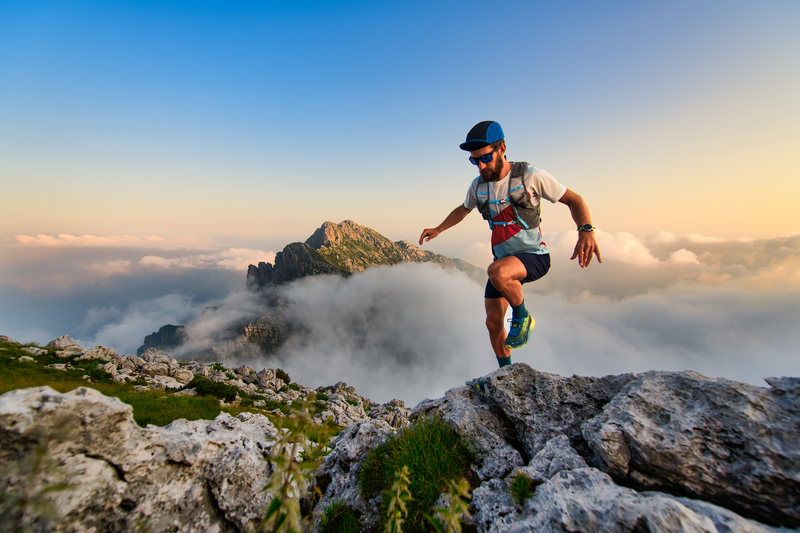
94% of researchers rate our articles as excellent or good
Learn more about the work of our research integrity team to safeguard the quality of each article we publish.
Find out more
ORIGINAL RESEARCH article
Front. Genet. , 26 September 2022
Sec. Epigenomics and Epigenetics
Volume 13 - 2022 | https://doi.org/10.3389/fgene.2022.998147
This article is part of the Research Topic RNA-binding Proteins in Cancer: Advances in Translational Research View all 7 articles
Background: RNA modification is one of the epigenetic mechanisms that regulates post-transcriptional gene expression, and abnormal RNA modifications have been reported to play important roles in tumorigenesis. N7-methylguanosine (m7G) is an essential modification at the 5′ cap of human mRNA. However, a systematic and pan-cancer analysis of the clinical relevance of m7G related regulatory genes is still lacking.
Methods: We used univariate Cox model and Kaplan-Meier analysis to generate the forest plot of OS, PFI, DSS and identified the correlation between the altered expression of m7G regulators and patient survival in 33 cancer types from the TCGA and GTEx databases. Then, the “estimate” R-package, ssGSEA and CIBERSORT were used to depict the pan-cancer immune landscape. Through Spearman’s correlation test, we analyzed the correlation between m7G regulators and the tumor microenvironment (TME), immune subtype, and drug sensitivity of the tumors, which was further validated in NSCLC. We also assessed the changes in the expression of m7G related regulatory genes in NSCLC with regards to the genetic and transcriptional aspects and evaluated the correlation of METTL1 and WDR4 expression with TMB, MSI and immunotherapy in pan-cancer.
Results: High expression of most of the m7G regulators was significantly associated with worse prognosis. Correlation analyses revealed that the expression of majority of the m7G regulators was correlated with tumor immune infiltration and tumor stem cell scores. Drug sensitivity analysis showed that the expression of CYFP1,2 was closely related to drug sensitivity for various anticancer agents (p < 0.001). Analysis of the pan-cancer immune subtype revealed significant differences in the expression of m7G regulators between different immune subtypes (p < 0.001). Additionally, the types and proportions of mutations in METTL1 and WDR4 and their relevance to immunotherapy were further described.
Conclusion: Our study is the first to evaluate the correlation between the altered expression of m7G regulators and patient survival, the degree of immune infiltration, TME and drug sensitivity in pan-cancer datasets.
Similar to the epigenetic code formed by DNA and histone modifications, post-transcriptional modifications in RNA profoundly affect cellular functions and fate, often termed as “RNA epigenetics” or “epitranscriptome,” and have attracted wide attention in recent years (He, 2010). There are over 150 known RNA modifications, which include N6-methyladenosine (m6A), N7-methylguanosine (m7G), 5-methylcytidine (m5C), pseudouridine (Ψ), N6,2′-O-dimethyladenosine (m6Am), N1-methyladenosine (m1A), alternative polyadenylation (APA), 2′-O-methylated nucleotides (Nm), N4-acetylcytidine (ac4C), adenosine-to-inosine RNA editing (I), and cytidine-to-uridine RNA editing (U), all of which contribute to normal development and homeostasis and their dysregulation is known to cause various diseases including cancer (Boccaletto et al., 2022; Zhao et al., 2017b; Frye et al., 2018; Roundtree et al., 2017; Barbieri et al., 2017; Barbieri and Kouzarides, 2020). Previous studies have focused more on m6A, one of the most common modified forms in eukaryotic cells, which have been demonstrated to play a role in embryogenesis, neurogenesis, hematopoiesis, and tumorigenesis in some studies (Zhao et al., 2017a; Zhang et al., 2017; Barbieri et al., 2017; Xu et al., 2021a). However, in recent years, modifications in non-m6A forms have also attracted increasing attention. For example, Xu et al. (2021b) investigated the potential relationship between regulators of non-m6a RNA modification and the clinical characteristics, TME status and GBM subtypes, and identified that three non-m6a RNA modification patterns were associated with distinct biological pathways and clinical characteristics.
m7G is widespread in numerous RNA cap structures as well as in tRNA, rRNA, mRNA and miRNA, is known to affect all the stages of RNA processing including splicing, export, decay, and controls the mRNA translation (Galloway and Cowling, 2019; Enroth et al., 2019; Zhang et al., 2019a; Malbec et al., 2019; Pandolfini et al., 2019). m7G modification processes are evolutionarily conserved, which are necessary for normal development (Malbec et al., 2019). Recently, a growing number of studies have implicated an important role of m7G-related regulators in the onset and progression of a variety of cancers, which were reported to be closely associated with patient prognosis. For example, METTL1 mediates m7G modification on tRNA, drives oncogene transformation and tumor formation by upregulating the translation of specific mRNAs such as growth-promoting proteins (Orellana et al., 2021). EIF3D is a subunit of the EIF3 complex with Cap-binding activity (Lee et al., 2016). EIF3D is elevated in gallbladder cancer (GBC) and promotes the proliferation and migration of malignant tumor cells (Zhang et al., 2021). Currently, lung cancer is one of the most prevalent malignancies worldwide and the leading cause of cancer-related death (Sung et al., 2021; Siegel et al., 2022). Recent studies reporting the targeting of specific m7G-related regulators have shown that m7G and lung cancer were closely related. METTL1-WDR4 mediates tRNA modifications and tRNA-decoded codon usage, which promotes mRNA translation leading to the proliferation and invasion of lung cancer cells. Furthermore, the WDR4/PML axis is also overactive in lung cancer and promotes tumor progression in the immunosuppressive and pre-metastatic microenvironment (Ma et al., 2021; Wang et al., 2017). EIF4G1, a scaffolding protein that anchors eIF4E, eIF4A1, the eIF3 complex, etc., is selectively upregulated in tumors, which is associated with enhanced Cap-independent initiation, especially in LUAD. The dramatically elevated expression of EIF4G1 also positively correlates with the expression of different cell cycle-related proteins (Wu and Wagner, 2021).
In this study, we analyzed the m7G-related regulators in pan-cancer datasets, and further evaluated the correlation of the differentially expressed m7G-related regulators with patient survival. Next, we specifically mapped the immune landscape in each cancer and analyzed the correlation between the differentially expressed m7G regulators and the TME across different cancers and drug sensitivity. Our findings may provide further direction for future studies exploring novel mechanisms and therapeutic targets in cancer.
The normal tissue samples from genotype tissue expression (GTEx) and TCGA-GDC pan-cancer data including the transcriptional data (FKPM values) from 33 cancer types, immunophenotype data, stemness scores (calculated by DNA methylation and RNA expression, respectively), clinical variables, and genetic and transcriptional changes in the m7G-related genes were downloaded from UCSC Xena (https://xenabrowser.net/). The transcriptional data were recalculated with a unifying pipeline. Abbreviations for the different types of cancer are listed in Supplementary Table S1. Five m7G regulator gene sets were obtained from the GSEA’s MSigDB Home database (http://www.gsea-msigdb.org/gsea/index.jsp) as shown in Supplementary Table S2. The transcriptional and clinical data from GSE78220 (Hugo et al., 2016) based on the GPL11154 platform for pre-treated melanoma patients was obtained from the GEO database (https://www.ncbi.nlm.nih.gov/geo/). The IMvigor210 trial is a single-arm phase II study of atezolizumab in patients with metastatic urothelial carcinoma (Mariathasan et al., 2018), data from the IMvigor210 trial were obtained using the IMvigor210CoreBiologies R package (http://research-pub.gene.com/IMvigor210CoreBiologies/). We also used http://cis.hku.hk/TISIDB/ (Ru et al., 2019) to analyze the correlation between the expression of METTL1 and WDR4 and the expression of immunoinhibitors across pan-cancer.
The gene symbol of transcriptional data was obtained from Ensembl (https://asia.ensembl.org/index.html). Based on previous studies, we selected 28 cancer types that had more than five contiguous normal specimens (after merging with GTEx) among the 33 cancer types in further comparative analysis (Yu et al., 2020; Zhang et al., 2020a). The “corrplot” R package was used to analyze the correlation between the m6A regulators and m7G regulators. The R-package “limma,” “ggplot2,” and “reshape2” were used to investigate the correlation between the m7G regulators and the immune subtypes. The immune score, stromal score, and estimate score of pan-cancers were generated using the “estimate” R-package. The gene expression and drug sensitivity data were obtained from the CellMiner database (https://discover.nci.nih.gov/cellminer/home.do) and were filtered by clinical trials or FDA approved standards. p-value < 0.05 was considered as statistically significant.
The prognosis for overall survival (OS), progression-free interval (PFI) and disease-specific survival (DSS) of pan-cancer patients were compared with the expression level of m7G-related genes. Kaplan–Meier curves were generated to display the differences in the OS of patients. The univariate Cox proportional hazards regression models’ analyses were obtained for different samples from each type of cancer by “survival” and “survminer” R package.
We first quantified the level of enrichment of m7g-related genes in each sample by scoring with single-sample gene-set enrichment analysis (ssGSEA) (Shen et al., 2019). The proportions of 22 human immune cell subsets were calculated by CIBERSORT (Newman et al., 2015). The ssGSEA score and immune cell proportions were used to evaluate the correlation between the activity of m7g-related genes and the immune cell infiltration level in pan-cancer dataset, and the “limma” R-package was used to construct the corresponding heatmap.
The alteration in the expression of METTL1 and WDR4 in the TCGA pan-cancer database was examined using the cBioportal database (https://www.cbioportal.org/). The “maftools” R package was employed to generate the pan-cancer mutation annotation format for the data downloaded from the UCSC Xena. The tumor mutation burden (TMB) score for each patient within pan-cancer was calculated and analyzed for its correlation with METTL1 and WDR4 expression, respectively. Mutation and microsatellite instability analysis (MSI) is characterized by a wide range of microsatellite sequence polymorphisms due to mutations in DNA polymerase, which is used as an indicator of genetic instability for cancer detection (Li et al., 2020). The MSI score of METTL1 and WDR4 for each patient within pan-cancer was also investigated in the same way as the TMB score.
The mixed-effects linear models were used to compare the gene expression between the pan-cancer samples and their adjacent normal samples. The heatmap was created to visualize the differential gene expression between the tumor and normal tissues across all cancer types, while box plots were used to show the gene expression in each cancer type. Spearman correlation was used to perform the correlation analysis included in this study. Wilcox test or Kruskal-Wallis test was used to compare the different groups depending on the number of comparisons. All statistical analyses were performed using the R version 4.1.3 and all the reported p-values were two-tailed and p < 0.05 was considered as statistically significant.
We obtained 41 m7G-related regulatory genes by searching GSEA’s MSigDB Home database and classified them into three classes based on their molecular function and biological process: N7 methylation (WDR4, BUD23, METTL1, TRMT112, RAMAC, RNMT); CAP-dependent translational initiation (Lin et al., 2007; Van Der Kelen et al., 2009) (EIF4G1, NCK1, MIF4GD, SLBP, EIF3D); and CAP formation genes (SNUPN, EIF4A1, EIF4E, NCBP2, CYFIP1, LARP1, IFIT5, EIF4E1B, GEMIN5, CYFIP2, AGO2, LSM1, DCPS, EIF4E3, NCBP2L, NCBP1, NCBP3, EIF3D, EIF4G3, EIF4E2, DCP2, NUDT1, NUDT10, NUDT11, NUDT16, NUDT16L1, NUDT3, NUDT4, NUDT4B, NUDT5, NUDT7), respectively. After analyzing the differential expression of 41 genes in 33 tumors and their matched normal tissues, 10 genes (EIF4A1, EIF4E, EIF4E1B, EIF4E3, NCBP2L, NUDT10, NUDT11, NUDT3, NUDT4B, NUDT7) with no significant difference in their expression (logFC absolute value <2) were eliminated. The remaining 31 genes were subjected to further pan-cancer analyses (Figure 1A).
FIGURE 1. Box plot and heat map of the differential expression of 41 m7G regulators in 18 cancer types. (A) Box plot showing the expression of 41 m7G regulators in 18 TCGA cancer tissues. (B) Heat map showing the differential expression of 31 m7G regulators across 18 cancer types.
The m7G related gene expression levels in pan-caner and normal samples were mapped in Figure 1B; Supplementary Figure S1. Most of the m7G regulators had a significantly higher expression in the tumor samples than the normal tissues, especially the N7 methylation genes. Correspondingly, there were some genes that were part of the CAP formation genes, such as NCBP3, NUDT4, CYFIP2, SNUPN, and EIF4E2, which were weakly expressed in most tumor tissues and highly expressed in normal tissues (Figure 1B; Supplementary Figure S1). Moreover, the expression of most of the CAP-dependent translational initiation genes was significantly increased in the tumor cells.
Previous studies have reported that m7G-related regulators, in particular, METTL1 and WDR4, were associated with the prognosis of cancer patients (Tian et al., 2019; Zeng et al., 2021). However, only a few studies evaluated the influence of other m7G regulators on patient prognosis. For survival analysis, all 33 cancer types were tested with univariate Cox proportional hazards regression models, and p-value < 0.05 was considered as a statistically significant association. As shown in Figure 2A, WDR4, NUDT1, BUD23 were high-risk factors in KIRC, LICH, GBM, and LGG patients. However, they were found to be low-risk factors in BLCA and DLBC patients. Kaplan-Meier survival curves showing the correlation between the 31 genes in the 33 cancers and OS, PFI and DSS are presented in Supplementary Figure S3. The results showed that the expression of the same gene in the same cancer did not result in significant differences in their OS, DSS, and PFI. Each m7G regulator was significantly associated with patient prognosis across multiple cancer types, and overall survival as the best indicator of endpoint could precisely differentiate the two risk groups. As shown in Figure 2, a general pattern was observed, wherein the elevated expression of a majority of the m7G regulators was associated with poorer survival and vice versa. For example, METTL1 was highly expressed in most tumor tissues (Supplementary Figure S1), and a univariate Cox analysis showed that the high expression of METTL1 was significantly associated with poor survival in cancer patients (Figure 2). Kaplan-Meier analysis in KIRC, MESO, and LGG patients clearly showed that increased expression of METTL1 was significantly associated with a shorter OS, PFI and DSS (Figures 2B,C). However, the expression of CYFIP2, IFIT5, and NUDT4 were negatively correlated with the prognosis of most cancer patients (Figure 2). The low expression of CYFIP2 was associated with a significantly worse prognosis in KIRC, PAAD, and THYM patients (p < 0.001) (Figures 2B,C). The low expression of IFIT5 was significantly associated with a poor prognosis in LGG, MESO, and SKCM patients (p < 0.001) (Figures 2B,C). Finally, the low expression of NUDT4 was significantly associated with a worse prognosis in ACC, KIRC, and COAD patients (p < 0.001) (Figures 2B,C).
FIGURE 2. Forest map and survival analysis of m7G regulators in multiple cancers. (A) The forest map showing the overall survival risk ratio of 31 m6A-related genes across 33 TCGA cancer types. (B) Analysis of the correlation of m7G-related regulators with OS across multiple cancer types. (C) Analysis of the correlation of m7G-related regulators with PFI across multiple cancer types (*p < 0.05,**p < 0.01, and ***p < 0.001).
As we can see from Figures 3A,B, the expression levels of most of the m7G regulators were negatively correlated with the immune score, stromal cell score and ESTIMATE score. For example, the expression of AGO2 gene was significantly negatively correlated with the immune score in SARC, implying that increased expression of AGO2 resulted in lower immune cell infiltration in SARC patients. However, the expression of IFIT5 and NCK1 were positively correlated with the immune score in most cancers. To explore the relationship between changes in the overall levels of m7G regulators and the tumor immune landscape, we used the ssGSEA score to quantify the activity or enrichment levels of the 31 m7g-related genes in the cancer samples (Supplementary Table S3) and calculated the correlation between ssGSEA score and the tumor immune infiltration. As shown in Figure 3C, m7G-related genes were correlated to tumor-associated immune cell infiltration in different cancers, and had cancer species specificity. Among them, the Mast cells resting, Macrophages M2, Dendritic cells resting, T cells CD4 memory resting, T cells CD8, Plasma cells, and B cells naïve drew our attention, which were negatively correlated with the enrichment levels of m7G-related genes in most cancer types. Additionally, we found that the expression of most of the m7G regulators were positively correlated with RNAss (RNA methylation based stemness score) and DNAss (DNA methylation based stemness score) in 33 TCGA cancer types (Figures 3D,E). Then we explored the two groups (m6A and m7G) of regulators using deconvolution algorithm and found a positive correlation between them as shown in Figure 3F. The m6A regulators were associated with the degree of immune cell infiltration in several cancer types (Li et al., 2021). We also found that the expression of the m7G methyltransferase WDR4, was positively correlated with the expression of some of the m6A methyltransferases such as METTL13, WTAP, RBM15, and RBM15B. But another m7G methyltransferase regulator TRMT112, was negatively correlated with the expression of all m6A methyltransferases. The correlation of the individual m7G regulators is also shown in Figure 3F, where many of them were positively correlated with each other. We found that WDR4 expression was positively correlated with the expression of most of the regulators except NUDT16L1, NUDT16, NUDT4, CYFIP2, and IFIT5, suggesting its important role in the formation of m7G formation relative to METTL1. In addition, as shown in Figure 3G, the expression of m7G-related genes in pan-cancer was significantly different in immune subtypes C1 (wound healing), C2 (IFN-γ dominant), C3 (inflammatory), C4 (lymphocyte depletion), C5 (immune quiet) and C6 (TGF-β dominant) (p < 0.001).
FIGURE 3. Correlation analysis between the expression of m7G-related regulators and the pan-cancer immune microenvironment with further analysis of m6A. (A) Correlation between the expression of 31 m7G methylation regulators and the immune score. (B) Correlation between the expression of 31 m7G methylation regulators and the stromal cell score. (C) Correlation between the enrichment level of m7G regulators (m7G geneset score) and tumor-associated immune cells in different cancers calculated by CIBERSORT. (D) Correlation between the expression of 31 m7G regulators and RNAss. (E) Correlation between the expression of 31 m7G regulators and DNAss. (F) The blue and red dots indicate that the expression of the m7G methylation regulators is positive and negative in relation to m6A methylation regulators, respectively. (G) The expression of m7G regulators within different immune infiltrate subtypes across all cancer types. The expression of m7G regulators within different immune infiltrate subtypes across all cancer types.
We analyzed the relationship between 31 regulatory m7G genes and drug sensitivity using the Cellminer database, and the top 25 drugs with significant correlation to their associated gene expression are presented in Figure 4. Of these, 16 drugs were significantly associated with the CYFIP gene family. CYFIP1 expression was negatively correlated with drug sensitivity towards bendamustine, XK-469, etoposide, teniposide, valrubicin, epirubicin, carmustine, BN-2629, imexon, pipobroman, melphalan, and mitoxantrone. And the expression of CYFIP2 was significantly positively correlated with the sensitivity towards nelarabine, XK-469, batracylin, and chelerythrine. The expression of DCP2 and NCK1 was positively correlated with drug sensitivity to nelarabine, while NUDT16 expression was negatively correlated with sensitivity to nelarabine. Higher expression of EIF3D and SLBP was correlated with greater drug sensitivity to hydroxyurea and amonafide, respectively. Higher expression of METTL1 and WDR4 were correlated with greater drug sensitivity to 5-fluoro-deoxy-Uri and cladribine, respectively.
FIGURE 4. Scatterplots showing the association between the expression of m7G regulators and drug sensitivity (Z-score from CellMiner interface) using NCI-60 cell line data.
The pooled analysis of the incidence of somatic mutations in the above 31 m7G regulators showed a relatively high mutation frequency in both the LUAD and LUSC cohorts. Among the 561 LUAD samples, 97 samples (17.29%) had mutations in the m7G regulators (Figure 5A). Of these, EIF4G3 had the highest mutation frequency (3%), followed by LARP1 (2%), while the three N7 methylation genes, namely BUD23, RAMAC, and TRMT112, were not mutated. Among 491 LUSC samples, 83 samples (16.9%) had mutations in the m7G regulators (Figure 5B). EIF4G3 also showed the highest mutation frequency (2%), followed by GEMIN5 (2%). Similarly, in LUAD, three m7G methyltransferase genes, namely BUD23, RAMAC, and METTL1, had no mutations.
FIGURE 5. Correlation analysis between the expression of 31 m7G regulators and clinical features in LUAD and LUSC. (A) Mutation frequencies of 31 m7G regulators in 97 and 83 patients with LUAD. (B) Mutation frequencies of 31 m7G regulators in 97 and 83 patients with LUSC from the TCGA cohort. (C) Frequencies of CNV gain, loss, and non-CNV among m7G regulators in LUAD patients. (D) Chromosomal locations of CNV changes in m7G regulators in LUAD patients. (E) Frequencies of CNV gain, loss, and non-CNV among m7G regulators in LUSC. (F) Chromosomal locations of CNV changes in m7G regulators in LUSC patients. (G) Differences in the expression of m7G regulators across different immune subtypes of LUAD. (H) Differences in the expression ofm7G regulators across different immune subtypes of LUSC.
We then examined the somatic copy number changes and found predominant copy number changes in all the 31 m7G regulators. In the LUAD samples, copy number variants (CNV) were generally elevated in AGO2, METTL1, MIF4GD, NCK1, RAMAC, while it was generally lower in CYFIP2, EIF4E2, DCP2, NUDT4, NCBP3 as shown in Figure 5C. While in the LUSC samples, CNV was generally elevated in EIF4G1, NCBP2, AGO2, LSM1, MIF4GD, and decreased in EIF4G3, IFIT5, EIF4E2 as shown in Figure 5E. Figures 5D,F shows the CNV changes in m7G regulators and their position on the chromosomes in LUAD and LUSC samples, respectively. Figure 5G shows the expression of most of the m7G regulators except EIF4G3, GEMIN5, LARP1, NUDT4, and RNMT, in different immune types C1 (wound healing), C3 (inflammation), C2 (IFN-gamma dominant), C4 (lymphocyte depletion), and C6 (TGF-beta dominant) in LUAD samples. Furthermore, the clinical subgroup analysis in Supplementary Figure S4A showed significant differences in the expression of CYFIP2, EIF3D, DCP2, NUDT16, SLBP, and RNMT in pathologic T-stage LUAD. The expression of METTL1, WDR4, NCBP3, DCP2, NUDT1, EIF4G1, BUD23, and RNMT in LUAD was significantly different in the pathological stage N. The expression of EIF3D in LUAD was significantly different in the pathological stage M0/M1. Figure 5H shows the expression of m7G regulators in residues in the immune types C1 (wound healing), C3 (inflammation) LUSC, excluding the eight genes, namely, DCPS, GEMIN5, LARP1, NCBP1, SNUPN, NUDT16L, NUDT4, and MIF4GD. Furthermore, the clinical subgroup analysis displayed in Supplementary Figure S4B showed that the expression of AGO2, CYFIP2, NUDT1, NUDT16, and NUDT4 in LUSC was significantly different in the pathologic T phase. The expression of CYFIP1, GEMIN5, LARP1, NVBP1, NCBP3, NUDT16L1, and BUD23 in LUSC was significantly different in the pathological stage N. The expression of GEMIN5, DCP2, and RNMT in LUSC was significantly different in the pathological stage M0/M1.
Supplementary Figure S5A shows patients with high expression of WDR4, BUD23, METTL1, TRMT112, RAMAC, RNMT, EIF4G1, NCK1, MIF4GD, SLBP, EIF3D, SNUPN, NCBP2, LARP1, IFIT5, GEMIN5, AGO2, LSM1, DCPS, and NCBP1, with the exception of four negatively correlated genes (CYFIP1, CYFIP2, DCP2, NUDT16) and five unrelated genes (EIF4G3, IFIT5, NCBP3, NUDT16L1, NUDT4, MIF4GD). METTL1, AGO2, EIF4G3, NCBP2, NCBP3, NUDT1, NUDT16, NUDT4, NUDT5, EIF4G1, SLBP, BUD23, TRMT112, RAMAC, and RNMT were positively correlated with DNAss (p < 0, 05) in LUAD, while the expression of CYFIP1, CYFIP2, EIF4E2, GEMIN5, and IFIT5 in LUAD patients was negatively correlated with their DNAss score (p < 0.05). As shown in Supplementary Figure S5B, the expression of AGO2, WDR4, BUD23, METTL1, TRMT112, RAMAC, RNMT, SLBP, MIF4GD, EIF4G1, NUDT1, NUDT16L1, NUDT5, DCPS, EIF3D, GEMIN5, LARP1, LSM1, NCBP1, NCBP2, and SNUPN in LUSC patients was significantly positively associated with the RNAss score in LUSC (p < 0.05), with the exception of four negatively correlated genes (CYFIP2, EIF4G3, IFIT5, NUDT4) and six uncorrelated genes (CYFIP1, EIF4E2, NCBP3, DCP2, NUDT16, NCK1). Expression of WDR4, BUD23, METTL1, TRMT112, RAMAC, RNMT, AGO2, EIF3D, LSM1, NCBP2, NCBP3, SNUPN, NUDT1, NUDT16L1, EIF4G1, and MIF4GD were positively correlated with DNAss scores (p < 0.05) in LUSC, while the expression of CYFIP2, IFIT5, LARP1, and DCP2 were negatively correlated with the DNAss score in LUSC patients (p < 0.05). The expression of CYFIP1, CYFIP2, IFIT5, DCP2, NUDT4, and NCK1 in LUAD patients correlated positively with LUAD microenvironment scores (p < 0.05). However, the expression of BUD23, METTL1, TRMT112, RAMAC, SLBP, WDR4, DCPS, EIF3D, LARP1, LSM1, NCBP2, NCBP3, SNUPN, NUDT1, NUDT16L1, NUDT5, and EIF4G1 were negatively correlated with the micro-environmental assessment scores in LUAD patients (p < 0.05) (Figure 5A). Expression of WDR4, BUD23, METTL1, TRMT112, RAMAC, RNMT, SLBP, MIF4GD, EIF4G1, NUDT5, NUDT16L1, NUDT1, SNUPN, NCBP1, NCBP2, NCBP3, LSM1, GEMIN5, EIF3D, and AGO2 were negatively associated with the microenvironment score (p < 0.05) in LUSC, while the expression of CYFIP2, EIF4E2, IFIT5, DCP2, and NUDT4 were positively associated with the immune score, stromal score and estimation score in the LUSC samples (p < 0.05) (Supplementary Figure S5B).
Using the cBioportal database, we examined the pan-cancer changes in METTL1 and WDR4 in the TCGA database. As shown in Figure 6A, the results reflected that the highest frequency of change in METTL1 was approximately 18% in sarcoma, while 5.5% in LUAD and 1% in LUSC. Among the different types of genetic alterations, amplification was the most common. Figure 6B shows that the highest alteration frequency in WDR4 was in endometrial carcinoma of the uterine body, about 4.9%, while it was 1.8% in LUAD and 1.82% in LUSC. Gene mutation was the most common type of alteration. The TMB radar plot of METTL1 in Figure 6C shows the significant positive correlation of METTL1 mutations with STAD, PRAD, LUSC, LUAD, LIHC, LGG, KIRC, KICH, HNSC, and BRCA, while there was a significant negative correlation with THCA. The METTL1-MSI radar plot in Figure 6D shows the significant positive correlation of MSI with STAD, THCA, PRAD, MESO, KIRP, KICH, HNSC, and BRCA, while there was a negative correlation with THCA. The TMB radar plot of WDR4 in Figure 6E shows significant positive correlation of WDR4 mutation with STAD, PRAD, LUSC, LUAD, LIHC, LGG, KIRC, KICH, HNSC, and BRCA, while there was a significant negative correlation with THCA. The WDR4-MSI radar plot in Figure 6F shows significant positive correlations of MSI with UVM, STAD, THCA, SARC, READ PRAD, MESO, KIRP, KICH, HNSC, DLBC, and BRCA, while there was negative correlation with READ and COAD.
FIGURE 6. Mutational landscape of METTL1 and WDR4 in cancer. (A) METTL1 mutation frequency in multiple TCGA pan-cancer studies according to the cBioPortal database. (B) WDR4 mutation frequency in several TCGA pan-cancer studies according to the cBioPortal database. (C) Radar plot visualizing the relationship between METTL1 expression and TMB. Radar plot visualizing the relationship between WDR4 expression and TMB. (D) Radar plot visualizing the relationship between METTL1 expression and MSI. Radar chart visualizing the relationship between WDR4 expression and MSI. (E) The relationship between METTL1 expression and immunoinhibitors in pan-cancer. (F) The relationship between WDR4 expression and immunoinhibitors in pan-cancer. (G) The expression levels of METTL1 were significantly different in four groups of IMvigor rather than GSE78220. (H) The expression levels of WDR4 were significantly different in three groups of GSE78220 rather than IMvigor.
To investigate the relationship between METTL1/WDR4 expression and immunotherapy response in pan-cancer, we first explored the correlation between the expression of METTL1 and WDR4 with the expression of immunoinhibitors in the TISIDB database (Figures 6E,F). CD274 programmed death protein ligand 1 (PD-L1, CD274, B7-H1) has been shown to play a role in the regulation of immune responses and peripheral tolerance, and play a critical role in the induction and maintenance of tolerance to autoimmunity (Fabrizio et al., 2018; Huang et al., 2021). As shown in Figures 6E,F, the expression levels of METTL1, WDR4, and CD274 was inconsistent across different tumors. METTL1 showed a negative correlation while WDR4 was more positively correlated overall. We next chose the IMvigor210 and GSE78220 cohort to explore the association of METTL1 and WDR4 levels with the outcome of different immunotherapy. In GSE78220, the clinical outcomes were divided into CR (Complete response), PR (Partial response), and PD (Progressive disease), and the expression level of WDR4 was statistically significant in the different groups. A lower expression level of WDR4 was associated with a positive immunotherapy response (p = 0.026) in Figure 6G. However, in the clinical groups in Imvigor, which were divided into the following four groups: CR, PD, PR, and SD (Stable disease), a higher expression level of METTL1 was associated with a positive immunotherapy response (p = 0.077) in Figure 6H. These results were consistent with the above findings.
Previously, m7G was thought to be a representative of the CAP structure at the 5′ end of most mammalian mRNAs, which kept it stable during genetic inheritance (Furuichi, 2015). Our study evaluated the differential expression of the m7G regulators and their association with the immune landscape, including the TME, immune subtypes, and stem cell scores in 33 cancer types from the TCGA pan-cancer dataset. Then, the findings were validated in NSCLC. The expression of the m7G regulators in the tumor and normal tissues were significantly different as shown in Supplementary Figure S1, which suggest the potential role of these differentially expressed genes in tumorigenesis. Many CAP-dependent translational initiation genes were associated with transcription initiation of mRNA, which reflects the high transcription requirements of tumor tissues.
m6A, a widely studied form of RNA methylation modification, is essential for regulating RNA transcription, processing and translation, which in turn affects cellular metabolic activities. Different m6A modification patterns are known to interact with the immune phenotypes of tumors, including immune rejection, immune activation, and immune inertness, thus, estimating the m6A pattern of a tumor might enable the characterization of TME infiltration and provide guidance for improved immunotherapy strategies (Du et al., 2021; Zhang et al., 2020b). In the present study, we analyzed the correlation between m6A and m7G regulators using a deconvolution algorithm and found that most of the genes in the two groups showed a positive correlation with each other. This suggests that some common factors between the two groups may work together in one pathway. For example, the altered expression of TRMT112 could affect the tumor suppressive role of WBSCR22 in pancreatic cancer (PC), leading to tumor evolution (Khan et al., 2022). van Tran et al. (2019) identified METTL5 as the enzyme responsible for 18S rRNA m6A modification and showed that it formed a heterodimeric complex with the known methyltransferase activator TRMT112, in order to be metabolically stable in cells. Therefore, we hypothesized that identifying and blocking some common regulators in both the methylation modification pathways might be more effective than individually blocking some of the key genes.
We next examined the expression of m7G regulators in different immune-infiltrating subtypes of TME and their correlation with different immune subtypes. Specifically, METTL1, WDR4, CYFIP2, NUDT5, EIF4G1, NCK1, MIF4GD, SLBP, IFIT5, and NUDT1 were found to be significantly associated with the immune subtypes (p ≤ 0.01). METTL1, WDR4, EIF3D, and NUDT1 were found to be associated with the more aggressive immune subtypes C1 and C2. However, CYFIP1 was significantly associated with the C6 subtype. We speculated that the increased expression of CYFIP1, METTL1, WDR4, EIF3D, and NUDT1 predicted poor prognosis, and CYFIP1 had stronger correlation to the TGF-β immune signature, and higher lymphocytic infiltration than the other regulators, with an even distribution of type I and type II T cells. Moreover, NCBP3 showed increased expression in the immune subtype C3, which indicated a better prognosis and longer survival, suggesting that it may have an inhibitory role in cancers. However, CYFIP2, EIF4G3, IFIT15, NCBP2, NUDT16, NUDT4, and MIF4GD showed the highest expression in the immunologically quiescent C5 subtype, which was associated with a better prognosis.
The interaction between tumor cells and stromal components forms a functionally complex TME (Schaaf et al., 2018; Hinshaw and Shevde, 2019). Cancer-associated fibroblasts (CAFs) are mainly distributed around blood vessels or in the fibrous interstitium around tumors, and secrete cytokines, ECM components and related enzyme molecules. CAFs expressing the fibroblast activation protein induces a pro-inflammatory and pro-angiogenic microenvironment, promotes proliferation and increases stemness characteristics in a variety of cancer cells (Mao et al., 2021; Peltier et al., 2022). There are a variety of immune infiltrating cells in the TME, among which CD8+ or cytotoxic T lymphocytes (CTL) play a tumor-killing function. Wu et al. (2022) demonstrated that NELFB in CD8+ T lymphocytes played an important role in antitumor immunity associated with TCF1, promoting TCF1-bound transcriptional enhancers and promoter chromatin accessibility. Generally, M1-type macrophages play pro-inflammatory and anti-tumor functions, but tumor-associated macrophages (TAMs) in the TME are M2-type. Xu et al. (2021a) elucidated the mechanism by which hypoxia and glioma affected autophagy and M2-like macrophage polarization through exosomes, thereby promoting the formation of an immunosuppressive microenvironment.
According to the immune estimation algorithm, m7G regulators also correlated differentially with immune cell infiltration in the TCGA 33 Pan-cancer atlas. We found that the m7G-related genes were mainly negatively correlated with the stroma scores (Supplementary Figure S3), of which CAFs were the major players. Since the expression of most of the m7G regulators were negatively associated with immune scores in the TCGA 33 Pan Cancer Atlas, we next investigated the correlation between the overall expression level and immune cells. After calculating the ssGSEA score representing the activity of 31 m7G-regulated genes in the pan-cancer cohort, we found that the ssGSEA score was negatively correlated with higher infiltration of some immune cells especially Mast cells, T cells CD4 memory resting, T cells CD8 and B cells, these cell types have been shown by multiple studies to hinder tumor progression and promote a hyperimmune state of the tumor microenvironment and affect patient outcomes (Lichterman and Reddy, 2021; Lohr et al., 2013; Zhang et al., 2019b; Fu and Jiang, 2018; Wouters and Nelson, 2018). These results suggested that in future studies, one must consider linking changes in individual genes and changes in overall regulatory genes to analyze the possible effects of m7G methylation, which may lead to more realistic results.
In general, we found that the m7G-related gene expressions were correlated with the levels of immune or stromal infiltration, tumor purity, and prognosis. A previous study reported that in T cell acute lymphoblastic leukemia, the epigenetic loss of NUDT16 mediated the activation of C-MYC and promoted tumor progression (Anadon et al., 2017). Notably, certain genes that were positively associated with immune infiltration were also involved in tumor progression. For example, IFIT5 was shown to promote tumor cell invasion and migration by inducing EMT and downregulating miR-99a in bladder cancer (Huang et al., 2019). Therefore, the selection of specific genes as immune-related markers with specific molecular functions should be carried out and tested in tumor signaling pathways in order not to isolate oneself from reality.
Cancer stem cells (CSC) promote cancer progression due to their ability to self-renew and to invade and mediate therapeutic resistance (Schonberg et al., 2014). The expression of most genes was positively associated with both DNAss and RNAss scores. But IFIT5 and CYFIP2 were significantly negatively correlated with DNAss in some tumors. Moreover, the dysregulated expression of m7G regulators were generally significantly associated with OS, DSS and PFI in patients from the TCGA dataset in this study (Supplementary Figure S3). Obviously, the prognosis of patients with the same tumor correlated with the expression of multiple regulators. The Cellminer drug sensitivity analysis confirmed the significant correlation of almost all m7G regulators with sensitivity towards several of the currently used anti-cancer agents. CYFIP1 is one of the components of the CYFIP1-EIF4E-FMR1 complex, which enables its binding to the mRNA cap, mediating translational repression (Santini et al., 2017). Elevated CYFIP1 expression is known to suppress the drug sensitivity to bendamustine, XK-469, etoposide, teniposide, valrubicin, epirubicin, imexon, pipobroman, melphalan, and mitoxantrone. However, CYFIP1 expression has been negatively correlated with patient prognosis in several cancer types (KIRC, KIRP, UCEC), suggesting the tumor repressive role of CYFIP1. Oguro-Ando et al. (2015) showed that the overexpression of CYFIP1 in mammals dysregulated the mTOR signaling pathway. After treatment with rapamycin, the morphological abnormalities of neurons arising due to CYFIP1 overexpression were rescued in mice. Quantitative studies have shown that patients with renal tumors (ccRCC) benefitted from treatment with mTOR inhibitors such as everolimus and temsirolimus (Voss et al., 2014; Dong et al., 2019). CYFIP1 overexpression may play an important role in cancers with a predominantly altered mTOR signaling pathway.
Recent studies have mainly focused on the METTL1 and WDR4 complex, which are known to play important role in the modification of tRNAs (Dai et al., 2021). METTL1 has been reported to be highly expressed in a variety of cancers and was associated with tumor initiation, metastasis and chemo-sensitivity. Moreover, some studies have shown that METTL1 was associated with the immunosuppressive tumor microenvironment and stemness indices, and that its expression reflected the sensitivity of immune checkpoint blockade (ICB) therapy (Ma et al., 2021; Tian et al., 2019; Dai et al., 2021; Liu et al., 2019; Gao et al., 2022). WDR4 is also involved in a variety of cellular functions, including signal transduction, cell cycle promotion, and apoptotic cell death process (Lin et al., 2018; Michaud et al., 2000). Some studies showed that the aberrant expression of WDR4 was observed in various malignant cancers and was significantly associated with the overall survival outcomes. The expression level of WDR4 is also strongly associated with tumor immunity, such as immune scores and tumor-infiltrating immune cells (Zeng et al., 2021). In our study, we found that the expression levels of METTL1 and WDR4 were correlated with immunoinhibitors in pan-cancer datasets. Using data sets from previous studies (IMvigor210 and GSE78220 cohort), we further verified that the expression levels of METTL1 and WDR4 were related to the efficacy of immunotherapy. TMB is a valid predictive marker for tumor immunotherapy, effectively identifies and distinguishes individuals that could benefit from immunotherapy (Chan et al., 2019). Our TMB and MSI analyses for METTL1 and WDR4 reflected the mutation frequency and proportion at the molecular level in different tumors and showed the correlation between mutation load and tumor progression. METTL1 could serve as a predictive biomarker for immunotherapy efficacy in BRCA, STAD, PRAD, and LUSC, while WDR4 had similar role in BRCA, THYM, STAD, and LGG. High mutation rates of METTL1 and WDR4 showed significant correlations in certain tumors, and the combination of the two biomarkers could be used to construct a precise model for predicting tumor progression and response to immunotherapy in BRCA, STAD, and LGG.
By examining the m7G regulators in NSCLC, we observed that the expression of these genes varied in different immune subtypes within the same cancer. Thus, we hypothesized that the tumor suppressive or promoting role of these genes were subtype-specific and subsequently confirmed it in further clinical subgroup analyses. In our study, high expression of WDR4, EIF4G1 and SLBP were significantly associated with a poor OS (p < 0.001) and increased tumor stem cell score in LUAD. Meanwhile, they were associated with lower immune scores, confirming the negative impact of their increased expression on patient survival. Similarly, in LUSC, higher expression of AGO2, EIF3D, and LSM1 were positively correlated with tumor stem cell score but negatively correlated with immune infiltration degrees, indicating a poor prognosis. TMB and CNV analysis were performed to investigate the possible mechanisms underlying the altered expression of the m7G regulators. We found that the TMB of m7G regulators was low in both LUAD and LUSC patients, and the predominant alteration was mutation followed by gene amplification. However, in terms of CNV, LUAD, and LUSC had significant different alterations. Through the combination of TMB and clinical subgroup analysis, we concluded that CNV was not the sole factor influencing the expression of m7G regulators in both LUAD and in LUSC.
In summary, based on our systematic analyses of m7G regulators, we demonstrated the correlation between the differential expression of m7G regulators and patient survival, cancer immune landscape and tumor microenvironment, and concluded that the altered expression of WDR4, METTTL1, NUDT1, IFIT5, and CYFIP2 were associated with poor prognosis in cancer patients. Excluding NCBP3, NUDT4, CYFIP2, SNUPN, EIF4E2, and BUD23, most m7G regulators were upregulated in the primary tumors, all of which were associated with at least two of the following traits: more aggressive immune subtype; lower degree of immune infiltration; and a poor survival. The increased expression of WDR4, METTTL1, NUDT1, and CYFIP2 enhanced the sensitivity of some anti-cancer drugs such as hydroxyurea, L-asparaginase, 5-fluoro-deoxy-uridine 10mer, and chelerythrine. Upregulated expression of the above genes was often associated with a poor prognosis, their increased expression seemed to improve the sensitivity of patients to specific drugs. The expression levels of METTL1 and WDR4 was associated with immunotherapy and they could serve as potential prognostic markers. Furthermore, an individual analysis of NSCLC patients revealed that multiple genes (validated METTTL1 and WDR4, as well as EIF4G1, SLBP, AGB2, EIF3D, and LSM1) might play important roles in NSCLC progression, which could serve as potential biomarkers for predicting patient prognosis and response to immunotherapy. However, the oncogenic or tumor suppressing role of the individual m7G regulators depends on their specific molecular functions, thus it would be necessary to investigate their individual functional role in different types of cancer.
Publicly available datasets were analyzed in this study. Thenames of the repository/repositories and accession number(s) can be found in the article/Supplementary Material.
The project was designed by C-MH, JT, and X-JZ. JT, X-JZ, and SX give administrative support. Provision of study materials or patients by QW, Y-MW, and S-XZ. Collection and assembly of data by C-MH, X-DZ, and S-XZ. Data analysis and interpretation was carried out by C-MH and X-DZ. All authors read and approved the final manuscript. C-MH, X-DZ, and S-XZ contributed equally to this work. X-JZ and JT are the corresponding authors.
This work was supported by Wu Jie ping Medical Foundation, Special Fund of Burning Rock Testing for Precision Diagnosis and Treatment of Thoracic and Abdominal Tumors (320.6750.18137) and Shanghai Shenkang hospital development center, Clinical science and technology innovation project, Quality control and clinical pathway of surgical diagnosis and treatment of pulmonary nodules (SHDC2015641).
The authors declare that the research was conducted in the absence of any commercial or financial relationships that could be construed as a potential conflict of interest.
All claims expressed in this article are solely those of the authors and do not necessarily represent those of their affiliated organizations, or those of the publisher, the editors and the reviewers. Any product that may be evaluated in this article, or claim that may be made by its manufacturer, is not guaranteed or endorsed by the publisher.
The Supplementary Material for this article can be found online at: https://www.frontiersin.org/articles/10.3389/fgene.2022.998147/full#supplementary-material
SUPPLEMENTARY FIGURE S1 | Boxplot of m7G regulators differential expression between cancer and adjacent normal tissues. The blue boxplots indicate the normal tissues. The red boxplots indicate the cancer tissues.
SUPPLEMENTARY FIGURE S2 | The forest map of PFI and DSS in 31 m7G-related genes across 33 TCGA cancer types. (A) The forest map of PFS hazard ratio of 31 m7G-related genes across 33 TCGA cancer types. (B) The forest map of DSS hazard ratio of 31 m7G-related genes across 33 TCGA cancer types. (C) Survival correlation analysis of m7G related regulators in DSS across multiple cancer types. (D) The correlation relationship among the expression of 31 m7G methylation regulators and tumor purity. (E) Correlation between the expression of 31 m7G methylation regulators and the stromal cell score.
SUPPLEMENTARY FIGURE S3 | Survival analysis of m7G related regulators across multiple cancer types. The red line in the photos indicates high expression and the blue line in the photos indicates low expression.
SUPPLEMENTARY FIGURE S4 | Correlation analysis between 31 m7G regulators expression and clinical characteristics in LUAD and LUSC, respectively.
SUPPLEMENTARY FIGURE S5 | Correlation analysis of m7G regulators and LUAD, LUSC microenvironment, and stem cell scores.(A) The correlation relationship between m7G methylation regulators expression and immune score, stromal cell score, stem cell score and immune microenvironment in LUAD. (B) The correlation relationship between m7G methylation regulators expression and immune score, stromal cell score, stem cell score, and immune microenvironment in LUSC.
Anadón, C., van Tetering, G., Ferreira, H. J., Moutinho, C., Martínez-Cardús, A., Villanueva, A., et al. (2017). Epigenetic loss of the RNA decapping enzyme NUDT16 mediates C-MYC activation in T-cell acute lymphoblastic leukemia. Leukemia 31 (7), 1622–1625. doi:10.1038/leu.2017.99
Barbieri, I., and Kouzarides, T. (2020). Role of RNA modifications in cancer. Nat. Rev. Cancer. 20 (6), 303–322. doi:10.1038/s41568-020-0253-2
Barbieri, I., Tzelepis, K., Pandolfini, L., Shi, J., Millán-Zambrano, G., Robson, S. C., et al. (2017). Promoter-bound METTL3 maintains myeloid leukaemia by m6A-dependent translation control. Nature 552 (7683), 126–131. doi:10.1038/nature24678
Boccaletto, P., Stefaniak, F., Ray, A., Cappannini, A., Mukherjee, S., Purta, E., et al. (2022). Modomics: A database of RNA modification pathways. 2021 update. Nucleic Acids Res. 50 (D1), D231–D235. doi:10.1093/nar/gkab1083
Chan, T. A., Yarchoan, M., Jaffee, E., Swanton, C., Quezada, S. A., Stenzinger, A., et al. (2019). Development of tumor mutation burden as an immunotherapy biomarker: Utility for the oncology clinic. Ann. Oncol. 30 (1), 44–56. doi:10.1093/annonc/mdy495
Dai, Z., Liu, H., Liao, J., Huang, C., Ren, X., Zhu, W., et al. (2021). N7-Methylguanosine tRNA modification enhances oncogenic mRNA translation and promotes intrahepatic cholangiocarcinoma progression. Mol. Cell 81 (16), 3339–3355. doi:10.1016/j.molcel.2021.07.003
Dong, Y., Eskandari, R., Ray, C., Granlund, K. L., Santos-Cunha, L. D., Miloushev, V. Z., et al. (2019). Hyperpolarized MRI visualizes warburg effects and predicts treatment response to mTOR inhibitors in Patient-Derived ccRCC xenograft models. Cancer Res. 79 (1), 242–250. doi:10.1158/0008-5472.CAN-18-2231
Du, A., Wu, X., Gao, Y., Jiang, B., Wang, J., Zhang, P., et al. (2021). M6A Regulator-Mediated methylation modification patterns and tumor microenvironment infiltration characterization in acute myeloid leukemia. Front. Immunol. 12, 789914. doi:10.3389/fimmu.2021.789914
Enroth, C., Poulsen, L. D., Iversen, S., Kirpekar, F., Albrechtsen, A., and Vinther, J. (2019). Detection of internal N7-methylguanosine (m7G) RNA modifications by mutational profiling sequencing. Nucleic Acids Res. 47 (20), e126. doi:10.1093/nar/gkz736
Fabrizio, F. P., Trombetta, D., Rossi, A., Sparaneo, A., Castellana, S., and Muscarella, L. A. (2018). Gene code CD274/PD-L1: From molecular basis toward cancer immunotherapy. Ther. Adv. Med. Oncol. 10, 175883591881559. doi:10.1177/1758835918815598
Frye, M., Harada, B. T., Behm, M., and He, C. (2018). RNA modifications modulate gene expression during development. Science 361 (6409), 1346–1349. doi:10.1126/science.aau1646
Fu, C., and Jiang, A. (2018). Dendritic cells and CD8 t cell immunity in tumor microenvironment. Front. Immunol. 9, 3059. doi:10.3389/fimmu.2018.03059
Furuichi, Y. (2015). Discovery of m7G-cap in eukaryotic mRNAs. Proc. Jpn. Acad. Ser. B Phys. Biol. Sci. 91 (8), 394–409. doi:10.2183/pjab.91.394
Galloway, A., and Cowling, V. H. (2019). MRNA cap regulation in mammalian cell function and fate. Biochimica Biophysica Acta (BBA) - Gene Regul. Mech. 1862 (3), 270–279. doi:10.1016/j.bbagrm.2018.09.011
Gao, Z., Xu, J., Zhang, Z., Fan, Y., Xue, H., Guo, X., et al. (2022). A comprehensive analysis of METTL1 to immunity and stemness in Pan-Cancer. Front. Immunol. 13, 795240. doi:10.3389/fimmu.2022.795240
He, C. (2010). Grand challenge commentary: RNA epigenetics? Nat. Chem. Biol. 6 (12), 863–865. doi:10.1038/nchembio.482
Hinshaw, D. C., and Shevde, L. A. (2019). The tumor microenvironment innately modulates cancer progression. Cancer Res. 79 (18), 4557–4566. doi:10.1158/0008-5472.CAN-18-3962
Huang, J., Lo, U. G., Wu, S., Wang, B., Pong, R. C., Lai, C. H., et al. (2019). The roles and mechanism of IFIT5 in bladder cancer epithelial-mesenchymal transition and progression. Cell Death Dis. 10 (6), 437. doi:10.1038/s41419-019-1669-z
Huang, R., Murugesan, K., Montesion, M., Pavlick, D. C., Mata, D. A., Hiemenz, M. C., et al. (2021). Pan-cancer landscape of CD274 (PD-L1) copy number changes in 244 584 patient samples and the correlation with PD-L1 protein expression. J. Immunother. Cancer 9 (5), e002680. doi:10.1136/jitc-2021-002680
Hugo, W., Zaretsky, J. M., Sun, L., Song, C., Moreno, B. H., Hu-Lieskovan, S., et al. (2016). Genomic and transcriptomic features of response to Anti-PD-1 therapy in metastatic melanoma. Cell 165 (1), 35–44. doi:10.1016/j.cell.2016.02.065
Kelen, K., Beyaert, R., Inzé, D., and Veylder, L. (2009). Translational control of eukaryotic gene expression. Crit. Rev. Biochem. Mol. Biol. 44 (4), 143–168. doi:10.1080/10409230902882090
Khan, A. A., Huang, H., Zhao, Y., Li, H., Pan, R., Wang, S., et al. (2022). WBSCR22 and TRMT112 synergistically suppress cell proliferation, invasion and tumorigenesis in pancreatic cancer via transcriptional regulation of ISG15. Int. J. Oncol. 60 (3). doi:10.3892/ijo.2022.5314
Lee, A. S., Kranzusch, P. J., Doudna, J. A., and Cate, J. H. (2016). EIF3d is an mRNA cap-binding protein that is required for specialized translation initiation. Nature 536 (7614), 96–99. doi:10.1038/nature18954
Li, K., Luo, H., Huang, L., Luo, H., and Zhu, X. (2020). Microsatellite instability: A review of what the oncologist should know. Cancer Cell Int. 20, 16. doi:10.1186/s12935-019-1091-8
Li, R., Yin, Y. H., Ji, X. L., Liu, X., Li, J. P., and Qu, Y. Q. (2021). Pan-Cancer prognostic, immunity, stemness, and anticancer drug sensitivity characterization of N6-Methyladenosine RNA modification regulators in human cancers. Front. Mol. Biosci. 8, 644620. doi:10.3389/fmolb.2021.644620
Lichterman, J. N., and Reddy, S. M. (2021). Mast cells: A new frontier for cancer immunotherapy. Cells 10 (6), 1270. doi:10.3390/cells10061270
Lin, J. C., Hsu, M., and Tarn, W. Y. (2007). Cell stress modulates the function of splicing regulatory protein RBM4 in translation control. Proc. Natl. Acad. Sci. U.S.A. 104 (7), 2235–2240. doi:10.1073/pnas.0611015104
Lin, S., Liu, Q., Lelyveld, V. S., Choe, J., Szostak, J. W., and Gregory, R. I. (2018). Mettl1/Wdr4-Mediated m7G tRNA methylome is required for normal mRNA translation and embryonic stem cell self-renewal and differentiation. Mol. Cell 71 (2), 244–255. doi:10.1016/j.molcel.2018.06.001
Liu, Y., Yang, C., Zhao, Y., Chi, Q., Wang, Z., and Sun, B. (2019). Overexpressed methyltransferase-like 1 (METTL1) increased chemosensitivity of colon cancer cells to cisplatin by regulating miR-149-3p/S100A4/p53 axis. Aging 11 (24), 12328–12344. doi:10.18632/aging.102575
Lohr, M., Edlund, K., Botling, J., Hammad, S., Hellwig, B., Othman, A., et al. (2013). The prognostic relevance of tumour-infiltrating plasma cells and immunoglobulin kappa C indicates an important role of the humoral immune response in non-small cell lung cancer. Cancer Lett. 333 (2), 222–228. doi:10.1016/j.canlet.2013.01.036
Ma, J., Han, H., Huang, Y., Yang, C., Zheng, S., Cai, T., et al. (2021). METTL1/WDR4-mediated m7G tRNA modifications and m7G codon usage promote mRNA translation and lung cancer progression. Mol. Ther. 29 (12), 3422–3435. doi:10.1016/j.ymthe.2021.08.005
Malbec, L., Zhang, T., Chen, Y. S., Zhang, Y., Sun, B. F., Shi, B. Y., et al. (2019). Dynamic methylome of internal mRNA N7-methylguanosine and its regulatory role in translation. Cell Res. 29 (11), 927–941. doi:10.1038/s41422-019-0230-z
Mao, X., Xu, J., Wang, W., Liang, C., Hua, J., Liu, J., et al. (2021). Crosstalk between cancer-associated fibroblasts and immune cells in the tumor microenvironment: New findings and future perspectives. Mol. Cancer. 20 (1), 131. doi:10.1186/s12943-021-01428-1
Mariathasan, S., Turley, S. J., Nickles, D., Castiglioni, A., Yuen, K., Wang, Y., et al. (2018). TGFβ attenuates tumour response to PD-L1 blockade by contributing to exclusion of T cells. Nature 554 (7693), 544–548. doi:10.1038/nature25501
Michaud, J., Kudoh, J., Berry, A., Bonne-Tamir, B., Lalioti, M. D., Rossier, C., et al. (2000). Isolation and characterization of a human chromosome 21q22.3 gene (WDR4) and its mouse homologue that code for a WD-repeat protein. Genomics 68 (1), 71–79. doi:10.1006/geno.2000.6258
Newman, A. M., Liu, C. L., Green, M. R., Gentles, A. J., Feng, W., Xu, Y., et al. (2015). Robust enumeration of cell subsets from tissue expression profiles. Nat. Methods. 12 (5), 453–457. doi:10.1038/nmeth.3337
Oguro-Ando, A., Rosensweig, C., Herman, E., Nishimura, Y., Werling, D., Bill, B. R., et al. (2015). Increased CYFIP1 dosage alters cellular and dendritic morphology and dysregulates mTOR. Mol. Psychiatry 20 (9), 1069–1078. doi:10.1038/mp.2014.124
Orellana, E. A., Liu, Q., Yankova, E., Pirouz, M., De Braekeleer, E., Zhang, W., et al. (2021). METTL1-mediated m7G modification of Arg-TCT tRNA drives oncogenic transformation. Mol. Cell 81 (16), 3323–3338. doi:10.1016/j.molcel.2021.06.031
Pandolfini, L., Barbieri, I., Bannister, A. J., Hendrick, A., Andrews, B., Webster, N., et al. (2019). METTL1 promotes let-7 MicroRNA processing via m7G methylation. Mol. Cell 74 (6), 1278–1290. doi:10.1016/j.molcel.2019.03.040
Peltier, A., Seban, R. D., Buvat, I., Bidard, F. C., and Mechta-Grigoriou, F. (2022). Fibroblast heterogeneity in solid tumors: From single cell analysis to whole-body imaging. Seminars Cancer Biol. [Epub ahead of print]. doi:10.1016/j.semcancer.2022.04.008
Roundtree, I. A., Evans, M. E., Pan, T., and He, C. (2017). Dynamic RNA modifications in gene expression regulation. Cell 169 (7), 1187–1200. doi:10.1016/j.cell.2017.05.045
Ru, B., Wong, C. N., Tong, Y., Zhong, J. Y., Zhong, S., Wu, W. C., et al. (2019). Tisidb: An integrated repository portal for tumor-immune system interactions. Bioinformatics 35 (20), 4200–4202. doi:10.1093/bioinformatics/btz210
Santini, E., Huynh, T. N., Longo, F., Koo, S. Y., Mojica, E., D’Andrea, L., et al. (2017). Reducing eIF4E-eIF4G interactions restores the balance between protein synthesis and actin dynamics in fragile X syndrome model mice. Sci. Signal. 10 (504). doi:10.1126/scisignal.aan0665
Schaaf, M. B., Garg, A. D., and Agostinis, P. (2018). Defining the role of the tumor vasculature in antitumor immunity and immunotherapy. Cell Death Dis. 9 (2), 115. doi:10.1038/s41419-017-0061-0
Schonberg, D. L., Lubelski, D., Miller, T. E., and Rich, J. N. (2014). Brain tumor stem cells: Molecular characteristics and their impact on therapy. Mol. Aspects Med. 39, 82–101. doi:10.1016/j.mam.2013.06.004
Shen, S., Wang, G., Zhang, R., Zhao, Y., Yu, H., Wei, Y., et al. (2019). Development and validation of an immune gene-set based Prognostic signature in ovarian cancer. EBioMedicine 40, 318–326. doi:10.1016/j.ebiom.2018.12.054
Siegel, R. L., Miller, K. D., Fuchs, H. E., and Jemal, A. (2022). Cancer statistics, 2022. CA A Cancer J. Clin. 72 (1), 7–33. doi:10.3322/caac.21708
Sung, H., Ferlay, J., Siegel, R. L., Laversanne, M., Soerjomataram, I., Jemal, A., et al. (2021). Global cancer statistics 2020: GLOBOCAN estimates of incidence and mortality worldwide for 36 cancers in 185 countries. CA A Cancer J. Clin. 71 (3), 209–249. doi:10.3322/caac.21660
Tian, Q. H., Zhang, M. F., Zeng, J. S., Luo, R. G., Wen, Y., Chen, J., et al. (2019). METTL1 overexpression is correlated with poor prognosis and promotes hepatocellular carcinoma via PTEN. J. Mol. Med. 97 (11), 1535–1545. doi:10.1007/s00109-019-01830-9
van Tran, N., Ernst, F., Hawley, B. R., Zorbas, C., Ulryck, N., Hackert, P., et al. (2019). The human 18S rRNA m6A methyltransferase METTL5 is stabilized by TRMT112. Nucleic Acids Res. 47 (15), 7719–7733. doi:10.1093/nar/gkz619
Voss, M. H., Bastos, D. A., Karlo, C. A., Ajeti, A., Hakimi, A. A., Feldman, D. R., et al. (2014). Treatment outcome with mTOR inhibitors for metastatic renal cell carcinoma with nonclear and sarcomatoid histologies. Ann. Oncol. 25 (3), 663–668. doi:10.1093/annonc/mdt578
Wang, Y. T., Chen, J., Chang, C. W., Jen, J., Huang, T. Y., Chen, C. M., et al. (2017). Ubiquitination of tumor suppressor PML regulates prometastatic and immunosuppressive tumor microenvironment. J. Clin. Invest. 127 (8), 2982–2997. doi:10.1172/JCI89957
Wouters, M., and Nelson, B. H. (2018). Prognostic significance of Tumor-Infiltrating b cells and plasma cells in human cancer. Clin. Cancer Res. 24 (24), 6125–6135. doi:10.1158/1078-0432.CCR-18-1481
Wu, B., Zhang, X., Chiang, H. C., Pan, H., Yuan, B., Mitra, P., et al. (2022). RNA polymerase II pausing factor NELF in CD8+ T cells promotes antitumor immunity. Nat. Commun. 13 (1), 2155. doi:10.1038/s41467-022-29869-2
Wu, S., and Wagner, G. (2021). Deep computational analysis details dysregulation of eukaryotic translation initiation complex eIF4F in human cancers. Cell Syst. 12 (9), 907–923. doi:10.1016/j.cels.2021.07.002
Xu, J., Gao, Z., Liu, K., Fan, Y., Zhang, Z., Xue, H., et al. (2021a). The non-N6-methyladenosine epitranscriptome patterns and characteristics of tumor microenvironment infiltration and mesenchymal transition in glioblastoma. Front. Immunol. 12, 809808. doi:10.3389/fimmu.2021.809808
Xu, J., Zhang, J., Zhang, Z., Gao, Z., Qi, Y., Qiu, W., et al. (2021b). Hypoxic glioma-derived exosomes promote M2-like macrophage polarization by enhancing autophagy induction. Cell Death Dis. 12 (4), 373. doi:10.1038/s41419-021-03664-1
Yu, S., Wu, Y., Li, C., Qu, Z., Lou, G., Guo, X., et al. (2020). Comprehensive analysis of the SLC16A gene family in pancreatic cancer via integrated bioinformatics. Sci. Rep. 10 (1), 7315. doi:10.1038/s41598-020-64356-y
Zeng, H., Xu, S., Xia, E., Hirachan, S., Bhandari, A., and Shen, Y. (2021). Aberrant expression of WDR4 affects the clinical significance of cancer immunity in pan-cancer. Aging 13 (14), 18360–18375. doi:10.18632/aging.203284
Zhang, B., Wu, Q., Li, B., Wang, D., Wang, L., and Zhou, Y. L. (2020a). m6A regulator-mediated methylation modification patterns and tumor microenvironment infiltration characterization in gastric cancer. Mol. Cancer. 19 (1), 53. doi:10.1186/s12943-020-01170-0
Zhang, C., Chen, Y., Sun, B., Wang, L., Yang, Y., Ma, D., et al. (2017). m6A modulates haematopoietic stem and progenitor cell specification. Nature 549 (7671), 273–276. doi:10.1038/nature23883
Zhang, F., Xiang, S., Cao, Y., Li, M., Ma, Q., Liang, H., et al. (2021). Correction: EIF3D promotes gallbladder cancer development by stabilizing GRK2 kinase and activating PI3K-akt signaling pathway. Cell Death Dis. 13 (1), 19. doi:10.1038/s41419-021-04465-2
Zhang, L. S., Liu, C., Ma, H., Dai, Q., Sun, H. L., Luo, G., et al. (2019a). Transcriptome-wide mapping of internal N7-methylguanosine methylome in mammalian mRNA. Mol. Cell 74 (6), 1304–1316. doi:10.1016/j.molcel.2019.03.036
Zhang, S. C., Hu, Z. Q., Long, J. H., Zhu, G. M., Wang, Y., Jia, Y., et al. (2019b). Clinical implications of Tumor-Infiltrating immune cells in breast cancer. J. Cancer 10 (24), 6175–6184. doi:10.7150/jca.35901
Zhang, X., Shao, S., and Li, L. (2020b). Characterization of class-3 semaphorin receptors, neuropilins and plexins, as therapeutic targets in a Pan-Cancer study. Cancers 12 (7), 1816. doi:10.3390/cancers12071816
Zhao, B. S., Roundtree, I. A., and He, C. (2017a). Post-transcriptional gene regulation by mRNA modifications. Nat. Rev. Mol. Cell Biol. 18 (1), 31–42. doi:10.1038/nrm.2016.132
Keywords: pan-cancer analysis, m7G regulators, tumor microenevironment, survival, immune score, drug sensitivity
Citation: He C-M, Zhang X-D, Zhu S-X, Zheng J-J, Wang Y-M, Wang Q, Yin H, Fu Y-J, Xue S, Tang J and Zhao X-J (2022) Integrative pan-cancer analysis and clinical characterization of the N7-methylguanosine (m7G) RNA modification regulators in human cancers. Front. Genet. 13:998147. doi: 10.3389/fgene.2022.998147
Received: 19 July 2022; Accepted: 25 August 2022;
Published: 26 September 2022.
Edited by:
Ann-Kristin Östlund Farrants, Stockholm University, SwedenReviewed by:
Jin Chen, Wenzhou Medical University, ChinaCopyright © 2022 He, Zhang, Zhu, Zheng, Wang, Wang, Yin, Fu, Xue, Tang and Zhao. This is an open-access article distributed under the terms of the Creative Commons Attribution License (CC BY). The use, distribution or reproduction in other forums is permitted, provided the original author(s) and the copyright owner(s) are credited and that the original publication in this journal is cited, in accordance with accepted academic practice. No use, distribution or reproduction is permitted which does not comply with these terms.
*Correspondence: Jian Tang, tangjian1108@yeah.net; Xiao-Jing Zhao, drzhaoxiaojing@163.com
†These authors have contributed equally to this work
Disclaimer: All claims expressed in this article are solely those of the authors and do not necessarily represent those of their affiliated organizations, or those of the publisher, the editors and the reviewers. Any product that may be evaluated in this article or claim that may be made by its manufacturer is not guaranteed or endorsed by the publisher.
Research integrity at Frontiers
Learn more about the work of our research integrity team to safeguard the quality of each article we publish.