite. To observe the microsatellite instability and predict the immunotherapy efficacy of patients in three groups, we further calculated and compared the MSI scores, and found that MSI scores were significantly different among the three groups (p < 0.0001 for all; Figure 2F). Specifically, MSI scores are higher in the C1 group than in C2 and C3 groups. Furthermore, to evaluate the correlation between the TME and those three groups, we calculated the immune score, matrix score, and microenvironment score using XCELL, and analyzed their difference. As shown in Figure 2G, the immune cell infiltration in the three groups, indicated by activated myeloid dendritic cells, T lymphocytes, endothelial cells, hematopoietic stem cells, tumor-derived fibroblasts, neutrophils, monocytes infiltration and immune scores, stromal scores, and microenvironment scores, is different in the three groups. These results indicated that our model using these 45 MMP-related genes could effectively classify GC patients into different groups with different immunologic and prognostic features.
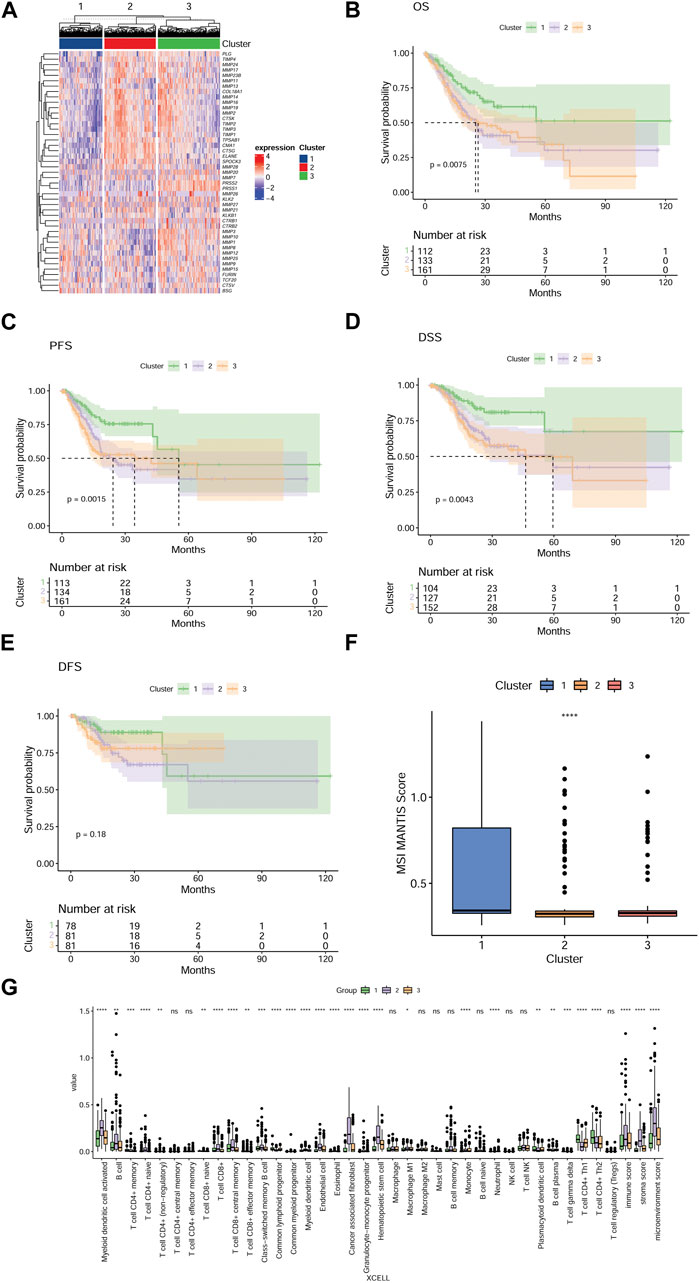
FIGURE 2. The three MMP-related groups in GC patients have significant differences in prognosis and tumor microenvironment characteristics (A) A heat map shows the expression levels of 45 MMP-related genes in three groups, and each row in the heat map represents one MMP-related gene. The degree of expression of the gene varies among the three groups, the higher the expression level, the redder the color, and the lower the bluer. Kaplan-Meier survival analysis comparing the differences in OS (B), PFS (C), DSS (D), and DFS (E) among the three groups of patients. (F) Box plots depict the MSI scores of the three groups of patients. (G) Box plots showing differences in the tumor microenvironment among the three groups of patients. *p < 0.05; **p < 0.01; ***p < 0.001; ****p < 0.0001. Ns, not significant.
Establishment of an MMP scoring system for GC patients
To downscale the prognosis and the differences in TME of different patients, we established the MMP scoring system to make the subsequent clinical analysis more concise and clearer. We identified the DEGs among the three groups using the Boruta algorithm and obtained the signature A genes and signature B genes. A heat map was depicted to directly visualize the expression level of the 126 most abundant DEGs among the three groups (Figure 3A). All GC patients were then scored using the principal component analysis (PCA) algorithm. According to the median of the scores, GC patients were divided into a high MMP score group and a low MMP score group. To delineate the correlation between the MMP score, the survival status of the patients, and the MMP-related groups, Figure 3B was depicted. As the result, the patients in the C1 group with the best prognosis are those with low MMP scores, while the patients in the C2 and C3 groups with relatively poor prognosis are mostly those with high MMP scores. Aiming at further analyzing the relationship between MMP scores and prognosis, the results are shown in Figures 3C–F. Low MMP scores are significantly associated with OS (p = 0.0052), PFS (p = 0.00074), DSS (p = 0.0013), and DFS (p = 0.023). For the sake of identifying the difference in microsatellite instability and predicting the efficacy of immunotherapy, we analyzed the MSI scores in different MMP patterns. We showed that the difference in MSI scores was significant between the two groups (p < 0.0001; Figure 3G), consistent with those from the C1, C2, and C3 groups. That is, the low MMP score group with a good survival outcome had a higher MSI score as did the C1 group. In the case of analyzing and validating the predictive efficacy of the MMP scoring system, we further calculated the AUC of our model in predicting OS, PFS, DSS, and DFS (Figures 3H–K), and showed that MMP scores are effective in predicting survival at 12, 24, 36, 48 and 60 months in patients with GC. To compare and identify the discrepancy in TME of different MMP scoring groups, again we calculated the infiltration ratios of multiple immune cells, the immune score, the stromal score, and the microenvironmental score using Xcell (Figure 3L). We showed that lower MMP scores were associated with lower infiltration of myeloid dendritic cells, B lymphocytes, cytotoxic T lymphocytes, monocytes, macrophages, tumor-derived fibroblasts, and lower immune, stromal, and microenvironment scores, indicating an inextricable relationship between MMP score and tumor microenvironment.
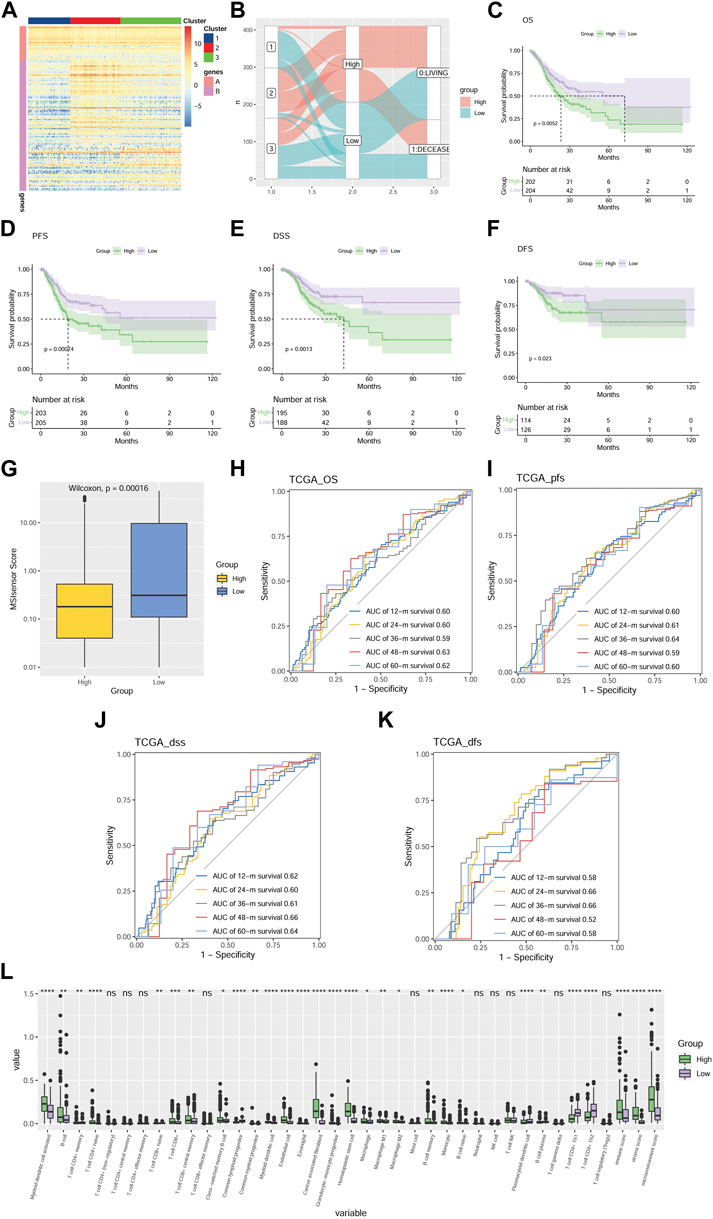
FIGURE 3. Development of an MMP scoring system for GC patients (A) Heat map depicts the expression situation of the 126 most abundant DEGs in the genome in three different gene clusters. Those DEGs are divided into Signature A and B genes. Each row represents one DEG. The higher the expression level of a DEG, the redder the color, and the lower the bluer. (B) Alluvial plots depicting the relationship between three molecular models, MMP scores, and survival status. Kaplan-Meier survival analysis comparing the differences in OS (C), PFS (D), DSS (E), and DFS (F) among the three groups. (G) Box plots show the difference in MSI scores between the two groups. ROC is based on MMP scores for OS (H), PFS (I), DSS (J), and DFS (K). (L) Box plot compares the discrepancy of immune infiltration level of various immune cells and immune score, stromal score, and microenvironmental score in TME between two groups of patients with MMP scores. The higher the immune infiltration and the immune score, stromal score, and microenvironmental score, the higher the value. *p < 0.05; **p < 0.01; ***p < 0.001; ****p < 0.0001. Ns, not significant.
Association of MMP scores with clinicopathological features in patients GC
To analyze the correlation between MMP scores and major clinicopathological features in patients with GC and validate the clinical value of the MMP scoring system, we delineate the box plots. As shown in Figure 4, all the clinicopathological features, except for the N stage and M stage, are associated with the MMP scores. The MMP scores were significantly lower in patients in stage T1 than those in stages T2, T3, and T4, significantly higher in patients with G3 than those with G1 and G2, significantly lower in patients in stage I than those in stages II, III and IV, significantly lower in patients in group C1 than those in groups C2 and C3. These results indicate that MMP scores are closely related to major clinicopathological characteristics of gastric cancer.
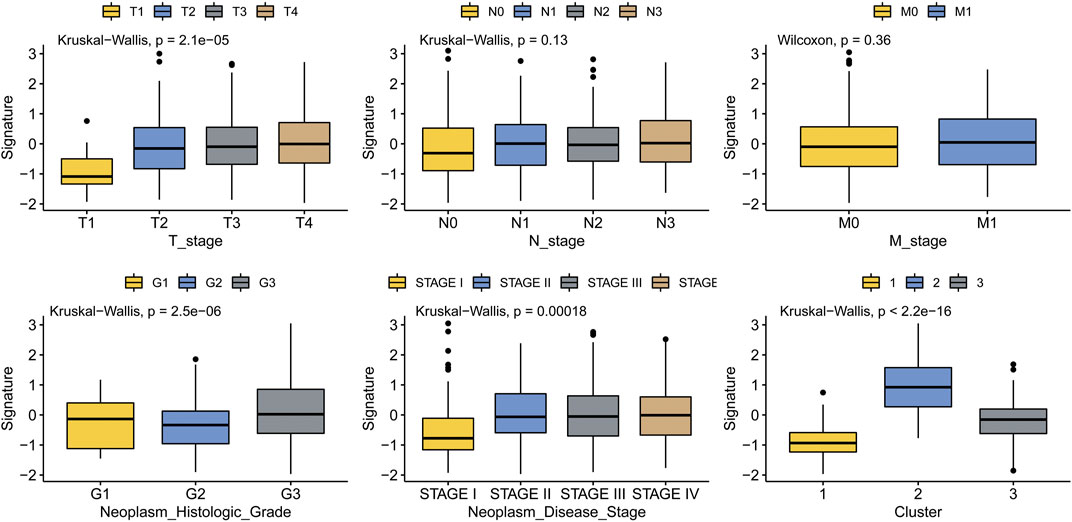
FIGURE 4. Association of MMP scores with clinicopathological features in patients with gastric cancer.
Gene set enrichment analysis of DEGs
Aiming at further understanding the terms (i.e., biochemical processes, molecular functions, and cellular components), signaling pathways involved in the low MMP score and high MMP score groups and speculating the roles they play in the development of GC, we performed KEGG and GO enrichment analysis of the DEGs using gene set enrichment analysis (GESA); significantly enriched terms or pathways are illustrated using the bubble plots in Figures 5A, B. KEGG enrichment analysis showed that various signaling pathways, including DNA replication, proteasome, homologous recombination, and mismatch repair, were enriched in the low MMP score group. While various pathways, including dilated cardiomyopathy, tyrosine metabolism, calcium signaling pathway, and ECM receptor interaction, were significantly enriched in the high MMP score group. GO enrichment analysis demonstrated that DNA strand elongation, nuclear replication fork, histone exchange, and nucleoid and kinetochore organization terms were enriched in the low MMP score group, whereas the immunoglobulin complex, phagocytosis recognition, complement activation, and antigen binding terms were enriched in the high MMP score group.
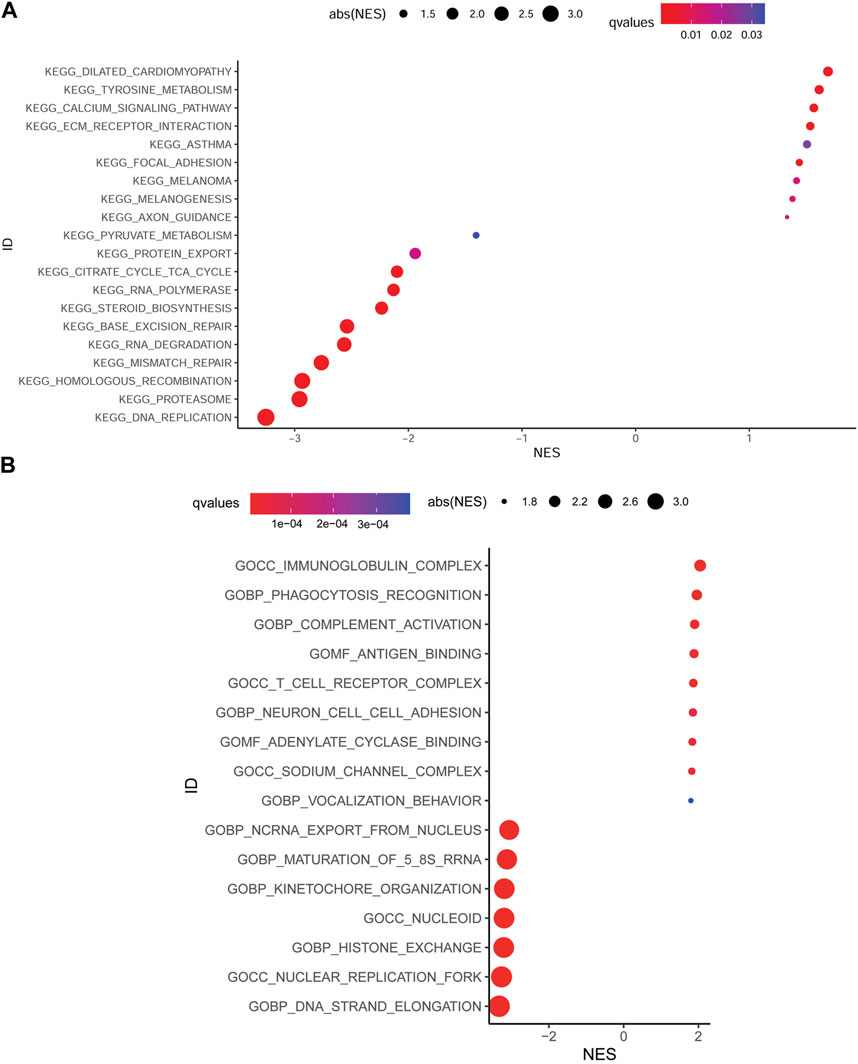
FIGURE 5. Bubble plots depicting the enrichment of KEGG (A) and GO (B) in different score groups of MMP.
Gene mutations in different MMP score groups
A gene mutation is a type of contributing factor in the development of GC, different gene mutations might play different roles. For the sake of further identifying the role of different genes on gastric cancer, we analyze and compare gene mutations between patients in the two MMP score groups. The data were presented as gene mutation maps as shown in Figures 6A, B. The highest mutation rates were found in TTN, TP53, MUC16, and LRP1B genes, which were present in both the high and low MMP score groups. Specifically, the four genes in the low MMP score group had higher mutation rates than those in the high MMP score group. Moreover, to vividly compare the overall gene mutation counts in these two MMP score groups, we drew a box plot that delineates that the low MMP score group has higher overall mutation counts than the high MMP score group (Figure 6C). To verify whether the mutation counts in different genes were statistically significant between the two MMP score groups, we applied the logistic binary regression analysis. As shown in Figure 6D, we found that the high MMP score group tends to have fewer mutation genes.
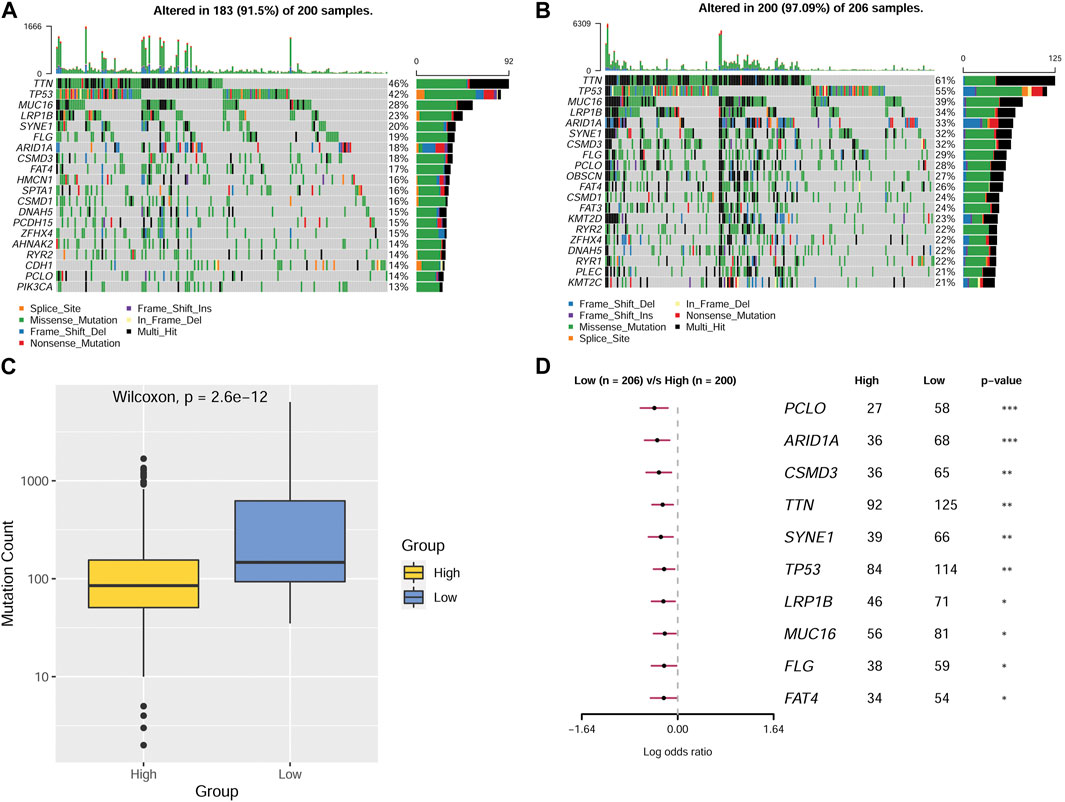
FIGURE 6. Mutations in patients from different MMP scoring groups (A) Mutations in MMP high-scoring group. (B)Mutations in MMP low-scoring group. (C) Mutation counts in different MMP scoring groups. (D) Forest plot depicting statistical differences of various genes in different MMP scoring groups.
MMP scores and drug treatment
Drug therapy plays an important role in the clinical diagnosis and treatment of GC. Aiming at finding out the efficacy and sensitivity of various chemotherapy and targeted therapy drugs on GC, we compared the predicted IC50 values of eight different drugs, including sorafenib, sunitinib, cisplatin, gefitinib, vincristine, vorinostat, and gemcitabine, between the two MMP score groups (Figure 7A). We found that the predicted IC50 values of gefitinib in the low MMP score group were smaller than those in the high MMP score group. In contrast, the predicted IC50 values for sorafenib, sunitinib, vincristine, and vorinostat were relatively lower in the high MMP score group. Aiming at analyzing the efficacy and suitability of immunotherapy and the TME disorder, TIDE score, immune dysfunction, and rejection were taken into full consideration. We found that patients with higher MMP scores tend to have relatively higher TIDE scores, suggesting that patients in the high MMP score group are more prone to immune dysfunction and rejection (Figure 7B).
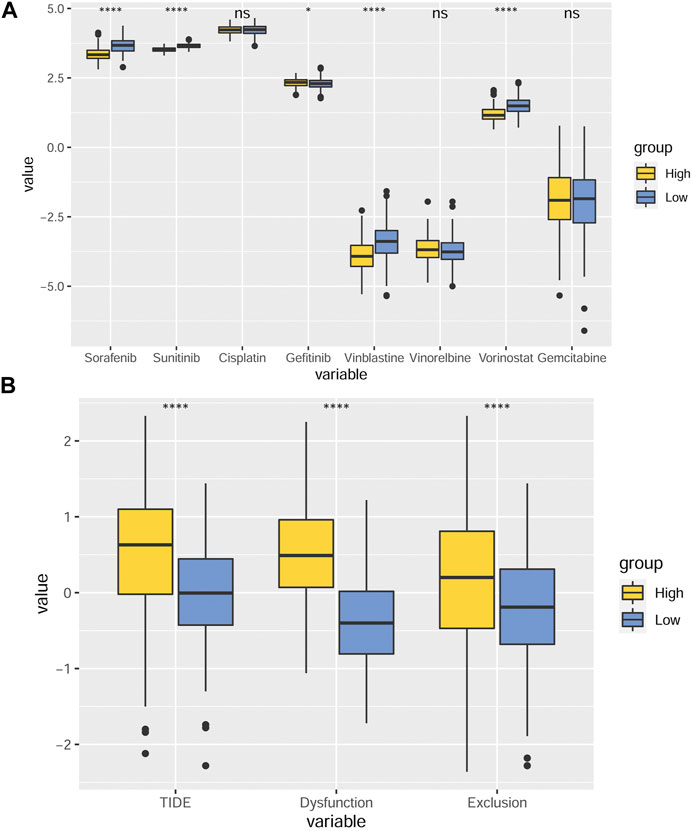
FIGURE 7. MMP scores and drug IC50 inference (A) Box plots depicting the differences in predicted IC50 values for sorafenib, sunitinib, cisplatin, gefitinib, vincristine, vorinostat, and gemcitabine between the different MMP scoring groups. (B) Differences in TIDE scores, immune dysfunction, and rejection in different MMP scoring groups. *p < 0.05; **p < 0.01; ***p < 0.001; ****p < 0.0001. Ns, not significant.
Validation of the MMP scoring system using multiple independent datasets
To identify the robustness of the MMP scoring system and validate that MMP low scores patients have a more optimistic outcome, we applied the MMP scoring system to four external datasets from the GEO database (GSE26901, GSE13861, GSE26899, and GSE66229) and divided patients in these 4 datasets into high and low MMP score groups. Next, we compared the high and low MMP score groups for each dataset using the log-rank survival analysis; the results are shown in Figures 8A–D. Consistently, these results all show that the OS of patients with GC in the low MMP score group is longer than that of patients in the high MMP score group.
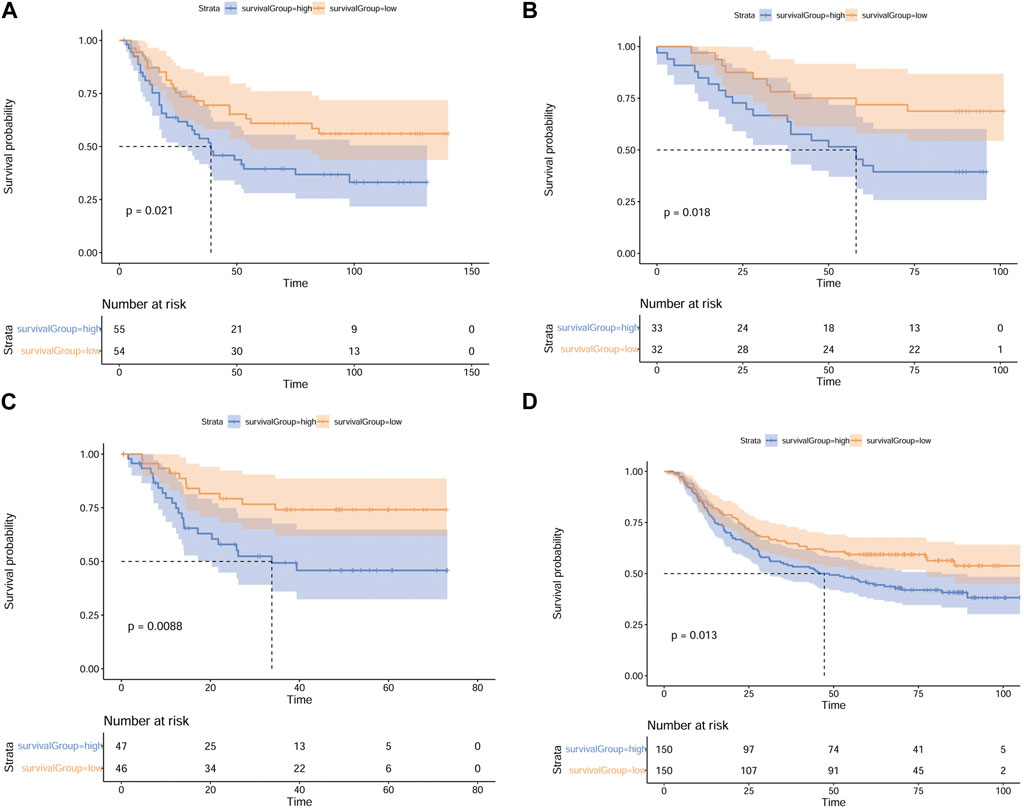
FIGURE 8. Validation of the MMP scoring system using 4 external datasets. Full validation of the MMP scoring system by four datasets GSE26901. (A), GSE13861 (B), GSE26899 (C), and GSE66229 (D) in the GEO database.
Discussion
In this study, we systematically and comprehensively analyzed the clinical significance of MMP-related genes and explored their association with tumor microenvironments in patients with GC. We then established an MMP scoring system and explored how the MMP score was associated with prognosis, disease characteristics, and drug treatment effects in patients with GC. Finally, we verified the robustness of the MMP scoring system using external datasets. Since patients with different MMP scores have significantly different clinical traits, genetic mutation characteristics, metabolic characteristics, and drug response characteristics, we believe that clinicians can apply the MMP scoring system to each GC patient for predicting the survival prognosis, and use more effective drugs in the treatment. At the same time, physicians can find more targeted therapeutic drugs and treatment methods through different metabolic pathways and genetic mutations based on the MMP scoring system to improve patients’ prognosis and life quality in the future.
After establishing the MMP-related groups, we compared the survival, MSI scores, and tumor microenvironmental characteristics between the 3 groups. Regarding survival, patients in the C1 group had the best prognoses. Correspondingly, C1 group patients own the highest MSI score. The results above are consistent with previous studies showing that GC patients with high MSI scores have a better prognosis than patients with low MSI gastric cancer of the same pathological type (Baniak et al., 2016; Polom et al., 2018), indicating the robustness and usefulness of the MMP-related groups. The tumor microenvironment plays a pivotal role in tumor progression by interacting with tumor cells. Different studies have demonstrated the inextricable link between tumor-infiltrating lymphocytes and the prognosis of GC (Hu et al., 2019). We found that the TME characteristics of the different MMP-related groups differed. The results of our current research are in line with previous studies showing that the lower the level of CD4+ T lymphocyte infiltration, the worse the prognosis of the patient and the more advanced the stage of the tumor would be (Li et al., 2019). Our findings are also supported by a study containing 1524 GC patients that explored the relationship between tumor-derived fibroblasts and clinical features, which showed that higher tumor-derived fibroblast infiltration was associated with a less favorable prognosis (Zeng et al., 2019). Another research, which showed that tumor-associated mononuclear macrophages promote rapid growth and distant metastasis of GC through the secretion of cytokines and growth factors (Qian and Pollard, 2010), is also consistent with our findings. Similarly, a direct correlation between low infiltration of myeloid dendritic cells and good prognoses of malignancy was observed in a large-scale study (Bai et al., 2021), further demonstrating the validity of our MMP-related groups.
A thorough analysis of this MMP scoring system was carried out and we showed that the MMP score could be used as an appropriate indicator for the assessment of the prognosis of GC. This conclusion is supported by the results of other studies exploring the relationship between MMP expression and pathological features of GC. For example, Rybarczyk et al. (2012) found that higher expression of MMP was directly associated with higher infiltration of immune cells and greater lymph node metastasis in GC. Patients in the low MMP score group had higher MSI scores, consistent with a previous study exploring the relationship between MSI and the prognosis of GC (Kim et al., 2017). Because PD-1 inhibitors are more effective for GC patients with high MSI scores (Zhang et al., 2020a), MMP scores have the potential to predict patient response to immunotherapy. Patients with low MMP scores respond better to anti-PD-1 treatment. Immune cells and stromal cells are the main components of the tumor microenvironment, so we used Xcell to estimate the ratios of infiltrated immune cells and stromal cells, and calculate the immune score and stromal score (Yoshihara et al., 2013). High immune scores are significantly associated with high-stage, low differentiation, and poor prognosis of GC (Zeng et al., 2018). This undoubtedly supported our findings. At the same time, we found that low MMP score patients with low stroma scores have better overall survival, consistent with a previous study showing that stroma scores can be used as an independent predictor of prognosis in GC (Mao et al., 2020). Further, based on the stroma score and immune score, a microenvironmental score was constructed by other researchers to compare various features of GC and it was found that a low microenvironmental score predicted a better prognosis of gastric cancer and a lower inflammatory response (Zhou et al., 2017). Correspondingly in our study, the low MMP score patients with better prognoses have the same lower microenvironmental score. In summary, those ramifications demonstrate that the MMP score can be used as a reliable indicator to predict the prognosis of patients with GC.
Studies on the relationship between the tumor stage, tumor differentiation, immune cell infiltration, and prognosis of GC have shown that the more advanced the TNM stage of GC is (Ahn et al., 2010), the worse the differentiation (Wanebo et al., 1993), the less degree of infiltration (Marchet et al., 2008), the more pessimistic the outcome will be. A high MMP score is associated with unpromising clinicopathological characteristics, indicating that the MMP scoring system can be used as an appropriate assessment in clinical practice.
The development and rapid growth of GC and distant metastasis involve a complex array of biological changes. As a result, the impact of MMP as a key factor in GC should be taken seriously. Many researchers have conducted various studies on the biological behaviors of GC through the alteration of different signaling pathways, BP, MF, and CC by MMP. MMP-2 enhances the invasion and metastasis of GC through the NF-KB pathway (Wang et al., 2014b). PPARγ, Cx43, and Grhl2 negatively regulate the expression of MMP-2, thereby inhibiting the progression of GC (He et al., 2007). Silymarin et al. reduced the expression of MMP-2 and MMP-9 by down-regulating the P38MAPK signaling pathway, thereby inhibiting the invasion and metastasis of human GC SGC7901 cells (Lu et al., 2021). In our study, we found that MMP could also alter the biological characteristics of GC through various pathways, implying that MMP-related genes are involved in a wide range of biological alterations. In addition, since the DNA replication, proteasome, DNA strand elongation, as well as histone exchange terms or pathways, were significantly enriched in the low MMP score group, we speculated that these altered biological behaviors might be associated with a good prognosis of GC. Conversely, tyrosine metabolism, calcium signaling pathway, ECM receptor interaction, and immunoglobulin complex terms or pathways may be indicative of a worse prognosis for GC. However, the mechanism of these alterations in signaling pathways or terms (i.e., BP, CC, and MF) affecting the progression of GC remains to be validated.
It is well known that genetic mutations can be involved in the development of gastric cancer by altering several physiological and biochemical processes. Based on the mutations of various genes in the two MMP score groups and the corresponding prognoses, TTN, TP53, MUC16, and LRP1B genes might play important roles in influencing the outcomes and clinicopathological features of GC. One study showed that GC patients with wild-type TP53 had a shorter OS and 1.39 times the risk of death than patients with TP53 mutations (Deng et al., 2021). Consistently, in our study, most of the patients in the MMP high group contained wild-type TP53 and their survival prognoses were significantly worse, further supporting the validity and reliability of the MMP scoring system. In a joint study of the relationship between mutations in MUC4, MUC16, TTN, and the prognosis of GC, it was found that the higher the number of mutations in these three genes is, the longer the OS of the patients and correspondingly the higher the TMB (Yang et al., 2020) will be. In addition, a high mutation in TTN predicts a good ICB treatment outcome (Jia et al., 2019). Our data also showed that patients in the low MMP score group with a better prognosis tend to have higher TTN mutations. What’s more, patients in the low MMP score group with higher MSI scores and lower TIDE scores are likely to have better ICB treatment outcomes, coinciding with the aforementioned study (Jia et al., 2019). After comparing the expression of the MUC16 gene in GC patients, some researchers concluded that high expression of the MUC16 gene was significantly associated with a poorer prognosis (Streppel et al., 2012; Jonckheere and Van Seuningen, 2018). While other researchers found that the survival rate of MUC16 mutant patients was higher than that of MUC16 wild-type patients (Li et al., 2018). Those results are consistent with our study. We speculated that the mutation of MUC16 could result in a decrease in its expression, thereby improving the prognosis of GC. A gene sequencing analysis of Chinese GC patients showed that TP53, ARID1A, and LRP1B genes were the top 3 genes containing the most mutations (Yu et al., 2021), which is similar to our findings. However, there are no studies investigating the correlation between LRP1B gene mutation and the prognosis of GC. Based on the results of our data, we speculated that a high mutation in the LRP1B gene might be associated with a good prognosis in GC. Therefore, our data can be used as a reference for the study of the relationship between different gene mutations and GC prognoses. Moreover, it will provide new ideas for screening better prognostic markers for GC in the future.
Identification of new drugs for the treatment of GC has been a clinical priority. The current chemotherapy regimens recommended by the CSCO are SOX, XELOX, and FOLFOX. In clinical practice, however, not all patients benefit from these chemotherapy regimens. Mutations in the KRAS and BRAF genes, decreased expression of HER-2 in many cases of GC patients, and the fact that bevacizumab is not suitable for most patients, have hindered the application of chemotherapy and targeted therapy in GC. Our study suggests that patients in different MMP score groups might benefit from different drugs. For example, gefitinib is more suitable for patients with low MMP scores, while sorafenib, sunitinib, vincristine, and vorinostat might be more suitable for patients with high MMP scores. Our MMP scoring system could be used to predict drug efficacy, and thus improve the efficacy of chemotherapy or targeted therapy for GC treatment. However, further clinical trials and laboratory validation are still needed. In recent years, immunotherapy has become increasingly popular and it has been elevated from second or even third-line treatment to first-line treatment. To predict and assess the prognosis of ICB treatment, TIDE scores were calculated based on the immune dysfunction of tumor tissue infiltrating CD8+ T cells and immune rejection (Jiang et al., 2018). The higher the TIDE score is, the less effective the immunotherapy and the shorter the survival after receiving the treatment will be. Our results imply that immunotherapy might be more appropriate for patients in the low MMP score group, consistent with the aforementioned finding that patients in the low MMP score group with higher MSI scores were more likely to benefit from ICB treatment. This observation further demonstrates the potential of the MMP score to assess and predict the efficacy of ICB treatment in patients with GC. Similarly, we found that immune dysfunction and rejection of tumor-infiltrated cytotoxic T lymphocytes profoundly affect the prognosis of GC. Although patients in the high MMP score group had more CD8+ T lymphocytes in their tumor microenvironment, due to the presence of immune dysfunction and rejection, the number of immune cells that are actually effective in eliminating tumor cells is greatly reduced, thus affecting the prognosis of GC.
Finally, the MMP scoring system applied in other GC patients from the GEO database (GSE26901, GSE13861, GSE26899, and GSE66229) validates that the low MMP score group has better prognoses, indicating the robustness, reliability, and usefulness of the MMP scoring system in GC.
Our research initially concludes that MMP influences the progression of GC through the database as well as statistical software analysis, which provides a preliminary verification and framework of the significant role of MMP in the development of GC. In the next step, multiple experiments such as Western blot for exploring the expression of MMP in different stages of GC, MTT assay for elucidating and demonstrating the effectiveness of those 8 drugs mentioned above in inhibiting GC cells, the mechanism of signaling pathways of KEGG and GO affecting GC progression will be carried out to further validate the indispensable role MMP plays in the development of GC.
Conclusion
In summary, the MMP scoring system proposed in this study provides a comprehensive and integrated assessment of the prognosis, clinicopathological features, tumor microenvironment, and ICB treatment outcomes of patients with GC. There is a significant difference regarding prognosis between the high and low MMP scoring groups. A low MMP score predicts a better prognosis. Therefore, the MMP scoring system could potentially facilitate the diagnosis and personalized treatment of GC patients in clinical practice.
Materials and methods
RNA sequencing (RNA-seq) profiles and matched complete clinical information (age, sex, survival status, staging, and staging) were retrieved from TCGA (https://www.cancer.gov/tcga) for STAD (n = 411). TCGA is a landmark cancer genomicsprogram, which molecularly characterized over 20,000 primary cancer and matched normal samples spanning 33 cancer types. This joint effort between NCI and the National Human Genome Research Institute began in 2006, bringing together researchers from diverse disciplines and multiple institutions.
Establishment of MMP-related molecular model group
The Molecular Signatures Database database (https://www.gsea-msigdb.org/gsea/msigdb/index.jsp) is a resource of tens of thousands of annotated gene sets for use with GSEA software, from this website, we can browse, investigate and download gene sets (Subramanian et al., 2005). Two gene sets in the database were selected, where REACTOME_ACTIVATION_OF_MATRIX_METALLOPROTEINASES was contributed by Reactome (https://reactome.org/PathwayBrowser/#/R-HSA-1592389) and WP_MATRIX_METALLOPROTEINASES was contributed by WikiPathways (http://www.wikipathways.org/instance/WP129_r117774). We defined 45 genes in total involved in these two gene sets as MMP-related genes in GC. Then we use the ConsensionClusterPlus package in R software to estimate the number of unsupervised classes in the TCGA-STAD dataset (Wilkerson and Hayes, 2010). The PCA in the ggplot2 package in R software was then applied to downscale the expression profile subsets of the above genes.
MMP-related groups in GC patients have significant differences regarding prognosis and tumor microenvironment characteristics
Heat maps were plotted for these three MMP-related groups and the survminer package in R software was applied to plot survival curves for OS, PFS, DSS, and DFS and box line plots for MSI scores. MSI score was calculated through MANTIS software, which is a tool for identifying microsatellite instability in paired tumor-normal patient samples (Bonneville et al., 2017; Kautto et al., 2017). Cell-type enrichment analysis was performed on each GC sample by XCell software (https://xcell.ucsf.edu/). XCell is a web tool that performs cell type enrichment analysis from gene expression data for 64 immune and stroma cell types, which uses a novel method that integrates the advantages of gene set enrichment with deconvolution approaches (Aran et al., 2017). The levels of infiltrated immune cells were compared between groups by the Kruskal-Wallis test and visualized with box plots.
Development of an MMP scoring system for GC patients
The limma package in R was used to identify the DEGs in these three groups (C1, C2, and C3), with thresholds of adjusted p < 0.05 and |Log2FC| > 1 (Ritchie et al., 2015). The DEGs positively and negatively correlated with cluster features are named MMP gene signatures A and B, respectively. The dimensionality reduction of MMP gene signatures A and B was achieved using the Boruta algorithm, and the MMP score was calculated according to the following formula: MMP score = ∑PC1A—∑PC1B, where PC1A represents the first component of signature A and PC1B indicates the first component of signature B (Zhang et al., 2020b). The ggalluvial package was used to draw the Alluvial diagram to visualize the three groups regarding MMP scores and survival status (Brunson, 2017). The survminer and survivalROC packages were then used to plot survival curves of OS, PFS, DSS, and DFS and ROC curves for the model. The levels of infiltrated immune cells were then compared between the high and low MMP score groups by the Kruskal-Wallis test. Finally, box plots of MSI scores were plotted for the high and low MMP score groups.
Association of MMP scores with clinicopathological features of GC
We fused the patients’ clinical data with MMP scores and applied R software to draw box plots depicting the relationship between T, N, M, G, TNM Stages, three MMP-related groups, and MMP scores.
Gene probe enrichment analysis of DEGs
KEGG and GO enrichment analyses were performed using GSEA software for the high and low MMP groups. Pathways with FDR < 0.05 were considered significantly enriched and enriched signaling pathways were represented using plot bubble maps using the ggPub package. GSEA software uses a computational method that determines whether an a priori defined set of genes shows statistically significant, concordant differences between two biological states (Subramanian et al., 2005).
Gene mutations in different MMP score groups
The mutation data in GC patients were downloaded from the TCGA database. The data were applied to the maftools package to depict the mutation maps for patients in the high and low MMP score groups. Maftools package offers a multitude of analysis and visualization modules that are commonly used in cancer genomic studies, including driver gene identification, pathway, signature, enrichment, and association analyses (Mayakonda et al., 2018). The numbers of mutations were represented using the box plots. The number of mutated genes that differed between the two groups was represented using the Forest plots. p < 0.05 was considered statistically significant.
MMP scores and drug IC50 inference
The database information collated from the pRRophetic package in R was invoked to calculate the IC50 values for the eight drugs, which were visualized using the box plots. In the pRRophetic package, we need only provide the baseline gene expression and IC50, the predicted drug sensitivity is then calculated (Paul G et al., 2014). TIDE stands for Tumor Immune Dysfunction and Exclusion. It is a computational framework developed to evaluate the potential of tumor immune escape from the gene expression profiles of cancer samples. The TIDE score, scores for immune dysfunction and rejection were calculated for both groups of patients using the TIDE website (http://tide.dfci.harvard.edu/login/) and plotted using the box plots (Fu et al., 2020).
Independent cohort validation of the MMP scoring system
Survival curves for the GC patients in four validation datasets in the GEO database (https://www.ncbi.nlm.nih.gov/geo/) were plotted by applying the survminer package and tested using the Log Rank method. GEO is an international public repository that archives and freely distributes microarray, next-generation sequencing, and other forms of high-throughput functional genomics data submitted by the research community (Kumar et al., 2019). We selected four datasets (GSE26901、GSE13861、GSE26899、GSE66229) about gene expression profiles of GC from GEO for the validation group. (GSE26901 from the Kosin University College of Medicine, GSE13861 from the Yonsei University Severance Hospital, GSE26899 from the Korea University Guro Hospital, and GSE66229 from the Asian Cancer Research Group) (Cho et al., 2011; Oh et al., 2018; Nshizirungu et al., 2021).
Data availability statement
The original contributions presented in the study are included in the article/supplementary material, further inquiries can be directed to the corresponding authors.
Author contributions
All authors contributed to the study conception and design. Material preparation, data collection and analysis were performed by HaX, JH, and HiX. The first draft of the manuscript was written by JH, CY and JY, and all authors commented on previous versions of the manuscript. All authors read and approved the final manuscript.
Funding
This work was supported by the Scientific and Technological Development Program of Jilin Province, China (No. 20200404134YY), the Natural Science Foundation of Jilin Province, China (No. 20210101256JC) and Jilin Provincial Research Foundation of China Grants (Nos. 2018SCZ014, 2019SCZ049).
Conflict of interest
The authors declare that the research was conducted in the absence of any commercial or financial relationships that could be construed as a potential conflict of interest.
Publisher’s note
All claims expressed in this article are solely those of the authors and do not necessarily represent those of their affiliated organizations, or those of the publisher, the editors and the reviewers. Any product that may be evaluated in this article, or claim that may be made by its manufacturer, is not guaranteed or endorsed by the publisher.
References
Ahn, H. S., Lee, H. J., Hahn, S., Kim, W. H., Lee, K. U., Sano, T., et al. (2010). Evaluation of the seventh American joint committee on cancer/international union against cancer classification of gastric adenocarcinoma in comparison with the sixth classification. CANCER-AM CANCER Soc. 116 (24), 5592–5598. doi:10.1002/cncr.25550
Albertini, A., Raoux, D., Neumann, F., Rossat, S., Tabet, F., Pedeutour, F., et al. (2017). Analyse des mutations des gènes RAS par technique cobas ® au sein d’une structure de pathologie libérale: Étude médico-économique et moléculaire comparative avec une plateforme labellisée INCa. B CANCER 104 (7-8), 662–674. doi:10.1016/j.bulcan.2017.05.005
Aran, D., Hu, Z., and Butte, A. J. (2017). xCell: digitally portraying the tissue cellular heterogeneity landscape. GENOME Biol. 18 (1), 220. doi:10.1186/s13059-017-1349-1
Bai, D., Feng, H., Yang, J., Yin, A., Lin, X., Qian, A., et al. (2021). Genomic analysis uncovers prognostic and immunogenic characteristics of ferroptosis for clear cell renal cell carcinoma. Mol. Ther. - Nucleic Acids 25, 186–197. doi:10.1016/j.omtn.2021.05.009
Baniak, N., Senger, J. L., Ahmed, S., Kanthan, S. C., and Kanthan, R. (2016). Gastric biomarkers: A global review. WORLD J. Surg. Oncol. 14 (1), 212. doi:10.1186/s12957-016-0969-3
Bonneville, R., Krook, M. A., Kautto, E. A., Miya, J., Wing, M. R., Chen, H. Z., et al. (2017). Landscape of microsatellite instability across 39 cancer types. JCO Precis. Oncol. 2017, 1–15. doi:10.1200/PO.17.00073
Bray, F., Ferlay, J., Soerjomataram, I., Siegel, R. L., Torre, L. A., and Jemal, A. (2018). Global cancer statistics 2018: GLOBOCAN estimates of incidence and mortality worldwide for 36 cancers in 185 countries. CA Cancer J. Clin. 68 (6), 394–424. doi:10.3322/caac.21492
Brunson, J. C. (2017), ggalluvial: Layered grammar for alluvial plots. J. Open Source Softw. 5, 2017. doi:10.21105/joss.02017
Cho, J. Y., Lim, J. Y., Cheong, J. H., Park, Y., Yoon, S., Kim, S. M., et al. (2011). Gene expression signature–based prognostic risk score in gastric cancer. Clin. CANCER Res. 17 (7), 1850–1857. doi:10.1158/1078-0432.CCR-10-2180
Choi, I. J., Kook, M. C., Kim, Y. I., Cho, S. J., Lee, J. Y., Kim, C. G., et al. (2018). Helicobacter pylori therapy for the prevention of metachronous gastric cancer. N. Engl. J. Med. 378 (12), 1085–1095. doi:10.1056/NEJMoa1708423
Ciliberto, D., Staropoli, N., Caglioti, F., Gualtieri, S., Fiorillo, L., Chiellino, S., et al. (2015). A systematic review and meta-analysis of randomized trials on the role of targeted therapy in the management of advanced gastric cancer: Evidence does not translate? CANCER Biol. Ther. 16 (8), 1148–1159. doi:10.1080/15384047.2015.1056415
Deng, W., Hao, Q., Vadgama, J., and Wu, Y. (2021). Wild-type TP53 predicts poor prognosis in patients with gastric cancer. J. Cancer Sci. Clin. Ther. 5 (1), 134–153. doi:10.26502/jcsct.50790107
Ekstrom, A. M., Hansson, L. E., Signorello, L. B., Lindgren, A., Bergstrom, R., and Nyren, O. (2000). Decreasing incidence of both major histologic subtypes of gastric adenocarcinoma--a population-based study in Sweden. Br. J. Cancer 83 (3), 391–396. doi:10.1054/bjoc.2000.1205
Fu, J., Li, K., Zhang, W., Wan, C., Zhang, J., Jiang, P., et al. (2020). Large-scale public data reuse to model immunotherapy response and resistance. GENOME Med. 12 (1), 21. doi:10.1186/s13073-020-0721-z
He, Q., Chen, J., Lin, H. L., Hu, P. J., and Chen, M. H. (2007). Expression of peroxisome proliferator-activated receptor gamma, E-cadherin and matrix metalloproteinases-2 in gastric carcinoma and lymph node metastases. Chin. Med. J. Engl. 120 (17), 1498–1504. doi:10.1097/00029330-200709010-00007
Hu, K., Wang, S., Wang, Z., Li, L., Huang, Z., Yu, W., et al. (2019). Clinicopathological risk factors for gastric cancer: A retrospective cohort study in China. BMJ OPEN 9 (9), e030639. doi:10.1136/bmjopen-2019-030639
Jia, Q., Wang, J., He, N., He, J., and Zhu, B. (2019). Titin mutation associated with responsiveness to checkpoint blockades in solid tumors. JCI Insight 4 (10), e127901–e127940. doi:10.1172/jci.insight.127901
Jiang, P., Gu, S., Pan, D., Fu, J., Sahu, A., Hu, X., et al. (2018). Signatures of T cell dysfunction and exclusion predict cancer immunotherapy response. Nat. Med. 24 (10), 1550–1558. doi:10.1038/s41591-018-0136-1
Jonckheere, N., and Van Seuningen, I. (2018). Integrative analysis of the cancer genome atlas and cancer cell lines encyclopedia large-scale genomic databases: MUC4/MUC16/MUC20 signature is associated with poor survival in human carcinomas. J. Transl. Med. 16 (1), 259. doi:10.1186/s12967-018-1632-2
Kang, M., Choi, H., Oshima, M., Cheong, J., Kim, S., Lee, J. H., et al. (2018). Author Correction: Estrogen-related receptor gamma functions as a tumor suppressor in gastric cancer. Nat. Commun. 9 (1), 3599. doi:10.1038/s41467-018-06061-z
Kautto, E. A., Bonneville, R., Miya, J., Yu, L., Krook, M. A., Reeser, J. W., et al. (2017). Performance evaluation for rapid detection of pan-cancer microsatellite instability with MANTIS. Oncotarget 8 (5), 7452–7463. doi:10.18632/oncotarget.13918
Kim, K. J., Lee, T. H., Kim, J. H., Cho, N. Y., Kim, W. H., and Kang, G. H. (2017). Deletion in HSP110 T17: Correlation with wild-type HSP110 expression and prognostic significance in microsatellite-unstable advanced gastric cancers. Hum. Pathol. 67, 109–118. doi:10.1016/j.humpath.2017.08.001
Kumar, S. U., Kumar, D. T., Siva, R., Doss, C., and Zayed, H. (2019). Integrative bioinformatics approaches to map potential novel genes and pathways involved in ovarian cancer. Front. Bioeng. Biotechnol. 7, 391. doi:10.3389/fbioe.2019.00391
Li, L., Kang, D., Huang, Z., Zhan, Z., Feng, C., Zhou, Y., et al. (2019). Multimodal multiphoton imaging for label-free monitoring of early gastric cancer. BMC CANCER 19 (1), 295. doi:10.1186/s12885-019-5497-4
Li, X., Pasche, B., Zhang, W., and Chen, K. (2018). Association of MUC16 mutation with tumor mutation load and outcomes in patients with gastric cancer. JAMA Oncol. 4 (12), 1691–1698. doi:10.1001/jamaoncol.2018.2805
Lu, S., Zhang, Z., Chen, M., Li, C., Liu, L., and Li, Y. (2021). Erratum: Silibinin inhibits the migration and invasion of human gastric cancer SGC7901 cells by downregulating MMP-2 and MMP-9 expression via the p38MAPK signaling pathway. Oncol. Lett. 21 (5), 415. doi:10.3892/ol.2021.12676
Mao, M., Yu, Q., Huang, R., Lu, Y., Wang, Z., and Liao, L. (2020). Stromal score as a prognostic factor in primary gastric cancer and close association with tumor immune microenvironment. Cancer Med. 9 (14), 4980–4990. doi:10.1002/cam4.2801
Marchet, A., Mocellin, S., Ambrosi, A., de Manzoni, G., Di Leo, A., Marrelli, D., et al. (2008). The prognostic value of N-ratio in patients with gastric cancer: Validation in a large, multicenter series. Eur. J. Surg. Oncol. 34 (2), 159–165. doi:10.1016/j.ejso.2007.04.018
Mayakonda, A., Lin, D. C., Assenov, Y., Plass, C., and Koeffler, H. P. (2018). Maftools: Efficient and comprehensive analysis of somatic variants in cancer. GENOME Res. 28 (11), 1747–1756. doi:10.1101/gr.239244.118
Mimori, K., Fukagawa, T., Kosaka, Y., Ishikawa, K., Iwatsuki, M., Yokobori, T., et al. (2008). A large-scale study of MT1-MMP as a marker for isolated tumor cells in peripheral blood and bone marrow in gastric cancer cases. Ann. Surg. Oncol. 15 (10), 2934–2942. doi:10.1245/s10434-008-9916-z
Murphy, D. A., and Courtneidge, S. A. (2011). The 'ins' and 'outs' of podosomes and invadopodia: Characteristics, formation and function. Nat. Rev. Mol. Cell BIO 12 (7), 413–426. doi:10.1038/nrm3141
Nshizirungu, J. P., Bennis, S., Mellouki, I., Sekal, M., Benajah, D. A., Lahmidani, N., et al. (2021). Reproduction of the cancer genome atlas (TCGA) and asian cancer research group (ACRG) gastric cancer molecular classifications and their association with clinicopathological characteristics and overall survival in Moroccan patients. Dis. MARKERS 2021, 9980410. doi:10.1155/2021/9980410
Oh, S. C., Sohn, B. H., Cheong, J. H., Kim, S. B., Lee, J. E., Park, K. C., et al. (2018). Clinical and genomic landscape of gastric cancer with a mesenchymal phenotype. Nat. Commun. 9 (1), 1777. doi:10.1038/s41467-018-04179-8
Paul G, J. C. N., Stephanie, H. R., and Huang, R. S. (2014). Clinical drug response can be predicted using baseline gene expression levels and in vitro drug sensitivity in cell lines. GENOME Biol. 15 (3), R47. doi:10.1186/gb-2014-15-3-r47
Polom, K., Marano, L., Marrelli, D., De Luca, R., Roviello, G., Savelli, V., et al. (2018). Meta-analysis of microsatellite instability in relation to clinicopathological characteristics and overall survival in gastric cancer. Br. J. Surg. 105 (3), 159–167. doi:10.1002/bjs.10663
Qian, B. Z., and Pollard, J. W. (2010). Macrophage diversity enhances tumor progression and metastasis. Cell 141 (1), 39–51. doi:10.1016/j.cell.2010.03.014
Ritchie, M. E., Phipson, B., Wu, D., Hu, Y., Law, C. W., Shi, W., et al. (2015). Limma powers differential expression analyses for RNA-sequencing and microarray studies. NUCLEIC ACIDS Res. 43 (7), e47. doi:10.1093/nar/gkv007
Rybarczyk, P., Gautier, M., Hague, F., Dhennin-Duthille, I., Chatelain, D., Kerr-Conte, J., et al. (2012). Transient receptor potential melastatin-related 7 channel is overexpressed in human pancreatic ductal adenocarcinomas and regulates human pancreatic cancer cell migration. Int. J. CANCER 131 (6), E851–E861. doi:10.1002/ijc.27487
Scherer, R. L., McIntyre, J. O., and Matrisian, L. M. (2008). Imaging matrix metalloproteinases in cancer. CANCER METAST Rev. 27 (4), 679–690. doi:10.1007/s10555-008-9152-9
Solov'Eva, N. I., Timoshenko, O. S., Kugaevskaia, E. V., Andreeva, I., and Zavalishina, L. E. (2014) Key enzymes of degradation and angiogenesis as a factors of tumor progression in squamous cell carcinoma of the cervix. Bioorg Khim 40 (6), 743–751.
Streppel, M. M., Vincent, A., Mukherjee, R., Campbell, N. R., Chen, S. H., Konstantopoulos, K., et al. (2012). Mucin 16 (cancer antigen 125) expression in human tissues and cell lines and correlation with clinical outcome in adenocarcinomas of the pancreas, esophagus, stomach, and colon. Hum. Pathol. 43 (10), 1755–1763. doi:10.1016/j.humpath.2012.01.005
Subramanian, A., Tamayo, P., Mootha, V. K., Mukherjee, S., Ebert, B. L., Gillette, M. A., et al. (2005). Gene set enrichment analysis: A knowledge-based approach for interpreting genome-wide expression profiles. Proc. Natl. Acad. Sci. U. S. A. 102 (43), 15545–15550. doi:10.1073/pnas.0506580102
Wadhwa, R., Song, S., Lee, J. S., Yao, Y., Wei, Q., and Ajani, J. A. (2013). Gastric cancer-molecular and clinical dimensions. Nat. Rev. Clin. Oncol. 10 (11), 643–655. doi:10.1038/nrclinonc.2013.170
Wanebo, H. J., Kennedy, B. J., Chmiel, J., Steele, G. J., Winchester, D., and Osteen, R. (1993). Cancer of the stomach. A patient care study by the American College of Surgeons. Ann. Surg. 218 (5), 583–592. doi:10.1097/00000658-199321850-00002
Wang, H. L., Zhou, P. Y., Zhang, Y., and Liu, P. (2014). Relationships between abnormal MMP2 expression and prognosis in gastric cancer: A meta-analysis of cohort studies. Cancer Biother Radiopharm. 29 (4), 166–172. doi:10.1089/cbr.2014.1608
Wang, Y., Wu, H., Wu, X., Bian, Z., and Gao, Q. (2014). Interleukin 17A promotes gastric cancer invasiveness via NF-κB mediated matrix metalloproteinases 2 and 9 expression. PLOS ONE 9 (6), e96678. doi:10.1371/journal.pone.0096678
Wilkerson, M. D., and Hayes, D. N. (2010). ConsensusClusterPlus: A class discovery tool with confidence assessments and item tracking. BIOINFORMATICS 26 (12), 1572–1573. doi:10.1093/bioinformatics/btq170
Wu, H., Lin, W., and Tsai, K. (2014). Advances in molecular biomarkers for gastric cancer: miRNAs as emerging novel cancer markers. EXPERT Rev. Mol. Med. 16, e1. doi:10.1017/erm.2013.16
Yang, Y., Zhang, J., Chen, Y., Xu, R., Zhao, Q., Guo, W., et al. (2020). MUC4, MUC16, and TTN genes mutation correlated with prognosis, and predicted tumor mutation burden and immunotherapy efficacy in gastric cancer and pan-cancer. Clin. Transl. Med. 10 (4), e155. doi:10.1002/ctm2.155
Yoshihara, K., Shahmoradgoli, M., Martinez, E., Vegesna, R., Kim, H., Torres-Garcia, W., et al. (2013). Inferring tumour purity and stromal and immune cell admixture from expression data. Nat. Commun. 4, 2612. doi:10.1038/ncomms3612
Yu, P., Wang, Y., Yu, Y., Wang, A., Huang, L., Zhang, Y., et al. (2021). Deep targeted sequencing and its potential implication for cancer therapy in Chinese patients with gastric adenocarcinoma. ONCOLOGIST 26 (5), e756–e768. doi:10.1002/onco.13695
Zeng, D., Li, M., Zhou, R., Zhang, J., Sun, H., Shi, M., et al. (2019). Tumor microenvironment characterization in gastric cancer identifies prognostic and immunotherapeutically relevant gene signatures. CANCER Immunol. Res. 7 (5), 737–750. doi:10.1158/2326-6066.CIR-18-0436
Zeng, D., Zhou, R., Yu, Y., Luo, Y., Zhang, J., Sun, H., et al. (2018). Gene expression profiles for a prognostic immunoscore in gastric cancer. Br. J. Surg. 105 (10), 1338–1348. doi:10.1002/bjs.10871
Zhang, M., Fan, Y., Che, X., Hou, K., Zhang, C., Li, C., et al. (2020). 5-FU-Induced upregulation of exosomal PD-L1 causes immunosuppression in advanced gastric cancer patients. Front. Oncol. 10, 492. doi:10.3389/fonc.2020.00492
Zhang, X., Shi, M., Chen, T., and Zhang, B. (2020). Characterization of the immune cell infiltration landscape in head and neck squamous cell carcinoma to aid immunotherapy. Mol. Ther. Nucleic Acids 22, 298–309. doi:10.1016/j.omtn.2020.08.030
Zhou, M., Wang, H., Zhu, J., Chen, W., Wang, L., Liu, S., et al. (2016). Cause-specific mortality for 240 causes in China during 1990-2013: A systematic subnational analysis for the global burden of disease study 2013. LANCET 387 (10015), 251–272. doi:10.1016/S0140-6736(15)00551-6
Keywords: gastric cancer, MMP-matrix metalloproteinase, immunogenic profile, clinical diagnosis, immunotherapy, clinical treatment, genomics
Citation: Yuan C, Yuan J, Xiao H, Li H, Jiang Y, Zhai R, Zhai J, Xing H and Huang J (2023) Genomic analysis of matrix metalloproteinases affecting the prognosis and immunogenic profile of gastric cancer. Front. Genet. 14:1128088. doi: 10.3389/fgene.2023.1128088
Received: 20 December 2022; Accepted: 04 April 2023;
Published: 18 April 2023.
Edited by:
Jyoti Sharma, Institute of Bioinformatics (IOB), IndiaReviewed by:
Udhaya Kumar. S, Baylor College of Medicine, United StatesRajaguru Aradhya, Amrita Vishwa Vidyapeetham University, India
Copyright © 2023 Yuan, Yuan, Xiao, Li, Jiang, Zhai, Zhai, Xing and Huang. This is an open-access article distributed under the terms of the Creative Commons Attribution License (CC BY). The use, distribution or reproduction in other forums is permitted, provided the original author(s) and the copyright owner(s) are credited and that the original publication in this journal is cited, in accordance with accepted academic practice. No use, distribution or reproduction is permitted which does not comply with these terms.
*Correspondence: Hua Xing, eGluZ2hAamx1LmVkdS5jbg==; Jiannan Huang, aHVhbmdqbjE3QG1haWxzLmpsdS5lZHUuY24=
†These authors have contributed equally to this work and share first authorship