- National Engineering Laboratory for Animal Breeding, Key Laboratory of Animal Genetics and Breeding of the Ministry of Agriculture, College of Animal Science and Technology, China Agricultural University, Beijing, China
The Yangyuan donkey is a domestic animal breed mainly distributed in the northwest region of Hebei Province. Donkey body shape is the most direct production index, can fully reflect the donkey’s growth status, and is closely related to important economic traits. As one of the main breeding selection criteria, body size traits have been widely used to monitor animal growth and evaluate the selection response. Molecular markers genetically linked to body size traits have the potential to accelerate the breeding process of animals via marker-assisted selection. However, the molecular markers of body size in Yangyuan donkeys have yet to be explored. In this study, we performed a genome-wide association study to identify the genomic variations associated with body size traits in a population of 120 Yangyuan donkeys. We screened 16 single nucleotide polymorphisms that were significantly associated with body size traits. Some genes distributed around these significant SNPs were considered candidates for body size traits, including SMPD4, RPS6KA6, LPAR4, GLP2R, BRWD3, MAGT1, ZDHHC15, and CYSLTR1. Gene Ontology and Kyoto Encyclopedia of Genes and Genomes (KEGG) pathway analyses indicated that these genes were mainly involved in the P13K-Akt signaling pathway, Rap1 signaling pathway, regulation of actin cytoskeleton, calcium signaling pathway, phospholipase D signaling pathway, and neuroactive ligand-receptor interactions. Collectively, our study reported on a list of novel markers and candidate genes associated with body size traits in donkeys, providing useful information for functional gene studies and offering great potential for accelerating Yangyuan donkey breeding.
Introduction
Domestic donkeys (Equus asinus) have facilitated the movement of goods and people for millennia (Todd et al., 2022), providing a significant contribution to the development of human societies (Seyiti and Kelimu, 2021). With more than 4,000 years of donkey breeding history, China has one of the most abundant donkey genetic resources in the world (Wang et al., 2022). Yangyuan donkeys are mainly distributed in the northwestern part of Hebei Province of China, and are a medium-sized meat-and-servitude breed that was developed through long-term terroir domestication and generations of selective breeding by local farmers. It has been listed as one of 24 locally protected donkey breeds. Donkey populations have declined significantly over the past decade worldwide (Seyiti and Kelimu, 2021), and many local donkey breeds with excellent performance are currently threatened with extinction (Zeng et al., 2019), including the Yangyuan donkeys. Considering marginal livestock production worldwide (Maestrini et al., 2020), donkey products have become scarce and expensive. Therefore, breeding high-yielding varieties to improve the production performance of meat (Li et al., 2021), milk (Souroullas et al., 2018), and hides (Bennett and Pfuderer, 2020) with high economic and nutritional value may be an effective way to promote the sustainable development of the donkey industry.
As a complementary tool for conventional breeding, molecular marker-assisted selection has accelerated the breeding process of various domestic animals (Christensen and Lund, 2010; Ding et al., 2017). The increasing use of high-throughput sequencing technology has made single nucleotide polymorphisms (SNPs) highly attractive as molecular markers, which have been widely used in the field of animal breeding (Choi et al., 2017; Tsai et al., 2020). Genome-wide association studies (GWASs) are currently used to examine SNPs to determine genetic factors that affect quantitative traits (Wang W. et al., 2016). In addition, GWASs can simultaneously test millions of SNPs in comparatively wide chromosomal regions by comparing the frequencies of genetic variants in phenotypically different individuals and can estimate whether the locus is associated with the target trait (Dai et al., 2013; Gorlova et al., 2022). Due to advances in next-generation sequencing (NGS), GWAS has been carried out extensively on various livestock, such as pigs (Wu et al., 2019), cattle (Pausch et al., 2017), and sheep (Zhang T. et al., 2019). However, little in-depth breeding research has been conducted on donkeys due to their undervalued status. Previous studies have started uncovering the genetic basis of body size variations in donkeys, revealing a limited number of polymorphic sites of candidate genes, including IGF1(Lai et al., 2021), CYP4A11 (Wang et al., 2022), and TBX3 (Wang et al., 2021); however, a large amount of genomic information is waiting to be explored.
In this study, we detected significant SNP markers and candidate genes associated with body size traits within a population of 120 Yangyuan jennies using GWAS. GO and the KEGG pathway analyses were conducted. Our results may serve as a reference for molecular marker-assisted breeding in donkeys.
Materials and methods
Sample collection and phenotyping
A total of 120 donkeys were selected for whole-genome sequencing in Yangyuan County, Hebei Province, China. All donkeys were adult jennies with unknow pedigree information. The animal feed consisted of grass and hay ad libitum and water. Blood samples were taken from the jugular vein and rapidly stored at −80°C. Genomic DNA was extracted from the blood using a TIANamp genomic DNA kit (TIANGEN, DP304, China). The quality and integrity of the extracted DNA were examined based on the A260/A280 ratio and 1% agarose gel electrophoresis. We also conducted measurements of the body height, body length, chest circumference, and shin girth. EXCEL 2019 was used to generate the descriptive statistics of the four body size traits.
Sequencing and quality control
Genomic libraries with an insert size of ∼400 bp were constructed and sequenced using an Illumina NovaSeq instrument (2 × 150 paired-end mode), which was conducted by the Personalbio Biotechnology Co., Ltd. (Shanghai, China). FastQC was used to provide quality control checks on the raw sequence data (http://www.bioinformatics.babraham. ac.uk/projects/fastqc). Fastp (Chen et al., 2018) was used to perform data filtering to provide clean data for downstream analysis with the following criteria: 1) removal of the joint contamination at the 3′end; 2) sliding window quality pruning, where if the average Q value ≤ 20, then the bases in the window would be marked as discarded and the window would be moved forward by one base; and 3) if the length of any of the double-end reads was ≤50 bp, the double-end reads would be removed.
Mapping
High-quality trimmed read pairs were aligned to the reference donkey genome (GenBank: PRJNA431818) by Burrows-Wheeler Aligner (BWA, versin0.7.12-r1039) with default parameters and SAM files were generated (Li and Durbin, 2009). The SAM files were sequenced using Picard1.107 software. Duplicates were removed using the MarkDuplicates command in the Picard package. Thus, if multiple paired reads had the same chromosome coordinates after comparison, only the paired reads with the highest score would be retained, and the reads near InDel were most prone to mapping errors. To minimize SNPs caused by mapping errors, it was necessary to rematch the reads near InDel to improve the accuracy of SNP calling, using the IndelRealigner command in the Genome Analysis Toolkit (GATK) program to rematch all of the reads near InDel to improve the accuracy of SNP prediction. SNP prediction accuracy was improved by using the IndelRealigner command in the GATK program to re-compare all of the reads near InDel. For all 120 samples, mapping statistics based on high-quality mapped reads for each accession included the average coverage depth and the proportion of the donkey genome covered by different read depths.
SNP calling and annotating
High-quality mapped reads were used for variant calling with GATK (version 3.8) (Zhu et al., 2015). To improve the accuracy of SNP calling, two steps were conducted, including using GATK to output files that contained all possible InDels and using IndelRealigner to re-compare all InDels near reads. The SNPs that met the following criteria were filtered out to ensure reliability: 1) Fisher test of strand bias (FS) ≤ 60; 2) HaplotypeScore ≤13.0; 3) Mapping Quality (MQ) ≥ 40; 4) Quality Depth (QD) ≥ 2; 5) ReadPosRankSum ≥ −8.0; 6) MQRankSum > −12.5; 7) Read depth (DP) > 4; and 8) --maf 0.01; 9) --max-missing 0.7. All SNPs were annotated using ANNOVAR (Wang et al., 2010) software with default parameter settings.
Population structure and phylogenetic analysis
PCA of the 120 samples was conducted with GCTA (Yang et al., 2011) software. To evaluate the phylogenetic relationships between individuals, a maximum likelihood (ML) tree was built based on the SNPs using Fasttree software (Price et al., 2009), with n = 1,000 bootstrap replications. We used PopLDdecay (Zhang C. et al., 2019) software with default parameters to calculate linkage disequilibrium (LD) decay.
GWAS and candidate gene search
GWASs for body size traits, including body height, body length, chest circumference, and shin girth, were performed using linear mixed models (LMMs) by the efficient mixed-model association expedited (EMMAX) software (http://genetics.cs.ucla.edu/emmax.) (Kang et al., 2010). The model is
where
The Manhattan and quantile-quantile plot (Q-Q) plots were drawn by R packages. The SNPs reached the genome-wide significant level -log10 (p-value) = 7 were determined as the significant loci. The search for candidate genes was extended from 50 kb upstream and downstream from the significant SNPs.
GO and KEGG pathway enrichment analyses
To provide insight into the functional enrichment of the candidate genes, we performed GO enrichment analysis (Ashburner et al., 2000) and the KEGG pathway (Kanehisa et al., 2004). GO enrichment analysis was performed using InterProScan. A hypergeometric p-value was calculated where the background was set as genes in the entire genome. GO terms with p-value <0.05 were considered significantly enriched, and GO enrichment analysis elucidated the molecular function, biological process, and cellular components. KEGG pathway analysis was performed using KEGG Automatic Annotation Server (KAAS) to predict the main biological pathways involved in the candidate genes.
Results
Descriptive statistics for body size traits
The body height, body length, chest circumference, and shin girth were collected from 120 Yangyuan jennies. The raw measurement data are detailed in Supplementary Table S1. Ranking from high to low according to body height, we divided the population into two groups, the top 60 donkeys are the larger ones, and the bottom 60 donkeys are the smaller ones. The order of the samples in Supplementary Table S1 is the result of ranking according to body height. The general descriptive statistics of the body size traits are shown in Table 1. The average body height was 139.33 cm with a standard deviation of 10.94, the average body length was 138.62 cm with a standard deviation of 10.43, the average chest circumference was 138.62 cm with a standard deviation of 9.68, and the average shin girth was 15.93 cm with a standard deviation of 1.19.
Genome sequencing and SNPs identification
To sequence the Yangyuan donkey genome, libraries with 400 bp inserts were sequenced and 861 Gb of raw sequence data were produced. The quality of the raw data is shown in Supplementary Table S2, with Q20 ≥ 96.7, Q30 ≥ 91.48, and GC content from 43.08 to 44.00, which confirmed the high quality of the sequence data. The construction of the library and sequencing were successful. To enhance the quality of the data, Fastp was used to generate high quality data. In total, 718 Gb of clean data were generated. The filtered clean data were aligned to the reference genome, and the mapping rate was 99.57%–99.79% (Supplementary Table S3), implying that the mapping rate could be used for subsequent variant detection. In general, by using next-generation sequencing technology, and after filtering and mapping, a total of 9,735,956 SNPs were identified using the Illumina NovaSeq sequencing platform with an average sequencing depth of ∼4.1 (Supplementary Table S4). Their distribution on the chromosomes is shown in Figure 1, and the SNP annotation results are shown in Table 2. We found that 65.8% of the SNPs were concentrated in the intergenic region, 31.48% of the SNPs were concentrated in the intronic region, 0.91% of the SNPs were located upstream, 0.8% of the SNPs were concentrated downstream, and only 0.98% of the SNPs were located in the exon region.
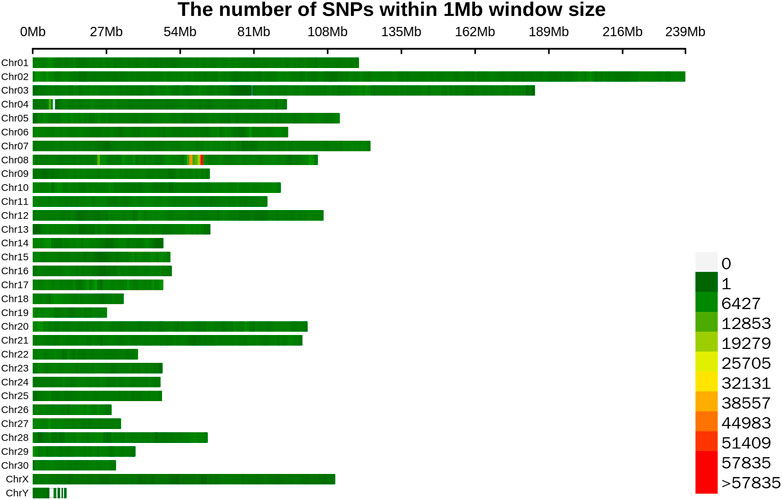
FIGURE 1. Distribution map of SNPs on different chromosomes. Different colors represent the number of SNPs in the 1 Mb window.
Population structure and phylogenetic analysis
We performed principal component analysis (PCA) with 9,735,956 SNPs to analyze the population structure of 120 Yangyuan donkeys. PCA revealed the relationship between the first two principal components, and we found that most of the individuals were clustered together and only a few individuals showed outlier distribution, which demonstrated that the genetic background of the population was relatively uniform (Figure 2A). The phylogenetic tree showed that the samples were mixed together without obvious clustering into separate groups, which was consistent with PCA (Figure 2B).
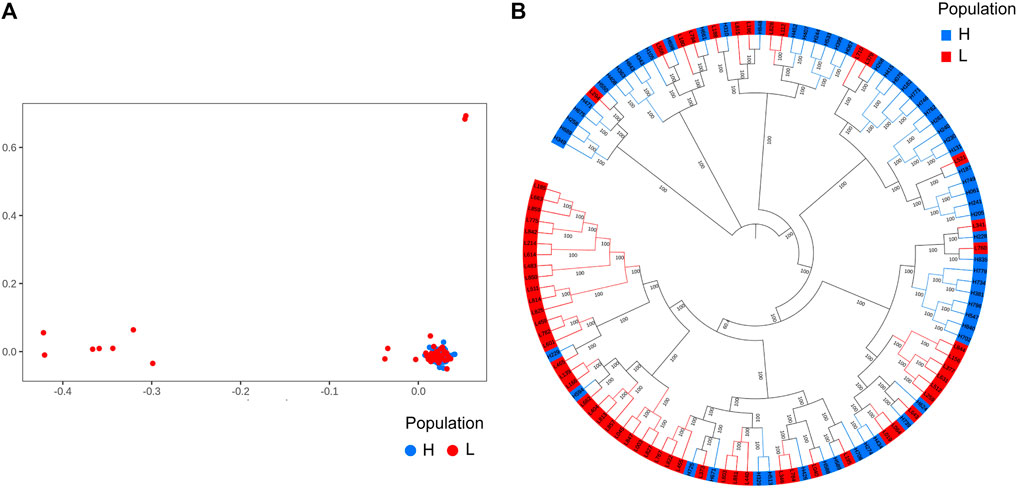
FIGURE 2. Population structure analysis results. A total of 9,735,956 SNPs and 120 donkeys were used to perform the analysis. The 120 donkeys are ranked in descending order of body height, the top 60 donkeys are the larger ones, denoted by H, and the bottom 60 donkeys are the smaller ones, denoted by L. (A). Principal component analysis results. (B). Phylogenetic tree.
Genome-wide association study of body size traits
Based on the detected SNPs, correlation analysis was performed according to the SNP and body height, body length, chest circumference, and shin girth traits to identify the markers and candidate genes that were closely associated with the target traits. In total, 16 SNPs reached a significance threshold of 7, and most of the SNPs were within the intronic, intergenic, and downstream locations of chromosomes 8, 13, and 31. The Manhattan plots for the four body size traits and corresponding Q-Q plots of the p-values against the expected p-values are presented in Figure 3. According to the LD analysis results (Supplementary Figure S1), all positional candidate genes were identified for 50 kb before and after the most significant SNP. Detailed information regarding the significant SNPs identified using GWAS and the putative candidate genes are presented in Table 3, including sphingomyelin phosphodiesterase 4 (SMPD4), ribosomal protein S6 kinase A6 (RPS6KA6), lysophosphatidic acid receptor 4 (LPAR4), glucagon-like peptide 2 receptor (GLP2R), bromodomain, and the WD repeat domain containing 3 (BRWD3), magnesium transporter 1 (MAGT1), zinc finger DHHC-type palmitoyltransferase 15 (ZDHHC15), and cysteinyl leukotriene receptor 1 (CYSLTR1). Other genes located in the 50-kb genome region flanking the significantly associated SNPs are shown in Supplementary Table S5.
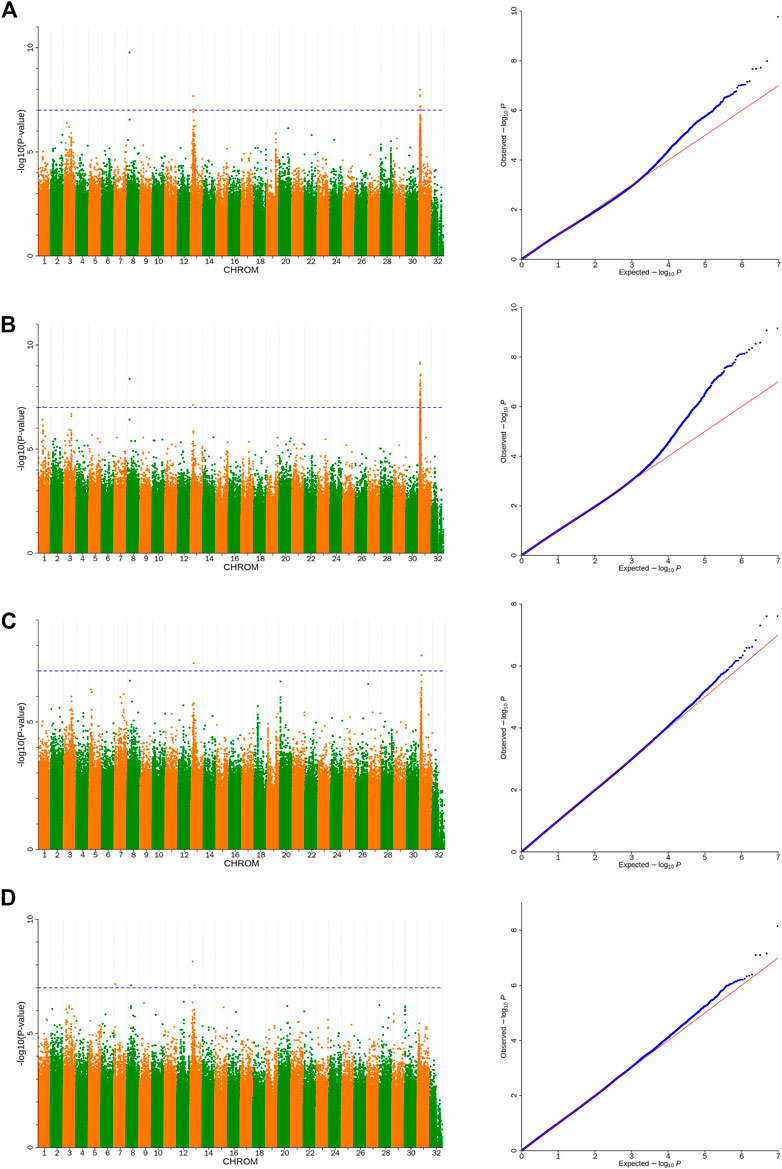
FIGURE 3. Manhattan and Q-Q plots of body size traits (A). Body height, (B). Body length, (C). Chest circumference, (D). Shin girth). Significant p-value threshold set at p = 10−7. A total of 16 significant SNPs are labeled at the top of the Manhattan plot (left). Q-Q plots are displayed as scatter plots of observed and expected log p-values (right).
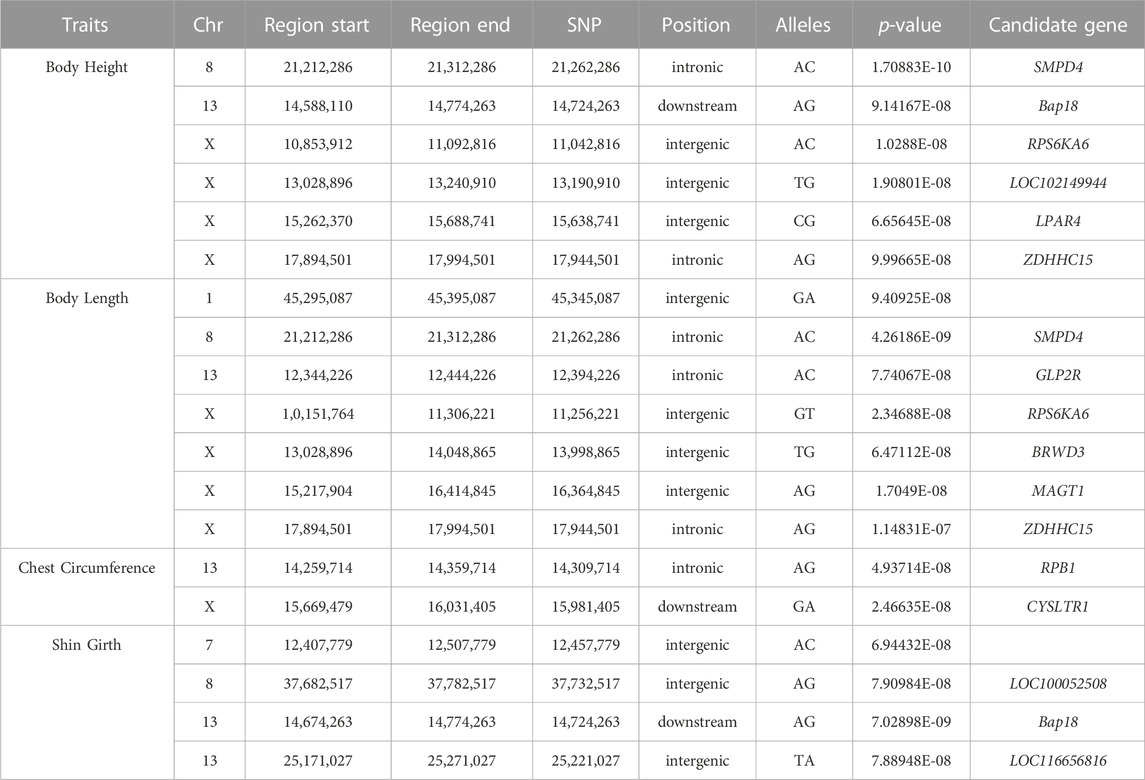
TABLE 3. Candidate genes significantly associated with body size traits as revealed by genome-wide associated study.
Bioinformatic analysis of the candidate genes
The GO function annotation and KEGG pathway enrichment analyses were utilized to explore the biological functions of the candidate genes. The GO enrichment analysis results were classified based on the molecular function, biological process, and cellular components. The top 10 GO terms with the smallest p-value, indicating the most significant enrichment, were selected for display, and the results are shown in Figure 4. According to the KEGG enrichment results, the KEGG pathways with the smallest FDR values, which were the most enriched, are illustrated in Figure 5.
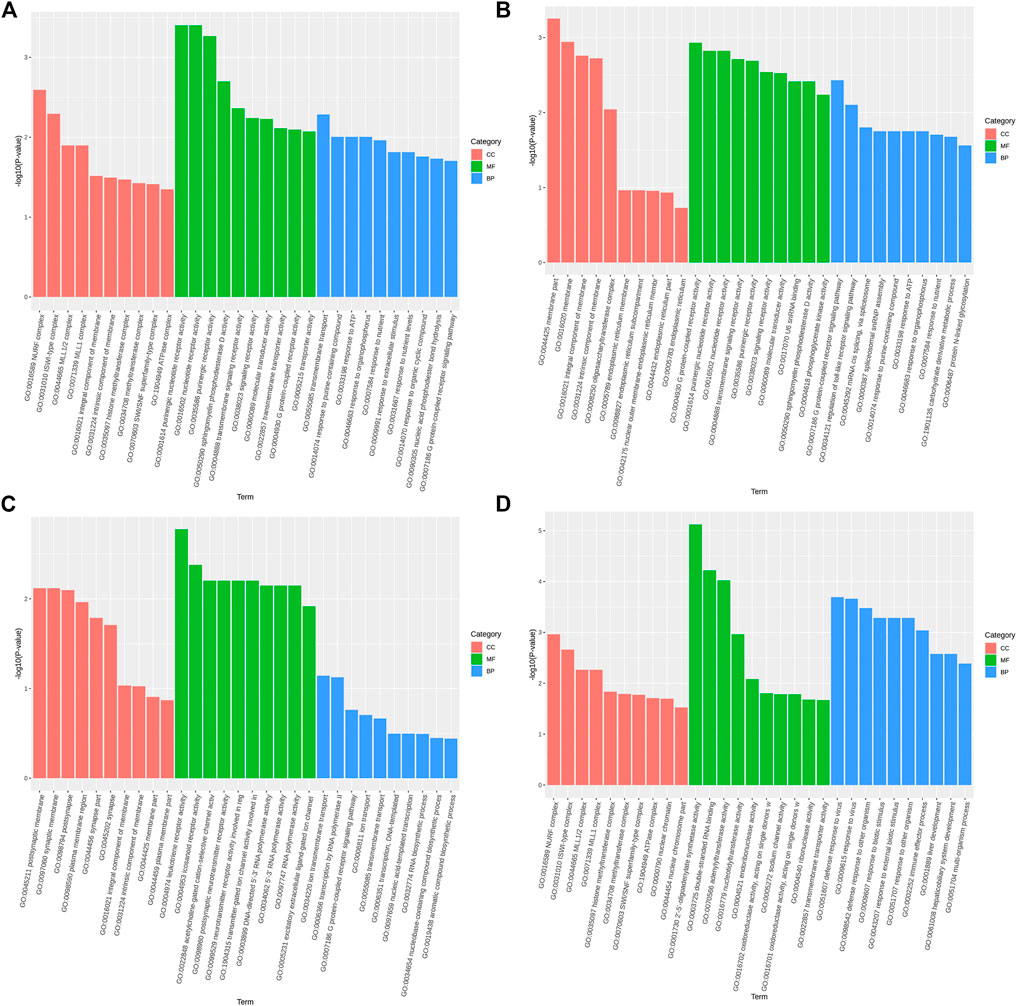
FIGURE 4. Top 10 significantly enriched GO terms of cellular component (CC), molecular function (MF), and biological process (BP). (A). Body height, (B). Body length, (C). Chest circumference, (D). Shin girth.
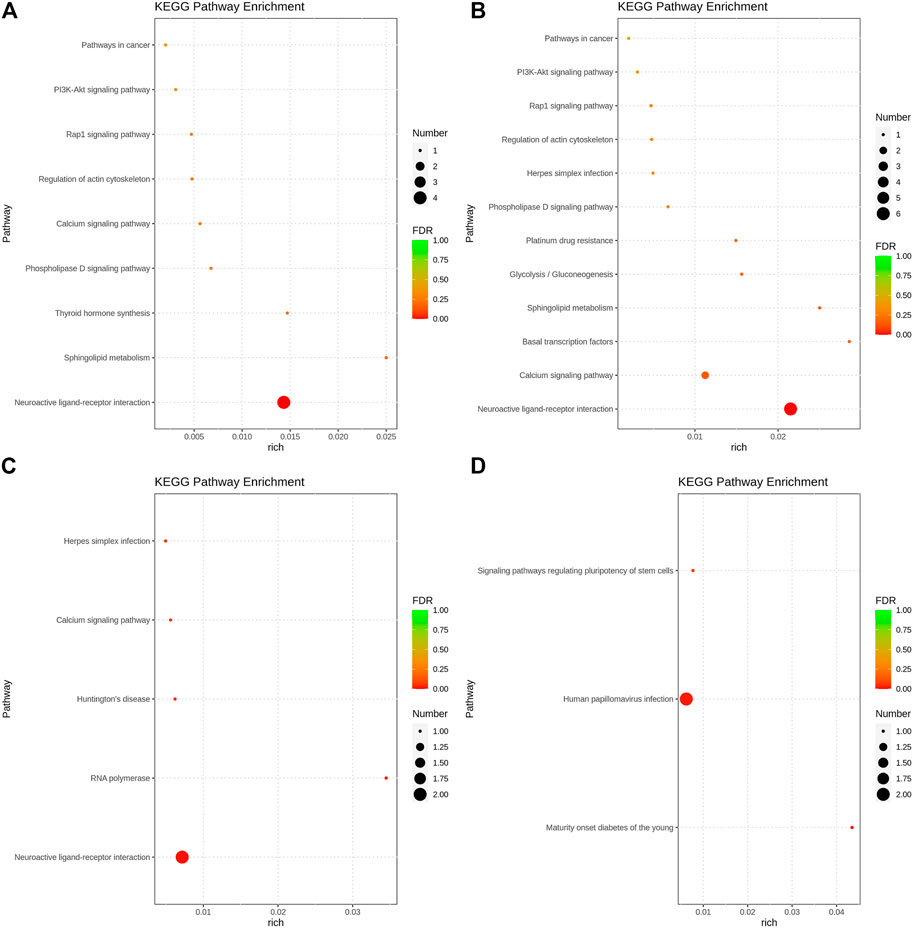
FIGURE 5. Bubble chart of KEGG pathway analysis results. (A). Body height, (B). Body length, (C). Chest circumference, (D). Shin girth.
Discussion
The Yangyuan donkey is considered a valued Chinese indigenous breed, famous for its high disease resistance performance and strong adaptability. The donkey industry has been vigorously developed in many areas of northern China; however, the genetic mechanisms of body size traits of these donkeys have rarely been studied. Due to the long average generation interval (5 years) of donkeys, traditional approaches to donkey breeding yield slow progress. Undoubtedly, compared to conventional breeding, marker-assisted selection (MAS) could accelerate breeding programs (Ding et al., 2017). Molecular markers such as SSRs and SNPs are abundant in animal genomes (Campos Mantello et al., 2019). In the breeding of animals, body size trait is an important index for evaluating the growth status (Asiamah et al., 2020), and earlier studies have found a strong correlation between body size and meat production in animals (Takasuga, 2016). In the case of donkey breeding, it was essential to identify molecular markers associated with body size traits.
With the development of molecular markers, the GWAS approach has been used extensively to understand the genetic basis of complex traits in animals (Tam et al., 2019), and is widely considered a powerful approach for exploring marker genes for complex traits at the SNP level (Wang et al., 2016). In this research, we focused on the differences among body size traits in Yangyuan donkeys, and we noticed significant body size differences, even within the same population. Prior to the GWAS, we performed a principal component analysis. The results of PCA showed the outlier phenomenon of some small-sized donkeys, which might due to the inclusion of several hybrid donkeys with similar phenotypic characteristics to Yangyuan donkeys. We eliminated this stratification by using the PCA value of each sample as covariance in GWAS. By performing GWAS in 120 Yangyuan donkeys using SNPs detected via whole-genome sequencing, we reported some significantly correlated SNPs and candidate genes that could serve as potential candidates associated with body size traits. By annotating candidate regions, sphingomyelin phosphodiesterase 4 (SMPD4) was found to be a candidate gene greatly associated with body height and body length. SMPD4 is a sphingomyelinase from lipid rafts that hydrolyzes sphingomyelin into phosphorylcholine and ceramide, and regulates membrane composition in lipid rafts (Wu et al., 2010). The loss of function of SMPD4 will induce endoplasmic reticulum (ER) stress, autophagy, impaired sphingolipids homeostasis, cell cycle dysregulation, and this could partially explain the severe growth failure and microcephaly in SMPD4-related disorder (Magini et al., 2019; Bijarnia-Mahay et al., 2022). SMPD4 causes several developmental abnormalities through the sphingolipid-related pathway, serving as a hint for our subsequent variation study of how it may affect body size traits in donkeys. Magnesium transporter 1 (MagT1) was considered a candidate related to chest circumference. MagT1 contributes to the maintenance of Mg homeostasis at the cellular level, playing a crucial role in bone metabolism and in the regulation of bone cell functions (Castiglioni et al., 2018). A key event in bone formation is the differentiation of MSC into osteoblasts, a process that involves Mg and its transport (Castiglioni et al., 2018). Research showed that regulating the expression of MagT1 was closely related to the osteogenic differentiation of BMSCs, and MagT1 was upregulated in hMSC differentiation (Lin et al., 2019). In rat MSC, silencing MagT1 blunted osteogenic differentiation (Zheng et al., 2016). However, little is known about the role of MagT1 in bone, and further experiments are needed to verify whether SNPs in MagT1 will affect its expression and whether MagT1 causes differences in donkey body size by affecting bone formation.
GO analysis showed that in terms of cellular components, the candidates focused on the components of chromosome remodeling complex, lysine methyltransferase, and membrane. Molecular function enrichment analysis highlighted the membrane protein activities, and biological processes were mainly enriched in response to certain organic substances and ion channel activity. According to KEGG analysis, pathways were mainly enriched on the P13K-Akt signaling pathway, the Rap1 signaling pathway, the regulation of actin cytoskeleton, calcium signaling pathway, phospholipase D signaling pathway, and neuroactive ligand-receptor interactions. Previous studies have shown that by regulating the P13K-Akt signaling pathway, the proliferation and differentiation of bone marrow mesenchymal stem cells and bone formation could be promoted (Ledergerber et al., 1985; Zhang et al., 2017). Blocking the PI3K/AKT signaling pathway was found to not only impair chondrocyte differentiation but also inhibit longitudinal bone growth (Ulici et al., 2008). The calcium signaling pathway in bone has been extensively studied, as calcium is a necessary factor for bone cell proliferation and differentiation. Based on the above analysis, we hypothesized that SNPs caused differences in the body size traits of Yangyuan donkeys by affecting the pathways related to bone growth and metabolism, and the enrichment analysis results provided guidance for our subsequent functional studies.
In summary, from the GWAS results for the body size traits with SNPs detected from the whole-genome sequence in 120 Yangyuan donkeys, we identified 16 SNPs significantly associated with body size traits. All of the results provided good ideas for further revealing the Yangyuan donkey body size trait mechanisms, improving donkey production performance, and conducting marker assisted selection in donkey breeding.
Data availability statement
The data presented in the study are deposited in the NCBI repository, accession number PRJNA898246.
Ethics statement
The animal study was reviewed and approved by Institutional Review Board (or Ethics Committee) of China Agricultural University Laboratory Animal Welfare and Animal Experimental Ethical Inspection Committee (protocol code: AW-311022-2-1, date of approval: 1 August 2021). Animal Experiment Guidelines and Regulations of China Agricultural University (Permit No. SCXK: 2012-0766, China). Written informed consent was obtained from the owners for the participation of their animals in this study.
Author contributions
SZ, SS and SW conceived and planned the study. SS and SW wrote the manuscript. SS, SW, NL, SC, SD, YG, XW and YC performed the sample collection and phenotyping. All authors reviewed the manuscript. The author(s) read and approved the final manuscript.
Funding
The work was supported by Inner Mongolia central guidance local scientific research project fund, Hebei donkey industry innovation fund.
Acknowledgments
We thank LetPub (www.letpub.com) for its linguistic assistance during the preparation of this manuscript.
Conflict of interest
The authors declare that the research was conducted in the absence of any commercial or financial relationships that could be construed as a potential conflict of interest.
Publisher’s note
All claims expressed in this article are solely those of the authors and do not necessarily represent those of their affiliated organizations, or those of the publisher, the editors and the reviewers. Any product that may be evaluated in this article, or claim that may be made by its manufacturer, is not guaranteed or endorsed by the publisher.
Supplementary material
The Supplementary Material for this article can be found online at: https://www.frontiersin.org/articles/10.3389/fgene.2023.1112377/full#supplementary-material
References
Ashburner, M., Ball, C. A., Blake, J. A., Botstein, D., Butler, H., Cherry, J. M., et al. (2000). Gene ontology: tool for the unification of biology. The gene ontology consortium. Nat. Genet. 25 (1), 25–29. doi:10.1038/75556
Asiamah, C., Xue, Y., Lu, L. L., Zou, K., Zhao, Z., and Su, Y. (2020). Evaluation of growth performance on family breeding of the leizhou black duck: A preliminary study. Vet. Med. Sci. 6 (3), 500–510. doi:10.1002/vms3.263
Bennett, R., and Pfuderer, S. (2020). The potential for new donkey farming systems to supply the growing demand for hides. Anim. (Basel) 10 (4), 718. doi:10.3390/ani10040718
Bijarnia-Mahay, S., Somashekar, P. H., Kaur, P., Kulshrestha, S., Ramprasad, V. L., Murugan, S., et al. (2022). Growth and neurodevelopmental disorder with arthrogryposis, microcephaly and structural brain anomalies caused by Bi-allelic partial deletion of SMPD4 gene. J. Hum. Genet. 67 (3), 133–136. doi:10.1038/s10038-021-00981-3
Campos Mantello, C., Boatwright, L., da Silva, C. C., Scaloppi, E. J., de Souza Goncalves, P., Barbazuk, W. B., et al. (2019). Deep expression analysis reveals distinct cold-response strategies in rubber tree (Hevea brasiliensis). BMC Genomics 20 (1), 455. doi:10.1186/s12864-019-5852-5
Castiglioni, S., Romeo, V., Locatelli, L., Cazzaniga, A., and Maier, J. A. M. (2018). TRPM7 and MagT1 in the osteogenic differentiation of human mesenchymal stem cells in vitro. Sci. Rep. 8 (1), 16195. doi:10.1038/s41598-018-34324-8
Chen, S., Zhou, Y., Chen, Y., and Gu, J. (2018). fastp: an ultra-fast all-in-one FASTQ preprocessor. Bioinformatics 34 (17), i884–i890. doi:10.1093/bioinformatics/bty560
Choi, T., Lim, D., Park, B., Sharma, A., Kim, J. J., Kim, S., et al. (2017). Accuracy of genomic breeding value prediction for intramuscular fat using different genomic relationship matrices in Hanwoo (Korean cattle). Asian-Australas J. Anim. Sci. 30 (7), 907–911. doi:10.5713/ajas.15.0983
Christensen, O. F., and Lund, M. S. (2010). Genomic prediction when some animals are not genotyped. Genet. Sel. Evol. 42, 2. doi:10.1186/1297-9686-42-2
Dai, H., Zhao, Y., Qian, C., Cai, M., Zhang, R., Chu, M., et al. (2013). Weighted SNP set analysis in genome-wide association study. PLoS One 8 (9), e75897. doi:10.1371/journal.pone.0075897
Ding, R., Quan, J., Yang, M., Wang, X., Zheng, E., Yang, H., et al. (2017). Genome-wide association analysis reveals genetic loci and candidate genes for feeding behavior and eating efficiency in Duroc boars. PLoS One 12 (8), e0183244. doi:10.1371/journal.pone.0183244
Gorlova, O. Y., Xiao, X., Tsavachidis, S., Amos, C. I., and Gorlov, I. P. (2022). SNP characteristics and validation success in genome wide association studies. Hum. Genet. 141 (2), 229–238. doi:10.1007/s00439-021-02407-8
Kanehisa, M., Goto, S., Kawashima, S., Okuno, Y., and Hattori, M. (2004). The KEGG resource for deciphering the genome. Nucleic Acids Res. 32, D277–D280. doi:10.1093/nar/gkh063
Kang, H. M., Sul, J. H., Service, S. K., Zaitlen, N. A., Kong, S. Y., Freimer, N. B., et al. (2010). Variance component model to account for sample structure in genome-wide association studies. Nat. Genet. 42 (4), 348–354. doi:10.1038/ng.548
Lai, Z., Wu, F., Li, M., Bai, F., Gao, Y., Yu, J., et al. (2021). Tissue expression profile, polymorphism of IGF1 gene and its effect on body size traits of Dezhou donkey. Gene 766, 145118. doi:10.1016/j.gene.2020.145118
Ledergerber, B., Bettex, J. D., Joos, B., Flepp, M., and Luthy, R. (1985). Effect of standard breakfast on drug absorption and multiple-dose pharmacokinetics of ciprofloxacin. Antimicrob. Agents Chemother. 27 (3), 350–352. doi:10.1128/AAC.27.3.350
Li, H., and Durbin, R. (2009). Fast and accurate short read alignment with Burrows-Wheeler transform. Bioinformatics 25 (14), 1754–1760. doi:10.1093/bioinformatics/btp324
Li, M., Zhu, M., Chai, W., Wang, Y., Fan, D., Lv, M., et al. (2021). Determination of lipid profiles of Dezhou donkey meat using an LC-MS-based lipidomics method. J. Food Sci. 86 (10), 4511–4521. doi:10.1111/1750-3841.15917
Lin, S., Yang, G., Jiang, F., Zhou, M., Yin, S., Tang, Y., et al. (2019). A magnesium-enriched 3D culture system that mimics the bone development microenvironment for vascularized bone regeneration. Adv. Sci. (Weinh) 6 (12), 1900209. doi:10.1002/advs.201900209
Maestrini, M., Molento, M. B., Mancini, S., Martini, M., Angeletti, F. G. S., and Perrucci, S. (2020). Intestinal strongyle genera in different typology of donkey farms in tuscany, central Italy. Vet. Sci. 7 (4), 195. doi:10.3390/vetsci7040195
Magini, P., Smits, D. J., Vandervore, L., Schot, R., Columbaro, M., Kasteleijn, E., et al. (2019). Loss of SMPD4 causes a developmental disorder characterized by microcephaly and congenital arthrogryposis. Am. J. Hum. Genet. 105 (4), 689–705. doi:10.1016/j.ajhg.2019.08.006
Pausch, H., Emmerling, R., Gredler-Grandl, B., Fries, R., Daetwyler, H. D., and Goddard, M. E. (2017). Meta-analysis of sequence-based association studies across three cattle breeds reveals 25 QTL for fat and protein percentages in milk at nucleotide resolution. BMC Genomics 18 (1), 853. doi:10.1186/s12864-017-4263-8
Price, M. N., Dehal, P. S., and Arkin, A. P. (2009). FastTree: Computing large minimum evolution trees with profiles instead of a distance matrix. Mol. Biol. Evol. 26 (7), 1641–1650. doi:10.1093/molbev/msp077
Seyiti, S., and Kelimu, A. (2021). Donkey industry in China: Current aspects, suggestions and future challenges. J. Equine Vet. Sci. 102, 103642. doi:10.1016/j.jevs.2021.103642
Souroullas, K., Aspri, M., and Papademas, P. (2018). Donkey milk as a supplement in infant formula: Benefits and technological challenges. Food Res. Int. 109, 416–425. doi:10.1016/j.foodres.2018.04.051
Takasuga, A. (2016). PLAG1 and NCAPG-LCORL in livestock. Anim. Sci. J. 87 (2), 159–167. doi:10.1111/asj.12417
Tam, V., Patel, N., Turcotte, M., Bosse, Y., Pare, G., and Meyre, D. (2019). Benefits and limitations of genome-wide association studies. Nat. Rev. Genet. 20 (8), 467–484. doi:10.1038/s41576-019-0127-1
Todd, E. T., Tonasso-Calviere, L., Chauvey, L., Schiavinato, S., Fages, A., Seguin-Orlando, A., et al. (2022). The genomic history and global expansion of domestic donkeys. Science 377 (6611), 1172–1180. doi:10.1126/science.abo3503
Tsai, H. Y., Janss, L. L., Andersen, J. R., Orabi, J., Jensen, J. D., Jahoor, A., et al. (2020). Genomic prediction and GWAS of yield, quality and disease-related traits in spring barley and winter wheat. Sci. Rep. 10 (1), 3347. doi:10.1038/s41598-020-60203-2
Ulici, V., Hoenselaar, K. D., Gillespie, J. R., and Beier, F. (2008). The PI3K pathway regulates endochondral bone growth through control of hypertrophic chondrocyte differentiation. BMC Dev. Biol. 8, 40. doi:10.1186/1471-213X-8-40
Wang, K., Li, M., and Hakonarson, H. (2010). ANNOVAR: functional annotation of genetic variants from high-throughput sequencing data. Nucleic Acids Res. 38 (16), e164. doi:10.1093/nar/gkq603
Wang, G., Li, M., Zhou, J., An, X., Bai, F., Gao, Y., et al. (2021). A novel A > G polymorphism in the intron 2 of TBX3 gene is significantly associated with body size in donkeys. Gene 785, 145602. doi:10.1016/j.gene.2021.145602
Wang, G., Wang, F., Pei, H., Li, M., Bai, F., Lei, C., et al. (2022). Genome-wide analysis reveals selection signatures for body size and drought adaptation in Liangzhou donkey. Genomics 114 (6), 110476. doi:10.1016/j.ygeno.2022.110476
Wang, W., Zhang, T., Wang, J., Zhang, G., Wang, Y., Zhang, Y., et al. (2016). Genome-wide association study of 8 carcass traits in Jinghai Yellow chickens using specific-locus amplified fragment sequencing technology. Poult. Sci. 95 (3), 500–506. doi:10.3382/ps/pev266
Wang, Z., Cabrera, M., Yang, J., Yuan, L., Gupta, B., Liang, X., et al. (2016). Genome-wide association analysis identifies genetic loci associated with resistance to multiple antimalarials in Plasmodium falciparum from China-Myanmar border. Sci. Rep. 6, 33891. doi:10.1038/srep33891
Wu, B. X., Clarke, C. J., and Hannun, Y. A. (2010). Mammalian neutral sphingomyelinases: regulation and roles in cell signaling responses. Neuromolecular Med. 12 (4), 320–330. doi:10.1007/s12017-010-8120-z
Wu, P., Wang, K., Yang, Q., Zhou, J., Chen, D., Liu, Y., et al. (2019). Whole-genome re-sequencing association study for direct genetic effects and social genetic effects of six growth traits in Large White pigs. Sci. Rep. 9 (1), 9667. doi:10.1038/s41598-019-45919-0
Yang, J., Lee, S. H., Goddard, M. E., and Visscher, P. M. (2011). GCTA: a tool for genome-wide complex trait analysis. Am. J. Hum. Genet. 88 (1), 76–82. doi:10.1016/j.ajhg.2010.11.011
Zeng, L., Dang, R., Dong, H., Li, F., Chen, H., and Lei, C. (2019). Genetic diversity and relationships of Chinese donkeys using microsatellite markers. Arch. Anim. Breed. 62 (1), 181–187. doi:10.5194/aab-62-181-2019
Zhang, H., Jiang, C., Li, M., Wang, X., Tian, F., Fang, X., et al. (2017). CXCR4 enhances invasion and proliferation of bone marrow stem cells via PI3K/AKT/NF-κB signaling pathway. Int. J. Clin. Exp. Pathol. 10 (9), 9829–9836.
Zhang, C., Dong, S. S., Xu, J. Y., He, W. M., and Yang, T. L. (2019). PopLDdecay: a fast and effective tool for linkage disequilibrium decay analysis based on variant call format files. Bioinformatics 35 (10), 1786–1788. doi:10.1093/bioinformatics/bty875
Zhang, T., Gao, H., Sahana, G., Zan, Y., Fan, H., Liu, J., et al. (2019). Genome-wide association studies revealed candidate genes for tail fat deposition and body size in the Hulun Buir sheep. J. Anim. Breed. Genet. 136 (5), 362–370. doi:10.1111/jbg.12402
Zheng, J., Mao, X., Ling, J., Chen, C., and Zhang, W. (2016). Role of magnesium transporter subtype 1 (MagT1) in the osteogenic differentiation of rat bone marrow stem cells. Biol. Trace Elem. Res. 171 (1), 131–137. doi:10.1007/s12011-015-0459-4
Keywords: Yangyuan donkey, body size traits, SNP, GWAS, candidate genes
Citation: Song S, Wang S, Li N, Chang S, Dai S, Guo Y, Wu X, Cheng Y and Zeng S (2023) Genome-wide association study to identify SNPs and candidate genes associated with body size traits in donkeys. Front. Genet. 14:1112377. doi: 10.3389/fgene.2023.1112377
Received: 30 November 2022; Accepted: 14 February 2023;
Published: 28 February 2023.
Edited by:
Yun Li, Ocean University of China, ChinaReviewed by:
Lijun Shi, Institute of Animal Sciences (CAAS), ChinaQuratulain Hanif, Forman Christian College, Pakistan
Copyright © 2023 Song, Wang, Li, Chang, Dai, Guo, Wu, Cheng and Zeng. This is an open-access article distributed under the terms of the Creative Commons Attribution License (CC BY). The use, distribution or reproduction in other forums is permitted, provided the original author(s) and the copyright owner(s) are credited and that the original publication in this journal is cited, in accordance with accepted academic practice. No use, distribution or reproduction is permitted which does not comply with these terms.
*Correspondence: Shenming Zeng, zengsm@cau.edu.cn
†These authors have contributed equally to this work