- 1Regenerative Medicine Clinic, State Key Laboratory of Experimental Hematology, National Clinical Research Center for Blood Diseases, Haihe Laboratory of Cell Ecosystem, Institute of Hematology and Blood Diseases Hospital, Chinese Academy of Medical Sciences & Peking Union Medical College, Tianjin, China
- 2Center for Stem Cell Medicine and Department of Stem Cell & Regenerative Medicine, State Key Laboratory of Experimental Hematology, National Clinical Research Center for Blood Diseases, Haihe Laboratory of Cell Ecosystem, Institute of Hematology and Blood Diseases Hospital, Chinese Academy of Medical Sciences and Peking Union Medical College, Tianjin, China
Acute myeloid leukemia (AML) is a heterogeneous hematological malignancy with dismal prognosis. Identification of better biomarkers remained a priority to improve established stratification and guide therapeutic decisions. Therefore, we extracted the RNA sequence data and clinical characteristics of AML from The Cancer Genome Atlas (TCGA) and Genotype-Tissue Expression database (GTEx) to identify the key factors for prognosis. We found UNC93B1 was highly expressed in AML patients and significantly linked to poor clinical features (p < 0.05). We further validated the high expression of UNC93B1 in another independent AML cohort from GEO datasets (p < 0.001) and performed quantitative PCR of patient samples to confirm the overexpression of UNC93B1 in AML (p < 0.005). Moreover, we discovered high level of UNC93B1 was an independent prognostic factor for poorer outcome both in univariate analysis and multivariate regression (p < 0.001). Then we built a nomogram model based on UNC93B1 expression, age, FAB subtype and cytogenetic risk, the concordance index of which for predicting overall survival was 0.729 (p < 0.001). Time-dependent ROC analysis for predicting survival outcome at different time points by UNC93B1 showed the cumulative 2-year survival rate was 43.7%, and 5-year survival rate was 21.9%. The differentially expressed genes (DEGs) between two groups divided by UNC93B1 expression level were enriched in innate immune signaling and metabolic process pathway. Protein–protein interaction (PPI) network indicated four hub genes (S100A9, CCR1, MRC1 and CD1C) interacted with UNC93B1, three of which were also significantly linked to inferior outcome. Furthermore, we discovered high UNC93B1 tended to be infiltrated by innate immune cells, including Macrophages, Dendritic cells, Neutrophils, Eosinophils, and NK CD56dim cells. We also found UNC93B1 had a significantly positive correlation with CD14, CD68 and almost all Toll-like receptors. Finally, we revealed negatively correlated expression of UNC93B1 and BCL2 in AML and conjectured that high-UNC93B1 monocytic AML is more resistant to venetoclax. And we found high MCL-1 expression compensated for BCL-2 loss, thus, we proposed MCL-1 inhibitor might overcome the resistance of venetoclax in AML. Altogether, our findings demonstrated the utility of UNC93B1 as a powerful poor prognostic predictor and alternative therapeutic target.
Introduction
Acute myeloid leukemia (AML) is a heterogenous malignancy of bone marrow which is characterized by clonal expansion and differentiation arrest in myeloid progenitor cells (De Kouchkovsky and Abdul-Hay, 2016), patients used to have rarely optional treatment, largely depending on cytarabine + anthracycline (7 + 3) intensive chemotherapy (Maganti et al., 2018), the most common treatment for AML. Although transplantation is an effectively curable therapy for AML patients, relapse is common and associated with quite poor prognosis (Christopher et al., 2018). Evidence suggested that AML relapse after transplantation was associated with dysregulated pathways that might involve in immune response, such as the process of antigen presentation (Christopher et al., 2018). Diagnosis and treatment of AML have improved over the past few decades, but overall survival (OS) remains less than 50% (Walter and Estey, 2015), and the overall 5-year survival is still low, about 24% (Shallis et al., 2019). Tumor escape in AML is expected to be reversible and an urgent problem needed to be solved. Current strategies offer alternative immunotherapeutic options, and the adaptive arm of the immune system is largely harnessed (Au et al., 2020), such as checkpoint inhibitors PD1/PD-L1 antibodies, which has been lagging far behind solid tumors for its limited efficacy in AML (Liu, 2021). Recently, accumulating evidences suggest that innate immunity might also play an important role in hematopoietic malignancies (Tettamanti et al., 2022), however, the specific mechanisms for some of these events remained unclear.
UNC-93 homolog B1 (UNC93B1), encoding an ER protein with 12 transmembrane domains (Maschalidi et al., 2017), is a key regulator of nucleotide-sensing toll-like receptors (TLRs) (Pelka et al., 2018) that sense invading pathogens and deliver them from the endoplasmic reticulum to their respective endosomal signaling (Kim et al., 2008). Toll-like receptors are the key components of the innate immune system (Sulaiman et al., 2017) and trigger a host defense response (Akira et al., 2006). UNC93B1 is associated with multiple immune diseases, such as systemic lupus erythematosus, influenza and herpes simplex encephalitis (Casrouge et al., 2006; Nakano et al., 2010; Lafferty et al., 2014). Furthermore, It has been reported that UNC93B1 is linked to human tumors such as oral squamous cell carcinomas (Wagai et al., 2019), colon cancer (Zhao et al., 2019), CML (Shokeen et al., 2018), lymphoma (Wang et al., 2016). And recent study revealed that UNC93B1 phenotype is related to survival outcomes after unrelated bone marrow transplantation (Uchino et al., 2021), however, the role of UNC93B1 in AML remains elusive.
This study is aimed at describing the clinical implications and survival-predicted functions of UNC93B1 in AML, and the potential interaction between AML blasts’ metabolism and innate immune response, considering the innate immune system such as innate immune cells and TLR signaling may overcome the current barriers in AML treatment.
Materials and methods
Data acquisition and processing
The RNA-seq data in transcripts per million (TPM) format and relevant clinical information from the TCGA AML cohort were obtained from the TCGA data portal (https://portal.gdc.cancer.gov/projects) (Colaprico et al., 2016). A total of 173 patients with AML and 70 normal people (The GTEx Consortium atlas of, 2020) were included in the TGCA LAML program, and Log2 transformation of the TPM RNA-seq data was performed before further intrasample comparison. 153 AML patients were eligible and extracted for survival analysis after excluding patients without clinical survival outcome.
Quantitative real-time PCR of patient samples
Bone barrow mononuclear cells (BMNC) were Isolated from six AML patients and three healthy donors by Histopaque®-10771 (Sigma-Aldrich) standard procedure. All participants provided written informed consent. The study was approved by ethics review boards of our institution. The clinical information of enrolled patients was listed in Supplementary Table S1. Total RNA of BMNC was isolated with TRIzol reagent (Life Technologies) and then reverse transcribed to complementary DNA (cDNA) using the Evo M-MLV RT Kit (AG) with gDNA Clean. The cDNAs were then mixed with SYBR reagent, Applied Biosystems real-time PCR system were used for gene expression analysis, and the expression level were normalized to GAPDH. The primer sequences for UNC93B1 and GAPDH are shown in Supplementary Table S2.
Establishment of risk-scoring model
Patients were divided into two groups based on the median cutoff value of UNC93B1 expression, and the power of UNC93B1 expression to distinguish AML from healthy individuals was estimated using the receiver operating characteristic (ROC) curve drawn by the pROC software (Schlicker et al., 2007). Various alternative clinical events were analyzed using univariate Cox analysis to evaluate their potential impact on overall survival (OS) in AML patients. Furthermore, a multivariate Cox regression model with independent prognostic factors was applied to construct the final prognostic predictors. Independent factors were then recruited to build the nomogram prognostic model (Motwani et al., 2018). Additionally, calibration (Karapanagiotis et al., 2018) was used to estimate the predictive power of the nomogram model. The nomogram and calibration curve were constructed using the “RMS” R package. Finally, the time-dependent receiver operating characteristic (ROC) analysis (Krischer et al., 2019) was performed to assess the predicted value of UNC93B1 in the prognostic model.
Identification of differentially expressed genes
Based on the median expression of UNC93B1 mRNA, AML patients were divided into two groups (UNC93B1-lower and UNC93B1-higher groups). The differentially expressed genes (DEGs) between the high-UNC93B1 and low-UNC93B1 groups were identified by DESeq2R (Love et al., 2014) package. DEGs were considered here as an adjusted p value < 0.05 and an absolute logarithmic 2-fold change (|log2 FC|) >2.0.
Functional pathway analysis
Functional Gene Ontology annotations were performed using R-package clusterProfiler (Yu et al., 2012), including biological process (BP), cellular component (CC), and molecular function (MF) (Gene Ontology Consortium, 2021), as well as Kyoto Encyclopedia of Genes and Genomes (KEGG) analysis (Kanehisa et al., 2022). To further evaluate the gene function, we also conducted the Gene Set Enrichment Analysis (GSEA) analysis (Szklarczyk et al., 2019) using hallmark gene sets (h.all.v7.2. symbols.gmt) from the MsigDB and it was defined as a statistically significant item with the p value was less than 0.05.
Protein–protein interactions network construction and hub genes identification
Protein–protein interaction (PPI) networks can discover and annotate the biological interactions between cellular proteins. In this study, potential protein–protein interactions were predicted by the website of STRING (https://string-db.org/) (Szklarczyk et al., 2019). The PPI for up-regulated DEGs were constructed and visualized by Cytoscape (version 3.7.1) (Shannon et al., 2003). Then hub genes were found using three different algorithms. Finally, the ggplot2 package was used to map the correlation of UNC93B1 and the expression of the hub genes in AML.
Correlation analysis of immune infiltration and immune related genes
To explore the association between UNC93B1 expression levels and abundance of infiltrating immune cells, we used GSVA (version 1.34.0) to perform the ssGSEA algorithm and identified the qualified correlation of infiltrated immune cells and UNC93B1 expression by Pearson correlation coefficient (Bindea et al., 2013; Hänzelmann et al., 2013). A total of 24 subtypes of infiltrating immune cells were involved, including dendritic cells (DCs), activated dendritic cells (aDCs), immature dendritic cells (iDCs), plasmacytoid dendritic cells (pDCs), B cells, T cells, CD8 positive (CD8+) T cells, T helper cells, T central memory (Tcm) cells, T effector memory (Tem) cells, T follicular helper (Tfh) cells, T gamma delta (Tgd) cells, Th1 cells, Th17 cells, Th2 cells, regulatory T (Treg) cells, cytotoxic cells, eosinophils, macrophages, mast cells, neutrophils, NK cells, NK CD56 (bright) cells, and NK CD56 (dim) cells. Next, we draw a scatterplot in R by ggplot2 to show a quantitative correlation of UNC93B1-expressed level and well-recognized surface markers of specific immune cells (CD14, CD68), which were widely discussed in monocytes/macrophages.
Statistical analysis
Statistical analysis was carried out based on the R package (https://www.r-project.org/, version 3.6.3). Statistically significant was defined as a two-tailed p-value of <0.05. Differences in clinical features based on UNC93B1 expression were defined by the Kruskal–Wallis tests and the Wilcoxon rank sum tests. The Pearson χ2 test was used to detect differences in clinical features between low and high UNC93B1-expressed AML. The survival curve was plotted using the Kaplan-Meier (KM) method, and the logarithmic rank p-value < 0.05 was considered statistically significant. Univariate and multivariate Cox regression analysis were selected to determine prognostic factors. Risk ratios (HR) and 95% confidence intervals (CI) for survival-related genes or events were calculated.
Results
High expression of UNC93B1 and its implicated clinical characteristics in AML
To explore the expression landscape and potential indication of UNC93B1, the mRNA levels of this molecular in tumors and normal tissues were extracted from TCGA database. Pan-cancer analysis revealed that UNC93B1 was widely and highly expressed in most malignancies (Figure 1A), especially in AML (p < 0.001, Figure 1B). We validated the high expression level of UNC93B1 in another GEO datasets (GSE13159, p < 0.001, Figure 1C).
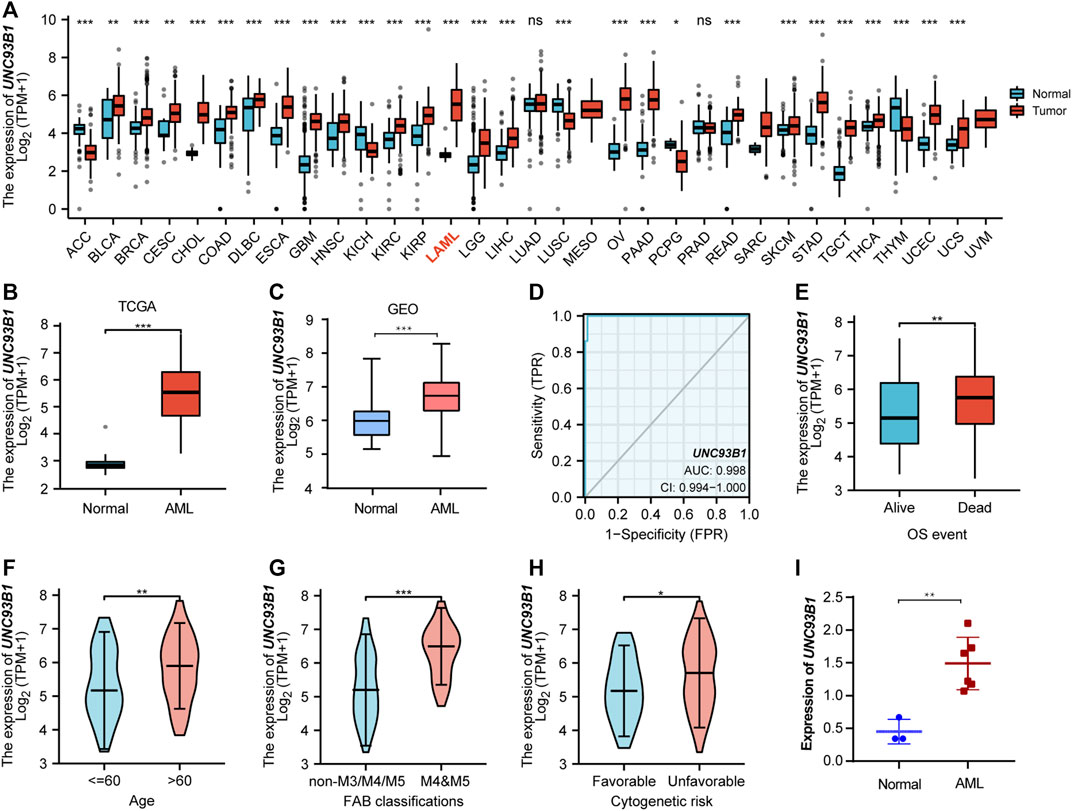
FIGURE 1. High UNC93B1 expression was linked to inferior clinical features in AML. (A) Level of UNC93B1 expression in different tumors from TCGA and GTEx database. (B) Expression levels of UNC93B1 in AML (n = 173) and normal samples (n = 70). (C) Differential expression of UNC93B1 expression between AML patients from GSE13159 (n = 252) and normal control from GSE42519 (n = 44). (D) Receiver operating characteristic analysis (ROC) of UNC93B1 in AML. (E) Expression levels of UNC93B1 in different final OS events. (F) Association of UNC93B1 expression and age. (G) High expression of UNC93B1 in M4/M5 leukemia according to French-American-British (FAB) classification. (H) High level of UNC93B1 relative to GAPDH in AML patients (n = 6) compared to healthy donors (n = 3) using qPCR. (I) Association of high UNC93B1 expression and unfavorable Cytogenetics according to 2017 European Leukemia Net (ELN) genetic risk stratification guidelines of AML. Wilcoxon rank sum test was used between two groups of unpaired samples and Kruskal–Wallis rank sum test was analyzed among multiple groups of samples. ns: p ≥ 0.05, *p < 0.05, **p < 0.01, ***p < 0.001.
Intriguingly, UNC93B1 expression distinguished AML from healthy individuals with a predictive power of 0.998 (95% confidence interval, CI = 0.994–1.000), as shown by AUC values analyzed by the ROC curve (Figure 1D). Therefore, we doubted whether UNC93B1 expression in AML patients is clinically relevant. We surprisingly observed UNC93B1 expression is linked to final OS events (p < 0.01, Figure 1E) and other clinical characteristics (Table 1). The UNC93B1 expression level was higher in AML patients with older age (age > 60, p < 0.01, Figure 1F), non-M3 FAB classification (p < 0.05, Supplementary Figure S1A), especially M4/M5 FAB subtype (p < 0.001, Figure 1G), in which monocytes were prominent. Unexpectedly, unfavorable cytogenetic risk including intermediate or poor cytogenetic risk, which indicated inferior survival in 2017 European Leukemia Net (ELN) genetic risk stratification had higher level of UNC93B1 (p < 0.05, Figure1H). Moreover, among the favorable cytogenetic risk, upregulated UNC93B1 was associated with inv (16) (p < 0.01, Supplementary Figure S1B), which results in the fusion oncoproteins CBFβ-SMMHC. As we all known, AML subtype M4-with eosinophilia (M4-eo) is classified by AML blasts with inv (16) that have a myelomonocytes with immature basophilic granules (Sangle and Perkins, 2011), which is consistent with our results that UNC93B1 is higher in M4/M5 FAB subtypes leukemia. Furthermore, we performed quantitative real-time PCR (qRT-PCR) of our clinical samples to confirm that UNC93B1 were high-expressed in AML-M4/M5 FAB subtypes (p < 0.005, Figure 1I). Whereas, there is no association with gender, WBC counts, FLT3, IDH1, RAS and NPM1 mutation status (Table1). Taken together, high UNC93B1 expression was closely linked to unfavorable clinical characteristics and deserved further exploration in AML.
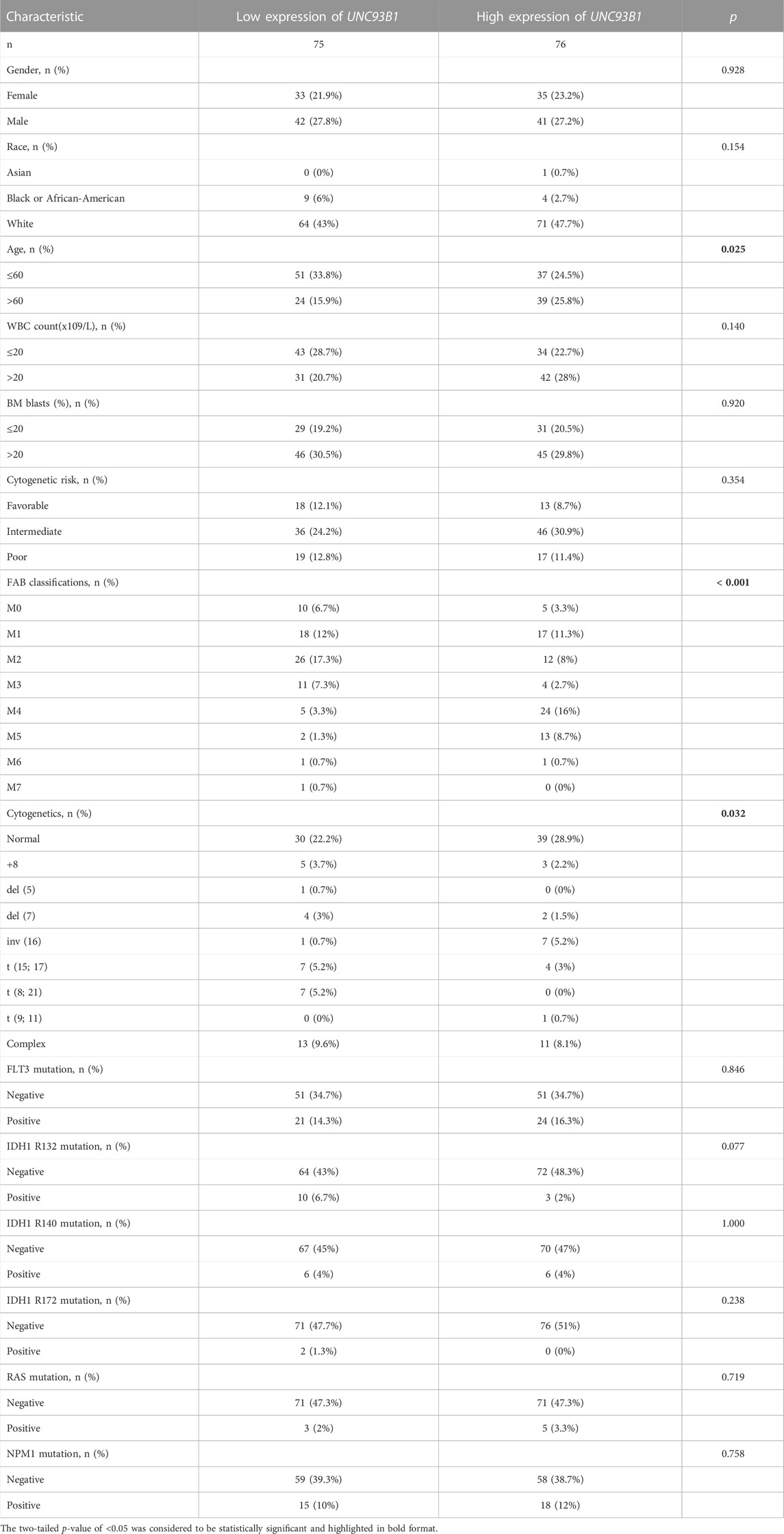
TABLE 1. Clinical characteristics of AML patients in two groups divided by the level of UNC93B1 expression.
High UNC93B1 expression predicts worse prognosis in AML
To explore the prognostic value of UNC93B1 in AML, we draw the overall survival (OS) curve using the Kaplan-Meier method. Patients with higher levels of UNC93B1 than the median expression value had a worse overall survival (HR = 2.08, p = 0.001, Figure 2A). Furthermore, we rationally proposed UNC93B1 level can also distinctly reflect survival outcomes of different subgroups, and the analysis showed higher UNC93B1 level had a more pronounced shortens of survival in AML patients with older age (age > 60, HR: 10.98, p = 0.021, Figure 2B), non-M3 FAB subtype (HR: 1.90, p = 0.004, Figure 2C), WBC counts in peripheral blood ≤ 20 x10^9/L (HR: 2.76, p = 0.001, Figure 2D), and unfavorable cytogenetic risk (including Intermediate cytogenetic risk and Poor cytogenetic risk, HR = 2.49, p = 0.001, Figure 2E). Additionally, higher expression of UNC93B1 also a poor survival factor in AML patients with normal karyotype (HR: 2.29, p = 0.008, Figure 2F), which is predominant in intermediate-risk group (Lin et al., 2017). Interestingly, we found high expression of UNC93B1 just linked to worse prognosis in AML patients without FLT3 (p < 0.05, Supplementary Figure S2A), IDH1 (p < 0.05, Supplementary Figures S2B–D) or NRAS (p < 0.05, Supplementary Figure S2H) mutation, once acquired mutations of these genes, UNC93B1 expression level had no effects on patient’s survival outcome (p > 0.05, Supplementary Figures S2E–G). Meanwhile, there is a significantly negative correlation between UNC93B1 level and OS of both subgroups divided by BM blasts and NPM1 (p < 0.05, Supplementary Figures S2I–L).
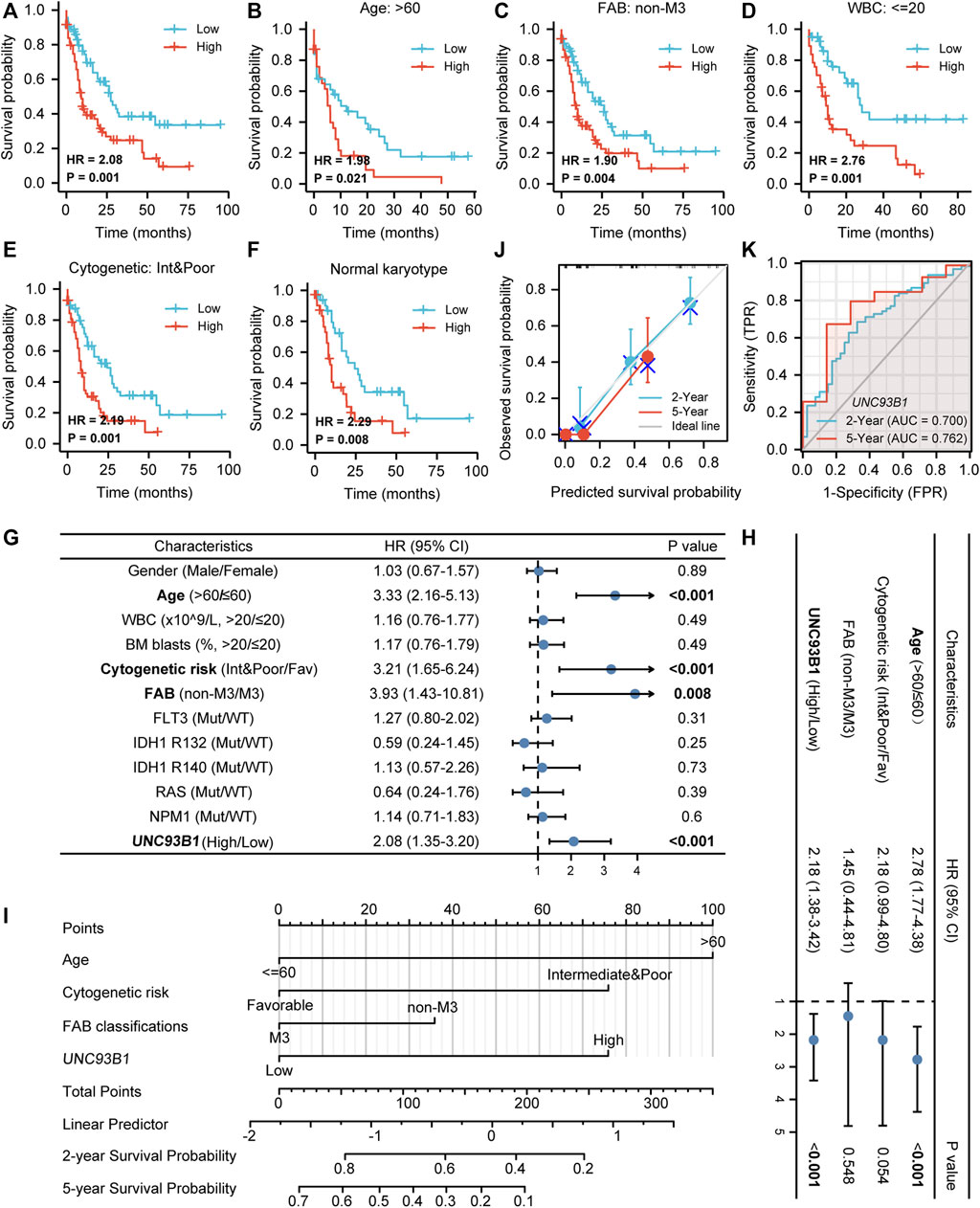
FIGURE 2. Unfavorable prognostic value of high UNC93B1 expression in AML. (A) KM curve analysis of overall survival (OS) between high and low UNC93B1 expression Groups divided by median expression value of UNC93B1 in AML. (B–F) Prognostic value of UNC93B1 expression in each subgroup divided by clinical features. (B) AML patients with age > 60 years old, (C) AML patients with all other subtypes except for M3 according to French-American-British (FAB) classification, (D) AML patients with WBC counts in peripheral blood <20 x10^9/L, (E) AML patients with unfavorable (intermediate and poor) Cytogenetic risk classification according to 2017 European Leukemia Net (ELN) genetic risk stratification guidelines of AML, (F) AML patients with Normal karyotype. (G) Forest plot of Univariate analyses of OS (H) Forest plot of Multivariate analyses of OS. (I) Nomogram model integrates UNC93B1 and other prognostic factors in AML. (J) Calibration curve of nomogram. (K)The Receiver operating characteristic analysis (ROC) by UNC93B1 in predicting 2-year and 5-year OS of AML. Mut: mutation; wt: wild type; Int: intermediate; Fav: favorable; non-M3 including M0, M1, M2, M4, M5, M6 and M7 of FAB classification.
To further identify the indicated prognosis of UNC93B1 in AML and other survival-related factors, we performed univariate and multivariate Cox regression analyses (Supplementary Table S3). Univariate analysis indicated that older age (age > 60, HR: 3.33, 95% CI: 2.16–5.13, p < 0.001), unfavorable cytogenetic risk (including Intermediate cytogenetic risk and Poor cytogenetic risk, HR: 3.21, 95% CI: 1.65–6.24, p < 0.001), non-M3 FAB subtype (HR: 3.93, 95% CI: 1.43–10.81, p = 0.008) and higher UNC93B1 levels (HR: 2.08, 95% CI: 1.35–3.20, p < 0.001) predicted poor survival (Figure 2G). Multivariate Cox regression analyses showed that except for age > 60 (HR: 2.78, 95% CI: 1.77–4.38, p < 0.001), expression level of UNC93B1 (HR: 2.18, 95% CI: 1.38–3.42, p < 0.001) was the only factor which have clinically meaningful effects to overall survival (Figure 2H). Thus, UNC93B1 is an independent prognostic factor found in both univariate and multivariate analysis.
Additionally, a nomogram model was built using age, FAB subtype, cytogenetic risk and UNC93B1 gene expression, which were independent prognostic factors in univariable analysis, to provide the model’s quantitative predictive power for survival outcomes in AML patients (Figure 2I). The predictive OS concordance index (C-index) was 0.729 (95% CI: 0.701–0.756, p < 0.001, Figure 2I), indicating that these four univariable factors had a certain predictive accuracy for OS. Calibration analysis was performed to further assess the predictive value of the nomogram model, in which the calibration curves showed the agreement between the predicted OS and the actual proportion of OS at different time points, and the 5-year median survival rate is sharply decreased compared to 2-year median survival rate (Figure 2J), probably in part due to the higher relapse rate of AML within 2 years clinically. Thus, this nomogram model is well calibrated and had an accuracy to predict the patients’ clinical outcomes. Finally, time-dependent analysis of sensitivity, specificity, and ROC curve was constructed to evaluate UNC93B1 for predicting survival outcome at 2 years or 5 years, and the cumulative 2-year survival rate is 43.7% (2-year AUC = 0.70, 95% CI: 0.60–0.80), while the cumulative 2-year survival rate was dramatically decreased to 21.9% (5-year AUC = 0.76, 95% CI:0.59–0.94, Figure 2K), which is consistent with clinical data. In summary, the survival-predictive model constructed here is significant for AML and UNC93B1 served as an independent prognostic factor for poorer outcome.
Differentially expressed gene analysis and functional signaling pathway enrichment in AML
To explore the potential mechanisms of UNC93B1 in leukemogenesis, we first identified the DEGs between two AML populations divided by high and low UNC93B1 expression. In total, 614 DEGs (|log2(FC)| >2 and adjusted p value < 0.05) were obtained and shown in volcano plots (Figure 3A; Supplementary Table S4), including 342 upregulated genes and 272 downregulated genes. To elucidate the intracellular signaling pathways influenced by the DEGs, we performed GO and KEGG analysis. Firstly, we investigated the biological function of the up-regulated DEGs, the top five of BP enrichment items, CC enrichment items, MF enrichment items as well as top 5 KEGG pathways were obtained from GO annotation (Figure 3B; Supplementary Table S5). We can find two main signaling sets were enriched, one set is immune-related signaling, especially the innate immune associated pathways, such as antigen processing and presentation, macrophage activation, pattern recognition receptor activity, Phagosome and Toll-like receptor signaling pathway were significantly enriched (Figure 3B; Figure 3D; Supplementary Table S5). Another notable signaling cascade was metabolism-related set, including NADPH oxidase complex, carbohydrate binding, lipopeptide binding, superoxide-generating NADPH oxidase activity (Figure 3B; Figure 3E; Supplementary Table S5). Moreover, to better understand the mutual connection among genes and the aforementioned two main signaling sets, we performed an interactive analysis of two main enriched signaling sets and its corresponding genes (Figure 3D-E). We discovered that CD14, TLR8, TLR7, ITGAM, NCF2, TREM2, CYBB, CLEC7A, CD209, NCF1, SLC11A1 and MARCO shared by at least two pathways in innate immune-related sets (Figure 3D); while NCF2, CYBB, NCF1B, NCF1C and NCF1 were commonly enriched in metabolism-related sets (Figure 3E).
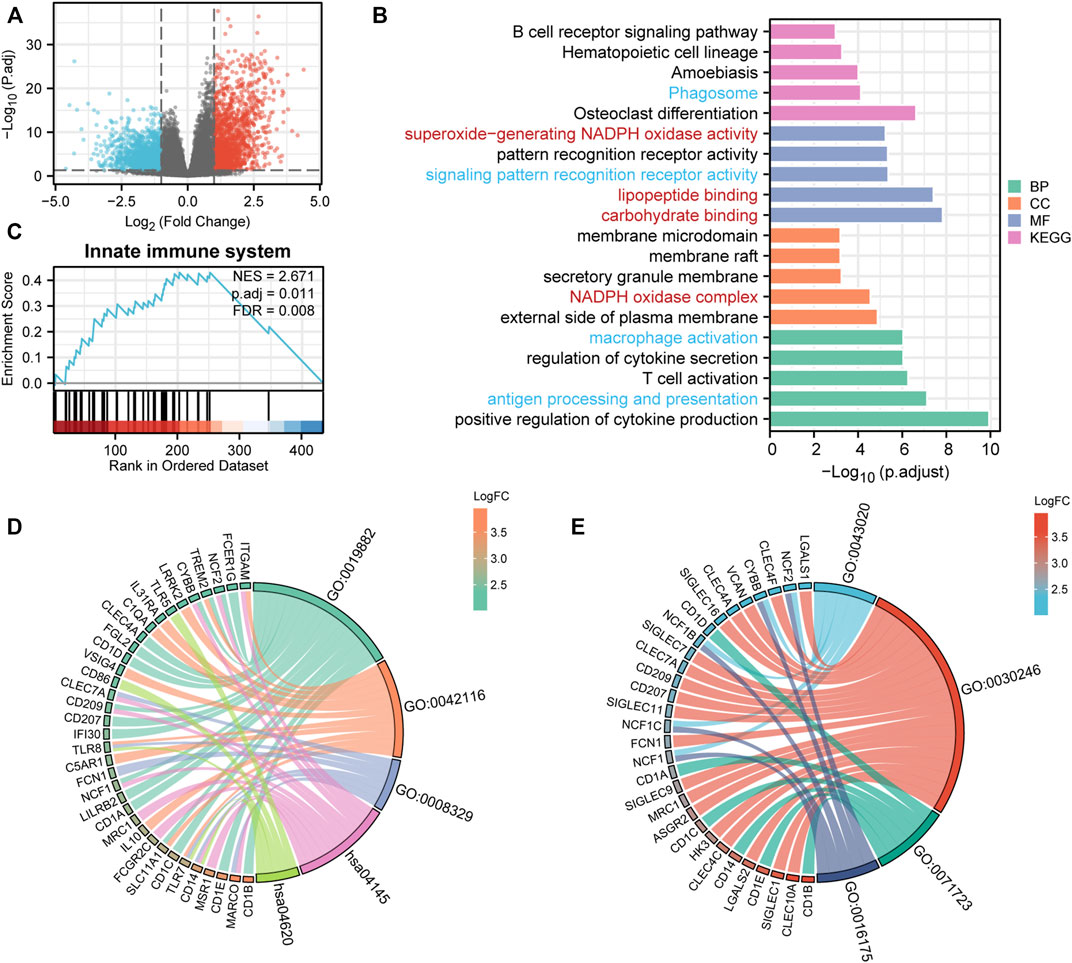
FIGURE 3. DEG analysis and Functional enrichment analyses of DEGs. (A) Volcano plot of DEGs. (B) GO and KEGG pathway of up-regulated DEGs. Red context represents innate-immune signaling sets and blue means metabolic-related pathway sets. (C) Enriched in innate immune system by GSEA analysis. immune system. (D–E) Interactive analysis of enriched pathways in GO/KEGG and corresponding genes. (D) Innate-immune signaling sets and the corresponding genes. GO:0019882, antigen processing and presentation; GO:0042116, macrophage activation; GO:0008329, signaling pattern recognition receptor activity; hsa04145, Phagosome; hsa04620, Toll-like receptor signaling pathway. (E) Metabolic-related signaling sets and the corresponding genes. GO:0043020, NADPH oxidase complex; GO:0030246, carbohydrate binding; GO:0071723, lipopeptide binding; GO:0016175, superoxide-generating NADPH oxidase activity.
To validate the activation of innate immune signaling in UNC93B1-high expressed AML, we next use GSEA analysis to investigate the enrichment pathway, and finally, we found four significantly activated pathways, which were innate immune system (NES = 2.67, p = 0.01, Figure 3C), immunoregulatory interactions between a lymphoid and a non-lymphoid cell (NES = 2.60, p = 0.01, Supplementary Figures S3A), neutrophil degranulation (NES = 2.23, p = 0.01, Supplementary Figures S3B) and adaptive immune system (NES = 2.94, p = 0.01, Supplementary Figure S3C; Supplementary Table S6). So, we assumed that immune system, especially innate immune signaling might play an important role in AML with high level of UNC93B1.
Identification of hub genes connected to UNC93B1
To determine possible proteins directly interact with UNC93B1, protein-protein interaction (PPI) analyses were used. We calculated all of the 342 up-regulated genes and the top 20 hub genes were obtained by the maximal clique centrality (MCC, Supplementary Figures S4A), maximum neighborhood component (MNC, Supplementary Figures S4B), and density of maximum neighborhood component (DMNC, Supplementary Figures S4C) algorithms respectively. Clearly, four common hub genes (S100A9, CCR1, MRC1 and CD1C) shared from the aforementioned three gene lists (Figure 4A), and all of these four genes are related to innate immune response. To gain further deep insight in these molecules, we next turn to interrogate the expression and survival outcome of these four genes. All hub genes were upregulated in AML patients compared to healthy individuals (p < 0.05, Figure 4B). Furthermore, increased expression of S100A9, CCR1 and MRC1 were also associated with poor survival in AML (p < 0.05, Figure 4E). Additionally, UNC93B1 expression positively correlates with S100A9, CCR1, MRC1 and CD1C (p < 0.001, Figure 4C), and the detailed co-expression pattern were shown in Figure 4D.
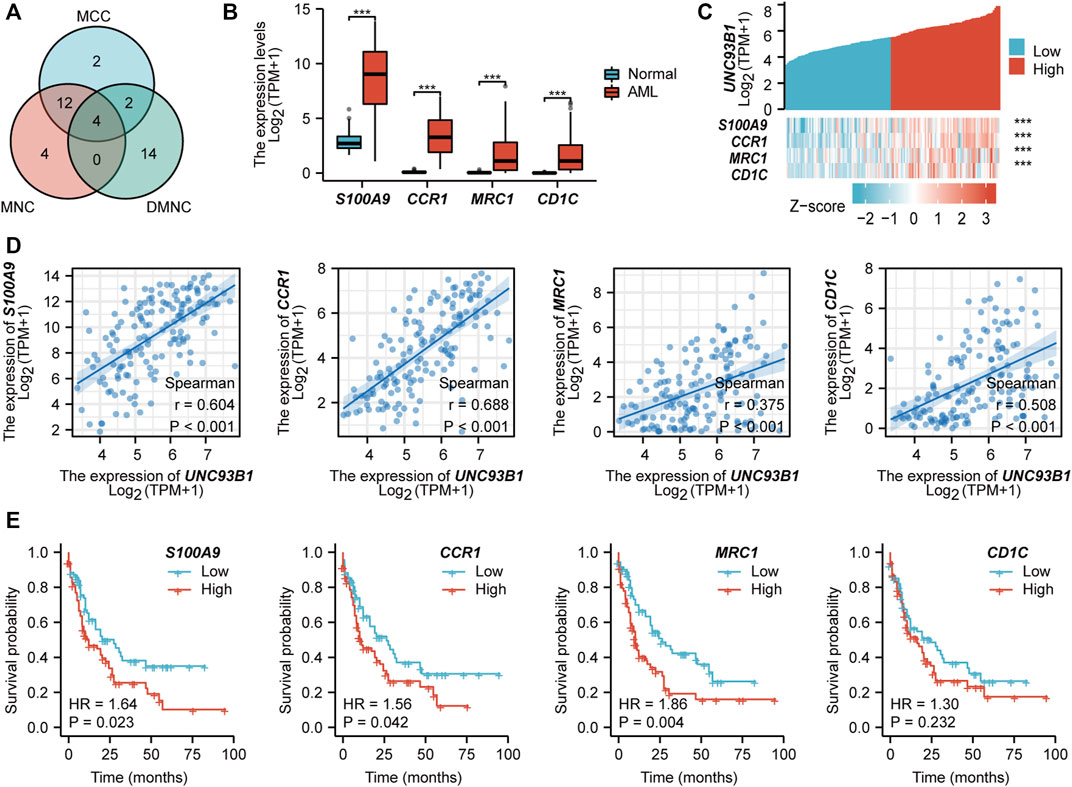
FIGURE 4. PPI network construction and clinical significance of hub genes. (A) Venn diagram of hub genes obtained from three different algorithms. (B) Expression of common hub genes (S100A9, CCR1, MRC1, CD1C) in AML(n = 173) and normal individuals(n = 70). (C) Co-expression pattern of UNC93B1 and four hub genes. (D) Quantified expression correlation of UNC93B1 and four hub genes. (E) Prognostic value of hub genes in AML. (*p < 0.05, **p < 0.01, ***p < 0.001).
Correlation analysis between UNC93B1 and immune cell infiltration
To investigate the association between UNC93B1 expression and AML-infiltrating immune cells, we studied a total of 24 immune cell subtypes. The results displayed that AML with high expression of UNC93B1 had a significant trend to be infiltrated by dendritic cells (DC), immature dendritic cells (iDC), Macrophages, Neutrophils, Eosinophils, NK CD56(dim) cells, Effective Memory T Cell (Tem), Th17 cells and Treg cells (Figure 5A), most of which were responsible for innate immune system instead of adaptive immune. The refined details of a quantified analysis of UNC93B1 and infiltrated innate immune cells (DC, iDC, macrophages, neutrophils, Eosinophils and NK CD56dim cells) by Spearman’s correlation are shown in Figure 5B. More strikingly, we found the level of UNC93B1 expression was positively corelated with macrophages (r = 0.572, p < 0.001), and regulatory T cells (Tregs, r = 0.41, p < 0.001) in AML (Figure 5E), which were responsible for suppressing functional T cell activation and regulating immune systems. We also discovered UNC93B1 expression levels positively correlated with CD14 (r = 0.672, p < 0.001, Figure 5C), a surface antigen that is preferentially expressed on monocytes and a molecule associated to shorter overall survival and lower complete remission rate in AML (Solary et al., 1992; Bradstock et al., 1994). CD68, generally represented macrophages and associated with a lower complete remission in AML (Liu et al., 2022), were also found to be higher in UNC93B1-high expressed AML patients (r = 0.618, p < 0.001, Figure 5C), which further validated the role of innate immune cells in AML pathogenesis and therapy response. TLRs, the most important family of receptors in the early to middle stages of innate immunity response, play a crucial role in recognizing microorganisms and augmenting the inflammatory mediators (Shey et al., 2010; Takeuchi and Akira, 2010; Trejo-de la et al., 2014; Schnetzke et al., 2015), were all extremely upregulated in AML (p < 0.05, Figure 5D), consistent with dysfunctional innate immune response in AML. Unsurprisingly, the level of UNC93B1 was positively and significantly correlated with CD14, CD68, and almost all of TLRs except for TLR3 (p < 0.001, Figure 5C; Figure 5F).
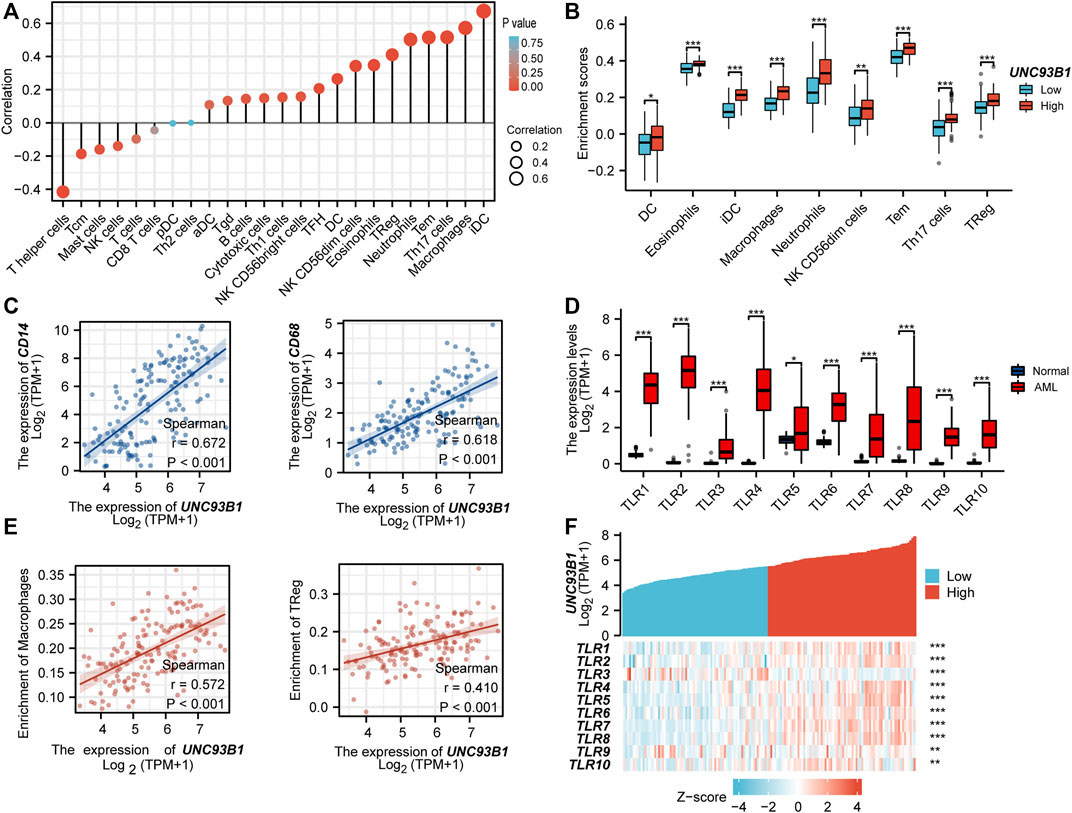
FIGURE 5. UNC93B1 gene expression related to innate immune cell infiltration and innate-immune related molecules. (A) Correlation of UNC93B1 expression and 24 kinds of infiltrated immune cells. (B) High UNC93B1 expression enriched more immune cells, especially innate immune cells. (C) Positive correlation of UNC93B1 expression level with CD14 and CD68. (D) High expression of TLR family in AML (n = 173) compare with normal individuals (n = 70). (E) Positive correlation of UNC93B1 expression level with macrophages and regulatory T cells (Tregs). (F) Expression correlation between UNC93B1 and Toll-like receptors (TLRs) genes in AML. (r means Spearman’s correlation coefficient. *p < 0.05, **p < 0.01, ***p < 0.001.)
UNC93B1 expression in AML is associated with innate immune and metabolic process
To refine the potential mechanism of UNC93B1 on leukemogenesis, we quantified the correlation of expression between UNC93B1 and the genes related to innate immune response-activating signal transduction (Figure 6B) and cytokine metabolic process (Figure 6C), both were significantly enriched in GO/KEGG (Figure 3B). Gene lists related to each pathway were shown in Figure 6A, and CLEC7A, TLR7, TLR8 played important roles in both signaling. C-Type Lectin Domain Family 7 Member A (CLEC7A), encoding a glycoprotein that is a small type II membrane receptor with extracellular C-type lectin-like domain folding and a cytoplasmic domain with an activated motif based on immune receptor tyrosine. Gene Ontology (GO) annotations associated with this gene include carbohydrate binding and pattern recognition receptor activity, which is consistent with the mutually shared genes in aforementioned immune and metabolic pathway. What’s more, TLR7 and TLR8 predominantly expressed in dendritic cells, TLR8 is also expressed in monocytes, macrophages, neutrophils, and Tregs (Gorden et al., 2005; Peng et al., 2005). TLR7 activation triggers the production of interferon-α (Gorden et al., 2005), and TLR8 activation leads to the production of immunomodulatory cytokines, such as interleukin-12, interleukin-18, and pro-inflammatory cytokines, such as tumor necrosis factor alpha and interleukin-1β (Cristescu et al., 2018), which is associated with leukemic microenvironment and immune tolerance (Pyzer et al., 2017). Therefore, the high expression of TLRs on leukemia cells and the consequences of TLR activation might be a potentially therapeutic target.
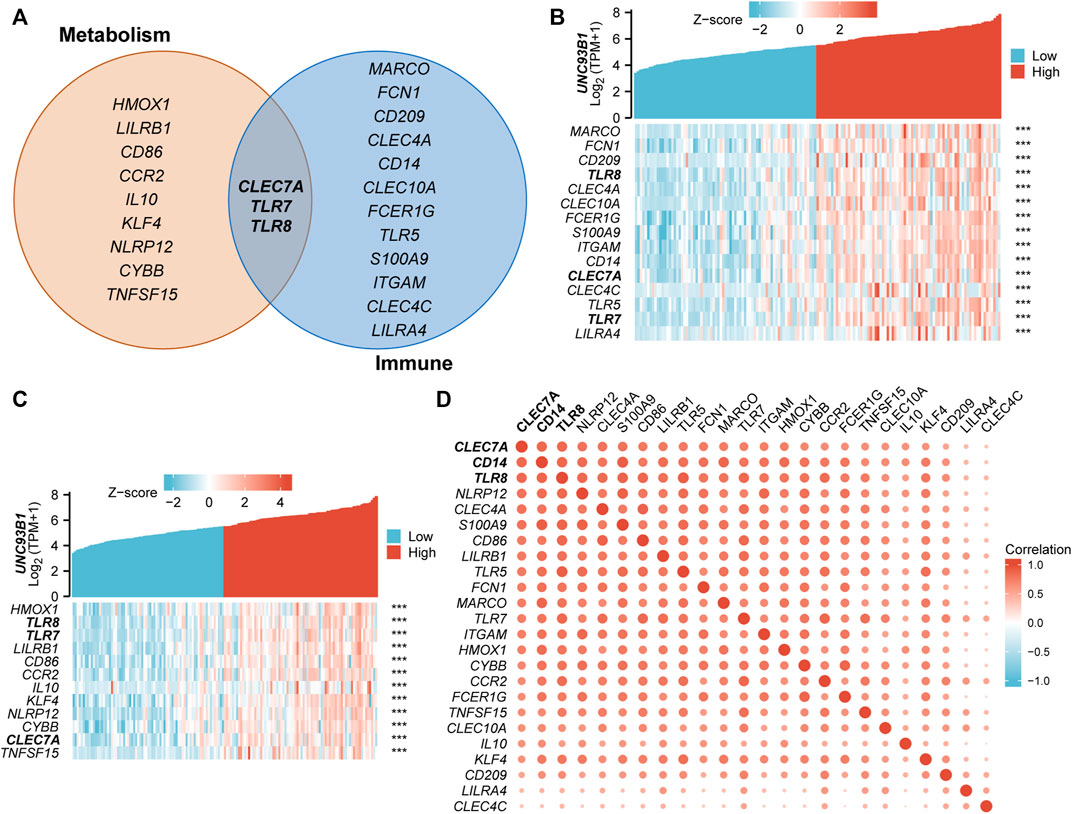
FIGURE 6. Association of UNC93B1 with innate immune and metabolic process. (A) Venn diagram of genes enriched in innate immune response-activating signal transduction pathway (right) and cytokine metabolic process signaling (left). (B) Co-expression heatmap of UNC93B1 and innate immune response-activating signal transduction related genes. (C) Co-expression heatmap of UNC93B1 and cytokine metabolic process related genes. (D) Positive correlation of genes’ expression corresponding to innate immune response-activating signal transduction and cytokine metabolic process.
There have been multiple studies that indicate innate immune cells undergo substantial metabolic reprogramming following activation (Angiari et al., 2020). Meanwhile, various metabolic enzymes involved in glycolysis and mitochondrial metabolic pathways have been found to play a vital role in influencing innate immune cell function (Li et al., 2019). So, we supposed the coupling of metabolic process and innate immune responses play an essential role in the AML development, especially in the patients with high-expressed UNC93B1. And we draw the correlation heat maps of the key regulators in metabolic process and innate immune pathways (Figure 6D), which demonstrated obvious co-expression patterns. Therefore, we conclude that immunometabolism has taken on a different meaning in AML by unknown immune-metabolism interactions, for example, contributions of key metabolic factors contribute to immune cell development or fate; in turn, responses of immune cells resulted in metabolic reprogramming. Altogether, metabolic system and innate immune affected AML patients with aberrantly high-expressed UNC93B1 through combined strategies.
UNC93B1 is preferentially high expressed on monocytic AML and associated with losing expression of venetoclax target BCL2
As we show in this work, UNC93B1 is high-expressed in AML, especially in M4/M5 (Figure 1G). Recent studies have shown that AML hierarchy composition as a determinant of response to targeted therapy (Zeng et al., 2022), and Monocytic AML (M5) is intrinsically resistant to venetoclax + azacytidine (Pei et al., 2020). We then further analyzed the expression of UNC93B1 in AML and found that the level of UNC93B1 is significantly higher in FAB-M5 patients than any other FAB-subtype patient (p < 0.05, Figure 7A-B), which is consistent with the positive correlation expression of UNC93B1 and CD14, CD68 shown in Figure 5C. As we demonstrated in Figure 3B, another signaling enriched by UNC93B1 were metabolic pathways, and AML blasts might switch from BCL2-dependence to MCL1-dependence to drive energy metabolism under specific circumstances (Pei et al., 2020), we next focused on expression of BCL2 and MCL-1 in AML. Contrary to the increasing expression of UNC93B1 in FAB-M5 AML, BCL2 expression decreased remarkably in FAB-M5 AML (p < 0.05, Figures 7C–D). However, MCL1 presented similar expression pattern to UNC93B1 in AML, tended to be high-expressed in FAB-M5 (p < 0.05, Figures 7E–F). To clear the quantitative expression correlation of UNC93B1, BCL2 and MCL1, we performed Spearman Rank Correlation Analysis, and as expected, we revealed significantly negative correlation of UNC93B1 expression and BCL2 expression (r = −0.345, p < 0.001, Figure 7G), meanwhile, consistent with the previous results, MCL-1 expression is positively related to UNC93B1 level in AML (r = 0.347, p < 0.001, Figure 7H). Given that venetoclax (VEN) is an inhibitor specific to BCL2 and some studies have discovered that the level of BCL2 expression is closely related to VEN response in vitro (Souers et al., 2013; Pan et al., 2014), we hypothesized high-UNC93B1 AML might be resistant to venetoclax, and selective MCL1 inhibitor, such as VU661013 (Ramsey et al., 2018), alone or in combination with AZA might be effective in venetoclax-resistant AML and high-UNC93B1 AML. Together, these data indicated that high-UNC93B1 expressed AML cells shows greater dependence on MCL1 than BCL2 for survival, and UNC93B1 might be a biomarker to predicting drug response in acute myeloid leukemia.
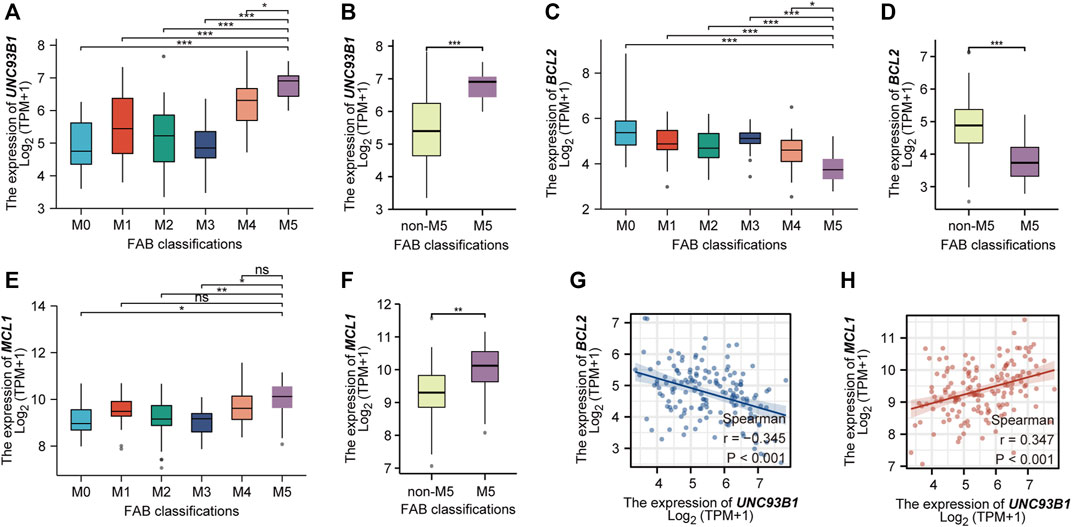
FIGURE 7. High expression of UNC93B1 in FAB-M5 AML and association with losing expression of venetoclax target BCL2. (A) The expression level of UNC93B1 in different FAB subtypes (M0-M5) of AML. M6 and M7 were excluded because of small numbers (n ≤ 3). (B) The expression level of UNC93B1 in FAB-M5 AML and non-M5 AML. non-M5 including M0, M1, M2, M3, M4, M6 and M7 of FAB classification. (C) The expression level of BCL2 in different FAB subtypes (M0-M5) of AML. (D) The expression level of BCL2 in FAB-M5 AML and non-M5 AML. (E)The expression level of MCL1 in different FAB subtypes (M0-M5) of AML. (F) The expression level of MCL1 in FAB-M5 AML and non-M5 AML. (G) The negative correlation of UNC93B1 expression and BCL-2 expression in AML. (H) The positive correlation of UNC93B1 expression and MCL1 expression in AML. (r means Spearman’s correlation coefficient. Wilcoxon rank sum test was used between two groups of unpaired samples, ns: p ≥ 0.05, *p < 0.05, **p < 0.01, ***p < 0.001.)
Discussion
Acute myeloid leukemia (AML) is a heterogeneous group of hematologic malignancies with low survival rates and with most patients eventually relapsing and dying of progressive disease. Resistance to chemotherapy and relapse after HSCT were major obstacles in the cure of AML patients, and is often attributed to the immune escape of AML blasts (Dermime et al., 1997; Masuda et al., 2007; Vago et al., 2009; Stölzel et al., 2012; Dama et al., 2019). Compared to the rapid development of immunotherapies in solid tumors, PD1/PD-L1 antibodies showed limited clinical activity in AML (Zhang et al., 2009; Zhou et al., 2010; Zhou et al., 2011), we still have a long way to develop immunotherapies for myeloid leukemia. Recent lines of investigation suggest that innate immunity might play an important role in hematopoietic malignancies (Tettamanti et al., 2022).
The ER membrane protein uncoordinated 93 homolog B1 (UNC93B1) plays an important role in regulating intracellular TLR signaling, which is vital in innate immune system. Few studies reported that UNC93B1 had a relation with some specific type of solid tumor (Wagai et al., 2019; Zhao et al., 2019). However, it is remains elusive whether UNC93B1 has an impact on AML.
We first investigated the association between UNC93B1 expression and clinical features of AML. Surprisingly, UNC93B1 expression observed in AML was aberrantly up-regulated, which correlated with adverse clinical characteristics and poor survival. Next, we used the integrated bioinformatics analysis of DEGs to explore the potential pathogenic mechanisms of UNC93B1. GO/KEGG and GSEA analyses consistently pointed to dysregulated innate immune signaling in AML patients with high-UNC93B1 expression. Therefore, we proposed that UNC93B1 is involved in innate immune system, especially TLR signaling pathway, which may contribute to the poor outcomes in AML.
In addition, we demonstrated that S100A9, CCR1, MRC1 and CD1C act as hub genes for high-UNC93B1 AML, and three (S100A9, CCR1, MRC1) of them were also related to poor survival of AML. S100A9 is a calcium- and zinc-binding protein that plays an essential role in regulating inflammatory processes and immune response. CCR1, Macrophage Inflammatory Protein 1-Alpha Receptor, participated in recruiting the effector immune cells to the inflammatory site. MRC1, Macrophage Mannose Receptor 1-Like Protein 1, involve in mediating the endocytosis of glycoproteins by macrophages. CD1C, T-Cell Surface Glycoprotein CD1c, involved in Dendritic Cells Developmental Lineage Pathway and Innate Immune System. Thus, all hubs we obtained here were innate immune related genes. Furthermore, high levels of innate immune cells infiltration and wide expression of TLR signaling genes also validated innate immune activation, which further support our hypothesis.
Additionally, we found the higher expression of UNC93B1, the more innate immune cells infiltrated such as macrophages and dendric cells. Instead of activating functional effector T cells, UNC93B tended to recruit regulatory T cells, which were responsible for the initiation and development of acute and chronic leukemia (Moon et al., 2011; Bachireddy et al., 2015; Wan et al., 2020), and mediated immune escape of myeloblast in de novo AML (Wan et al., 2020). It is widely believed that metabolism and immune cell function are related (Brown and Byersdorfer, 2020), and innate immune training is associated with metabolic reprogramming (Bandyopadhaya et al., 2016). So, we next investigated the cross-talk between innate immune and metabolic process, unexpectedly, we found they were positively correlated. Finally, we supposed that a large fraction of immune cells infiltrated in AML are from the innate arm of the immune system. These classical innate immune cells (macrophages, DCs, neutrophils, Basophils, Eosinophils, Mast cells and NK cells) (Bandyopadhaya et al., 2016) offers an alternative immunotherapeutic option to attack tumor cells, and targeting TLRs or metabolism signaling dysregulated by UNC93B1 might be an effective treatment too. We believed that more tailored immunometabolism-therapeutic strategies for the future of AML treatment deserved to be deeply explored.
A selective inhibitor of BCL-2, Venetoclax (VEN), has shown the advancement in the treatment of AML patients, especially in older patients (Konopleva et al., 2016). The combination of hypomethylating drugs with venetoclax had been approved by FDA for de novo AML patients over the age of 75 or who are not suitable for intensive chemotherapy (Konopleva et al., 2016). However, the widespread use of Venetoclax presented us with new challenges to drug resistance, especially in patients with relapsed/refractory AML (DiNardo et al., 2018; Maiti et al., 2021). Here, we show that UNC93B1 is associated with Venetoclax resistance in AML due to BCL2 loss and discuss the potential of MCL-1 inhibitors to overcome the resistance.
Our results provide the first proof-of-principle that UNC93B1 is an innate immune related gene, which can serve as prognostic and therapeutic biomarkers. However, several limitations exist with our studies. Firstly, we investigated the diagnostic and prognostic effect of UNC93B1 from the public TCGA AML datasets, which needs to be validated in larger clinical cohorts in the future. Secondly, the interaction and underlying molecular mechanisms between UNC93B1 and the innate immune response lack biological validation. Last but not least, the significance of UNC93B1 in predicting venetoclax resistance and the simultaneous loss of BCL2 in high-UNC93B1 monocytic AML subpopulations need further experiments. Therefore, clinical trials of TLR inhibitors, MCL-1 inhibitors, innate immune cell therapy and further functional validation in the laboratory are required to overcome the above shortcomings.
Conclusion
We identified a novel gene (UNC93B1) for AML, which serve as a critical candidate of leukemic biomarker and prognostic predictor. Importantly, innate immune response and metabolism signaling dysregulated by UNC93B1 deserved further explorations to define its therapeutic potential, and immuno-metabolism combined strategies might hold great prospect for various applications in AML.
Data availability statement
The original contributions presented in the study are included in the article/Supplementary Material, further inquiries can be directed to the corresponding author.
Ethics statement
The studies involving human participants were reviewed and approved by Institute of Hematology and Blood Diseases Hospital, Chinese Academy of Medical Sciences. The patients/participants provided their written informed consent to participate in this study. Written informed consent was obtained from the individual(s) for the publication of any potentially identifiable images or data included in this article.
Author contributions
QL, designed the experiments; QL, and JS, performed the study and wrote the paper; HP, ZG, JZ, WL, LZ, and LF, gave some critical suggestions; YC, WY, contributed to the data analysis. JS gave an approval of the final manuscript.
Funding
This work was supported by the National Natural Science Foundation of China (82270145).
Conflict of interest
The authors declare that the research was conducted in the absence of any commercial or financial relationships that could be construed as a potential conflict of interest.
Publisher’s note
All claims expressed in this article are solely those of the authors and do not necessarily represent those of their affiliated organizations, or those of the publisher, the editors and the reviewers. Any product that may be evaluated in this article, or claim that may be made by its manufacturer, is not guaranteed or endorsed by the publisher.
Supplementary material
The Supplementary Material for this article can be found online at: https://www.frontiersin.org/articles/10.3389/fgene.2023.1063227/full#supplementary-material
SUPPLEMENTARY FIGURE S1 | Association of UNC93B1 expression and clinical characteristics.(A) High level of UNC93B1 in non-M3 leukemia compared to M3 leukemia according to French-American-British (FAB) classification. (B) Differential expression of UNC93B1 according to cytogenetics. ns: p ≥ 0.05, *p < 0.05, **p < 0.01, ***p < 0.001.
SUPPLEMENTARY FIGURE S2 | Survival analysis of UNC93B1 in different subgroups based on clinical characteristics.(A) AML patients without FLT3 mutation. (B–D) AML patients without IDH1 mutation. (E) AML patients with FLT3 mutation. (F) AML patients with IDH1 R132 mutation. (G) AML patients with IDH1 R140 mutation. (H) AML patients without RAS mutation. (I) AML patients with BM blasts (%) ≤ 20%. (J) AML patients with BM blasts (%) > 20%. (K) AML patients without NPM1 mutation. (L) AML patients with NPM1 mutation.
SUPPLEMENTARY FIGURE S3 | GSEA analysis of DEGs.(A) Enriched in immunoregulatory interactions between a lymphoid and a non-lymphoid cell, (B) Enriched in neutrophil degranulation, (C) Enriched in adaptive immune system.
SUPPLEMENTARY FIGURE S4 | PPI network construction.(A-C) Top 20 hub genes selected from the PPI network of up-regulated encoding DEGs calculated by (A) MCC, (B) MNC, (C) DMNC algorithms respectively. MCC, maximal clique centrality; MNC, maximum neighborhood component; DMNC, density of maximum neighborhood component.
SUPPLEMENTARY TABLE S1 | Clinical information of AML patients involved in this study.
SUPPLEMENTARY TABLE S2 | Primer sequence in qPCR.
SUPPLEMENTARY TABLE S3 | Univariate and multivariate Cox regression analyses of survival-related factors in AML.
SUPPLEMENTARY TABLE S4 | Differentially expressed genes (DEGs) identified between UNC93B1-high AML and UC993B1-low AML.
SUPPLEMENTARY TABLE S5 | GO and KEGG pathway analysis of up-regulated genes in UNC93B1-high AML.
SUPPLEMENTARY TABLE S6 | Gene Set Enrichment Analysis.
References
Akira, S., Uematsu, S., and Takeuchi, O. (2006). Pathogen recognition and innate immunity. Cell 124 (4), 783–801. doi:10.1016/j.cell.2006.02.015
Angiari, S., Runtsch, M. C., Sutton, C. E., Palsson-McDermott, E. M., Kelly, B., Rana, N., et al. (2020). Pharmacological activation of pyruvate kinase M2 inhibits CD4(+) T cell pathogenicity and suppresses autoimmunity. Cell metab. 31 (2), 391–405. e8. doi:10.1016/j.cmet.2019.10.015
Au, K. M., Park, S. I., and Wang, A. Z. (2020). Trispecific natural killer cell nanoengagers for targeted chemoimmunotherapy. Sci. Adv. 6 (27), eaba8564. doi:10.1126/sciadv.aba8564
Bachireddy, P., Burkhardt, U. E., Rajasagi, M., and Wu, C. J. (2015). Haematological malignancies: At the forefront of immunotherapeutic innovation. Nat. Rev. Cancer 15 (4), 201–215. doi:10.1038/nrc3907
Bandyopadhaya, A., Tsurumi, A., Maura, D., Jeffrey, K. L., and Rahme, L. G. (2016). A quorum-sensing signal promotes host tolerance training through HDAC1-mediated epigenetic reprogramming. Nat. Microbiol. 1, 16174. doi:10.1038/nmicrobiol.2016.174
Bindea, G., Mlecnik, B., Tosolini, M., Kirilovsky, A., Waldner, M., Obenauf, A. C., et al. (2013). Spatiotemporal dynamics of intratumoral immune cells reveal the immune landscape in human cancer. Immunity 39 (4), 782–795. doi:10.1016/j.immuni.2013.10.003
Bradstock, K., Matthews, J., Benson, E., Page, F., and Bishop, J. (1994). Prognostic value of immunophenotyping in acute myeloid leukemia. Australian Leukaemia Study Group. Blood 84 (4), 1220–1225. doi:10.1182/blood.v84.4.1220.bloodjournal8441220
Brown, R. A., and Byersdorfer, C. A. (2020). Metabolic pathways in alloreactive T cells. Front. Immunol. 11, 1517. doi:10.3389/fimmu.2020.01517
Casrouge, A., Zhang, S. Y., Eidenschenk, C., Jouanguy, E., Puel, A., Yang, K., et al. (2006). Herpes simplex virus encephalitis in human UNC-93B deficiency. Science 314 (5797), 308–312. doi:10.1126/science.1128346
Christopher, M. J., Petti, A. A., Rettig, M. P., Miller, C. A., Chendamarai, E., Duncavage, E. J., et al. (2018). Immune escape of relapsed AML cells after allogeneic transplantation. N. Engl. J. Med. 379 (24), 2330–2341. doi:10.1056/NEJMoa1808777
Colaprico, A., Silva, T. C., Olsen, C., Garofano, L., Cava, C., Garolini, D., et al. (2016). TCGAbiolinks: An R/bioconductor package for integrative analysis of TCGA data. Nucleic Acids Res. 44 (8), e71. doi:10.1093/nar/gkv1507
Cristescu, R., Mogg, R., Ayers, M., Albright, A., Murphy, E., Yearley, J., et al. (2018). Pan-tumor genomic biomarkers for PD-1 checkpoint blockade-based immunotherapy. Science 362 (6411), eaar3593. doi:10.1126/science.aar3593
Dama, P., Tang, M., Fulton, N., Kline, J., and Liu, H. (2019). Gal9/Tim-3 expression level is higher in AML patients who fail chemotherapy. J. Immunother. cancer 7 (1), 175. doi:10.1186/s40425-019-0611-3
De Kouchkovsky, I., and Abdul-Hay, M. (2016). Acute myeloid leukemia: A comprehensive review and 2016 update. Blood Cancer J. 6 (7), e441. doi:10.1038/bcj.2016.50
Dermime, S., Mavroudis, D., Jiang, Y. Z., Hensel, N., Molldrem, J., and Barrett, A. J. (1997). Immune escape from a graft-versus-leukemia effect may play a role in the relapse of myeloid leukemias following allogeneic bone marrow transplantation. Bone marrow Transplant. 19 (10), 989–999. doi:10.1038/sj.bmt.1700778
DiNardo, C. D., Rausch, C. R., Benton, C., Kadia, T., Jain, N., Pemmaraju, N., et al. (2018). Clinical experience with the BCL2-inhibitor venetoclax in combination therapy for relapsed and refractory acute myeloid leukemia and related myeloid malignancies. Am. J. Hematol. 93 (3), 401–407. doi:10.1002/ajh.25000
Gene Ontology Consortium (2021). The gene Ontology resource: Enriching a GOld mine. Nucleic acids Res. 49 (D1), D325–D334. doi:10.1093/nar/gkaa1113
Gorden, K. B., Gorski, K. S., Gibson, S. J., Kedl, R. M., Kieper, W. C., Qiu, X., et al. (2005). Synthetic TLR agonists reveal functional differences between human TLR7 and TLR8. J. Immunol. Baltim. Md 1950) 174 (3), 1259–1268. doi:10.4049/jimmunol.174.3.1259
Hänzelmann, S., Castelo, R., and Guinney, J. (2013). Gsva: Gene set variation analysis for microarray and RNA-seq data. BMC Bioinforma. 14, 7. doi:10.1186/1471-2105-14-7
Kanehisa, M., Sato, Y., and Kawashima, M. (2022). KEGG mapping tools for uncovering hidden features in biological data. Protein Sci. a Publ. Protein Soc. 31 (1), 47–53. doi:10.1002/pro.4172
Karapanagiotis, S., Pharoah, P. D. P., Jackson, C. H., and Newcombe, P. J. (2018). Development and external validation of prediction models for 10-year survival of invasive breast cancer. Comparison with PREDICT and CancerMath. Clin. Cancer Res. 24 (9), 2110–2115. doi:10.1158/1078-0432.CCR-17-3542
Kim, Y. M., Brinkmann, M. M., Paquet, M. E., and Ploegh, H. L. (2008). UNC93B1 delivers nucleotide-sensing toll-like receptors to endolysosomes. Nature 452 (7184), 234–238. doi:10.1038/nature06726
Konopleva, M., Pollyea, D. A., Potluri, J., Chyla, B., Hogdal, L., Busman, T., et al. (2016). Efficacy and biological correlates of response in a phase II study of venetoclax monotherapy in patients with acute myelogenous leukemia. Cancer Discov. 6 (10), 1106–1117. doi:10.1158/2159-8290.CD-16-0313
Krischer, J. P., Liu, X., Vehik, K., Akolkar, B., Hagopian, W. A., Rewers, M. J., et al. (2019). Predicting islet cell autoimmunity and type 1 diabetes: An 8-year TEDDY study progress report. Diabetes Care 42 (6), 1051–1060. doi:10.2337/dc18-2282
Lafferty, E. I., Flaczyk, A., Angers, I., Homer, R., d'Hennezel, E., Malo, D., et al. (2014). An ENU-induced splicing mutation reveals a role for Unc93b1 in early immune cell activation following influenza A H1N1 infection. Genes Immun. 15 (5), 320–332. doi:10.1038/gene.2014.22
Li, X., Gong, W., Wang, H., Li, T., Attri, K. S., Lewis, R. E., et al. (2019). O-GlcNAc transferase suppresses inflammation and necroptosis by targeting receptor-interacting serine/threonine-protein kinase 3. Immunity 50 (3), 1115–1190. e6. doi:10.1016/j.immuni.2019.03.008
Lin, P. H., Li, H. Y., Fan, S. C., Yuan, T. H., Chen, M., Hsu, Y. H., et al. (2017). A targeted next-generation sequencing in the molecular risk stratification of adult acute myeloid leukemia: Implications for clinical practice. Cancer Med. 6 (2), 349–360. doi:10.1002/cam4.969
Liu, H. (2021). Emerging agents and regimens for AML. J. Hematol. Oncol. 14 (1), 49. doi:10.1186/s13045-021-01062-w
Liu, Z. H., Wang, P. P., and Yan, X. J. (2022). The expression and clinical correlation of the CD68 factor in acute myeloid leukemia patients. Zhongguo shi yan xue ye xue za zhi 30 (1), 77–83. doi:10.19746/j.cnki.issn.1009-2137.2022.01.013
Love, M. I., Huber, W., and Anders, S. (2014). Moderated estimation of fold change and dispersion for RNA-seq data with DESeq2. Genome Biol. 15 (12), 550. doi:10.1186/s13059-014-0550-8
Maganti, H. B., Jrade, H., Cafariello, C., Manias Rothberg, J. L., Porter, C. J., Yockell-Lelièvre, J., et al. (2018). Targeting the MTF2-MDM2 Axis sensitizes refractory acute myeloid leukemia to chemotherapy. Cancer Discov. 8 (11), 1376–1389. doi:10.1158/2159-8290.CD-17-0841
Maiti, A., Rausch, C. R., Cortes, J. E., Pemmaraju, N., Daver, N. G., Ravandi, F., et al. (2021). Outcomes of relapsed or refractory acute myeloid leukemia after frontline hypomethylating agent and venetoclax regimens. Haematologica 106 (3), 894–898. doi:10.3324/haematol.2020.252569
Maschalidi, S., Nunes-Hasler, P., Nascimento, C. R., Sallent, I., Lannoy, V., Garfa-Traore, M., et al. (2017). UNC93B1 interacts with the calcium sensor STIM1 for efficient antigen cross-presentation in dendritic cells. Nat. Commun. 8 (1), 1640. doi:10.1038/s41467-017-01601-5
Masuda, K., Hiraki, A., Fujii, N., Watanabe, T., Tanaka, M., Matsue, K., et al. (2007). Loss or down-regulation of HLA class I expression at the allelic level in freshly isolated leukemic blasts. Cancer Sci. 98 (1), 102–108. doi:10.1111/j.1349-7006.2006.00356.x
Moon, H. W., Kim, B. H., Park, C. M., Hur, M., Yun, Y. M., Kim, S. Y., et al. (2011). CD4+CD25highFoxP3+ regulatory T-cells in hematologic diseases. Korean J. laboratory Med. 31 (4), 231–237. doi:10.3343/kjlm.2011.31.4.231
Motwani, S. S., McMahon, G. M., Humphreys, B. D., Partridge, A. H., Waikar, S. S., and Curhan, G. C. (2018). Development and validation of a risk prediction model for acute kidney injury after the first course of cisplatin. J. Clin. Oncol. 36 (7), 682–688. doi:10.1200/JCO.2017.75.7161
Nakano, S., Morimoto, S., Suzuki, S., Watanabe, T., Amano, H., and Takasaki, Y. (2010). Up-regulation of the endoplasmic reticulum transmembrane protein UNC93B in the B cells of patients with active systemic lupus erythematosus. Rheumatol. Oxf. Engl. 49 (5), 876–881. doi:10.1093/rheumatology/keq001
Pan, R., Hogdal, L. J., Benito, J. M., Bucci, D., Han, L., Borthakur, G., et al. (2014). Selective BCL-2 inhibition by ABT-199 causes on-target cell death in acute myeloid leukemia. Cancer Discov. 4 (3), 362–375. doi:10.1158/2159-8290.CD-13-0609
Pei, S., Pollyea, D. A., Gustafson, A., Stevens, B. M., Minhajuddin, M., Fu, R., et al. (2020). Monocytic subclones confer resistance to venetoclax-based therapy in patients with acute myeloid leukemia. Cancer Discov. 10 (4), 536–551. doi:10.1158/2159-8290.CD-19-0710
Pelka, K., Bertheloot, D., Reimer, E., Phulphagar, K., Schmidt, S. V., Christ, A., et al. (2018). The chaperone UNC93B1 regulates toll-like receptor stability independently of endosomal TLR transport. Immunity 48 (5), 911–922. e7. doi:10.1016/j.immuni.2018.04.011
Peng, G., Guo, Z., Kiniwa, Y., Voo, K. S., Peng, W., Fu, T., et al. (2005). Toll-like receptor 8-mediated reversal of CD4+ regulatory T cell function. Science 309 (5739), 1380–1384. doi:10.1126/science.1113401
Pyzer, A. R., Stroopinsky, D., Rajabi, H., Washington, A., Tagde, A., Coll, M., et al. (2017). MUC1-mediated induction of myeloid-derived suppressor cells in patients with acute myeloid leukemia. Blood 129 (13), 1791–1801. doi:10.1182/blood-2016-07-730614
Ramsey, H. E., Fischer, M. A., Lee, T., Gorska, A. E., Arrate, M. P., Fuller, L., et al. (2018). A novel MCL1 inhibitor combined with venetoclax rescues venetoclax-resistant acute myelogenous leukemia. Cancer Discov. 8 (12), 1566–1581. doi:10.1158/2159-8290.CD-18-0140
Sangle, N. A., and Perkins, S. L. (2011). Core-binding factor acute myeloid leukemia. Archives pathology laboratory Med. 135 (11), 1504–1509. doi:10.5858/arpa.2010-0482-RS
Schlicker, A., Rahnenführer, J., Albrecht, M., Lengauer, T., and Domingues, F. S. (2007). GOTax: Investigating biological processes and biochemical activities along the taxonomic tree. Genome Biol. 8 (3), R33. doi:10.1186/gb-2007-8-3-r33
Schnetzke, U., Spies-Weisshart, B., Yomade, O., Fischer, M., Rachow, T., Schrenk, K., et al. (2015). Polymorphisms of Toll-like receptors (TLR2 and TLR4) are associated with the risk of infectious complications in acute myeloid leukemia. Genes Immun. 16 (1), 83–88. doi:10.1038/gene.2014.67
Shallis, R. M., Wang, R., Davidoff, A., Ma, X., and Zeidan, A. M. (2019). Epidemiology of acute myeloid leukemia: Recent progress and enduring challenges. Blood Rev. 36, 70–87. doi:10.1016/j.blre.2019.04.005
Shannon, P., Markiel, A., Ozier, O., Baliga, N. S., Wang, J. T., Ramage, D., et al. (2003). Cytoscape: A software environment for integrated models of biomolecular interaction networks. Genome Res. 13 (11), 2498–2504. doi:10.1101/gr.1239303
Shey, M. S., Randhawa, A. K., Bowmaker, M., Smith, E., Scriba, T. J., de Kock, M., et al. (2010). Single nucleotide polymorphisms in toll-like receptor 6 are associated with altered lipopeptide- and mycobacteria-induced interleukin-6 secretion. Genes Immun. 11 (7), 561–572. doi:10.1038/gene.2010.14
Shokeen, Y., Sharma, N. R., Vats, A., Taneja, V., Minhas, S., Jauhri, M., et al. (2018). Identification of prognostic and susceptibility markers in chronic myeloid leukemia using next generation sequencing. Ethiop. J. health Sci. 28 (2), 135–146. doi:10.4314/ejhs.v28i2.5
Solary, E., Casasnovas, R. O., Campos, L., Béné, M. C., Faure, G., Maingon, P., et al. (1992). Surface markers in adult acute myeloblastic leukemia: Correlation of CD19+, CD34+ and cd14+/DR--phenotypes with shorter survival. Groupe d'Etude immunologique des leucémies (GEIL). Leukemia 6 (5), 393–399.
Souers, A. J., Leverson, J. D., Boghaert, E. R., Ackler, S. L., Catron, N. D., Chen, J., et al. (2013). ABT-199, a potent and selective BCL-2 inhibitor, achieves antitumor activity while sparing platelets. Nat. Med. 19 (2), 202–208. doi:10.1038/nm.3048
Stölzel, F., Hackmann, K., Kuithan, F., Mohr, B., Füssel, M., Oelschlägel, U., et al. (2012). Clonal evolution including partial loss of human leukocyte antigen genes favoring extramedullary acute myeloid leukemia relapse after matched related allogeneic hematopoietic stem cell transplantation. Transplantation 93 (7), 744–749. doi:10.1097/TP.0b013e3182481113
Sulaiman, G., Cooke, A., Ffrench, B., Gasch, C., Abdullai, O. A., O'Connor, K., et al. (2017). MyD88 is an essential component of retinoic acid-induced differentiation in human pluripotent embryonal carcinoma cells. Cell Death Differ. 24 (11), 1975–1986. doi:10.1038/cdd.2017.124
Szklarczyk, D., Gable, A. L., Lyon, D., Junge, A., Wyder, S., Huerta-Cepas, J., et al. (2019). STRING v11: Protein-protein association networks with increased coverage, supporting functional discovery in genome-wide experimental datasets. Nucleic acids Res. 47 (D1), D607–d13. doi:10.1093/nar/gky1131
Takeuchi, O., and Akira, S. (2010). Pattern recognition receptors and inflammation. Cell 140 (6), 805–820. doi:10.1016/j.cell.2010.01.022
Tettamanti, S., Pievani, A., Biondi, A., Dotti, G., and Serafini, M. (2022). Catch me if you can: How AML and its niche escape immunotherapy. Leukemia 36 (1), 13–22. doi:10.1038/s41375-021-01350-x
The GTEx Consortium atlas of (2020). The GTEx Consortium atlas of genetic regulatory effects across human tissues. Science 369 (6509), 1318–1330. doi:10.1126/science.aaz1776
Trejo-de la, O. A., Hernández-Sancén, P., and Maldonado-Bernal, C. (2014). Relevance of single-nucleotide polymorphisms in human TLR genes to infectious and inflammatory diseases and cancer. Genes Immun. 15 (4), 199–209. doi:10.1038/gene.2014.10
Uchino, K., Vu Quang, L., Mizuno, S., Horio, T., Yamamoto, H., Hanamura, I., et al. (2021). Donor UNC-93 Homolog B1 genetic polymorphism predicts survival outcomes after unrelated bone marrow transplantation. Genes Immun. 22 (1), 35–43. doi:10.1038/s41435-021-00122-y
Vago, L., Perna, S. K., Zanussi, M., Mazzi, B., Barlassina, C., Stanghellini, M. T., et al. (2009). Loss of mismatched HLA in leukemia after stem-cell transplantation. N. Engl. J. Med. 361 (5), 478–488. doi:10.1056/NEJMoa0811036
Wagai, S., Kasamatsu, A., Iyoda, M., Hayashi, F., Hiroshima, K., Yoshimura, S., et al. (2019). UNC93B1 promotes tumoral growth by controlling the secretion level of granulocyte macrophage colony-stimulating factor in human oral cancer. Biochem. biophysical Res. Commun. 513 (1), 81–87. doi:10.1016/j.bbrc.2019.03.172
Walter, R. B., and Estey, E. H. (2015). Management of older or unfit patients with acute myeloid leukemia. Leukemia 29 (4), 770–775. doi:10.1038/leu.2014.216
Wan, Y., Zhang, C., Xu, Y., Wang, M., Rao, Q., Xing, H., et al. (2020). Hyperfunction of CD4 CD25 regulatory T cells in de novo acute myeloid leukemia. BMC cancer 20 (1), 472. doi:10.1186/s12885-020-06961-8
Wang, J. Q., Beutler, B., Goodnow, C. C., and Horikawa, K. (2016). Inhibiting TLR9 and other UNC93B1-dependent TLRs paradoxically increases accumulation of MYD88L265P plasmablasts in vivo. Blood 128 (12), 1604–1608. doi:10.1182/blood-2016-03-708065
Yu, G., Wang, L. G., Han, Y., and He, Q. Y. (2012). clusterProfiler: an R package for comparing biological themes among gene clusters. Omics a J. Integr. Biol. 16 (5), 284–287. doi:10.1089/omi.2011.0118
Zeng, A. G. X., Bansal, S., Jin, L., Mitchell, A., Chen, W. C., Abbas, H. A., et al. (2022). A cellular hierarchy framework for understanding heterogeneity and predicting drug response in acute myeloid leukemia. Nat. Med. 28 (6), 1212–1223. doi:10.1038/s41591-022-01819-x
Zhang, L., Gajewski, T. F., and Kline, J. (2009). PD-1/PD-L1 interactions inhibit antitumor immune responses in a murine acute myeloid leukemia model. Blood 114 (8), 1545–1552. doi:10.1182/blood-2009-03-206672
Zhao, J., Xue, Y., Pan, Y., Yao, A., Wang, G., Li, D., et al. (2019). Toll-like receptor 3 agonist poly I:C reinforces the potency of cytotoxic chemotherapy via the TLR3-UNC93B1-IFN-β signaling axis in paclitaxel-resistant colon cancer. J. Cell. physiology 234 (5), 7051–7061. doi:10.1002/jcp.27459
Zhou, Q., Munger, M. E., Highfill, S. L., Tolar, J., Weigel, B. J., Riddle, M., et al. (2010). Program death-1 signaling and regulatory T cells collaborate to resist the function of adoptively transferred cytotoxic T lymphocytes in advanced acute myeloid leukemia. Blood 116 (14), 2484–2493. doi:10.1182/blood-2010-03-275446
Keywords: acute myeloid leukemia, UNC93B1, prognostic and therapeutic biomarker, innate immune, metabolism, BCL2
Citation: Li Q, Pan H, Gao Z, Li W, Zhang L, Zhao J, Fang L, Chu Y, Yuan W and Shi J (2023) High-expression of the innate-immune related gene UNC93B1 predicts inferior outcomes in acute myeloid leukemia. Front. Genet. 14:1063227. doi: 10.3389/fgene.2023.1063227
Received: 06 October 2022; Accepted: 06 January 2023;
Published: 18 January 2023.
Edited by:
Jun Qian, Jiangsu University Affiliated People’s Hospital, ChinaReviewed by:
Weiying Gu, First People’s Hospital of Changzhou, ChinaTing-Juan Zhang, Jiangsu University Affiliated People’s Hospital, China
Copyright © 2023 Li, Pan, Gao, Li, Zhang, Zhao, Fang, Chu, Yuan and Shi. This is an open-access article distributed under the terms of the Creative Commons Attribution License (CC BY). The use, distribution or reproduction in other forums is permitted, provided the original author(s) and the copyright owner(s) are credited and that the original publication in this journal is cited, in accordance with accepted academic practice. No use, distribution or reproduction is permitted which does not comply with these terms.
*Correspondence: Jun Shi, c2hpanVuQGloY2Ftcy5hYy5jbg==