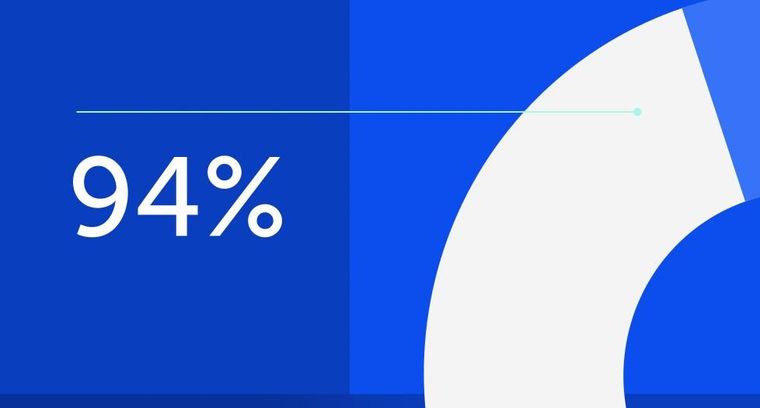
94% of researchers rate our articles as excellent or good
Learn more about the work of our research integrity team to safeguard the quality of each article we publish.
Find out more
ORIGINAL RESEARCH article
Front. Genet., 20 April 2023
Sec. Genetics of Common and Rare Diseases
Volume 14 - 2023 | https://doi.org/10.3389/fgene.2023.1122985
Introduction: Exome sequencing has a diagnostic yield ranging from 25% to 70% in rare diseases and regularly implicates genes in novel disorders. Retrospective data reanalysis has demonstrated strong efficacy in improving diagnosis, but poses organizational difficulties for clinical laboratories.
Patients and methods: We applied a reanalysis strategy based on intensive prospective bibliographic monitoring along with direct application of the GREP command-line tool (to “globally search for a regular expression and print matching lines”) in a large ES database. For 18 months, we submitted the same five keywords of interest [(intellectual disability, (neuro)developmental delay, and (neuro)developmental disorder)] to PubMed on a daily basis to identify recently published novel disease–gene associations or new phenotypes in genes already implicated in human pathology. We used the Linux GREP tool and an in-house script to collect all variants of these genes from our 5,459 exome database.
Results: After GREP queries and variant filtration, we identified 128 genes of interest and collected 56 candidate variants from 53 individuals. We confirmed causal diagnosis for 19/128 genes (15%) in 21 individuals and identified variants of unknown significance for 19/128 genes (15%) in 23 individuals. Altogether, GREP queries for only 128 genes over a period of 18 months permitted a causal diagnosis to be established in 21/2875 undiagnosed affected probands (0.7%).
Conclusion: The GREP query strategy is efficient and less tedious than complete periodic reanalysis. It is an interesting reanalysis strategy to improve diagnosis.
Exome sequencing (ES) is now used for routine diagnostic testing. ES has a diagnostic yield ranging from 25% to 70% (Stark et al., 2016; Tan et al., 2017; Clark et al., 2018), depending on the type of disorder, the presence of consanguinity, and the strategy used (solo or trio). Clinical laboratories have rapidly developed an attraction to the substantial benefits of data reanalysis, since ES regularly identifies causative variants in a large number of genes responsible for ultra-rare Mendelian disorders not yet associated to human disorders, particularly in highly heterogeneous diseases such as developmental disorders (DDs) or intellectual disability (ID) (Boycott et al., 2017; Wenger et al., 2017; Hartley et al., 2020).
The strategy of ES data reanalysis for undiagnosed individuals has demonstrated high efficiency. Complete retrospective reanalysis of clinical ES data after a defined period of time has elapsed, along with pipeline updates (especially to the OMIM and ClinVar databases), leads to an additional diagnostic yield ranging from 10.5% to 32% (Table 1) (Costain et al., 2018; Ewans et al., 2018; Nambot et al., 2018; Baker et al., 2019; Li et al., 2019; Salfati et al., 2019). A recent review of published reanalysis papers reported 10% diagnostic yield, but with considerable heterogeneity due to the delay in reanalysis (after or before 24 months), which is the limitation to OMIM-morbid genes or research extension (Dai et al., 2022). The major reasons for novel diagnosis are not only the ongoing discovery of novel genes involved in human diseases (Boycott et al., 2017) but also novel annotations of well-known OMIM-morbid genes extending their clinical phenotypes (Fokstuen et al., 2016). However, genes that are newly associated with human disorders are not instantly implemented in the commonly used public databases (OMIM, ClinVar, etc.) or in laboratory databases, which limits the power of reanalysis in the diagnostic setting. Diagnostic yield may indeed be found to increase significantly, from 30% to more than 40% in DD/ID, when reanalysis is extended to translational research involving data-sharing for candidate genes (Bruel et al., 2019). Because systematically performing complete reanalysis of ES data represents a significant challenge for clinical laboratories, a semi-automated reanalysis pipeline that interrogates various databases could facilitate efficient re-evaluation of undiagnosed individuals using up-to-date literature; this could be of significant value to clinical laboratories (Costain et al., 2018).
TABLE 1. Manuscripts focusing on a reanalysis strategy with diagnostic yield and delay. ES: exome sequencing; GS: genome sequencing; NR: not reported.
In addition to reanalysis of complete ES data, several targeted strategies can be applied on request. ES data can thus be interrogated using GREP, a command-line tool used to “globally search for a regular expression and print matching lines” that makes it possible to search for specific expressions in files (https://www.gnu.org/software/grep/manual/grep.html). By default, a GREP query will search for lines containing a given string pattern in a file or the standard input, but the query can be customized for enhanced specificity. The GREP command-line tool has been used in cancer applications to look for gene fusion or Alu insertion in unique patients or cohorts with similar disorders (Panagopoulos et al., 2014; Bujakowska et al., 2015; Panagopoulos et al., 2015). After identifying a balanced translocation t(10; 17) (q22; q21) following the use of cytogenetic techniques in an affected individual with retroperitoneal leiomyoma, Panagopoulos et al. (2015) identified a breakpoint in exon 3 of KAT6B, localized in the 10q22.2 region. A GREP query of this KAT6B exon sequence identified a unique chimeric sequence of 101 nucleotides composed of 43 nucleotides from this exon and 60 nucleotides from the KANSL1 gene, localized in the 17q21 region. A similar approach was applied to a large cohort following the identification by cytogenetic techniques of a balanced translocation t(4; 19) (q35; q13) in an affected individual with small round cell sarcoma. The same group identified a CIC–DUX4 fusion transcript that was not detected by several other algorithms designed to identify fusion (Panagopoulos et al., 2014). A GREP query on a known junction sequence of an Alu insertion in MAK was applied in a cohort of 1,847 samples (data from targeted sequencing or ES) of individuals with retinitis pigmentosa; this enabled the discovery of five affected individuals with the same GREP term (Bujakowska et al., 2015). In rare diseases, targeted GREP queries can be performed over ES or GS data on request and/or for specific purposes (Panagopoulos et al., 2014; Bujakowska et al., 2015; Panagopoulos et al., 2015). Nevertheless, intensive use of GREP query strategies for certain genes or variants has never been reported.
We present an innovative reanalysis strategy combining intensive prospective medical bibliographic monitoring with subsequent use of rapid GREP querying in a large ES database and international data-sharing to improve diagnostic yield and reduce diagnostic delay in individuals with DD/ID.
Since 2013, our clinical laboratory has performed ES in 5,459 individuals (4,170 probands and 1,289 affected or unaffected relatives), referred by several French university hospitals. Among the 4,170 probands, 3,771 were referred for DD and/or ID; 896/3,771 (23.7%) probands in this DD/ID group had a positive molecular diagnosis and 2875/3,771 (76.3%) had no causal diagnosis. Among the relatives, 98 belonged to a cutaneous mosaicism group, 23 to a cancer group, and 1,168 to the diverse DD/ID group. The ES methods employed have been previously reported (Tran Mau-Them et al., 2021).
All patients were informed of the continuous updating of their genomic data with regard to advances in knowledge and of the fact that they could be contacted again in the future in the event of the identification of new results useful for their health. All patients gave their informed consent for this procedure.
From April 2019 to October 2020, manual monitoring of medical bibliographies was performed every day by one medical biologist. The biologist searched the PubMed database for the following terms: intellectual disability, (neuro)developmental delay, and (neuro)developmental disorder. The PubMed search output was sorted using the “most recent” display option, and only the 10 articles displayed on the first page were considered. When an article described a novel gene–disease association or broadened a known gene–disease phenotype, we considered this article to be relevant. In addition, the gene name was searched on OMIM to look for any existing association with human disorders. Finally, the gene in question was searched in the ES database using the GREP command-line tool.
Use of the daily GREP query strategy started in April 2019, with a basic GREP query used to search for every rare variant (variant allele frequency <1%) of genes of interest in all the vcf files available (hg19). These vcf files are text files storing gene sequence variants with a mandatory minimum of eight columns and custom annotation. The custom annotation included general population frequency and occurrence [in the Exome Sequencing Project (ESP), Exome Aggregation Consortium (ExAC), Genome Aggregation Database (gnomAD) exome/genome, and a control sample count from our own database]; a batch sample count; several pathogenicity scores [Polyphen (Adzhubei et al., 2010), GERP (Cooper et al., 2005), Grantham (Grantham, 1974), CADD (Kircher et al., 2014), misZ, and pLI]; the OMIM pathology name, number, and mode of transmission; and lastly, the ClinVar classification. This basic GREP query performed by the biologist is referred to as v1 in the manuscript figures and tables. For example, the basic GREP query “grep -w GENE1 *.tsv > GENE1.tsv” searches for the exact expression “GENE1” in all files ending with “.tsv” and creates a new file “GENE1.tsv”. The -w option is included so that the query searches for whole-word matches for GENE1 and will not print lines such as GENE10, GENE11, etc.
With the increasing amount of ES data (novel or reanalysis) available, the number of lines printed increases accordingly, leading to hardly readable files. To improve this command-line tool and make the resulting file more biologist-friendly, in early 2020, we developed a bash script set up for our specific working environment, referred to as v2. The novel command-line script only requires the gene name as an argument, meaning that the biologist only has to type “GENE1” (example query: “getthatgene.bash GENE1”, compared to the more complex v1 GREP query. The output of the v2 GREP query was reformatted using a python3 script called by the bash script. The most important steps included 1) removal of exome reanalysis duplicates to retain only the most recently analyzed file, and 2) filling of gaps in the additional annotations with dots to produce an intelligible spreadsheet (Figure 1A). Three novel annotations were added during versioning of the database, namely, the and observed/expected with lower/upper threshold, Splicing Prediction Pipeline, (Leman et al., 2022) and the CCR (Havrilla et al., 2019) scores. Finally, in October 2020, we performed an end-point GREP query by searching again for all the genes previously searched with the daily GREP queries to see whether we could recover any missed diagnoses due to the time that had elapsed between the initial daily GREP queries and the end-point GREP query.
FIGURE 1. (A) Tabular files produced by a basic GREP query (top) and custom script query (bottom) for SUZ12. Note the absence of headers, the duplicate lines, and the disordered columns in the file resulting from the basic GREP query, which are corrected in the version output by the in-house script. (B) Workflow of the fast GREP query strategy.
A positive GREP query was defined by the identification of a candidate variant and a negative GREP query by its absence.
Although our ES analysis currently includes SNVs, CNVs, and mitochondrial variants from off-target sequences (Garret et al., 2019), only rare SNVs from nuclear genomes were searched for using these command line queries.
For all variants recovered using the GREP queries, interpretation was focused on the same variant type (missense variants in the same protein domain or protein-truncating variants) and mode of inheritance as reported in the manuscripts from PubMed. For the remaining candidate variants of interest, patient phenotypes were compared to the new disease–gene associations; only variants identified in the 3,771 individuals with DD/ID and their 1,168 relatives were considered. Candidate variants (i.e., those with compatible genotype–phenotype correlation) were confirmed using a second independent method (Sanger sequencing or quantitative PCR) and then shared via international collaborative platforms (GeneMatcher) (Sobreira et al., 2015) to strengthen the community’s knowledge of genotype–phenotype correlations since only one article had reported these new disease–gene associations in most cases. The workflow for the rapid GREP query strategy is summarized in Figure 1B.
Variant classification was based on the ACMG–AMP classification (Richards et al., 2015).
From April 2019 to October 2020, prospective medical bibliographic monitoring identified 128 genes implicated in DD/ID, mainly from publications in the American Journal of Human Genetics (30/128 genes), the Journal of Medical Genetics (10/128 genes), Brain (10/128 genes), Clinical Genetics (8/128 genes), and Genetics in Medicine (8/128 genes) (Figure 2; Supplementary Table S1). Among these 128 genes, 100 were associated with a novel human disorder (of which 37 were still not classified as morbid in the OMIM database and one was not reported in OMIM at all), nine had already been associated with a distinct human disorder, and 19 represented updates to the genotype/phenotype correlation of a known human disorder (Figure 3). The number of relevant manuscripts identified per week ranged from 0 to more than 5 (Figure 4A).
FIGURE 2. Number of positive and negative GREP queries arising from manuscripts published in various journals.
FIGURE 3. Number of genes resulting from positive and negative GREP queries with different levels of association with human disorders. Black indicates a GREP query run in 2019 and gray a query run in 2020. Evolution of the number of exomes in the database and number of genes queried with positive and variants of unknown significance patients.
FIGURE 4. (A) Number of weeks with the specified number of manuscripts resulting in a GREP query. (B) Delay in months between positive GREP query and diagnostic report. (C) Number of lines of output printed by GREP queries for each of the 128 genes, for daily queries using the v1 and v2 scripts and end-point GREP queries using the v2 script. Note the drastic difference between v1 and v2.
The time interval between a positive GREP query and delivery of the diagnostic report to the clinician ranged from 1 to 11 weeks (overall mean and SD: 4.2 ± 3.1; mean in 2019: 3.4 ± 2.2; mean in 2020: 4.5 ± 3.5; Figure 4B). For the daily GREP queries, the average time was 2.6 months (SD ± 1.8), compared with 6.6 months (SD ± 3.4) for the end-point GREP queries.
We also calculated the number of printed lines resulting from GREP queries using v1 and v2. Overall, the v1 script resulted in 52 genes and 82,156 lines of output (mean: 1,579 output lines/gene) and the v2 script resulted in in 76 genes and 11,953 lines of output (mean: 157 output lines/gene). This represented a drastic reduction (mean: 10-fold) in the number of output lines between v1 and v2. There was a slight increase in the number of lines of output between the two sets of v2 searches (mean difference: 1.1 times) due to the increase in the number of exomes in the database between the first v2 queries and the end-point GREP queries (Figure 4C). This use of the GREP query retrieved 36 genes in 2019 (starting from April) and 92 genes in 2020 (from January to October), for ES data covering a grand total of 5,459 individuals.
From the daily GREP queries, we obtained results of interest for 38 variants (29 premature stop codons and nine missenses) in 25/128 genes (20%) in 37 individuals (Figure 5). After Sanger validation and family segregation, 18 variants were classified as pathogenic or likely pathogenic (P/LP), 15 as variants of unknown significance (VUS), and four as benign or likely benign (B/LB). In three genes, we identified both P/LP variants and VUS (MAPK8IP3, SCAF4, and SUZ12), and in one gene, we identified P/LP, VUS, and B/LB variants (ZNF292) (Table 2).
FIGURE 5. Workflow and version of GREP query used according to the amount of ES data searched, with the number and classification of identified variants, genes, and individuals. A CSNK2B variant was first classified as of unknown significance but subsequently reclassified as likely pathogenic after a later publication. VUS: variant of unknown significance.
TABLE 2. Candidate variants identified using GREP queries, with classification after parental segregation. NA: not available; P/LP: pathogenic/likely pathogenic; VUS: variants of unknown significance; LB: likely benign.
All 18 P/LP variants in the 16 genes were identified in 18 previously undiagnosed DD/ID affected individuals, with diagnostic odysseys ranging from 6 months to 6 years. Thirteen of the 16 genes had not previously been reported to be involved in human disorders in the OMIM database and therefore were not annotated in the pipeline and had not been considered in the first diagnostic analysis. The other three genes were known to be involved in human disorders with an OMIM number, but with a different phenotype (KMT2D, MN1, and SETD1A). In one of the individuals, a patient with epileptic encephalopathy and a family history of long QT and with a previously identified KCNQ1 pathogenic variant, we also identified an HPDL causative variant [p.(Ala116Cysfs*81)], leading to a dual diagnosis. The GREP queries enabled the reclassification as likely pathogenic of two variants initially classified as VUS by the solo ES analysis (NR4A2 and TET3).
Fifteen variants (12 truncating and three missense) were classified as VUS, mainly because they were inherited from asymptomatic parents (10/15 individuals), a situation not described in the original manuscripts (Table 2). For 3/15 VUS cases, the parental segregation was not available (MAPK8IP3 and ZNF292). One of the 15 VUSs was secondarily reclassified as likely pathogenic after the publication of additional data 7 months after the initial GREP query (CSNK2B). One of the 15 VUSs was a de novo truncating variant in CUL3. Two VUSs were identified in individuals with previously identified causative variants: an inherited SCAF4 truncating variant [p.(Glu1071Glyfs*12)] in a fetus carrying a pathogenic IGF2 variant, and an inherited SYT1 missense variant [p.(Phe350Tyr)] in an individual carrying a SRCAP pathogenic variant. For 6/11 genes, international collaborations are ongoing to better characterize the genotype/phenotype of the affected individuals and could lead to reclassification of these variants (BAZ2B, CUL3, SCAF4, SUZ12, TAOK2, and ZNF292) as causative.
After the end-point GREP queries, we identified additional results of interest for 18 variants (10 missense, seven truncating, and one in-frame deletion) in 11 additional genes and four variants in genes with previous positive GREP query results, in 16 additional individuals (Figure 5; Table 2). After Sanger validation and family segregation, five variants were classified as P/LP (in three genes for three affected individuals), eight as VUS (in eight genes for eight affected individuals), and five as B/LB (in four genes for five affected individuals). The five P/LP variants identified the three genes had not been considered in the initial analysis for two reasons. First, the queries for SMPD4 and WDFY3 were carried out using the v1 script, probably leading to output that was too complicated for interpretation (1,259 variants in the v1 GREP query for SMPD4 and 1,433 for WDFY3). Second, the query for NUP188 was carried out in February 2020 using the v1 script, but the individual in question was only added to our exome database in August 2020, so the variants could not have been detected previously. They would also not have been detected in a diagnostic setting, since this gene was not implemented as an OMIM-morbid gene at this time.
After the end-point GREP queries, additional genes were found to harbor additional candidate variants, namely, TAOK1 (P/LP variants and VUS), CDC42BPB and TOMM70 (VUS and B/LB variants), and TET3 (P/LP and B/LB variants).
Among the five P/LP variants identified in the end-point GREP queries, four were identified in two previously undiagnosed individuals with DD/ID, with diagnostic odysseys ranging from 4 months (NUP188) to 3 years (SMPD4). In one of the three individuals with an overgrowth history and a previously identified pathogenic CNV in chromosome 16 (containing TAOK2), we also identified a WDFY3 causative variant [p.(Lys1705fs)], leading to a dual diagnosis. Eight variants (five missense and three truncating) identified in the end-point GREP queries were classified as VUS because they were inherited from asymptomatic parents in 6/8 individuals, which was not as described in the original manuscript. For two de novo variants, international collaborations are ongoing to better characterize the genotype/phenotype of the affected individuals and could lead to reclassification of these variants (BRD4 and TAOK1) as causative.
Altogether, we identified 56 variants in 36 different genes in 53 affected individuals by performing the daily and end-point GREP queries (Figure 5; Table 2). Moreover, all the VUSs could be considered likely pathogenic because they harbored the same type (protein truncating variant or missense located in the same domains) as in the relevant published manuscript.
This study is the first to present the feasibility and value of a reanalysis strategy combining intensive medical bibliographic monitoring with the use of a rapid GREP query applied to large ES data for the diagnosis of individuals with DD/ID. Altogether, this strategy identified 56 variants in 36 different genes in 53/3,771 affected probands (1.4%), including a causative variant in 21/53 (39.6%), rising to 44/53 (83%) when considering reclassified variants and VUS. Therefore, GREP queries for only 128 genes during a period of 18 months permitted a causal diagnosis to be established in 21/2,875 undiagnosed affected probands (0.7%). This yield is probably an underestimate because the initial cohort was heterogeneous and because the use of only a few keywords [ID, (neuro)developmental delay/disorder] will not have encompassed the full spectrum of the cohort (10% being unaffected by ID/DD). Moreover, with the majority of the candidate genes being responsible for ultra-rare diseases, this strategy would probably prove to be more effective if the number of undiagnosed affected individuals was much greater.
The novel diagnoses were mainly established in genes newly implicated in human diseases (32/36 genes). These genes had not been reported to be involved in human disorders in the OMIM database at the time of the initial clinical ES analysis and thus, could not be retained by the usual diagnostic analysis. Since these variants were present in the vcf files used in the initial ES analysis, a translational research analysis extended to non-OMIM (morbid) genes could focus on these candidate genes (Fokstuen et al., 2016). However, some variants appear to be very difficult to interpret, especially missense variants [39% of variants (22/56) identified in our GREP queries]. Moreover, all the VUSs could be considered likely pathogenic because they harbored the same type (protein truncating variant or missense located in the same domains) as in the relevant published manuscript. However, in most cases, with only one manuscript reporting these variants, additional observations of genotype–phenotype correlation are warranted to definitively implicate these genes and variants in human disorders. Most of these variants have been shared through various data-sharing systems, leading to ongoing international collaborations with the aim of further characterizing the genotype–phenotype correlation and/or conducting functional analysis. As expected, one category of variants remains easier to interpret in research analysis, i.e., truncating variants in genes with pLI > 0.9 or o/e < 0.3 (66% of variants identified in our GREP queries).
In a very small number of genes (4/36), variants were identified in well-known OMIM-morbid genes (CUL3, KMT2D, MN1, and SETD1A) but had not been initially considered in the first analysis because the phenotype of the referred individual and/or the mechanism of the variant was completely different from what was known. For instance, heterozygous CUL3 variants have been associated with pseudohypoaldosteronism type IIIE (MIM # 614496) due to an in-frame deletion of exon 9, and only recently have loss-of-function (LoF) variants been found to be involved in overall developmental delay (Nakashima et al., 2020). Heterozygous LoF variants in KMT2D are involved in Kabuki syndrome type 1 (MIM #147920), but specific heterozygous variants in exons 38 and 39 are likely to act in a dominant negative mechanism (Cuvertino et al., 2020). Fusion transcripts in MN1 are involved in meningioma (MIM # 607174), but specific truncating variants are thought to act in a dominant negative mechanism (Mak et al., 2020; Miyake et al., 2020). Missense variants outside protein domains in SETD1A, and of unclear effect, have been associated with early-onset epilepsy with or without ID (MIM #618832) (Yu et al., 2019), whereas LoF variants have been associated with a novel neurodevelopmental syndrome (MIM #611052) (Kummeling et al., 2021).
The major aspect of interest of this strategy remains the rapid translation from published results in PubMed to a diagnostic report, with a mean time between online publication in PubMed and diagnostic report of 4.2 months on average (SD ± 3.1). Indeed, the total number of genes found to be newly involved in human disorders represents a mean of 38 (31–49) genes per month (i.e., one gene per day) in 2019/2020 according to OMIM statistics (Boycott et al., 2017). However, the time that elapses between online publication in PubMed and OMIM indexing can be up to several months, which delays the annotation of these genes and hampers their interpretation in clinical routine practice. It also requires periodic pipeline updates with information from different databases (OMIM, ClinVar, HGMD, and denovoDB) and reanalysis to improve diagnosis. Despite their recognized utility (Nambot et al., 2018; Bruel et al., 2019), periodic reanalysis strategies in diagnostic and research settings may represent a significant challenge for clinical laboratories. These appear to be very time-consuming, with systematization being possible only if a sufficiently large translational research team is available to work in partnership with the clinical laboratory and physicians. A strategy combining intensive prospective bibliographic monitoring and targeted GREP queries appears to be a good compromise for the workload of clinical laboratories, especially since the usual diagnostic pipelines rely on updated data to aid in the interpretation of genes newly found to be involved in human disorders and/or flagged with pathogenic information in databases. In addition, this strategy enables faster diagnoses than periodic reanalysis. The time delay appears to be significantly reduced in the daily strategy, compared to published periodic reanalysis strategies, where it currently ranges from 6 to 18 months (Figure 1A) (Costain et al., 2018; Ewans et al., 2018; Nambot et al., 2018; Baker et al., 2019; Li et al., 2019; Salfati et al., 2019) or may be conducted on physicians’ request. Nevertheless, manuscript selection via intensive medical bibliographic monitoring could also be time-consuming for biologists. Ideally, this strategy should be combined with periodic reanalysis since we cannot guarantee that all novel implications and phenotypes have been investigated, despite thorough bibliographic monitoring.
To monitor relevant bibliographies, we periodically performed searches on PubMed using five relevant clinical keywords for DD/DI. Among the 128 genes retained in the results of the GREP queries, 66/128 (51.5%) were from articles published in five different journals in the field of human genetics (Am J Hum Genet; Brain; J Med Genet; Clin Genet; and Genet Med) (Supplementary Table S1). To improve the strategy, the choice of keywords for literature monitoring is essential. They must belong to the clinical area of the cohort studied. Indeed, our keywords were suitable for DD/DI but did not capture a wide range of genetic diseases and would not be suitable for other databases. Limiting their number or combining them would reduce the chances of identifying suitable candidate genes and therefore reduce the scope of the GREP query strategy. Increasing their number would probably lead to the collection of additional manuscripts to be read, which would be more time-consuming and therefore difficult to implement in routine diagnosis. An alternative could be to subscribe to RSS feeds from selected journals in the field of interest (Sobreira et al., 2015). This strategy requires the selection of appropriate journals and subsequent filtering of manuscripts of interest from these journals (Dubuque, 2011; Beller et al., 2018; Marshall and Wallace, 2019). Ultimately, the identification of manuscripts could be automated via a direct search in PubMed, which would facilitate the search and save time (Bohle, 2018). While retrieving manuscripts from appropriate journals is one component of the strategy, it must be acknowledged that systematic review of these manuscripts to identify the few manuscripts of interest is another. Indeed, identifying the name of a particular gene in a manuscript title does not imply that the article presents a novel gene–pathology association. To address this caveat, several text mining software tools have been developed, each with advantages and disadvantages (Rani et al., 2015; Van der Mierden et al., 2019).
In addition, updates to public and private databases could lead to update description of variants or changes in classification. Since a GREP query is performed on existing ES data, these variants, despite being detected, could be lacking crucial annotation for correct interpretation. The GREP query strategy only requires a priori knowledge of the genes of interest, since it is based on targeted querying of large-scale ES data. This GREP strategy also has great potential utility if collaborators send requests to one another regarding unpublished candidate genes in order to identify recurrences and establish phenotype–genotype correlations. For example, we identified strong candidate variants in one undiagnosed individual (namely, compound heterozygosity in DOHH, with molecular and clinical overlap) after a national collaborative call. However, whatever the mode of selection of the genes of interest, the effectiveness of the GREP strategy is all the greater when the disease is more heterogeneous, when the annual rate of identification of relevant new causal genes is high, and when large amounts of ES data are available. Accordingly, DD and ID are likely the best groups of rare diseases in which to apply this strategy. Nevertheless, efficient reanalysis strategies have also been reported in other rare disorders, such as sudden death, DD/ID, epilepsy, and Mendelian disorders (Costain et al., 2018; Ewans et al., 2018; Nambot et al., 2018; Baker et al., 2019; Li et al., 2019; Salfati et al., 2019).
Another advantage of the GREP query strategy is its ease of setup, since the GREP command-line tool is available in every Linux terminal. One disadvantage is the multiplicity of pipeline versions and/or reanalysis of ES data. Indeed, if different vcf files originating from the same affected individual, but with different versions of the pipeline (and most likely with additional annotations), are searched using a GREP query, then the output file resulting from the query will consist of multiples lines pertaining to the same variants (Figure 1B). Since vcf files can change in terms of the number of fields (due to the addition of novel in silico scores, databases, etc.), the duplicated lines for each variant will not present the same information; i.e., there will be missing information in some columns due to pipeline updates (Figure 1B). This issue is compounded when searches are carried out for multiple individuals who have benefited from different versions of the pipeline. Implementation of an in-house script can overcome this issue (Figure 1B), facilitating interpretation by decreasing variant redundancy in the results of the basic GREP query (with an 11-fold mean decrease between the basic and custom GREP query). However, even without a custom script, a basic GREP query can already provide files that are interpretable for biologists, and a GREP query remains easy to set up for routine use. The periodicity at which the GREP query was run (every day) was defined a priori in our study. While we could not define a mean time for the availability of manuscripts of interest in PubMed, the question of the periodicity of the PubMed inquiry is important, since a daily search will identify most of the relevant manuscripts but could be time-consuming. A monthly search would appear to be more practical in a diagnostic setting, with the drawback of the accumulation of manuscripts to either discard or retain. With this monthly periodicity, the OMIM update list could be used. However, this list is generally out of date: for example, the first new clinical synopses in January 2022 is based on a manuscript published in 2020 (a 2-year delay). In addition, the new entry list merely describes the relevant gene, without including the information that this gene is linked to a human disorder. Therefore, a clinician adopting this strategy would have to search every new entry to find out whether this is linked to a human disorder falling into their area of expertise.
Sometimes, several relevant manuscripts (up to four) are added to PubMed on the same day or on different days of the same week (up to seven manuscripts over 4 days of the same week), whereas sometimes no manuscripts are relevant for weeks (up to eight) at a time. Therefore, there is a fine line between setting a loose threshold that will miss some manuscripts and daily GREP querying that will lead to work overload. A compromise could be a one-off search capturing the most recent 20 articles or searches at two time points capturing 10 articles.
In conclusion, a reanalysis strategy combining intensive bibliographic monitoring and rapid GREP queries of a large ES database offers promising added value in increasing diagnostic yield and reducing diagnostic delay in rare diseases. Nevertheless, this strategy remains time-consuming, and automated bibliographic monitoring tools to pinpoint genes of interest will be welcomed, as these would lead to even faster diagnosis.
The data presented in the study are deposited in the https://www.ncbi.nlm.nih.gov/clinvar/?term=SUB12859036 repository, accession number SUB12859036.
The studies involving human participants were reviewed and approved by DC 2011-1332. Written informed consent to participate in this study was provided by the participants’ legal guardian/next of kin. Written informed consent was obtained from the minor(s)’ legal guardian/next of kin for the publication of any potentially identifiable images or data included in this article.
FT contributed to conceptualization, design, and writing. AO and YD wrote the Informatics script. A-LB, AP, AVi, AGo, AGu, AS, AVe, CR, CE, CC, CP, CD, DL, DG, EL, ET, HS, IM, JG, JTh, JD, JL, LPe, LL, LF, MT, MD, MW, MG, M-LJ, NH, RD, SN, SMe, SMo, VB, and YC performed data analysis and/or contributed to data acquisition. CT and YD contributed to the revision of the manuscript.
The authors thank the families for taking part in the study. The authors thank the University of Burgundy Centre de Calcul (CCuB) for technical support and management of the informatics platform, and the GeneMatcher platform for data-sharing. Several of the authors of this publication are members of the European Reference Network for Developmental Anomalies and Intellectual Disability (ERN-ITHACA; FTMT, ASDP, AV, LF). The authors thank the Free Software Foundation for the GREP software (Copyright © 1999–2002, 2005, 2008–2021 Free Software Foundation, Inc.). The authors also wish to thank Suzanne Rankin (Dijon Bourgogne University Hospital) for reviewing the English manuscript.
The authors declare that the research was conducted in the absence of any commercial or financial relationships that could be construed as a potential conflict of interest.
All claims expressed in this article are solely those of the authors and do not necessarily represent those of their affiliated organizations, or those of the publisher, the editors, and the reviewers. Any product that may be evaluated in this article, or claim that may be made by its manufacturer, is not guaranteed or endorsed by the publisher.
The Supplementary Material for this article can be found online at: https://www.frontiersin.org/articles/10.3389/fgene.2023.1122985/full#supplementary-material
Adzhubei, I. A., Schmidt, S., Peshkin, L., Ramensky, V. E., Gerasimova, A., Bork, P., et al. (2010). A method and server for predicting damaging missense mutations. Nat. Methods 7, 248–249. doi:10.1038/nmeth0410-248
Baker, S. W., Murrell, J. R., Nesbitt, A. I., Pechter, K. B., Balciuniene, J., Zhao, X., et al. (2019). Automated clinical exome reanalysis reveals novel diagnoses. J. Mol. Diagn 21, 38–48. doi:10.1016/j.jmoldx.2018.07.008
Beller, E., Clark, J., Tsafnat, G., Adams, C., Diehl, H., Lund, H., et al. (2018). Making progress with the automation of systematic reviews: Principles of the international collaboration for the automation of systematic reviews (ICASR). Syst. Rev. 7, 77. doi:10.1186/s13643-018-0740-7
Bohle, S. (2018). Plutchik": Artificial intelligence chatbot for searching NCBI databases. J. Med. Libr. Assoc. 106, 501–503. doi:10.5195/jmla.2018.500
Boycott, K. M., Rath, A., Chong, J. X., Hartley, T., Alkuraya, F. S., Baynam, G., et al. (2017). International cooperation to enable the diagnosis of all rare genetic diseases. Am. J. Hum. Genet. 100, 695–705. doi:10.1016/j.ajhg.2017.04.003
Bruel, A. L., Nambot, S., Quéré, V., Vitobello, A., Thevenon, J., Assoum, M., et al. (2019). Increased diagnostic and new genes identification outcome using research reanalysis of singleton exome sequencing. Eur. J. Hum. Gene 27, 1519–1531. doi:10.1038/s41431-019-0442-1
Bujakowska, K. M., White, J., Place, E., Consugar, M., and Comander, J. (2015). Efficient in silico identification of a Common insertion in the MAK gene which causes retinitis pigmentosa. PLoS One 10, e0142614. doi:10.1371/journal.pone.0142614
Clark, M. M., Stark, Z., Farnaes, L., Tan, T. Y., White, S. M., Dimmock, D., et al. (2018). Meta-analysis of the diagnostic and clinical utility of genome and exome sequencing and chromosomal microarray in children with suspected genetic diseases. NPJ Genom Med. 3, 16. doi:10.1038/s41525-018-0053-8
Cooper, G. M., Stone, E. A., Asimenos, G., Green, E. D., Batzoglou, S., et al. NISC Comparative Sequencing Program (2005). Distribution and intensity of constraint in mammalian genomic sequence. Genome Res. 15, 901–913. doi:10.1101/gr.3577405
Costain, G., Jobling, R., Walker, S., Reuter, M. S., Snell, M., Bowdin, S., et al. (2018). Periodic reanalysis of whole-genome sequencing data enhances the diagnostic advantage over standard clinical genetic testing. Eur. J. Hum. Genet. 26, 740–744. doi:10.1038/s41431-018-0114-6
Cuvertino, S., Hartill, V., Colyer, A., Garner, T., Nair, N., Al-Gazali, L., et al. (2020). A restricted spectrum of missense KMT2D variants cause a multiple malformations disorder distinct from Kabuki syndrome. Genet. Med. 22, 867–877. doi:10.1038/s41436-019-0743-3
Dai, P., Honda, A., Ewans, L., McGaughran, J., Burnett, L., Law, M., et al. (2022). Recommendations for next generation sequencing data reanalysis of unsolved cases with suspected mendelian disorders: A systematic review and meta-analysis. Genet. Med. 24, 1618–1629. doi:10.1016/j.gim.2022.04.021
Dubuque, E. M. (2011). Automating academic literature searches with RSS feeds and google Reader(™). Behav. Anal. Pract. 4, 63–69. doi:10.1007/BF03391776
Ewans, L. J., Schofield, D., Shrestha, R., Zhu, Y., Gayevskiy, V., Ying, K., et al. (2018). Whole-exome sequencing reanalysis at 12 months boosts diagnosis and is cost-effective when applied early in Mendelian disorders. Genet. Med. 20, 1564–1574. doi:10.1038/gim.2018.39
Fokstuen, S., Makrythanasis, P., Hammar, E., Guipponi, M., Ranza, E., Varvagiannis, K., et al. (2016). Experience of a multidisciplinary task force with exome sequencing for Mendelian disorders. Hum. Genomics 10, 24. doi:10.1186/s40246-016-0080-4
Garret, P., Bris, C., Procaccio, V., Amati-Bonneau, P., Vabres, P., Houcinat, N., et al. (2019). Deciphering exome sequencing data: Bringing mitochondrial DNA variants to light. Hum. Mutat. 40, 2430–2443. doi:10.1002/humu.23885
Grantham, R. (1974). Amino acid difference formula to help explain protein evolution. Science 185, 862–864. doi:10.1126/science.185.4154.862
Hartley, T., Lemire, G., Kernohan, K. D., Howley, H. E., Adams, D. R., and Boycott, K. M. (2020). New diagnostic approaches for undiagnosed rare genetic diseases. Annu. Rev. Genomics Hum. Genet. 21, 351–372. doi:10.1146/annurev-genom-083118-015345
Havrilla, J. M., Pedersen, B. S., Layer, R. M., and Quinlan, A. R. (2019). A map of constrained coding regions in the human genome. Nat. Genet. 51, 88–95. doi:10.1038/s41588-018-0294-6
Kircher, M., Witten, D. M., Jain, P., O'Roak, B. J., Cooper, G. M., and Shendure, J. (2014). A general framework for estimating the relative pathogenicity of human genetic variants. Nat. Genet. 46, 310–315. doi:10.1038/ng.2892
Kummeling, J., Stremmelaar, D. E., Raun, N., Reijnders, M. R. F., Willemsen, M. H., Ruiterkamp-Versteeg, M., et al. (2021). Characterization of SETD1A haploinsufficiency in humans and Drosophila defines a novel neurodevelopmental syndrome. Mol. Psychiatry 26, 2013–2024. doi:10.1038/s41380-020-0725-5
Leman, R., Parfait, B., Vidaud, D., Girodon, E., Pacot, L., Le Gac, G., et al. (2022). SPiP: Splicing Prediction Pipeline, a machine learning tool for massive detection of exonic and intronic variant effects on mRNA splicing. Hum. Mutat. 43, 2308–2323. doi:10.1002/humu.24491
Li, J., Gao, K., Yan, H., Xiangwei, W., Liu, N., Wang, T., et al. (2019). Reanalysis of whole exome sequencing data in patients with epilepsy and intellectual disability/mental retardation. Gene 700, 168–175. doi:10.1016/j.gene.2019.03.037
Mak, C. C. Y., Doherty, D., Lin, A. E., Vegas, N., Cho, M. T., Viot, G., et al. (2020). MN1 C-terminal truncation syndrome is a novel neurodevelopmental and craniofacial disorder with partial rhombencephalosynapsis. Brain 143, 55–68. doi:10.1093/brain/awz379
Marshall, I. J., and Wallace, B. C. (2019). Toward systematic review automation: A practical guide to using machine learning tools in research synthesis. Syst. Rev. 8, 163. doi:10.1186/s13643-019-1074-9
Miyake, N., Takahashi, H., Nakamura, K., Isidor, B., Hiraki, Y., Koshimizu, E., et al. (2020). Gain-of-Function MN1 truncation variants cause a recognizable syndrome with craniofacial and Brain abnormalities. Am. J. Hum. Genet. 106, 13–25. doi:10.1016/j.ajhg.2019.11.011
Nakashima, M., Kato, M., Matsukura, M., Kira, R., Ngu, L. H., Lichtenbelt, K. D., et al. (2020). De novo variants in CUL3 are associated with global developmental delays with or without infantile spasms. J. Hum. Genet. 65, 727–734. doi:10.1038/s10038-020-0758-2
Nambot, S., Thevenon, J., Kuentz, P., Duffourd, Y., Tisserant, E., Bruel, A. L., et al. (2018). Clinical whole-exome sequencing for the diagnosis of rare disorders with congenital anomalies and/or intellectual disability: Substantial interest of prospective annual reanalysis. Genet. Med. 20, 645–654. doi:10.1038/gim.2017.162
Panagopoulos, I., Gorunova, L., Bjerkehagen, B., and Heim, S. (2015). Novel KAT6B-KANSL1 fusion gene identified by RNA sequencing in retroperitoneal leiomyoma with t(10;17)(q22;q21). PLoS One 10, e0117010. doi:10.1371/journal.pone.0117010
Panagopoulos, I., Gorunova, L., Bjerkehagen, B., and Heim, S. (2014). The "grep" command but not FusionMap, FusionFinder or ChimeraScan captures the CIC-DUX4 fusion gene from whole transcriptome sequencing data on a small round cell tumor with t(4;19)(q35;q13). PLoS One 9, e99439. doi:10.1371/journal.pone.0099439
Rani, J., Shah, A. B., and Ramachandran, S. (2015). PubMed.mineR: an R package with text-mining algorithms to analyse PubMed abstracts. J. Biosci. 40, 671–682. doi:10.1007/s12038-015-9552-2
Richards, S., Aziz, N., Bale, S., Bick, D., Das, S., Gastier-Foster, J., et al. (2015). Standards and guidelines for the interpretation of sequence variants: A joint consensus recommendation of the American college of medical genetics and genomics and the association for molecular pathology. Genet. Med. 17, 405–424. doi:10.1038/gim.2015.30
Salfati, E. L., Spencer, E. G., Topol, S. E., Muse, E. D., Rueda, M., Lucas, J. R., et al. (2019). Re-analysis of whole-exome sequencing data uncovers novel diagnostic variants and improves molecular diagnostic yields for sudden death and idiopathic diseases. Genome Med. 11, 83. doi:10.1186/s13073-019-0702-2
Sobreira, N., Schiettecatte, F., Valle, D., and Hamosh, A. (2015). GeneMatcher: A matching tool for connecting investigators with an interest in the same gene. Hum. Mutat. 36, 928–930. doi:10.1002/humu.22844
Stark, Z., Tan, T. Y., Chong, B., Brett, G. R., Yap, P., Walsh, M., et al. (2016). A prospective evaluation of whole-exome sequencing as a first-tier molecular test in infants with suspected monogenic disorders. Genet. Med. 18, 1090–1096. doi:10.1038/gim.2016.1
Tan, T. Y., Dillon, O. J., Stark, Z., Schofield, D., Alam, K., Shrestha, R., et al. (2017). Diagnostic impact and cost effectiveness of whole-exome sequencing for ambulant children with suspected monogenic conditions. JAMA Pediatr. 171, 855–862. doi:10.1001/jamapediatrics.2017.1755
Tran Mau-Them, F., Duffourd, Y., Vitobello, A., Bruel, A. L., Denommé-Pichon, A. S., Nambot, S., et al. (2021). Interest of exome sequencing trio-like strategy based on pooled parental DNA for diagnosis and translational research in rare diseases. Mol. Genet. Genomic Med. 9, e1836. doi:10.1002/mgg3.1836
Van der Mierden, S., Tsaioun, K., Bleich, A., and Leenaars, C. H. C. (2019). Software tools for literature screening in systematic reviews in biomedical research. ALTEX 36, 508–517. doi:10.14573/altex.1902131
Wenger, A. M., Guturu, H., Bernstein, J. A., and Bejerano, G. (2017). Systematic reanalysis of clinical exome data yields additional diagnoses: Implications for providers. Genet. Med. 19, 209–214. doi:10.1038/gim.2016.88
Keywords: GREP, intellectual disability, developmental anomalies, genomic database, diagnostic improvement, exome sequencing (ES), data reanalysis
Citation: Tran Mau-Them F, Overs A, Bruel A-L, Duquet R, Thareau M, Denommé-Pichon A-S, Vitobello A, Sorlin A, Safraou H, Nambot S, Delanne J, Moutton S, Racine C, Engel C, De Giraud d’Agay M, Lehalle D, Goldenberg A, Willems M, Coubes C, Genevieve D, Verloes A, Capri Y, Perrin L, Jacquemont M-L, Lambert L, Lacaze E, Thevenon J, Hana N, Van-Gils J, Dubucs C, Bizaoui V, Gerard-Blanluet M, Lespinasse J, Mercier S, Guerrot A-M, Maystadt I, Tisserant E, Faivre L, Philippe C, Duffourd Y and Thauvin-Robinet C (2023) Combining globally search for a regular expression and print matching lines with bibliographic monitoring of genomic database improves diagnosis. Front. Genet. 14:1122985. doi: 10.3389/fgene.2023.1122985
Received: 13 December 2022; Accepted: 13 February 2023;
Published: 20 April 2023.
Edited by:
Mahmood Rasool, King Abdulaziz University, Saudi ArabiaReviewed by:
Ammar Husami, Cincinnati Children’s Hospital Medical Center, United StatesCopyright © 2023 Tran Mau-Them, Overs, Bruel, Duquet, Thareau, Denommé-Pichon, Vitobello, Sorlin, Safraou, Nambot, Delanne, Moutton, Racine, Engel, De Giraud d’Agay, Lehalle, Goldenberg, Willems, Coubes, Genevieve, Verloes, Capri, Perrin, Jacquemont, Lambert, Lacaze, Thevenon, Hana, Van-Gils, Dubucs, Bizaoui, Gerard-Blanluet, Lespinasse, Mercier, Guerrot, Maystadt, Tisserant, Faivre, Philippe, Duffourd and Thauvin-Robinet. This is an open-access article distributed under the terms of the Creative Commons Attribution License (CC BY). The use, distribution or reproduction in other forums is permitted, provided the original author(s) and the copyright owner(s) are credited and that the original publication in this journal is cited, in accordance with accepted academic practice. No use, distribution or reproduction is permitted which does not comply with these terms.
*Correspondence: Frédéric Tran Mau-Them, ZnJlZGVyaWMudHJhbi1tYXUtdGhlbUB1LWJvdXJnb2duZS5mcg==
Disclaimer: All claims expressed in this article are solely those of the authors and do not necessarily represent those of their affiliated organizations, or those of the publisher, the editors and the reviewers. Any product that may be evaluated in this article or claim that may be made by its manufacturer is not guaranteed or endorsed by the publisher.
Research integrity at Frontiers
Learn more about the work of our research integrity team to safeguard the quality of each article we publish.