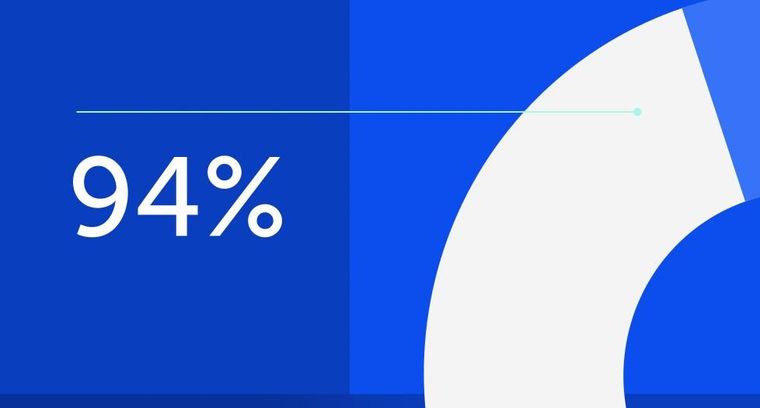
94% of researchers rate our articles as excellent or good
Learn more about the work of our research integrity team to safeguard the quality of each article we publish.
Find out more
ORIGINAL RESEARCH article
Front. Genet., 05 April 2023
Sec. Immunogenetics
Volume 14 - 2023 | https://doi.org/10.3389/fgene.2023.1119017
Background: Anti-neutrophil cytoplasmic antibody (ANCA)-associated vasculitis (AAV) is a systemic autoimmune disease that may lead to end-stage renal disease. However, few specifific biomarkers are available for AAV-related renal injury. The aim of this study was to identify important biomarkers and explore new immune subtypes of AAV-related renal injury.
Methods: In this study, messenger RNA expression profiles for antibody-associated vasculitis and AAV-associated kidney injury were downloaded from the Gene Expression Omnibus database. Weighted gene co-expression network analysis (WGCNA) was performed to identify the most relevant module genes to AAV. Key module genes from WGCNA were then intersected with AAV- and nephropathy-related genes from the Genecards database to identify key genes for AAV-associated kidney injury. Subsequently, the expression of key genes was validated in independent datasets and the correlation of genes with clinical traits of kidney injury was verified by the Nephroseq database. Finally, non-negative matrix factorization (NMF) clustering was performed to identify the immune subtypes associated with the key genes.
Results: Eight co-key genes (AGTR2, ANPTL2, BDKRB1, CSF2, FGA, IL1RAPL2, PCDH11Y, and PGR) were identifified, and validated the expression levels independent datasets. Receiver operating characteristic curve analysis revealed that these eight genes have major diagnostic value as potential biomarkers of AAV-related renal injury. Through our comprehensive gene enrichment analyses, we found that they are associated with immune-related pathways. NMF clustering of key genes identified two and three immune-related molecular subtypes in the glomerular and tubular data, respectively. A correlation analysis with prognostic data from the Nephroseq database indicated that the expression of co-key genes was positively co-related with the glomerular filtration rate.
Discussion: Altogether, we identifified 8 valuable biomarkers that firmly correlate with the diagnosis and prognosis of AAV-related renal injury. These markers may help identify new immune subtypes for AAV-related renal injury.
Antineutrophil cytoplasmic antibody (ANCA)-associated vasculitis (AAV) is a group of systemic autoimmune diseases typified by inflammation, the destruction of small- and medium-sized blood vessels, and the presence of circulating ANCAs. Primarily, AAV includes microscopic polyangiitis (MPA), granulomatosis with polyangiitis (GPA), and eosinophilic granulomatosis with polyangiitis. AAV usually causes damage to multiple organs at the same time, with the lungs and kidneys being most commonly affected and patients typically presenting with pulmonary hemorrhage and acute kidney injury. Patients with untreated AAV experience rapid progression of their disease, and the cause of death is primarily renal or respiratory failure (Cornec et al., 2016). The occurrence and progression of AAV correlate with multiple factors from the point of view of science and research, such as genetic susceptibility, cellular and environmental factors, and infection (Lee et al., 2012; Lyons et al., 2019). Genes and genetics are involved in the development of AAV autoimmunogenesis (Jennette et al., 2013; Jennette and Falk, 2014a; Kallenberg, 2014a; Jennette and Falk, 2014b). In recent years, immunosuppressive therapy has significantly improved the prognosis of patients with AAV (Kallenberg, 2014b), but early detection of AAV, evaluation of the treatment response, and outcome prediction are still considered challenging.
AAV-related renal injury mainly manifests as symptoms of hematuria, proteinuria, and abnormal renal function, and generally results in a rapid deterioration of renal function. Several studies have shown that severe AAV carries a high mortality rate, approaching 80% in untreated patients (Shi, 2017). The 5-year survival rates for patients with GPA and MPA, respectively, are 74%–91% and 45%–76% (Robson et al., 2015). A study of children with AAV in China found that AAV occurs most commonly in adolescent girls. Renal impairment was the most common symptom, with 58.8% of patients progressing to end-stage renal disease within a median period of 3 months (Wu et al., 2021a). It is clear that renal involvement is potentially life-threatening for patients, and exploring the molecular mechanisms underlying the onset and progression of AAV-related kidney injury and identifying new therapeutic targets could contribute to the early diagnosis and treatment of this disease and improve the prognosis.
More recently, high-throughput sequencing technologies have generated massive bioinformatics data, facilitating access to more accurate gene-expression profiles, the identification of disease-related genes and drug targets, and the analysis of the pathogenesis of complex diseases. Bioinformatics analysis has significant advantages for understanding the pathophysiological mechanisms of hereditary AAV. Numerous bioinformatics datasets in the Gene Expression Omnibus (GEO) database have been analyzed to uncover the pathogenesis of AAV. However, there are still relatively few bioinformatics studies of biomarkers and key pathways for AAV-related kidney injury.
In this study, we used bioinformatics approaches to screen for key genes participating in AAV-related kidney injury from multiple AAV dataset sources. In addition, using Gene Ontology (GO)/Kyoto Encyclopedia of Genes and Genomes (KEGG) and gene set enrichment analysis (GSEA) pathway analyses, the signaling pathways in which key genes were significantly enriched were clustered. Non-negative matrix factorization (NMF) and single-sample GSEA (ssGSEA) were performed to explore new subtypes of AAV-related kidney injury and immune infiltration. This study aimed to identify key genes and pathways to explore new subtypes of AAV-related kidney injury and provide potential candidate biomarkers for diagnosis, survival prediction, and drug targets for AAV-related kidney injury.
We searched the GEO database (https://www.ncbi.nlm.nih.gov/geo/) (Barrett et al., 2013) for human AAV-, ANCA-associated glomerulonephritis (AAGN), and ANCA-associated tubulointerstitial nephritis (AATN)- related gene profiles using the keywords “ANCA,” “AAV,” “AAGN,” and “AATN.” Data from 30 AAV patients in the GSE129752 dataset (Gill et al., 2021) based on the GPL10999 platform (Illumina Genome Analyzer IIx, Homo sapiens) were used for subsequent analysis.The GSE158163 dataset (Grayson et al., 2018) is based on the GPL16791 platform (Illumina HiSeq 2500, H. sapiens), from which we extracted data from 16 control samples (virus negative and positive control groups) for subsequent analysis. The GSE104948 dataset is based on the GPL22945 platform (Affymetrix Human Genome U133 Plus 2.0 Array) and the GPL24120 platform (Affymetrix Human Genome U133A Array), and we extracted glomerular expression data from 21 normal and 22 AAGN patient samples. The GSE104954 dataset (Grayson et al., 2018) was also based on the GPL22945 platform and the GPL24120 platform, and we extracted renal tubular expression data from 21 control and 21 AATN patients (Figure 1).
FIGURE 1. Study workflow. Anti-neutrophil cytoplasmic antibody–associated vasculitis (AAV), weighted gene co-expression network analysis (WGCNA), gene set enrichment analysis (GSEA), Gene Ontology (GO), Kyoto Encyclopedia of Genes and Genomes (KEGG), and non-negative matrix factorization (NMF).
For the RNA sequencing data, the sequencing count values of the GSE129752 and GSE158163 datasets were downloaded and converted to Fragments Per Kilobase of exon model per Million mapped fragments (FPKM) values using the biomaRt package in R. Since the optimal input matrix type for WGCNA was FPKM, the FPKM values were no longer converted to TPM values. Next, the FPKM matrices of the two datasets were subjected to missing value completion and data cleaning. We removed rows (genes) with NA values greater than 50% and columns (samples) with NA values greater than 50%, and furthermore, we used the impute.knn function of the R package impute to perform missing value completion by setting the Number of neighbors to K to complete the missing data. The expression values were taken as log2 (Exp+1) and the mean value of the duplicated genes was taken as their expression. We then combined the two datasets by limma and removed the batch effect, and analyzed the data distribution before and after combining the two datasets by principal component analysis (PCA). The anosim function in the vegan package of the R language was used to calculate the P and R values. For the microarray data, we used Perl scripts to preprocess the raw matrices of GSE104948 and GSE104954. Probe identification numbers were converted to gene symbols and empty probes were removed based on the annotation information contained in each platform file. When multiple probes were matched to the same gene, the average expression value was used to determine the expression level of the gene. If there is a large variation in the expression of a gene, log (Exp+1) is used to measure the expression level.
The “WGCNA” R package (Langfelder and Horvath, 2008) was used to identify the key modules most closely related to AAV in the matrix of merged GSE129752 and GSE158163 expression profiles. We extracted the AAV group’s pediatric vasculitis activity score (PVAS) scores from the original dataset as input data for WGCNA. A sample clustering dendrogram was constructed using the cluster function, and the topological overlap matrix (TOM) was constructed using the pickSoftThreshold function by WGCNA to determine the optimal soft threshold. We used candidate power values (1–20) to determine the average connectivity and independence of various modules. Dynamic shear trees were used to identify gene modules. Next, we measured the association between modules and sample traits using gene significance (GS) values and module membership (MM) values, and key modules were identified. We set |GS| to >0.3 and |MM| to >0.7 to filter hub genes in accordance with the official WGCNA guidelines and prior application examples so as to obtain the genes most relevant to the traits in the key module (Langfelder and Horvath, 2008; Tang et al., 2018). We performed GSEA (Wu et al., 2021b) using the KEGG and REACTOME gene sets in the GSEA dataset [c2.cp.v7.2.symbols.gmt (curated)]. A false discovery rate of <0.25 and p.adjust <0.05 (Subramanian et al., 2005) were set as the screening criteria for enrichment pathways.
The module hub genes of WGCNA were intersected with the AAV-related key genes and nephropathy-related key genes in the Genecards database (Supplementary Table S1) to identify co-key genes of AAV. The “bioconductor” package “org.Hs.e.g.,.db” (Carlson, 2022) and “clusterProfiler” package were installed in R (R Foundation for Statistical Computing, Vienna, Austria), which was used for GO (Ashburner et al., 2000) analysis and KEGG (Kanehisa and Goto, 2000) pathway enrichment analysis while adopting p < 0.05 and p.adjust <0.05 as criteria to screen the co-key genes.
The expression levels of co-key genes in the glomerular dataset GSE104948 and the tubular dataset GSE104954 were identified. We extracted the gene expression information after preprocessing the data and performed the Wilcoxon rank sum test. Then, we used the “PRROC” (Gatto et al., 2014) package in R to examine the diagnostic efficacy of co-key genes by performing receiver operating characteristic (ROC) curve analysis.
NMF (Gaujoux and Seoighe, 2010) clustering was performed on the eight co-key genes in the GSE104948 and GSE104954 datasets to identify potential features of interest in the gene-expression profiles. The distinct molecular subtypes of AAV-related renal injury were identified based on a cophenetic and consensus matrix. These distinct molecular subtypes were systematically assessed by ssGSEA analysis using the “GSVA” (Hänzelmann et al., 2013) R package to explore the immune infiltration and mechanisms of different subtypes of AAV-related renal injury.
The Nephroseq database (http://v5.nephroseq.org/) (Zheng et al., 2017) is an online, free public platform. A correlation analysis of co-key genes and clinical features using the Nephroseq database was performed to evaluate the clinical prognostic value of key genes for AAV-related renal injury.
The WGCNA package was performed to identify the key modules most closely related to AAV in the matrix of merged GSE129752 and GSE158163 expression profiles (which contained a total of 21,113 genes). Differences in the integrated dataset after removal of batch effects were mainly driven by intrinsic heterogeneity between the Disease and Control groups (Supplementary Figure S1). The control and AAV sets in WGCNA were automatically clustered into different branches (Figure 2A). The merged shear height of the dynamic shear tree was 0.85 for module identification. The minimum number of genes in each network module was set to 50, resulting in a total of 21 gene modules (Figure 2B). The most strongly correlated positive and negative modules were chosen as critical modules for discerning the pathogenesis of AAV traits. Our results indicate that the brown module (containing a total of 7,290 genes) was significantly negatively correlated with the AAV trait (cor = −0.29, p = 0.05) (Figure 2C). By setting |GS| > 0.3 and |MM| > 0.7 as criteria to screen the critical module genes for the pathogenesis of the AAV traits, we identified 462 genes associated with AAV pathogenesis (Figure 2D).
FIGURE 2. Weighted gene co-expression network analysis using GSE129752 and GSE111459. (A) Sample-trait clustering heatmap. (B) Dynamic shearing tree merging similar module genes. (C) Module-trait correlation heatmap. (D) Scatterplot for correlation between gene module membership in the brown module and gene significance.
We performed a comprehensive GSEA analysis of the screened brown module genes to discover the function and pathways of the key modules and explore the pathogenesis of AAV. The results showed (Figure 3A) that module genes were significantly enriched in the following immune- and inflammation-related pathways: antigen processing and presentation, autoimmune thyroid disease, immunomodulatory interactions, natural killer cell–mediated cytotoxicity, MAPK signaling pathways, and Leishmania protozoa infection. It has been suggested that immune, infection, and cellular responses are critical for the progression of AAV-related renal injury, which was consistent with the existing knowledge about AAV, and the accuracy of the module genes was also verified.
FIGURE 3. Gene set enrichment analysis (GSEA) enrichment analysis and validation of co–key genes in independent datasets. (A) GSEA enrichment results. (B) Venn diagram screening for co-key genes. (C, E) Expression of co-key genes in the GSE104948 and GSE104954 datasets for kidney injury in anti-neutrophil cytoplasmic antibody–associated vasculitis (AAV) (*p < 0.05, **p < 0.01, ***p < 0.001; ns, not statistically significant). (D, F) Receiver operating characteristic curves of eight key genes specific to kidney injury in AAV in GSE104948 and GSE104954.
The WGCNA module hub genes were then intersected with the AAV-related key genes and nephropathy-related key genes in the Genecards database to identify key genes of AAV-related renal injury. We identified a total of 11 co-key genes, including PGR, AGTR2, BDKRB1, FGA, 1L1RAPL2, ANGPTL2, PCDH11Y, CSF2, ADAMTSL1, MIRLET7E, and SAA4 (Figure 3B); then, we validated the expression levels of these co-key genes in the ANCA glomerular dataset GSE104948 and the ANCA tubular dataset GSE104954 to verify that they have similar expression profiles in independent datasets. Since the expression profiles of MIRLET7E, ADAMTSL1 and SAA4 were missing in the independent dataset, we only validated the expression profiles of the remaining eight genes. Our results indicated that the expression levels of PGR, AGTR2, BDKRB1, FGA, ANGPTL2, and PCDH11Y in the GSE104948 expression profile data were not significantly different between the control and AAGN groups (Figure 3B). However, the expression levels of 1L1RAPL2 (p < 0.001) and CSF2 (p < 0.05) were significantly lower in the AAGN group than in the control group (Figure 3C).
In the tubular dataset GSE104954, the expression levels of PGR, BDKRB1, FGA, 1L1RAPL2, ANGPTL2, and CSF2 were significantly downregulated in patients with AATN (Figure 3E). We examined the diagnostic sensitivity and specificity of the eight hub genes by performing ROC curve analysis. In the glomerular dataset GSE104948, the area under the ROC curve (AUC) values for 1L1RAPL2 and CSF2 were both >0.7, suggesting that these genes have good diagnostic efficacy as AAGN markers, with the larger AUC value being 0.816 (1L1RAPL2) (Figure 3D). In the tubular dataset GSE104954, as shown in Figure 3F, the AUC values of all six genes were >0.7 (PGR: 0.850, BDKRB1: 0.706, FGA: 0.796, 1L1RAPL2: 0.808, ANGPTL2: 0.780, and CSF2: 0.730), suggesting that these six genes could be AATN biomarkers with high specificity. These genes have good diagnostic sensitivity and specificity for AAV-related renal injury.
The biological functions and key signaling pathways of the eight candidate key genes were analyzed using GO and KEGG enrichment analyses. Our results showed that the co-key genes were mainly enriched for GO entries, including G protein–coupled peptide receptor activity, transcription factor activity, chemoattractant activity, receptor antagonist activity, endoplasmic reticulum lumen, platelet alpha granule lumen, extrinsic apoptotic signaling pathway, hormone secretion, vascular processes in the circulatory system, heterotypic cell–cell adhesion regulation, and other processes (Figure 4A). The KEGG pathways involved those designated for complement and coagulation cascades, the renin–angiotensin system, neuroactive ligand–receptor interaction, acute myeloid leukemia, the Fc epsilon RI signaling pathway, and others (Figure 4B).
FIGURE 4. Gene enrichment analysis of co–key genes for AAV related renal injury (A) Gene Ontology enrichment analysis of co-key genes. (B) Kyoto Encyclopedia of Genes and Genomes enrichment analysis of co-key genes.
To further explore whether the identified co-key genes are meaningful across renal injury subtypes, we performed NMF clustering with the eight co-key genes for molecular subtype identification using the glomerular dataset GSE104948 and the tubular dataset GSE104954. In the glomerular dataset GSE104948, a sharp decrease in the cophenetic correlation coefficient at rank r = 2 indicated that the co-key genes could classify glomerular disease in AAV-related kidney injury into a pair of subgroups (Figures 5A, C). Meanwhile, in the tubular dataset GSE104954, the highest cophenetic correlation coefficient at rank r = 3 suggested that the co-key genes could classify renal tubular disease in AAV-related kidney injury into three subgroups (Figures 5B, D). Importantly, these findings may offer a novel approach to the classification of AAV-related kidney injury. Next, we further investigated the immune infiltration among these five subtypes. The results of ssGSEA showed that, between both subtypes of AAGN, subtype 1 had a higher level of infiltration by effector memory CD4 T-cells and memory B-cells than subtype 2, suggesting that each cell type has a crucial role in the progression of subtype 1 AAGN renal injury (Figure 5E). Separately, the distribution of the immune infiltration of 28 immune cells in the three subtypes of AATN is shown in Supplementary Figure S2; notably, CD56dim natural killer infiltration in subtype 1 of AATN was significant (p = 0.048), and neutrophil cell infiltration in subtype 2 was significant (p = 0.0097), while memory B-cell infiltration was significant in subtype 3 (p = 0.0024). This indicated that these three cell types have a significant effect on the progression of the three subtypes of AATN.
FIGURE 5. Non-negative matrix factorization clustering with eight co–key genes or molecular subtype identification in the glomerular dataset GSE104948 and the tubular dataset GSE104954. (A, C) The sharp decrease in the cophenetic correlation coefficient at rank r = 2 indicates that co-key genes could classify glomerular disease in anti-neutrophil cytoplasmic antibody–associated vasculitis (AAV)-related kidney injury into two subgroups. (B, D) In the tubular dataset GSE104954, the highest cophenetic correlation coefficient at rank r = 3 suggested that the co-key genes could classify renal tubular disease in AAV-related kidney injury into three subgroups. (E). Immune cell infiltration of AAV-glomerular injury molecular subtypes. Immune infiltration analysis of molecular subtypes endows subtypes with immunological significance to the disease. High expression of both subtypes in a particular immune cell may suggest that the immune cell was essential to disease development The statistical differences obtained by comparing one subtypes with another subtype imply that a certain subtype plays a major role in that single immune cell.
In summary, we determined that AGTR2, ANGPTL2, BDKRB1, CSF2, FGA, IL1RAPL2, PCDH11Y, and PGR are key genes in patients with AAV-related kidney injury, with diagnostic sensitivity and specificity. We further validated the relationship between co-key genes and clinical traits in the Nephroseq database to assess the association between co-key genes and prognostic factors of AAV-related kidney injury. Low expression of co-key genes was associated with a low glomerular filtration rate (BDKRB1: r = 0.338, p < 0.001; PCDH11Y: r = 0.287, p < 0.001; IL1RAPL2: r = 0.243, p < 0.001) (Figures 6A–H), suggesting that the expression of these genes may participate in the process of AAV-related kidney injury.But it is not clear whether its correlation can predict its poor prognosis, which requires further experiments and large clinical cohorts for validation.
FIGURE 6. Association of co–key genes and clinical features in the Nephroseq database. (A–H) Correlation of eight co-key genes with the glomerular filtration rates of patients.
We identified 11 key genes of AAV-related renal injury. Eight of these genes were validated in independent datasets. These eight genes have good diagnostic efficacy suggests that they can serve as novel biomarkers and provide a new way to classify AAV-related renal injury. In addition, a comprehensive functional and pathway enrichment approach revealed that the biological mechanisms mediating the development of AAV-related renal injury are interrelated. Currently, there is a lack of effective, non-invasive biomarkers for AAV-related kidney injury; therefore, further exploration of the underlying mechanisms of the biological processes responsible for AAV-related kidney injury would contribute to the early diagnosis and treatment of the disease and improve the prognosis. The co-expressed genes identified in this study by integrating multiple approaches may be critical for elucidating the pathogenesis of AAV-related kidney injury.
A total of 462 key genes of AAV-related renal injury were screened using WGCNA. Multi-dataset integration analysis identified and validated eight co-key genes. GSEA showed that the key genes were significantly enriched in immune- and inflammation-related pathways. The key genes identified herein were those mainly enriched for GO entries, including G protein–coupled peptide receptor activity, transcription factor activity, chemoattractant activity, and other processes. The KEGG pathways of interest included those indicated for complement and coagulation cascades, the renin–angiotensin system, and others. The complement system plays a vital role in the development of AAV (Jennette and Falk, 2014a; Chen et al., 2017). Through bioinformatics techniques, we found that AGTR2, ANGPTL2, BDKRB1, CSF2, FGA, IL1RAPL2, PCDH11Y, and PGR are differently expressed in AAV patients and healthy people. In the validation of the independent microarray dataset, our results showed that 1L1RAPL2 and CSF2 expressed significantly lower levels in the GSE104948 expression profile data than the control group. In the tubular dataset GSE104954, PGR, BDKRB1, FGA, 1L1RAPL2, ANGPTL2, and CSF2 were significantly downregulated in AATN patients. Suggesting that the six genes have distinct differences in the renal tubules. Similarly, in the ROC curve analysis, the AUC values of all six genes were >0.7 in the renal tubular dataset, suggesting that these six genes could be AATN biomarkers with high specificity. These genes have good diagnostic sensitivity and specificity for AAV-related renal injury.The mechanism may be related to the following gene functions, but requires robust experiment to validate.
Among the eight key genes identified for AAV kidney injury, CSF2 is involved in the pathogenesis of several inflammatory and autoimmune diseases, and play an essential role in regulating the pro-inflammatory function of monocytes in the pathogenesis of AAV-related renal injury (Krebs et al., 2017; VVilar et al., 2020; Rousselle et al., 2022). Rousselle (Jing et al., 2022) found that CSF2 increased the ability of ANCA-stimulated monocytes to produce interleukin (IL)-1β and promote Th17 effector cell polarization. FGA is a major plasma protein coagulation factor in the regulation of wound healing, tissue regeneration, and the inflammatory response. ANGPTL2 is an influential member of the angiopoietin-like protein in angiogenesis, lipid metabolism, and the maintenance of tissue homeostasis. Fang et al. (Fang et al., 2020) observed that irbesartan protects the kidney by inhibiting ANGPTL2 and protecting the podocytes. AGTR2 is a type of angiotensin II receptor whose activation leads to nitric oxide release and subsequent hyperpolarization, exerting anti-inflammatory and anti-fibrotic responses and inhibiting proliferation (Ghadhanfar et al., 2017). Activation of BDKRB1, a subtype of the bradykinin receptor, is induced by injury, pain, and intense inflammatory responses (Hofman et al., 2016; Girolami et al., 2021), making it a possible therapeutic target in chronic inflammation. Kakoki et al. (Kakoki et al., 2007) showed that BDKRB1 plays an essential role in DNA damage, apoptosis, morphological and functional changes in the kidneys, and mortality during renal ischemia/reperfusion injury. IL1RAPL2 is a molecule in the IL1R family with different biological effects on immune and inflammatory responses (Boraschi and Tagliabue, 2013). There is a lack of studies about IL1RAPL2 and PGR in relation to kidney disease. Although their molecular mechanisms are not completely understood, we believe that this may be the first time that they have been identified as specific biomarkers of AAV-related renal injury, and further exploration of their effects on the mechanism of AAV-related renal injury is warranted.
We performed NMF clustering with the eight co-key genes for molecular subtype identification using the glomerular dataset GSE104948 and the tubular dataset GSE104954. In the glomerular dataset GSE104948, the co-key genes could classify in AAV-related kidney glomerular injury into two distinct subgroups. Meanwhile, in the tubular dataset GSE104954, the co-key genes could classify renal tubular disease in AAV-related kidney injury into three subgroups. Immune infiltration analysis of molecular subtypes endows subtypes with immunological significance to the disease, and immune cells associated with subtypes may potentially be significant to the disease. The statistical differences obtained by comparing subtypes with each other imply that a certain subtype plays a major role in a single immune cell. Analyzing the immune infiltration of different subtypes showed that effector memory CD4 T-cells and memory B-cells have an essential role in the progression of AAGN subtype 1. Meanwhile, CD56dim natural killer cells, neutrophils, and memory B-cells had a significant effect on the progression of the three subtypes of AATN. CD4+ effector T-cells mediate the production of neutrophil chemotactic factors through the release of IL-17A. Velden et al. detected numerous polymorphonuclear neutrophils expressing IL-17 in the glomerular and tubular mesenchyme. During the acute phase of the disease, neutrophils are an essential early intrinsic source of IL-17, helping to mediate ongoing renal inflammatory injury (Nogueira et al., 2010; Velden et al., 2012). B-cells can present antigens to T-cells, stimulate T-cell activation, and secrete pro-inflammatory factors (such as IL-6 and tumor necrosis factor) (McClure et al., 2018; Herrnstadt and Steinmetz, 2021). These results suggest that immune cells play an influential role in the progression of AAV-related renal injury (Lee, 2018; Soukou et al., 2021). The expression of these key genes positively correlates with the glomerular filtration rate, and their expression levels may be instructive for the prognosis of AAV-related renal injury.
In summary, this study systematically explored the potential mechanisms of AAV-related renal injury through comprehensive bioinformatics analysis and screened a number of biological genes and pathways that may be useful in the search for new biomarkers and therapeutic targets in AAV-related renal injury.
We found that AGTR2, ANGPTL2, BDKRB1, CSF2, FGA, IL1RAPL2, PCDH11Y, and PGR are potential novel biomarkers of AAV-related renal injury. These genes may be involved in the progression of AAV-related kidney injury through immune- and inflammation-related pathways. The co-key genes could be used to classify AAV-related kidney injury into different injury subtypes with differential patterns of immune infiltration that may closely relate to the pathogenesis of AAV-related kidney injury. Further exploration of the co-key genes’ effects on the molecular mechanism of AAV-related renal injury is warranted.
The original contributions presented in the study are included in the article/Supplementary Material, further inquiries can be directed to the corresponding authors.
YX and ZC designed the research. LL, KY, FC, JX, ZC, and YX performed the experiments. LL, KY, FC, and ZC analyzed the data. LL, KY, and ZC wrote the draft manuscript. YX and ZC supervised the research and performed writing (review and editing). All authors verified the data and approved the final version of the manuscript.
This work was supported by grants from National Natural Science Foundation of China (No. 82070720), Fujian Provincial Health Technology Project (No. 2021ZQNZD004), Fujian Province Traditional Chinese Medicine Research Project (No. 2021zyjc09) and Fujian Province Finance Project (2020B009). YX was supported by Fujian Research and Training Grants for Young and Middle_aged Leaders in Healthcare.
The authors declare that the research was conducted in the absence of any commercial or financial relationships that could be construed as a potential conflict of interest.
All claims expressed in this article are solely those of the authors and do not necessarily represent those of their affiliated organizations, or those of the publisher, the editors and the reviewers. Any product that may be evaluated in this article, or claim that may be made by its manufacturer, is not guaranteed or endorsed by the publisher.
The Supplementary Material for this article can be found online at: https://www.frontiersin.org/articles/10.3389/fgene.2023.1119017/full#supplementary-material
SUPPLEMENTARY TABLE S1 | List of key AAV- and nephropathy-related genes in the Genecards database.
Ashburner, M., Ball, C. A., Blake, J. A., Botstein, D., Butler, H., Cherry, J. M., et al. (2000). Gene ontology: Tool for the unification of biology. The gene Ontology consortium. Nat. Genet. 25, 25–29. doi:10.1038/75556
Barrett, T., Wilhite, S. E., Ledoux, P., Evangelista, C., Kim, I. F., Tomashevsky, M., et al. (2013). NCBI GEO: Archive for functional genomics data sets-update. Nucleic Acids Res. 41, D991–D995. doi:10.1093/nar/gks1193
Boraschi, D., and Tagliabue, A. (2013). The interleukin-1 receptor family. Sem. Immunol. 25, 394–407. doi:10.1016/j.smim.2013.10.023
Chen, M., Jayne, D. R. W., and Zhao, M. H. (2017). Complement in ANCA-associated vasculitis: Mechanisms and implications for management. Nat. Rev. Nephrol. 13 (6), 359–367. doi:10.1038/nrneph.2017.37
Cornec, D., Cornec-Le Gall, E., Fervenza, F. C., and Specks, U. (2016). ANCAassociated vasculitis - clinical utility of using ANCA specificity to classify patients. Nat. Rev. Rheumatol. 12 (10), 570–579. doi:10.1038/nrrheum.2016.123
Fang, L. N., Zhong, S., Huang, L. J., Lu, B., Shen, L. W., Tang, F. Y., et al. (2020). Protective effect of irbesartan by inhibiting ANGPTL2 expression in diabetic kidney disease. Curr. Med. Sci. 40 (6), 1114–1120. doi:10.1007/s11596-020-2304-z
Gatto, L., Breckels, L. M., Wieczorek, S., Burger, T., and Lilley, K. S. (2014). Mass-spectrometry-based spatial proteomics data analysis using pRoloc and pRolocdata. Bioinformatics 30 (9), 1322–1324. doi:10.1093/bioinformatics/btu013
Ghadhanfar, E., Alsalem, A., Al-Kandari, S., Naser, J., Babiker, F., and Al-Bader, M. (2017). The role of ACE2, angiotensin-(1-7) and Mas1 receptor Axis in glucocorticoid-induced intrauterine growth restriction. Reprod. Biol. Endocrinol. 15 (1), 97. doi:10.1186/s12958-017-0316-8
Gill, E. E., Smith, M. L., Gibson, K. M., Morishita, K. A., Lee, A. H. Y., Falsafi, R., et al. (2021). Different disease endotypes in phenotypically similar vasculitides affecting small-to-medium sized blood vessels. Front. Immunol. 12, 638571. doi:10.3389/fimmu.2021.638571
Girolami, J.-P., Bouby, N., Richer-Giudicelli, C., and Alhenc-Gelas, F. (2021). Kinins and kinin receptors in cardiovascular and renal diseases. Pharmaceuticals 14 (3), 240. doi:10.3390/ph14030240
Grayson, P. C., Eddy, S., Taroni, J. N., Lightfoot, Y. L., Mariani, L., Parikh, H., et al. (2018). Metabolic pathways and immunometabolism in rare kidney diseases. Ann. Rheum. Dis. 77 (8), 1226–1233. doi:10.1136/annrheumdis-2017-212935
Hänzelmann, S., Castelo, R., and Guinney, A. G. S. V. A. (2013). Gsva: Gene set variation analysis for microarray and RNA-seq data. BMC Bioinforma. 14 (7), 7. doi:10.1186/1471-2105-14-7
Herrnstadt, G. R., and Steinmetz, O. M. (2021). The role of treg subtypes in glomerulonephritis. Cell Tissue Res. 385 (2), 293–304. doi:10.1007/s00441-020-03359-7
Hofman, Z., de Maat, S., Hack, C. E., and Maas, C. B. (2016). Bradykinin: Inflammatory product of the coagulation system. Clin. Rev. Allergy Immunol. 51 (2), 152–161. doi:10.1007/s12016-016-8540-0
Jennette, J. C., and Falk, R. J. (2014). B cell-mediated pathogenesis of ANCA-mediated vasculitis. Seminars Immunopathol. 36 (3), 327–338. doi:10.1007/s00281-014-0431-y
Jennette, J. C., Falk, R. J., Hu, P., and Xiao, H. (2013). Pathogenesis of antineutrophil cytoplasmic autoantibody-associated small-vessel vasculitis. Annu. Rev. Pathology-mechanisms Dis. 8 (1), 139–160. doi:10.1146/annurev-pathol-011811-132453
Jennette, J. C., and Falk, R. J. (2014). Pathogenesis of antineutrophil cytoplasmic autoantibody-mediated disease. Nat. Rev. Rheumatol. 10 (8), 463–473. doi:10.1038/nrrheum.2014.103
Jing, X., Lu1, S., Wang1, X., Liang, M., Dong, C., Zhang, X., et al. (2022). Serum proteomic analysis identififies SAA1, FGA, SAP, and CETP as new biomarkers for eosinophilic granulomatosis with polyangiitis. Front. Immunol. 13, 86035. doi:10.3389/fimmu.2022.866035
Kakoki, M., McGarrah, R. W., Kim, H. S., and Smithies, O. (2007). Bradykinin B1 and B2 receptors both have protective roles in renal ischemia/reperfusion injury. Proc. Natl. Acad. Sci. U. S. A. 104 (18), 7576–7581. doi:10.1073/pnas.0701617104
Kallenberg, C. (2014). Advances in pathogenesis and treatment of ANCA-associated vasculitis. Discov. Med. 18 (99), 195–201.
Kallenberg, C. G. (2014). Key advances in the clinical approach to ANCA-associated vasculitis. Nat. Rev. Rheumatol. 10 (8), 484–493. doi:10.1038/nrrheum.2014.104
Kanehisa, M., and Goto, S. (2000). Kegg: Kyoto Encyclopedia of genes and Genomes. Nucleic Acids Res. 28, 27–30. doi:10.1093/nar/28.1.27
Krebs, C. F., Schmidt, T., Riedel, J-H., and Panzer, U. (2017). T helper type 17 cells in immune-mediated glomerular disease. Nat. Rev. Nephrol. 13 (10), 647–659. doi:10.1038/nrneph.2017.112
Langfelder, P., and Horvath, S. (2008). Wgcna: An R package for weighted correlation network analysis. BMC Bioinforma. 9, 559. doi:10.1186/1471-2105-9-559
Lee, G. R. (2018). The balance of Th17 versus treg cells in autoimmunity. Int. J. Mol. Sci. 19 (3), 730. doi:10.3390/ijms19030730
Lee, Y. H., Choi, S. J., Ji, J. D., and Song, G. G. (2012). CTLA-4 and TNF-α promoter-308 A/G polymorphisms and ANCA-associated vasculitis susceptibility: A meta-analysis. Mol. Biol. Rep. 39 (1), 319–326. doi:10.1007/s11033-011-0741-2
Lyons, P. A., Peters, J. E., Alberici, F., Liley, J., Coulson, R. M. R., Astle, W., et al. (2019). Genome-wide association study of eosinophilic granulomatosis with polyangiitis reveals genomic loci stratified by ANCA status. Nat. Commun. 10 (1), 5120. doi:10.1038/s41467-019-12515-9
McClure, M., Gopaluni, S., Jayne, D., and Jones, R. (2018). B cell therapy in ANCAAssociated vasculitis: Current and emerging treatment options. Nat. Rev. Rheumatol. 14 (10), 580–591. doi:10.1038/s41584-018-0065-x
Nogueira, E., Hamour, S., Sawant, D., Henderson, S., Mansfield, N., Chavele, K. M., et al. (2010). Serum IL-17 and IL-23 levels and autoantigen-specific TH17 cells are elevated in patients with ANCA-associated vasculitis. Nephrol. Dial. Transpl. 25 (7), 2209–2217. doi:10.1093/ndt/gfp783
Gaujoux, R., and Seoighe, C. (2010). A flexible R package for nonnegative matrix factorization. BMC Bioinforma. 11, 367. doi:10.1186/1471-2105-11-367
Robson, J., Doll, H., Suppiah, R., Flossmann, O., Harper, L., Hoglund, P., et al. (2015). Damage in the anca-associated vasculitides: Long-term data from the European vasculitis study group (EUVAS) therapeutic trials. Ann. Rheum. Dis. 74 (1), 177–184. doi:10.1136/annrheumdis-2013-203927
Rousselle, A., Sonnemann, J., Amann, K., Mildner, A., Lodka, D., Kling, L., et al. (2022). CSF2-dependent monocyte education in the pathogenesis of ANCA-induced glomerulonephritis. Ann. Rheum. Dis. 81 (8), 1162–1172. doi:10.1136/annrheumdis-2021-221984
Shi, L. (2017). Anti-neutrophil cytoplasmic antibody-associated vasculitis: Prevalence, treatment, and outcomes. Rheumatol. Int. 37 (11), 1779–1788. doi:10.1007/s00296-017-3818-y
Soukou, S., Huber, S., and Krebs, C. F. (2021). T cell plasticity in renal autoimmune disease. Cell Tissue Res. 385 (2), 323–333. doi:10.1007/s00441-021-03466-z
Subramanian, A., Tamayo, P., Mootha, V. K., Mukherjee, S., Ebert, B. L., Gillette, M. A., et al. (2005). Gene set enrichment analysis: A knowledge-based approach for interpreting genome-wide expression profiles. Proc. Natl. Acad. Sci. 102, 15545–15550. doi:10.1073/pnas.0506580102
Tang, J., Kong, D., Cui, Q., Wang, K., Zhang, D., Gong, Y., et al. (2018). Prognostic genes of breast cancer identified by gene Co-expression network analysis. Front. Oncol. 8, 374. doi:10.3389/fonc.2018.00374
Velden, J., Paust, H. J., Hoxha, E., Turner, J. E., Steinmetz, O. M., Wolf, G., et al. (2012). Renal IL-17 expression in human ANCA-associated glomerulonephritis. Am. J. Physiol. Ren. Physiol. 302 (12), F1663–F1673. doi:10.1152/ajprenal.00683.2011
Vvilar, R., Fish, R. J., Casini, A., and Neerman-Arbez, M. (2020). Fibrin(ogen) in human disease: Both friend and foe. Haematologica 105 (2), 284–296. doi:10.3324/haematol.2019.236901
Wu, J., Pei, Y., Rong, L., Zhuang, H., Zeng, S., Chen, L., et al. (2021). Clinicopathological analysis of 34 cases of primary antineutrophil cytoplasmic antibody-associated vasculitis in Chinese children. Front. Pediatr. 9, 656307. doi:10.3389/fped.2021.656307
Wu, T., Hu, E., Xu, S., Chen, M., Guo, P., Dai, Z., et al. (2021). clusterProfiler 4.0: A universal enrichment tool for interpreting omics data. Innovation 2 (3), 100141. doi:10.1016/j.xinn.2021.100141
Keywords: ANCA-associated vasculitis, renal injury, bioinformatics, biomarkers, immune subtypes, WGCNA
Citation: Lin L, Ye K, Chen F, Xie J, Chen Z and Xu Y (2023) Identification of new immune subtypes of renal injury associated with anti-neutrophil cytoplasmic antibody–associated vasculitis based on integrated bioinformatics analysis. Front. Genet. 14:1119017. doi: 10.3389/fgene.2023.1119017
Received: 08 December 2022; Accepted: 27 March 2023;
Published: 05 April 2023.
Edited by:
Danillo G. Augusto, University of North Carolina at Charlotte, United StatesReviewed by:
Francesco Puppo, University of Genoa, ItalyCopyright © 2023 Lin, Ye, Chen, Xie, Chen and Xu. This is an open-access article distributed under the terms of the Creative Commons Attribution License (CC BY). The use, distribution or reproduction in other forums is permitted, provided the original author(s) and the copyright owner(s) are credited and that the original publication in this journal is cited, in accordance with accepted academic practice. No use, distribution or reproduction is permitted which does not comply with these terms.
*Correspondence: Zhimin Chen, Y2hlbnptMDkwNUAxNjMuY29t; Yanfang Xu, eHV5YW5mYW5nOTlAaG90bWFpbC5jb20=
†These authors have contributed equally to this work
Disclaimer: All claims expressed in this article are solely those of the authors and do not necessarily represent those of their affiliated organizations, or those of the publisher, the editors and the reviewers. Any product that may be evaluated in this article or claim that may be made by its manufacturer is not guaranteed or endorsed by the publisher.
Research integrity at Frontiers
Learn more about the work of our research integrity team to safeguard the quality of each article we publish.