- 1Institute for Community Medicine, University Medicine Greifswald, Greifswald, Germany
- 2Department of Psychiatry and Psychotherapy, University Medicine Greifswald, Greifswald, Germany
- 3DZHK (German Center for Cardiovascular Research), Partner Site Greifswald, Greifswald, Germany
- 4German Center for Neurodegenerative Diseases DZNE, Site Rostock/Greifswald, Greifswald, Germany
A decreased estimated glomerular filtration rate (eGFR) leading to chronic kidney disease is a significant public health problem. Kidney function is a heritable trait, and recent application of genome-wide association studies (GWAS) successfully identified multiple eGFR-associated genetic loci. To increase statistical power for detecting independent associations in GWAS loci, we improved our recently developed quasi-adaptive method estimating SNP-specific alpha levels for the conditional analysis, and applied it to the GWAS meta-analysis results of eGFR among 783,978 European-ancestry individuals. Among known eGFR loci, we revealed 19 new independent association signals that were subsequently replicated in the United Kingdom Biobank (n = 408,608). These associations have remained undetected by conditional analysis using the established conservative genome-wide significance level of 5 × 10–8. Functional characterization of known index SNPs and novel independent signals using colocalization of conditional eGFR association results and gene expression in cis across 51 human tissues identified two potentially causal genes across kidney tissues: TSPAN33 and TFDP2, and three candidate genes across other tissues: SLC22A2, LRP2, and CDKN1C. These colocalizations were not identified in the original GWAS. By applying our improved quasi-adaptive method, we successfully identified additional genetic variants associated with eGFR. Considering these signals in colocalization analyses can increase the precision of revealing potentially functional genes of GWAS loci.
Introduction
Glomerular filtration rate estimated from serum creatinine (eGFR) is used to quantify kidney function and define chronic kidney disease (CKD). CKD defined by low eGFR <60 ml/min/1.73 m2 is strongly associated with an increased risk of major adverse clinical outcomes such as end-stage kidney disease (ESKD), cardiovascular (CV) outcomes, and mortality (Go et al., 2004; Chronic Kidney Disease PrognosisMatsushita et al., 2010; Hemmelgarn et al., 2010; Astor et al., 2011; Bello et al., 2011; Gansevoort et al., 2011; Gansevoort et al., 2013; Weiner et al., 2014; Matsushita et al., 2015). A better understanding of the biological mechanisms underlying kidney function is a prerequisite for initiating targeted treatments and reducing patient mortality, comorbidity, and associated healthcare costs. eGFR is a heritable trait with estimated h2 = 39%, and recent application of genome-wide association studies (GWAS) successfully identified multiple eGFR-associated genetic loci (Okada et al., 2012; Pattaro et al., 2012; Mahajan et al., 2016; Pattaro et al., 2016; Hishida et al., 2018; Kanai et al., 2018; Lee et al., 2018; Wuttke et al., 2019). Allelic heterogeneity within a GWAS locus is a common characteristic of complex traits and conditional analyses successfully identified multiple independent associations with eGFR. For instance, Gorski et al. (2017) (Gorski et al., 2017) detected 57 independent signals among the 49 loci. Morris et al. (2019) (Morris et al., 2019) delineated 127 distinct signals across the 93 loci. Hellwege et al. (2019) (Hellwege et al., 2019) discovered 18 independent signals at 15 loci, and Wuttke et al. (2019) (Wuttke et al., 2019) identified 253 independent SNPs at 228 loci explaining 7.3% of the eGFR variation.
To identify an independent signal, the SNPs of a locus are conditioned by the known significant associations. In case individual genotypes of a sample are available, the genotypes of known signals are added as covariates to the association model. Alternatively, these conditional associations can be approximated by using summary statistics and an appropriate linkage disequilibrium (LD) panel. Usually, the established genome-wide significance level of 5 × 10–8 was applied as a significance threshold for the conditional analysis, which is also the significance level for the primary GWAS. Since the conditional analysis is applied on a specific genomic region and not on a genome-wide scale, 5 × 10–8 is too conservative and implies a loss of power. In Ghasemi et al. (2021) (Ghasemi et al., 2021), we developed a quasi-adaptive method to determine SNP-specific significance levels in conditional analysis.
Although GWAS have discovered multiple eGFR-associated loci, the underlying genes that influence genetic associations have often remained unknown. Integration of GWAS signals and expression quantitative trait loci (eQTL) studies (Nica and Dermitzakis, 2013) to estimate the relation between gene expression of nearby genes and eGFR, termed colocalization (Giambartolomei et al., 2014), allows the identification of candidate genes and improves the functional interpretation of GWAS results. For instance, FGF5, CDKL5, TPSAN33, and METTL10 colocalized with the eGFR-associated loci in kidney-specific tissues (Graham et al., 2019), and Wuttke et al. (2019) (Wuttke et al., 2019) detected 17 underlying genes expressed in kidney tissues including UMOD, KNG1, and FGF5.
Here, we improved and applied our quasi-adaptive method to the publically available GWAS meta-analysis results of 783,978 European-ancestry individuals (Wuttke et al., 2019) of the CKDGen Consortium to uncover additional independent signals for eGFR. Replication of the identified novel independent signals was conducted using individual-level participant data of the United Kingdom Biobank (UKBB) (Bycroft et al., 2018). The UKBB was not included in the primary GWAS meta-analysis, and thus represents an independent dataset for replication. We run colocalization analyses based on associations with eGFR and with gene expression (eQTLs) in cis across 49 human tissues included in the Genotype-Tissue Expression (GTEx) project v827, as well as the microdissected human glomerular and tubulo-interstitial kidney portions from 187 individuals from the NEPTUNE study (Gillies et al., 2018). Since the presence of multiple independent signals within a GWAS locus reduces power of colocalization, we provided the colocalization analyses with conditional eGFR-association analysis and eQTL to detect potential causal genes and compared these results to the unconditional approach. Our colocalization analyses used the latest version of GTEx-v8 compared to the GTEx-v6 in the previous report of eGFR (Wuttke et al., 2019).
The emerging list of novel eGFR-associated variants and genes influencing kidney disease etiology facilitate CKD targeted treatment and prevention.
Methods
Additional independent eGFR-associated signals identification by quasi-adaptive method
We obtained the CKDGen Consortium 2019 eGFR-association GWAS meta-analysis results for European-ancestry (Wuttke et al., 2019) from https://ckdgen.imbi.uni-freiburg.de. The downloaded file included chromosome, position (b37), SNP rsid, effect allele, non-effect allele, effect allele frequency, beta, standard error, p-value, and sample size for each variant. Wuttke et al. (2019) (Wuttke et al., 2019) identified 253 independent genome-wide-significant eGFR-associated SNPs through approximate conditional analyses implemented in GCTA (Yang et al., 2011) (GCTA COJO Slct algorithm) across 228 European-ancestry-specific and replicated loci. To identify additional independent eGFR-associated secondary signals, we applied our quasi-adaptive method to the aforementioned GWAS meta-analysis with 8,885,712 genetic variants and 783,978 individuals. The method incorporated LD structure from individual-level genotype data of 15,000 randomly selected European-ancestry participants of the UKBB (Bycroft et al., 2018). The selected UKBB LD reference sample underwent the same data preparation procedure as described in (Wuttke et al., 2019) and (Teumer et al., 2019), except for the minor allele frequency (MAF) cut-off. We excluded SNPs with a MAF <0.0001. The final dataset for estimating the LD structure included 13,558 unrelated European-ancestry individuals and 36, 228, 692 genetic variants. We used the published 228 replicated index SNPs (i.e., variants with the smallest p-value of a locus) as the basis for applying our method (Wuttke et al., 2019). A one megabase window around the index SNPs was considered as primary loci. Overlapping loci at which two adjacent index SNPs were less than one megabase apart or with pairwise correlation
The pre-weight
The quasi-adaptive method is applied on
SNP(
(Ghasemi et al., 2021) showed that Equation 1 has the overall best power in detecting secondary signals while controlling the family-wise error rate (FWER) at the
Improved quasi-adaptive method to identify multiple independent eGFR-associated signals
The original quasi-adaptive method was developed to determine one independent signal (secondary signal) with the smallest conditional p-value smaller than the correspondingly assigned
The improved method is an iterative process that is subsequently performed to detect higher-order independent signals (applied to loci with confirmed independent signals from the previous steps) until no additional independent signals are found. Finding higher-order independent signals keeps the FWER at the
Due to the complexity of the LD structure of the major histocompatibility complex (MHC) region, this region was excluded from the search for independent signals as also in the main GWAS (Wuttke et al., 2019).
Replication of the results in the UK biobank dataset
The novel independent eGFR-associated signals were tested for replication by conditional association analyses using the individual-level data of the UKBB (Bycroft et al., 2018) cohort. This cohort was not included in the initial GWAS of eGFR, and thus represents an independent dataset for replication. The phenotype definition, quality control, and analyses were performed using the same methods and scripts of the main GWAS (Teumer et al., 2019; Wuttke et al., 2019). As independent signals were identified from samples of European ancestry, conditional analyses were restricted to 408,608 UKBB participants of European ancestry with approximately 19 million autosomal SNPs that met the inclusion criteria of MAF ≥0.001 and imputation quality score > 0.3. For replication of each category of independent signals (secondary, tertiary, and beyond) across loci, a conditional analysis was conducted by including sex- and age-adjusted residual of log (eGFR), the first 15 genetic principal components, and the allele dosages of all corresponding conditioned SNPs as covariates in a mixed-model association method as implemented in BOLT-LMM, v2.3.2 (Loh et al., 2005). Within each locus, conditional analysis was performed for replication of an identified independent signal by conditioning on a known index SNP and (if present) on other known or replicated independent signals identified before the corresponding independent signal. Of note, non-replicated signals identified before the independent signal under investigation were excluded from the conditional analysis. Supplementary Table S2 shows the list of known index SNPs and known and novel independent signals with the list of covariates (SNPs) used for replication. Bonferroni correction of 0.05/9, 0.05/8, 0.05/6, 0.05/3, and 0.05, correcting for the number of tested SNPs per conditional analysis, was applied to assess the significance of the replication of secondary signals, tertiary signals, signals of fourth, signals of fifth, and signal of sixth, respectively.
Colocalization of eGFR signals with gene expression in cis
In the first instance, colocalization analyses were run for known index SNPs and novel independent signals using unconditional eGFR association analyses in the UKBB and expression quantitative trait (eQTL) studies (Nica and Dermitzakis, 2013). eQTL were quantified from 49 human tissues included in the GTEx project v8 release (Aguet et al., 2019), and the microdissected human glomerular and tubulointerstitial kidney portions from 187 individuals from the NEPTUNE study (Gillies et al., 2018). For colocalization, the effect alleles for GWAS and eQTLs were harmonized, and tissue gene pairs with eQTL data were identified within
Secondly, we re-run the colocalization analyses using conditional eGFR association analyses and the eQTL studies. Conditional analysis was performed for a known index SNP by adjusting for all known and novel independent signals and for a novel independent signal by conditioning on a known index SNP and (if present) on other known or novel independent signals within the corresponding locus. Supplementary Table S2 shows the list of covariates (SNPs) used in the eGFR association. We defined a variant as a colocalized signal (same causal variant underlying both the GWAS and eQTL association) if the posterior probability (PP) of a variant was greater than 80%.
Results
Novel eGFR-associated multiple conditionally independent signals
To detect additional eGFR-associated independent signals, our method was applied on 190 loci derived from the GWAS meta-analysis (Wuttke et al., 2019) (Methods and Supplementary Table S1). Our method identified in total 87 independent signals, including 53 secondary signals (Supplementary Table S3), 20 tertiary signals (Supplementary Table S4), 10 signals of fourth (Supplementary Table S5), three signals of fifth (Supplementary Table S6), and one signal of sixth (Supplementary Table S7), of which 27 were novel (Table 1). Of note, all novel SNPs were secondary or higher-order signals. We have listed the differences between the previous analysis (Wuttke et al., 2019) and our analysis in Supplementary Tables S3-S7 in a column labeled “Known”. At a locus, an SNP detected by our method was considered known (yes) if it was exactly the independent signal or in high LD (
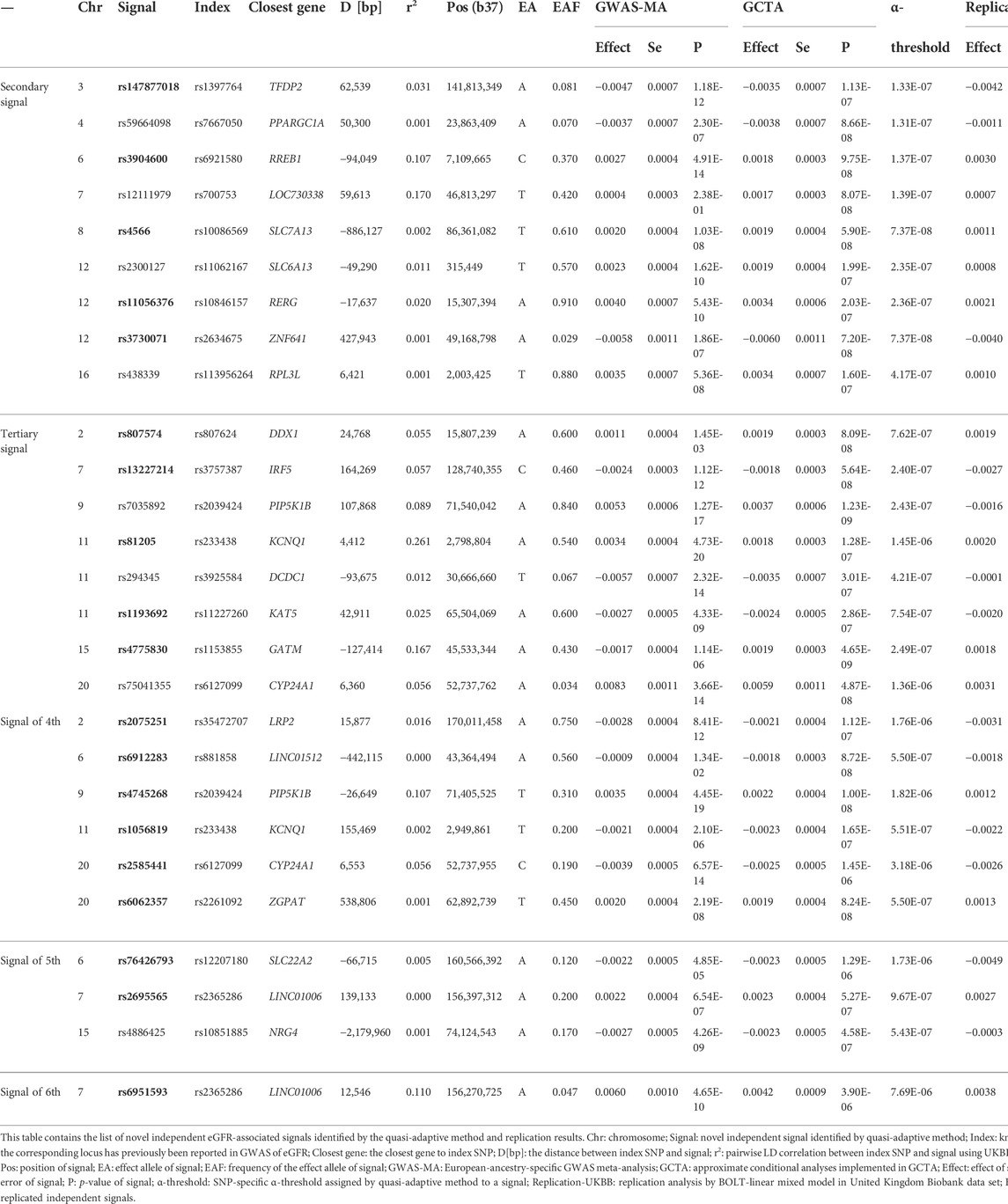
TABLE 1. Summary of novel independent eGFR-associated signals identified by quasi-adaptive method and replication results.
Replication of novel multiple independent signals in European-ancestry individuals
To assess the validity of our newly identified independent signals, we conducted conditional eGFR-association analyses using individual-level genotype data among 408,608 European-ancestry participants of the UKBB as independent replication (Methods). For 27 novel independent signals, we conducted 27 conditional analyses (Supplementary Table S2). In total, replication was achieved for 19 signals (Five secondary signals, five tertiary signals, six signals of fourth, two signals of fifth, and one signal of sixth) after applying multiple testing corrections (Methods, Table 1 and Figure 1A). Of note, seven of these signals achieved genome-wide significant conditional p-values, and additional four signals were nominally significant (p < 0.05) in the replication analysis. Effect estimates for the replicated signals showed a strong correlation (r2 = 0.937) with the discovery results (Figure 1B).
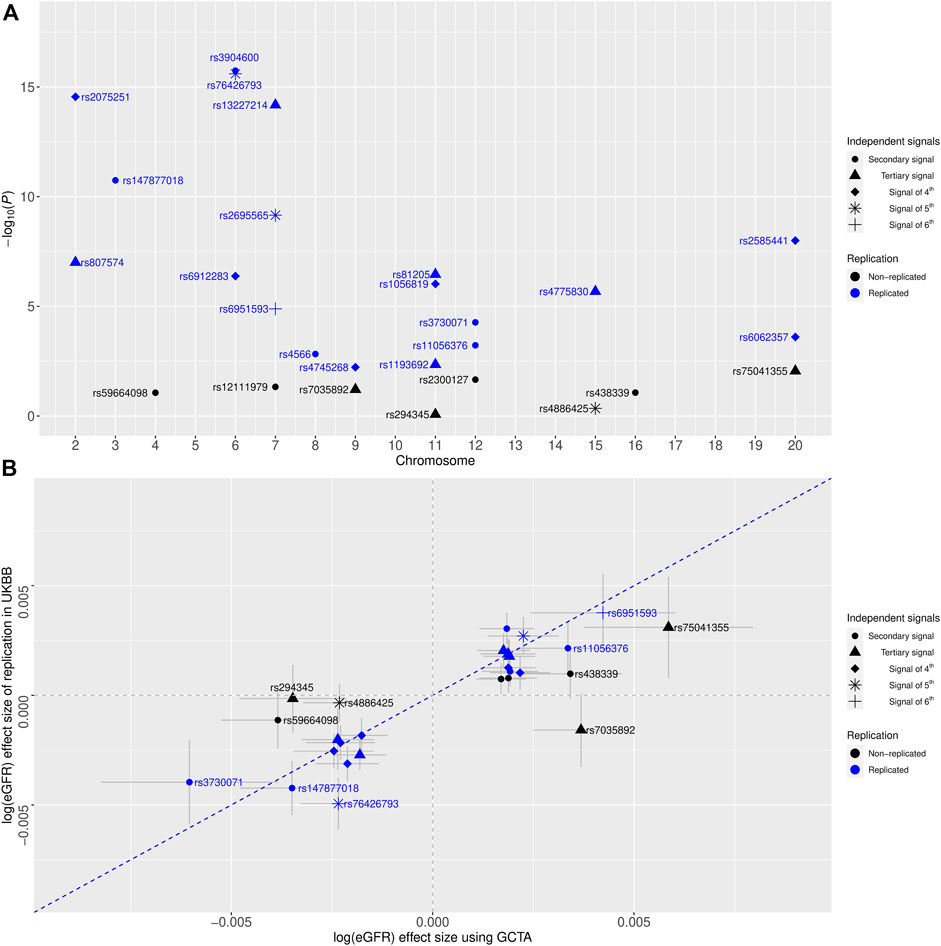
FIGURE 1. (A) Replication of eGFR-associated multiple independent signals identified by the quasi-adaptive method using the United Kingdom Biobank (UKBB) genotype data among European-ancestry individuals. The x-axis shows the chromosome number, and the y-axis is the
For better comparison, the regional association plots were generated for the unconditional associations and the conditional associations with the highlighted known index and the novel independent signal separately (Supplementary Figures S1-S57). Of note, the new independent signals rs3904600, rs13227214, rs81205, rs2075251, rs2695565, and rs6951593 (identified by the quasi-adaptive method based on the meta-analysis of the previous GWAS of eGFR (Wuttke et al., 2019)) showed smaller p-values in their unconditional analysis within the UKBB compared to their corresponding index SNP (Supplementary Figures S4, S19, S22, S31, S52, S55).
Colocalization with gene expression
Colocalization analyses were performed with eQTLs in cis across 51 tissues, including kidney cortex, glomerular, and tubulointerstitial for the 17 known eGFR-associated index SNPs as well as for the 19 new independent signals using unconditional and conditional eGFR results (Methods and Supplementary Table S2).
Using unconditional eGFR associations, we identified 56 genes mapping to 13 out of 17 index SNPs for which cis-eQTL in at least one tissue colocalized with an eGFR-associated signal with a high PP (
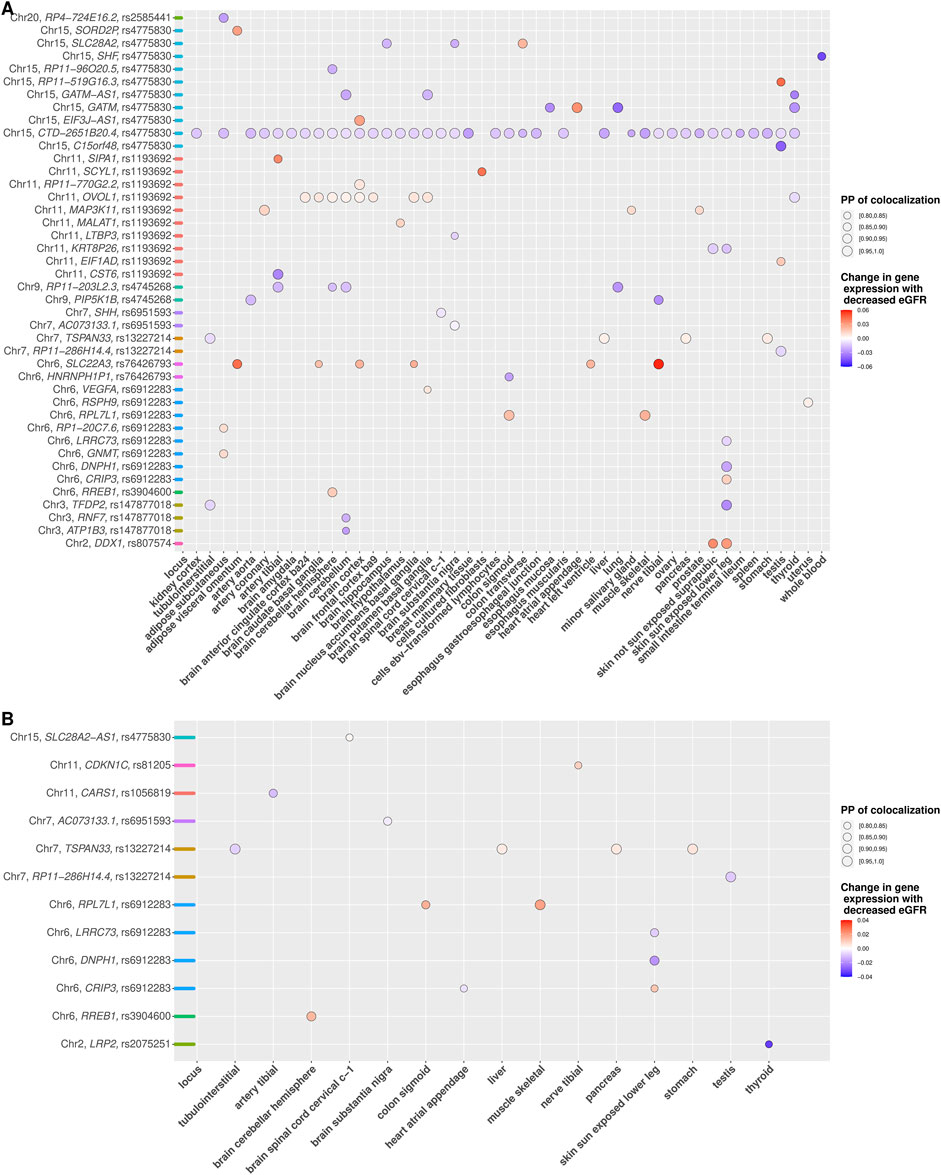
FIGURE 2. (A,B) Colocalization of eGFR association of novel independent signals with gene expression (cis eQTLs) across tissues. (A and B) depict colocalization results based on unconditional and conditional eGFR association analyses, respectively. Gene with at least one posterior probability of colocalization (PP
To determine more robust evidence of colocalization, we re-run the colocalization for each known index SNP using the corresponding conditional eGFR association. We identified 53 genes mapping to 11 index SNPs for which cis-eQTL in at least one tissue colocalized with an eGFR-associated signal with a high PP (Supplementary Table S9 and Supplementary Figure S59). We identified 10 genes that colocalized with four index SNPs exclusively using conditional associations, which would have remained undetected if only colocalization of unconditional associations had been considered (Table 2). Comparing colocalization for index SNPs based on unconditional with conditional associations across all tissues revealed consistent results for 45 genes mapping to eight index SNPs (Supplementary Table S10), which means that multiple independent signals did not affect the colocalization analyses at these loci. On the other hand, 11 genes mapping to six index SNPs were detected only by colocalization using unconditional association, indicating that multiple independent signals at these loci affected the colocalization analyses for the corresponding index SNPs (Supplementary Table S11).
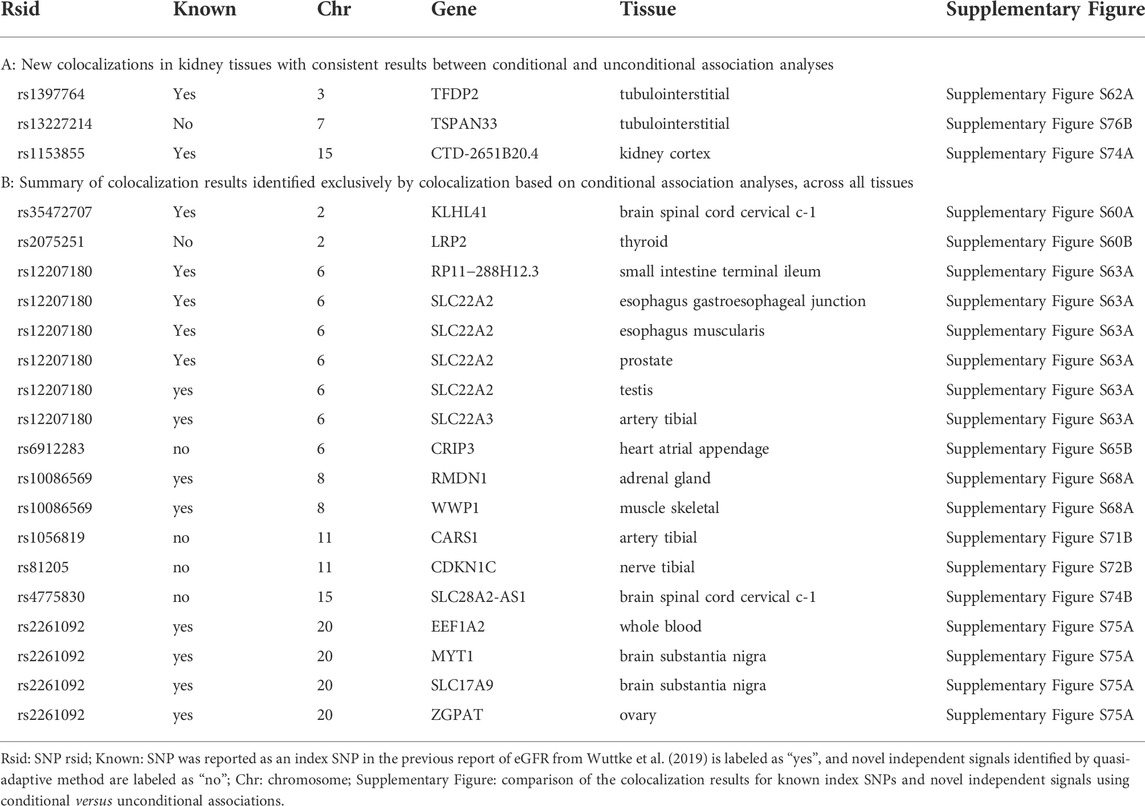
TABLE 2. Summary of colocalization of eGFR association known index SNPs and novel independent signals with posterior probability (PP ≥ 80%). (A-B) contain summary of colocalization of eGFR association known index SNPs and novel independent signals with a high posterior probability of colocalization (PP) ≥ 80% in at least one tissue.
Colocalization for each new independent signal using conditional association analysis mapped 12 genes to eight of the 19 independent signals with colocalization PP ≥ 80% in at least one tissue (Supplementary Table S9 and Figure 2B). We identified eight genes mapping to 4 novel independent signals with consistent results between colocalization based on unconditional and conditional associations, indicating accurate colocalization results for novel independent signals at these loci (Supplementary Table S10). In addition, five genes mapping to 5 novel independent signals were identified exclusively by colocalization using conditional associations, which would have remained undetected if only colocalization using unconditional associations had been considered (Table 2 and Figure 2B). On the other hand, 34 genes mapping to 9 novel independent signals were detected only by colocalization using unconditional associations, indicating that colocalization using unconditional association has less power to detect accurate results at these loci (Supplementary Table S11).
The complete comparison of the colocalization results for known index SNPs and novel independent signals using conditional versus unconditional associations are provided in Supplementary Figures S60-S76.
Discussion
Application of our recently developed quasi-adaptive method to the publicly available GWAS meta-analysis results of eGFR among 783,978 European-ancestry individuals (Wuttke et al., 2019) and subsequent replication in additional 408,608 individuals from UKBB identified 19 novel independent eGFR association signals. These signals included five secondary signals, five tertiary signals, six signals of fourth, two signals of fifth, and one signal of sixth. These results would have gone undetected by conditional analysis applying the commonly used but too conservative genome-wide significance level of 5 × 10–8. Of note, the individuals included in the LD reference sample were also part of the replication stage, but an influence of the results is very unlikely because of the substantially larger sample size in the replication analysis, and the different methods applied (summary statistics with LD reference vs individual level conditional analysis).
Some previous reports on eGFR support our findings. For instance, our secondary signal rs147877018 was previously discovered as an eGFR-associated signal through conditional analysis implemented in GCTA (at locus-wide significance, p < 10–5)20. In addition, Wuttke et al. (2019) (Wuttke et al., 2019) reported ADCY6 as a novel eGFR candidate gene in humans by performing a nested candidate gene analysis in mice. ADCY6 has not been reported to contain genome-wide significant eGFR-associated SNPs or to be located near known loci. However, in our study, the secondary signal rs3730071 was discovered near ADCY6 (Supplementary Figure S13).
Colocalization of eGFR-associated known index SNPs and novel independent signals and gene expression implicate specific potential functional genes for follow-up. We investigated the kidney by using cis-eQTL dataset from the publicly available GTEx project (Aguet et al., 2019). However, the human kidney tissues have been poorly covered by the GTEx study, and only the kidney cortex with small sample size is included in this dataset. To overcome this limitation, we also investigated kidney tissue by using a cis-eQTL dataset from microdissected human glomerular and tubulointerstitial kidney portions from 187 individuals from the NEPTUNE study (Gillies et al., 2018).
The presence of multiple independent GWAS signals at a locus violates the assumption required by the applied colocalization method (one causal variant for each locus) and likely reduces the power to detect accurate colocalization results. In this context, Wu. et al. (2019) (Wu et al., 2019) showed that for a locus with multiple GWAS signals and/or multiple eQTL signals for the same gene, integration of conditional GWAS association and conditional eQTL led to more robust evidence of colocalization. Our project provides conditional eGFR association tests conducted in the UKBB individual-level genotype dataset. These tests were used to improve the colocalization analyses of the known index SNPs and novel independent signals to identify plausible effector genes related to eGFR. Our findings could be improved by adding the conditional eQTLs data, which may have affected our ability to colocalize signals. It is worth noting that the conditional eQTLs data are not available in our study.
The consistent results between colocalization using unconditional and conditional associations at a locus with multiple independent signals confirm that the colocalization based on unconditional association has enough power to detect accurate colocalization. On the other hand, inconsistent results indicate that colocalization based on unconditional association is affected by the presence of other independent signals at a locus and has less power to detect true colocalization. Therefore, we suggest more accurate results based on colocalization analyses using conditional association and eQTLs, revealing the plausible candidate genes after eliminating the potential effect of other multiple signals.
For instance, in tubulointerstitial and kidney cortex we revealed the known index SNPs rs1397764 and rs1153855 as the shared underlying variants for colocalization of lower eGFR with lower expression of TFDP2 and CTD−2651B20.4, respectively. This was identified by colocalization based on both unconditional and conditional association analyses (Table 2A and Supplementary Figures S58, S59). Across other tissues, we suggest SLC22A2 as a plausible candidate gene colocalized with index SNP rs12207180, which was detected only after eliminating the effect of other multiple signals at the locus (Table 2B and Supplementary Figure S59). TFDP2, CTD−2651B20.4, and SLC22A2 were exclusively identified by our colocalization and have not been reported in the previous report of eGFR (Wuttke et al., 2019). TFDP2 encodes E2F dimerization partner (DP)-2, which forms heterodimers with the E2F transcription factors resulting in transcriptional activation of cell cycle-regulated genes. Although the role of TFDP2 in the context of renal disease has not been reported, several genetic associations in or near TFDP2 have been reported in previous GWAS of eGFR and CKD (Kottgen et al., 2010; Pattaro et al., 2016; Hellwege et al., 2019; Morris et al., 2019; Wuttke et al., 2019). In addition, TFDP2 was identified as a prioritized gene for eGFR by performing a transcriptome-wide association study (TWAS) and a summary Mendelian randomization test (Doke et al., 2021). Furthermore, the expression of TFDP2 was associated with the eGFR index variant, specifically in kidney-specific eQTL associations (Graham et al., 2019). CTD−2651B20.4 is a protein-kinase, interferon-inducible double-stranded RNA-dependent inhibitor, and repressor of (P58 repressor) (PRKRIR) pseudogene with Ensembl version identifier ENSG00000259433.2. There is no explicit function for CTD−2651B20.4, and it has not been reported to contain or be located near associated variants with phenotypes, diseases, and traits in humans or other species. SLC22A2 is specifically expressed in the kidney and plays a critical role in the renal secretion of various cationic compounds (Aoki et al., 2008). SLC22A2 encodes the polyspecific organic cation transporter (OCT2) and mediates tubular uptake of organic compounds including creatinine in the basolateral membrane of renal tubular epithelial cells (Urakami et al., 2004). SLC22A2 has been reported to contain or to be located near genetic associations in multiple GWAS of eGFR and CKD (Kottgen et al., 2010; Mahajan et al., 2016; Morris et al., 2019; Wuttke et al., 2019).
Our colocalization of novel independent signals suggests rs13227214 as the shared underlying variant for colocalization of lower eGFR with lower expression of TSPAN33in tubulointerstitial tissue, which was robustly identified based on both unconditional and conditional association analyses (Table 2A and Figure 2). Furthermore, in thyroid and nerve tibial tissue, we suggest LRP2 and CDKN1C as the plausible candidate genes colocalized with rs2075251 and rs81205, respectively, which were detected only by colocalization based on conditional associations (Table 2B and Figure 2B). TSPAN33, LRP2, and CDKN1C were identified exclusively by our colocalization of novel independent signals and would have remained undetected if only colocalization of the corresponding index SNPs rs3757387, rs35472707, and rs233438 were considered at these loci (Supplementary Figure S67, Supplementary Figure S60, and Supplementary Figure S72). TSPAN33 is a member of the tetraspanin family and encodes a transmembrane protein. TSPAN33 is highly expressed in the kidney and TSPAN33 mRNA is detectable in the kidney by both microarray and qPCR (Luu et al., 2013). Furthermore, in colocalization analysis of kidney-specific eQTL association (kidney cortex (Ko et al., 2017), glomerulus, and tubule-interstitial compartments (Gillies et al., 2018), TPSAN33 showed significant colocalization with the eGFR association (Graham et al., 2019). LRP2 encodes the megalin receptor (Nielsen and Christensen, 2010) and connected to its seed gene DAB2, through protein–protein interaction (Hosaka et al., 2009). Chasman et al. (2012) identified LRP2 related to the kidney function through connection with the previously known eGFR gene DAB2 and prior biological knowledge about megalin system in kidney function (Chasman et al., 2012). CDKN1C expressed in the heart, brain, lung, skeletal muscle, kidney, pancreas and testis. Up-regulation of miR-199a-5p through suppressing CDKN1C might promote cell proliferation in autosomal dominant polycystic kidney disease tissues (Sun et al., 2015), which is a genetic disorder characterized by the growth of numerous cysts in the kidney often causes renal failure with many serious complications.
In summary, we have extended our quasi-adaptive method toward identifying multiple independent SNPs within a locus, applied this method to an eGFR meta-analysis result, and discovered and replicated novel eGFR-associated SNPs. Using these results, we revealed plausible candidate genes for eGFR by colocalization, partly undetected using standard approaches. These findings will help improve the understanding of biological mechanisms underlying kidney function and may subsequently help reducing the burden of CKD.
Data availability statement
The datasets presented in this study can be found in online repositories. The names of the repository/repositories and accession number(s) can be found in the article and Supplementary Material.
Ethics statement
The studies involving human participants were reviewed and approved by the ethics committee of the respective studies provided the summary statistics included in this project. Written informed consent for participation was not required for this study in accordance with the national legislation and the institutional requirements.
Author contributions
SG, TB, and AT contributed to conception and design of the study. SG performed the statistical analysis. AT supervised the project. AT and HG acquired funding for the analyses. SG wrote the first draft of the manuscript. All authors contributed to manuscript revision, read, and approved the submitted version.
Acknowledgments
We acknowledge support for the Article Processing Charge by the German Research Foundation and the Open Access Publication Fund of the University of Greifswald. This research has been conducted using the United Kingdom Biobank resource under application number 20272.
Conflict of interest
HG has received travel grants and speakers honoraria from Fresenius Medical Care, Neuraxpharm, Servier and Janssen Cilag as well as research funding from Fresenius Medical Care not related to the current project.
The remaining authors declare that the research was conducted in the absence of any commercial or financial relationships that could be construed as a potential conflict of interest.
Publisher’s note
All claims expressed in this article are solely those of the authors and do not necessarily represent those of their affiliated organizations, or those of the publisher, the editors and the reviewers. Any product that may be evaluated in this article, or claim that may be made by its manufacturer, is not guaranteed or endorsed by the publisher.
Supplementary material
The Supplementary Material for this article can be found online at: https://www.frontiersin.org/articles/10.3389/fgene.2022.997302/full#supplementary-material
References
Aguet, F., et al. (2019). The GTEx Consortium atlas of genetic regulatory effects across human tissues. bioRxiv, 787903. doi:10.1101/787903
Aoki, M., Terada, T., Kajiwara, M., Ogasawara, K., Ikai, I., Ogawa, O., et al. (2008). Kidney-specific expression of human organic cation transporter 2 (OCT2/SLC22A2) is regulated by DNA methylation. Am. J. Physiol. Ren. Physiol. 295 (1), F165–F170. doi:10.1152/ajprenal.90257.2008
Astor, B. C., Matsushita, K., Gansevoort, R. T., van der Velde, M., Woodward, M., Levey, A. S., et al. (2011). Lower estimated glomerular filtration rate and higher albuminuria are associated with mortality and end-stage renal disease. A collaborative meta-analysis of kidney disease population cohorts. Kidney Int. 79, 1331–1340. doi:10.1038/ki.2010.550
Bello, A. K., Hemmelgarn, B., Lloyd, A., James, M. T., Manns, B. J., Klarenbach, S., et al. (2011). Associations among estimated glomerular filtration rate, proteinuria, and adverse cardiovascular outcomes. Clin. J. Am. Soc. Nephrol. 6 (6), 1418–1426. doi:10.2215/CJN.09741110
Bycroft, C., Freeman, C., Petkova, D., Band, G., Elliott, L. T., Sharp, K., et al. (2018). The UK biobank resource with deep phenotyping and genomic data. Nature 562 (7726), 203–209. doi:10.1038/s41586-018-0579-z
Chasman, D. I., Fuchsberger, C., Pattaro, C., Teumer, A., Boger, C. A., Endlich, K., et al. (2012). Integration of genome-wide association studies with biological knowledge identifies six novel genes related to kidney function. Hum. Mol. Genet. 21 (24), 5329–5343. doi:10.1093/hmg/dds369
Chronic Kidney Disease PrognosisMatsushita van der Velde, M., Astor, B. C., Woodward, M., Levey, A. S., et al. (2010). Association of estimated glomerular filtration rate and albuminuria with all-cause and cardiovascular mortality in general population cohorts: A collaborative meta-analysis. Lancet 375, 2073–2081. doi:10.1016/S0140-6736(10)60674-5
Doke, T., Huang, S., Qiu, C., Liu, H., Guan, Y., Hu, H., et al. (2021). Transcriptome-wide association analysis identifies DACH1 as a kidney disease risk gene that contributes to fibrosis. J. Clin. Investig. 131 (10), e141801. doi:10.1172/JCI141801
Gansevoort, R. T., Correa-Rotter, R., Hemmelgarn, B. R., Jafar, T. H., Heerspink, H. J. L., Mann, J. F., et al. (2013). Chronic kidney disease and cardiovascular risk: Epidemiology, mechanisms, and prevention. Lancet 382 (9889), 339–352. doi:10.1016/S0140-6736(13)60595-4
Gansevoort, R. T., Matsushita, K., van der Velde, M., Astor, B. C., Woodward, M., Levey, A. S., et al. (2011). Lower estimated GFR and higher albuminuria are associated with adverse kidney outcomes. A collaborative meta-analysis of general and high-risk population cohorts. Kidney Int. 80, 93–104. doi:10.1038/ki.2010.531
Ghasemi, S., Teumer, A., Wuttke, M., and Becker, T. (2021). Assessment of significance of conditionally independent GWAS signals. Bioinformatics 37 (20), 3521–3529. doi:10.1093/bioinformatics/btab332
Giambartolomei, C., Vukcevic, D., Schadt, E. E., Franke, L., Hingorani, A. D., Wallace, C., et al. (2014). Bayesian test for colocalisation between pairs of genetic association studies using summary statistics. PLoS Genet. 10, e1004383. doi:10.1371/journal.pgen.1004383
Gillies, C. E., Putler, R., Menon, R., Otto, E., Yasutake, K., Nair, V., et al. (2018). An eQTL landscape of kidney tissue in human nephrotic syndrome. Am. J. Hum. Genet. 103, 232–244. doi:10.1016/j.ajhg.2018.07.004
Go, A. S., Chertow, G. M., Fan, D., McCulloch, C. E., and Hsu, C. y. (2004). Chronic kidney disease and the risks of death, cardiovascular events, and hospitalization. N. Engl. J. Med. 351 (13), 1296–1305. doi:10.1056/NEJMoa041031
Gorski, M., Most, P. J. v. d., Teumer, A., Chu, A. Y., Li, M., Mijatovic, V., et al. (2017). Corrigendum: 1000 genomes-based meta-analysis identifies 10 novel loci for kidney function. Sci. Rep. 7, 46835. doi:10.1038/srep46835
Graham, S. E., Nielsen, J. B., Zawistowski, M., Zhou, W., Fritsche, L. G., Gabrielsen, M. E., et al. (2019). Sex-specific and pleiotropic effects underlying kidney function identified from GWAS meta-analysis. Nat. Commun. 10, 1847. doi:10.1038/s41467-019-09861-z
Hellwege, J. N., Velez Edwards, D. R., Giri, A., Qiu, C., Park, J., Torstenson, E. S., et al. (2019). Mapping eGFR loci to the renal transcriptome and phenome in the VA Million Veteran Program. Nat. Commun. 10, 3842. doi:10.1038/s41467-019-11704-w
Hemmelgarn, B. R., Manns, B. J., Lloyd, A., James, M. T., Klarenbach, S., Quinn, R. R., et al. (2010). Relation between kidney function, proteinuria, and adverse outcomes. JAMA 303, 423–429. doi:10.1001/jama.2010.39
Herold, C., Steffens, M., Brockschmidt, F. F., Baur, M. P., and Becker, T. (2009). Intersnp: Genome-wide interaction analysis guided by a priori information. Bioinformatics 25, 3275–3281. doi:10.1093/bioinformatics/btp596
Hishida, A., Nakatochi, M., Akiyama, M., Kamatani, Y., Nishiyama, T., Ito, H., et al. (2018). Genome-wide association study of renal function traits: Results from the Japan multi-institutional collaborative cohort study. Am. J. Nephrol. 47, 304–316. doi:10.1159/000488946
Hosaka, K., Takeda, T., Iino, N., Hosojima, M., Sato, H., Kaseda, R., et al. (2009). Megalin and nonmuscle myosin heavy chain IIA interact with the adaptor protein disabled-2 in proximal tubule cells. Kidney Int. 75, 1308–1315. doi:10.1038/ki.2009.85
Kanai, M., Akiyama, M., Takahashi, A., Matoba, N., Momozawa, Y., Ikeda, M., et al. (2018). Genetic analysis of quantitative traits in the Japanese population links cell types to complex human diseases. Nat. Genet. 50, 390–400. doi:10.1038/s41588-018-0047-6
Kang, G., Ye, K., Liu, N., Allison, D. B., and Gao, G. (2009). Weighted multiple hypothesis testing procedures. Stat. Appl. Genet. Mol. Biol. 8, Article23–22. doi:10.2202/1544-6115.1437
Ko, Y. A., Yi, H., Qiu, C., Huang, S., Park, J., Ledo, N., et al. (2017). Genetic-variation-driven gene-expression changes highlight genes with important functions for kidney disease. Am. J. Hum. Genet. 100 (6), 940–953. doi:10.1016/j.ajhg.2017.05.004
Kottgen, A., Pattaro, C., Boger, C. A., Fuchsberger, C., Olden, M., Glazer, N. L., et al. (2010). New loci associated with kidney function and chronic kidney disease. Nat. Genet. 42, 376–384. doi:10.1038/ng.568
Lee, J., Lee, Y., Park, B., Won, S., Han, J. S., and Heo, N. J. (2018). Genome-wide association analysis identifies multiple loci associated with kidney disease-related traits in Korean populations. PLoS One 13 (3), e0194044. doi:10.1371/journal.pone.0194044
Loh, P.-R., Tucker, G., Bulik-Sullivan, B. K., Vilhjalmsson, B. J., Finucane, H. K., Salem, R. M., et al. (2005). Efficient Bayesian mixed model analysis increases association power in large cohorts. Nat. Genet. 47, 284–290. doi:10.1038/ng.3190
Luu, V. P., Hevezi, P., Vences-Catalan, F., Maravillas-Montero, J. L., White, C. A., Casali, P., et al. (2013). TSPAN33 is a novel marker of activated and malignant B cells. Clin. Immunol. 149 (3), 388–399. doi:10.1016/j.clim.2013.08.005
Mahajan, A., Rodan, A. R., Le, T. H., Gaulton, K. J., Haessler, J., Stilp, A. M., et al. (2016). Trans-ethnic fine mapping highlights kidney-function genes linked to salt sensitivity. Am. J. Hum. Genet. 99 (3), 636–646. doi:10.1016/j.ajhg.2016.07.012
Matsushita, K., Coresh, J., Sang, Y., Chalmers, J., Fox, C., Guallar, E., et al. (2015). Estimated glomerular filtration rate and albuminuria for prediction of cardiovascular outcomes: A collaborative meta-analysis of individual participant data. Lancet. Diabetes Endocrinol. 3, 514–525. doi:10.1016/S2213-8587(15)00040-6
Morris, A. P., Le, T. H., Wu, H., Akbarov, A., van der Most, P. J., Hemani, G., et al. (2019). Trans-ethnic kidney function association study reveals putative causal genes and effects on kidney-specific disease aetiologies. Nat. Commun. 10, 29. doi:10.1038/s41467-018-07867-7
Nica, A. C., and Dermitzakis, E. T. (2013). Expression quantitative trait loci: Present and future. Philos. Trans. R. Soc. Lond. B Biol. Sci. 368 (1620), 20120362. doi:10.1098/rstb.2012.0362
Nielsen, R., and Christensen, E. I. (2010). Proteinuria and events beyond the slit. Pediatr. Nephrol. 25, 813–822. doi:10.1007/s00467-009-1381-9
Okada, Y., Sim, X., Go, M. J., Wu, J. Y., Gu, D., Takeuchi, F., et al. (2012). Meta-analysis identifies multiple loci associated with kidney function-related traits in east Asian populations. Nat. Genet. 44, 904–909. doi:10.1038/ng.2352
Pattaro, C., Kottgen, A., Teumer, A., Garnaas, M., Boger, C. A., Fuchsberger, C., et al. (2012). Genome-wide association and functional follow-up reveals new loci for kidney function. PLoS Genet. 8, e1002584. doi:10.1371/journal.pgen.1002584
Pattaro, C., Teumer, A., Gorski, M., Chu, A. Y., Li, M., Mijatovic, V., et al. (2016). Genetic associations at 53 loci highlight cell types and biological pathways relevant for kidney function. Nat. Commun. 7, 10023. doi:10.1038/ncomms10023
Sun, L., Zhu, J., Wu, M., Sun, H., Zhou, C., Fu, L., et al. (2015). Inhibition of MiR-199a-5p reduced cell proliferation in autosomal dominant polycystic kidney disease through targeting CDKN1C. Med. Sci. Monit. 21, 195–200. doi:10.12659/MSM.892141
Teumer, A., Li, Y., Ghasemi, S., Prins, B. P., Wuttke, M., Hermle, T., et al. (2019). Genome-wide association meta-analyses and fine-mapping elucidate pathways influencing albuminuria. Nat. Commun. 10, 4130–4219. doi:10.1038/s41467-019-11576-0
Urakami, Y., Kimura, N., Okuda, M., and Inui, K. i. (2004). Creatinine transport by basolateral organic cation transporter hOCT2 in the human kidney. Pharm. Res. 21, 976–981. doi:10.1023/b:pham.0000029286.45788.ad
Weiner, D. E., Tighiouart, H., Amin, M. G., Stark, P. C., MacLeod, B., Griffith, J. L., et al. (2014). Chronic kidney disease as a risk factor for cardiovascular disease and all-cause mortality: A pooled analysis of community-based studies. J. Am. Soc. Nephrol. 15 (5), 1307–1315. doi:10.1097/01.asn.0000123691.46138.e2
Wu, Y., Broadaway, K. A., Raulerson, C. K., Scott, L. J., Pan, C., Ko, A., et al. (2019). Colocalization of GWAS and eQTL signals at loci with multiple signals identifies additional candidate genes for body fat distribution. Hum. Mol. Genet. 28 (24), 4161–4172. doi:10.1093/hmg/ddz263
Wuttke, M., Li, Y., Sieber, K. B., Feitosa, M. F., Gorski, M., et al. (2019). A catalog of genetic loci associated with kidney function from analyses of a million individuals. Nat. Genet. 51 (6), 957–972. doi:10.1038/s41588-019-0407-x
Keywords: estimated glomerular filtration rate (eGFR), genome-wide association studies (GWAS), expression quantitative trait loci (eQTL), conditional association analysis, SNP-specific alpha-level, colocalization
Citation: Ghasemi S, Becker T, Grabe HJ and Teumer A (2022) Discovery of novel eGFR-associated multiple independent signals using a quasi-adaptive method. Front. Genet. 13:997302. doi: 10.3389/fgene.2022.997302
Received: 18 July 2022; Accepted: 13 October 2022;
Published: 31 October 2022.
Edited by:
Yaowu Liu, Southwestern University of Finance and Economics, ChinaReviewed by:
Elise Flynn, Columbia University, United StatesRyan Sun, University of Texas MD Anderson Cancer Center, United States
Weiqiu Cheng, University of Oslo, Norway
Copyright © 2022 Ghasemi, Becker, Grabe and Teumer. This is an open-access article distributed under the terms of the Creative Commons Attribution License (CC BY). The use, distribution or reproduction in other forums is permitted, provided the original author(s) and the copyright owner(s) are credited and that the original publication in this journal is cited, in accordance with accepted academic practice. No use, distribution or reproduction is permitted which does not comply with these terms.
*Correspondence: Sahar Ghasemi, c2FoYXIuZ2hhc2VtaUB1bmlrbGluaWstZnJlaWJ1cmcuZGU=; Alexander Teumer, YXRldW1lckB1bmktZ3JlaWZzd2FsZC5kZQ==