- International Institute of Tropical Agriculture (IITA), Ibadan, Nigeria
The parasitic weed, Striga is a major biological constraint to cereal production in sub-Saharan Africa (SSA) and threatens food and nutrition security. Two hundred and twenty-three (223) F2:3 mapping population involving individuals derived from TZdEI 352 x TZEI 916 were phenotyped for four Striga-adaptive traits and genotyped using the Diversity Arrays Technology (DArT) to determine the genomic regions responsible for Striga resistance in maize. After removing distorted SNP markers, a genetic linkage map was constructed using 1,918 DArTseq markers which covered 2092.1 cM. Using the inclusive composite interval mapping method in IciMapping, twenty-three QTLs influencing Striga resistance traits were identified across four Striga-infested environments with five stable QTLs (qGY4, qSC2.1, qSC2.2, qSC5, and qSC6) detected in more than one environment. The variations explained by the QTLs ranged from 4.1% (qSD2.3) to 14.4% (qSC7.1). Six QTLs each with significant additive × environment interactions were also identified for grain yield and Striga damage. Gene annotation revealed candidate genes underlying the QTLs, including the gene models GRMZM2G077002 and GRMZM2G404973 which encode the GATA transcription factors, GRMZM2G178998 and GRMZM2G134073 encoding the NAC transcription factors, GRMZM2G053868 and GRMZM2G157068 which encode the nitrate transporter protein and GRMZM2G371033 encoding the SBP-transcription factor. These candidate genes play crucial roles in plant growth and developmental processes and defense functions. This study provides further insights into the genetic mechanisms of resistance to Striga parasitism in maize. The QTL detected in more than one environment would be useful for further fine-mapping and marker-assisted selection for the development of Striga resistant and high-yielding maize cultivars.
Introduction
Maize is one of the world’s most important food and feed crops and plays an important role in ensuring food security. Striga hermonthica (purple witchweed) is an obligate hemiparasitic plant that parasitizes the root systems of cereals, causing significant reduction in maize yield in the savannas of sub-Saharan Africa (SSA). Losses in maize yield due to Striga infestation could reach as high as 100%, particularly when infestation is severe during the vegetative growth stage (Akaogu et al., 2019). The severity of infestation is influenced by the type of maize cultivar, weather conditions, and the severity of infestation. The parasitic weed penetrates the roots of maize plants with its haustoria cells to derive nutrients, thus depriving host plants of resources necessary for growth (Parker and Riches, 1993). The increasing Striga problems cause an annual loss of US $7–10 billion per annum to the livelihoods of African farmers, and the elevated levels in vast areas further worsen food insecurity, hunger, and poverty for millions of subsistence farmers (Rodenburg et al., 2005; Jamil et al., 2022). Effective control of S. hermonthica is essential for food security and poverty alleviation for small-holder subsistence farmers in SSA. Commonly used control methods include chemical, cultural and biological measures, which have achieved little or no success. Under prevailing field conditions in SSA, especially in the savannas, the use of Striga-resistant maize varieties has been found to be the most effective, economical, and eco-friendly approach for the control and prevention of yield losses due to Striga parasitism (Akaogu et al., 2019). Also, host plant resistance could be deployed as a vital component of an integrated control strategy for mitigating the menace of the parasitic weed. In Striga research, a maize plant is characterized as resistant when the parasite is unable to penetrate through the endodermis of the host plant, thus disallowing xylem-xylem connections for continuity after attachment of the plant to the host (Atera et al., 2015), whereas tolerance refers to the extent to which the Striga damage effects on the maize plants are mitigated (Rodenburg et al., 2017; Badu-Apraku et al., 2020b).
Quantitative trait loci (QTL) mapping has become an important tool for dissecting the genetic architecture of polygenic traits, facilitating the identification of genomic regions underlying traits of interest, the distribution of gene effects and the relative importance of additive, dominance, and epistatic effects (Vengadessan et al., 2013). With the development of sequencing technology, high-density SNPs are now used in constructing genetic maps for increasing precision (Zhang et al., 2022). Several QTL studies have been conducted to understand the molecular genetics of complex traits under both biotic and abiotic stresses in maize, including drought (Tuberosa et al., 2002; Almeida et al., 2013), low soil nitrogen (Ribeiro et al., 2018), maize grey leaf spot disease (Berger et al., 2014), Fusarium ear rot (Maschietto et al., 2017), maize streak virus (Ladejobi et al., 2018), and Striga parasitism (Amusan et al., 2008; Badu-Apraku et al., 2020a; Badu-Apraku et al., 2020b). However, information is limited on the molecular genetics of Striga resistance. The use of marker-assisted selection (MAS) could be a very effective strategy in breeding for Striga resistance/tolerance (Badu-Apraku and Fakorede, 2017). Molecular markers associated with Striga resistance alleles would be invaluable because plant breeders could use such markers during selection to identify the resistance loci in existing populations or to pyramid resistance into new populations. However, the success of MAS depends on the identification of the accurate locations of the QTL and the identification of tightly linked molecular markers.
Wild relatives of maize (teosintes and Tripsacum dactyloides) have been used to develop genetically improved maize with resistance to Striga species, which are particularly prevalent in Africa (Rich and Ejeta, 2008). Novel resistance genes identified in the wild perennial maize relative, teosinte (Zea diploperennis) by International Institute of Tropical Agriculture (IITA) maize breeders (Kling et al., 2000) have been introgressed into early and extra-early maturing maize inbred lines (Amegbor et al., 2017). For example, the early-maturing Striga resistant and drought-tolerant maize inbred line, TZdEI 352 derived from a biparental cross involving the normal endosperm white maize population TZEW Pop DT STR and the Z. diploperennis has displayed increased grain yield and durable Striga resistance/tolerance (Akaogu et al., 2019). Results of genetic studies conducted by Akaogu et al. (2019) revealed that the additive-dominance model was adequate in describing observed variations in the number of emerged Striga plants in a population derived from a cross between TZdEI 352 and the susceptible inbred TZEEI 11. Hence, the digenic epistatic model was adopted for the inheritance of resistance to Striga damage (Akaogu et al., 2019). In contrast, dominance effects were higher than the additive effects for the number of emerged Striga plants across locations signifying that non-additive gene action conditioned the inheritance of Striga resistance. It was proposed that the inbred TZdEI 352 could serve as an invaluable parent for hybrid development in Striga endemic agro-ecologies of SSA. Furthermore, TZdEI 352 has significant and positive general combining ability (GCA) effects for grain yield in Striga, drought and low N environments, significant negative GCA effects for the stay-green characteristic under drought and low N, as well as negative and significant GCA effects for Striga damage and number of emerged Striga plants under Striga-infested conditions. Inbred TZdEI 352 is presently serving as an invaluable multiple stress tolerant parent for hybrid development in Striga endemic agro-ecologies of SSA. This inbred line was therefore an ideal genotype for QTL discovery for Striga resistance. Application of molecular markers provides a powerful tool for mapping quantitative traits loci and improving complex traits through marker-assisted selection (MAS). QTLs/markers have been identified for Striga resistance traits by maize breeders at the IITA and the International Maize and Wheat Improvement Centre (CIMMYT). However, most of the QTLs/markers have minor effects, though linked to genes associated with plant defense mechanisms under Striga infestation (Badu-Apraku et al., 2020a; Adewale et al., 2020; Badu-Apraku et al., 2020b; Gowda et al., 2021; Stanley et al., 2021). There is, therefore, the need to identify more Striga resistance QTLs particularly using germplasm extracted from wild maize sources (Zea diploperennis) to ensure successful resistance breeding through gene pyramiding. The objectives of this study were to (i) map QTLs for Striga resistance using a maize population derived from a cross involving the Striga resistant early maturing inbred, TZdEI 352, and the Striga susceptible early maturing line, TZEI 916, (ii) identify candidate genes associated with the Striga resistance QTLs.
Materials and methods
Plant materials
The contrasting inbred lines used in the present study were selected based on preliminary evaluation of the lines (Adewale et al., 2020) and genetic studies involving these inbred lines under Striga-infested environments at Mokwa, Nigeria in 2017 and 2018. The two early maturing white maize inbred lines varied significantly in their responses under artificial Striga infestation. The parental inbred line TZdEI 352 (TZE-W POP STR 107 S6 24/254-1/2-1/1-1/1-2/2-1/1) is Striga resistant, while line TZE1 916 ((TZE COMP 5-W DT C7 x TZEI 31) S6 inb 39-1/1-1/2-2/2-2/2-1/1) is highly susceptible to Striga parasitism. The inbred TZEI 916 has negative and significant GCA effect for grain yield, positive and significant GCA effect for Striga damage as well as positive GCA effect for number of emerged Striga counts under Striga infestation. The Striga resistant parent TZdEI 352 also possesses drought and low soil N tolerance alleles and it is one of the parental lines involved in the development of the outstanding multiple stress-tolerant hybrid (HAKIMI 3) commercialized in Nigeria. It is also involved in several other hybrids and open-pollinated varieties in the pipeline for release in several countries in West and Central Africa. Crosses were made between TZdEI 352 and TZEI 916 to obtain 223 F2:3 families at the IITA-Ibadan breeding nursery during the 2018 and 2019 growing seasons. The inbred TZdEI 352 was crossed to TZEI 916 to obtain F1 progenies. The F1 progenies derived from the bi-parental cross involving TZdEI 352 and TZEI 916 were screened using SSR molecular markers to identify true-to-type hybrids for the development of the F2 mapping population. Thirty-four F1 progenies identified to be true-to-type were advanced to the F2 stage through selfing. The harvested F2 seeds were planted for sample collection for genotyping and advancement to the F2:3 stage for field phenotyping. The F2:3 mapping population was used to identify QTL/genomic regions responsible for resistance/tolerance to Striga parasitism.
Field experiment and trait evaluation
A segregating population consisting of 223 F2:3 families and the two parental lines (TZdEI 352 and TZEI 916) were evaluated at two Striga endemic locations: Mokwa (9018′N, 504′E, 457 m altitude) and Abuja (90 16′N, 70 20′E, 300 m altitude) in the Southern Guinea savanna zones of Nigeria. Field trials were conducted during the 2019 and 2020 growing seasons at each location. The experimental design used in the different environments was 15 x 15 lattice design. The experimental field at Mokwa has a luvisol soil type, while that at Abuja has a ferric luvisol soil type (Soil Survey Staff, 1999). The trials were replicated twice for evaluations in each of the four environments. Each experimental unit consisted of a 1-row plot 3 m long, with row spacing of .75 m and intra-row spacing of .4 m. The fields for artificial Striga infestation at Mokwa and Abuja were injected with ethylene gas about 2 weeks before planting to induce suicidal germination of Striga seeds in the soil. The artificial Striga infestation was carried out as proposed by the IITA Maize Program (Kim, 1991). About a week before inoculation, the Striga seeds were thoroughly mixed with finely sieved sand at the ratio of 1:99 by weight to ensure rapid and uniform infestation. A standard scoop calibrated to deliver about 5,000 germinable Striga seeds per hole was used for the artificial infestation. Three maize seeds were placed in the same hole with the Striga seeds and thinned to two plants per hill at 2 weeks after emergence to obtain the target population density of 66,666 plants per ha. Fertilizer application on the maize plots was delayed till about 30 days after planting to subject the maize plants to stress, a condition that was expected to favour the production of strigolactones, which enhances good germination of Striga seeds and attachment of Striga plants to the roots of host plants. At this stage of plant growth, 30 kg N/ha, and 30 kg each of P and K were applied as NPK 15-15-15. The reduced rate of fertilizer application was necessary because Striga emergence decreases at high N rate (Kim, 1991). Data were recorded on the number of emerged Striga plants and host plant damage severity at 10 weeks after planting (Kim, 1991; Badu-Apraku et al., 2020b). The number of ears per plant (EPP) was computed by dividing the total number of ears harvested per plot by the number of plants in a plot at harvest. Grain yield (kg ha− 1) was estimated from field weight of ears harvested per plot, assuming a shelling percentage of 80% (that is, 800 g grain/kg ear weight), adjusted to moisture content of 15%. These four traits measured (grain yield, host plant damage, number of emerged Striga plants and ears per plant) are the primary traits of interest in selecting for resistance to Striga parasitism in maize (Menkir and Kling, 2007; Adewale et al., 2020).
Statistical analysis of phenotypic data
Statistical analysis of the phenotypic data was performed using the SAS software version 9.3 and R software version 4.0.5. Analysis of variance (ANOVA) was first carried out for each environment. Thereafter, combined ANOVA across environments (locations) was conducted with PROC GLM in SAS using a random statement with the TEST option (SAS Institute, 2011). The replications and blocks within replications were considered as random and the F2:3 families as fixed effects. Correlation analysis among the traits was carried out using package “corrplot” in the R software. The broad sense heritability (H2) was estimated using the formula:
DNA extraction, genotyping and data filtering process
Young, healthy leaf tissues were obtained from the F2:3 individuals and bulk samples from the parental lines. The samples were freeze-dried and used for genomic DNA extraction following the DArT protocol. The extracted DNA was tested by electrophoresis in 1% agarose gel and analyzed on the ND-1000 spectrophotometer platform (NanoDrop, Wilmington, DE, United States) for concentration and purity. The mapping population was genotyped using 15,048 DArTseq markers for the QTL identification. Low-quality data filtering was carried out by eliminating markers with minor allele frequency of less than 5%, missing data greater than 10%, and those that were monomorphic for the parents. Segregation distortion was determined using Chi-square (χ2) test for goodness-of-fit and an expected ratio of 1:2:1 for the F2:3 mapping population. Markers significantly deviating (p < .05) from Mendelian segregation were eliminated. After quality filtering, a total of 1,918 quality SNP markers were used for the QTL analysis.
Construction of genetic linkage map, QTL analysis and candidate gene annotation
Recombination fractions and LOD scores were performed using the R qtl package (http://www.rqtl.org) and the order of the markers were clustered into bin. The correct order of the markers across each chromosome was verified through the pairwise marker linkage analysis using the “est.rf” function. Genetic linkage maps were then constructed using R/QTL 2. The genetic distances were estimated using the “est.map” function with “kosambi” distance from the R/qtl package (Broman et al., 2003; Arends et al., 2010). Quantitative trait loci analysis was performed using the inclusive composite interval mapping (ICIM-ADD) method in IciMapping V4.2 (Meng et al., 2015). The ICIM approach utilizes a strategy in which a stepwise regression is firstly performed, so markers with significant effect on QTL are selected. ICIM-ADD method was used with the multi-environmental model built in QTL IciMapping (Li et al., 2015; Singhal et al., 2021). The parameters of QTL analysis were set as follows: logarithm of odds (LOD) = 1,000 permutations, step = 1 cM, and PIN = .001. The confidence interval of each QTL was determined by LOD > 3. The software also estimated the proportion of phenotypic variation, additive and dominance effects explained by each QTL for a trait. QTL × environment interaction (QEI) mapping for multi-environmental trials (MET) was carried out using ICIM-ADD (Meng et al., 2015; Wang et al., 2022). Sources of favourable alleles were determined depending on signs of the QTL additive effects and the traits of interest. For instance, positive additive effects for grain yield and ears per plant and negative additive effects for Striga damage and Striga emergence counts indicated that the favourable alleles were derived from the resistant parent and vice versa. The QTLs detected in more than one environment were regarded as stable (Shang et al., 2015). QTL were classified as major when the phenotypic variance was more than 10% and minor when less than 10% (Collard et al., 2005). QTL detected under different environments for the same trait were regarded as the same QTL when the distances of their peaks were less than 10 cM (Ma et al., 2018; Zhang et al., 2020). QTL naming conventions was followed as described by McCouch et al. (1997) and Yang et al. (2020). The name of each QTL was defined starting with a lowercase ‘q’, then the trait name in uppercase, thereafter by the chromosome number where the QTL was detected. For instance, qSD2 refers to a QTL identified for Striga damage (SD) on chromosome 2. Candidate genes within the main additive QTLs confidence intervals and their corresponding molecular functions were retrieved using the B73 reference genome (Woodhouse et al., 2021). The candidate genes were mined from the sequences of the identified QTL related to the plant defense functions.
Results
Phenotypic evaluation
The analysis of variance showed significant variation among the 223 F2:3 families and their parents for grain yield and all other Striga adaptive traits across artificial Striga-infested environments (Table 1). Significant effects of the environment and genotype × environment interaction were observed for measured traits except genotype × environment interaction for Striga emergence counts. Similarly, the parents and the F2:3 individuals displayed high level of variability for the Striga resistance adaptive traits in each environment (Table 2; Figure 1). All the traits followed normal distribution and both absolute values of skewness and kurtosis were less than 1.0. Transgressive segregation was observed in both directions of normal distribution for the studied traits. Among the F2:3 individuals, grain yield varied from 317 kg/ha to 5,380 kg/ha, with the smallest mean of 1,646, largest mean of 3,388 and largest standard deviation of 1245.39. Ears per plant differed from .01 to .95 with the smallest mean of .74, largest mean of 1.17 and largest standard deviation of .30, Striga damage varied from 1 to 9 with the smallest mean of 3.6, largest mean of 5.5 and largest standard deviation of 1.17, while Striga count differed from 0 to 4.96 with the smallest mean of 1.25, largest mean of 3.35 and largest standard deviation of .90. Broad-sense heritability of the traits ranged from .33 for ears per plant to .60 for grain yield. Results of correlation analysis among the Striga adaptive traits across the four research environments revealed strong negative association of grain yield with Striga damage rating and Striga emergence counts but significant positive relationship with ears per plant (Figure 2). Ears per plant displayed negative association with Striga damage rating and Striga emergence counts. Striga damage rating also revealed positive association with Striga emergence counts.
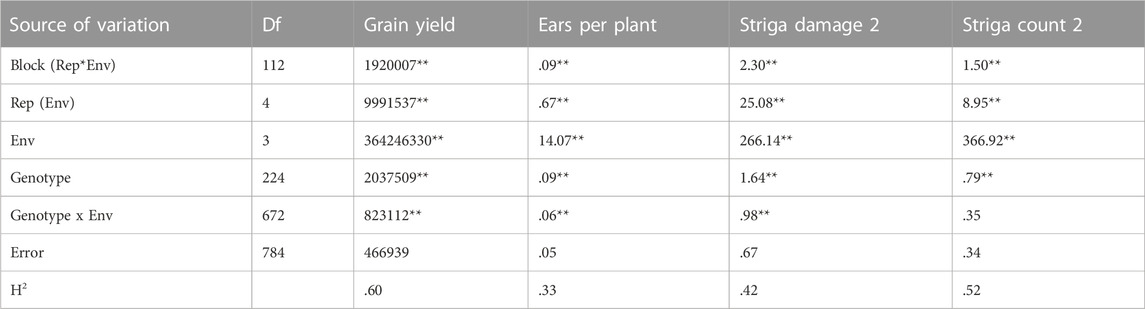
TABLE 1. Mean squares of F2:3 mapping population evaluated under artificial Striga infestation across four environments at Abuja and Mokwa in the 2019 and 2020 growing seasons.
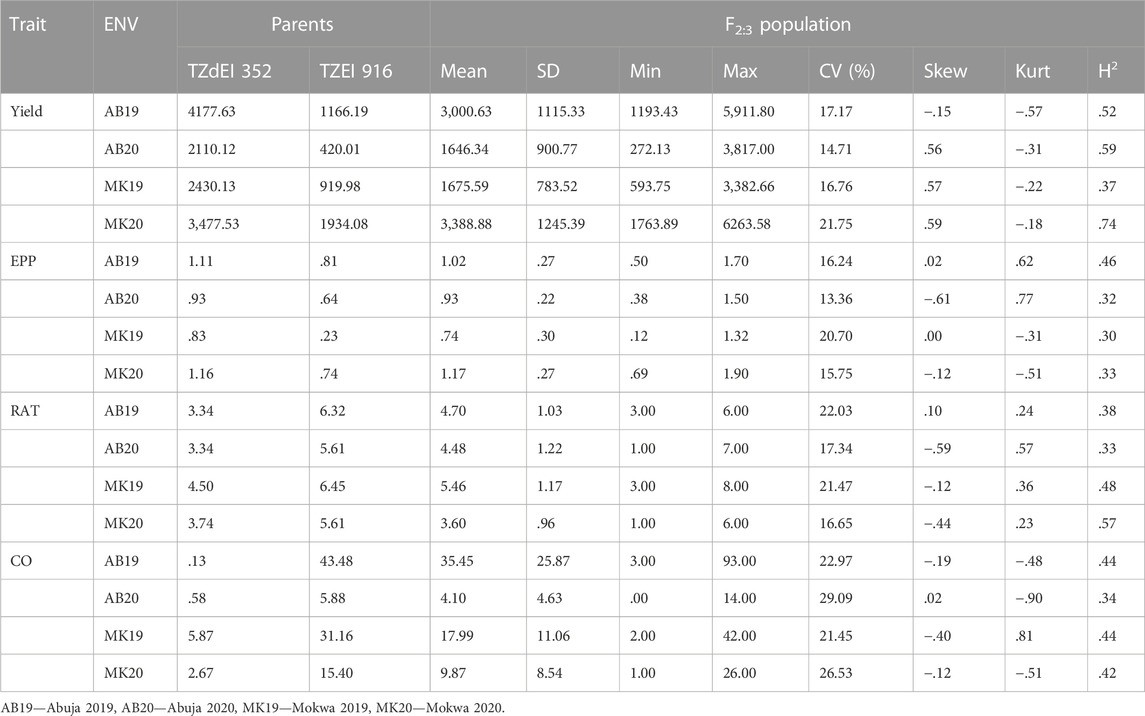
TABLE 2. Summary statistics of the parental lines and F2:3 individuals under artificial Striga infestation.
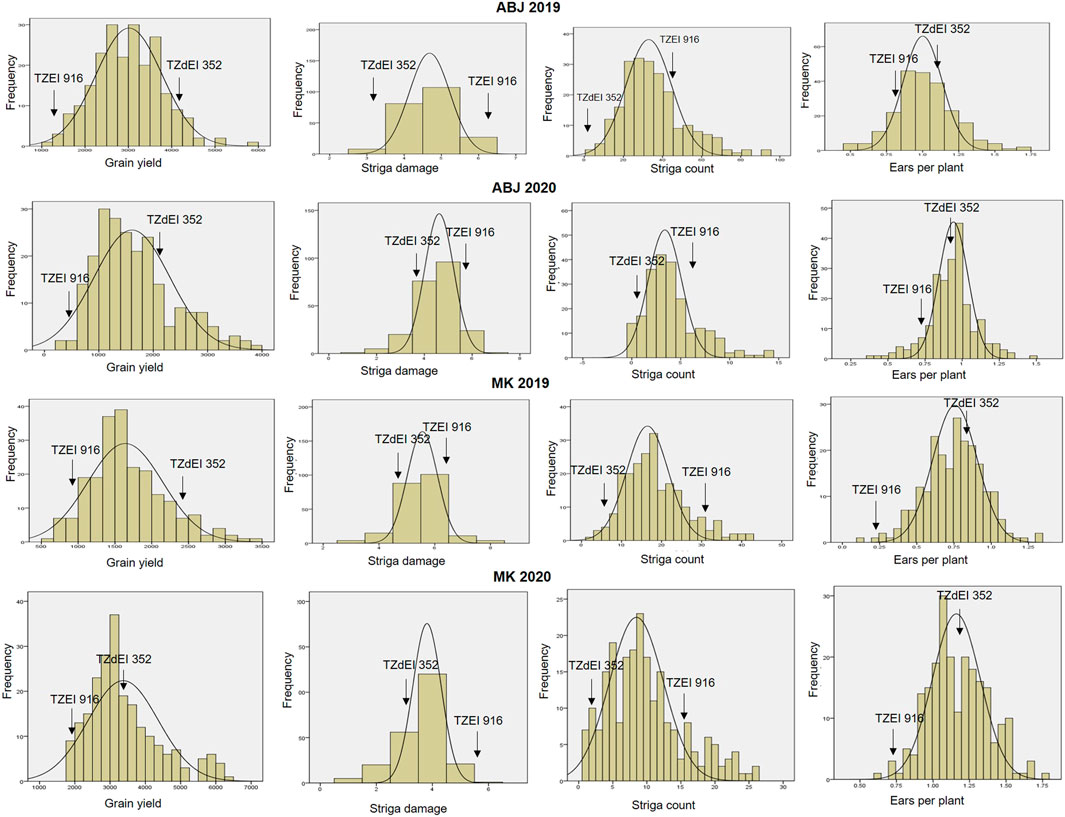
FIGURE 1. Frequency distribution of grain yield, number of ears per plant, Striga damage and number of emerged Striga plants among the F2:3 mapping population individuals, 2019–2020. ABJ—Abuja, MK—Mokwa.
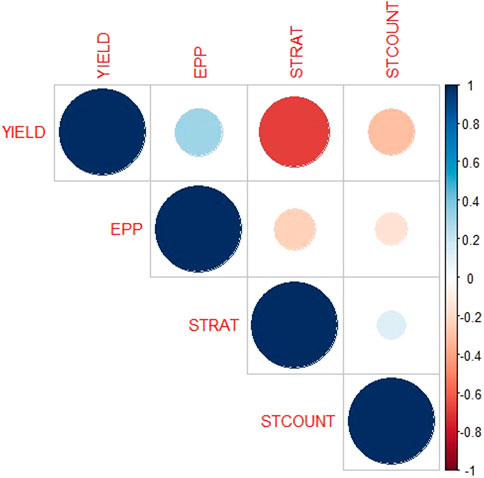
FIGURE 2. Relationship among Striga adaptive traits in the F2:3 mapping population across four Striga-infested environments. YIELD—Grain yield, EPP—number of ears per plant, STRAT—Striga damage rating, STCOUNT—Striga emergence counts. Gradient bars represent the correlation coefficients.
Linkage map
The genetic map of the F2:3 population contained 1,918 polymorphic markers distributed across the 10 maize chromosomes with a total coverage length of 2092.1 cM. The length of each chromosome varied from 150 cM for chromosome 10–307 cM for chromosome 1 (Table 3; Figure 3). The highest number of markers (306) was recorded on chromosome 1, whereas chromosome 5 recorded the least number of markers (117). The average interval size was 23.3 cM. The mean linkage group length was 209.21 cM with a mean of 191.9 cM loci. The genetic linkage map of the F2:3 mapping population displaying stable QTLs as well as QTLs similar to those reported in previous studies are presented in Supplementary Figure S1. The order of markers on the genetic map conforms to the physical position of the marker and is also supported by pairwise marker linkage analysis (Supplementary Figure S2).
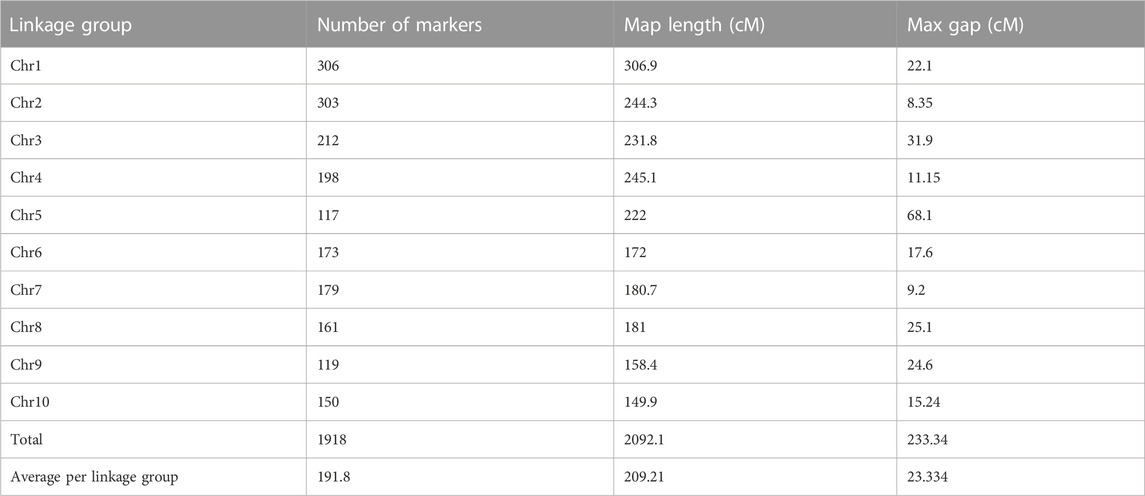
TABLE 3. DArT markers linkage map of F2:3 mapping population derived from the cross TZdEI 352 x TZEI 916.
QTL identification for Striga resistance adaptive traits
QTL mapping was carried out using the inclusive composite interval mapping method to identify QTLs for Striga resistance traits and phenotypic BLUE values across environments were used to reduce the variation effects of the environments. A total of 23 QTLs were detected for the Striga adaptive traits in the F2:3 population across four environments (that is, Abuja in 2019 and 2020; Mokwa in 2019 and 2020) (Table 4). Five stable QTLs including one for grain yield (qGY4), and four for Striga emergence counts (qSC2.1, qSC2.2, qSC5 and qSC6), were detected in more than one environment. For grain yield, four QTLs qGY2, qGY4, qGY5 and qGY8 were detected on chromosomes 2, 4, 5, and 8, respectively. The LOD scores of these QTLs ranged from 4.0 to 6.2, and the proportion of phenotypic variance explained (PVE) ranged from 8.2% to 11.6%. The highest PVE of 11.6% was recorded by qGY5. The QTLs qGY2 and qGY8 displayed negative additive effects, whereas qGY4 and qGY5 displayed positive additive effects for grain yield. Most of the QTLs identified across environments were also found in individual environments. For instance, the QTL qGY2 was also detected in MK19, qGY4 both in AB19 and MK20, qGY5 in MK20 and qGY8 in AB20. Eight QTLs qSD2.1, qSD2.2, qSD2.3, qSD3.1, qSD3.2, qSD5, qSD8 and qSD10 were identified for Striga damage on chromosomes 2, 3, 5, 8 and 10 across the four environments. These QTLs individually explained 4.1%–10.2% phenotypic variation, with LOD scores ranging from 2.6 to 3.9. The highest PVE of 10.2% was recorded by qSD5. Of the eight QTLs identified for Striga damage across environments, only qSD2.2, qSD3.1, qSD3.2, and qSD5 displayed negative additive effects. The QTL qSD2.2 was also found in individual environment AB20; qSD2.3 and qSD3.1 were also identified in AB19. Nine QTLs qSC2.1, qSC2.2, qSC3, qSC5, qSC6, qSC7.1, qSC7.2, qSC8 and qSC9 were identified for number of emerged Striga counts on chromosomes 2, 3, 5, 6, 7, 8, and 9 across environments. The LOD scores of the identified QTLs varied from 3.0 to 5.4, with PVE ranging from 4.6% to 14.4%. The QTL qSC7.1 recorded the highest PVE of 14.4%. Of the nine QTLs, only qSC2.2, qSC3, qSC5, and qSC7.2 displayed negative additive effects. The QTLs qSC2.1, qSC2.2, qSC5, qSC7.1, qSC8 and qSC9 were also identified in AB19, qSC2.1, qSC2.2 and qSC3 were identified in MK19, and qSC2.1 in MK20. Two QTLs qEP1 and qEP6 were identified on chromosomes 1 and 6 for number of ears per plant. LOD score of 2.7 and PVE of 7.0% were obtained for qEP1, while qEP7 recorded LOD score of 3.9 and PVE of 10.3%. Pleiotropic effects were observed for qGY5 and qSD5 located on the same position on chromosome 5 as well as qSD3 and qSC3 found within the same confidence interval on chromosome 3. Significant G × E interaction effects were identified for all studied traits except Striga emergence counts. Using the MET functionality of the Ici-QTL mapping software, six MET-QTLs each were identified for grain yield and Striga damage as well as one for ears per plant (Table 5). Three MET-QTLs (eqGY-2, eqGY-5 and eqGY-8) detected for grain yield were located close to the main additive effects QTLs (qGY-2, qGY-5 and qGY-8). Similarly, the MET-QTLs (eqSD2.1) is closely located to the main additive effects QTL (qSD2.1) for Striga damage. These MET-QTLs displayed the same signs of additive effects as the main effects QTLs.
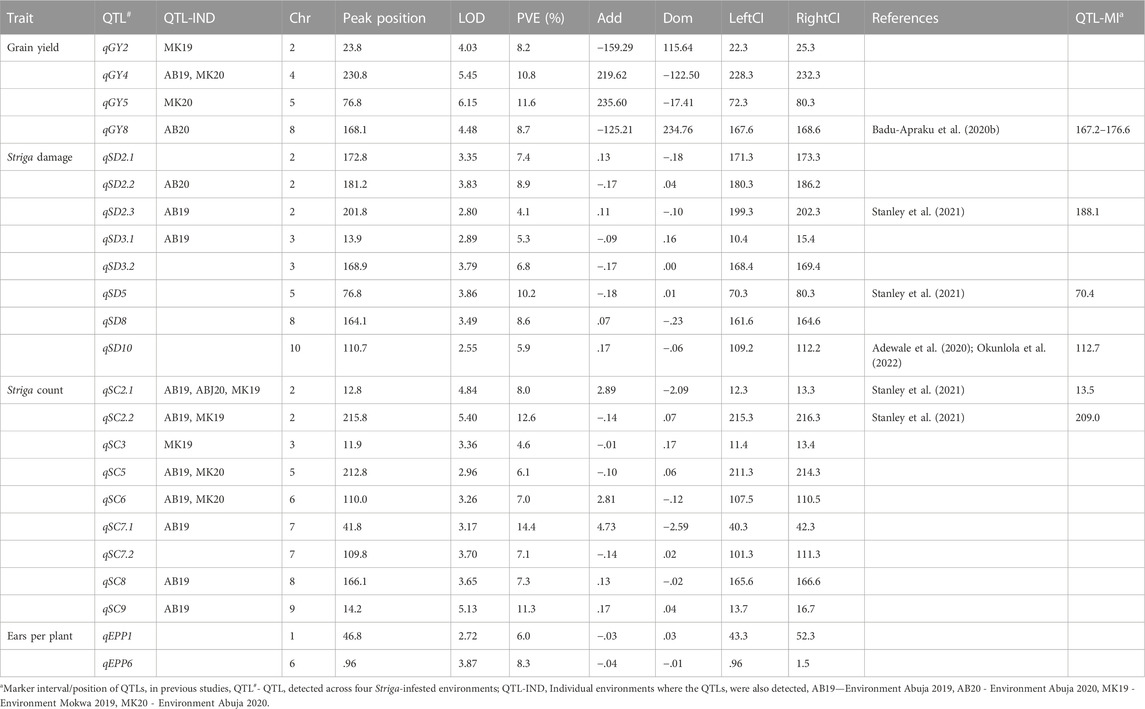
TABLE 4. Quantitative trait loci identified from F2:3 mapping population derived from TZdEI 352 x TZEI 916 across four artificial Striga infested environments in Nigeria, 2019-2020.
Candidate gene prediction
Based on the 23 main additive effect QTLs detected for four Striga adaptive traits using the QTL mapping, a total of 279 protein coding genes were identified within the confidence interval of each of the identified QTLs (Supplementary Table S3), according to the maize gene annotation database accessible at Maize GDB (https://www.maizegdb.org). Of these, 36 candidate genes were functionally annotated to be associated with the Striga resistance QTLs detected (Table 6). Inside the putative QTL qGY2 is enclosed the gene GRMZM2G475678, ereb61 (locus–2:21824408-24239657) which encodes the ethylene-responsive transcription factor 61. Other genes enclosed in this QTL include GRMZM5G805505, GRMZM2G077002, GRMZM2G178998, GRMZM2G055180, GRMZM2G123387, GRMZM2G404973, and GRMZM2G054795 which encode AP2-EREBP-transcription factor 87, C2C2-GATA-transcription factor 5, NAC-transcription factor 131, EREBP-transcription factor 198, WRKY-transcription factor 101, C2C2-GATA-transcription factor 11 and C2C2-YABBY-transcription factor 1 respectively. On chromosome 4, one putative QTL qGY4 (locus - 4:228266384-232427685) contained three genes GRMZM2G085751, GRMZM2G164359 and GRMZM2G052102 which encodes the bHLH-transcription factor 24, C3H-transcription factor 343 - putative RING zinc finger domain superfamily protein and bZIP-transcription factor 120 - basic leucine zipper 19, respectively. Similarly, the QTL qGY8 (locus - 8:167208138-168572930) located .47 Mb upstream of the QTL peak possesses the gene models GRMZM2G030762, GRMZM2G065971 which encodes the bHLH-transcription factor 55 and magnesium transporter 8. Inside the pleiotropic QTLs qGY 5 and qSD 5 (locus 5:68355981-77117931) located on chromosome 5, are five genes cdpk22, nrt3, myb161, bhlh129 and bzip14. These genes encode calcium-dependent kinase, nitrate transporter, MYB, bHLH as well as the bZIP-transcription factors, respectively. The QTLs qSD3.1 and qSC3 (locus 3:11798990-14131442) as well as qSD2 (locus 2:201773609-203194405) were associated with the MYB transcription factors. On chromosome 10, QTL qSD10 located 2.2 Mb downstream of the QTL peak contains the genes GRMZM2G425798, GRMZM2G313756 and GRMZM2G023708 encoding the AP2-EREBP-transcription factor 149, bhlh100 - bHLH-transcription factor 100 and AP2-EREBP-transcription factor 125. The QTL qSC2 (locus 2:12757631–13953770) which encodes the genes GRMZM2G024898, GRMZM2G038722, GRMZM2G014534 and GRMZM2G055204 were associated with WRKY-transcription factor 70, MYB20 transcription factor 13, MYB-related-transcription factor 36 and AP2-EREBP-transcription factor 18, respectively. Similarly, QTLs qSC2 (locus 2:215446898-2159609600), qSC7 (locus 7:39974564-43809836) and qEPP1 (locus 1:43333475–46957478) which houses the genes GRMZM2G383841, GRMZM2G075715 and GRMZM2G021095 encodes the bHLH-transcription factor 147, ARF-transcription factor 37 as well as LBD-transcription factor 4, respectively. On chromosome 5, qSC5 (locus 5:211699547–213923453) contains genes GRMZM2G130459 and GRMZM2G036092 encoding the AP2-EREBP-transcription factor 187 and bHLH-transcription factor 30. The QTL qSC8 (locus 8:165139590–166861798), around .76 Mb upstream region of the QTL peak contains the genes GRMZM2G134073 and GRMZM2G371033 encodes the NAC and SBP transcription factors, respectively. On chromosome 9, QTL qSC9 (locus 9 9:13152043–14927006) contains genes GRMZM2G156006 and GRMZM2G402156 which encode the AP2-EREBP-transcription factor 207 and MYB-related-transcription factor 19, respectively.
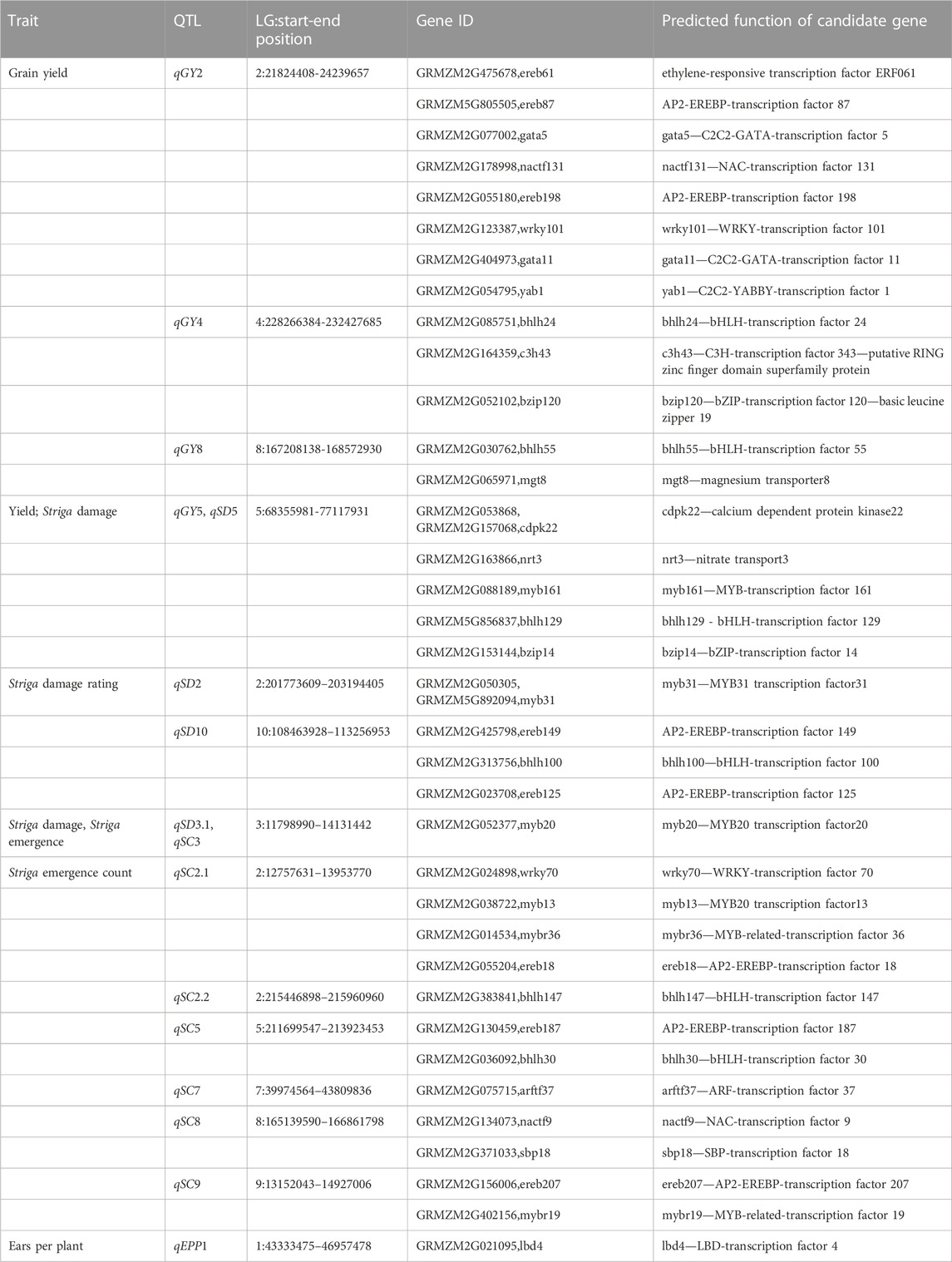
TABLE 6. Key candidate genes associated with QTLs detected from the F2:3 mapping population evaluated under artificial Striga infestation.
Discussion
The introgression of novel genes for Striga resistance from wild relative of maize, Zea diploperennis L. into other genetic backgrounds using molecular markers has great potential for the development of high-yielding and Striga resistant maize genotypes. The significant differences observed among individuals of the F2:3 mapping population for measured traits revealed high level of variability and the suitability for QTL mapping analysis. Frequency distribution and box plot analysis revealed that the studied traits were normally distributed in the F2:3 mapping population. Skewness and kurtosis for the four measured traits were less than 1.0 indicating normal distribution of traits and the suitability of the data for QTL analysis. Some of the F2:3 individuals displayed phenotypes which were beyond the parental limits indicating the possible role of transgressive segregation and additive genes from the two parental lines (Mondal and Badigannavar, 2019). Transgressive segregation was observed in both directions of normal distribution for the studied traits. The presence of transgressive segregation suggested genetic recombination, implying that both favourable and unfavourable alleles for the traits were distributed between the parents. Transgressive segregation observed for measured traits also suggested the possible existence of multiple QTLs and QTL × QTL interaction or epistatic interaction (Chattopadhyay et al., 2019).
In bi-parental mapping, the detection of robust QTLs for traits of interest requires significant differences between the two parents. The Striga resistant parent TZdEI 352 and the Striga susceptible parent TZEI 916 used in our study varied significantly in terms of grain yield, Striga damage, Striga emergence count and number of ears per plant. The wide range of variation in the present population revealed the possibility for selection of lines with resistance to Striga and increased grain yield in maize. The genetic map of the F2:3 population contained 1,918 polymorphic markers distributed across the 10 maize chromosomes with a total coverage length of 2092.1 cM. The overall coverage length reported in the present study are similar to those reported by Badu-Apraku et al. (2020a) and Badu-Apraku et al. (2020b), who found total coverage lengths of 2016 cM and 2076 cM, respectively, in studies focusing on the identification of QTLs for Striga resistance in two extra-early maturing maize mapping populations. Similarly, Yang et al. (2020) reported total genome coverage length of 2,315 cM across the 10 maize chromosomes using 119 polymorphic simple sequence repeats pairs.
The results of QTL mapping rely on the type of population, nature of traits, number of samples, marker density and QTL mapping techniques used. Good understanding of these factors is important in designing QTL mapping experiments and determining optimal procedures for data analysis especially when dealing with highly polygenic traits such as Striga resistance (Su et al., 2017). In the present study, we identified a total of 23 QTLs for grain yield, number of ears per plant, number of emerged Striga plants and Striga damage ratings in a population involving 223 F2:3 families derived from the maize biparental cross TZdEI 352 x TZEI 916 across four artificial Striga-infested environments. The QTLs qGY5 and qSD5 showed co-localization of pleiotropic effects. These QTLs accounted for phenotypic variations ranging from 4.1% (qSD2.3) to 14.4% (qSC7.1), indicating the complex nature of the Striga resistance traits studied. Five (qGY4, qSC2.1, qSC2.2, qSC5 and qSC6) of these QTLs were detected in more than one environment. The QTL identified in more than one environment would be useful for further fine-mapping and marker assisted selection. Some of the QTLs identified in this study have been reported in previous studies. For example, QTLs qSD2.3 and qSD5 detected for Striga damage, qSC2.1 and qSC2.2 for Striga emergence counts in this study have been previously reported by Stanley et al. (2021). The authors identified significant SNP markers S5_70442824 and S2_188120710 to be associated with Striga damage ratings as well as S2_135038935 and S2_208978140 associated with number of emerged Striga plants in a genome-wide association study to detect SNP markers linked with Striga resistance traits in late maturing maize germplasm. Similarly, the QTL qGY-8 has been previously reported by Badu-Apraku et al. (2020a); qSD-10 by Adewale et al. (2020) and Okunlola et al. (2022). Six major effect QTLs qGY4, qGY5, qSD5, qSC2.2, qsc7.1 and qsc9 were identified on chromosomes 2, 4, 5, 7, and 9, explaining phenotypic variance varying from 10.2% to 14.4%. These QTLs are promising for the introgression of favorable alleles to improve Striga resistance in maize through marker-assisted selection. Identification of stable genomic regions inside QTLs will serve as guides in the selection of traits more efficiently. However, genotype × environment interaction QTLs are also important as they significantly influence the total phenotypic variance and additive effect of the main effect QTL located inside or close to them. In our study we detected significant genotype × environment interaction for grain yield, Striga damage and number of ears per plant. Similar signs of additive effects observed for some of the MET-QTLs and main effect QTLs revealed that these MET-QTLs had positive effects on the total additive value of the Striga resistance adaptive traits. This implied that these MET-QTLs had positive effect on the Striga resistance alleles which improved the phenotypic expression resulting in increased grain yield and reduced Striga damage under Striga infestation.
The QTL qGY2 associated with grain yield is linked to gene models GRMZM2G077002 and GRMZM2G404973, C2C2-GATA-transcription factors. The GATA transcription factors are widely involved in the regulation of plant developmental and growth processes, including seed germination, development, light-mediated signalling, and regulation of plant nitrogen metabolism (An et al., 2020). GATA motifs have been detected in regulatory regions of many genes involved in nitrogen assimilation, such as nitrate reductase, nitrite reductase, and glutamine synthetase (Hudson et al., 2011). Therefore, GATA transcription factors play a potential role in coordinating nutrient assimilation and vegetative growth. Similarly, the QTLs qGY2 and qSC8 were identified to be linked with the gene models GRMZM2G178998 and GRMZM2G134073, respectively (NAC transcription factors). The NAC transcription factors play important roles in the regulation of transcriptional reprogramming associated with plant stress responses (Nuruzzaman et al., 2013). Genes in the NAC family regulate a wide range of developmental processes, including seed development, embryo development, shoot apical meristem formation, fiber development, and cell division. The NAC transcription proteins participate in the regulation of immune signalling pathways to execute critical functions in plant immunity (Yuan et al., 2019). When plants are attacked by pathogens, they perceive the pathogen-derived signals and often activate an efficient and complicated but fine-tuned network of defense hormone-mediated signalling pathways (Peng et al., 2018; Yuan et al., 2019).
The QTLs qGY5 and qSD5 found to be associated with the gene models GRMZM2G053868 and GRMZM2G157068 both encode the nitrate transporter protein. Nitrate is a major source of N in higher plants. A large proportion of NO3 acquired by plants from the soil is actively transported through NO3 transporters (NRTs) (Bai et al., 2013). To cope with low or high nitrate concentrations in the soil, plant roots have developed high-affinity and low-affinity nitrate uptake systems involving certain nitrate transporter genes. The nitrate transporter (NRT3) proteins are partner proteins that interact with most NRT2 proteins and contribute to high-affinity nitrate uptake (Guo et al., 2020). Thus, the NRT2 genes combine with the NRT3 genes to enable plants to cope with variable nitrate supplies, thereby improving nitrogen use efficiency. Adewale et al. (2020) in a genome-wide association study to identify markers linked to Striga resistance in early maturing tropical maize, found marker SNP S10_133224759 close to the functional gene GRMZM2G164743 (bin 10.05), which encodes an ammonium transporter protein (amt5). Further studies on the nitrate transporter candidate genes could help to understand the regulatory mechanism of Striga resistance in the roots of maize plants and determine their usefulness in selecting genotypes with resistance to Striga parasitism.
The QTL qSC8 associated with Striga emergence count was found to be linked with the gene model, GRMZM2G371033, SBP-transcription factor. The SBP-transcription factors are very important for plant growth, development, and defense response as they regulate the specific downstream gene expression, such as the phase transition from vegetative to reproductive stage, leaf development, plant hormone signaling, toxin resistance, copper deficiency response, temperature, and drought stress tolerance (Jiang et al., 2021). The gene model GRMZM2G075715, ARF-transcription factor was associated with the QTL qSC7 identified for Striga emergence count. Auxin response transcription factors play key roles in plant development, particularly in the regulation of gene expression (Saidi and Hajibarat, 2020; Pratt and Zhang, 2021). Auxin is a plant hormone involved in various stages of plant growth and development, such as apical dominance, tropisms, and vascular patterning. Auxin plays key roles in cell division and cell expansion during the developmental stages and in regulation of a variety of physiological processes including lateral root initiation and shoot elongation (Di et al., 2016). The QTL qEPP1 associated with ears per plant was found to be linked with the candidate gene GRMZM2G021095, LBD-transcription factor. The lateral organ boundaries domain (LBD) genes, are plant-specific transcription factor family which play crucial roles in controlling plant architecture, regulating lateral organ development, morphogenesis, and stress tolerance in plants (Majer and Hochholdinger, 2011; Wang et al., 2021). The LBD genes are expressed in a band of cells at the adaxial base of all lateral organs formed from the shoot apical meristem and at the base of lateral roots. The LBD genes also regulate plant cell wall thickening and secondary growth (Chen et al., 2017).
Several well-studied plant transcription factor families, including ethylene response factor (ERF), basic-domain leucine-zipper protein (bZIP), MYB, WRKY, NAC, and members of the basic helix-loop-helix (bHLH) transcription factors associated with defense responses in plants were identified in our study to be associated with Striga resistance adaptive traits. Stanley et al. (2021) identified the bHLH transcription factors, putative leucine-rich repeat protein and bZIP transcription factors in a GWAS to identify Striga resistance linked markers using the IITA intermediate and late maturing maize inbred lines under artificial Striga infestation. Similarly; Gowda et al. (2021) found the bHLH transcription factors to be associated with Striga resistance traits in maize. These transcription factor families regulate gene expression in response to a range of biotic stimuli, including microbes (fungi, oomycetes, bacteria) and insects, and downstream defense signalling hormones such as salicylic acid, jasmonic acid, and ethylene (Thatcher et al., 2012). The key candidate genes identified in our study should be further validated to ascertain their influence in improving resistance to Striga parasitism in tropical maize germplasm.
Conclusion
The results of this study further elucidated the understanding and deployment of molecular markers in Striga resistance breeding. Twenty-three QTLs were detected for four traits associated with Striga resistance across four different environments at two locations over 2 years using the F2:3 mapping population. The phenotypic variance explained by each QTL ranged from 4.1% to 14.4%. The QTL qSC2.1 was consistently detected in three individual environments and across environments. Our results further revealed the genetic basis of Striga resistance traits in maize and will be useful for the marker-assisted selection of Striga-resistant maize genotypes, laying the foundation for the fine mapping and cloning of the gene underlying the stable QTLs identified in more than one environment in this study.
Data availability statement
The raw data used/analyzed in this manuscript will be made available by the authors upon request.
Author contributions
BB-A: Conceptualization, resources, design of experiment, data collection, writing—review and editing; SA: Data collection, curation, phenotypic, and genotypic data analysis, writing—original draft; MG: Resources, writing—review and editing; AP: Writing—review and editing, QO: Leaf sample collection, writing—review and editing. All authors read and approved the final version of the manuscript.
Funding
This research was supported by the Bill & Melinda Gates Foundation [OPP1134248] under the Stress Tolerant Maize for Africa (STMA) project.
Acknowledgments
The authors are also grateful to the IITA Maize Improvement Programme and Bioscience staff for providing technical assistance.
Conflict of interest
The authors declare that the research was conducted in the absence of any commercial or financial relationships that could be construed as a potential conflict of interest.
Publisher’s note
All claims expressed in this article are solely those of the authors and do not necessarily represent those of their affiliated organizations, or those of the publisher, the editors and the reviewers. Any product that may be evaluated in this article, or claim that may be made by its manufacturer, is not guaranteed or endorsed by the publisher.
Supplementary material
The Supplementary Material for this article can be found online at: https://www.frontiersin.org/articles/10.3389/fgene.2023.1012460/full#supplementary-material
References
Adewale, S. A., Badu-Apraku, B., Akinwale, R. O., Paterne, A. A., Gedil, M., and Garcia-Oliveira, A. L. (2020). Genome-wide association study of Striga resistance in early maturing white tropical maize inbred lines. BMC Plant Biol. 20, 203–216. doi:10.1186/s12870-020-02360-0
Akaogu, I. C., Badu-Apraku, B., Tongoona, P., Ceballos, H., Gracen, V., Offei, S. K., et al. (2019). Inheritance of Striga hermonthica adaptive traits in an early-maturing white maize inbred line containing resistance genes from Zea diploperennis. Plos One 138, 546–552. doi:10.1111/pbr.12707
Almeida, G. D., Makumbi, D., Magorokosho, C., Nair, S., Borém, A., Ribaut, J. M., et al. (2013). QTL mapping in three tropical maize populations reveals a set of constitutive and adaptive genomic regions for drought tolerance. Theor. Appl. Genet. 126 (3), 583–600. doi:10.1007/s00122-012-2003-7
Alvarado, G., López, M., Vargas, M., Pacheco, A., Rodríguez, F., Burgueño, J., et al. (2016). META-R (multi environment trial analysis with R for windows). Version 6.0. Available at: http://hdl.handle.net/11529/10201 International Maize and Wheat Improvement Center.
Amusan, I. O., Rich, P. J., Menkir, A., Housley, T., and Ejeta, G. (2008). Resistance to Striga hermonthica in a maize inbred line derived from Zea diploperennis. New Phytol. 178, 157–166. doi:10.1111/j.1469-8137.2007.02355.x
An, Y., Zhou, Y., Han, X., Shen, C., Wang, S., Liu, C., et al. (2020). The GATA transcription factor GNC plays an important role in photosynthesis and growth in poplar. J. Exp. Bot. 71 (6), 1969–1984. doi:10.1093/jxb/erz564
Arends, D., Prins, P., Jansen, R. C., and Broman, K. W. (2010). R/qtl: High-throughput multiple QTL mapping. J. Bioinform 26 (23), 2990–2992. doi:10.1093/bioinformatics/btq565
Atera, E. A., Onyango, J. C., Thanh, P. T., Ishii, T., and Itoh, K. (2015). Identification of QTL for Striga hermonthica resistance using backcross population derived from a cross between Oryza sativa (cv. Nipponbare) and O. rufipogon. J. Agric. Sci. 7 (2), 99–105. doi:10.5539/jas.v7n2p99
Badu-Apraku, B., Adewale, S., Paterne, A., Gedil, M., and Asiedu, R. (2020a). Identification of QTLs controlling resistance/tolerance to Striga hermonthica in an extra-early maturing yellow maize population. Agronomy 10, 1168. doi:10.3390/agronomy10081168
Badu-Apraku, B., Adewale, S., Paterne, A. A., Gedil, M., Toyinbo, J., and Asiedu, R. (2020b). Identification of QTLs for grain yield and other traits in tropical maize under Striga infestation. Plos One 15 (9), e0239205. doi:10.1371/journal.pone.0239205
Badu-Apraku, B., and Fakorede, M. A. B. (2017). Advances in genetic enhancement of early and extra-early maize for sub-Saharan Africa. Cham, Switzerland: Springer.
Bai, H., Euring, D., Volmer, K., Janz, D., and Polle, A. (2013). The nitrate transporter (NRT) gene family in Poplar. Plos One 8 (8), e72126. doi:10.1371/journal.pone.0072126
Berger, D. K., Carstens, M., Korsman, J. N., Middleton, F., Kloppers, F. J., Tongoona, P., et al. (2014). Mapping QTL conferring resistance in maize to gray leaf spot disease caused by Cercospora Zeina. BMC Genet. 15, 60. doi:10.1186/1471-2156-15-60
Broman, K. W., Wu, H., Sen, S., and Churchill, G. A. (2003). R/qtl: QTL mapping in experimental crosses. J. Bioinform. 19, 889–890. doi:10.1093/bioinformatics/btg112
Chattopadhyay, K., Behera, L., Bagchi, T. B., Sardar, S. S., Moharana, N., Patra, N. R., et al. (2019). Detection of stable QTLs for grain protein content in rice (Oryza sativa L.) employing high throughput phenotyping and genotyping platforms. Sci. Rep. 9, 3196. doi:10.1038/s41598-019-39863-2
Chen, X., Wang, J., Zhao, M., and Yuan, H. (2017). Identification and expression analysis of lateral organ boundaries domain (LBD) transcription factor genes in Fragaria vesca. Can. J. Plant Sci. 98 (2), 288–299. doi:10.1139/cjps-2017-0098
Collard, B., Jahufer, M., Brouwer, J., and Pang, E. (2005). An introduction to markers, quantitative trait loci (QTL) mapping and marker-assisted selection for crop improvement: The basic concepts. Euphytica 142, 169–196. doi:10.1007/s10681-005-1681-5
Di, D. W., Wu, L., Zhang, L., An, C. W., Zhang, T. Z., Luo, P., et al. (2016). Functional roles of Arabidopsis CKRC2/YUCCA8 gene and the involvement of PIF4 in the regulation of auxin biosynthesis by cytokinin. Sci. Rep. 6, 36866. doi:10.1038/srep36866
Gowda, M., Makumbi, D., Das, B., Nyaga, C., Kosgei, T., Crossa, J., et al. (2021). Genetic dissection of Striga hermonthica (Del.) Benth. resistance via genome-wide association and genomic prediction in tropical maize germplasm. Theor. Appl. Genet. 134, 941–958. doi:10.1007/s00122-020-03744-4
Guo, B., Li, Y., Wang, S., Li, D., Lv, C., and Xu, R. (2020). Characterization of the nitrate transporter gene family and functional identification of HvNRT2.1 in barley (Hordeum vulgare L.). Plos One 15, e0232056. doi:10.1371/journal.pone.0232056
Hudson, D., Guevara, D., Yaish, M. W., Hannam, C., Long, N., Clarke, J. D., et al. (2011). GNC and CGA1 modulate chlorophyll biosynthesis and glutamate synthase (GLU1/Fd-GOGAT) expression in Arabidopsis. PLoS One 6, e26765. doi:10.1371/journal.pone.0026765
Jamil, M., Wang, J. Y., Yonli, D., Ota, T., Berqdar, L., Traore, H., et al. (2022). Striga hermonthica suicidal germination activity of potent strigolactone analogs: Evaluation from laboratory bioassays to field trials. Plants 11, 1045. doi:10.3390/plants11081045
Jiang, X., Chen, P., Zhang, X., Liu, Q., and Li, H. (2021). Comparative analysis of the SPL gene family in five rosaceae species: Fragaria vesca, Malus domestica, Prunus persica, Rubus occidentalis, and Pyrus pyrifolia. Open Life Sci. 16, 160–171. doi:10.1515/biol-2021-0020
Kim, S. K. (1991). “Breeding maize for Striga tolerance and the development of a eld infestation technique,” in Combating Striga in Africa: Proceedings of an InternationalWorkshop organized by IITA, ICRISAT and IDRC, Ibadan, Nigeria, 22–24Aug. 1988 (IITA), 96–108.
Kling, J. G., Fajemisin, J. M., Badu-Apraku, B., Diallo, A., Menkir, A., and Melake-Berhan, A. (2000). “Striga resistance breeding in maize,” in Breeding for Striga resistance in cereals. Editors B. I. G. Haussmann, D. E. Hess, M. L. Koyama, L. Grivet, H. F. W. Rattunde, and H. H. Geiger (Weikersheim, Germany: Margraf Verlag), 103–118.
Ladejobi, O., Salaudeen, M. T., Kumar, L., Menkir, A., Adesoye, A., Atiri, G., et al. (2018). Mapping of QTLs associated with recovery resistance to streak virus disease in maize. Ann. Agric. Sci. 63, 115–121. doi:10.1016/j.aoas.2018.05.006
Li, S., Wang, J., and Zhang, L. (2015). Inclusive composite interval mapping of QTL by environment interactions in biparental populations. PLoS One 10, e0132414. doi:10.1371/journal.pone.0132414
Ma, L., Ijaz, B., Wang, Y-M., and Hua, J-P. (2018). Dynamic QTL analysis and validation for plant height using maternal and paternal backcrossing populations in upland cotton. Euphytica 214, 167. doi:10.1007/s10681-018-2243-y
Majer, C., and Hochholdinger, F. (2011). Defining the boundaries: Structure and function of LOB domain proteins. Trends Plant Sci. 16, 47–52. doi:10.1016/j.tplants.2010.09.009
Maschietto, V., Colombi, C., Pirona, R., Pea, G., Strozzi, F., Marocco, A., et al. (2017). QTL mapping and candidate genes for resistance to Fusarium ear rot and fumonisin contamination in maize. BMC Plant Biol. 17, 20. doi:10.1186/s12870-017-0970-1
McCouch, S. R., Cho, Y., Yano, M., Paul, E., Blinstrub, M., Morishima, H., et al. (1997). Report on QTL nomenclature. Rice Genet. Newlett. 14, 11–13.
Meng, L., Li, H., Zhang, L., and Wang, J. (2015). QTL IciMapping: Integrated software for genetic linkage map construction and quantitative trait locus mapping in biparental populations. Crop J. 3 (3), 269–283. doi:10.1016/j.cj.2015.01.001
Menkir, A., and Kling, J. G. (2007). Response to recurrent selection for resistance to Striga hermonthica (Del.) Benth in a tropical maize population. Crop Sci. 47 (2), 674–682. doi:10.2135/cropsci2006.07.0494
Mondal, S. A., and Badigannavar, A. M. (2019). Identification of major consensus QTLs for seed size and minor QTLs for pod traits in cultivated groundnut (Arachis hypogaea L.). 3 Biotech. 9, 347. doi:10.1007/s13205-019-1881-7
Nuruzzaman, M., Sharoni, A. M., and Kikuchi, S. (2013). Roles of NAC transcription factors in the regulation of biotic and abiotic stress responses in plants. Front. Microbiol. 4, 248. doi:10.3389/fmicb.2013.00248
Okunlola, G., Badu-Apraku, B., Ariyo, J., Agre, J., Offernedo, Q., and Ayo-Vaughan, Q. (2022). Genome-wide association studies of Striga resistance in extra-early maturing quality protein maize inbred lines. G3 (Bethesda), jkac237. doi:10.1093/g3journal/jkac237
Parker, C., and Riches, C. R. (1993). Parasitic weeds of the world: Biology and control. Wallingford, UK: CAB International, 332. ISBN: 9780851988733.
Peng, Y., van Wersch, R., and Zhang, Y. (2018). Convergent and divergent signaling in PAMP-triggered immunity and effector-triggered immunity. Mol. Plant-Microbe Interact. 31, 403–409. doi:10.1094/MPMI-06-17-0145-CR
Pratt, I. S., and Zhang, B. (2021). Genome-wide identification of ARF transcription factor gene family and their expression analysis in Sweet Potato. Int. J. Mol. Sci. 22 (17), 9391. doi:10.3390/ijms22179391
Ribeiro, P. F., Badu-Apraku, B., Gracen, V. E., Danquah, E. Y., Garcia-Oliveira, A. L., Asante, M. D., et al. (2018). Identification of quantitative trait loci for grain yield and other traits in tropical maize under high and low soil-nitrogen environments. Crop Sci. 58, 321–331. doi:10.2135/cropsci2017.02.0117
Rich, P. J., and Ejeta, G. (2008). Towards effective resistance to Striga in African maize. Plant Signal. Behav. 3 (9), 618–621. doi:10.4161/psb.3.9.5750
Rodenburg, J., Bastiaans, L., Weltzien, E., and Hess, D. E. (2005). How can field selection for Striga resistance and tolerance in Sorghum be improved? Field crop. Res. 93, 34–50. doi:10.1016/j.fcr.2004.09.004
Rodenburg, J., Cissoko, M., Kayongo, N., Dieng, I., Bisikwa, J., Irakiza, R., et al. (2017). Genetic variation and host-parasite specificity of Striga resistance and tolerance in rice: The need for predictive breeding. New Phytol. 214 (3), 1267–1280. doi:10.1111/nph.14451
Saidi, A., and Hajibarat, Z. (2020). Computational study of environmental stress-related transcription factor binding sites in the promoter regions of maize auxin response factor (ARF) gene family. Not. Sci. Biol. 12 (3), 646–657. doi:10.15835/nsb12310823
Shang, L., Liu, F., Wang, Y., Abduweli, A., Cai, S., Wang, K., et al. (2015). Identification of stable QTLs controlling fiber traits properties in multi-environment using recombinant inbred lines in Upland cotton (Gossypium hirsutum L.). Euphytica 205 (3), 877–888. doi:10.1007/s10681-015-1434-z
Singhal, T., Satyavathi, C. T., Singh, S. P., Kumar, A., Sankar, S. M., Bhardwaj, C., et al. (2021). Multi-environment quantitative trait loci mapping for grain iron and zinc content using bi-parental recombinant inbred line mapping population in pearl millet. Front. Plant Sci. 12, 659789. doi:10.3389/fpls.2021.659789
Soil Survey Staff (1999). Soil taxonomy: A basic system of soil classification for making and interpreting soil survey. 2nd ed. Washington, US: Agricultural Handbook 436, Natural Resource Conservation Service USDA, Washington, US Government Printing Office, 869–873.
Stanley, A. E., Menkir, A., Ife, B., Paterne, A. A., Unachukwu, N. N., Meseka, S., et al. (2021). Association analysis for resistance to Striga hermonthica in diverse tropical maize inbred lines. Sci. Rep. 11, 24193. doi:10.1038/s41598-021-03566-4
Su, C., Wang, W., Gong, S., Zuo, J., Li, S., and Xu, S. (2017). High density linkage map construction and mapping of yield trait QTLs in maize (Zea mays) using the genotyping-by-sequencing (GBS) technology. Front. Plant Sci. 8, 706. doi:10.3389/fpls.2017.00706
Thatcher, L. F., Powell, J. J., Aitken, E. A. B., Kazan, K., and Manners, J. M. (2012). The lateral organ boundaries domain transcription factor LBD20 functions in fusarium wilt susceptibility and jasmonate signaling in Arabidopsis. Plant Physiol. 160 (1), 407–418. doi:10.1104/pp.112.199067
Tuberosa, R., Salvi, S., Sanguineti, M. C., Landi, P., Maccaferri, M., and Conti, S. (2002). Mapping QTLs regulating morpho-physiological traits and yield: Case studies, shortcomings and perspectives in drought-stressed maize. Ann. Bot. (Lond.) 89, 941–963. doi:10.1093/aob/mcf134
Vengadessan, V., Rai, K. N., Bapu, J. R. K., Hash, C. T., Bhattacharjee, R., Senthilvel, S., et al. (2013). Construction of genetic linkage map and QTL analysis of sink-size traits in Pearl Millet (Pennisetum glaucum). Int. Sch. Res. Not. 2013, 1–14. Article ID 471632. doi:10.5402/2013/471632
Wang, H., Jia, J., Cai, Z., Duan, M., Jiang, Z., Xia, Q., et al. (2022). Identification of quantitative trait loci (QTLs) and candidate genes of seed iron and zinc content in soybean [Glycine max (L.) Merr.]. BMC Genom 23, 146. doi:10.1186/s12864-022-08313-1
Wang, Z., Zhang, R., Cheng, Y., Lei, P., Song, W., Zheng, W., et al. (2021). Genome-wide identification, evolution, and expression analysis of LBD transcription factor family in bread wheat (Triticum aestivum L.). Front. Plant Sci. 12, 721253. doi:10.3389/fpls.2021.721253
Woodhouse, M. R., Cannon, E. K., Portwood, J. L., Harper, L. C., Gardiner, J. M., Schaeffer, M. L., et al. (2021). A pan-genomic approach to genome databases using maize as a model system. BMC Plant Biol. 21, 385. doi:10.1186/s12870-021-03173-5
Yang, J., Liu, Z., Chen, Q., Qu, Y., Tang, J., Lubberstedt, T., et al. (2020). Mapping of QTL for grain yield components based on a DH population in maize. Sci. Rep. 10, 7086. doi:10.1038/s41598-020-63960-2
Yuan, X., Wang, H., Cai, J., Li, D., and Song, F. (2019). NAC transcription factors in plant immunity. Phytopathol. Res. 1, 3. doi:10.1186/s42483-018-0008-0
Zhang, X., Guan, Z., Li, Z., Liu, P., Ma, L., Zhang, Y., et al. (2020). A combination of linkage mapping and GWAS brings new elements on the genetic basis of yield-related traits in maize across multiple environments. Theor. Appl. Genet. 133 (10), 2881–2895. doi:10.1007/s00122-020-03639-4
Keywords: Striga resistance, QTL x environment, candidate genes, marker assisted selction, wild maize
Citation: Badu-Apraku B, Adewale S, Paterne A, Offornedo Q and Gedil M (2023) Mapping quantitative trait loci and predicting candidate genes for Striga resistance in maize using resistance donor line derived from Zea diploperennis. Front. Genet. 14:1012460. doi: 10.3389/fgene.2023.1012460
Received: 05 August 2022; Accepted: 02 January 2023;
Published: 12 January 2023.
Edited by:
Casper Nyaradzai Kamutando, University of Zimbabwe, ZimbabweReviewed by:
Sudhakar Reddy Palakolanu, International Crops Research Institute for the Semi-Arid Tropics (ICRISAT), IndiaAbdulwahab Saliu Shaibu, Bayero University Kano, Nigeria
Copyright © 2023 Badu-Apraku, Adewale, Paterne, Offornedo and Gedil. This is an open-access article distributed under the terms of the Creative Commons Attribution License (CC BY). The use, distribution or reproduction in other forums is permitted, provided the original author(s) and the copyright owner(s) are credited and that the original publication in this journal is cited, in accordance with accepted academic practice. No use, distribution or reproduction is permitted which does not comply with these terms.
*Correspondence: B. Badu-Apraku, b.badu-apraku@cgiar.org