- 1State Key Laboratory of Genetic Engineering, School of Life Sciences and Human Phenome Institute, Fudan University, Shanghai, China
- 2Grandomics Biosciences Institute, Wuhan, China
- 3Shanghai Zoological Park, Shanghai, China
- 4Shanghai Jiao Tong University School of Medicine, Shanghai, China
- 5Cancer Institute, Shanghai Cancer Center, Fudan University, Shanghai, China
- 6Centro de Investigación en Enfermedades Tropicales, Facultad de Microbiología, Universidad de Costa Rica, San José, Costa Rica
Sericinus montelus (Lepidoptera, Papilionidae, Parnassiinae) is a high-value ornamental swallowtail butterfly species widely distributed in Northern and Central China, Japan, Korea, and Russia. The larval stage of this species feeds exclusively on Aristolochia plants. The Aristolochia species is well known for its high levels of aristolochic acids (AAs), which have been found to be carcinogenic for numerous animals. The swallowtail butterfly is among the few that can feed on these toxic host plants. However, the genetic adaptation of S. montelus to confer new abilities for AA tolerance has not yet been well explored, largely due to the limited genomic resources of this species. This study aimed to present a chromosome-level reference genome for S. montelus using the Oxford Nanopore long-read sequencing, Illumina short-read sequencing, and Hi-C technology. The final assembly was composed of 581.44 Mb with an expected genome size of 619.27 Mb. Further, 99.98% of the bases could be anchored onto 30 chromosomes. The N50 of contigs and scaffolds was 5.74 and 19.12 Mb, respectively. Approximately 48.86% of the assembled genome was suggested to be repeat elements, and 13,720 protein-coding genes were predicted in the current assembly. The phylogenetic analysis indicated that S. montelus diverged from the common ancestor of swallowtails about 58.57–80.46 million years ago. Compared with related species, S. montelus showed a significant expansion of P450 gene family members, and positive selections on eloa, heatr1, and aph1a resulted in the AA tolerance for S. montelus larva. The de novo assembly of a high-quality reference genome for S. montelus provided a fundamental genomic tool for future research on evolution, genome genetics, and toxicology of the swallowtail butterflies.
Introduction
Butterflies account for the high biodiversity of terrestrial organisms, with more than 18,000 species recorded worldwide (Espeland et al., 2018). Butterflies develop a life cycle through a complete metamorphosis spanning four stages: egg, larva, pupa, and adult (Figure 1). More than half a century ago, butterflies were widely used as important model species to investigate the impact of habitat destruction, pest control, and climatic changes. Further, the study of butterflies has progressed tremendously in other diverse fields: evolution, embryology, mimicry, toxicology, genetics, population dynamics, and biodiversity conservation (Heikkila et al., 2012; Kawahara and Breinholt, 2014).
Sericinus montelus (Lepidoptera, Papilionidae, Parnassiinae) is a common species of swallowtail butterfly with high ornamental values native only to Northern and Central China, Japan, Korea, and Russia (Li et al., 2016). In the last few years, S. montelus was used to study its four stages of life and aspects such as natural history, breeding, mobility, climate change, and habitat loss (Li et al., 2016). The larvae of S. montelus are monophagous insects that feed on Aristolochia contorta (Li et al., 2016). However, the host plant notoriously contains toxic substances such as aristolochic acid (AA), which is carcinogenic to many animals (Arlt et al., 2002; Chen et al., 2012). In mammal models, AAs are metabolically converted into form reactive intermediates, and these intermediates have the potential to bind to DNA and exert mutagenic and carcinogenic effects (Lebeau et al., 2001).
Many biological aspects of S. montelus remain unclear to date. For example, why do the S. montelus larvae only feed on a single host plant Aristolochia; which functional genes and metabolic pathways are essential for S. montelus to be AA tolerant; and how S. montelus is tolerant to the genotoxicity of AAs. Indeed, comprehensive genomic data analyses are needed to address these questions. Therefore, the assembly of a high-quality chromosome-level reference genome for S. montelus can provide a genomic tool to fill this gap.
Whole-genome sequencing provides a high-resolution and comprehensive collection of an individual’s genetic variations for target species at the base-by-base level (Ng and Kirkness, 2010). The sequencing and assembly of a complex genome for insect species rapidly and in a cost-effective manner has become possible with the development of third-generation sequencing technology (Oxford Nanopore and PacBio sequencing technologies) (Lu et al., 2019; Yang et al., 2020). Moreover, high-throughput chromosome conformation capture (Hi-C) is an effective technology (Belaghzal et al., 2017) to identify genome-wide interactions between and within chromosomes. The Hi-C technology is a powerful tool to improve the genome assembly to the chromosome level (Belaghzal et al., 2017).
This study reported a high-quality chromosome-level reference genome of S. montelus using the Oxford Nanopore long reads, Illumina short reads, and Hi-C scaffolding technology. This was the most contiguous reference genome generated for the swallowtail butterflies so far. Comparative genome analyses provided evidence to identify candidate functional genes and metabolic pathways related to AA tolerance. The genome of S. montelus reported in this study might provide important data for further investigations on the evolution biology of the swallowtail butterflies, complexity of host-plant shifts, and genomic aspects of key affiliated genes for the adaptation to AA tolerance.
Materials and methods
DNA collection and sequencing
A fifth instar larval stage of S. montelus was collected from Beijing mountainous area. High–molecular weight genomic DNA was extracted using the SDS method, followed by purification with Qiagen genomic kit following the standard protocol. DNA purity was then assessed based on OD 260/280 and OD 260/230 ratios detected using NanoDrop One/OneC Microvolume UV-Vis Spectrophotometer (Thermo Fisher Scientific, USA). DNA concentration was measured using a Qubit 3.0 Fluorometer (Invitrogen, USA).
Illumina library with an insert size of 350 bp was prepared using a TruSeq Nano DNA HT sample preparation kit (Illumina, USA). DNA fragments were then blunted with an A-base overhang and ligated to sequencing adapters for Illumina sequencing with further polymerase chain reaction (PCR) amplification. Finally, PCR products were purified (AMPure XP system), and libraries were analyzed for size distribution using an Agilent 2100 Bioanalyzer and quantified using real-time PCR. After the aforementioned quality control steps, the library was sequenced on an Illumina NovaSeq 6000 platform in a paired-end sequencing model. Further, 2 µg of DNA was used for Nanopore library construction. The size selection (20 kb) was performed using a BluePippin system (Sage Science, USA). Next, both ends of DNA fragments were repaired and an A-ligation reaction was conducted using an NEBNext Ultra II End Repair/dA-tailing Kit (Cat# E7546). The adapter in the LSK109 kit was used for further ligation reaction, and a Qubit 3.0 Fluorometer (Invitrogen, USA) was used to quantify the size of library fragments. Sequencing was then performed on a Nanopore PromethION (Oxford Nanopore Technologies, United Kingdom) at Grandomics (Wuhan, China).
Hi-C library construction
A Hi-C library was constructed from a fifth instar larval stage following the previously reported protocols (Lafontaine et al., 2021). In brief, freshly harvested tissues were cut into 2-cm pieces and vacuum infiltrated in nuclei isolation buffer supplemented with 2% formaldehyde. Glycine and additional vacuum infiltration were used to stop the process. The fixed tissue was then grounded to a powder before resuspending it in a nuclei isolation buffer to obtain a nuclear suspension. One hundred units of DpnII were used to digest the purified nuclei, followed by marking with biotin-14-dCTP. Biotin-14-dCTP from non-ligated DNA ends was removed owing to the exonuclease activity of T4 DNA polymerase. The ligated DNA was sheared into 300–600 bp fragments and then blunt-end repaired and A-tailed, followed by purification through biotin-streptavidin-mediated pull-down. Finally, the Hi-C library was quantified using the aforementioned steps and then sequenced at Illumina NovaSeq 6000 platform.
Genome size estimation
Raw data generated from Illumina sequencing were quality controlled using fastp v0.20.0 (Chen et al., 2018), and low-quality reads, adapters, and reads containing N were filtered. Specifically, the reads were filtered under the following conditions: 1) reads with ≥10% unidentified nucleotides N); 2) reads with >10 nucleotides aligned to the adapter, allowing ≤10% mismatch; 3) reads with >50% bases having Phred quality <5; 4) putative PCR duplicates generated by PCR amplification removed in the library construction process (reads 1 and 2 of two paired-end reads that were completely identical). A total of 100,000 reads were selected randomly and compared against the sequences deposited in the NT database (nucleotide sequence database) to verify the presence of contamination (Sayers et al., 2022) using blastn v2.12.0+ (Mount, 2007). After quality control, the clean reads were used to count the number of 17-mers using jellyfish2 v2.3.0 (Marcais and Kingsford, 2011) and the distributions were then used to estimate the genome size with GenomeScope v2.0b (Vurture et al., 2017).
Chromosome-level genome assembly and validation
The raw reads of Oxford Nanopore were corrected using NextDenovo v2.5.0 (https://github.com/Nextomics/NextDenovo) with seed length cutoff set to 23 kb, and the corrected reads were then assembled using SMARTdenovo v1.0.0 (Liu et al., 2021) with parameters “-k 21 -J 3000” to generate the primary contigs. Three rounds of iterative polishing using Nextpolish v1.2.3 (Hu et al., 2020) were implemented based on Oxford Nanopore reads and Illumina short reads to enhance the continuity and base accuracy of the assembly. Subsequently, the polished assembly was decontaminated. The reads aligned in terms of depth and GC content were calculated on contigs using a 10-kb window bin. The contigs having 80% bins with GC less than 0.2 or greater than 0.6 were selected. Then, the contigs were aligned to the NT database (ftp://ftp.ncbi.nlm.nih.gov/blast/db/), and contigs aligned to bacterial and viral species were removed. After genome decontamination, the assembly was used to evaluate the quality of the Hi-C library and valid read pairs were identified following the Hic-Pro pipeline v2.8.1 (Servant et al., 2015). These valid read pairs were then used to calculate the interactions between every two regions of the whole genome to generate an interaction matrix, which was used to cluster, order, and orient the contigs onto a designated number of chromosomes using LACHESIS version-201701 (Burton et al., 2013) with default settings. In the LACHESIS step, manual curation was used to reduce assembly errors based on the whole-genome interaction matrix signal. In the contig clustering process, contigs were split into a 50-kb interaction matrix. The contigs were interrupted by manual curation when the interaction bins of one contig were clustered into different chromosomes. In contig ordering and orientation steps, manual curation was used to ensure that the contig was in the right position in chromosomes based on the interaction matrix score.
The quality of the assembly was validated using different strategies based on contiguity, correctness, and completeness (3C) criteria (Molina-Mora et al., 2020). For contiguity, the statistics about total assembled length, total number of contigs and scaffolds, and contig and scaffold N50 (N50 value was calculated at the median of the total length by sorting all contigs with length) were calculated. Three analyses were performed to assess completeness: i) BUSCO v5.1.3 (Manni et al., 2021) with insect_odb10 datasets was used to evaluate the assembly of expected genes; ii) RNA sequencing (RNA-seq) reads were mapped back to the genome with hisat2 v2.1.0 (Kim et al., 2019) with default settings to evaluate the mapping rate and coverage of the gene region of the assembly; iii) valid Hi-C reads were mapped back to the pseudo-chromosomes, and the interaction matrix between 100-kb bins was calculated based on the mapping results; iv) Nanopore long reads and Illumina short reads were, respectively, mapped back to the assembly using minimap2 v2.17-r954-dirty (Li, 2018) and bwa v0.7.16a-r1181 (Li and Durbin, 2009) to calculate the mapping rate and coverage; and iv) DepthSizer v1.6.3 (Chen et al., 2022) was used to establish the collapsed repeats in the assembly and the completeness of the genome assembly. In the last case, the mapping results were used to evaluate the genome’s accuracy or correctness. Finally, the mapped reads were used to detect variants by employing samtools v1.13 (Li et al., 2009) and bcftools v1.9 (Li, 2011), and homozygous SNPs and indels were considered as genomic errors.
RNA collection and sequencing
RNA-seq was achieved for four samples representing four stages of S. montelus (egg, larva, pupa, and adult; Figure 1) to assist in the gene predictions in the genome annotation step. Total RNA from each sample was collected using an RNeasy Plus Mini Kit (Qiagen, Germany). The RNA purity was checked using a NanoDrop One/OneC spectrophotometer (Thermo Fisher Scientific). RNA degradation and contamination were monitored using 1% agarose gels. The RNA concentration was measured using a Qubit RNA Assay Kit of a Qubit 3.0 Fluorometer (Life Technologies, CA, USA). The RNA integrity was assessed using an RNA Nano 6000 Assay Kit of a Bioanalyzer 2100 system (Agilent Technologies, CA, USA). The RNA quality for the RNA samples with an integrity value more than 8.0 was used for constructing the cDNA library. The paired-end library was prepared using an Illumina TruSeq Sample Preparation Kit and sequenced on a NovaSeq6000 platform (Illumina). The raw data were filtered by removing reads containing adapters, Ns, and low-quality reads using fastp v0.20.0 (Chen et al., 2018).
Genome annotation
The repeat sequences, including tandem repeat and transposable elements (TEs) residing in the genome, were investigated. The tandem repeats were identified using GMATA v2.2 (Wang and Wang, 2016) and Tandem Repeats Finder (TRF) v4.07b (Benson, 1999). Briefly, GMATA (Wang and Wang, 2016) was used to identify simple sequence repeats (SSRs), and TRF (Benson, 1999) was used to recognize all tandem repeat elements in the whole genome. The TEs residing in the genome were identified using a combination of ab initio and homology-based methods. To this end, MITE-hunter (Han and Wessler, 2010) and RepeatModel2 v1.0.11 (Flynn et al., 2020) were used to identify possible TEs with default parameters and create a repeat library. The library was then classified according to homologous deposits in the Repbase v14.02 (Bao et al., 2015). RepeatMasker v1.331 (Tarailo-Graovac and Chen, 2009) was applied to search for known and novel TEs by mapping sequences against the de novo repeat and Repbase library for further identification of the repeats throughout the genome. Overlapping TEs belonging to the same repeat class were collated and combined.
The protein-coding genes were predicted by integrating ab initio, homology-based, and RNA-seq-based strategies. For homology prediction, GeMoMa v1.6.1 (Keilwagen et al., 2019) was used to determine the gene structure based on the alignments of homologous peptides from five related species, including Bombyx mori (GCF_014905235.1), Danaus plexippus (GCF_009731565.1), Papilio glaucus (GCA_000931545.1), Papilio machaon (GCF_912999745.1), and Papilio xuthus (GCF_000836235.1) mapped back to the assembly. For RNA-seq-based gene prediction, clean RNA-seq data were mapped back to the reference genome using STAR v2.7.3a (Dobin et al., 2013). The transcripts were then assembled using Stringtie v1.3.4d (Pertea et al., 2015), and open reading frames (ORFs) were predicted using PASA v2.3.3 (Haas et al., 2008). The PASA results were also used as a training set to train profiles for de novo prediction tools including Augustus v3.3.1 (Stanke et al., 2006) and SNAP (Korf, 2004). Subsequently, Augustus and SNAP were used to predict possible protein-coding regions with default parameters. Finally, EVidenceModeler v1.1.1 (EVM) (Haas et al., 2008) was used to produce an integrated gene set, and genes harboring TEs were removed using the TransposonPSI package (http://transposonpsi.sourceforge.net/). The miscoded genes were further filtered. The untranslated regions (UTRs) and alternative splicing regions were determined using PASA based on RNA-seq assemblies. The longest transcripts were retained for each locus, and the regions outside of the ORFs were designated UTRs.
The non-coding RNAs (ncRNA), including micro RNAs (miRNAs), ribosomal RNAs (rRNAs), small nuclear RNAs (snRNAs), and transfer RNAs (tRNAs), were determined with the Rfam database (release 13.0) (Kalvari et al., 2017) using Infernal v1.1.3 software (Nawrocki, 2014). tRNA was identified using tRNAscan-SE v2.0 software (Chan et al., 2021). The subunits of rRNA were predicted using RNAmmer v1.2 software (Lagesen et al., 2007).
The functional annotation of the predicted protein-coding genes was carried out by aligning them with homologs deposited in public databases. Briefly, the predicted proteins were searched against homologs deposited in SwissProt (https://ftp.uniprot.org/pub/databases/uniprot/), NR (ftp://ftp.ncbi.nlm.nih.gov/blast/db/FASTA/nr.gz), KEGG (https://www.kegg.jp/kegg/download/), and KOG (http://ftp//ftp.ncbi.nih.gov/pub/COG/KOG/) using the blastall v2.2.26 blastp model (Mount, 2007) with an E-value cutoff of 1e-05. Descriptions, gene ontologies, and KEGG pathways were extracted from the top hits.
Gene family and phylogenetic analysis
The orthogroups between S. montelus and relative species that seemed to originate from common ancestor sequences were investigated. To this end, genome sequences, GFF3 file, CDS, and protein sequences of relative species, including P. xuthus, Papilio polytes, P. machaon, P. glaucus, Papilio binaor, Melitaea cinxia, Junonia coenia, Heliconius melpomene, D. plexippus, and B. mori, were downloaded from NCBI (Kitts et al., 2015). For each species, the longest transcript for each gene was extracted from the genome and GFF3 file, and miscoded genes and genes exhibiting premature termination were discarded by checking whether the coding sequence accorded with the gene model. These genes whose CDS did not begin with a start codon or did not end with a stop codon, or whose CDS length was not a multiple of three, or genes with a stop codon in the middle of the CDS were discarded. Following the extraction of protein sequences, they were aligned in pairs using the blastall blastp model (Mount, 2007), with an E-value cutoff of 1e-05. The inter-genome orthologous, intra-genome paralogous, and single-copy gene pairs were further identified using OrthMCL v2.0.9 (Li et al., 2003).
After identifying orthologous gene sets, a molecular phylogenetic analysis was performed using the shared single-copy genes. Briefly, the coding sequences were extracted from the single-copy families, and each orthogroup was globally aligned using Mafft v7.313 (Katoh et al., 2002) with default settings. Poorly aligned sequences were then eliminated using Gblocks v0.91b (Castresana, 2000), and the GTR + GAMMA substitution model of RAxML v8.2.10 (Stamatakis, 2015) was used for the phylogenetic tree construction with 1000 times of ultrafast bootstrap resampling. The generated tree file was displayed using Figtree v1.4.4 (http://tree.bio.ed.ac.uk/software/figtree/). MCMCTREE v4.9e included in the PAML4 (Yang, 2007) package was used to estimate the divergent time based on the phylogenetic tree. The calibration times were obtained from the TimeTree database (http://www.timetree.org/) by placing soft bounds at the split node of B. mori (114 Mya), P. glaucus–J.coenia (76–106 Mya), and D. plexippus–J. coenia (77–107 Mya) (Kumar et al., 2017).
Significant expansion or contraction of specific gene families is often associated with the adaptive divergence of closely related species. Hence, CAFÉ v4.0 (De Bie et al., 2006) was used to perform a gene family expansion and contraction based on the results of OrthMCL and estimated divergence times. The enrichment analysis for the expanded gene family members was implemented using clusterProfiler v4.0 (Wu et al., 2021). After the identification, the gene lists were analyzed with functional enrichment using GO terms and KEGG pathways. The significantly enriched determinants were extracted individually for comparison against model organisms.
Positive selection analysis
According to the neutral theory of molecular evolution, the ratio of non-synonymous substitution rate (Ka) and synonymous substitution rate (Ks) of protein-coding genes can be used to identify genes showing the signatures of natural selection. Therefore, the branch-site model in the codeml model (Yang, 2007) was used to estimate possible adaptations that resided in the proteins in the S. montelus genome with orthologous genes. Likelihood ratio tests were performed between the null hypothesis (model = 2, NSsites = 2, fix_omega = 1, omega = 1) and alternative hypothesis (model = 2, NSsites = 2, fix_omega = 0, omega = 1.5) to determine the fitness differences between these two models. Genes with a p-value < 0.05 under the branch-site model were considered positively selected genes. The p values were also adjusted using the Benjamini–Hochberg method (Benjamini and Hochberg, 1995), given the multiple tests. The process was repeated twice for each gene to test the convergence of the HMM models. The three-dimensional structure for the two candidates of positively selected genes was predicted using AlphaFold2 (Bouatta et al., 2021) and visualized using PyMOL (www.pymol.org/pymol).
The function enrichment analysis of positive selection genes in GO terms and KEGG pathways was conducted using clusterProfiler v4.0. The significant enrichment pathways were defined with a p-value < 0.05. For RNA-seq data, the value of fragments per kilobase per million mapped reads (FPKM) was used to characterize the gene expression profile at a different stage with the stringtie v1.3.4 software, and heatmap was plotted with HeatMapper (http://www.heatmapper.ca/expression/).
Results and discussion
Chromosome-level genome assembly
A total of 25.9-Gb Illumina short reads (Table 1) were produced and used to estimate the size and heterozygosity of the S. montelus genome. The main peak was 33 according to the distribution of 17-mers (Supplementary Figure S1), and the deduced size and heterozygosity of the S. montelus genome were approximately 619.27 Mb and 0.8% (Supplementary Table S1), respectively.
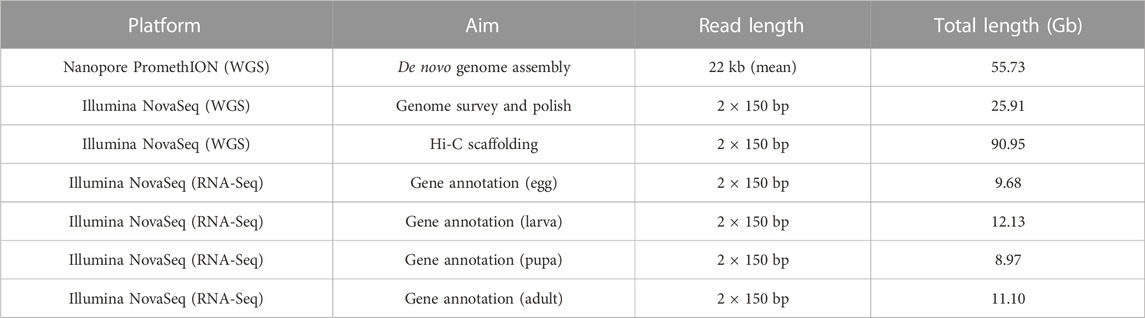
TABLE 1. Raw data of S. montelus generated in the present study using Nanopore and Illumina technology.
Subsequently, more than two million Oxford Nanopore long reads, approximately 55.7 Gb and representing nearly 100× of the estimated genome size, were generated and used for de novo genome assembly. Briefly, the long reads were first self-corrected using Nextdenovo and then assembled into primary contigs using SMARTdenovo. Considering the high error rate of Oxford Nanopore long reads, the consensus of the primary contigs was then iteratively improved using the long reads and Illumina short reads for three rounds. After genome decontamination, the final genome size was nearly 581.4 Mb, with a contig N50 of approximately 5.74 Mb (Table 2).
Karyotype is a written proof of chromosome numbers of what a cytogeneticist observes from species. Hi-C is a remarkable technology for genome scaffolding. It is a chromosome conformation capture method used to detect genome-wide chromatin interactions in many plant and animal genomes. More than 600 million of Illumina short reads (approximately 90.9 Gb representing about 150× of the estimated genome size) were generated (Hi-C strategy) to scaffold the contigs. After quality control and filtering, 152,896,732 uniquely and validly mapped paired-end reads were retained and used to construct the Hi-C interaction matrix. The chromosome number between 28 and 32 was used with Lachesis and SALSA to guide the genome scaffolding. Finally, the Hi-C matrix map showed that 30 can clustered succeed and each cluster had intra- and interchromosomal matrix data. Based on the matrix, 275 contigs of 566 Mb, representing more than 99.9% of the assembly, were orientated and anchored to 30 pseudo-chromosomes (Supplementary Table S2). The pseudo-chromosome LG04 was identified as a Z chromosome with collinear blocks.
This strategy was followed because of the high error rate (∼15%) of Nanopore reads, in which the primary assembly contig assembled using SMARTdenovo was polished by combining with high-accuracy Illumina reads (Ge et al., 2019). Further, Hi-C is an impressive technology for genome scaffolding. It is a chromosome conformation capture method used to detect genome-wide chromatin interactions in many plant and animal genomes. It is widely applied in improving the de novo assembled contigs into chromosome-level genome assembly, as previously reported (Yang et al., 2020).
The quality of the final assembly was estimated using different strategies. For contiguity, the contig N50 and scaffold N50 were 5.74 and 19.12 Mb (Table 2), respectively, with 99.4% complete BUSCOs in the final genome. For completeness, the size of the final chromosome-level genome was about 581.44 Mb, which represented nearly 93.9% of the estimated genome size using GenomeScope and 98.94% of the estimated genome size (587.66 Mb) using DepthSizer with a combined sequencing length and the single-copy read depth software. More than 98.84% of the whole-genome sequencing short reads were mapped back to the assembly (Supplementary Table S3), and the percentage of homozygous alternative bases was estimated to be less than 7.6e-6 (Supplementary Table S4) as part of the correctness assessment. Generally, these bases indicate errors since normal alternatives should be heterozygous. Also, for completeness, short reads of RNA-seq for the four different phases, including egg, larva, pupa, and adult, were mapped back to the assembly, and the mapping rate ranged from 95.24% to 96.89% (Supplementary Table S3). The completeness using BUSCO showed that nearly 99.4% of the complete benchmark genes could be identified in the assembly, within which about 98.0% and 1.4% were single-copy and duplicated, respectively (Supplementary Figure S2). Only 0.5% of the benchmark genes were missed in the assembly. All short reads from the Hi-C library were mapped back to the final assembly, and the results showed that the interactions between nearby regions were more intensive than those between distant ones (Supplementary Figure S3), conforming to the premise of Hi-C scaffolding. Furthermore, a consistent synteny was found between the assemblies of S. montelus and the relative species B. mori and P. binaor (Figure 2).
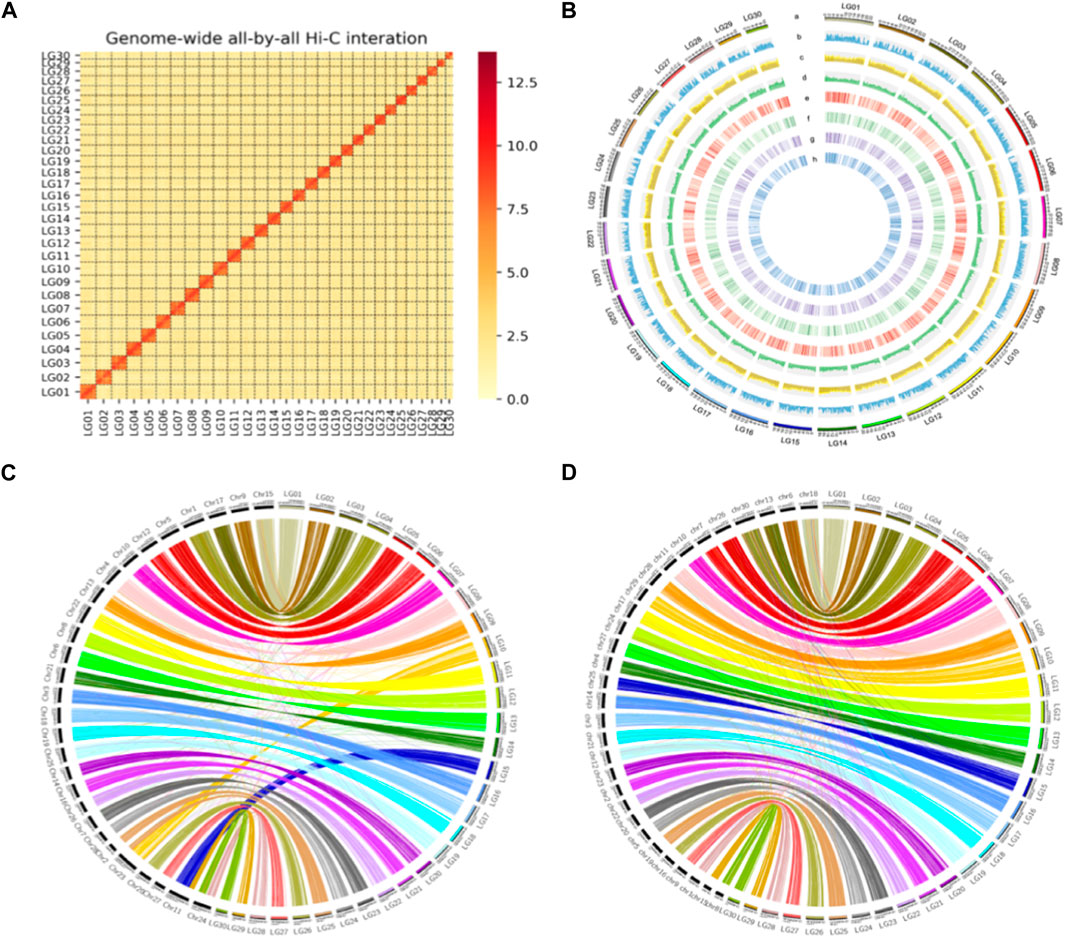
FIGURE 2. High quality de novo assembly of S. montelus. (A) Interaction heatmap between any two regions of the whole genome intra- and interchromosome with Hi-C data. (B) Whole-genome feature distribution of S. montelus with 1-Mb-wide bins. a, Chromosome (30 chromosomes); b, gene density (13,720 protein-coding genes); c, repeat density (repeat content 48.9%); d, GC content distribution (average GC content 35.9%); e, egg stage gene expression (75% of genes have expression); f, larva stage gene expression (84% expression); g, pupa stage gene expression (77% expression); h, adult stage gene expression (86% expression). (C) Protein-coding gene synteny between S. montelus (right) and Bombyx mori (left). (D) Protein-coding gene synteny between S. montelus (right) and P. binaor (left).
Compared with the de novo genome assemblies generated for butterflies (Table 3), the quality of the present assembly was one of the best (Lu et al., 2019; Yang et al., 2020). Briefly, a total of 55.7 and 30.5 Gb raw data were generated for the Chinese peacock butterfly Papilio bianor and dead-leaf butterfly Kallima inachus and used for de novo assembly, and the final contig N50 was 5.5 and 3.3 Mb, respectively (Lu et al., 2019; Yang et al., 2020). The BUSCO assessments showed that approximately 96.3% and 97.3% of complete benchmark genes were recovered for the two species, respectively (Luan et al., 2015; Turi et al., 2018). For S. montelus, 55.7 Gb raw data were used for the de novo assembly, and the contig N50 and BUSCO assessments reached 5.7 Mb and 99.4%, respectively. The high-quality genome assembly of S. montelus might provide a basic understanding of genome evolution, toxicology, and AA tolerance in future analysis.
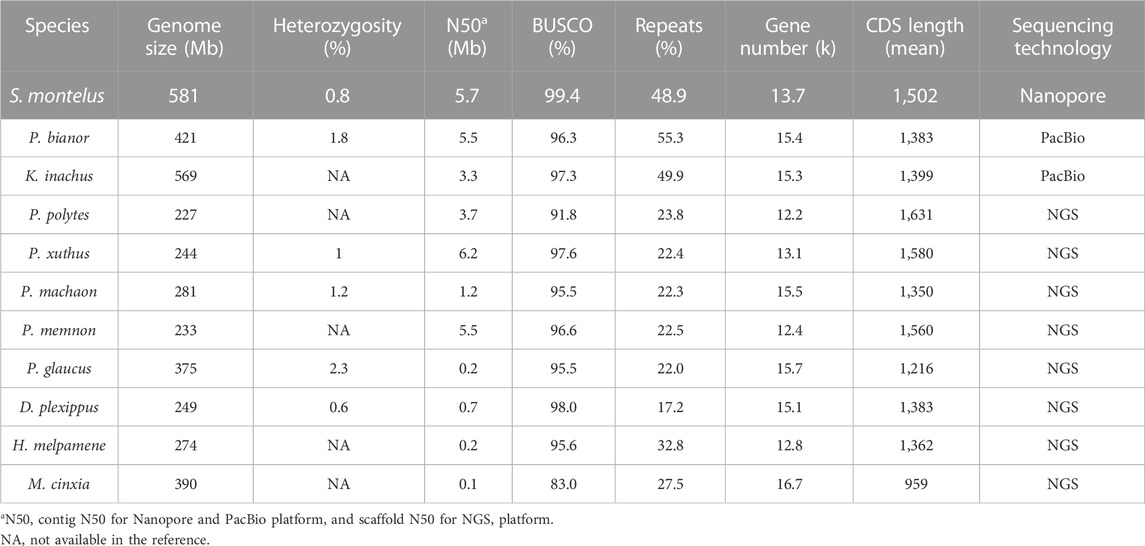
TABLE 3. Comparison of basic characteristics of S. montelus genome assembly and annotation with published butterfly genomes.
Identification of repeats and protein-coding genes
A total of 284.1 Mb accounting for approximately 48.9% of the whole assembly were identified as repeat elements by adopting both the de novo and homology-based strategies (Table 3). Generally, the type I TE long interspersed nuclear element (LINE) was the most represented in the genome, and about one-fifth of the genome was composed of LINEs (Supplementary Table S5). The second most represented TEs residing in the genome was a long terminal repeat, accounting for nearly one-tenth of the genome. The other most represented REs were DNA repeats and short interspersed nuclear elements, which accounted for about 8.8% and 3.7% of the genome, respectively.
A total of 13,720 protein-coding genes were identified in the genome by combining the results of three strategies, including the de novo, homology-based, and expression-based gene prediction methods. Of these, 12,422 genes (representing 90.5% of total genes) were annotated by at least one database (Table 4). Specifically, the descriptions were obtained for 12,422 and 9,955 genes from Nr and SwissProt, which accounted for nearly 90.1% and 72.6% of total genes, respectively. A total of 8,518 genes were annotated by KOG functional protein database, 6,382 genes by GO, and 6,861 genes by KEGG (Table 4). The ncRNA was predicted using the published database of Rfam (Daub et al., 2015). A total of 148 rRNAs, 194 miRNAs, 3,326 tRNAs, and 166 snRNAs were identified (Supplementary Table S6).
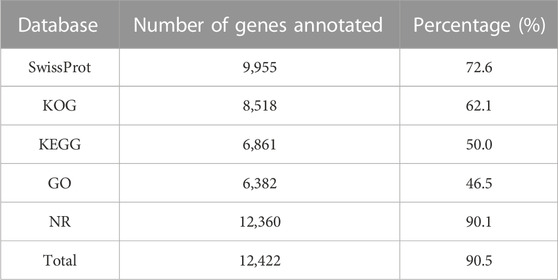
TABLE 4. Annotation summary of the predicted protein-coding genes residing in the S. montelus genome in the SwissProt, KOG, KEGG, GO, and NR databases.
Regarding the content of repeat sequences, the number of elements residing in S. montelus genome was similar to that of K. inachus (48.9% vs. 49.9%), slightly less than that of P. bianor (55.3%) but much higher than that of P. xuthus (22.4%), P. machaon (32.3%), P. polytes (34.0%), and others (Schmeiser et al., 2009; Stiborova et al., 2011) (Table 3). In S. montelus, 13.7 k genes were identified. Compared with other species, the gene number for our model was less than that of K. inachus (15.3 k), P. bianor (15.4 k), and P. machaon (15.5 k), but more than that of P. xuthus (13.1 k) and P. polytes (12.2 k). The de novo genome assemblies of S. montelus, K. inachus, and P. bianor were all based on the long-read sequencing technologies, but short reads were used for the other species such as P. xuthus, P. machaon, and P. polytes. Thus, it seemed that long-read sequencing technologies recovered more repeats residing in the genomes that short-read technologies might have expectedly missed. The long-read sequencing technologies had the advantage of resolving repetitive regions in the de novo assembly. For example, in a recent study, the Telomere-to-Telomere (T2T) Consortium used long-read sequencing technologies to finish a gapless human T2T-CHM13 genome and addressed 8% of the genome with the most repeated regions (Nurk et al., 2022).
On the contrary, frameshifts may occur for long-read raw assemblies because of the high error rate compared with Illumina assemblies (Koren et al., 2019). This situation can affect gene prediction and total gene counts (Molina-Mora et al., 2020). To address this, polishing was used as a key step to improve the genome base-pair accuracy to QV50 by mapping long and short reads back to the assembly. Thus, after the short-read correction process, total gene numbers and average CDS length resulted in similar counts and lengths for all the compared genomes, independently of the sequencing technology.
Possible adaptive gene family expansions of S. Montelus
In the present study, 14,986 gene families were identified from the predicted protein-coding genes of S. montelus, and the sequences were downloaded from public databases for relative species. Of these gene families, 3071 single-copy (one-to-one) ortholog gene families were used to construct their phylogeny relationships and divergence times. The results showed that all Papilio species formed a monophyletic group, and S. montelus diverged from the common ancestor of all Papilio species about 58.57–80.46 million years ago (Mya) (Figure 3). All other butterflies, including D. plexippus, H. melpomene, J. coenia, and M. cinxia, formed another single clade, with the moth B. mori forming the basal branch, and diverged about 100.68–119.97 Mya (Figure 3). All these phylogenetic relationships were fully supported by 2000 times of ultrafast bootstrap resampling. Given the number of members for each gene family and the divergence times among these species, the gene family dynamics during evolution history were deduced. For S. montelus, 682 expansion gene families and 371 contraction gene families were found. The enrichment analysis about the genes related to expansion families showed that several KEGG pathways, including ascorbate and aldarate metabolism, retinol metabolism, metabolism of xenobiotics by cytochrome P450, and others, were significantly enriched (Figure 4 and Supplementary Table S7). A total of 98 cytochrome P450 genes in the S. montelus genome (Supplementary Table S8) and 86 cytochrome P450 genes in the P. polytes genome were annotated compared with those in B. mori, which is a major insect model for research (Kawamoto et al., 2019), and another common species of swallowtail butterfly P. polytes, which does not feed on Aristolochia plants. Cytochrome P450 is a large superfamily of heme-thiolate proteins, including clan2, clan3, clan4, and clan mitochondrial subtypes (https://kaikobase.dna.affrc.go.jp/curation/P450.html), in the S. montelus genome (Fig. S5). In the present study, clan3 showed a large expansion (Table 5). P450 expansions mainly occurred from gene duplication (Cheng et al., 2017). The large P450 cluster from clan3, which contained nine CYP332A1 genes, was located in a cluster on the same chromosome (Supplementary Figure S6).
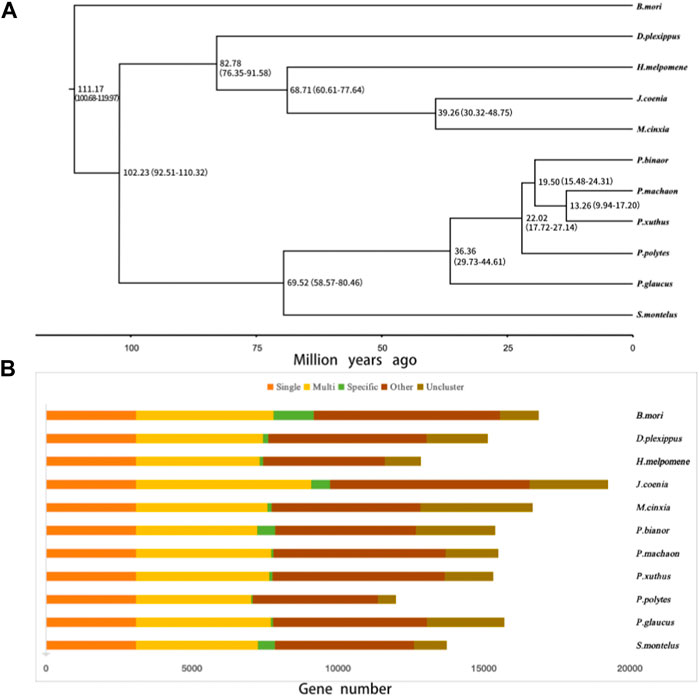
FIGURE 3. Phylogenetic tree and the orthogroup based on S. montelus and relative species. (A) Phylogenetic tree of S. montelus with other species using 3071 single-copy ortholog genes. (B) Orthogroup between S. montelus and relative species. Single means single-copy orthologous genes; Multi means multiple-copy orthologous genes; Specific means genes from a unique gene family; Other means genes that do not belong to Single, Multi, or Specific; and Uncluster means unclustered genes.
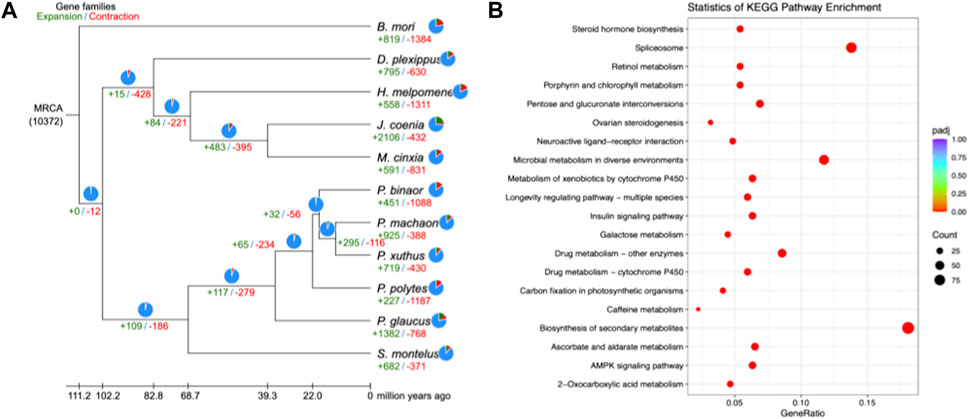
FIGURE 4. Gene family dynamics in S. montelus and relative species. (A) Expansions and contractions of gene families residing in S. montelus and its relatives. The green color represents expansion gene families and numbers. The red color represents contraction gene families and numbers. (B) Top 20 pathways with members of gene families enriched in S. montelus.
The P450 enzymes are a diverse class of genes that play important roles in the metabolism of endogenous and foreign chemicals (Feyereisen, 1999). Thus, the members of the insect P450 superfamily may participate in the adaptation processes, including insecticide resistance and tolerance to plant toxins (Scott et al., 1998). In this sense, AAs have been demonstrated to be highly correlated with aristolochic acid nephropathy and even associated urothelial malignancies; in mammals such as mice and rats, hepatic cytochrome P450s may contribute to the detoxication of these plant toxins (Stiborova et al., 2011; Luan et al., 2015). Therefore, given that the larvae of S. montelus mainly fed on Aristolochia, the significant expansion of P450 members in this species to increase the tolerance level was not unexpected. The immunogold signals showed that AAs were observed all over the cells of mouse renal tubules and accumulated in the mitochondria and peroxisomes (Li et al., 2014). Thus, the significant expansion of genes related to peroxisomes might help attenuate the toxic effects of AAs. The impact of AAs on other pathways, such as galactose metabolism (Nie et al., 2015; Hu et al., 2017), caffeine metabolism (Nie et al., 2015), and MAPK signaling pathway (Chen et al., 2019), have also been reported. Although the molecular mechanisms were not well known, it was hypothesized that the expansion of family members associated with these pathways might be related to AA tolerance. For transcriptome analysis, mRNAs in different stages (egg, larva, pupa, and adult), including P450 gene families, were quantified. The results showed that 47 of 98 P450 genes had a higher expression level in the larval stage compared with the other stages (Supplementary Figure S7). Of the 47 higher-expression genes, 37 were significantly overexpressed, including 19 CYP3 clan genes, 10 CYP4 clan genes, 5 mitochondrial clan P450 genes, and 3 CYP2 clan genes.
Subsequently, a positive selection analysis was conducted. Positive selection is the process by which new advantageous genetic variants sweep a population. Thus, possible amino-acid adaptations residing in the protein-coding genes within the S. montelus genome were identified. Using the best BLAST hit strategy, 13,081 possible ortholog groups were found among these species. By adopting the branch-site model with 8474 orthologous genes presented in S. montelus, 115 of these orthologous genes were identified to be probably subject to positive selection during the adaptation of S. montelus (Supplementary Table S9). The functional analysis showed that these genes, including ppib (peptidyl-prolyl cis-trans isomerase B), qtrt1 (queuine tRNA-ribosyltransferase), and rps4 (small subunit ribosomal protein S4e), might participate in several KEGG pathways, such as Notch signaling pathway, Toll and Imd signaling pathway, and amino sugar and nucleotide sugar metabolism. However, the enrichment analysis showed that no other pathways were significantly enriched (Supplementary Table S10).
Several genes in the list of 115 elements under positive selection were reported to be involved in AA exposure in previous studies on mammals. The elongin A (eloa) and HEAT repeat containing 1 (heatr1) could be altered by the overexposure of human renal epithelial cells to AAs (Li et al., 2021). In this case, the expression levels of eloa increased after treatment with AA, unlike heatr1, which resulted in an underexpression. Moreover, in the same study, the expression level of aph1b, which should be one of the homologous genes of gamma-secretase subunit APH-1A (aph1a), also increased. Therefore, these genes may be related to the interactions between the organism and AAs (Li et al., 2021). The directional alterations in the metabolism of some amino acids may impact the efficacy of promoting tolerance to AAs (Stepka et al., 2021). For example, the eukaryotic transcription process mediated by RNA polymerase II (pol II) may be paused by several situations such as DNA damage (Van Houten and Kisker, 2014). In this case, the elongin complex can mediate the ubiquitylation and degradation of the largest subunit of pol II (Rpb1) to stimulate transcription elongation (Yasukawa et al., 2008). The elongin is composed of two small regulatory B and C subunits and a transcriptionally active A subunit, which is coded by eloa. In vivo, AAs can be reduced to an electrophilic cyclic N-acylnitrenium ion and preferentially form DNA adducts and cause damage (Schmeiser et al., 2009). Therefore, it was presumed that the modifications of two amino acids in the coil regions of the A subunit (Supplementary Figure S8) might promote the efficacy of the elongin and accelerate the DNA-damage-induced ubiquitylation and subsequent degradation of the Rpb1 complex. The γ-secretase complex participated in the cleavage of amyloid precursor protein into amyloid beta-peptide (Aβ), and the protein Aph1 encoded by aph1 was an important scaffold protein in the γ-secretase complex (Watanabe et al., 2022). The functional analysis showed that the γ-secretase complex containing Aph1b produced more Aβ42 than the complex containing Aph1a (Serneels et al., 2009), and the Aph1 mutant analysis suggested the regulatory function of Aph1 in γ-secretase activity (Watanabe et al., 2022). Two mutations within the helix regions of Aph1a detected in S. montelus might enhance the catalytic activity of the γ-secretase complex (Supplementary Figure S8), although the adaptative mechanisms in AA endurance are yet unknown. Heart1 may act as an integrative hub of pre-rRNA transcription and processing (Prieto and McStay, 2007). The downregulation of heatr1 can lead to the increased expression of p53 (Turi et al., 2018), which is a critical gene in carcinogenesis and frequently mutated in AA-induced urothelial tumors (Slade et al., 2009). It was hypothesized that positive selection might alter the efficiency of the genes and lead to relatively stable p53 expression levels in S. montelus, as the activation of p53 might promote renal injury in acute AA nephropathy (Zhou et al., 2010). However, these regulatory relationships were only testified in mammals. The actual molecular events in insects, such as S. montelus, warrant further investigations.
Conclusion
This study reported a high-quality ornamental butterfly genome S. montelus with Nanopore long reads sequencing and Hi-C scaffolding technologies. The relationships and divergence times between S. montelus and relative species were identified based on the de novo assembly. S. montelus was separated from the common ancestor of swallowtails about 58.57–80.46 Mya. A comparison of the protein-coding genes in the species and relatives showed that a series of adaptations might happen in the S. montelus genome. The gene family expansion and expression might highlight this as an area for future research on the adaptation to the feeding habits of the larval phase of the species. Three of the 115 genes in previous studies with AA tolerance in mammals were positively selected in S. montelus. Thus, this study provided not only novel biological insights but also useful butterfly data for further genetic and comparative genomics analyses with this species or closely related ones.
Data availability statement
The datasets presented in this study can be found in online repositories. The names of the repository/repositories and accession number(s) can be found in the article/Supplementary Material.
Author contributions
YL, YZ, and LS conceived, designed, and supervised the study. JL, HW, QY, and JZ performed the sample collection and data analysis. JL, HW, and JM. drafted the manuscript and participated in the revision. All authors contributed to the manuscript and approved this final version of the manuscript for publication.
Conflict of interest
The authors declare that the research was conducted in the absence of any commercial or financial relationships that could be construed as a potential conflict of interest.
Publisher’s note
All claims expressed in this article are solely those of the authors and do not necessarily represent those of their affiliated organizations, or those of the publisher, the editors and the reviewers. Any product that may be evaluated in this article, or claim that may be made by its manufacturer, is not guaranteed or endorsed by the publisher.
Supplementary material
The Supplementary Material for this article can be found online at: https://www.frontiersin.org/articles/10.3389/fgene.2023.1107353/full#supplementary-material
References
Arlt, V. M., Stiborova, M., and Schmeiser, H. H. (2002). Aristolochic acid as a probable human cancer hazard in herbal remedies: A review. Mutagenesis 17, 265–277. doi:10.1093/mutage/17.4.265
Bao, W., Kojima, K. K., and Kohany, O. (2015). Repbase Update, a database of repetitive elements in eukaryotic genomes. Mob. DNA 6, 11. doi:10.1186/s13100-015-0041-9
Belaghzal, H., Dekker, J., and Gibcus, J. H. (2017). Hi-C 2.0: An optimized Hi-C procedure for high-resolution genome-wide mapping of chromosome conformation. Methods 123, 56–65. doi:10.1016/j.ymeth.2017.04.004
Benjamini, Y., and Hochberg, Y. (1995). Controlling the false discovery rate: A practical and powerful approach to multiple testing. J. R. Stat. Soc. Ser. B Methodol. 57, 289–300. doi:10.1111/j.2517-6161.1995.tb02031.x
Benson, G. (1999). Tandem repeats finder: A program to analyze DNA sequences. Nucleic Acids Res. 27, 573–580. doi:10.1093/nar/27.2.573
Bouatta, N., Sorger, P., and AlQuraishi, M. (2021). Protein structure prediction by AlphaFold2: Are attention and symmetries all you need? Acta Crystallogr. D. Struct. Biol. 77, 982–991. doi:10.1107/S2059798321007531
Burton, J. N., Adey, A., Patwardhan, R. P., Qiu, R., Kitzman, J. O., and Shendure, J. (2013). Chromosome-scale scaffolding of de novo genome assemblies based on chromatin interactions. Nat. Biotechnol. 31, 1119–1125. doi:10.1038/nbt.2727
Castresana, J. (2000). Selection of conserved blocks from multiple alignments for their use in phylogenetic analysis. Mol. Biol. Evol. 17, 540–552. doi:10.1093/oxfordjournals.molbev.a026334
Chan, P. P., Lin, B. Y., Mak, A. J., and Lowe, T. M. (2021). tRNAscan-SE 2.0: improved detection and functional classification of transfer RNA genes. Nucleic Acids Res. 49, 9077–9096. doi:10.1093/nar/gkab688
Chen, C. H., Dickman, K. G., Moriya, M., Zavadil, J., Sidorenko, V. S., Edwards, K. L., et al. (2012). Aristolochic acid-associated urothelial cancer in Taiwan. Proc. Natl. Acad. Sci. U. S. A. 109, 8241–8246. doi:10.1073/pnas.1119920109
Chen, I. H., Luo, H. L., Su, Y. L., Huang, C. C., Chiang, P. H., Yu, C. C., et al. (2019). Aristolochic acid affects upper tract urothelial cancer behavior through the MAPK pathway. Molecules 24, 3707. doi:10.3390/molecules24203707
Chen, S. H., Rossetto, M., van der Merwe, M., Lu-Irving, P., Yap, J. S., Sauquet, H., et al. (2022). Chromosome-level de novo genome assembly of Telopea speciosissima (New South Wales waratah) using long-reads, linked-reads and Hi-C. Mol. Ecol. Resour. 22, 1836–1854. doi:10.1111/1755-0998.13574
Chen, S., Zhou, Y., Chen, Y., and Gu, J. (2018). fastp: an ultra-fast all-in-one FASTQ preprocessor. Bioinformatics 34, i884–i890. doi:10.1093/bioinformatics/bty560
Cheng, T., Wu, J., Wu, Y., Chilukuri, R. V., Huang, L., Yamamoto, K., et al. (2017). Genomic adaptation to polyphagy and insecticides in a major East Asian noctuid pest. Nat. Ecol. Evol. 1, 1747–1756. doi:10.1038/s41559-017-0314-4
Daub, J., Eberhardt, R. Y., Tate, J. G., and Burge, S. W. (2015). Rfam: Annotating families of non-coding RNA sequences. Methods Mol. Biol. 1269, 349–363. doi:10.1007/978-1-4939-2291-8_22
De Bie, T., Cristianini, N., Demuth, J. P., and Hahn, M. W. (2006). Cafe: A computational tool for the study of gene family evolution. Bioinformatics 22, 1269–1271. doi:10.1093/bioinformatics/btl097
Dobin, A., Davis, C. A., Schlesinger, F., Drenkow, J., Zaleski, C., Jha, S., et al. (2013). Star: Ultrafast universal RNA-seq aligner. Bioinformatics 29, 15–21. doi:10.1093/bioinformatics/bts635
Espeland, M., Breinholt, J., Willmott, K. R., Warren, A. D., Vila, R., Toussaint, E. F. A., et al. (2018). A comprehensive and dated phylogenomic analysis of butterflies. Curr. Biol. 28, 770–778. doi:10.1016/j.cub.2018.01.061
Feyereisen, R. (1999). Insect P450 enzymes. Annu. Rev. Entomol. 44, 507–533. doi:10.1146/annurev.ento.44.1.507
Flynn, J. M., Hubley, R., Goubert, C., Rosen, J., Clark, A. G., Feschotte, C., et al. (2020). RepeatModeler2 for automated genomic discovery of transposable element families. Proc. Natl. Acad. Sci. U. S. A. 117, 9451–9457. doi:10.1073/pnas.1921046117
Ge, H., Lin, K., Shen, M., Wu, S., Wang, Y., Zhang, Z., et al. (2019). De novo assembly of a chromosome-level reference genome of red-spotted grouper (Epinephelus akaara) using nanopore sequencing and Hi-C. Mol. Ecol. Resour. 19, 1461–1469. doi:10.1111/1755-0998.13064
Haas, B. J., Salzberg, S. L., Zhu, W., Pertea, M., Allen, J. E., Orvis, J., et al. (2008). Automated eukaryotic gene structure annotation using EVidenceModeler and the Program to Assemble Spliced Alignments. Genome Biol. 9, R7. doi:10.1186/gb-2008-9-1-r7
Han, Y., and Wessler, S. R. (2010). MITE-hunter: A program for discovering miniature inverted-repeat transposable elements from genomic sequences. Nucleic Acids Res. 38, e199. doi:10.1093/nar/gkq862
Heikkila, M., Kaila, L., Mutanen, M., Pena, C., and Wahlberg, N. (2012). Cretaceous origin and repeated tertiary diversification of the redefined butterflies. Proc. Biol. Sci. 279, 1093–1099. doi:10.1098/rspb.2011.1430
Hu, J., Fan, J., Sun, Z., and Liu, S. (2020). NextPolish: A fast and efficient genome polishing tool for long-read assembly. Bioinformatics 36, 2253–2255. doi:10.1093/bioinformatics/btz891
Hu, X., Shen, J., Pu, X., Zheng, N., Deng, Z., Zhang, Z., et al. (2017). Urinary time- or dose-dependent metabolic biomarkers of aristolochic acid-induced nephrotoxicity in rats. Toxicol. Sci. 156, 123–132. doi:10.1093/toxsci/kfw244
Kalvari, I., Argasinska, J., Quinonesolvera, N., Nawrocki, E. P., Rivas, E., Eddy, S. R., et al. (2017). Rfam 13.0: Shifting to a genome-centric resource for non-coding RNA families. Nucleic Acids Res. 46, D335–D342. doi:10.1093/nar/gkx1038
Katoh, K., Misawa, K., Kuma, K., and Miyata, T. (2002). Mafft: A novel method for rapid multiple sequence alignment based on fast fourier transform. Nucleic Acids Res. 30, 3059–3066. doi:10.1093/nar/gkf436
Kawahara, A. Y., and Breinholt, J. W. (2014). Phylogenomics provides strong evidence for relationships of butterflies and moths. Proc. Biol. Sci. 281, 20140970. doi:10.1098/rspb.2014.0970
Kawamoto, M., Jouraku, A., Toyoda, A., Yokoi, K., Minakuchi, Y., Katsuma, S., et al. (2019). High-quality genome assembly of the silkworm, Bombyx mori. Insect Biochem. Mol. Biol. 107, 53–62. doi:10.1016/j.ibmb.2019.02.002
Keilwagen, J., Hartung, F., and Grau, J. (2019). GeMoMa: Homology-Based gene prediction utilizing intron position conservation and RNA-seq data. Methods Mol. Biol. 1962, 161, doi:10.1007/978-1-4939-9173-0_9
Kim, D., Paggi, J. M., Park, C., Bennett, C., and Salzberg, S. L. (2019). Graph-based genome alignment and genotyping with HISAT2 and HISAT-genotype. Nat. Biotechnol. 37, 907–915. doi:10.1038/s41587-019-0201-4
Kitts, P. A., Church, D. M., Thibaud-Nissen, F., Choi, J., Hem, V., Sapojnikov, V., et al. (2015). Assembly: A resource for assembled genomes at NCBI. Nucleic Acids Res. 44, D73–D80. doi:10.1093/nar/gkv1226
Koren, S., Phillippy, A. M., Simpson, J. T., Loman, N. J., and Loose, M. (2019). Reply to 'Errors in long-read assemblies can critically affect protein prediction. Nat. Biotechnol. 37, 127–128. doi:10.1038/s41587-018-0005-y
Kumar, S., Stecher, G., Suleski, M., and Hedges, S. B. (2017). TimeTree: A resource for timelines, timetrees, and divergence times. Mol. Biol. Evol. 34, 1812–1819. doi:10.1093/molbev/msx116
Lafontaine, D. L., Yang, L., Dekker, J., and Gibcus, J. H. (2021). Hi-C 3.0: Improved protocol for genome-wide chromosome conformation capture. Curr. Protoc. 1, e198. doi:10.1002/cpz1.198
Lagesen, K., Hallin, P., Rodland, E. A., Staerfeldt, H. H., Rognes, T., and Ussery, D. W. (2007). RNAmmer: Consistent and rapid annotation of ribosomal RNA genes. Nucleic Acids Res. 35, 3100–3108. doi:10.1093/nar/gkm160
Lebeau, C., Arlt, V. M., Schmeiser, H. H., Boom, A., Verroust, P. J., Devuyst, O., et al. (2001). Aristolochic acid impedes endocytosis and induces DNA adducts in proximal tubule cells. Kidney Int. 60, 1332–1342. doi:10.1046/j.1523-1755.2001.00938.x
Li, H. (2011). A statistical framework for SNP calling, mutation discovery, association mapping and population genetical parameter estimation from sequencing data. Bioinformatics 27, 2987–2993. doi:10.1093/bioinformatics/btr509
Li, H., and Durbin, R. (2009). Fast and accurate short read alignment with Burrows-Wheeler transform. Bioinformatics 25, 1754–1760. doi:10.1093/bioinformatics/btp324
Li, H., Handsaker, B., Wysoker, A., Fennell, T., Ruan, J., Homer, N., et al. (2009). The sequence alignment/map format and SAMtools. Bioinformatics 25, 2078–2079. doi:10.1093/bioinformatics/btp352
Li, H. (2018). Minimap2: Pairwise alignment for nucleotide sequences. Bioinformatics 34, 3094–3100. doi:10.1093/bioinformatics/bty191
Li, L., Stoeckert, C. J., and Roos, D. S. (2003). OrthoMCL: Identification of ortholog groups for eukaryotic genomes. Genome Res. 13, 2178–2189. doi:10.1101/gr.1224503
Li, X., Luo, Y., Yang, H., Yang, Q., Settele, J., and Schweiger, O. (2016). On the ecology and conservation of Sericinus montelus (Lepidoptera: Papilionidae) - its threats in xiaolongshan forests area (China). PLoS One 11, e0150833. doi:10.1371/journal.pone.0150833
Li, X. W., Yokota, S., Wang, D., Wang, X., Shoyama, Y., and Cai, S. Q. (2014). Localization of aristolochic acid in mouse kidney tissues by immunohistochemistry using an anti-AA-I and AA-II monoclonal antibody. Am. J. Chin. Med. 42, 1453–1469. doi:10.1142/S0192415X14500918
Li, Y., Xu, H., Cai, D., Zhu, S., Liu, X., Zhao, Y., et al. (2021). Integration of transcriptomic, proteomic and metabolomic data to reveal the biological mechanisms of AAI injury in renal epithelial cells. Toxicol Vitro 70, 105054. doi:10.1016/j.tiv.2020.105054
Liu, H., Wu, S., Li, A., and Ruan, J. (2021). SMARTdenovo: A de novo assembler using long noisy reads. Gigabyte 1, 1–9. doi:10.46471/gigabyte.15
Lu, S., Yang, J., Dai, X., Xie, F., He, J., Dong, Z., et al. (2019). Chromosomal-level reference genome of Chinese peacock butterfly (Papilio bianor) based on third-generation DNA sequencing and Hi-C analysis. Gigascience 8, giz128. doi:10.1093/gigascience/giz128
Luan, Y., Xing, G., Ren, J., and Gu, J. (2015). Role of hepatic cytochrome P450 enzymes in the detoxication of aristolochic acid I; effects on DNA adduct, mutation, and tumor formation. Genes Environ. 37, 11. doi:10.1186/s41021-015-0010-z
Manni, M., Berkeley, M. R., Seppey, M., and Zdobnov, E. M. (2021). BUSCO: Assessing genomic data quality and beyond. Curr. Protoc. 1, e323. doi:10.1002/cpz1.323
Marcais, G., and Kingsford, C. (2011). A fast, lock-free approach for efficient parallel counting of occurrences of k-mers. Bioinformatics 27, 764–770. doi:10.1093/bioinformatics/btr011
Molina-Mora, J. A., Campos-Sanchez, R., Rodriguez, C., Shi, L., and Garcia, F. (2020). High quality 3C de novo assembly and annotation of a multidrug resistant ST-111 Pseudomonas aeruginosa genome: Benchmark of hybrid and non-hybrid assemblers. Sci. Rep. 10, 1392. doi:10.1038/s41598-020-58319-6
Mount, D. W. (2007). Using the basic local alignment search tool (BLAST). CSH Protoc. 2007, pdb.top17, doi:10.1101/pdb.top17
Nawrocki, E. P. (2014). Annotating functional RNAs in genomes using Infernal. Methods Mol. Biol. 1097, 163–197. doi:10.1007/978-1-62703-709-9_9
Ng, P. C., and Kirkness, E. F. (2010). Whole genome sequencing. Methods Mol. Biol. 628, 215–226. doi:10.1007/978-1-60327-367-1_12
Nie, W., Lv, Y., Yan, L., Chen, X., and Lv, H. (2015). Prediction and characterisation of the system effects of aristolochic acid: A novel joint network analysis towards therapeutic and toxicological mechanisms. Sci. Rep. 5, 17646. doi:10.1038/srep17646
Nurk, S., Koren, S., Rhie, A., Rautiainen, M., Bzikadze, A. V., Mikheenko, A., et al. (2022). The complete sequence of a human genome. Science 376, 44–53. doi:10.1126/science.abj6987
Pertea, M., Pertea, G. M., Antonescu, C. M., Chang, T. C., Mendell, J. T., and Salzberg, S. L. (2015). StringTie enables improved reconstruction of a transcriptome from RNA-seq reads. Nat. Biotechnol. 33, 290–295. doi:10.1038/nbt.3122
Prieto, J. L., and McStay, B. (2007). Recruitment of factors linking transcription and processing of pre-rRNA to NOR chromatin is UBF-dependent and occurs independent of transcription in human cells. Genes Dev. 21, 2041–2054. doi:10.1101/gad.436707
Sayers, E. W., Bolton, E. E., Brister, J. R., Canese, K., Chan, J., Comeau, D. C., et al. (2022). Database resources of the national center for biotechnology information. Nucleic Acids Res. 50, D20–D26. doi:10.1093/nar/gkab1112
Schmeiser, H. H., Stiborova, M., and Arlt, V. M. (2009). Chemical and molecular basis of the carcinogenicity of Aristolochia plants. Curr. Opin. Drug Discov. Devel 12, 141–148.
Scott, J. G., Liu, N., and Wen, Z. (1998). Insect cytochromes P450: Diversity, insecticide resistance and tolerance to plant toxins. Comp. Biochem. Physiol. C Pharmacol. Toxicol. Endocrinol. 121, 147–155. doi:10.1016/s0742-8413(98)10035-x
Serneels, L., Van Biervliet, J., Craessaerts, K., Dejaegere, T., Horre, K., Van Houtvin, T., et al. (2009). gamma-Secretase heterogeneity in the Aph1 subunit: relevance for Alzheimer's disease. Science 324, 639–642. doi:10.1126/science.1171176
Servant, N., Varoquaux, N., Lajoie, B. R., Viara, E., Chen, C. J., Vert, J. P., et al. (2015). HiC-pro: An optimized and flexible pipeline for Hi-C data processing. Genome Biol. 16, 259. doi:10.1186/s13059-015-0831-x
Slade, N., Moll, U. M., Brdar, B., Zorić, A., and Jelaković, B. (2009). p53 mutations as fingerprints for aristolochic acid: an environmental carcinogen in endemic (Balkan) nephropathy. Mutat. Res. 663, 1–6. doi:10.1016/j.mrfmmm.2009.01.005
Stamatakis, A. (2015). Using RAxML to infer phylogenies. Curr. Protoc. Bioinforma. 51 (6 14 11), 1–6. doi:10.1002/0471250953.bi0614s51
Stanke, M., Keller, O., Gunduz, I., Hayes, A., Waack, S., and Morgenstern, B. (2006). Augustus: Ab initio prediction of alternative transcripts. Nucleic Acids Res. 34, W435–W439. doi:10.1093/nar/gkl200
Stepka, P., Vsiansky, V., Raudenska, M., Gumulec, J., Adam, V., and Masarik, M. (2021). Metabolic and amino acid alterations of the tumor microenvironment. Curr. Med. Chem. 28, 1270–1289. doi:10.2174/0929867327666200207114658
Stiborova, M., Mares, J., Levova, K., Pavlickova, J., Barta, F., Hodek, P., et al. (2011). Role of cytochromes P450 in metabolism of carcinogenic aristolochic acid I: Evidence of their contribution to aristolochic acid I detoxication and activation in rat liver. Neuro Endocrinol. Lett. 32 (1), 121–130.
Tarailo-Graovac, M., and Chen, N. (2009). Using RepeatMasker to identify repetitive elements in genomic sequences. Curr. Protoc. Bioinforma. Chapter 4, Unit 4.10. doi:10.1002/0471250953.bi0410s25
Turi, Z., Senkyrikova, M., Mistrik, M., Bartek, J., and Moudry, P. (2018). Perturbation of RNA Polymerase I transcription machinery by ablation of HEATR1 triggers the RPL5/RPL11-MDM2-p53 ribosome biogenesis stress checkpoint pathway in human cells. Cell Cycle 17, 92–101. doi:10.1080/15384101.2017.1403685
Van Houten, B., and Kisker, C. (2014). Transcriptional pausing to scout ahead for DNA damage. Proc. Natl. Acad. Sci. U. S. A. 111, 3905–3906. doi:10.1073/pnas.1402020111
Vurture, G. W., Sedlazeck, F. J., Nattestad, M., Underwood, C. J., Fang, H., Gurtowski, J., et al. (2017). GenomeScope: Fast reference-free genome profiling from short reads. Bioinformatics 33, 2202–2204. doi:10.1093/bioinformatics/btx153
Wang, X., and Wang, L. (2016). Gmata: An integrated software package for genome-scale SSR mining, marker development and viewing. Front. Plant Sci. 7, 1350. doi:10.3389/fpls.2016.01350
Watanabe, H., Yoshida, C., Hidaka, M., Ogawa, T., Tomita, T., and Futai, E. (2022). Specific mutations in Aph1 cause gamma-secretase activation. Int. J. Mol. Sci. 23, 507. doi:10.3390/ijms23010507
Wu, T., Hu, E., Xu, S., Chen, M., Guo, P., Dai, Z., et al. (2021). clusterProfiler 4.0: A universal enrichment tool for interpreting omics data. Innov. (Camb) 2, 100141. doi:10.1016/j.xinn.2021.100141
Yang, J., Wan, W., Xie, M., Mao, J., Dong, Z., Lu, S., et al. (2020). Chromosome-level reference genome assembly and gene editing of the dead-leaf butterfly Kallima inachus. Mol. Ecol. Resour. 20, 1080–1092. doi:10.1111/1755-0998.13185
Yang, Z. (2007). Paml 4: Phylogenetic analysis by maximum likelihood. Mol. Biol. Evol. 24, 1586–1591. doi:10.1093/molbev/msm088
Yasukawa, T., Kamura, T., Kitajima, S., Conaway, R. C., Conaway, J. W., and Aso, T. (2008). Mammalian Elongin A complex mediates DNA-damage-induced ubiquitylation and degradation of Rpb1. EMBO J. 27, 3256–3266. doi:10.1038/emboj.2008.249
Keywords: sericinus montelus, aristolochic acid tolerance, genome assembly, oxford nanopore sequencing, hi-c
Citation: Li J, Wang H, Zhu J, Yang Q, Luan Y, Shi L, Molina-Mora JA and Zheng Y (2023) De novo assembly of a chromosome-level reference genome of the ornamental butterfly Sericinus montelus based on nanopore sequencing and Hi-C analysis. Front. Genet. 14:1107353. doi: 10.3389/fgene.2023.1107353
Received: 24 November 2022; Accepted: 27 February 2023;
Published: 08 March 2023.
Edited by:
Eugenia Poliakov, National Eye Institute (NIH), United StatesReviewed by:
Richard John Edwards, University of New South Wales, AustraliaXinhai Ye, Zhejiang University, China
Copyright © 2023 Li, Wang, Zhu, Yang, Luan, Shi, Molina-Mora and Zheng. This is an open-access article distributed under the terms of the Creative Commons Attribution License (CC BY). The use, distribution or reproduction in other forums is permitted, provided the original author(s) and the copyright owner(s) are credited and that the original publication in this journal is cited, in accordance with accepted academic practice. No use, distribution or reproduction is permitted which does not comply with these terms.
*Correspondence: José Arturo Molina-Mora, am9zZS5tb2xpbmFtb3JhQHVjci5hYy5jcg==; Yuanting Zheng, emhlbmd5dWFudGluZ0BmdWRhbi5lZHUuY24=
†These authors have contributed equally to this work