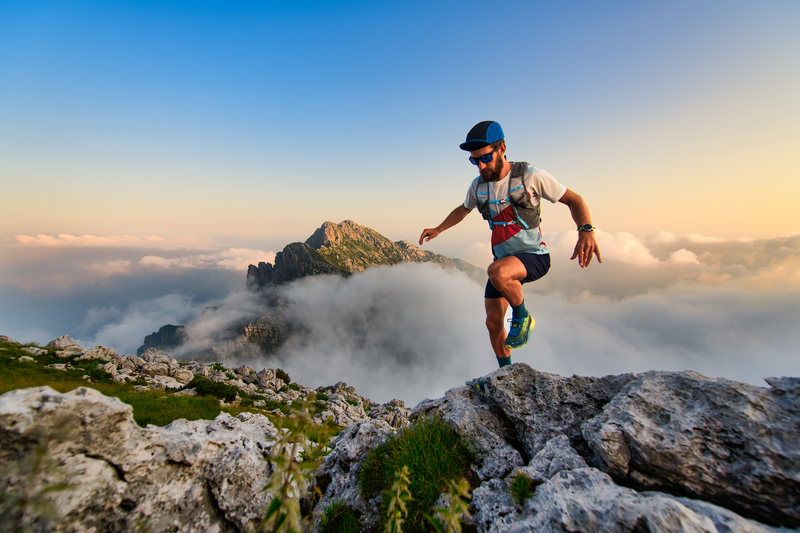
95% of researchers rate our articles as excellent or good
Learn more about the work of our research integrity team to safeguard the quality of each article we publish.
Find out more
ORIGINAL RESEARCH article
Front. Genet. , 06 September 2022
Sec. Computational Genomics
Volume 13 - 2022 | https://doi.org/10.3389/fgene.2022.989327
This article is part of the Research Topic Bioinformatics Analysis of Omics Data for Biomarker Identification in Clinical Research, Volume II View all 53 articles
Colorectal cancer (CRC) is a common malignant tumor worldwide. Lipid metabolism is a prerequisite for the growth, proliferation and invasion of cancer cells. However, the lipid metabolism-related gene signature and its underlying molecular mechanisms remain unclear. The aim of this study was to establish a lipid metabolism signature risk model for survival prediction in CRC and to investigate the effect of gene signature on the immune microenvironment. Lipid metabolism-mediated genes (LMGs) were obtained from the Molecular Signatures Database. The consensus molecular subtypes were established using “ConsensusClusterPlus” based on LMGs and the cancer genome atlas (TCGA) data. The risk model was established using univariate and multivariate Cox regression with TCGA database and independently validated in the international cancer genome consortium (ICGC) datasets. Immune infiltration in the risk model was developed using CIBERSORT and xCell analyses. A total of 267 differentially expressed genes (DEGs) were identified between subtype 1 and subtype 2 from consensus molecular subtypes, including 153 upregulated DEGs and 114 downregulated DEGs. 21 DEGs associated with overall survival (OS) were selected using univariate Cox regression analysis. Furthermore, a prognostic risk model was constructed using the risk coefficients and gene expression of eleven-gene signature. Patients with a high-risk score had poorer OS compared with patients in the low-risk score group (p = 3.36e-07) in the TCGA cohort and the validationdatasets (p = 4.03e-05). Analysis of immune infiltration identified multiple T cells were associated with better prognosis in the low-risk group, including Th2 cells (p = 0.0208), regulatory T cells (p = 0.0425), and gammadelta T cells (p = 0.0112). A nomogram integrating the risk model and clinical characteristics was further developed to predict the prognosis of patients with CRC. In conclusion, our study revealed that the expression of lipid-metabolism genes were correlated with the immune microenvironment. The eleven-gene signature might be useful for prediction the prognosis of CRC patients.
Colorectal cancer (CRC) is the third most common malignant cancer worldwide (Johdi and Sukor, 2020), which will project a total of 2.2 million new cases and 1.1 million deaths by 2030 (Douaiher et al., 2017). Therefore, early intervention for primary CRC contributes to clinical benefit outcomes (Koncina et al., 2020). At present, the main treatment methods for CRC are tumor resection, radiotherapy, chemotherapy, anti-angiogenic therapy, targeted therapy and immunotherapy (Rejhova et al., 2018; Piawah and Venook, 2019; Dariya et al., 2020; Johdi and Sukor, 2020). Although a certain degree of success has been achieved with these treatments, several significant challenges remain to be addressed. (Biller and Schrag, 2021). However, the etiology and molecular mechanisms of CRC are still unclear. Previous studies have identified prognostic and predictive molecular biomarkers for CRC based on DNA, RNA, or proteins, such as APC, VEGF-1 (Clarke, 2005; Das et al., 2017; Koncina et al., 2020). Liu et al. (2020) identified two risky (TIMP1 and LZTS3) and five protective prognostic genes (AXIN2, CXCL1, ITLN1, CPT2, and CLDN23) which provided more evidence for further application of novel diagnostic and prognostic biomarkers in CRC. A prognostic signature consisting of nine genes was established with good performance for the prediction of survival in CRC patients (Chen et al., 2019). Moreover, novel potential prognostic biomarkers still need to be explored for patient risk stratification and for the choice of best treatment options. Therefore, it is necessary to screen novel molecular therapeutic targets to improve the survival rate of CRC patients.
Lipids are essential components of biological membranes and are signaling molecules involved in cellular activities (Bian et al., 2021). Lipid metabolism plays an important role in maintaining of cellular homeostasis (Rohrig and Schulze, 2016; Bian et al., 2021). Numerous studies have demonstrated that lipid metabolism was involved in the progression, recurrence and tumor microenvironment (TME) of CRC (Lin et al., 2021). Dysregulation of lipid metabolism occurs in multiple cancers, including CRC (Dias et al., 2019; Haffa et al., 2019). Numerous bioactive secondary messengers trigger the activation of RAS, phosphoinositide 3-kinases (PI3Ks) and other signaling pathways to promote tumorigenesis (Yang et al., 2019; Moore et al., 2020). A previous study has shown that a total of 13 metabolites, including glycerophospholipids, were associated with a reduced risk of recurrence in CRC patients (Ose et al., 2021). Blocking metabolic reprogramming of tumor cells in obese mice improves anti-tumor immunity by impairing CD8+ T cell infiltration in the tumor microenvironment (Ringel et al., 2020). However, studies focusing on the characterization and risk signatures of lipid metabolism-related genes remain limited.
The aim of this study was to screen genes closely related to the prognosis of CRC using two published datasets. The 11-gene signature risk model provided a reference to distinguish high-risk groups in CRC patients with poor prognosis (Wang et al., 2021b). The workflow was illustrated in Figure 1.
FIGURE 1. The workflow of lipid metabolism-related signature identification. TCGA: The Cancer Genome Atlas. MSigDB: Molecular Signatures Database. ICGC: International Cancer Genome Consortium. LMGs: Lipid metabolism-associated genes.
RNA sequencing (RNA-seq) data and clinical features were retrieved from The Cancer Genome Atlas (TCGA) database (404 samples, TCGA-COAD, https://portal.gdc.cancer.gov/). Furthermore, the ICGC datasets (302 samples, COAD-US, https://dcc.icgc.org/releases/current/Projects/) were used to validate the risk model. RPKM is a widely used method for normalizing RNA-seq gene expression (Guo et al., 2013). All data were analyzed using RPKM expression profiles, including differentially expressed genes (DEGs), consensus clustering, analysis of tumor-infiltrating immune cells and univariate and multivariate Cox regression analyses. Lipid metabolism-associated genes (LMGs) were obtained from the Molecular Signature Database (MSigDB, http://gsea-msigdb.org). We selected the following keywords to select Lipid metabolism-associated genes (LMGs), including “lipid,” “lipid metabolism,” “metabolism of lipid,” “fat metabolism,” “fatty acid metabolism,” “metabolism of fat.” A total of 744 LMGs were selected from MSigDB and provided in the Supplementary Table S1.
Consensus analysis was performed using the “ConsensusClusterPlus” R package to assign patients with COAD into different clusters in the TCGA dataset. Subsequently, the DEGs were obtained between clusters with p < 0.05 and |log2 (fold change)| > 0.5 as the threshold using “limma” package in R software. The volcano plot was visualized by “ggplot2” R package.
Immune and stromal scores were calculated to evaluate cell infiltration levels in CRC. Estimation of Stromal and Immune cells in Malignant Tumor tissues using Expression data (ESTIMATE) algorithm (https://bioinformatics.mdanderson.org/estimate/) was adopted to measure stromal level (stromal scores), immunocyte infiltration degree (immune scores), and tumor purity using “estimate” R package (Li et al., 2020b; Guo et al., 2020).
The differences in immune infiltration subtypes were analyzed between the high- and low-risk groups using two-way ANOVA analysis. Furthermore, xCell algorithm was used to estimate 64 immune and stromal cell types from transcriptome data using “xCell” R package (Aran et al., 2017).
To explore the signaling pathway enrichment of overlapping genes, the Gene Ontology (GO) term and Kyoto Encyclopedia of Genes and Genomes (KEGG) pathway enrichment analyses were performed based on the online platform KOBAS (http://kobas.cbi.pku.edu.cn/index.php) with p < 0.05. The visualization was performed using “ggplot2” R package (Ito and Murphy, 2013).
Univariate Cox regression analysis was used to identify the lipid metabolism-related genes associated with prognosis of CRC with p < 0.05. The multivariate Cox regression analysis was performed to screen independent prognostic signature. Risk score for the signature was evaluated as following algorithm: Riskscore = Coefgene1*expressiongene1 + Coefgene2*expressiongene2 + Coefgene3*expressiongene3 + ...... + Coefgenen*expressiongenen (where “Coef” and “expression” are respectively the coefficient and RNA relative expression value, “gene” represents each selected gene range from 1 to n) (Wang et al., 2020b). Briefly, firstly, a robust likelihood-based survival modeling approach was used to narrow the number of genes from 21 key LMGs and the best genes were selected for the prognostic model using “survminer” and “survival” R package (Wang et al., 2021c). Secondly, multiple Cox regression analysis was performed to establish prognostic risk model using “survival” R package with a parameter of “direction = “both” (Wang et al., 2020b). Thirdly, the prognostic risk model of each sample was calculated with coefficient value of multiple Cox regression analysis using the following: Risk score = (−0.376743) × GGT5 + (−0.572140) × ASAH1 + (−0.484800) × HMGCL + (0.670476) × CD36 + (0.733487) × DPM2 + (−0.463117) × ACOX1 + (0.506670) × ANGPTL4 + (0.434523) × INSR + (−0.504028) × ADIPOQ + (0.615982) × ALDH1A3 + (−0.195776) × MMP1. The median parameter of risk score was 1.012238. The ROC curve was plotted using “survivalROC” R package with a parameter of “method = “KM” (Heagerty et al., 2000). The “pheatmap” R package (version 1.0.2, https://cran.r-project.org/web/packages/pheatmap/index.html) to show the heat map. The nomogram was formed to estimate the overall survival (OS) of CRC by using “rms” and “survival” package in R. The regression coefficients of the regression model was transformed into scores and plotted as a nomogram for prediction of prognosis. Moreover, the calibration curves were used to evaluate the precision of nomogram for the probability of actual occurrence versus prediction, with Hosmer-Lemeshow fit goodness test (Zhou et al., 2019).
A total of 744 LMGs were selected from MSigDB in Methods or Results and provided the terms in the Supplementary Table S1. Consensus clustering was conducted to divide the samples of 404 patients with CRC into subtypes. Optimal clustering stability was identified with K = 2 as the cut-off criterion (Supplementary Figures S1A,B). These samples were divided into two molecular subtypes including 251 samples in subtype 1 and 153 samples in subtype 2 (Figure 2A). To explore the dysregulated genes between two molecular subtypes, the differentially expressed genes (DEGs) visualized in volcano plot were screened by using “limma” R package (Ritchie et al., 2015). A total of 267 DEGs were identified with p < 0.05 and |Log2 (fold change)| > 0.5, including 153 upregulated DEGs and 114 downregulated DEGs (Figure 2B). Furthermore, univariate Cox regression analysis was performed to calculate the hazard ratio (HR) for OS. A total of 21 genes had a statistically significant effect on OS in CRC with overlapping DEGs (Figure 2C). The HR values of the 21 genes were calculated and shown in Figure 2D as potential molecular targets.
FIGURE 2. Identification of differentially expressed LMGs with consensus molecular subtypes and univariate Cox regression analysis. (A) Molecular subtype discrimination in TCGA. (B) Differentially expressed LMGs between subtype 1 and subtype 2. (C) Venn diagram showing 21 key LMGs (the intersection of the differentially expressed LMGs and the overall survival associated LMGs using the univariate Cox regression analysis). (D) Univariated cox proportional hazards analysis of key LMGs. HR, hazard ratio; CI, confidence interval. LMGs: Lipid metabolism-associated genes.
To establish a lipid metabolism-related gene signature, the predictive value of the risk score model was explored using the TCGA-COAD database as the training set. A total of 21 genes were intersection of those identified by differentially expression analysis and univariate Cox regression analysis. After that, a robust likelihood-based survival modeling approach was used to narrow the number of genes and select the best genes for the prognostic model using “survminer” and “survival” R package (Wang et al., 2021c). Finally, a total of 11 genes were screened to construct the risk model by using the multivariate Cox regression analysis with a parameter of “direction = both.” To evaluate the survival risk of patients with CRC, a prognostic risk model was constructed using risk coefficients and gene expression as described in previous studies (Zhang et al., 2020; Liu et al., 2021). Each patient’s risk score in the training was calculated using a mathematical algorithm. Firstly, we calculated the coefficient value of each genes using multiple Cox regression analysis. Secondly, risk score was calculated using coefficient value and expression of each gene. The mathematical algorithm in this study was described in the methods and materials section. The median risk score was 1.012238. By the median value of the risk score, all patients were divided into high-risk and low-risk groups according to the median of risk score. Patients with high-risk scores had higher mortality rate and poorer prognosis (Figure 3A, p = 3.36e-07). Supplementary Table S2 showed the number of patients along the overall survival and risk scores in TCGA and ICGC database. The risk score rank distribution of patients with CRC were shown in Figure 3B. The scatter represented the survival status and the time under the survival curve in each patient in Figure 3C. The risk scores between high- and low-risk groups in TCGA and ICGC database were added in Supplementary Table S3. The risk signature in the multivariate Cox model was illustrated in the forest plot (Figure 3D). The area under the curve (AUC) values of the ROC curve for 3-years and 5-years OS were 0.775 and 0.796, respectively (Figure 3E). The novel 11-gene prognostic signature was validated in 302 patients with CRC using the ICGC database. Survival analysis indicated that patients in the high-risk group had poorer prognosis (Figure 3F, p = 4.03e-05, Supplementary Table S2). The risk score rank distribution and survival status in each patient was shown in Figures 3G,H. The expression of the 11-gene signature and the risk score were visualized in Figure 3I. ROC analysis revealed that the risk model showed a good prediction accuracy, with the AUC of 0.767 (3-years overall survival) and AUC of 0.745 (5-years overall survival) (Figure 3J).
FIGURE 3. Development of risk model based on the 11 LMGs signature of CRC patients with the TCGA training cohort and ICGC validation database. (A) Kaplan-Meier survival plot for overall survival based on risk score of LMGs signature in TCGA cohort. (B) Risk score of CRC patients in high- and low risk groups in TCGA cohort. (C) Distribution of time under survival curve and survival status of each patient in TCGA cohort. (D) Multivariate Cox regression analysis of LMGs signature characteristics TCGA cohort. (E) ROC curve for 3 and 5 years overall survival TCGA cohort. (F) Kaplan-Meier survival plot showing overall survival using risk score of LMGs signature in ICGC validation database. (G) Risk score of CRC patients in risk groups in ICGC validation database. (H) Distribution of survival time and survival status of each patient in ICGC validation database. (I) The heatmap showing the gene expression of 11 LMGs signature and risk score in ICGC validation database. (J) ROC curve for 3 and 5 years overall survival in ICGC validation database. LMGs: Lipid metabolism-associated genes.
To explore the biological functions of the 21 genes, the Kyoto Encyclopedia of Genes and Genomes (KEGG) pathways and gene ontology (GO) terms were analyzed using KOBAS tools (http://kobas.cbi.pku.edu.cn). In the present study, these dysregulated DEGs were enriched in fatty acid metabolism and multiple cancer-related pathways including VEGF signaling, the PD-1 checkpoint pathway in cancer, the FoxO signaling pathway and Th1 and Th2 cell differentiation (Figure 4A). According to the GO term analysis, we found biological process related to nutrients, including lipid metabolic processes, fatty acid processes and toll-like receptor binding (Figure 4B).
FIGURE 4. Functional enrichment analysis. (A) Kyoto Encyclopedia of Genes and Genomes (KEGG) pathways enriched in the key LMGs. (B) Gene Ontology (GO) terms. “Ratio” presents the number of genes in our data/the number of all genes in terms or pathways. LMGs: Lipid metabolism-associated genes. The false discovery rate (FDR) < 0.05 was considered as threshold. The lower boundary value for the -log10(FDR) is 1.301 and the upper boundary value is infinite.
To predict the cell scores in the tumor microenvironment, the CIBERSORT and xCell algorithms were used to perform cell type enrichment analysis in CRC. The relative infiltration levels of various immune cell subsets were quantified using CIBERSORT algorithms (Chong et al., 2021). In the present study, a consistent result was observed in the risk score stratification (Figure 5A). Furthermore, several kinds of immune cells with high infiltration levels were significantly correlated with poorer prognosis of patients, including T follicular helper cells (Figure 5B, p = 0.048), mast activated cells (Figure 5C, p = 0.00715) and monocytes (Figure 5D, p = 0.031). In addition, the xCell method was used to estimate the abundance scores of 64 immune cell types using lipid metabolism-associated gene expression data. The stratification of abundance scores between the high- and low-risk groups for each patient was demonstrated in Figure 5E. Moreover, the correlation between immune cells and OS was assessed by using Kaplan-Meier survival with log-rank test. Our results demonstrated that the following cells with high abundance scores were associated with better prognosis, including Th2 cells (p = 0.0208, Figure 5F), regulatory T cells (Tregs) (p = 0.0425, Figure 5G), gamma delta T cells (Tgd cells) (p = 0.0112, Figure 5H) and GMP (p = 0.00493, Figure 5K). While other immune cells with high abundance scores, including chondrocytes (p = 0.0452, Figure 5I), endothelial cells (p = 0.0139, Figure 5J), mesangial cells (p = 0.0489, Figure 5L), mesenchymal stem cells (MSC, p = 0.0331, Figure 5M), Pericytes (p = 0.0234, Figure 5N), related to poorer prognosis.
FIGURE 5. Immune characteristics of risk groups. (A) Different immune cell levels between high-risk and low-risk groups using CIBERSORT analysis. Kaplan-Meier plot showing prognostic values of the risk signature between high- and low-risk groups in multiple immune cells using CIBERSORT analysis, including T follicular helper cells (B), mast activated cells (C), and monocytes (D). (E) The heatmap showing the abundance scores of immune cells in risk groups using xCell analysis. Kaplan-Meier plot illustrating prognostic values of the risk signature between high- and low-risk groups using xCell analysis in different immune cells, including Th2 cells (F), Tregs (G), Tgd cells (H), Chondrocytes (I), Endothelial cells (J), GMP (K), Mesangial cells (L), MSC (M), Pericytes (N). Th2: T helper 2 cells, Treg: Regulatory T cells. Tgd cells: gamma delta T cells. GMP: Granulocyte-macrophage progenitor, MSC: mesenchymal stem cells.
To evaluate the predictive value of risk model based on clinical features, the relationship between risk score and clinicopathological variables (age, gender, race, and stage) was calculated with the Student’s t-test and One-Way ANOVA test. Our results showed that the risk score contributed to different roles in the subgroups, including stage (Figure 6A, p = 0.0005), age (Supplementary Figure S2A, p = 0.1003), gender (Supplementary Figure S2B, p = 0.1932), race (Supplementary Figure S2C, p = 0.3185). Patients with early-stage CRC had a lower risk score compared with patients with advanced CRC (Figure 6A, p = 0.0216). The Kaplan-Meier survival curve showed that patients in the high-risk group had a dramatically shorter OS than those in the low-risk group in both early-stage CRC (Figure 6B, p = 0.0027) and advanced CRC (Figure 6C, p = 0.022).
FIGURE 6. Construction and calibration of nomogram for prognostic prediction in CRC patients. (A) The association between risk score and clinical stage. The prognostic value of the risk signature in patients with early/locally advanced CRC (stage I–III) (B) and advanced CRC (stage IV) (C). (D) The predictive nomogram based on risk score and clinical parameters for overall survival prediction at 1, 3, and 5 years. Calibration curve of the nomogram at 1 year (E), 3 years (F), and 5 years (G).
Risk score model status was incorporated into a nomogram model to predict the probability of overall survival at 1-, 3- and 5-years model. The point scale at the top of the nomogram model showed the score of every indicator, including age, gender, race, stage and risk score. All the points of each indicator were summed to estimate probability of OS at 1-, 3- and 5-years in nomogram plot (Figure 6D). Furthermore, the calibration curve was constructed to evaluate the performance of nomogram model. The C-index was 0.761 for OS prediction in training data, showing fair agreements between the nomogram prediction and actual observation for the 1-, 3- and 5-years OS (Figures 6E–G).
Colorectal cancer (CRC) is the second leading cause of death worldwide since 2020 (https://www.who.int/news-room/fact-sheets/detail/cancer). Previous studies showed prognostic models contributed to clinical decision and precision medicine (Zhang et al., 2020; Mohammed et al., 2021). The patients were divided into high- and low-risk groups for prediction of overall survival according to the risk stratification in the prognostic models (Lin et al., 2021). It is critical for improvement of the personalized therapies and the quality of life.
Intensively proliferating cancer cells need multiple metabolic patterns to get enough energy for new biomass synthesis (Warburg et al., 1927). A previous study demonstrated that lipid metabolism played critical roles in the main determinants of tumor progression (Bleve et al., 2020). Cancer cells show alterations of lipid metabolism, which lead to dysregulation of energy homeostasis, disruption of gene expression and signaling pathway (Huang and Freter, 2015; Pakiet et al., 2019). Numerous lipid molecules involved in lipid metabolism were considered as potential biomarkers, including serum polyunsaturated fatty acid metabolites (Zhang et al., 2017), cerotic acid (26:0) (Mika et al., 2017), γ-linolenic acid (18:3 n-6) (Kondo et al., 2011), and 12-keto-leukotriene B4 (Savari et al., 2014). However, the development of clinically useful lipid biomarkers requires a consistent research methodology. The aim of this study is to investigate the association between lipid metabolism and survival, and construct a lipid metabolism-based risk signature to improve the accuracy of prognosis prediction for survival in CRC patients.
Different colorectal cancer classification systems were identified by using microsatellite instability and highly expressed mesenchymal genes. However, these systems failed to incorporate with other subtypes (Wilkerson and Hayes, 2010). Consensus molecular subtypes were clustering algorithms with resampling and network-based approaches (Wilkerson and Hayes, 2010). Consensus molecular subtypes revealed prognostic value in metastatic colorectal cancer (Borelli et al., 2021). Here, the consensus molecular subtypes were established using “ConsensusClusterPlus” package in R software (Wilkerson and Hayes, 2010). In head and neck squamous cell carcinoma, the molecular features of different subtypes were evaluated for potentially effective therapeutic agents (Zhang et al., 2021). In gastric cancer, consensus molecular subtypes were associated with immune infiltration for prediction of survival (Yu et al., 2021a). In metastatic colorectal cancer, consensus molecular analysis demonstrated that the consensus molecular subtype 2 was the predominant subtype in left-sided and associated with the best outcome from the addition of bevacizumab to first-line chemotherapy (Mooi et al., 2018). Our results proved the molecular diversity of lipid metabolism-associated genes and provided different classification strategy for treatment allocation in CRC. A total of 267 differentially expressed genes were screened between cluster 1 subtype and cluster 2 subtype according to consensus molecular analysis. Furthermore, risk model was established using novel 11-gene signature from DEGs and revealed that high-risk group had poorer prognosis (p = 3.36e-07). The risk model was validated to divide patients into high- and low-risk groups for OS prediction (p = 4.03e-05). Similarly, a novel 4 gene prognostic signature revealed dramatically influence of clinical utility with risk model in colorectal cancer (Ahluwalia et al., 2019).
Numerous evidences from preclinical and clinical data support that the cancer stem cells (CSCs) are responsible for tumor recurrence (Peitzsch et al., 2017; Clarke, 2019). Lipid metabolism has been reported as potential target in bulk and CSCs, including CRC (Li et al., 2017; Choi et al., 2019). A previous study showed that blocking stearoyl-CoA desaturase 1 (SCD1) expression or function inhibited the survival of CSCs, but not bulk colorectal cancer cells in vitro and in vivo (Yu et al., 2021b). Stem colorectal cancer cells contained a distinctive lipid profile, with higher free MUFA and lower free SFA levels than bulk colorectal cancer cells through lipidomic profiling (Choi et al., 2019). Another study identified eicosapentaenoic acid, which decreased the cell number of the overall population of bulk colorectal cancer cells, but not of the stem colorectal cancer cells. Our results screened 11-gene signature of lipid metabolism for prediction of overall survival in colorectal cancer. Further in-depth studies are also warranted to elucidate the role of 11-gene signature on the behavior in bulk and stem colorectal cancer cells.
To further investigate the effect of nomogram on the predictive ability of survival, novel significant molecular signatures were screened for predicting OS in patients with CRC. The immune related signature showed better stratification and more precise immunotherapy in patients with CRC (Li et al., 2020a). A 13-gene metabolic signature was constructed to explore the association between metabolism and the immune microenvironment for prognostic prediction in stomach adenocarcinoma (Ye et al., 2021). In this study, a 11-lipid metabolism-related gene signature was established and showed improved prediction of OS for CRC patients, including GGT5, ASAH1, HMGCL, CD36, DPM2, ACOX1, ANGPTL4, INSR, ADIPOQ, ALDH1A3, and MMP1. GGT5 (Gamma-Glutamyltransferase 5) is a member of the gamma-glutamyl transpeptidase gene family involved in glutathione metabolism (Wickham et al., 2011). GGT5 was associated with immune cell infiltration and might be a potential immunological therapeutic target in gastric cancer (Wang et al., 2022). ASAH1 (N-Acylsphingosine Amidohydrolase 1) encodes a member of the acid ceramidase family of proteins and is involved in glycosphingolipid metabolism (Li et al., 1999). ASAH1 was used to build a risk model to reflect the dysregulated metabolic microenvironment in gastric cancer (Wen et al., 2020). HMGCL (3-Hydroxy-3-Methylglutaryl-CoA Lyase) is a mitochondrial enzyme and associated with HMG-CoA lyase deficiency (Menao et al., 2009). HMGCL was potential tumor suppressor gene and associated with poor prognosis in clear cell renal cell carcinoma (Cui et al., 2019). In colon adenocarcinoma, HMGCL was screened as prognosis-related metabolic gene using risk model analysis (Zhao et al., 2021). CD36 (CD36 Molecule) is a transmembrane glycoprotein that participates in adipose energy storage, and gut fat absorption (Smith et al., 2008; Tran et al., 2011; Wang and Li, 2019). A previous study demonstrated that ablation of CD36-mediated FA uptake attenuated tumor progression (Wang and Li, 2019). Moreover, CD36 was found to promote sterile inflammation and activate the protumor ability of tumor-associated immune cells (Wang and Li, 2019). DPM2 (Dolichyl-Phosphate Mannosyltransferase Subunit 2) serves as a donor of mannosyl residues on the lumenal side of the endoplasmic reticulum (Radenkovic et al., 2021). A total of 19 lipid metabolism-related genes were used to establish the risk predictive score model as a potential prognostic indicator of gastric cancer, including DPM2 (Wei et al., 2021). Similarly, our result showed that DPM2 was a lipid metabolism-related prognostic gene in colorectal cancer using risk model analysis. ACOX1 (Acyl-CoA Oxidase 1) is the first enzyme of the fatty acid beta-oxidation pathway (Ferdinandusse et al., 2007). A total of 10 key genes involved in the esophageal cancer progression were used to constructe a risk model for prediction of survival, including ACOX1 (Wang et al., 2021a). The higher expression levels of ACOX1 were related to poorer prognosis in esophageal squamous cell carcinoma (p = 0.0051), but better prognosis in esophageal adenocarcinoma (p = 0.01). Our results revealed that the high expression of ACOX1 had poorer prognosis in colorectal cancer. The correlation between ACOX1 and overall survival of color or rectal cancer will be investigated in the future. ANGPTL4 (Angiopoietin Like 4) encodes glycosylated protein containing a C-terminal fibrinogen domain (Kim et al., 2000). Overexpression of ANGPTL4 promoted glucose uptake and glycolysis activity in colorectal cancer cells (Zheng et al., 2021). High ANGPTL4 expression was associated with pathological stage and shorter overall survival and disease-free survival in patients with breast cancer (Zhao et al., 2020a). INSR (Insulin Receptor) is a member of receptor tyrosine kinase which mediates the pleiotropic actions of insulin (Kadowaki et al., 1990). NSR rs11668724 G > A exhibited an increased pancreatic cancer risk (OR = 0.89, p = 4.21 × 10−5) (Zhao et al., 2020b). Upregulation of INSR promoted tumorigenesis and metastasis in tongue squamous cell carcinoma (Sun et al., 2018). The effects of insulin were used to enhance the therapeutic effectiveness of chemotherapeutic drugs through downregulation of INSR signaling (Agrawal et al., 2019). ADIPOQ (Adiponectin, C1Q And Collagen Domain Containing) is expressed in adipose tissue exclusively and is involved in metabolic and hormonal processes (Ferguson et al., 2010). ADIPOQ induced cytotoxic autophagy in breast cancer cells. The ADIPOQ rs266729 G/C polymorphism led to low expression levels of adiponectin in CRC. Decreased levels of adiponectin were regarded as risk factor for CRC in metabolic syndrome patients (Divella et al., 2017). ALDH1A3 (Aldehyde Dehydrogenase 1 Family Member A3) catalyzed the formation of retinoic acid and played roles in a diverse range of biological characteristics within cancer stem cells (Hsu et al., 1994; Duan et al., 2016). MMP1 (Matrix Metallopeptidase 1) influenced the progression of uveal melanoma from stage 3 to stage 4 and was correlated with OS and disease-free survival (Wang et al., 2021b). In future studies, it is warranted to investigate the biological functions of these genes in CRC.
There are some limitations in our study. First, there is no relevant experimental verification owing to lack of conditions in our study. All CRC patients were obtained from public datasets. Second, our risk model needs to be evaluated in clinical setting. Large-scale multi-center cohort will be explored in the predictive performance of the lipid metabolism-mediated signature for risk stratification. The prognostic role of the model will be further evaluated in patients with CRC.
A valid and innovative 11-lipid metabolism gene signature model was constructed to predict the prognosis of CRC patients as an independent risk factors, including GGT5, ASAH1, HMGCL, CD36, DPM2, ACOX1, ANGPTL4, INSR, ADIPOQ, ALDH1A3, and MMP1. These dyregulated signature genes were involved in lipid metabolism pathway and Th1 and Th2 cell differentiation. Furthermore, our risk signature was correlated with high infiltration levels of T cells with better prognosis, including Th2 cells, Tregs, and Tgd cells.
Publicly available datasets were analyzed in this study. The names of the repository/repositories and accession number(s) can be found in the article/Supplementary Material.
ZZ: Supervision. F-RZ: Conceptualization. YH: Statistics and Writing-original draft preparation. JZ: Data curation and Writing-reviewing and Editing. HZ: Investigation. NX: Software, Methodology, Validation.
We owe thanks to the public database. We acknowledge the staff of GenePlus (Beijing, China) for their assistance to this study and we acknowledge Pengli Yu for the language editing.
Author JZ was employed by GenePlus-Beijing.
The remaining authors declare that the research was conducted in the absence of any commercial or financial relationships that could be construed as a potential conflict of interest.
All claims expressed in this article are solely those of the authors and do not necessarily represent those of their affiliated organizations, or those of the publisher, the editors and the reviewers. Any product that may be evaluated in this article, or claim that may be made by its manufacturer, is not guaranteed or endorsed by the publisher.
The Supplementary Material for this article can be found online at: https://www.frontiersin.org/articles/10.3389/fgene.2022.989327/full#supplementary-material
Technical details of consensus molecular subtypes analysis. (A) consensus index. (B) K = 2 was regarded as the optimal value in consensus molecular subtypes of colorectal cancer. CDF: Cumulative Distribution Function.
The association between risk score and clinical features, including age, (A) gender (B), and race (C). “≤60”: Patients less than or equal to 60 years of age; “>60”: Patients over 60 years of age.
Agrawal, S., Wozniak, M., Luc, M., Makuch, S., Pielka, E., Agrawal, A. K., et al. (2019). Insulin enhancement of the antitumor activity of chemotherapeutic agents in colorectal cancer is linked with downregulating PIK3CA and GRB2. Sci. Rep. 9 (1), 16647. doi:10.1038/s41598-019-53145-x
Ahluwalia, P., Mondal, A. K., Bloomer, C., Fulzele, S., Jones, K., Ananth, S., et al. (2019). Identification and clinical validation of a novel 4 gene-signature with prognostic utility in colorectal cancer. Int. J. Mol. Sci. 20 (15), E3818. doi:10.3390/ijms20153818
Aran, D., Hu, Z., and Butte, A. J. (2017). xCell: digitally portraying the tissue cellular heterogeneity landscape. Genome Biol. 18 (1), 220. doi:10.1186/s13059-017-1349-1
Bian, X., Liu, R., Meng, Y., Xing, D., Xu, D., and Lu, Z. (2021). Lipid metabolism and cancer. J. Exp. Med. 218 (1), e20201606. doi:10.1084/jem.20201606
Biller, L. H., and Schrag, D. (2021). Diagnosis and treatment of metastatic colorectal cancer: A review. JAMA 325 (7), 669–685. doi:10.1001/jama.2021.0106
Bleve, A., Durante, B., Sica, A., and Consonni, F. M. (2020). Lipid metabolism and cancer immunotherapy: Immunosuppressive myeloid cells at the crossroad. Int. J. Mol. Sci. 21 (16), E5845. doi:10.3390/ijms21165845
Borelli, B., Fontana, E., Giordano, M., Antoniotti, C., Lonardi, S., Bergamo, F., et al. (2021). Prognostic and predictive impact of consensus molecular subtypes and CRCAssigner classifications in metastatic colorectal cancer: A translational analysis of the TRIBE2 study. ESMO Open 6 (2), 100073. doi:10.1016/j.esmoop.2021.100073
Chen, L., Lu, D., Sun, K., Xu, Y., Hu, P., Li, X., et al. (2019). Identification of biomarkers associated with diagnosis and prognosis of colorectal cancer patients based on integrated bioinformatics analysis. Gene 692, 119–125. doi:10.1016/j.gene.2019.01.001
Choi, S., Yoo, Y. J., Kim, H., Lee, H., Chung, H., Nam, M. H., et al. (2019). Clinical and biochemical relevance of monounsaturated fatty acid metabolism targeting strategy for cancer stem cell elimination in colon cancer. Biochem. Biophys. Res. Commun. 519 (1), 100–105. doi:10.1016/j.bbrc.2019.08.137
Chong, W., Shang, L., Liu, J., Fang, Z., Du, F., Wu, H., et al. (2021). m(6)A regulator-based methylation modification patterns characterized by distinct tumor microenvironment immune profiles in colon cancer. Theranostics 11 (5), 2201–2217. doi:10.7150/thno.52717
Clarke, A. R. (2005). Studying the consequences of immediate loss of gene function in the intestine: Apc. Biochem. Soc. Trans. 33 (4), 665–666. doi:10.1042/BST0330665
Clarke, M. F. (2019). Clinical and therapeutic implications of cancer stem cells. Reply. Reply. N. Engl. J. Med. 381 (10), e19. doi:10.1056/NEJMc1908886
Cui, W., Luo, W., Zhou, X., Lu, Y., Xu, W., Zhong, S., et al. (2019). Dysregulation of ketone body metabolism is associated with poor prognosis for clear cell renal cell carcinoma patients. Front. Oncol. 9, 1422. doi:10.3389/fonc.2019.01422
Dariya, B., Aliya, S., Merchant, N., Alam, A., and Nagaraju, G. P. (2020). Colorectal cancer biology, diagnosis, and therapeutic approaches. Crit. Rev. Oncog. 25 (2), 71–94. doi:10.1615/CritRevOncog.2020035067
Das, V., Kalita, J., and Pal, M. (2017). Predictive and prognostic biomarkers in colorectal cancer: A systematic review of recent advances and challenges. Biomed. Pharmacother. 87, 8–19. doi:10.1016/j.biopha.2016.12.064
Dias, A. S., Almeida, C. R., Helguero, L. A., and Duarte, I. F. (2019). Metabolic crosstalk in the breast cancer microenvironment. Eur. J. Cancer 121, 154–171. doi:10.1016/j.ejca.2019.09.002
Divella, R., Daniele, A., Mazzocca, A., Abbate, I., Casamassima, P., Caliandro, C., et al. (2017). ADIPOQ rs266729 G/C gene polymorphism and plasmatic adipocytokines connect metabolic syndrome to colorectal cancer. J. Cancer 8 (6), 1000–1008. doi:10.7150/jca.17515
Douaiher, J., Ravipati, A., Grams, B., Chowdhury, S., Alatise, O., and Are, C. (2017). Colorectal cancer-global burden, trends, and geographical variations. J. Surg. Oncol. 115 (5), 619–630. doi:10.1002/jso.24578
Duan, J. J., Cai, J., Guo, Y. F., Bian, X. W., and Yu, S. C. (2016). ALDH1A3, a metabolic target for cancer diagnosis and therapy. Int. J. Cancer 139 (5), 965–975. doi:10.1002/ijc.30091
Ferdinandusse, S., Denis, S., Hogenhout, E. M., Koster, J., van Roermund, C. W., IJlst, L., et al. (2007). Clinical, biochemical, and mutational spectrum of peroxisomal acyl-coenzyme A oxidase deficiency. Hum. Mutat. 28 (9), 904–912. doi:10.1002/humu.20535
Ferguson, J. F., Phillips, C. M., Tierney, A. C., Perez-Martinez, P., Defoort, C., Helal, O., et al. (2010). Gene-nutrient interactions in the metabolic syndrome: Single nucleotide polymorphisms in ADIPOQ and ADIPOR1 interact with plasma saturated fatty acids to modulate insulin resistance. Am. J. Clin. Nutr. 91 (3), 794–801. doi:10.3945/ajcn.2009.28255
Guo, Y., Sheng, Q., Li, J., Ye, F., Samuels, D. C., and Shyr, Y. (2013). Large scale comparison of gene expression levels by microarrays and RNAseq using TCGA data. PLoS One 8 (8), e71462. doi:10.1371/journal.pone.0071462
Guo, Y., Wang, Y. L., Su, W. H., Yang, P. T., Chen, J., and Luo, H. (2020). Three genes predict prognosis in microenvironment of ovarian cancer. Front. Genet. 11, 990. doi:10.3389/fgene.2020.00990
Haffa, M., Holowatyj, A. N., Kratz, M., Toth, R., Benner, A., Gigic, B., et al. (2019). Transcriptome profiling of adipose tissue reveals depot-specific metabolic alterations among patients with colorectal cancer. J. Clin. Endocrinol. Metab. 104 (11), 5225–5237. doi:10.1210/jc.2019-00461
Heagerty, P. J., Lumley, T., and Pepe, M. S. (2000). Time-dependent ROC curves for censored survival data and a diagnostic marker. Biometrics 56 (2), 337–344. doi:10.1111/j.0006-341x.2000.00337.x
Hsu, L. C., Chang, W. C., Hiraoka, L., and Hsieh, C. L. (1994). Molecular cloning, genomic organization, and chromosomal localization of an additional human aldehyde dehydrogenase gene, ALDH6. ALDH6. Genomics. 24 (2), 333–341. doi:10.1006/geno.1994.1624
Huang, C., and Freter, C. (2015). Lipid metabolism, apoptosis and cancer therapy. Int. J. Mol. Sci. 16 (1), 924–949. doi:10.3390/ijms16010924
Ito, K., and Murphy, D. (2013). Application of ggplot2 to pharmacometric graphics. CPT. Pharmacometrics Syst. Pharmacol. 2, e79. doi:10.1038/psp.2013.56
Johdi, N. A., and Sukor, N. F. (2020). Colorectal cancer immunotherapy: Options and strategies. Front. Immunol. 11, 1624. doi:10.3389/fimmu.2020.01624
Kadowaki, T., Kadowaki, H., Rechler, M. M., Serrano-Rios, M., Roth, J., Gorden, P., et al. (1990). Five mutant alleles of the insulin receptor gene in patients with genetic forms of insulin resistance. J. Clin. Invest. 86 (1), 254–264. doi:10.1172/JCI114693
Kim, I., Kim, H. G., Kim, H., Kim, H. H., Park, S. K., Uhm, C. S., et al. (2000). Hepatic expression, synthesis and secretion of a novel fibrinogen/angiopoietin-related protein that prevents endothelial-cell apoptosis. Biochem. J. 346, 603–610. doi:10.1042/bj3460603
Koncina, E., Haan, S., Rauh, S., and Letellier, E. (2020). Prognostic and predictive molecular biomarkers for colorectal cancer: Updates and challenges. Cancers (Basel) 12 (2), E319. doi:10.3390/cancers12020319
Kondo, Y., Nishiumi, S., Shinohara, M., Hatano, N., Ikeda, A., Yoshie, T., et al. (2011). Serum fatty acid profiling of colorectal cancer by gas chromatography/mass spectrometry. Biomark. Med. 5 (4), 451–460. doi:10.2217/bmm.11.41
Li, C. M., Park, J. H., He, X., Levy, B., Chen, F., Arai, K., et al. (1999). The human acid ceramidase gene (ASAH): Structure, chromosomal location, mutation analysis, and expression. Genomics 62 (2), 223–231. doi:10.1006/geno.1999.5940
Li, J., Condello, S., Thomes-Pepin, J., Ma, X., Xia, Y., Hurley, T. D., et al. (2017). Lipid desaturation is a metabolic marker and therapeutic target of ovarian cancer stem cells. Cell Stem Cell 20 (3), 303–314. e305. doi:10.1016/j.stem.2016.11.004
Li, M., Wang, H., Li, W., Peng, Y., Xu, F., Shang, J., et al. (2020a). Identification and validation of an immune prognostic signature in colorectal cancer. Int. Immunopharmacol. 88, 106868. doi:10.1016/j.intimp.2020.106868
Li, Y., Deng, G., Qi, Y., Zhang, H., Gao, L., Jiang, H., et al. (2020b). Bioinformatic profiling of prognosis-related genes in malignant glioma microenvironment. Med. Sci. Monit. 26, e924054. doi:10.12659/MSM.924054
Lin, D., Fan, W., Zhang, R., Zhao, E., Li, P., Zhou, W., et al. (2021). Molecular subtype identification and prognosis stratification by a metabolism-related gene expression signature in colorectal cancer. J. Transl. Med. 19 (1), 279. doi:10.1186/s12967-021-02952-w
Liu, J., Ma, H., Meng, L., Liu, X., Lv, Z., Zhang, Y., et al. (2021). Construction and external validation of a ferroptosis-related gene signature of predictive value for the overall survival in bladder cancer. Front. Mol. Biosci. 8, 675651. doi:10.3389/fmolb.2021.675651
Liu, X., Bing, Z., Wu, J., Zhang, J., Zhou, W., Ni, M., et al. (2020). Integrative gene expression profiling analysis to investigate potential prognostic biomarkers for colorectal cancer. Med. Sci. Monit. 26, e918906. doi:10.12659/MSM.918906
Menao, S., Lopez-Vinas, E., Mir, C., Puisac, B., Gratacos, E., Arnedo, M., et al. (2009). Ten novel HMGCL mutations in 24 patients of different origin with 3-hydroxy-3-methyl-glutaric aciduria. Hum. Mutat. 30 (3), E520–E529. doi:10.1002/humu.20966
Mika, A., Kobiela, J., Czumaj, A., Chmielewski, M., Stepnowski, P., and Sledzinski, T. (2017). Hyper-elongation in colorectal cancer tissue - cerotic acid is a potential novel serum metabolic marker of colorectal malignancies. Cell. Physiol. biochem. 41 (2), 722–730. doi:10.1159/000458431
Mohammed, M., Mboya, I. B., Mwambi, H., Elbashir, M. K., and Omolo, B. (2021). Predictors of colorectal cancer survival using cox regression and random survival forests models based on gene expression data. PLoS One 16 (12), e0261625. doi:10.1371/journal.pone.0261625
Mooi, J. K., Wirapati, P., Asher, R., Lee, C. K., Savas, P., Price, T. J., et al. (2018). The prognostic impact of consensus molecular subtypes (CMS) and its predictive effects for bevacizumab benefit in metastatic colorectal cancer: Molecular analysis of the AGITG MAX clinical trial. Ann. Oncol. 29 (11), 2240–2246. doi:10.1093/annonc/mdy410
Moore, A. R., Rosenberg, S. C., McCormick, F., and Malek, S. (2020). RAS-Targeted therapies: Is the undruggable drugged? Nat. Rev. Drug Discov. 19 (8), 533–552. doi:10.1038/s41573-020-0068-6
Ose, J., Gigic, B., Brezina, S., Lin, T., Baierl, A., Geijsen, A., et al. (2021). Targeted plasma metabolic profiles and risk of recurrence in stage II and III colorectal cancer patients: Results from an international cohort consortium. Metabolites 11 (3), 129. doi:10.3390/metabo11030129
Pakiet, A., Kobiela, J., Stepnowski, P., Sledzinski, T., and Mika, A. (2019). Changes in lipids composition and metabolism in colorectal cancer: A review. Lipids Health Dis. 18 (1), 29. doi:10.1186/s12944-019-0977-8
Peitzsch, C., Tyutyunnykova, A., Pantel, K., and Dubrovska, A. (2017). Cancer stem cells: The root of tumor recurrence and metastases. Semin. Cancer Biol. 44, 10–24. doi:10.1016/j.semcancer.2017.02.011
Piawah, S., and Venook, A. P. (2019). Targeted therapy for colorectal cancer metastases: A review of current methods of molecularly targeted therapy and the use of tumor biomarkers in the treatment of metastatic colorectal cancer. Cancer 125 (23), 4139–4147. doi:10.1002/cncr.32163
Radenkovic, S., Fitzpatrick-Schmidt, T., Byeon, S. K., Madugundu, A. K., Saraswat, M., Lichty, A., et al. (2021). Expanding the clinical and metabolic phenotype of DPM2 deficient congenital disorders of glycosylation. Mol. Genet. Metab. 132 (1), 27–37. doi:10.1016/j.ymgme.2020.10.007
Rejhova, A., Opattova, A., Cumova, A., Sliva, D., and Vodicka, P. (2018). Natural compounds and combination therapy in colorectal cancer treatment. Eur. J. Med. Chem. 144, 582–594. doi:10.1016/j.ejmech.2017.12.039
Ringel, A. E., Drijvers, J. M., Baker, G. J., Catozzi, A., Garcia-Canaveras, J. C., Gassaway, B. M., et al. (2020). Obesity shapes metabolism in the tumor microenvironment to suppress anti-tumor immunity. Cell 183 (7), 1848–1866. e26. doi:10.1016/j.cell.2020.11.009
Ritchie, M. E., Phipson, B., Wu, D., Hu, Y., Law, C. W., Shi, W., et al. (2015). Limma powers differential expression analyses for RNA-sequencing and microarray studies. Nucleic Acids Res. 43 (7), e47. doi:10.1093/nar/gkv007
Rohrig, F., and Schulze, A. (2016). The multifaceted roles of fatty acid synthesis in cancer. Nat. Rev. Cancer 16 (11), 732–749. doi:10.1038/nrc.2016.89
Savari, S., Vinnakota, K., Zhang, Y., and Sjolander, A. (2014). Cysteinyl leukotrienes and their receptors: Bridging inflammation and colorectal cancer. World J. Gastroenterol. 20 (4), 968–977. doi:10.3748/wjg.v20.i4.968
Smith, J., Su, X., El-Maghrabi, R., Stahl, P. D., and Abumrad, N. A. (2008). Opposite regulation of CD36 ubiquitination by fatty acids and insulin: Effects on fatty acid uptake. J. Biol. Chem. 283 (20), 13578–13585. doi:10.1074/jbc.M800008200
Sun, J., Lu, Z., Deng, Y., Wang, W., He, Q., Yan, W., et al. (2018). Up-regulation of INSR/IGF1R by C-myc promotes TSCC tumorigenesis and metastasis through the NF-κB pathway. Biochim. Biophys. Acta. Mol. Basis Dis. 1864 (5), 1873–1882. doi:10.1016/j.bbadis.2018.03.004
Tran, T. T., Poirier, H., Clement, L., Nassir, F., Pelsers, M. M., Petit, V., et al. (2011). Luminal lipid regulates CD36 levels and downstream signaling to stimulate chylomicron synthesis. J. Biol. Chem. 286 (28), 25201–25210. doi:10.1074/jbc.M111.233551
Wang, F., Zhang, L., Xu, Y., Xie, Y., and Li, S. (2021a). Comprehensive analysis and identification of key driver genes for distinguishing between esophageal adenocarcinoma and squamous cell carcinoma. Front. Cell Dev. Biol. 9, 676156. doi:10.3389/fcell.2021.676156
Wang, J., and Li, Y. (2019). CD36 tango in cancer: Signaling pathways and functions. Theranostics 9 (17), 4893–4908. doi:10.7150/thno.36037
Wang, L. C., Chen, S. H., Shen, X. L., Li, D. C., Liu, H. Y., Ji, Y. L., et al. (2020a). M6A RNA methylation regulator HNRNPC contributes to tumorigenesis and predicts prognosis in glioblastoma multiforme. Front. Oncol. 10, 536875. doi:10.3389/fonc.2020.536875
Wang, S., Su, W., Zhong, C., Yang, T., Chen, W., Chen, G., et al. (2020b). An eight-CircRNA assessment model for predicting biochemical recurrence in prostate cancer. Front. Cell Dev. Biol. 8, 599494. doi:10.3389/fcell.2020.599494
Wang, T., Zhang, Y., Bai, J., Xue, Y., and Peng, Q. (2021b). MMP1 and MMP9 are potential prognostic biomarkers and targets for uveal melanoma. BMC Cancer 21 (1), 1068. doi:10.1186/s12885-021-08788-3
Wang, W., Wang, L., Xie, X., Yan, Y., Li, Y., and Lu, Q. (2021c). A gene-based risk score model for predicting recurrence-free survival in patients with hepatocellular carcinoma. BMC Cancer 21 (1), 6. doi:10.1186/s12885-020-07692-6
Wang, Y., Fang, Y., Zhao, F., Gu, J., Lv, X., Xu, R., et al. (2022). Identification of GGT5 as a novel prognostic biomarker for gastric cancer and its correlation with immune cell infiltration. Front. Genet. 13, 810292. doi:10.3389/fgene.2022.810292
Warburg, O., Wind, F., and Negelein, E. (1927). The metabolism of tumors in the body. J. Gen. Physiol. 8 (6), 519–530. doi:10.1085/jgp.8.6.519
Wei, X. L., Luo, T. Q., Li, J. N., Xue, Z. C., Wang, Y., Zhang, Y., et al. (2021). Development and validation of a prognostic classifier based on lipid metabolism-related genes in gastric cancer. Front. Mol. Biosci. 8, 691143. doi:10.3389/fmolb.2021.691143
Wen, F., Huang, J., Lu, X., Huang, W., Wang, Y., Bai, Y., et al. (2020). Identification and prognostic value of metabolism-related genes in gastric cancer. Aging (Albany NY) 12 (17), 17647–17661. doi:10.18632/aging.103838
Wickham, S., West, M. B., Cook, P. F., and Hanigan, M. H. (2011). Gamma-glutamyl compounds: Substrate specificity of gamma-glutamyl transpeptidase enzymes. Anal. Biochem. 414 (2), 208–214. doi:10.1016/j.ab.2011.03.026
Wilkerson, M. D., and Hayes, D. N. (2010). ConsensusClusterPlus: A class discovery tool with confidence assessments and item tracking. Bioinformatics 26 (12), 1572–1573. doi:10.1093/bioinformatics/btq170
Yang, J., Nie, J., Ma, X., Wei, Y., Peng, Y., and Wei, X. (2019). Targeting PI3K in cancer: Mechanisms and advances in clinical trials. Mol. Cancer 18 (1), 26. doi:10.1186/s12943-019-0954-x
Ye, Z., Zheng, M., Zeng, Y., Wei, S., Huang, H., Wang, Y., et al. (2021). A 13-gene metabolic prognostic signature is associated with clinical and immune features in stomach adenocarcinoma. Front. Oncol. 11, 612952. doi:10.3389/fonc.2021.612952
Yu, X., Yu, B., Fang, W., Xiong, J., and Ma, M. (2021a). Identification hub genes of consensus molecular subtype correlation with immune infiltration and predict prognosis in gastric cancer. Discov. Oncol. 12 (1), 41. doi:10.1007/s12672-021-00434-5
Yu, Y., Kim, H., Choi, S., Yu, J., Lee, J. Y., Lee, H., et al. (2021b). Targeting a lipid desaturation enzyme, SCD1, selectively eliminates colon cancer stem cells through the suppression of wnt and iNOTCH sSignaling. Cells 10 (1), 106. doi:10.3390/cells10010106
Zhang, D., Zheng, Y., Yang, S., Li, Y., Wang, M., Yao, J., et al. (2020). Identification of a novel glycolysis-related gene signature for predicting breast cancer survival. Front. Oncol. 10, 596087. doi:10.3389/fonc.2020.596087
Zhang, L. J., Chen, B., Zhang, J. J., Li, J., Yang, Q., Zhong, Q. S., et al. (2017). Serum polyunsaturated fatty acid metabolites as useful tool for screening potential biomarker of colorectal cancer. Prostagl. Leukot. Essent. Fat. Acids 120, 25–31. doi:10.1016/j.plefa.2017.04.003
Zhang, P., Li, S., Zhang, T., Cui, F., Shi, J. H., Zhao, F., et al. (2021). Characterization of molecular subtypes in head and neck squamous cell carcinoma with distinct prognosis and treatment responsiveness. Front. Cell Dev. Biol. 9, 711348. doi:10.3389/fcell.2021.711348
Zhao, F., Liu, Y., Gu, X., Zhang, B., Song, C., and Cui, B. (2021). Identification of sixteen metabolic genes as potential biomarkers for colon adenocarcinoma. J. BUON 26 (4), 1252–1259. https://www.jbuon.com/archive/26-4-1252.pdf.
Zhao, J., Liu, J., Wu, N., Zhang, H., Zhang, S., Li, L., et al. (2020a). ANGPTL4 overexpression is associated with progression and poor prognosis in breast cancer. Oncol. Lett. 20 (3), 2499–2505. doi:10.3892/ol.2020.11768
Zhao, L. L., Liu, H. L., Luo, S., Walsh, K. M., Li, W., and Wei, Q. (2020b). Associations of novel variants in PIK3C3, INSR and MAP3K4 of the ATM pathway genes with pancreatic cancer risk. Am. J. Cancer Res. 10 (7), 2128–2144.
Zheng, X., Liu, R., Zhou, C., Yu, H., Luo, W., Zhu, J., et al. (2021). ANGPTL4-Mediated promotion of glycolysis facilitates the colonization of fusobacterium nucleatum in colorectal cancer. Cancer Res. 81 (24), 6157–6170. doi:10.1158/0008-5472.CAN-21-2273
Keywords: colorectal cancer, lipid metabolism, signature, prognosis, biomarkers
Citation: Huang Y, Zhou J, Zhong H, Xie N, Zhang F-R and Zhang Z (2022) Identification of a novel lipid metabolism-related gene signature for predicting colorectal cancer survival. Front. Genet. 13:989327. doi: 10.3389/fgene.2022.989327
Received: 08 July 2022; Accepted: 15 August 2022;
Published: 06 September 2022.
Edited by:
Shibiao Wan, St. Jude Children’s Research Hospital, United StatesReviewed by:
Zeguo Sun, Icahn School of Medicine at Mount Sinai, United StatesCopyright © 2022 Huang, Zhou, Zhong, Xie, Zhang and Zhang. This is an open-access article distributed under the terms of the Creative Commons Attribution License (CC BY). The use, distribution or reproduction in other forums is permitted, provided the original author(s) and the copyright owner(s) are credited and that the original publication in this journal is cited, in accordance with accepted academic practice. No use, distribution or reproduction is permitted which does not comply with these terms.
*Correspondence: Fei-Ran Zhang, ZmVpcmFuLnpAMTI2LmNvbQ==; Zhanmin Zhang, em16aGFuZzIwMDhAMTI2LmNvbQ==
Disclaimer: All claims expressed in this article are solely those of the authors and do not necessarily represent those of their affiliated organizations, or those of the publisher, the editors and the reviewers. Any product that may be evaluated in this article or claim that may be made by its manufacturer is not guaranteed or endorsed by the publisher.
Research integrity at Frontiers
Learn more about the work of our research integrity team to safeguard the quality of each article we publish.