- 1Department of Anesthesiology, Clinical Medical College of Yangzhou University, Yangzhou, Jiangsu, China
- 2Department of Anesthesiology, Yangzhou University Affiliated Northern Jiangsu People’s Hospital, Yangzhou, Jiangsu, China
- 3Yangzhou Key Laboratory of Anesthesiology, Yangzhou, Jiangsu, China
Background: Intracerebral hemorrhage (ICH) is a stroke syndrome with high mortality and disability rates, but autophagy’s mechanism in ICH is still unclear. We identified key autophagy genes in ICH by bioinformatics methods and explored their mechanisms.
Methods: We downloaded ICH patient chip data from the Gene Expression Omnibus (GEO) database. Based on the GENE database, differentially expressed genes (DEGs) for autophagy were identified. We identified key genes through protein–protein interaction (PPI) network analysis and analyzed their associated pathways in Gene Ontology (GO) and the Kyoto Encyclopedia of Genes and Genomes (KEGG). Gene-motif rankings, miRWalk and ENCORI databases were used to analyze the key gene transcription factor (TF) regulatory network and ceRNA network. Finally, relevant target pathways were obtained by gene set enrichment analysis (GSEA).
Results: Eleven autophagy-related DEGs in ICH were obtained, and IL-1B, STAT3, NLRP3 and NOD2 were identified as key genes with clinical predictive value by PPI and receiver operating characteristic (ROC) curve analysis. The candidate gene expression level was significantly correlated with the immune infiltration level, and most of the key genes were positively correlated with the immune cell infiltration level. The key genes are mainly related to cytokine and receptor interactions, immune responses and other pathways. The ceRNA network predicted 8,654 interaction pairs (24 miRNAs and 2,952 lncRNAs).
Conclusion: We used multiple bioinformatics datasets to identify IL-1B, STAT3, NLRP3 and NOD2 as key genes that contribute to the development of ICH.
1 Introduction
Intracerebral hemorrhage (ICH) is a common stroke syndrome, accounting for approximately 15% of strokes, and nearly 50% of stroke-related deaths worldwide are related to ICH (Feigin et al., 2009; Biffi et al., 2016). ICH is caused by the sudden rupture of blood vessels caused by pathological accumulation of blood in the brain parenchyma (Jia et al., 2020). ICH injury is divided into primary and secondary injuries, with the former being caused by direct mechanical action of the hematoma (Fu et al., 2022). Edema around the hematoma occurs within hours of ICH, disrupting the blood–brain barrier and adjacent tissues and leading to secondary damage (Li et al., 2020). Second, mitochondrial dysfunction, neurotransmitter disturbance, microglial activation, and the release of inflammatory mediators are also important mechanisms for aggravating brain injury (Kim-Han et al., 2006). The death of nerve cells after ICH is closely related to the sequelae of ICH and death from ICH. Programmed cell death (PCD) refers to the autonomous and orderly death of cells controlled by genes to maintain the stability of the internal environment. PCD is an active suicidal behavior of cells (Huysmans et al., 2018). PCD, including autophagy, apoptosis and pyroptosis, plays an important role in neuronal cell death after ICH (Bobinger et al., 2018). Autophagy, as an important category of PCD, has been identified in ICH, but its mechanism in intracerebral hemorrhage remains unclear.
Autophagy is one of the important subcellular events occurring from eukaryotic cells to mammals, and the process of autophagy is highly conserved. Autophagy refers to the process in which cells can wrap their intracellular contents under stress and integrate with lysosomes to degrade into these contents into biomacromolecules, which are reused by cells (Ohsumi, 2014). Recent studies have shown that autophagy is closely related to the occurrence of various neurological diseases (Moujalled et al., 2021). In recent years, autophagy has been found to be closely related to secondary brain tissue damage after ICH (Duan et al., 2016; Zhang et al., 2021). After ICH occurs, thrombin is produced in the blood coagulation process, while the hematoma gradually degrades, releasing degradation products such as hemoglobin, heme and iron that invade the surrounding brain tissue. When iron overload and abnormal thrombin expression occur in brain tissue, autophagy is activated and involved in the brain protection process to reduce injury, remove harmful substances and maintain intracellular environmental homeostasis. The protective role of autophagy in ICH has been demonstrated (Wang et al., 2020; Li et al., 2021). However, the overactivation of autophagy, which activates microglia to produce proinflammatory factors and damages neurons, leads to the aggravation of secondary injury after ICH (Shi et al., 2018; Zhang et al., 2021). In summary, autophagy is extremely important for the progression of ICH, but the key genes involved in this process are still not clearly known. The diagnosis of the severity of ICH on the basis of autophagy-related gene expression is also a clinical blind spot. The key autophagy-related genes in ICH need to be identified.
To explore and identify potential biomarkers and the key autophagy-related genes in ICH, we obtained microarray and gene information from multiple databases and used the R statistical programming language for analysis. We selected DEGs in perihematomal tissue (PH) and contralateral normal tissue from intracerebral hemorrhage patients obtained from multiple sources as raw data. Then, four key genes were screened by analyzing the interactions and relationships of DEGs highly related to autophagy with the ROC curve method. Finally, we analyzed the impact of key genes on the immune microenvironment and the mechanisms by which these genes are regulated by transcription factors and non-coding RNAs. We innovatively used methods such as ceRNA network construction, motif-TF annotation and xCell to analyze autophagy after ICH. These results will contribute to the study of the mechanism of secondary injury following ICH and provide new ideas for the diagnosis and treatment of ICH in the clinic.
2 Materials and methods
2.1 Data download
The NCBI GEO Database (http://www.ncbi.nlm.nih.gov/geo/) is a repository of microarray, next-generation sequencing, and other high-throughput sequencing data (Edgar et al., 2002). The GSE24265 Series Matrix File was downloaded from the GEO public database, noted by the GPL570 annotation file, of which the expression profile data belonged to 11 samples, including the perihematomal areas, gray matters, and white matters of 7 patients in the healthy control group and 4 patients with ICH (Rosell et al., 2011). The GSE149317 Series Matrix File (only used to verify the expression level of key genes) was downloaded from the GEO public database, and the annotated File is GPL24688 (Yuan et al., 2020). The microarray data included 6 cases in the healthy control group and 6 patients in the ICH group. We used the R package limma to count the differentially expressed genes between ICH patient and healthy control samples (Ritchie et al., 2015). The screening conditions for differential genes were P.Value < 0.05 and |logFC| > 1. Using the GeneCards database (https://www.genecards.org/) (Stelzer et al., 2016), 7236 autophagy-related genes were obtained. The relevance scores of 269 genes were greater than 3, and these genes were chosen for analysis as an autophagy gene set. Another 1139 ICH-related genes were also obtained from the database. The flow chart of this study is shown in Figure 1.
2.2 Functional annotation
The R package clusterProfiler was used to comprehensively explore the functional correlation of these differentially expressed genes (Yu et al., 2012). GO and KEGG were used for the evaluation of relevant functional categories. GO and KEGG enriched pathways with both p values and q-values less than 0.05 were considered significant pathways. To comprehensively explore the functional correlation of differentially expressed genes, we also used the Metascape database (www.metascape.org) for gene annotation (Zhou et al., 2019). GO and KEGG were used to analyze the potential pathways of the selected genes. Min overlap ≥ 3 and p ≤ 0.01 were considered statistically significant.
2.3 Protein–protein interaction network analysis
The protein–protein interaction (PPI) information of genes was retrieved through the STRING database (Szklarczyk et al., 2021), and the confidence scores were set to ≥0.4. Cytoscape software was used to visualize the results, and the gene coexpression network was obtained. The MCODE algorithm of Cytoscape identified densely connected sets of genes in the PPI network.
2.4 Analysis of immune cell infiltration
Developed by the Dviraran team in 2017, xCell is a widely used method to evaluate immune cell types in the microenvironment (Aran et al., 2017). This method integrates the strengths of gene enrichment analysis via deconvolution to assess 64 cell types that include multiple adaptive and innate immune cells, hematopoietic progenitor cells, epithelial cells, and extracellular stromal cells, including 48 tumor microenvironment-related cells. With the R package xCell, we analyzed the patient data to infer the relative proportion of infiltrating immune cells and performed Pearson correlation analysis on the level of immune cell infiltration. Pearson correlation analysis was used to evaluate the immune cell content and the expression level of some key genes.
2.5 Transcription factor regulatory network analysis of key genes
The transcription initiation process of eukaryotes is very complex and often requires the assistance of various protein factors. TFs and RNA polymerase II form a transcription initiation complex and participate in the process of transcription initiation together. TFs can be divided into two categories according to their function. The first category is universal transcription factors, which, when acting together with RNA polymerase II to form the transcription initiation complex, can start transcription at the correct position. Another category is cis-acting elements, which are sequences present in sequences flanking genes that can affect gene expression. Cis-acting elements include promoters, enhancers, regulatory sequences, and inducible elements that participate in the regulation of gene expression. The cis-acting element itself does not encode any protein but provides an action site to interact with the trans-acting factor. This analysis was mainly performed using the R package cisTarget (https://resources.aertslab.org/cistarget/), in which we used mm9-500bp-upstream-7species.mc9nr.feather version 1.6.0 for the Gene-motif rankings database. The main TFs were predicted by the cisTarget function, when nesThreshold was 3, geneErnMethod was aprox, and geneErnMmaxRank was 5000.
2.6 Gene set enrichment analysis
According to a predefined set of genes, GSEA is a statistical procedure to rank genes according to their degree of differential expression in two types of samples and then test whether the predefined gene set is enriched at the top or bottom of the ranking list (Subramanian et al., 2005). In this study, GSEA was used to compare the discrepancies in signaling pathways between the high expression group and the low expression group and to explore the molecular mechanisms of the core genes of patients. The number of substitutions was 1000, and the substitution type was phenotype.
2.7 Analysis of the ceRNA network
Representing a new mode of gene expression regulation, ceRNA has attracted much attention in the academic community in recent years. Compared with the miRNA regulatory network, the ceRNA network is more elaborate and complex, involving more RNA molecules, including mRNAs, gene-coding pseudogenes, long non-coding RNAs, and miRNAs. In addition, we combined four databases, miRWalk, miRDB, TargetScan and ENCORI, to predict the interaction between key mRNAs and non-coding RNAs. Moreover, we selected coidentified targeted mRNAs for further analysis. Finally, ceRNA networks were established with the combination of mRNA–miRNA and miRNA–lncRNA interactions and visualized with Cytoscape.
3 Results
3.1 Identification of Hub genes
We downloaded the GSE24265 dataset from the NCBI GEO public database, which contained the data from a total of 11 individuals, including 7 in the healthy control group and 4 in the disease group. Through comparison with the healthy control group, we used the limma package to screen out a total of 341 upregulated genes and 144 downregulated genes in the patient samples (Figure 2A). Among them, 11 autophagy-related genes (all upregulated genes) were included (Figure 2B). Ultimately, we used these 11 autophagy-related differentially expressed genes as candidate gene sets for further analysis.
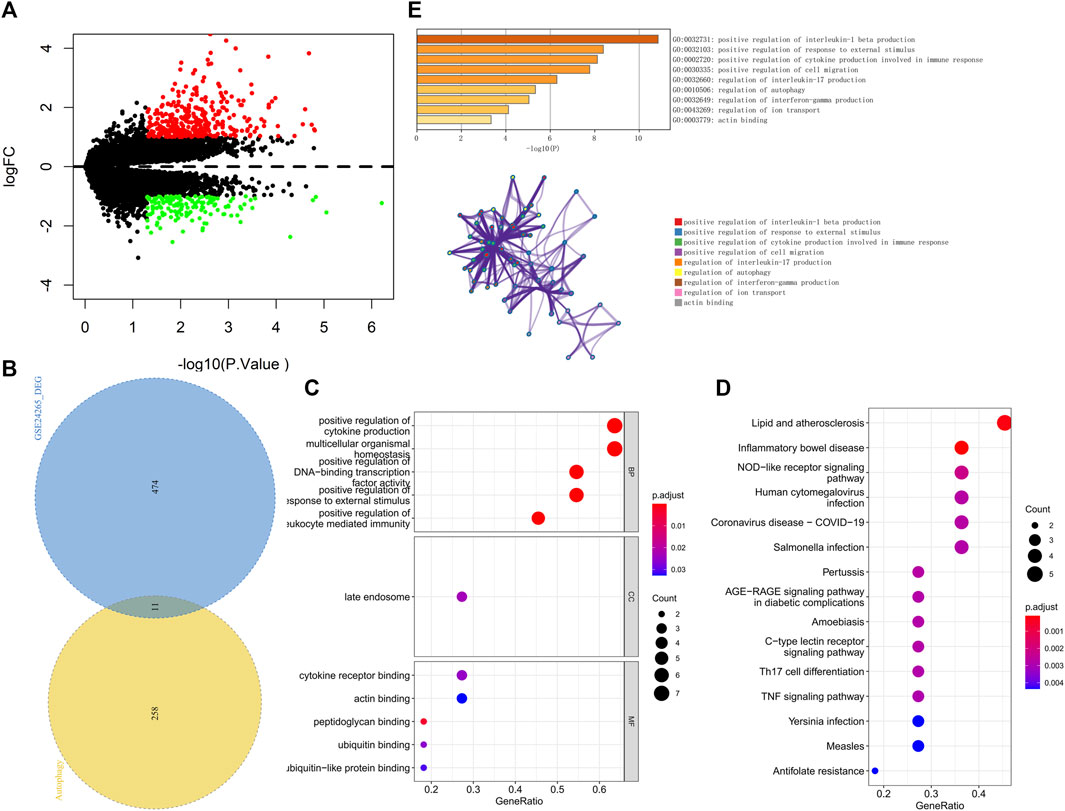
FIGURE 2. (A) The volcano plot of differentially expressed genes in the GSE24265 dataset. Red indicates the downregulation of differentially expressed genes, green indicates the upregulation of differentially expressed genes, and the screening conditions for differentially expressed genes were P.Value < 0.05 and |logFC| > 1. (B) Venn diagram of differentially expressed genes and autophagy-related genes. (C) GO enrichment results of differentially expressed genes, including BP, CC, and MF, sorted according to the number of genes enriched in the pathway. (D) KEGG enrichment results of differentially expressed genes sorted according to the number of genes enriched in the pathway. (E) GO-KEGG enrichment analysis of differential genes based on the Metascape database are shown above. The cluster networks composed of enriched pathways are shown below, where nodes sharing the same cluster are usually close to each other.
3.2 Functional enrichment analysis
We further performed pathway analysis on these 11 candidate genes. GO enrichment analysis showed that these candidate genes were mainly enriched in the positive regulation of cytokine production and cytokine receptor binding pathways (Figure 2C). KEGG enrichment analysis revealed that these candidate genes were mainly enriched in pathways such as lipid and atherosclerosis and the nucleotide-binding oligomerization domain (NOD)−like receptor signaling pathway (Figure 2D). The Metascape database was used for further pathway analysis of candidate genes. The results showed that these candidate genes were mainly enriched in positive regulation of interleukin-1 beta production, the regulation of interleukin-17 production and the regulation of autophagy pathways (Figure 2E).
3.3 Identification of key genes and ROC curve analysis
We found multiple protein interaction pairs among 11 candidate genes through the STRING online database. Moreover, five key genes, including IL1B, STAT3, IL6, NOD2 and NLRP3, were obtained by MCODE analysis in Cytoscape (Figure 3A). Then, we analyzed the expression levels of these five key genes in the GSE149317 dataset and found that the expression levels of interleukin-1beta (IL1B), signal transducer and activator of transcription 3 (STAT3), nucleotide-binding oligomerization domain containing 2 (NOD2) and NOD-1-like receptor pyrin domain containing three (NLRP3) were significantly higher in the ICH group than in the healthy control group (Figure 3B). The area under the receiver operating characteristic curve (AUC) for the four key genes was no less than 0.75 (Supplementary Figure S1). Based on the GSE24265 dataset, we once again analyzed the predictive power of these key genes for ICH. The results showed that the AUCs of IL1B, STAT3, NOD2 and NLRP3 were greater than 0.8 (Supplementary Figure S1).
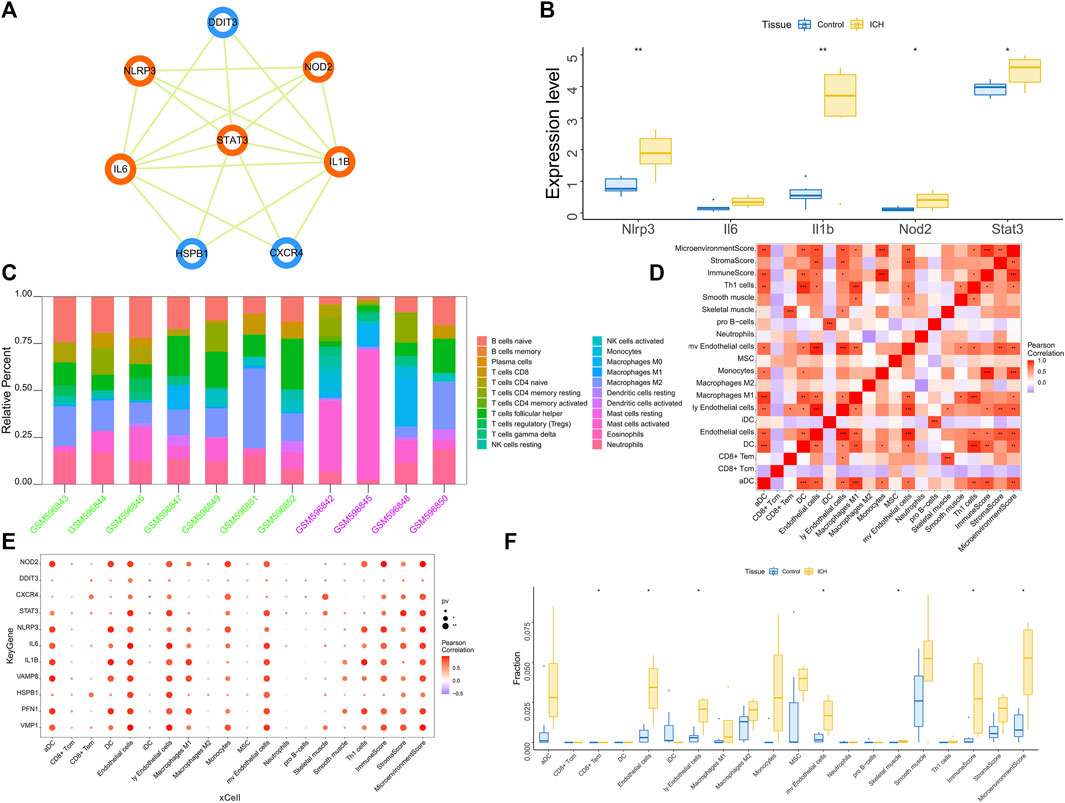
FIGURE 3. (A) Based on the Cytoscape software, the key clusters obtained by the protein interaction network MCODE algorithm, the orange genes are the key genes in the cluster. (B) The GSE149317 dataset validates the 5-based expression, with blue for the control group and yellow for the ICH group. The test method was ANOVA. (C) Relative percentages of 20 immune cell subsets per sample. On the horizontal axis, green is the healthy control group, and purple is the patient group. (D) Pearson correlation between 20 immune cells; purple indicates a negative correlation, and red indicates a positive correlation. The p value of the level of correlation is indicated by an asterisk: * for p < 0.05, ** for p < 0.01, and *** for p < 0.001. (E) Pearson correlation analysis of 11 candidate genes and 20 types of immune cells; purple indicates a negative correlation, and red indicates a positive correlation. (F) The difference in immune cell content between healthy controls and ICH patients (yellow indicates healthy controls, and blue indicates ICH patients); p < 0.05 was considered statistically significant.
3.4 Analyses of the immune microenvironment
The immune microenvironment is mainly composed of immune-related fibroblasts, immune cells, extracellular matrix, various growth factors, inflammatory factors and special physicochemical characteristics. The immune microenvironment significantly affects the diagnosis, survival outcome and clinical severity of disease. Analyzing the relationship between core genes and immune infiltration in the GSE24265 dataset, we further explored the potential molecular mechanisms affecting disease progression. The 20 most significant immune factors in the Wilcoxon test were selected for analysis. The research results showing the proportion of immune cells and the correlation with immunity are shown in Figures 3C, D. There were multiple significant correlation pairs between the expression level of candidate genes and the level of immune infiltration (Figure 3E). In addition, the levels of endothelial cells and Ly endothelial cells in the ICH group were higher than those in the healthy controls (Figure 3F). We further explored the relationship between key genes and immune cells and found that key genes were mostly positively correlated with immune cell infiltration levels. For example, Endothelial cells, MicroenvironmentScore, aDC, ly Endothelial cells and ImmuneScore were significantly positively correlated with 4 key genes, but CD8+ Tcm, iDC and pro B−cells were significantly negatively correlated with key genes (Supplementary Figure S2). We further obtained the correlations between these key genes and different immune factors from the TISIDB database, including immunomodulators, chemokines and cell receptors (Supplementary Figure S3). These data confirmed that these key genes are closely related to immune cell infiltration levels and play important roles in the immune microenvironment.
3.5 The correlation between key genes and ICH-related genes
We obtained 1,139 ICH-related pathogenic genes through the GeneCards database. Based on the GSE24265 dataset, we analyzed the expression levels of the 4 key genes and the top 20 genes in the Relevance score from GeneCards. Statistical analysis by ANOVA showed that the expression levels of these disease-related genes were significantly different between the healthy control group and the disease-related group. In addition, the expression levels of key genes were significantly correlated with the expression levels of multiple disease-related genes (Supplementary Figure S4).
3.6 Transcription factors of key genes
We applied these four key genes to the gene set for this analysis and found that they are regulated by a common mechanism including multiple transcription factors. Therefore, enrichment analysis (Figure 4), motif-TF annotation and the selection of important genes were performed for these transcription factors using accumulative recovery curves. The analysis results showed that the motif with the highest normalized enrichment score (NES: 7.70) was annotated as cisbp__M5082. Three genes were enriched in this motif, namely, IL1B, NLRP3 and NOD2. We displayed all enriched motifs and corresponding transcription factors of core genes (Supplementary File S1)
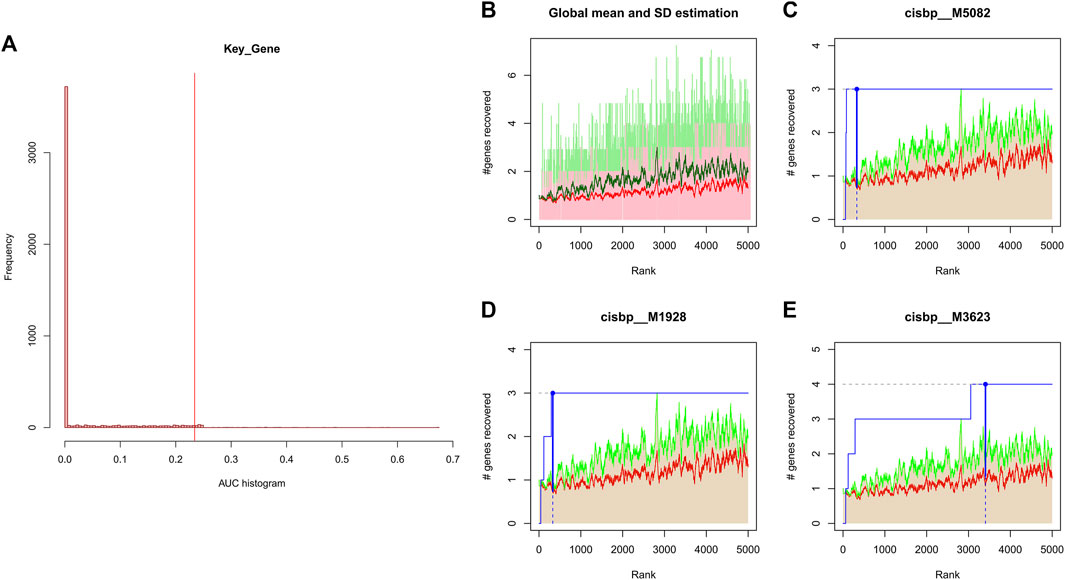
FIGURE 4. (A) Distribution of AUC values for enriched motifs, which were calculated from the recovery curves of key genes for motif ordering. (B–E) In the figure, the red line is the mean value of the recovery curve of each motif, the green line is the mean + standard deviation, and the blue line is the recovery curve of the current motif.
3.7 GSEA of key genes
We investigated the specific signaling pathways enriched by the 4 key genes and explored the underlying molecular mechanisms by which the core genes affect the progression of ICH. Some of these highly significant pathways were selected to be displayed in detail (Figure 5). The IL1B gene GO enrichment pathways were ERYTHROCYTE DEVELOPMENT, INTRACILIARY TRANSPORT INVOLVED IN CILIUM ASSEMBLY, etc. The IL1B gene KEGG enrichment pathways were CYTOKINE-CYTOKINE RECEPTOR INTERACTION, JAK STAT SIGNALING PATHWAY, etc. The NLRP3 gene GO enrichment pathways were COTRANSLATIONAL PROTEIN TARGETING TO MEMBRANE, 2 OXOGLUTARATE METABOLIC PROCESS, etc. The NLRP3 gene KEGG enrichment pathways were CALCIUM SIGNALING PATHWAY, PENTOSE AND GLUCURONATE INTERCONVERSIONS. The NOD2 gene GO enrichment pathways were CELLULAR METABOLIC COMPOUND SALVAGE, HISTONE H4 K16 ACETYLATION, etc. The NOD2 gene KEGG enrichment pathways were ANTIGEN PROCESSING AND PRESENTATION, AUTOIMMUNE THYROID DISEASE, etc. The STAT3 gene GO enrichment pathways were HEPATOCYTE DIFFERENTIATION, MRNA TRANSCRIPTION, etc. The STAT3 gene KEGG enrichment pathways were CALCIUM SIGNALING PATHWAY, PENTOSE AND GLUCURONATE INTERCONVERSIONS, etc.
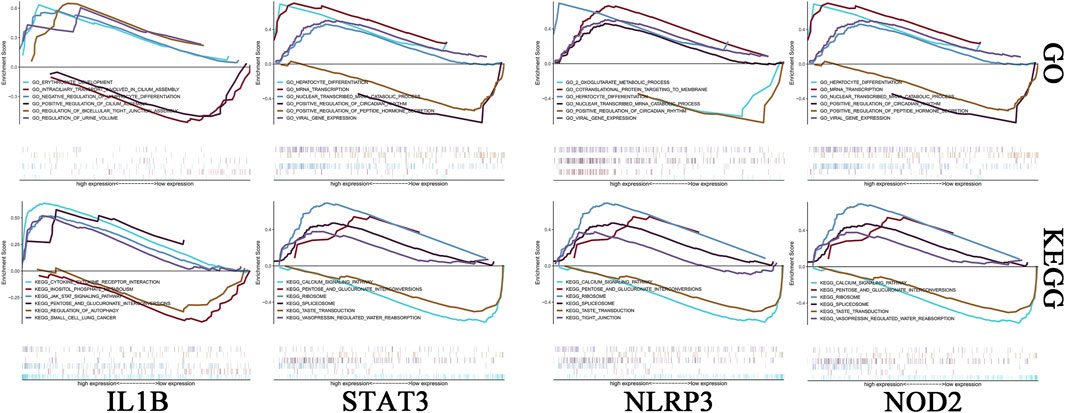
FIGURE 5. Enrichment analysis of key genes. The first row is the GO analysis, and the second row is the KEGG analysis. From left to right are IL-1B, STAT3, NLRP3 and NOD2.
3.8 Further ceRNA interaction and mining
The possible miRNAs and lncRNAs of the 4 key genes were obtained from the miRWalk database and ENCORI database, respectively. First, the four key mRNA-related mRNA–miRNA relationship pairs were extracted from the miRWalk database, but we retained only 67 mRNA–miRNA pairs (4 mRNAs and 66 miRNAs) that were validated in TargetScan or miRDB. Then, interacting lncRNAs were predicted based on these miRNAs, and a total of 8,654 pairs of interactions (24 miRNAs and 2,952 lncRNAs) were predicted. Finally, a ceRNA network was constructed by Cytoscape (V3.7) (Figure 6).
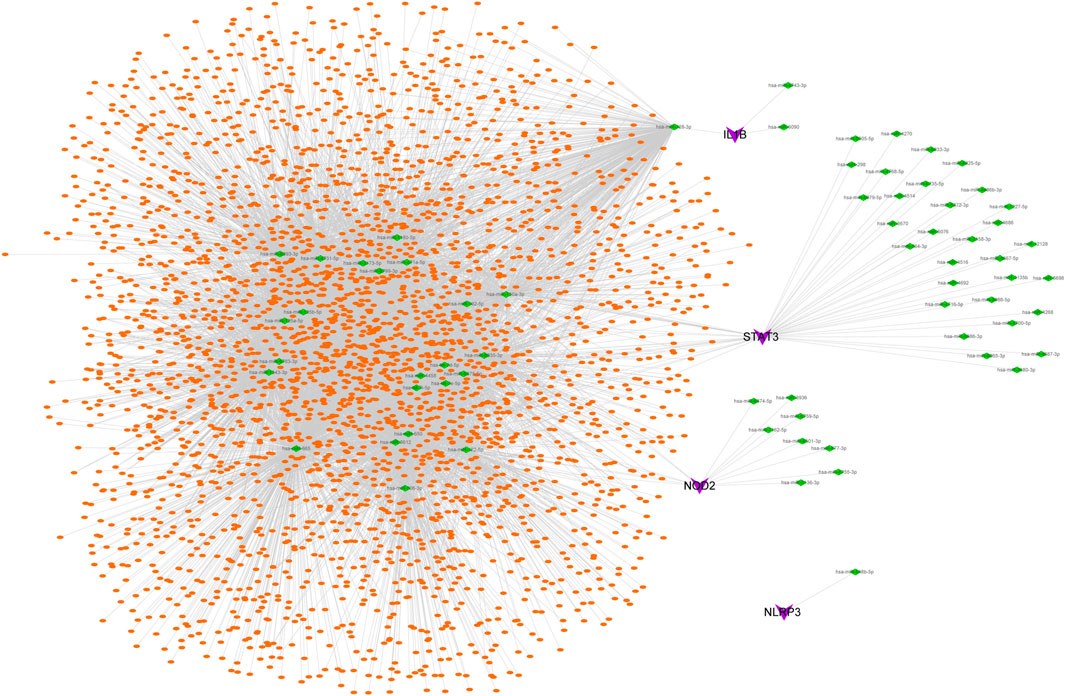
FIGURE 6. CeRNA network of key genes. Purple represents key genes, green represents miRNA, and orange represents lncRNA.
4 Discussion
Defined as a primary, non-traumatic intraparenchymal hemorrhage, ICH can lead to severe disability and is associated with a high fatality rate of 30%–50% within 6 months (Mayer and Rincon, 2005). The mortality rate of ICH within 30 days is 32%–50%, and only 28%–35% of patients who survive 3 months are able to live independently (Martini et al., 2012). As a subtype of stroke, the pathogenesis and treatment of ICH have been extensively studied, and there is still a lack of effective acute treatment. Autophagy, as an important regulatory mechanism of intracellular homeostasis, has been gradually recognized in ICH, but the regulatory effects of autophagy on intracellular homeostasis and the immune microenvironment after ICH remain to be further explored.
Inflammation in secondary injury after ICH is mainly due to the activation of and increase in inflammatory cells and inflammatory factors (Wang, 2010). After ICH, components in the blood, including blood cells, cytokines and various immune cells, quickly cross the blood–brain barrier and enter and accumulate at the center of the injured site. This is followed by the activation of infiltrated immune cells and immune cells of the central nervous system, including the polarization of macrophages and microglia, the activation of leukocytes and astrocytes, and brain tissue damage and repair (Xue and Del Bigio, 2000; Sheth and Rosand, 2014). Immune cells include peripheral blood-derived leukocytes and macrophages, innate microglia, astrocytes, and mast cells. Many studies have shown that leukocytes, macrophages, activated microglia, and astrocytes are the main cellular mediators of secondary injury in ICH (Illanes et al., 2011). These immune cells can release cytokines, chemokines, prostaglandins, proteases, ferrous iron, and other immunologically active molecules (Hua et al., 2006). The R package X cell analysis indicated that four key genes can cause macrophages, neutrophils and CD8+ T cells to infiltrate the lesions during ICH and can also promote the increase in related immune factors and aggravate the inflammatory response.
Although the pathogenesis of ICH has been extensively studied at the transcriptional level, there are some limitations of these studies. Most of the related research at the RNA level is on only the regulatory relationship between a single type of RNA, such as lncRNAs, miRNAs or mRNAs, and ICH, but little is known about the interaction of different RNAs in the development of ICH. The discovery of ceRNAs in recent years has solved this problem. ceRNA refers to RNA that has a miRNA binding site and can compete with mRNA to bind miRNA, thereby inhibiting the regulatory effect of miRNA on target genes (Ma et al., 2020). The ceRNA regulatory network rigorously integrates the mutual regulatory relationship between mRNA and non-coding RNA (ncRNA), providing significant help for the study of posttranscriptional mechanisms of diseases (Qi et al., 2015). Numerous studies have shown that the ceRNA regulatory network plays an important role in secondary injury following ICH (Liu et al., 2021; Wang et al., 2021; Yang et al., 2022). Based on multiple databases, the key gene-related ceRNA network described in this study shows miRNAs and related lncRNAs that play major regulatory roles.
This study identified IL-1B, STAT3, NOD2 and NLRP3 as key causative genes for secondary injury in ICH and demonstrated the critical role of autophagy in ICH. We combined two datasets, mainly using GO/KEGG analysis, immune infiltration analysis and ceRNA network construction, to screen key autophagy-related genes and analyze their mechanisms affecting ICH progression.
Recent studies have shown that cytokines, including proinflammatory cytokines and anti-inflammatory cytokines, play an important regulatory role in the course of various inflammatory-related diseases. IL-1B, a member of the interleukin 1 cytokine family, is a key proinflammatory factor that plays an important role in the body’s immune response and regulates inflammatory responses to brain injury (You et al., 2020). After inflammation occurs in the body, the secretion of IL-1B increases rapidly. In general, IL-1B has a proinflammatory effect in the local inflammatory response, causing vascular dilation and inducing the transfer of monocytes and neutrophils to the inflammatory site, resulting in a stress response and tissue damage (Schett et al., 2016). Our GSEA suggested that IL-1B was involved in the process of cytokine binding to its corresponding receptor, which also suggested that IL-1B plays an important role in the inflammatory response to ICH. In addition, KEGG analysis of IL-1B also showed enrichment of the Janus kinase-signal transducer and activator of transcription (JAK-STAT) pathway. Previously, researchers found that miRNAs/mRNAs changes in whole-blood samples for patients with ICH were important links with the JAK-STAT pathway (Cheng et al., 2020). The JAK-STAT pathway has also been associated with ICH progression in rat models (Ji et al., 2020). Our GSEA results also showed that STAT3, which is closely related to mRNA catabolism, is a key gene leading to ICH. The STAT protein family, which includes seven members, plays a key role in regulating cytokine-dependent inflammation and immunity. STAT3 is considered to be the most conserved and can be activated by various factors and stimuli, such as cytokines and chemokines. STAT3 is closely related to ischemic stroke and ischemia–reperfusion injury, and its high expression aggravates nerve damage (Zhu et al., 2021). Zhu H reported that STAT3 activation can promote the occurrence and development of inflammation, leading to increased cerebral edema after ICH and damage to neurons around the hematoma, and NLRP3 is a downstream molecule of STAT (Lee et al., 2006). In addition, the findings from mouse experiments suggest that NLRP3 is the key to the aggravation of ICH injury caused by STAT3 (Ji et al., 2022). Our results showed that NLRP3 was significantly upregulated in the brain tissues of ICH patients, and the AUC of NLRP3 was greater than 0.89, which indicates that NLRP3 is a key gene for ICH and has strong predictive value for ICH. NLRP3, a member of the intracytoplasmic pattern recognition receptor NOD-like receptors (NLRs), is an important part of the innate immune system and plays an important regulatory role in the process of innate immune inflammation. NLRP3 can sense tissue cell damage and is then activated by a variety of damage-associated molecular patterns (DAMPs) or pathogen-associated molecular patterns (PAMPs) (Mangan et al., 2018). Activated NLRP3 protein can form the NLRP3 inflammasome, which can cleave biologically inactive pro-IL-1B into IL-1B and exert its proinflammatory effect (Mangan et al., 2018). The last key gene identified in our analysis, NOD2, is also one of the main NLRs. As an important intracytoplasmic pattern recognition receptor, NOD2 is widely involved in the recognition of immune cells and the induction of inflammatory responses (Huang et al., 2013). Activated NOD2 receptors recruit the downstream signaling molecule receptor interacting protein 2 (RIP2), which can activate the non-canonical transcription factor nuclear factor-kappaB (NF-κB) and then transcribe NF-κB-dependent target genes, secreting inflammatory factors such as tumor necrosis factor-A (TNF-A) and IL-1B. Although many NOD2 studies have focused on inflammatory bowel disease, it has been shown that NOD2 is involved in the inflammatory response after cerebral ischemia, triggering an excessive inflammatory response and exacerbating brain injury (Kuban et al., 2017). This study is the first to suggest that NOD2 may be a key gene in the development of ICH. Our GSEA results suggest a high correlation of NOD2 with ANTIGEN PROCESSING AND PRESENTATION.
5 Conclusion
In this study, the existing ICH patient data in the GEO database were analyzed by combining autophagy-related genes in the GENE database, and 11 potential pathogenic genes were finally obtained. Finally, with diagnostic and predictive value, IL-1B, STAT3, NLRP3 and NOD2 were obtained through PPI analysis and ROC curve analysis. Then, based on the database and R package, we found that these 4 key genes cause immune cell infiltration into ICH lesions. GSEA revealed the specific signaling pathways involved in key genes, and we explored the possibility that these pathways might influence the development of ICH. The demonstration of TFs and ceRNA networks affecting key genes provides a theoretical basis for TFs and ncRNA in the regulation of the expression of these key genes. The identification of four key genes contributes to the understanding of the mechanism of ICH and provides potential targets and directions for the clinical treatment of ICH.
Data availability statement
The datasets presented in this study can be found in online repositories. The names of the repository/repositories and accession number(s) can be found in the article/Supplementary Material.
Author contributions
YX: Conceptualization, Methodology, Investigation, Software, Formal analysis. CW: Methodology, Investigation, Software, Formal analysis. YZ: Methodology, Writing—Original Draft. YG: Validation, Formal analysis. JG: Writing—Review & Editing, Resources. TH: Visualization, Project administration, Funding acquisition.
Funding
The present study was supported by the National Natural Science Foundation of China (grant nos. 82001170 and 82172190).
Conflict of interest
The authors declare that the research was conducted in the absence of any commercial or financial relationships that could be construed as a potential conflict of interest.
Publisher’s note
All claims expressed in this article are solely those of the authors and do not necessarily represent those of their affiliated organizations, or those of the publisher, the editors and the reviewers. Any product that may be evaluated in this article, or claim that may be made by its manufacturer, is not guaranteed or endorsed by the publisher.
Supplementary material
The Supplementary Material for this article can be found online at: https://www.frontiersin.org/articles/10.3389/fgene.2023.1032639/full#supplementary-material
SUPPLEMENTARY FIGURE S1 | The ROC curves of key genes suggest that the genes have good predictive performance for ICH. The first row is the GSE149317 dataset, and the second row is the GSE24265 dataset.
SUPPLEMENTARY FIGURE S2 | (A-D) Pearson correlation between key genes and immune cells. IL-1B, STAT3, NLRP3 and NOD2 are listed in alphabetical order. (E) Pearson correlation analysis of 4 key genes and 20 kinds of immune cells; purple indicates a negative correlation, and red indicates a positive correlation.
SUPPLEMENTARY FIGURE S3 | (A-D) Pearson correlations of key genes and various immune factors; purple indicates a negative correlation, and red indicates a positive correlation. Chemokine-related genes, receptor-related genes, immunoinhibitor-related genes, MHC-related genes and immunostimulator-related genes are listed in alphabetical order. The p value of the level of correlation is indicated by an asterisk: * for p < 0.05, ** for p < 0.01, and *** for p < 0.001.
SUPPLEMENTARY FIGURE S4 | (A) Differences in the expression of ICH disease-regulating genes; blue indicates healthy controls, and yellow indicates ICH patients. (B) The middle panel shows the Pearson correlation analysis of ICH disease-regulating genes and key genes. Blue indicates a negative correlation, and red indicates a positive correlation.
SUPPLEMENTARY FILE S1 | All the enriched motifs and corresponding transcription factors of core genes are displayed in the document.
References
Aran, D., Hu, Z., and Butte, A. J. (2017). xCell: digitally portraying the tissue cellular heterogeneity landscape. Genome Biol. 18, 220. doi:10.1186/s13059-017-1349-1
Biffi, A., Bailey, D., Anderson, C. D., Ayres, A. M., Gurol, E. M., Greenberg, S. M., et al. (2016). Risk factors associated with early vs delayed dementia after intracerebral hemorrhage. JAMA Neurol. 73, 969–976. doi:10.1001/jamaneurol.2016.0955
Bobinger, T., Burkardt, P., B Huttner, H., and Manaenko, A. (2018). Programmed cell death after intracerebral hemorrhage. Curr. Neuropharmacol. 16, 1267–1281. doi:10.2174/1570159X15666170602112851
Cheng, X., Ander, B. P., Jickling, G. C., Zhan, X., Hull, H., Sharp, F. R., et al. (2020). MicroRNA and their target mRNAs change expression in whole blood of patients after intracerebral hemorrhage. J. Cereb. Blood flow metab. Off. J. Int. Soc. Cereb. Blood Flow Metab. 40, 775–786. doi:10.1177/0271678X19839501
Duan, X., Wen, Z., Shen, H., Shen, M., and Chen, G. (2016). Intracerebral hemorrhage, oxidative stress, and antioxidant therapy. Oxid. Med. Cell. Longev. 2016, 1203285. doi:10.1155/2016/1203285
Edgar, R., Domrachev, M., and Lash, A. E. (2002). Gene expression Omnibus: NCBI gene expression and hybridization array data repository. Nucleic acids Res. 30, 207–210. doi:10.1093/nar/30.1.207
Feigin, V. L., Lawes, C. M., Bennett, D. A., Barker-Collo, S. L., and Parag, V. (2009). Worldwide stroke incidence and early case fatality reported in 56 population-based studies: A systematic review. Lancet Neurol. 8, 355–369. doi:10.1016/S1474-4422(09)70025-0
Fu, K., Xu, W., Lenahan, C., Mo, Y., Wen, J., Deng, T., et al. (2022). Autophagy regulates inflammation in intracerebral hemorrhage: Enemy or friend? Front. Cell Neurosci. 16, 1036313. doi:10.3389/fncel.2022.1036313
Hua, Y., Wu, J., Keep, R. F., Nakamura, T., Hoff, J. T., and Xi, G. (2006). Tumor necrosis factor-alpha increases in the brain after intracerebral hemorrhage and thrombin stimulation. Neurosurgery 58, 542–550; discussion 542-550. doi:10.1227/01.NEU.0000197333.55473.AD
Huang, J., Liu, B., Yang, C., Chen, H., Eunice, D., and Yuan, Z. (2013). Acute hyperglycemia worsens ischemic stroke-induced brain damage via high mobility group box-1 in rats. Brain Res. 1535, 148–155. doi:10.1016/j.brainres.2013.08.057
Huysmans, M., Buono, R. A., Skorzinski, N., Radio, M. C., De Winter, F., Parizot, B., et al. (2018). NAC transcription factors ANAC087 and ANAC046 control distinct aspects of programmed cell death in the arabidopsis columella and lateral root cap. Plant Cell 30, 2197–2213. doi:10.1105/tpc.18.00293
Illanes, S., Liesz, A., Sun, L., Dalpke, A., Zorn, M., and Veltkamp, R. (2011). Hematoma size as major modulator of the cellular immune system after experimental intracerebral hemorrhage. Neurosci. Lett. 490, 170–174. doi:10.1016/j.neulet.2010.11.065
Ji, X. C., Shi, Y. J., Zhang, Y., Chang, M. Z., and Zhao, G. (2020). Reducing suppressors of cytokine signaling-3 (SOCS3) expression promotes M2 macrophage polarization and functional recovery after intracerebral hemorrhage. Front. Neurol. 11, 586905. doi:10.3389/fneur.2020.586905
Ji, N., Wu, L., Shi, H., Li, Q., Yu, A., and Yang, Z. (2022). VSIG4 attenuates NLRP3 and ameliorates neuroinflammation via JAK2-STAT3-A20 pathway after intracerebral hemorrhage in mice. Neurotox. Res. 40, 78–88. doi:10.1007/s12640-021-00456-5
Jia, J., Wang, Z., Zhang, M., Huang, C., Song, Y., Xu, F., et al. (2020). SQR mediates therapeutic effects of H(2)S by targeting mitochondrial electron transport to induce mitochondrial uncoupling. Sci. Adv. 6, eaaz5752. doi:10.1126/sciadv.aaz5752
Kim-Han, J. S., Kopp, S. J., Dugan, L. L., and Diringer, M. N. (2006). Perihematomal mitochondrial dysfunction after intracerebral hemorrhage. Stroke 37, 2457–2462. doi:10.1161/01.STR.0000240674.99945.4e
Kuban, K. C., Joseph, R. M., O'Shea, T. M., Heeren, T., Fichorova, R. N., Douglass, L., et al. (2017). Circulating inflammatory-associated proteins in the first month of life and cognitive impairment at age 10 Years in children born extremely preterm. J. Pediatr. 180, 116–123.e1. doi:10.1016/j.jpeds.2016.09.054
Lee, S. T., Chu, K., Sinn, D. I., Jung, K. H., Kim, E. H., Kim, S. J., et al. (2006). Erythropoietin reduces perihematomal inflammation and cell death with eNOS and STAT3 activations in experimental intracerebral hemorrhage. J. Neurochem. 96, 1728–1739. doi:10.1111/j.1471-4159.2006.03697.x
Li, Z., Li, M., Shi, S. X., Yao, N., Cheng, X., Guo, A., et al. (2020). Brain transforms natural killer cells that exacerbate brain edema after intracerebral hemorrhage. J. Exp. Med. 217, e20200213. doi:10.1084/jem.20200213
Li, J., Wu, X., He, Y., Wu, S., Guo, E., Feng, Y., et al. (2021). PINK1 antagonize intracerebral hemorrhage by promoting mitochondrial autophagy. Ann. Clin. Transl. neurology 8, 1951–1960. doi:10.1002/acn3.51425
Liu, Z., Wu, X., Yu, Z., and Tang, X. (2021). Reconstruction of circRNA-miRNA-mRNA associated ceRNA networks reveal functional circRNAs in intracerebral hemorrhage. Sci. Rep. 11, 11584. doi:10.1038/s41598-021-91059-9
Ma, N., Tie, C., Yu, B., Zhang, W., and Wan, J. (2020). Identifying lncRNA-miRNA-mRNA networks to investigate Alzheimer's disease pathogenesis and therapy strategy. Aging 12, 2897–2920. doi:10.18632/aging.102785
Mangan, M. S. J., Olhava, E. J., Roush, W. R., Seidel, H. M., Glick, G. D., and Latz, E. (2018). Targeting the NLRP3 inflammasome in inflammatory diseases. Nat. Rev. Drug Discov. 17, 588–606. doi:10.1038/nrd.2018.97
Martini, S. R., Flaherty, M. L., Brown, W. M., Haverbusch, M., Comeau, M. E., Sauerbeck, L. R., et al. (2012). Risk factors for intracerebral hemorrhage differ according to hemorrhage location. Neurology 79, 2275–2282. doi:10.1212/WNL.0b013e318276896f
Mayer, S. A., and Rincon, F. (2005). Treatment of intracerebral haemorrhage. Lancet Neurol. 4, 662–672. doi:10.1016/S1474-4422(05)70195-2
Moujalled, D., Strasser, A., and Liddell, J. R. (2021). Molecular mechanisms of cell death in neurological diseases. Cell Death Differ. 28, 2029–2044. doi:10.1038/s41418-021-00814-y
Ohsumi, Y. (2014). Historical landmarks of autophagy research. Cell Res. 24, 9–23. doi:10.1038/cr.2013.169
Qi, X., Zhang, D. H., Wu, N., Xiao, J. H., Wang, X., and Ma, W. (2015). ceRNA in cancer: possible functions and clinical implications. J. Med. Genet. 52, 710–718. doi:10.1136/jmedgenet-2015-103334
Ritchie, M. E., Phipson, B., Wu, D., Hu, Y., Law, C. W., Shi, W., et al. (2015). Limma powers differential expression analyses for RNA-sequencing and microarray studies. Nucleic Acids Res. 43, e47. doi:10.1093/nar/gkv007
Rosell, A., Vilalta, A., García-Berrocoso, T., Fernández-Cadenas, I., Domingues-Montanari, S., Cuadrado, E., et al. (2011). Brain perihematoma genomic profile following spontaneous human intracerebral hemorrhage. PloS one 6, e16750. doi:10.1371/journal.pone.0016750
Schett, G., Dayer, J. M., and Manger, B. (2016). Interleukin-1 function and role in rheumatic disease. Nat. Rev. Rheumatol. 12, 14–24. doi:10.1038/nrrheum.2016.166
Sheth, K. N., and Rosand, J. (2014). Targeting the immune system in intracerebral hemorrhage. JAMA neurol. 71, 1083–1084. doi:10.1001/jamaneurol.2014.1653
Shi, H., Wang, J., Wang, J., Huang, Z., and Yang, Z. (2018). IL-17A induces autophagy and promotes microglial neuroinflammation through ATG5 and ATG7 in intracerebral hemorrhage. J. Neuroimmunol. 323, 143–151. doi:10.1016/j.jneuroim.2017.07.015
Stelzer, G., Rosen, N., Plaschkes, I., Zimmerman, S., Twik, M., Fishilevich, S., et al. (2016). The GeneCards suite: From gene data mining to disease genome sequence analyses. Curr. Protoc. Bioinforma. 54, 1. doi:10.1002/cpbi.5
Subramanian, A., Tamayo, P., Mootha, V. K., Mukherjee, S., Ebert, B. L., Gillette, M. A., et al. (2005). Gene set enrichment analysis: A knowledge-based approach for interpreting genome-wide expression profiles. Proc. Natl. Acad. Sci. U. S. A. 102, 15545–15550. doi:10.1073/pnas.0506580102
Szklarczyk, D., Gable, A. L., Nastou, K. C., Lyon, D., Kirsch, R., Pyysalo, S., et al. (2021). The STRING database in 2021: Customizable protein-protein networks, and functional characterization of user-uploaded gene/measurement sets. Nucleic acids Res. 49, D605–D612. doi:10.1093/nar/gkaa1074
Wang, L., Tian, M., and Hao, Y. (2020). Role of p75 neurotrophin receptor in neuronal autophagy in intracerebral hemorrhage in rats through the mTOR signaling pathway. Cell cycle (Georget. Tex) 19, 376–389. doi:10.1080/15384101.2019.1711318
Wang, H., Cao, X., Wen, X., Li, D., Ouyang, Y., Bao, B., et al. (2021). Transforming growth factor-β1 functions as a competitive endogenous RNA that ameliorates intracranial hemorrhage injury by sponging microRNA-93-5p. Mol. Med. Rep. 24, 499. doi:10.3892/mmr.2021.12138
Wang, J. (2010). Preclinical and clinical research on inflammation after intracerebral hemorrhage. Prog. Neurobiol. 92, 463–477. doi:10.1016/j.pneurobio.2010.08.001
Xue, M., and Del Bigio, M. R. (2000). Intracerebral injection of autologous whole blood in rats: Time course of inflammation and cell death. Neurosci. Lett. 283, 230–232. doi:10.1016/s0304-3940(00)00971-x
Yang, C., Wu, J., Lu, X., Xiong, S., and Xu, X. (2022). Identification of novel biomarkers for intracerebral hemorrhage via long noncoding RNA-associated competing endogenous RNA network. Mol. omics 18, 71–82. doi:10.1039/d1mo00298h
You, Y., Borgmann, K., Edara, V. V., Stacy, S., Ghorpade, A., and Ikezu, T. (2020). Activated human astrocyte-derived extracellular vesicles modulate neuronal uptake, differentiation and firing. J. Extracell. Vesicles 9, 1706801. doi:10.1080/20013078.2019.1706801
Yu, G., Wang, L. G., Han, Y., and He, Q. Y. (2012). clusterProfiler: an R package for comparing biological themes among gene clusters. Omics a J. Integr. Biol. 16, 284–287. doi:10.1089/omi.2011.0118
Yuan, J. J., Chen, Q., Xiong, X. Y., Zhang, Q., Xie, Q., Huang, J. C., et al. (2020). Quantitative profiling of oxylipins in acute experimental intracerebral hemorrhage. Front. Neurosci. 14, 777. doi:10.3389/fnins.2020.00777
Zhang, C., Qian, C., Yang, G., Bao, Y. X., and Qian, Z. M. (2021). Hepcidin inhibits autophagy in intracerebral hemorrhage models in vitro and in vivo. Mol. Cell. Neurosci. 111, 103589. doi:10.1016/j.mcn.2021.103589
Zhou, Y., Zhou, B., Pache, L., Chang, M., Khodabakhshi, A. H., Tanaseichuk, O., et al. (2019). Metascape provides a biologist-oriented resource for the analysis of systems-level datasets. Nat. Commun. 10, 1523. doi:10.1038/s41467-019-09234-6
Keywords: intracerebral hemorrhage, autophagy, immune infiltration, bioinformatics analysis, ceRNA network
Citation: Xiao Y, Zhang Y, Wang C, Ge Y, Gao J and Huang T (2023) The use of multiple datasets to identify autophagy-related molecular mechanisms in intracerebral hemorrhage. Front. Genet. 14:1032639. doi: 10.3389/fgene.2023.1032639
Received: 31 August 2022; Accepted: 23 March 2023;
Published: 03 April 2023.
Edited by:
Thayne Kowalski, Federal University of Rio Grande do Sul, BrazilReviewed by:
Mi Zhang, Huazhong University of Science and Technology, ChinaXiang Cao, Nanjing Drum Tower Hospital, China
Copyright © 2023 Xiao, Zhang, Wang, Ge, Gao and Huang. This is an open-access article distributed under the terms of the Creative Commons Attribution License (CC BY). The use, distribution or reproduction in other forums is permitted, provided the original author(s) and the copyright owner(s) are credited and that the original publication in this journal is cited, in accordance with accepted academic practice. No use, distribution or reproduction is permitted which does not comply with these terms.
*Correspondence: Ju Gao, Z2FvanVfMDAzQDE2My5jb20=; Tianfeng Huang, MTgwNTEwNjM0MDBAeXp1LmVkdS5jbg==