- 1Department of Clinical Laboratory, The First Affiliated Hospital of USTC, School of Basic Medical Sciences, Division of Life Science and Medicine, University of Science and Technology of China (UTSC), Hefei, Anhui, China
- 2Department of Pulmonary and Critical Care Medicine, Regional Medical Center for National Institute of Respiratory Diseases, Sir Run Run Shaw Hospital, School of Medicine, Zhejiang University, Hangzhou, China
Mammalian cells employ various adaptive responses to cope with multiple stresses to maintain homeostasis. Functional roles of non-coding RNAs (ncRNAs) in response to cellular stresses have been proposed, and systematical investigations about the crosstalk among distinct types of RNAs are required. Here, we challenged HeLa cells with thapsigargin (TG) and glucose deprivation (GD) treatments to induce endoplasmic reticulum (ER) and metabolic stresses, respectively. Ribosomal RNA (rRNA)-depleted RNA sequencing (RNA-seq) was then performed. Characterization of the RNA-seq data revealed a series of differentially expressed long non-coding RNAs (lncRNAs) and circular RNAs (circRNAs) with parallel changes responsive to both stimuli. We further constructed the lncRNA/circRNA-mRNA co-expressing network, competing endogenous RNA (ceRNA) network in the lncRNA/circRNA-miRNA-mRNA axis, and lncRNA/circRNA-RNA binding protein (RBP) interactome map. These networks indicated the potential cis and/or trans regulatory roles of lncRNAs and circRNAs. Moreover, Gene Ontology analysis demonstrated that these identified ncRNAs were associated with several essential biological processes known to be related to cellular stress responses. In conclusion, we systematically established functional regulatory networks of lncRNA/circRNA-mRNA, lncRNA/circRNA-miRNA-mRNA and lncRNA/circRNA-RBP to perceive the potential interactions and biological processes during cellular stresses. These results provided insights in ncRNA regulatory networks of stress responses and the basis for further identification of pivotal factors involved in cellular stress responses.
Introduction
Eukaryotic cells possess an extraordinary capacity to adapt to extra- or intracellular stimuli such as endoplasmic reticulum (ER) stress, heat shock, UV light, oxidative stress and nutrient starvation (Pakos-Zebrucka et al., 2016; Galluzzi et al., 2018; Bhardwaj et al., 2020). To cope with multiple stimuli, cells activate distinct cellular responses including the regulation of gene transcription, the DNA damage response (DDR), the unfolded protein response (UPR), mitochondria stress signaling and autophagy (Eisner et al., 2018; Galluzzi et al., 2018; Vihervaara et al., 2018; Hetz et al., 2020; Larsen et al., 2022). Constant exposures to stresses contribute to various diseases, such as diabetes mellitus, neurodegeneration, cardiovascular disorders and cancers (Urra et al., 2016; Ren et al., 2021; Larsen et al., 2022). Although a range of factors play potent roles in cellular stress responses, and their interactions have been established, the construction of distinct RNA regulatory networks is still in demand (Ermolaeva and Schumacher, 2014; Frakes and Dillin, 2017; Galluzzi et al., 2018; Melber and Haynes, 2018).
ER stress is characterized by the accumulation of unfolded or misfolded proteins in the ER lumen, which triggers the UPR to restore protein homeostasis (Urra et al., 2016; Hetz et al., 2020). Several chemicals including thapsigargin (TG), tunicamycin and dithiothreitol, could induce ER stress and activate the UPR (Feng et al., 2014; Keestra-Gounder et al., 2016). Under ER stress, mammalian cells make efforts in cellular responses via three distinct stress sensors: IRE1α, ATF6, and PERK (Hetz et al., 2020). For example, the activated IRE1α selectively splices the XBP1 mRNA to generate XBP1s, and XBP1s protein could regulate the transcription of target genes that are involved in protein folding (Yoshida et al., 2001; Hetz et al., 2020). Glucose deprivation (GD) is another stress that is accompanied by the metabolic oxidative stress, as glucose is the major energy source that generates ATP via glycolysis or mitochondrial oxidative metabolism (Zhang C. S. et al., 2017; Ren and Shen, 2019). Upon GD treatment, some primary metabolic pathways are quickly triggered to recover energy homeostasis and promote cell survival (Le et al., 2012; Li et al., 2022). For example, AMP-activated protein kinase (AMPK) mediated catabolism is activated, and the mammalian target of rapamycin (mTOR) mediated anabolism is decreased in response to GD-induced metabolic changes (Zhang C. S. et al., 2017; González et al., 2020).
In mammals, the majority of the genome is transcribed into a variety of non-coding RNAs (ncRNAs) with regulatory roles in physiology and diseases (Hu and Shan, 2016; Quinn and Chang, 2016; Wang et al., 2022). Lines of evidence have demonstrated that ncRNAs are indispensable regulators in various biological processes including transcriptional regulation, modulating alternative splicing, chromatin remodeling, and protein transportation (Quinn and Chang, 2016; Wang et al., 2022). MicroRNAs (miRNAs) are small ncRNAs that function mainly by binding to the 3′ untranslated regions (3′ UTRs) of targets to repress translation (Bartel, 2004; Jonas and Izaurralde, 2015). For example, miR-3648 is induced under ER stress and decreases the APC2 level to promote cell proliferation (Rashid et al., 2017). Additionally, several miRNAs could exert their functions by targeting the 5’ UTRs or coding regions of the corresponding genes (Liang et al., 2014; Li et al., 2016; Wang et al., 2022). Long ncRNAs (lncRNAs) are endogenously expressed RNA transcripts longer than 200 nucleotides (Quinn and Chang, 2016; Sun et al., 2018). LncRNAs have a broad range of biological functions, such as modulating alternative splicing and regulating translation (Quinn and Chang, 2016; Sun et al., 2018; Wang et al., 2022). The lncRNA LASTR is upregulated in hypoxic breast cancer and increases the fitness of breast cancer cells by regulating the activity of the U4/U6 recycling factor SART3 (De Troyer et al., 2020). The lncRNAs such as 5S-OT and MALAT1 modulate alternative splicing (Hu et al., 2016; Zhang X. et al., 2017). The lncRNA Caren represses the translation of Hint1 to inactivate DDR and activate mitochondrial biogenesis to antagonize heart failure (Sato et al., 2021). Circular RNAs (circRNAs) are covalently closed RNA molecules that are generated by back-splicing or other RNA circularization mechanisms (Kristensen et al., 2019; Liu et al., 2020; Chen et al., 2022). Most circRNAs are thought to be non-coding and exert their functions by mechanisms such as acting as miRNA sponges, modulating RNA binding proteins (RBPs), and regulating gene transcription (Hansen et al., 2013; Memczak et al., 2013; Li et al., 2015; Yang et al., 2017; Kristensen et al., 2019; Gao et al., 2020; Liu et al., 2020; Wang et al., 2021; Chen et al., 2022). The circRNA cPWWP2A retards diabetes-induced microvascular dysfunction by sequestering miR-579 from its targets, angiopoietin 1, occludin, and SIRT1 (Liu et al., 2019). CircACC1 is upregulated during metabolic stress and enhances the enzymatic activity of AMPK to modulate both glycolysis and fatty acid β-oxidation (Li et al., 2019). Accumulating evidence has demonstrated that ncRNAs could play diverse roles through forming complex regulatory networks including feedback loops, ceRNA networks, co-expressed networks, and RNA-protein complexes (Fu, 2014; Anastasiadou et al., 2018). For example, Kleaveland and colleagues characterized a ceRNA network centered on four ncRNAs—one lncRNA (Cyrano), one circRNA (CDR1as), and two miRNAs (miR-7 and miR-671) by using a panel of mouse knockouts (Kleaveland et al., 2018). Additionally, miR-143 and miR-145 are co-expressed miRNAs that have been extensively studied as potential tumor suppressors (Kent et al., 2014). The well-characterized lncRNA NEAT1, binds to various proteins such as TDP-43, KCNAB2, and WDR5, to exert its functional roles (Ahmed et al., 2018; An et al., 2018). Although several classes of ncRNAs including miRNAs, lncRNAs and circRNAs have been reported to play vital roles in response to cellular stresses (Leung and Sharp, 2010; Quinn and Chang, 2016; Chen et al., 2022), identifying more functional ncRNAs and constructing the interacted networks would provide further insights into stress responses.
Numerous studies have focused on one particular cellular stress (Galluzzi et al., 2018; González-Quiroz et al., 2020; Larsen et al., 2022), and to investigate functional ncRNAs in response to more than one stress condition, we performed high-throughput RNA sequencing (RNA-seq) of HeLa cells under TG or GD treatment, and identified differentially expressed lncRNAs and circRNAs in response to stresses. Then, we further established lncRNAs and circRNAs associated networks to characterize key regulators and provide novel insights into cellular stress responses.
Materials and methods
Cell culture
HeLa cells were purchased from the American Type Culture Collection (ATCC, http://www.atcc.org) and authenticated by short-tandem-repeat (STR) profiling. They were cultured under standard conditions with DMEM (Gibco, 11995065) containing 10% FBS (CLARK, FB25015), and 1% penicillin/streptomycin (Beyotime, C0222) at 37°C with 5% CO2. HeLa cells were determined with a PCR-based method and DAPI staining to ensure no contamination of mycoplasma.
Cellular stress treatments
To induce ER stress, HeLa cells were cultured with the DMEM medium containing 300 nM TG (Sigma, T9033) for 6 h. For glucose deprivation, cells were cultured with DMEM without glucose (Gibco, 11966025) at 37°C for 6 h.
Library preparation for ribo-minus RNA-seq
Total RNA was extracted by TRIzol reagent (Invitrogen, 15596026) according to the manufacturer’s instructions. The concentration and quality of extracted RNAs were verified by Nanodrop and gel electrophoresis, respectively. Libraries were constructed by the TruSeq Ribo Profile Library Prep Kit (Illumina, RPHMR12126) according to the manufacturer’s instructions. In brief, 10 μg total RNA was depleted rRNA with the Illumina Rio-Zero Gold Kit (Illumina, MRZE724) and next purified for end repair and 5′ adaptor ligation. Then, the reverse transcription was performed with random primers containing the 3′ adaptor and randomized hexamer sequences. Finally, complementary DNA (cDNA) was purified and amplified with a Thermal Cycler. The PCR products of 300–500 base pairs (bp) were purified, quantified and stored at −80°C before sequencing. The libraries were subjected to 150-nt paired-end sequencing generating a depth of 50–100 million read pairs with an Illumina Novaseq platform (Novogene).
Transcriptome data analysis
For data processing, the adaptors were trimmed with Cutadapt to obtain clean reads. The data quality was then checked with FastQC and the low-quality (Q value ≤ 20) reads were removed. The remaining reads were subsequently aligned to the human reference genome (hg19) with Bowtie2 (-v 1). For linear RNAs including lncRNAs and mRNAs, the corresponding reads were counted with BEDtools and read per million (RPM) was used to calculate levels for lncRNAs and mRNAs. LncRNAs with an average RPM ≥0.1 were used for further analysis and the DE lncRNAs were determined by DEseq2 with a criterion of fold change ≥2 or ≤0.5 and p-value < 0.05. The DE mRNAs were determined by DEseq2 with the cutoff (the average RPM ≥10, fold change ≥2 or ≤0.5 and p-value < 0.05). For circRNA prediction, find_circ and CIRI2 were applied to identify high-confidence BSJs with default parameters. Only circRNA candidates predicted by both pipelines were used for further investigations and CIRI2-annotated BSJ reads (BSJ reads ≥2) were used to calculate circRNA levels. The DE circRNAs were determined by DEseq2 with the cutoff (fold change ≥2 or ≤0.5, and p-value < 0.05).
PCR reactions
cDNA was synthesized from 500 ng total RNA with the GoScript Reverse Transcription System (Promega, A5000) according to the manufacturer’s protocol. For RT-PCR gels of XBP1 and FST mRNAs, amplification was performed with 30 cycles. Real-time quantitative PCR (RT-qPCR) was carried out with GoTaq SYBR Green qPCR Master Mix (Promega, A6001) on a QuantStudio Applied Biosystems (Thermo) according to standard procedures. All amplification curves reached the stationary stage before 35 cycles and the readings of the Ct value were obtained at the exponential stage. ACTB mRNA was used as an internal control. All PCR products were sequenced for confirmation and all primer sequences were included in Supplementary Table S3.
Plasmid construction and cell transfection
All plasmids for the luciferase reporter system were constructed with recombinant methods (Vazyme, c113-02). PRDM1 3′ UTR containing the binding sites of miR-9-5p and SOX12 3′ UTR containing the miR-744-5p binding sequences were PCR-amplified from the cDNA of HeLa cells and then inserted into the Firefly luciferase reporter vector pGL3-control (Promega, E1741) between XbaI (Thermo, FD0685) and FseI (NEB, R0588V) double-digested sites. Small interfering RNAs (siRNAs) targeting lncSLC25A1, TINCR and circBANP BSJ were synthesized by GenePharma (Shanghai, China). Transfection of plasmids and siRNAs was performed with Lipofectamine 2000 (Invitrogen, 11668019) according to the manufacturer’s protocol. Oligonucleotide sequences for primers used in plasmid construction and siRNAs are included in Supplementary Table S3.
Construction of the co-expression network
The lncRNA/circRNA-mRNA co-expression network was constructed according to the expression levels in our dataset. Briefly, 51 lncRNAs, 39 circRNAs, and 279 mRNAs sensitive to TG and GD treatments were used to construct the network with a criterion of Spearman R ≥ 0.95 or ≤ -0.95 and p-value < 0.01. The constructed network consisted of 29 lncRNAs, 20 circRNAs, and 131 mRNAs, and was visualized with Cytoscape (https://cytoscape.org/).
Construction of the ceRNA network
The ceRNA network was constructed based on the lncRNA/circRNA-miRNA-mRNA axis. The lncRNA-miRNA, circRNA-miRNA, and mRNA-miRNA interactions were predicted with TargetScanHuman (https://www.targetscan.org/vert_72/). Briefly, mRNAs, lncRNAs, or circRNAs with at least two putative binding sites for the individual miRNA were used to construct the network.
Dual-luciferase reporter assay
Briefly, 1×106 HeLa cells were co-transfected with 30 pmol siRNAs, 1 μg pGL3 Firefly luciferase plasmids and 100 ng pRL Renilla luciferase reporter vector (Promega, E2261). After transfection for 48 h, cells were lysed with passive lysis buffer on ice for 20 min and the luciferase activity was performed with Dual Luciferase Reporter Assay System Kit (Promega, E1910) according to the manufacturer’s protocol. The Firefly luciferase activities were measured and normalized to Renilla luciferase activities (F/R).
The RNA-RBP network
The RNA-RBP network was constructed based on the lncRNA-RBP and circRNA-RBP interactions predicted by RBPmap (http://rbpmap.technion.ac.il/). The lncRNA/circRNA-RBP interaction was determined by a criterion of the individual RNA sequence containing more than 2 motifs for RBP of interest (p-value < 10E-4). The RNA-RBP interactome map consisted of 46 lncRNAs, 17 circRNAs and 77 RBPs, and was visualized with Cytoscape.
Fluorescence in situ hybridization (FISH)
FISH was carried out as previously described with minor modifications (Hu et al., 2016). RNA probe antisense to linc00612 was generated by the Transcript Aid T7 High Yield Transcription Kit (Thermo, K0441) with the corresponding insertion into the T vector (Promega, A3600) as a template. The probe was then labeled with Alexa Fluor546, by using the ULYSIS Nucleic Acid Labeling Kit (Thermo, U21652), which added a fluor on every G of the probe to amplify the fluorescence intensity. The primers for the antisense probe amplification were included in Supplementary Table S3. HeLa cells were fixed with 4% PFA for 10 min at room temperature after washing with PBS twice. RNA probes were denatured at 80°C for 10 min and placed on ice immediately. Fixed cells were incubated with RNA probes mixed with 20 ng/μL human Cot-1 DNA (Invitrogen, 15279011), 500 ng/μL yeast total RNA (Invitrogen, AM7118) and 10 units/mL RNase inhibitor (Promega, N2615) in 2 × hybridization buffer (4 × SSC, 40% dextran sulfate) at 37°C for 15–17 h, protected from light. After two 10-min washes in SSCT (2 × SSC and 0.4% Tween 20) buffer, nuclei were stained with DAPI (Sigma, F6057). Finally, images were captured using the LSM 980 confocal microscope (Zeiss).
eCLIP-seq data analysis
Published eCLIP-seq data were obtained from Gene Expression Omnibus (GEO) database under the following accession numbers: GSE91952 (EIF4G2), GSE126263 (MSI1), GSE71096 (SRSF10), GSE69153 (RC3H1) and GSE107768 (FUBP3, DAZAP1, HNRNPA0 and PABPC4).
GO analysis
GO analysis of the DE mRNAs was performed using the GOrilla web-server with default parameters (http://cbl-gorilla.cs.technion.ac.il) (Eden et al., 2009). For data visualization, the plots were generated by the ggplot2 package in R software.
Statistical analysis
The physiological experiments were carried out in triplicates (N = 3), and statistical analysis of the data was performed with the two-tailed Student’s t-tests. Data were present as the mean from three independent experiments with SEM. The RNA-seq was performed with four replicates and statistical analysis for analysis was calculated by DEseq2.
Results
Global transcriptome analysis for cells under TG and GD treatments
To identify stress-related ncRNAs, we performed ribosomal RNA (rRNA) depleted RNA sequencing (RNA-seq) of human HeLa cells treated with TG (an ER stress inducer) or GD (a metabolic oxidative stress inducer). XBP1s is a well-characterized marker for ER stress and Follistatin (FST) is upregulated in response to GD (Yoshida et al., 2001; Gao et al., 2014). We first examined the levels of XBP1s and FST mRNAs, and found that XBP1s and FST detected by RT-PCR with specific primers were significantly increased upon TG and GD treatment, respectively (Supplementary Figure S1A). Then, we performed the bioinformatics to analyze lncRNAs and circRNAs in our dataset (Supplementary Figure S1B). To identify high-confidence back-splicing junctions (BSJs) of circRNAs, two published pipelines including CIRI2 (Gao et al., 2018) and find_circ (Memczak et al., 2013) were applied to annotate circRNAs (Supplementary Figure S1B). CircRNA candidates overlapped in these two pipelines were used for further investigations. Then, the differentially expressed (DE) circRNAs and lncRNAs were analyzed (Supplementary Figure S1B). Principal component analysis (PCA) plots with all identified transcripts also revealed that four biological replicates clustered together, and the controls, TG and GD groups were clearly separated (Supplementary Figure S1C), indicating reliability of RNA-seq and data analyses.
Differentially expressed lncRNAs and circRNAs in stress responses
We characterized 2,406 lncRNAs in our dataset, among which 179 (97 upregulated, 82 downregulated) and 193 (82 upregulated, 111 downregulated) DE lncRNAs were identified (fold change ≥2 or ≤0.5, p-value < 0.05) upon TG and GD treatments, respectively (Figure 1A and Supplementary Table S1). Among them, 26 and 25 lncRNAs were significantly increased and decreased in response to both TG and GD treatments (Figure 1B). Simultaneously, we discovered ∼1‰ BSJ reads in our dataset and identified a total of 6531 circRNAs (BSJ reads ≥2) (Supplementary Figure S1D). We determined 161 (70 upregulated, 91 downregulated) and 147 (51 upregulated, 96 downregulated) DE circRNAs (fold change ≥2 or ≤0.5, p-value < 0.05) upon TG and GD treatments, respectively (Figure 1C and Supplementary Table S2). Among them, 14 and 25 circRNAs were markedly increased and decreased in response to both TG and GD treatments (Figure 1D). Then, we focused on 51 lncRNAs and 39 circRNAs both dysregulated in response to ER and metabolic stresses (Figures 1B, D). We randomly selected 6 lncRNAs and 6 circRNAs among them for experimental validation. Real-time quantitative PCR (RT-qPCR) analysis demonstrated that lincPINT, lncSARS1, lncZFAS1, circCRIM1, circHBS1L, and circPTBP2 were significantly increased after TG and GD treatments (Figures 2A, B). Conversely, BASP1-AS1, lncNT5C3B, TINCR, circABL2, circBANP, and circCEP72 were significantly decreased upon TG and GD treatments (Figures 2A, B). For these candidates examined, RT-qPCR-mediated verification was highly consistent with the RNA-seq data (Figure 2C). Taken together, the DE lncRNAs and circRNAs might play essential roles in coping with cellular stresses.
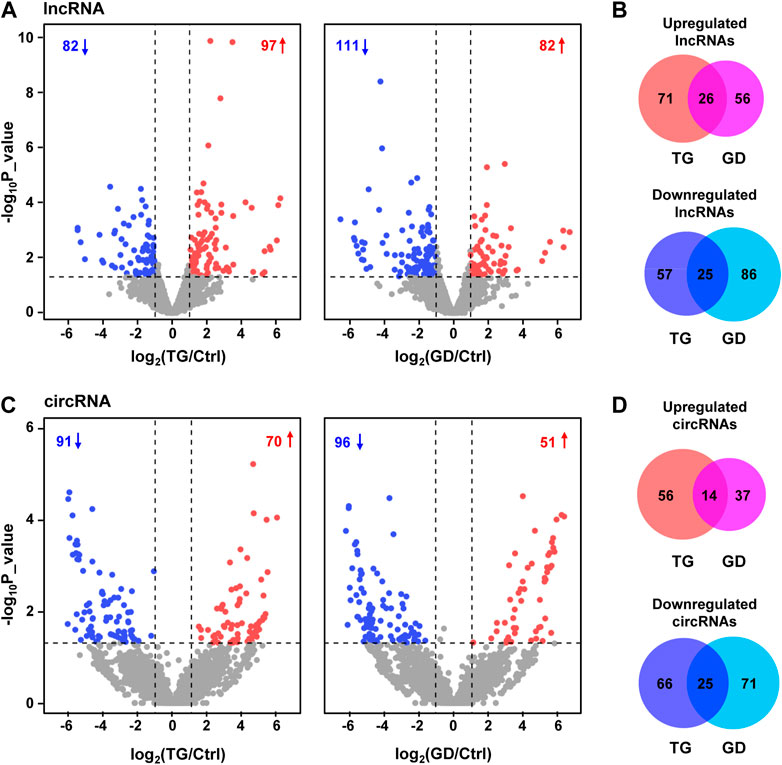
FIGURE 1. Transcriptome analyses of HeLa cells treated with TG and GD (A) Volcano plots displaying the differentially expressed lncRNAs in HeLa cells treated with TG and GD. Blue dots represent significantly downregulated lncRNAs and red dots represent significantly upregulated lncRNAs. Gray dots represent unchanged lncRNAs (B) Venn diagram revealing the overlap of dysregulated lncRNAs under TG and GD treatments (C) Volcano plots displaying the differentially expressed circRNAs in HeLa cells treated with TG and GD. Blue dots represent significantly downregulated circRNAs and red dots represent significantly upregulated circRNAs. Gray dots represent unchanged circRNAs (D) Venn diagram revealing the overlap of dysregulated circRNAs under TG and GD treatments.
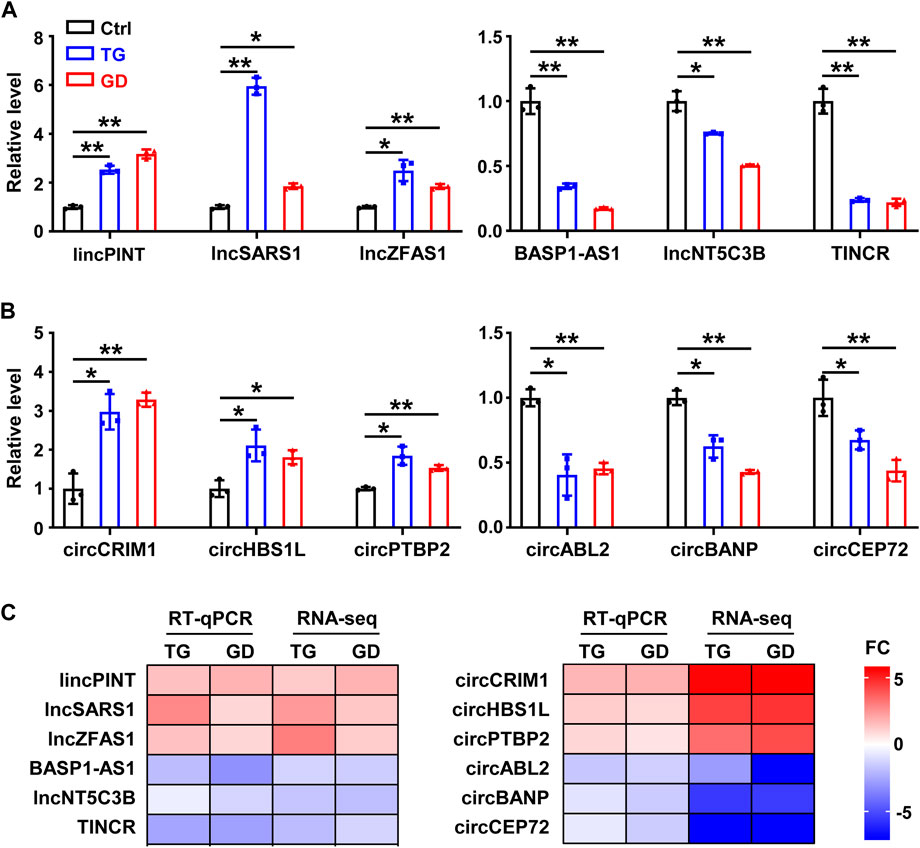
FIGURE 2. Experimental validation of lncRNAs and circRNAs in HeLa cells (A) RT-qPCR analysis of six differentially expressed lncRNAs in HeLa cells treated with TG and GD (B) RT-qPCR analysis of six differentially expressed circRNAs in HeLa cells treated with TG and GD (C) Heatmaps for the comparison of the RT-qPCR and RNA-seq. Red boxes represent upregulated candidates after TG or GD treatment and blue boxes represent downregulated candidates. FC, fold change. Data are representative of three independent experiments and shown as mean ± SEM. *p < 0.05; **p < 0.01 by two-tailed Student’s t-test.
Co-expression network for lncRNA/circRNA/mRNA
The functions of lncRNAs and circRNAs are tightly related to the roles of their co-expressed protein-coding genes (Quinn and Chang, 2016; Sheng et al., 2019; Chen et al., 2022). To investigate the roles of lncRNAs and circRNAs associated with ER and metabolic stresses, we constructed a co-expression network (Spearman R ≥ 0.95 or ≤ -0.95, p-value < 0.01) of lncRNAs, circRNAs and their co-expressed DE mRNAs (RPM ≥10, fold change ≥2 or ≤0.5, p-value < 0.05) for the 51 DE lncRNAs and 39 DE circRNAs identified in both stresses according to our RNA-seq data (Figure 3A). Our analysis revealed that 29 lncRNAs (17 downregulated, 12 upregulated) and 20 circRNAs (18 downregulated, 2 upregulated) interacted with 131 DE mRNAs (72 downregulated, 59 upregulated) (Figure 3A). Notably, we found that the genomic distances of all interacted nodes in the co-expressed network were more than 100 kilobases, indicating the trans roles of lncRNAs and circRNAs on the co-expressed mRNAs. RNA interference (RNAi) is a widely used approach to deplete lncRNA/circRNA of interest, although there are multiple other methods (Quinn and Chang, 2016; Zhang et al., 2021). We then performed loss of function studies of three candidates (two lncRNAs and one circRNA) using RNAi to validate the co-expressed correlation between DE lncRNAs/circRNAs and mRNAs (Figures 3B–D). Small interfering RNA (siRNA)-mediated TINCR silencing resulted in significantly decreased expressions of its co-expressed mRNAs (ERN1 and SOX12) in HeLa cells (Figure 3B). Consistently, lncSLC25A1 knockdown decreased its co-expressed targets (SOX12 and ATOH8), and circBANP depletion downregulated its co-expressed targets (ERN1 and ATF4) (Figures 3C, D). The heatmap further demonstrated that these 131 mRNAs were significantly dysregulated after TG and GD treatments (Supplementary Figure S2A). Gene Ontology (GO) analysis revealed that these mRNAs present in the co-expressed network significantly enriched in biological processes such as response to toxic substance, response to stress, response to extracellular stimulus, regulation of RNA metabolic process, programmed cell death, PERK-mediated unfolded protein response and cellular response to glucose starvation (Supplementary Figure S2B).
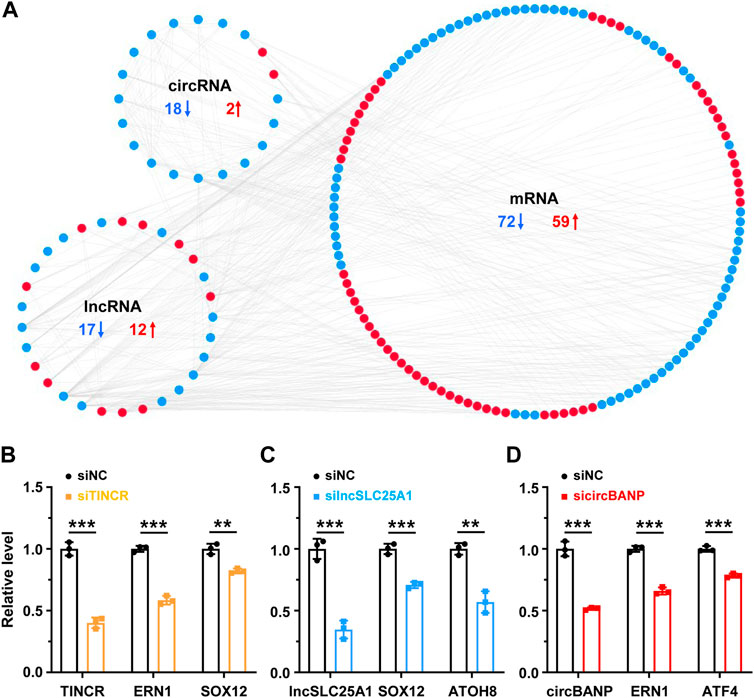
FIGURE 3. The co-expression network of lncRNA/circRNA/mRNA (A) The co-expression network between differentially expressed lncRNAs, circRNAs and mRNAs sensitive to both TG and GD treatments (correlation coefficient absolute value ≥ 0.95). Red, upregulated; blue, downregulated (B) RT-qPCR analysis of the knockdown efficiency of TINCR and expression levels of co-expressed ERN1 or SOX12 mRNA in HeLa cells treated with the siRNA against TINCR. siNC, siRNA with scrambled sequences; siTINCR, siRNA against TINCR (C) RT-qPCR analysis of the knockdown efficiency of lncSLC25A1 and expression levels of co-expressed SOX12 or ATOH8 mRNA in HeLa cells treated with the siRNA against lncSLC25A1. siNC, siRNA with scrambled sequences; silncSLC25A1, siRNA against lncSLC25A1 (D) RT-qPCR analysis of the knockdown efficiency of circBANP and expression levels of co-expressed ERN1 or ATF4 mRNA in HeLa cells treated with the siRNA against circBANP. siNC, siRNA with scrambled sequences; sicircBANP, siRNA against the junction sites of circBANP. Data are representative of three independent experiments and shown as mean ± SEM. **p < 0.01; ***p < 0.001 by two-tailed Student’s t-test.
Construction of ceRNA regulatory network
One of the molecular mechanisms for lncRNAs and circRNAs is to act as competing endogenous RNAs (ceRNAs), in which lncRNAs and circRNAs bind with miRNAs and decrease the corresponding binding of miRNAs on mRNA targets (Quinn and Chang, 2016; Chen et al., 2022). In order to explore the potential lncRNAs and circRNAs serving as ceRNAs responsive to cellular stresses via sequestering miRNAs and thus regulating mRNA targets, we constructed a ceRNA network among the DE lncRNAs, circRNAs, and mRNAs respond to both ER and metabolic stresses. 23 circRNAs, 2 lncRNAs, 48 miRNAs, and 32 mRNAs composed of the ceRNA regulatory network (Figure 4A). Among 32 mRNA targets, 17 were downregulated and 15 were upregulated in response to TG and GD treatments (Figure 4B). Then, we conducted GO analysis for the 32 mRNAs in the ceRNA network and found that they were associated with regulations of biological processes including transcription by RNA polymerase II, RNA metabolic process, macromolecule metabolic process, developmental process and cell differentiation (Figure 4C). To further verify the ceRNA roles of lncRNAs and circRNAs, we performed a dual-luciferase reporter assay in HeLa cells. In our network, circBANP interacted with miR-9-5p to derepress the PRDM1 level (Figure 4D). Knockdown of circBANP mediated by siRNA significantly reduced the luciferase activity of Firefly with the PRDM1 3’ UTR region containing the miR-9-5p binding site (Figure 4D). The lncSLC25A1-miR-744-5p-SOX12 axis exhibited a similar result according to our experiments (Figure 4D). Although, we have to point out that these were just results from overexpression and RNAi experiments, and further validations are required to examine the ceRNA regulatory network.
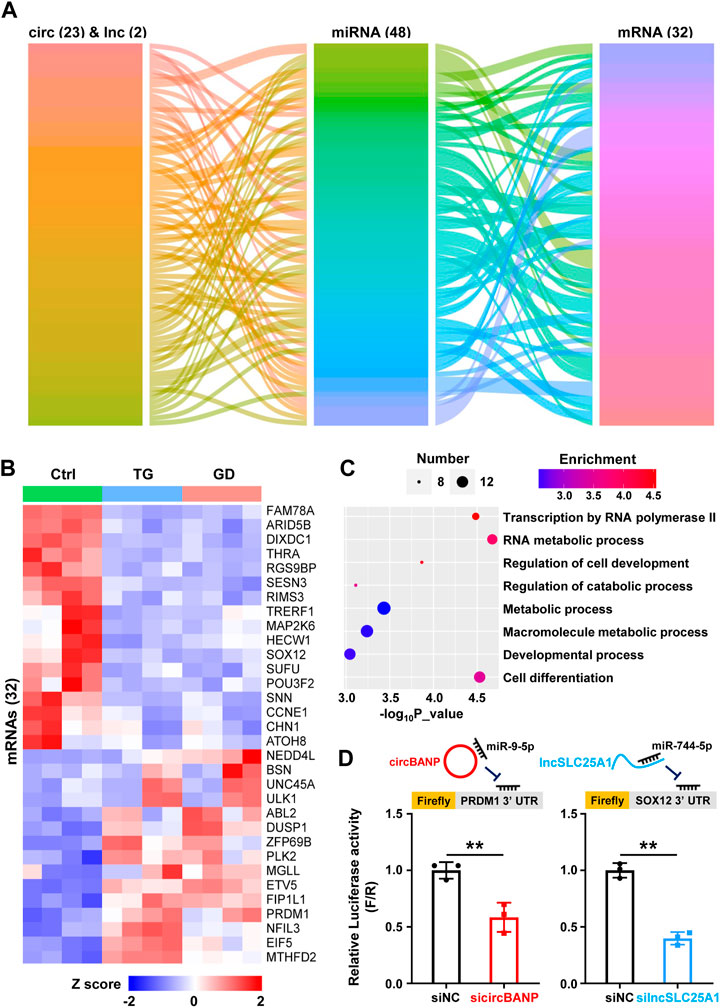
FIGURE 4. The ceRNA regulatory network (A) Alluvial ceRNA network was constructed based on the lncRNA/circRNA/mRNA-miRNA interactions among 51 lncRNAs and 39 circRNAs sensitive to both TG and GD treatments. All the interactions were predicted with TargetScanHuman 7.2 (B) The heatmap showing the levels of 32 mRNAs present in the above network in TG- or GD-treated HeLa cells. Red boxes represent upregulated mRNAs and blue boxes represent downregulated mRNAs (C) GO analysis revealing biological processes of 32 mRNAs present in the above network (D) The diagram of firefly luciferase reporters was shown (top). HeLa cells were co-transfected with sicircBANP or silncSLC25A1, the Renilla luciferase plasmid and Firefly luciferase reporter plasmids harboring the PRDM1 or SOX12 3′ UTR. The ratio of Firefly (F) to Renilla (R) in relative luciferase activity was plotted. siNC, siRNA with scrambled sequences; sicircBANP, siRNA against the junction sites of circBANP; silncSLC25A1, siRNA against lncSLC25A1. Data are representative of three independent experiments and shown as mean ± SEM. **p < 0.01 by two-tailed Student’s t-test.
RNA-RBP interaction network
Given that lncRNAs and circRNAs could interact with RBPs to exert their crucial functions (Chen et al., 2022; Wang et al., 2022), we predicted the potential binding RBPs of both DE lncRNAs and DE circRNAs in ER or metabolic stresses via RBPmap, a tool mapping the interacting RBPs for RNAs of interest (Paz et al., 2014), and constructed the lncRNA/circRNA-RBP network based on the predicted interactions (Figure 5A). The network consisted of 17 circRNAs, 46 lncRNAs and 77 RBPs (Figure 5A). We also noticed that among the network, linc00612 and circSTAU2 interacted with the most RBPs among lncRNAs and circRNAs, respectively (Figure 5A). Linc00612 interacted with 16 different RBPs such as SRSF8, HNRNPDL, PUM2, and circSTAU2 interacted with 6 distinct RBPs such as PABPC1, SART3, SRSF10 (Figures 5B, C). Furthermore, the mRNA levels of 77 RBPs were dynamic after TG and GD treatments (Supplementary Figure S3A). GO analysis for these 77 RBPs indicated that they were related to biological processes including RNA 3’ end processing, mRNA stability involved in response to stress, regulation of translation, gene silencing by miRNA, nuclear export, mRNA splicing via spliceosome, etc. (Supplementary Figure S3B). RNA fluorescence in situ hybridization (FISH) displayed that linc00612 mostly localized to the cytoplasm, and a small portion resided in the nucleus in HeLa cells (Figure 5D), implying that linc00612 might possess both cytoplasmic and nuclear roles. In addition, integrative analysis of published eCLIP-seq data of RBPs revealed that EIF4G2, FUBP3, HNRNPA0, and MSI1 demonstrated binding signals on linc00612 (Figure 5E).
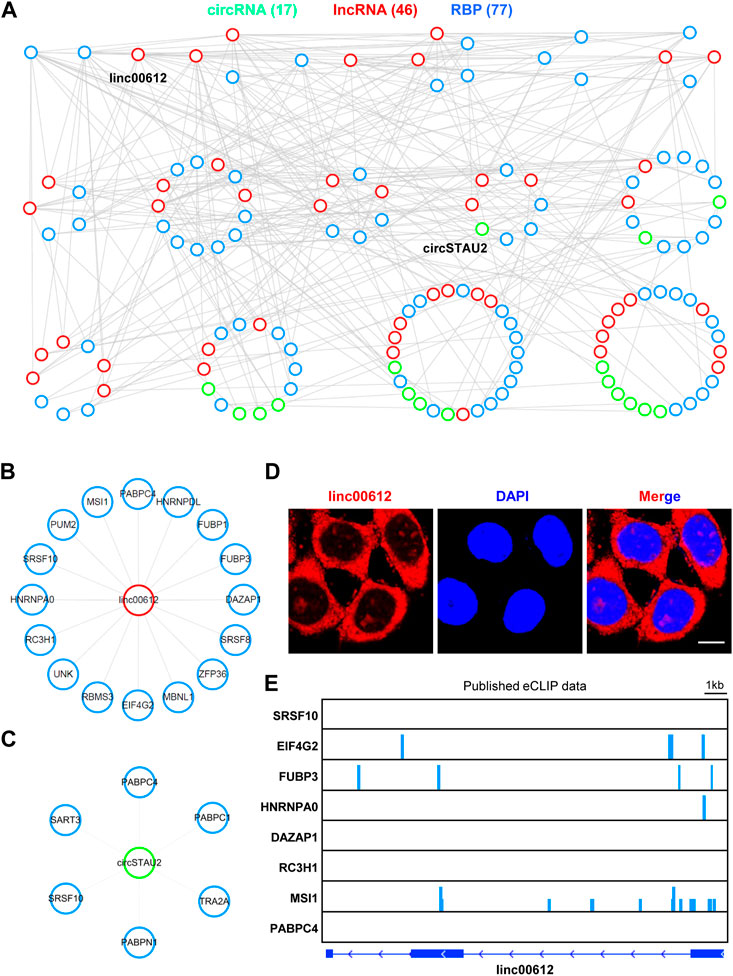
FIGURE 5. The RNA-RBP interactome map (A) The lncRNA/circRNA-RBP network was constructed according to the interactions among 51 lncRNAs and 39 circRNAs sensitive to both TG and GD treatments. All the interactions were predicted with RBPmap. The network was plotted by the ranked degrees. RBP, RNA binding protein (B) Linc00612 interacted with 16 RBPs in the above network (C) CircSTAU2 interacted with 6 RBPs in the above network (D) RNA FISH with the antisense probe showed the subcellular localization of linc00612 (red) in HeLa cells. Nuclei (blue) were stained with DAPI. Scale bar, 10 μm (E) The analyses of eight published eCLIP-seq data indicating the binding signals on linc00612.
Discussion
Prolonged exposures to stresses are tightly associated with numerous diseases including cancers and metabolic disorders (Urra et al., 2016; Galluzzi et al., 2018); thus, clarifying cellular responses and common responsive factors underneath multiple stresses is of great importance. Systematic investigations about stress response networks are still required (Galluzzi et al., 2018; Chen et al., 2021). Here, we have applied RNA-seq to identify the DE lncRNAs and circRNAs in two cellular stresses and tentatively revealed the regulatory interactions and potential functional mechanisms of these RNAs, shedding new insights into cellular responses and providing some indications for disease relevancies.
RNA-seq followed by bioinformatics analyses is a powerful tool to characterize aberrantly expressed protein-coding and non-coding genes at the transcriptome level (Mortazavi et al., 2008). The studies about stress-related RNAs are accumulating, and these transcripts may be correlated with stress or anti-stress roles (Settembre et al., 2011; Wiseman et al., 2022). Increasing evidence has demonstrated that lncRNAs and circRNAs along with mRNAs are extensively involved in cellular responses, with some of them being identified as stress sensors (Li et al., 2019; De Troyer et al., 2020; Hetz et al., 2020; Zhu et al., 2022). However, systematic identification of differentially expressed RNAs, especially circRNAs, under two distinct cellular stimuli, is limited.
In the present study, we have performed transcriptomic profiles of HeLa cells treated with TG or GD and identified 51 lncRNAs and 39 circRNAs sensitive to both stresses (Figure 1). We have validated 12 DE RNA candidates, which are also in high accordance with the RNA-seq (Figure 2). Several of them are known to have substantial involvements in physiology and diseases (Hong et al., 2020; Kretz et al., 2013; Marín-Béjar et al., 2017; Simchovitz et al., 2020; Zheng et al., 2020). For example, lincPINT, which is upregulated during TG or GD treatment, plays crucial roles in many diseases, such as neurodegeneration and cancers (Marín-Béjar et al., 2017; Simchovitz et al., 2020). TINCR is decreased in two stress conditions and has been reported to contribute to various cellular processes, including cell proliferation, apoptosis, autophagy, invasion and metastasis (Kretz et al., 2013; Zheng et al., 2020). CircCRIM1 has been reported to promote nasopharyngeal carcinoma (NPC) metastasis and docetaxel chemoresistance via serving as a ceRNA against miR-422a to improve the FOXQ1 level (Hong et al., 2020). All these results imply that these DE lncRNAs and circRNAs identified in both stresses might behave as pivotal regulators responsive to cellular stresses. To explore the regulatory functions of lncRNAs and circRNAs, we have constructed the co-expressed network, the ceRNA network, and the RNA-RBP interaction map (Figures 3–5). Furthermore, GO analysis has revealed the potential roles of lncRNAs and circRNAs present in these networks in the corresponding biological processes. Hopefully, this study can provide helpful aspects for future investigations of stress responses.
NcRNA-mRNA interaction is one of the most frequently studied molecular mechanisms of ncRNAs (Quinn and Chang, 2016; Chen et al., 2022). We have constructed a co-expressed network for 51 lncRNAs and 39 circRNAs sensitive to ER and metabolic stresses (Figure 3A). GO analysis has revealed mRNAs in the network are enriched on biological pathways such as response to toxic substance, response to stress, response to extracellular stimulus, programmed cell death, PERK-mediated unfolded protein response and cellular response to glucose starvation (Supplementary Figure S2B). These GOs strongly indicate the engagement of lncRNAs and circRNAs in stress responsive processes, and also to some degree provide proof for the constructed network.
We have also constructed a ceRNA network based on the lncRNA/circRNA-miRNA-mRNA interaction and performed experimental data to verify the ceRNA roles of lncRNAs and circRNAs (Figure 4). According to the result of GO analysis, 23 circRNAs, 2 lncRNA and 48 miRNAs composed of the network, which may participate in the processes including transcription by RNA polymerase II, RNA metabolic process and cell differentiation (Figure 4C). From a previous publication, miR-423-5p in the network is indeed upregulated under ER stress, and exerts its function by targeting CDKN1A (Dai et al., 2015). Although accumulating evidence is supportive of the ceRNA mechanism, concerns about this concept have been raised (Bosson et al., 2014; Denzler et al., 2014; Tay et al., 2014; Chen et al., 2022). The molecular ratio and the endogenous expression levels need to be carefully evaluated, and more examples with convincing physiological data should be provided to further prove ceRNA regulations.
RNA-protein interaction is another functional mechanism of RNAs (Licatalosi and Darnell, 2010; Chen et al., 2022). Increasing evidence has demonstrated that lncRNAs and circRNAs can exert their functions through modulating or sequestering one or more RBPs (Lee et al., 2016; Wang et al., 2021). Based on the RNA-protein interactions, we have constructed the RNA-RBP interactome map (Figure 5), and this type of interactome map is still scarce. In the network, linc00612 and circSTAU2 interact with the maximum number of proteins among lncRNAs and circRNAs, respectively (Figures 5A–C). Linc00612 has been reported to promote the progressions of osteosarcoma and bladder cancer (Miao et al., 2019; Zhou et al., 2020). Linc00612 binds to 16 RBPs with distinct functional roles, such as transcription (HNRNPDL, FUBP1), splicing (SRSF8, SRSF10, MBNL1), RNA stability (ZFP36, RC3H1, PABPC4), and translation (EIF4G2, UNK, MSI1). Cellular localization of an ncRNA is critical for its functionality. Linc00612 mostly localizes to the cytoplasm, which may be associated with its role in regulation of translation. Meanwhile, a small portion of linc00612 resides in the nucleus, which may be responsible for its regulatory roles in transcription and splicing. CircSTAU2 interacts with splicing-related proteins (TRA2A, SRSF10, SART3) and RNA stability-related proteins (PABPC1, PABPC4, PABPN1). Linc00612 and circSTAU2 both interact with SRSF10 and PABPC4, implying that both proteins may be key factors in response to cellular stresses.
We would also like to point out several limitations of this study. We cannot rule out that the DE lncRNAs/circRNAs identified under the cellular stresses tested have cell-type specificity. Although we have found a series of DE lncRNAs and circRNAs in cellular stress responses, investigations about functions of individual ncRNA of interest, and the molecular mechanism are required. Moreover, the lncRNA/circRNA regulatory networks need further validation with more experimental explorations.
Conclusion
In summary, our study has identified a number of DE lncRNAs and circRNAs responsive to ER and metabolic stresses, and has constructed associated regulatory networks to provide novel insights for functional and mechanistic explorations of ncRNAs under cellular stresses.
Data availability statement
The datasets presented in this study can be found in online repositories. The names of the repository/repositories and accession number(s) can be found in the article/Supplementary Material.
Author contributions
XW and GS designed and supervised this project. XW, XL, and JL performed experiments and bioinformatics analyses of RNA-seq data. XW, GS, and XL wrote the manuscript. All authors read and approved the final version of the article.
Funding
This study was supported by the National Key R&D Program of China (2019YFA0802600), the National Natural Science Foundation of China (31930019 and 32200431), and the China Postdoctoral Science Foundation (2022M713053).
Conflict of interest
The authors declare that the research was conducted in the absence of any commercial or financial relationships that could be construed as a potential conflict of interest.
Publisher’s note
All claims expressed in this article are solely those of the authors and do not necessarily represent those of their affiliated organizations, or those of the publisher, the editors and the reviewers. Any product that may be evaluated in this article, or claim that may be made by its manufacturer, is not guaranteed or endorsed by the publisher.
Supplementary material
The Supplementary Material for this article can be found online at: https://www.frontiersin.org/articles/10.3389/fgene.2023.1097571/full#supplementary-material
References
Ahmed, A. S. I., Dong, K., Liu, J., Wen, T., Yu, L., Xu, F., et al. (2018). Long noncoding RNA NEAT1 (nuclear paraspeckle assembly transcript 1) is critical for phenotypic switching of vascular smooth muscle cells. Proc. Natl. Acad. Sci. U. S. A. 115, E8660–E8667. doi:10.1073/pnas.1803725115
An, H., Williams, N. G., and Shelkovnikova, T. A. (2018). NEAT1 and paraspeckles in neurodegenerative diseases: A missing lnc found? Noncoding RNA Res. 3, 243–252. doi:10.1016/j.ncrna.2018.11.003
Anastasiadou, E., Jacob, L. S., and Slack, F. J. (2018). Non-coding RNA networks in cancer. Nat. Rev. Cancer 18, 5–18. doi:10.1038/nrc.2017.99
Bartel, D. P. (2004). MicroRNAs: genomics, biogenesis, mechanism, and function. Cell 116, 281–297. doi:10.1016/s0092-8674(04)00045-5
Bhardwaj, M., Leli, N. M., Koumenis, C., and Amaravadi, R. K. (2020). Regulation of autophagy by canonical and non-canonical ER stress responses. Semin. Cancer Biol. 66, 116–128. doi:10.1016/j.semcancer.2019.11.007
Bosson, A. D., Zamudio, J. R., and Sharp, P. A. (2014). Endogenous miRNA and target concentrations determine susceptibility to potential ceRNA competition. Mol. Cell 56, 347–359. doi:10.1016/j.molcel.2014.09.018
Chen, X., Zeh, H. J., Kang, R., Kroemer, G., and Tang, D. (2021). Cell death in pancreatic cancer: from pathogenesis to therapy. Nat. Rev. Gastroenterol. Hepatol. 18, 804–823. doi:10.1038/s41575-021-00486-6
Chen, L., Huang, C., and Shan, G. (2022). Circular RNAs in physiology and non-immunological diseases. Trends Biochem. Sci. 47, 250–264. doi:10.1016/j.tibs.2021.11.004
Dai, L., Huang, C., Chen, L., Shan, G., and Li, Z. (2015). Altered expression of microRNAs in the response to ER stress. Sci. Bull. 60, 202–209. doi:10.1007/s11434-014-0657-z
De Troyer, L., Zhao, P., Pastor, T., Baietti, M. F., Barra, J., Vendramin, R., et al. (2020). Stress-induced lncRNA LASTR fosters cancer cell fitness by regulating the activity of the U4/U6 recycling factor SART3. Nucleic Acids Res. 48, 2502–2517. doi:10.1093/nar/gkz1237
Denzler, R., Agarwal, V., Stefano, J., Bartel, D. P., and Stoffel, M. (2014). Assessing the ceRNA hypothesis with quantitative measurements of miRNA and target abundance. Mol. Cell 54, 766–776. doi:10.1016/j.molcel.2014.03.045
Eden, E., Navon, R., Steinfeld, I., Lipson, D., and Yakhini, Z. (2009). GOrilla: a tool for discovery and visualization of enriched GO terms in ranked gene lists. BMC Bioinforma. 10, 48. doi:10.1186/1471-2105-10-48
Eisner, V., Picard, M., and Hajnóczky, G. (2018). Mitochondrial dynamics in adaptive and maladaptive cellular stress responses. Nat. Cell Biol. 20, 755–765. doi:10.1038/s41556-018-0133-0
Ermolaeva, M. A., and Schumacher, B. (2014). Systemic DNA damage responses: organismal adaptations to genome instability. Trends Genet. 30, 95–102. doi:10.1016/j.tig.2013.12.001
Feng, Y. X., Sokol, E. S., Del Vecchio, C. A., Sanduja, S., Claessen, J. H., Proia, T. A., et al. (2014). Epithelial-to-mesenchymal transition activates PERK-eIF2α and sensitizes cells to endoplasmic reticulum stress. Cancer Discov. 4, 702–715. doi:10.1158/2159-8290.CD-13-0945
Frakes, A. E., and Dillin, A. (2017). The UPRER: sensor and coordinator of organismal homeostasis. Mol. Cell 66, 761–771. doi:10.1016/j.molcel.2017.05.031
Fu, X. D. (2014). Non-coding RNA: a new frontier in regulatory biology. Natl. Sci. Rev. 1, 190–204. doi:10.1093/nsr/nwu008
Galluzzi, L., Yamazaki, T., and Kroemer, G. (2018). Linking cellular stress responses to systemic homeostasis. Nat. Rev. Mol. Cell Biol. 19, 731–745. doi:10.1038/s41580-018-0068-0
Gao, X., Dong, H., Lin, C., Sheng, J., Zhang, F., Su, J., et al. (2014). Reduction of AUF1-mediated follistatin mRNA decay during glucose starvation protects cells from apoptosis. Nucleic Acids Res. 42, 10720–10730. doi:10.1093/nar/gku778
Gao, Y., Zhang, J., and Zhao, F. (2018). Circular RNA identification based on multiple seed matching. Brief. Bioinform 19, 803–810. doi:10.1093/bib/bbx014
Gao, L., Chang, S., Xia, W., Wang, X., Zhang, C., Cheng, L., et al. (2020). Circular RNAs from BOULE play conserved roles in protection against stress-induced fertility decline. Sci. Adv. 6, eabb7426. doi:10.1126/sciadv.abb7426
González, A., Hall, M. N., Lin, S. C., and Hardie, D. G. (2020). AMPK and TOR: The Yin and Yang of cellular nutrient sensing and growth control. Cell Metab. 31, 472–492. doi:10.1016/j.cmet.2020.01.015
González-Quiroz, M., Blondel, A., Sagredo, A., Hetz, C., Chevet, E., and Pedeux, R. (2020). When endoplasmic reticulum proteostasis meets the DNA damage response. Trends Cell Biol. 30, 881–891. doi:10.1016/j.tcb.2020.09.002
Hansen, T. B., Jensen, T. I., Clausen, B. H., Bramsen, J. B., Finsen, B., Damgaard, C. K., et al. (2013). Natural RNA circles function as efficient microRNA sponges. Nature 495, 384–388. doi:10.1038/nature11993
Hetz, C., Zhang, K., and Kaufman, R. J. (2020). Mechanisms, regulation and functions of the unfolded protein response. Nat. Rev. Mol. Cell Biol. 21, 421–438. doi:10.1038/s41580-020-0250-z
Hong, X., Liu, N., Liang, Y., He, Q., Yang, X., Lei, Y., et al. (2020). Circular RNA CRIM1 functions as a ceRNA to promote nasopharyngeal carcinoma metastasis and docetaxel chemoresistance through upregulating FOXQ1. Mol. Cancer 19, 33. doi:10.1186/s12943-020-01149-x
Hu, S., and Shan, G. (2016). LncRNAs in stem cells. Stem Cells Int. 2016, 2681925. doi:10.1155/2016/2681925
Hu, S., Wang, X., and Shan, G. (2016). Insertion of an Alu element in a lncRNA leads to primate-specific modulation of alternative splicing. Nat. Struct. Mol. Biol. 23, 1011–1019. doi:10.1038/nsmb.3302
Jonas, S., and Izaurralde, E. (2015). Towards a molecular understanding of microRNA-mediated gene silencing. Nat. Rev. Genet. 16, 421–433. doi:10.1038/nrg3965
Keestra-Gounder, A. M., Byndloss, M. X., Seyffert, N., Young, B. M., Chávez-Arroyo, A., Tsai, A. Y., et al. (2016). NOD1 and NOD2 signalling links ER stress with inflammation. Nature 532, 394–397. doi:10.1038/nature17631
Kent, O. A., McCall, M. N., Cornish, T. C., and Halushka, M. K. (2014). Lessons from miR-143/145: the importance of cell-type localization of miRNAs. Nucleic Acids Res. 42, 7528–7538. doi:10.1093/nar/gku461
Kleaveland, B., Shi, C. Y., Stefano, J., and Bartel, D. P. (2018). A network of noncoding regulatory RNAs acts in the mammalian brain. Cell 174, 350–362. doi:10.1016/j.cell.2018.05.022
Kretz, M., Siprashvili, Z., Chu, C., Webster, D. E., Zehnder, A., Qu, K., et al. (2013). Control of somatic tissue differentiation by the long non-coding RNA TINCR. Nature 493, 231–235. doi:10.1038/nature11661
Kristensen, L. S., Andersen, M. S., Stagsted, L. V. W., Ebbesen, K. K., Hansen, T. B., and Kjems, J. (2019). The biogenesis, biology and characterization of circular RNAs. Nat. Rev. Genet. 20, 675–691. doi:10.1038/s41576-019-0158-7
Larsen, B. D., Benada, J., Yung, P. Y. K., Bell, R. A. V., Pappas, G., Urban, V., et al. (2022). Cancer cells use self-inflicted DNA breaks to evade growth limits imposed by genotoxic stress. Science 376, 476–483. doi:10.1126/science.abi6378
Le, A., Lane, A. N., Hamaker, M., Bose, S., Gouw, A., Barbi, J., et al. (2012). Glucose-independent glutamine metabolism via TCA cycling for proliferation and survival in B cells. Cell Metab. 15, 110–121. doi:10.1016/j.cmet.2011.12.009
Lee, S., Kopp, F., Chang, T. C., Sataluri, A., Chen, B., Sivakumar, S., et al. (2016). Noncoding RNA NORAD regulates genomic stability by sequestering PUMILIO proteins. Cell 164, 69–80. doi:10.1016/j.cell.2015.12.017
Leung, A. K., and Sharp, P. A. (2010). MicroRNA functions in stress responses. Mol. Cell 40, 205–215. doi:10.1016/j.molcel.2010.09.027
Li, Z., Huang, C., Bao, C., Chen, L., Lin, M., Wang, X., et al. (2015). Exon-intron circular RNAs regulate transcription in the nucleus. Nat. Struct. Mol. Biol. 22, 256–264. doi:10.1038/nsmb.2959
Li, G., Wu, X., Qian, W., Cai, H., Sun, X., Zhang, W., et al. (2016). CCAR1 5’ UTR as a natural miRancer of miR-1254 overrides tamoxifen resistance. Cell Res. 26, 655–673. doi:10.1038/cr.2016.32
Li, Q., Wang, Y., Wu, S., Zhou, Z., Ding, X., Shi, R., et al. (2019). CircACC1 regulates assembly and activation of AMPK complex under metabolic stress. Cell Metab. 30, 157–173. doi:10.1016/j.cmet.2019.05.009
Li, Z., Ge, Y., Dong, J., Wang, H., Zhao, T., Wang, X., et al. (2022). BZW1 facilitates glycolysis and promotes tumor growth in pancreatic ductal adenocarcinoma through potentiating eIF2α phosphorylation. Gastroenterology 162, 1256–1271.e14. doi:10.1053/j.gastro.2021.12.249
Liang, W. C., Wang, Y., Xiao, L. J., Wang, Y. B., Fu, W. M., Wang, W. M., et al. (2014). Identification of miRNAs that specifically target tumor suppressive KLF6-FL rather than oncogenic KLF6-SV1 isoform. RNA Biol. 11, 845–854. doi:10.4161/rna.29356
Licatalosi, D. D., and Darnell, R. B. (2010). RNA processing and its regulation: global insights into biological networks. Nat. Rev. Genet. 11, 75–87. doi:10.1038/nrg2673
Liu, C., Ge, H. M., Liu, B. H., Dong, R., Shan, K., Chen, X., et al. (2019). Targeting pericyte-endothelial cell crosstalk by circular RNA-cPWWP2A inhibition aggravates diabetes-induced microvascular dysfunction. Proc. Natl. Acad. Sci. U. S. A. 116, 7455–7464. doi:10.1073/pnas.1814874116
Liu, X., Wang, X., Li, J., Hu, S., Deng, Y., Yin, H., et al. (2020). Identification of mecciRNAs and their roles in the mitochondrial entry of proteins. Sci. China Life Sci. 63, 1429–1449. doi:10.1007/s11427-020-1631-9
Marín-Béjar, O., Mas, A. M., González, J., Martinez, D., Athie, A., Morales, X., et al. (2017). The human lncRNA LINC-PINT inhibits tumor cell invasion through a highly conserved sequence element. Genome Biol. 18, 202. doi:10.1186/s13059-017-1331-y
Melber, A., and Haynes, C. M. (2018). UPRmt regulation and output: a stress response mediated by mitochondrial-nuclear communication. Cell Res. 28, 281–295. doi:10.1038/cr.2018.16
Memczak, S., Jens, M., Elefsinioti, A., Torti, F., Krueger, J., Rybak, A., et al. (2013). Circular RNAs are a large class of animal RNAs with regulatory potency. Nature 495, 333–338. doi:10.1038/nature11928
Miao, L., Liu, H. Y., Zhou, C., and He, X. (2019). LINC00612 enhances the proliferation and invasion ability of bladder cancer cells as ceRNA by sponging miR-590 to elevate expression of PHF14. J. Exp. Clin. Cancer Res. 38, 143. doi:10.1186/s13046-019-1149-4
Mortazavi, A., Williams, B. A., McCue, K., Schaeffer, L., and Wold, B. (2008). Mapping and quantifying mammalian transcriptomes by RNA-Seq. Nat. Methods 5, 621–628. doi:10.1038/nmeth.1226
Pakos-Zebrucka, K., Koryga, I., Mnich, K., Ljujic, M., Samali, A., and Gorman, A. M. (2016). The integrated stress response. EMBO Rep. 17, 1374–1395. doi:10.15252/embr.201642195
Paz, I., Kosti, I., Ares, M., Cline, M., and Mandel-Gutfreund, Y. (2014). RBPmap: a web server for mapping binding sites of RNA-binding proteins. Nucleic Acids Res. 42, W361–W367. doi:10.1093/nar/gku406
Quinn, J. J., and Chang, H. Y. (2016). Unique features of long non-coding RNA biogenesis and function. Nat. Rev. Genet. 17, 47–62. doi:10.1038/nrg.2015.10
Rashid, F., Awan, H. M., Shah, A., Chen, L., and Shan, G. (2017). Induction of miR-3648 upon ER stress and its regulatory role in cell proliferation. Int. J. Mol. Sci. 18, 1375. doi:10.3390/ijms18071375
Ren, Y., and Shen, H. M. (2019). Critical role of AMPK in redox regulation under glucose starvation. Redox Biol. 25, 101154. doi:10.1016/j.redox.2019.101154
Ren, J., Bi, Y., Sowers, J. R., Hetz, C., and Zhang, Y. (2021). Endoplasmic reticulum stress and unfolded protein response in cardiovascular diseases. Nat. Rev. Cardiol. 18, 499–521. doi:10.1038/s41569-021-00511-w
Sato, M., Kadomatsu, T., Miyata, K., Warren, J. S., Tian, Z., Zhu, S., et al. (2021). The lncRNA Caren antagonizes heart failure by inactivating DNA damage response and activating mitochondrial biogenesis. Nat. Commun. 12, 2529. doi:10.1038/s41467-021-22735-7
Settembre, C., Di Malta, C., Polito, V. A., Garcia Arencibia, M., Vetrini, F., Erdin, S., et al. (2011). TFEB links autophagy to lysosomal biogenesis. Science 332, 1429–1433. doi:10.1126/science.1204592
Sheng, Z., Wang, X., Xu, G., Shan, G., and Chen, L. (2019). Analyses of a panel of transcripts identified from a small sample size and construction of RNA networks in hepatocellular carcinoma. Front. Genet. 10, 431. doi:10.3389/fgene.2019.00431
Simchovitz, A., Hanan, M., Yayon, N., Lee, S., Bennett, E. R., Greenberg, D. S., et al. (2020). A lncRNA survey finds increases in neuroprotective LINC-PINT in Parkinson's disease substantia nigra. Aging Cell 19, e13115. doi:10.1111/acel.13115
Sun, Q., Hao, Q., and Prasanth, K. V. (2018). Nuclear long noncoding RNAs: Key regulators of gene expression. Trends Genet. 34, 142–157. doi:10.1016/j.tig.2017.11.005
Tay, Y., Rinn, J., and Pandolfi, P. P. (2014). The multilayered complexity of ceRNA crosstalk and competition. Nature 505, 344–352. doi:10.1038/nature12986
Urra, H., Dufey, E., Avril, T., Chevet, E., and Hetz, C. (2016). Endoplasmic reticulum stress and the hallmarks of cancer. Trends Cancer 2, 252–262. doi:10.1016/j.trecan.2016.03.007
Vihervaara, A., Duarte, F. M., and Lis, J. T. (2018). Molecular mechanisms driving transcriptional stress responses. Nat. Rev. Genet. 19, 385–397. doi:10.1038/s41576-018-0001-6
Wang, X., Li, J., Bian, X., Wu, C., Hua, J., Chang, S., et al. (2021). CircURI1 interacts with hnRNPM to inhibit metastasis by modulating alternative splicing in gastric cancer. Proc. Natl. Acad. Sci. U. S. A. 118, e2012881118. doi:10.1073/pnas.2012881118
Wang, X., Hua, J., Li, J., Zhang, J., Dzakah, E. E., Cao, G., et al. (2022). Mechanisms of non-coding RNA-modulated alternative splicing in cancer. RNA Biol. 19, 541–547. doi:10.1080/15476286.2022.2062846
Wiseman, R. L., Mesgarzadeh, J. S., and Hendershot, L. M. (2022). Reshaping endoplasmic reticulum quality control through the unfolded protein response. Mol. Cell 82, 1477–1491. doi:10.1016/j.molcel.2022.03.025
Yang, Y., Fan, X., Mao, M., Song, X., Wu, P., Zhang, Y., et al. (2017). Extensive translation of circular RNAs driven by N6-methyladenosine. Cell Res. 27, 626–641. doi:10.1038/cr.2017.31
Yoshida, H., Matsui, T., Yamamoto, A., Okada, T., and Mori, K. (2001). XBP1 mRNA is induced by ATF6 and spliced by IRE1 in response to ER stress to produce a highly active transcription factor. Cell 107, 881–891. doi:10.1016/s0092-8674(01)00611-0
Zhang, C. S., Hawley, S. A., Zong, Y., Li, M., Wang, Z., Gray, A., et al. (2017). Fructose-1,6-bisphosphate and aldolase mediate glucose sensing by AMPK. Nature 548, 112–116. doi:10.1038/nature23275
Zhang, X., Hamblin, M. H., and Yin, K. J. (2017). The long noncoding RNA Malat1: Its physiological and pathophysiological functions. RNA Biol. 14, 1705–1714. doi:10.1080/15476286.2017.1358347
Zhang, Y., Nguyen, T. M., Zhang, X. O., Wang, L., Phan, T., Clohessy, J. G., et al. (2021). Optimized RNA-targeting CRISPR/Cas13d technology outperforms shRNA in identifying functional circRNAs. Genome Biol. 22, 41. doi:10.1186/s13059-021-02263-9
Zheng, Z. Q., Li, Z. X., Guan, J. L., Liu, X., Li, J. Y., Chen, Y., et al. (2020). Long noncoding RNA TINCR-mediated regulation of acetyl-CoA metabolism promotes nasopharyngeal carcinoma progression and chemoresistance. Cancer Res. 80, 5174–5188. doi:10.1158/0008-5472.CAN-19-3626
Zhou, Y., Li, X., and Yang, H. (2020). LINC00612 functions as a ceRNA for miR-214-5p to promote the proliferation and invasion of osteosarcoma in vitro and in vivo. Exp. Cell Res. 392, 112012. doi:10.1016/j.yexcr.2020.112012
Keywords: er stress, glucose deprivation, lncRNA, circRNA, miRNA, ceRNA, RBP, network
Citation: Li X, Li J, Shan G and Wang X (2023) Identification of long non-coding RNA and circular RNA associated networks in cellular stress responses. Front. Genet. 14:1097571. doi: 10.3389/fgene.2023.1097571
Received: 14 November 2022; Accepted: 31 January 2023;
Published: 10 February 2023.
Edited by:
Sonam Dhamija, Council of Scientific and Industrial Research (CSIR), IndiaReviewed by:
Xiaowei Song, Changhai Hospital, ChinaYu-Hang Xing, Massachusetts General Hospital Cancer Center, United States
Copyright © 2023 Li, Li, Shan and Wang. This is an open-access article distributed under the terms of the Creative Commons Attribution License (CC BY). The use, distribution or reproduction in other forums is permitted, provided the original author(s) and the copyright owner(s) are credited and that the original publication in this journal is cited, in accordance with accepted academic practice. No use, distribution or reproduction is permitted which does not comply with these terms.
*Correspondence: Xiaolin Wang, d3hsMjAwODlAdXN0Yy5lZHUuY24=; Ge Shan, c2hhbmdlQHVzdGMuZWR1LmNu