- 1Key Laboratory for the Genetics of Developmental and Neuropsychiatric Disorders, Bio-X Institutes, Shanghai Jiao Tong University, Shanghai, China
- 2Shanghai Key Laboratory of Psychotic Disorders, Brain Science and Technology Research Center, Shanghai Jiao Tong University, Shanghai, China
- 3Asbios (Tianjin) Biotechnology Co., Ltd., Tianjin, China
- 4School of Mental Health, Jining Medical University, Jining, China
- 5Out-patient Department of West China Hospital, Sichuan University, Chengdu, China
- 6Mental Health Center of West China Hospital, Sichuan University, Chengdu, China
- 7Shanghai Center for Women and Children’s Health, Shanghai, China
The gut microbiome and host genetics are both associated with major depressive disorder (MDD); however, the molecular mechanisms among the associations are poorly understood, especially in the Asian, Chinese group. Our study applied linear discriminant analysis (LDA) effect size (LEfSe) and genome-wide association analysis in the cohort with both gut sequencing data and genomics data. We reported the different gut microbiota characteristics between MDD and control groups in the Chinese group and further constructed the association between host genetics and the gut microbiome. Actinobacteria and Pseudomonades were found more in the MDD group. We found significant differences in the ACE and Chao indexes of alpha diversity while no discrepancy in beta diversity. We found three associations between host genetics with microbiome features: beta diversity and rs6108 (p = 8.65 × 10–9), Actinobacteria and rs77379751 (p = 8.56 × 10–9), and PWY-5913 and rs1775633082 (p = 4.54 × 10–8). A species of the Romboutsia genus was co-associated with the species of Ruminococcus gnavus in an internetwork through four genes: METTL8, ITGB2, OTULIN, and PROSER3, with a strict threshold (p < 5 × 10–4). Furthermore, our findings suggested that the gut microbiome diversity might affect microRNA expression in the brain and influenced SERPINA5 and other spatially close genes afterward. These findings suggest new linkages between depression and gut microbiome in Asian, Chinese people, which might be mediated by genes and microRNA regulation in space distance.
Introduction
Depression is one of the leading causes of disability, affecting more than 300 million people of all ages globally (Koopman et al., 2017), and it is a common, complex, and debilitating condition with a lifetime prevalence of 20% worldwide (Cai et al., 2020a). However, it is still a challenge to understand the mechanism of depression in pathology (Malhi and Mann, 2018).
The heritability of major depressive disorder (MDD) has been shown by twin and adoption studies (Flint and Kendler, 2014). The risk of depression varied among people with different genetic backgrounds. A meta-analysis identified 44 independent and significant loci, based on genome-wide association studies (GWAS) with 135,458 cases and 344,901 controls (Wray et al., 2018). The importance of genetic heterogeneity in depression has been verified by studies (Lee S. H. et al., 2013; Wray et al., 2018; Cai et al., 2020a; Cai et al., 2020b). Although some results could not coincide exactly, replicable findings of current GWAS helped inform the pathophysiology of major depressive disorder (Howard et al., 2018; Wray et al., 2018; Howard et al., 2021).
A growing body of research has indicated the bidirectional communication between the gut microbiota and the host’s central nervous system (CNS), also known as the microbiome–gut–brain (MGB) axis, which might help elucidate the pathophysiology of depression (Evrensel and Tarhan, 2021; Simpson et al., 2021). The alpha diversity and beta diversity showed differences in patients with the major depressive disorder from control groups (Liu et al., 2016; Chen Z. et al., 2018; Huang et al., 2018; Chung et al., 2019). Actinobacteria and Bacteroidetes at the phylum level commonly showed significant differences between the depressive disorders and controls (Rong et al., 2019; Dong et al., 2021; Simpson et al., 2021). Although the exact mechanism of the MGB axis has not been clarified, some processes associated with the immune system, metabolites, the endocrine system, and neuronal systems have already been reported (Dinan and Cryan, 2015; Rogers et al., 2016; Cenit et al., 2017; Waclawikova and El Aidy, 2018; Martins-Silva et al., 2021).
The gut microbiota composition could be modulated by intrinsic host factors, and the microbial GWAS have demonstrated different microbiome–host genotype associations (Weissbrod et al., 2018; Dubois et al., 2019). The association between Actinobacteria and rs4988235 (a locus in the LCT-MCM6-ZRANB3 region), a typical example, was verified by high-level evidence, and meanwhile some other associations were reported in the same article (Qin et al., 2022). Although the association was supported by many sources of evidence, the mechanisms underlying the association are not fully understood.
microRNA (miRNA) was regarded as a mediator between the gut microbiome and intrinsic host factors. The expression of miR-294-5p, targeting some key genes in the kynurenine pathway, showed a difference between germ-free (GF) male mice and conventional mice, which was further normalized following colonization (Moloney et al., 2017). In the amygdala, the expression of miR-183-5p and miR-182-5p decreased in GF mice and was subsequently restored upon recolonization (Stilling et al., 2018). Furthermore, the miRNA expression in the brain and the gut microbiome is associated with depression or anxiety (Rosa et al., 2022). Antidepressant treatment for 8 weeks leads to increased plasma levels of miR-144-5p in patients with depression or anxiety compared to their pre-treatment baseline levels (Wang et al., 2015). Studies have researched the roles of miRNAs and microbiome in psychiatric disorders such as anxiety and depression (Meydan et al., 2016; Allen and Dwivedi, 2020; Huang and Wu, 2021).
This study aimed to construct the association between host genetics and the gut microbiome among MDD patients in the Asian group and find some hints of the specific mechanism underlying the association.
Materials and methods
Subjects
Patients with first-episode MDD and health controls (HCs) were recruited for this study. The participants were hospitalized patients recruited from the Mental Health Center, West China Hospital, Sichuan University, from January 2019 to October 2019. All samples were from Chengdu, Sichuan, China. We selected subjects with a normal body mass index (BMI, 18.5–22.9) to avoid bias, which might be caused by the influence of body weight on the intestinal flora.
The patients were diagnosed according to the Structured Clinical Interview for DSM-IV (SCID) by two psychiatrists (Aizawa et al., 2016). Patients aged less than 18 years or older than 45 years with organic etiology for their psychiatric symptoms, psychotic features, or intellectual disability were excluded.
According to the SCID, the HCs were 30 worker volunteers (aged 18–45 years), diagnosis of mental disorder was excluded by two psychiatrists, and their 24-item Hamilton Depression Rating Scale (HAMD-24) score was less than 7.
To further exclude the influence of physical diseases, subjects with the following diseases were excluded from our study: cardiovascular diseases (e.g., hypertension); digestive diseases (e.g., liver cirrhosis, irritable bowel syndrome, or inflammatory bowel disease); endocrine and metabolic diseases (e.g., diabetes mellitus, obesity, or fatty liver disease); drug or alcohol abuse; use of antibiotics, probiotics, prebiotics, or symbiotics during the 6 months before collection of fecal samples; known active bacterial, fungal, or viral infections; and obvious dietary preferences (e.g., vegetarians). A total of 51 eligible MDD patients and 30 HCs were recruited.
Fecal sample collection and DNA extraction
Fecal samples were immediately frozen on collection in a sterile plastic cup and stored at −80°C before analysis. Microbial genomic DNA was extracted using the QIAamp DNA Stool Mini Kit according to the manufacturer’s instructions (Qiagen, Hilden, Germany). The 16S rRNA V3-V4 amplicons were generated using the National Institutes of Health (NIH) Human Microbiome Project protocols (16S 454 Sequencing Protocol HMP Consortium; https://www.hmpdacc.org). The V3–V4 region of the bacteria 16S ribosomal RNA genes was amplified PCR.
16S rRNA gene sequencing analysis
Libraries were prepared and subjected to paired-end sequencing with Illumina MiSeq by following Illumina’s standard protocol (Bartram et al., 2011). These QIIME2 16S rRNA sequencing protocols were used to pick and analyze operational taxonomic units (OTUs) (Kuczynski et al., 2012). Sequences from this project were deposited in the NCBI Short Read Archive under BioProject ID PRJNA647236.
Bioinformatics and statistical analysis for gut microbiota
The sequence index file was used to identify and extract the sample data saved in the FASTQ format. Barcodes and the primers at the beginning and the end were used to identify and select sequence reads. The sequence number of each sample was normalized, and OTUs with 97% identity thresholds were used by the UPARSE (version 7.1 http://drive5.com/uparse/) software program. Chimeric sequences were identified and removed using UCHIME (version 4.1 http://drive5.com/uchime/). The taxonomy of each 16S rRNA gene sequence was analyzed by RDP Classifier (http://rdp.cme.msu.edu/) using the SILVA (SSU 138) 16S rRNA database at a confidence threshold of 70%. The further pathway abundances were predicted using PICRUSt2 (Douglas et al., 2020). Gut-microbiota-specific microbial characteristics (pathways or taxa) were analyzed using the linear discriminant analysis (LDA) effect size (LEfSe) method (http://huttenhower.sph.harvard.edu/galaxy/), which emphasizes both statistical significance and biological relevance.
Statistical analyses were performed using SPSS version 21(SPSS, Chicago, IL, United States). The t-test was used to compare the continuous variables following normal distribution. The Wilcoxon test was chosen for variables not following the normal distribution.
The α-diversity was calculated by the ACE, Chao, Simpson, and Shannon indexes. The β-diversity was calculated using the Bray–Curtis index as the distance method and reported according to principal co-ordinates analysis (PCoA) and non-metric multidimensional scaling (NMDS).
Host SNP genotyping and GWAS quality control
Genomic DNA materials were extracted using QIAamp DNA Blood Mini Kit (51106, Qiagen, Hilden, Germany) from the peripheral blood of patients. WES was performed with the sequencing platforms (Genesky Biotechnologies, Shanghai, China, and WuXi NextCODE, Shanghai, China). The total WES depth was 100×, and the filtering procedure was performed in accordance with the manners reported previously (Luan et al., 2021). Sequencing data were annotated according to the GRCh37/hg19 reference genome. All MDD samples were genotyped with SNP arrays intending to provide exon-wide coverage of common variation. GWAS quality control and imputation were performed according to published PGC procedures (Wray et al., 2018). GWAS quality control was conducted separately for each sample. SNPs were removed for missingness ≥0.02, case–control difference in SNP missingness ≥0.02, gender difference (male ≥0.9, female ≥0.2), minor allele frequency ≤0.05, linkage disequilibrium (r2) <0.5, deprivation Hardy–Weinberg equilibrium test in controls <1 × 10–6, PIHAT values > 0.2, and heterozygosity deviation of three standard deviations.
Genome-wide association analysis of gut microbiome features
We calculated the genetic principal components of ancestry from genome-wide genetic variants to estimate the population structure. PLINK 1.9 was used to perform a logistic regression model for taxa present in fewer than 90% of participants, adjusted for age, sex, and the first five genetic principal components of ancestry. For the taxa present in no fewer than 90% of participants, different pathways, and alpha diversity, we retained the quantitative value for analysis. The association assessment was fitted with the five genetic principal components of ancestry, age, and sex. Alpha diversity was calculated after randomly sampling 10,000 reads per sample.
The analysis for the genome-wide host genetic variants with beta diversity was performed using MicrobiomeGWAS (Hua et al., 2022), adjusted for covariates including the first five genetic principal components of ancestry, age, and sex.
The GWAS results were used to construct the network between genes and microbes or pathways. The lines in the constructed network represented the significant association between genes and microbes based on the p-values of the association test.
microRNA–gene interactions
The data on miRNAs and target genes were obtained from miRTarBase (Huang et al., 2022). The database has accumulated >2,200,449 verified miRNA–target interactions (MTIs) from 13,389 manually curated articles and CLIP-seq data. An optimized scoring system is adopted to enhance this update’s critical recognition of MTI-related articles and corresponding disease information.
Chromatin 3D model for associated genes
A chromatin 3D model based on molecular dynamics was used to discover the distance among genes in the nucleus center (Shi et al., 2020). The Euclidean distance was calculated to assess the possible interaction (closer proximity means more likely to influence each other). The 3D coordinate conversion was based on the Hi-C dataset of human embryonic stem cells (hESCs) with a resolution of 500 kb (bin size). We defined a close distance with means (M) minus 2 standard deviations (SD).
Ethical approval statement
All procedures that contributed to this study complied with the ethical standards of national and institutional committees on human experimentation and with the Declaration of Helsinki (1975), as revised in 2008. All procedures that involved human subjects and patients were approved by the Ethics Committee of West China Hospital (WCH) of Sichuan University (approval number: 2019-268). All participants signed an informed consent form after appropriate oral information and explanation were provided.
Results
Gut abundance differs between depression and healthy cohorts
The demographic details are shown in Supplementary Table S1. Sequencing the V3–V4 regions of the 16S rRNA gene generated approximately 70.6 million sequences (median ± MAD): ∼51211 ± 6148 sequence reads per subject, and the denoised dataset contained 1249 unique sequences, covering 26 phyla, 55 classes, 118 orders, 214 families, 466 genera, and 671 identified species. The general overview of the abundance at the order and genus levels is shown in Figures 1A, B.
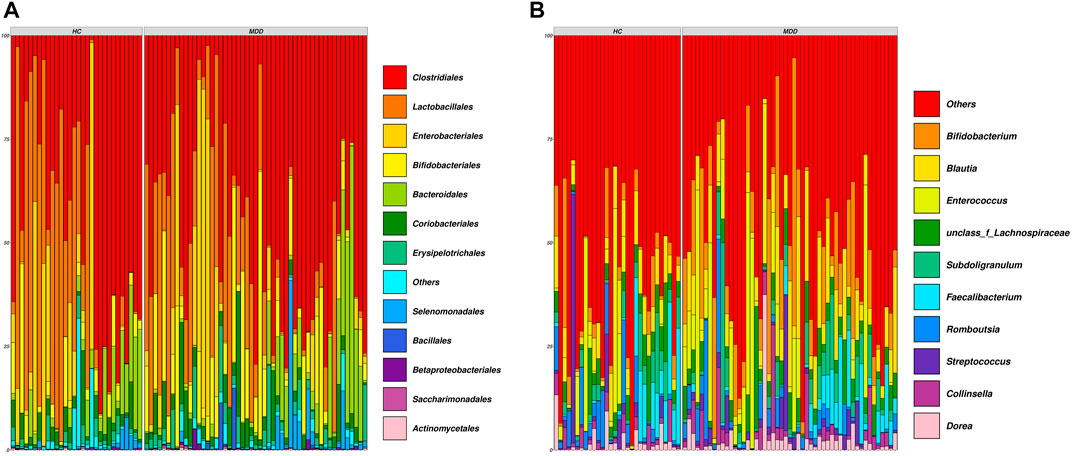
FIGURE 1. Composition of all samples in the cohort. (A) Family-level composition of samples. (B) Genus-level composition of samples. Each vertical line indicates one sample.
At the order level, Clostridiales, Lactobacillales, Enterobacteriales, and Bifidobacteriales were rich in both groups and showed no significance between groups. Actinobacteria and Pseudomonadales in the MDD group were higher than those in the HC group, while Rhizobiales, Mollicutes_RF39, Tenericutes, and Mollicutes were less in the MDD group (Figure 2A).
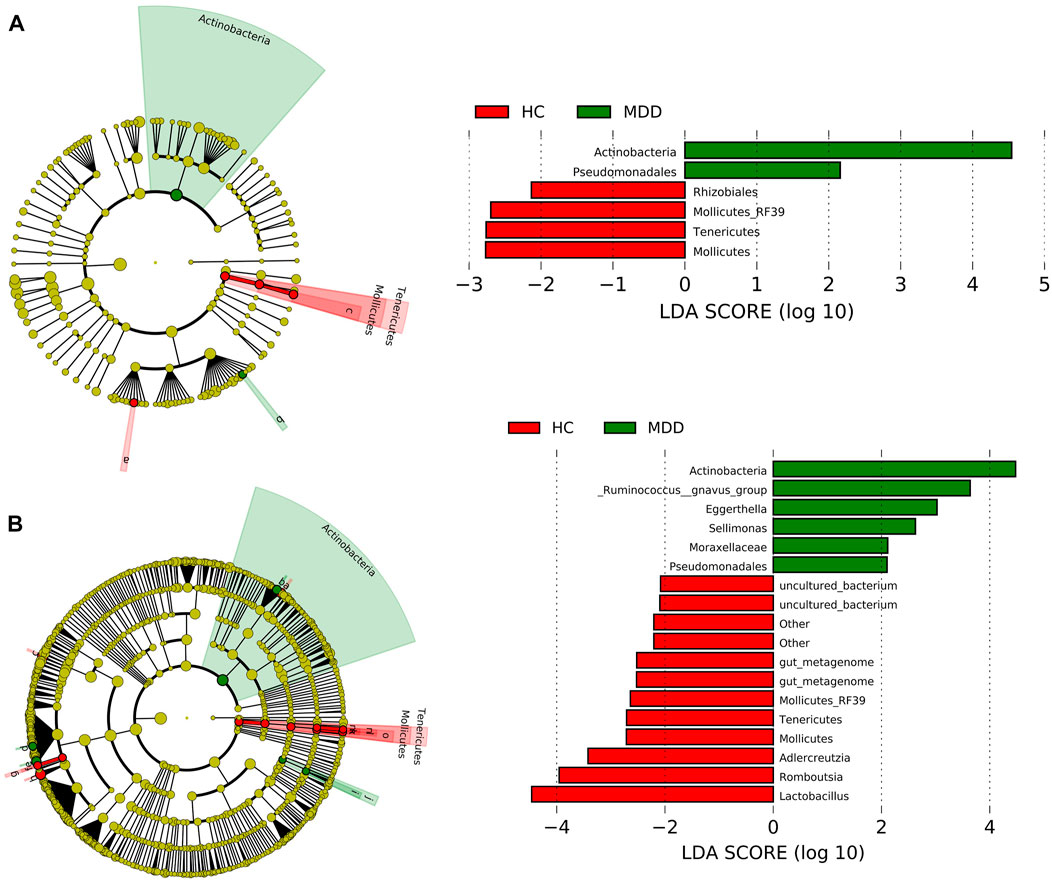
FIGURE 2. Linear discriminant analysis effect size (LEfSe) histograms and the cladograms for the comparisons of major depressive disorder (MDD) versus healthy controls (HCs). (A) LEfSe results at the order level. (B) LEfSe results at the genus level.
At the genus level, seven contents were higher in the MDD group, while 11 contents were higher in the control group (Figure 2B). Actinobacteria at the genus level showed the highest LDA score, 4.59 (p = 0.021), which indicated significant richness of Actinobacteria in the MDD group compared to the control group. Lactobacillus got the lowest LDA score, -4.42 (p = 0.037).
The structure of the depressed microbiome in individuals with depression differs from the control subjects
Local diversity (α-diversity) could be used to investigate the structure of the gut microbiome. The community richness and diversity indexes, including Chao, ACE, Shannon, and Simpson indexes, were calculated to estimate α-diversity. The ACE and Chao indexes were significantly higher in healthy groups (p = 0.010; p = 0.026), while the Shannon and Simpson indexes showed no difference (Figure 3A).
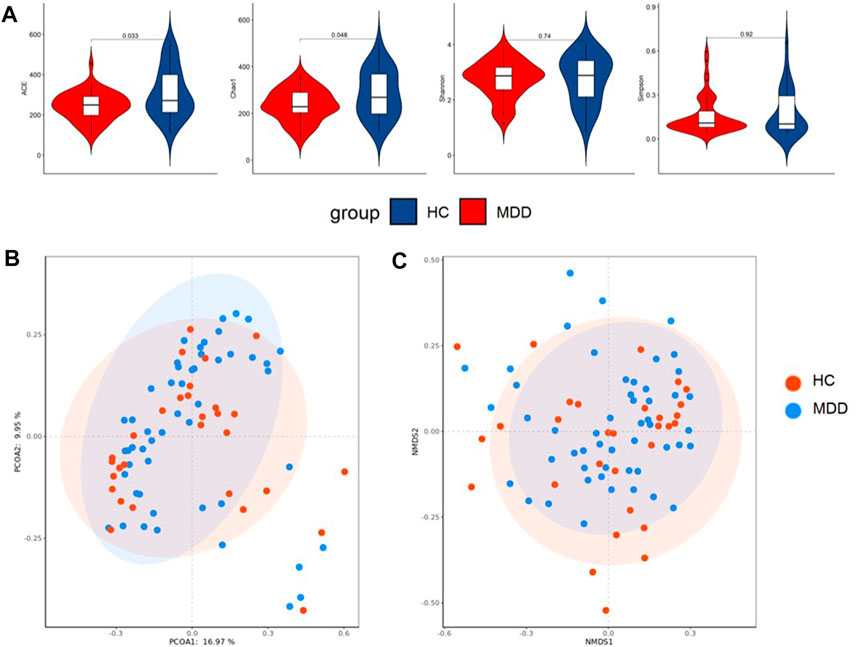
FIGURE 3. (A) Difference in alpha diversity between MDD and HC. (B) Result of principal co-ordinates analysis (PCoA) of beta diversity. (C) Result of non-metric multidimensional scaling (NMDS) of beta diversity.
PCoA and NMDS were used to test and visualize the difference in beta diversity between the control and case groups. Figure 3B indicates that PCoA showed no significant clustering between the cohorts (p = 0.143), and NMDS (Figure 3C) performed similar results.
The pathways predicted differ between depression and healthy cohorts
The pathways were predicted using PICRUSt2. The differences in the pathways are shown in Figure 4. Five pathways were much more enriched in MDD: PWY-5384, PWY-1061, PWY-5913, HSERMETANA-PWY, and OANTIGEN-PWY. The most enriched pathway in MDD was PWY-5384 (the LDA score was 2.792, p = 0.002), which involved sucrose phosphorylase and indicated a discrepant use of energy. There were two pathways which were much more abundant: RIBOSYN2-PWY and PWY-7013, with LDA scores of -2.69 (p = 0.005) and -2.69 (p = 0.033), respectively. The correlation between significantly different pathways and microbiota is shown in Supplementary Figure S1, and 54 microbes at different levels were significantly correlated with the predicted pathways (74 in total).
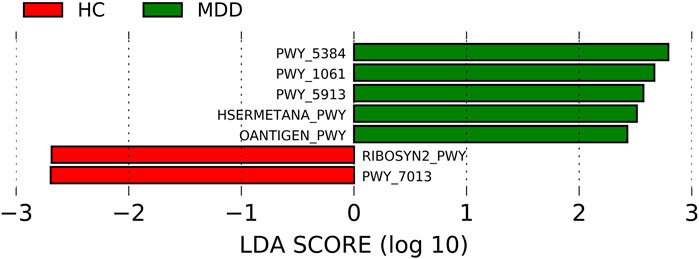
FIGURE 4. Linear discriminant analysis effect size (LEfSe) histograms for the comparisons of major depressive disorder (MDD) versus healthy controls of pathways.
Association of host genetics with gut microbiome
To examine the association of host genetic variants with alpha diversity, we performed GWAS for four indexes, but no genome-wide significant signal (p < 5 × 10–8) was found. The beta diversity GWAS was performed using MicrobiomeGWAS based on Bray–Curtis dissimilarity. We found that one locus was associated with beta diversity at a genome-wide significant level (Figure 5): rs6108 was associated with beta diversity with a p-value of 8.65 × 10–9. The rs6108 is a three prime UTR variant, at SERPINA5 gene. We subsequently performed discovery GWAS for individual gut microbes, which were significantly different. We found that only Actinobacteria, at the phylum level, was associated with host genetic variants (Figure 5) in one locus at the IFNL3 gene (rs77379751; p = 8.56 × 10–9). The variant at rs77379751 is a missense variant with a deleterious function and predicts scores in both ROVEN (-6.88, cutoff = -2.5) and SIFT (0.02, cutoff = 0.05). Further association was explored between different pathways and genetics. We found that only PWY-5913 was associated with CYP39A1 gene at the rs1775633082 locus (p = 4.536 × 10–8), passing the genome-wide test (Figure 5). The details of the genomic inflation factor for the GWAS data are shown in Supplementary Table S2.
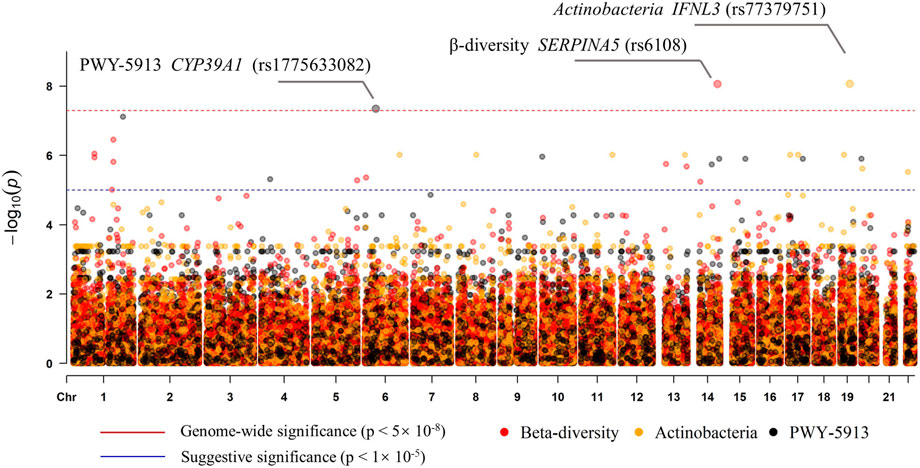
FIGURE 5. Manhattan plot of the associations with SNPs and the features (beta diversity, Actinobacteria, and PWY-5913).
The internetwork of genes and microbes or pathways
To further find the exact interaction between microbes/pathways and genes, we constructed the network between SNPs and microbes at the species level and the network between SNPs and pathways. We cannot find the connectivity at a rigorous level (p < 5 × 10–8). Considering the sample size, we took an effort at two suggestive significances (p < 5 × 10–4 and p < 1 × 10–3) to explore possible networks. A species (uncultured bacterium) of the Romboutsia genus was co-associated with another uncultured species of Ruminococcus gnavus, through METTL8 (rs3731986), ITGB2 (rs3746973), OTULIN (rs16903631), and PROSER3 (rs35178229), at a strict threshold (p < 5 × 10–4). While with a loose threshold (p < 1 × 10–3), three species ( belonging to Romboutsia genus, Ruminococcus gnavus, and Eggerthella genus) were co-associated through 12 genes at 13 loci (Figure 6A). The connectivity between the species of Ruminococcus gnavus and Eggerthella genus was only through USP28 (rs2465647). PWY-5384, PWY-1061, HSERMETANA-PWY, and OANTIGEN-PWY were co-associated through seven genes (Figure 6B), at the loose threshold (p < 1 × 10–3). Only PWY-5384 and OANTIGEN-PWY were co-associated with rs1644600 (a locus of GSG1L gene).
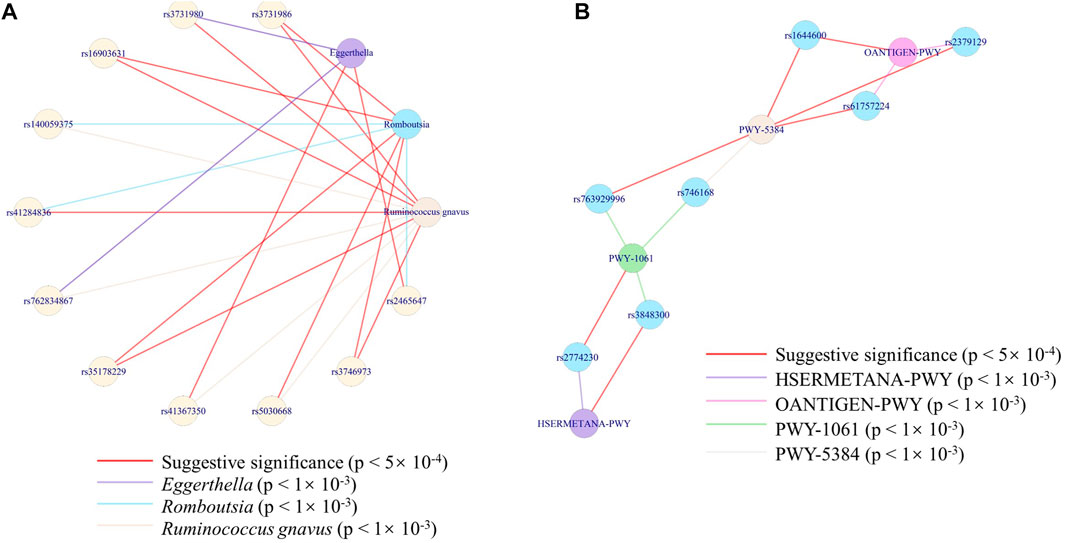
FIGURE 6. Network of the associations among SNPs and the features. (A) Network of the associations among three species with 12 genes at 13 loci. (B) Network of the associations among four pathways with six loci.
MiRNA–gene network based on a close 3D distance
We searched the microbe-associated genes (IFNL3 and SERPINA5) to explore whether they interacted with miRNAs that were reported with depression/anxiety and microbiota. SERPINA5 showed a target of mmu-miR-3095-3p, which was reported to be associated with anxiety-like behavior and microbiota (Rosa et al., 2022), while IFNL3 was not. Following the interaction, we caught the targets of mmu-miR-3095-3p (737 targets in miRTarBase). Considering the possibility of interaction, we screened the targets based on a defined close distance (M-2SD) of the 3D model. Eight genes were finally left: EGLN3, FNDC3A, MAP3K9, NFATC4, OTUB2, SERPINA5, SUPT16H, and TMEM179. Also, all eight genes were found as at least one target of miRNAs which were reported to be associated with depression/anxiety and microbiota (Figure 7).
Discussion
Our study showed the difference in microbiota between the patients with major depression disorder (MDD) and the controls. The phylum Actinobacteria was higher in MDD, which was consistent with prior results (Chen J. J. et al., 2018; Chen Z. et al., 2018; Chung et al., 2019; Lai et al., 2021). Some researchers reported that Bacteroidetes were higher in MDD (Jiang et al., 2015; Liu et al., 2016). At the order level, our results showed that Pseudomonades were higher in MDD, while other studies found results more on Enterobacteriaceae, despite the findings were not same (Jiang et al., 2015; Chen Z. et al., 2018). At the genus level, Actinobacteria was higher in MDD with the highest LDA score in our study, which was consistent with previous studies (Chung et al., 2019; Simpson et al., 2021).
As for alpha diversity, our results showed no difference in the Shannon index and Simpson index between MDD and control groups. Similarly, some studies found no difference between the MDD and control groups across all examined alpha diversity indexes (Chahwan et al., 2019; Mason et al., 2020), while some indicated that lower alpha diversity was found in depressive disorders using the Shannon index (Liu et al., 2016; Huang et al., 2018). The community richness was estimated by the Chao index and ACE index in our study, and both of them were significantly lower in the MDD group than in the control group, which was consistent with the previous results (Huang et al., 2018; Rong et al., 2019). Previous studies indicated that there would be significant differences in beta diversity between the MDD and controls, as shown by group clustering on PCoA (Chen Z. et al., 2018; Chung et al., 2019). However, we found no significant difference between the MDD and control groups using both PCoA and NMDS. Our findings were consistent with some other studies (Chahwan et al., 2019; Mason et al., 2020). On the whole taxa difference, our results were verified by previous results and gave some new findings. Based on the 16S rRNA data, predicted pathways also suggested some important insights. The differences in predicted pathways indicated the possible special mechanism of metabolism in the MDD group. The enriched pathways in MDD involved homogalacturonan biosynthesis, sucrose degradation, l-methionine biosynthesis, O-antigen building blocks biosynthesis, and the TCA cycle, which suggested a high level of glycometabolism and energy utilization. Some researchers have reported similar different factors of metabolic processes and emphasized the importance of the favorable metabolic status (Penninx and Lange, 2018; Wang et al., 2022). The significant correlations between predicted pathways and microbes were among 54 of 74 microbes at different levels, which showed that the key microbes were linked with key pathways. However, the correlation between PWY-5913 and microbes showed non-significance, which indicated that the functional-predicted pathways reflected an overall difference. The results suggested that microbiota could act not only through key individuals but also by affecting functional pathways as a whole.
The results of the association between microbiome characteristics and host genetic information indicated some specific links in the East Asian people. Our study used the threshold of p < 5 × 10–8 for the rigorous test, while the analysis discussed the nominal significance (p < 0.05) and suggestive significance (p < 5 × 10–5) to find results (Xu et al., 2020).
The association between Actinobacteria (at the phylum level) and rs77379751 (locus in the IFNL3 gene) showed a deleterious prediction score in both ROVEN and SIFT. IFNL3 gene encodes IFN-λ3 protein, which is the one of the members of the type Ⅲ IFN family (Lasfar et al., 2016). It is reported that IFN-λ could play a crucial part in immune mechanisms that protect mucosal surfaces from pathogenic microbes by directly interacting more specifically with epithelial cells (Durbin et al., 2013; Mahlakoiv et al., 2015). The association between IFN-λ and depression is rarely reported. The IFN family, especially IFN-α and IFN-γ, is commonly associated with neuroinflammation in depression. Our results showed a possible link between IFN-λ3 and depression, mediated by gut microbiome characteristics.
The association between beta diversity and the locus rs6108 indicated that genetic heterogeneity would influence gut microbiota in some way. Prior literature has reported some genetic variants that were associated with beta diversity, among which we found no significant association (p < 5 × 10–8), and these associations in our study could either survived Bonferroni correction (Bonder et al., 2016; Wang et al., 2016; Rothschild et al., 2018; Ruhlemann et al., 2018; Xu et al., 2020). For the various methods for sequencing and calculation of beta diversity, it is a challenge to verify and extrapolate the results across populations.
SERPINA5 was indicated to be associated with beta diversity of gut microbiota. SERPINA5 is a member of serpins. It was originally identified as an inhibitor of anticoagulant protease-activated protein C (aPC), and later it was shown with broad protease reactivity (Marlar and Griffin, 1980; Suzuki et al., 1983; Espana et al., 1989; Yang and Geiger, 2017). It is most mentioned that SERPINA5 plays a role in hemostasis, male fertility, and cancer protection (Carroll et al., 1997; Elisen et al., 1998; Uhrin et al., 2000; Bijsmans et al., 2011; Lee E. K. et al., 2013; Jing et al., 2014). Only a few studies reported that SERPINA5 had antimicrobial activity, which had been attributed to heparin-binding helix H (Malmstrom et al., 2009; Papareddy et al., 2014). However, few data in vivo supported the role of SERPINA5 in the defense against bacteria (Yang and Geiger, 2017). SERPINA5 was shown to be expressed in the hypothalamus (https://www.proteinatlas.org/ENSG00000188488-SERPINA5/brain), which might indicate a role in the hypothalamic–pituitary–adrenal (HPA) axis. It is reported that SERPINA5 was associated with Alzheimer’s disease (AD) and Parkinson’s disease (PD) (Hurley et al., 2015; Crist et al., 2021). Although the linkage between AD and PD was indicated, we found no results which suggested that SERPINA5 was associated with depression or gut microbiota.
Some studies reported that other proteins in the serpin family might be related to depression. SERPINA6 was involved in HPA axis regulation and was associated with antenatal and postpartum depression (Skalkidou et al., 2019). SERPINE1 and SERPING1 were suggested crucial in the promotion of depression in patients with ovarian cancer (Yi et al., 2019). The association between the serpin family and the gut microbiome is not clear. A report hypothesized that SERPINB5 (also known as maspin) might play a role in dysregulating the intestinal microbiota and inducing idiopathic inflammatory bowel disease (IBD)-related colorectal carcinoma (Gurzu and Jung, 2021). We all know about the linkage of inflammation with gut microbiota, but there was little evidence to show us the exact way that the serpin family works.
The network of microbiota and genes showed a complex regulation in the depression group. We found the co-association with four genes between the Romboutsia genus and Ruminococcus gnavus at the strict threshold (p < 5 × 10–4). It is reported that ITGB2 had a powerful influence on immune cell infiltration into the acute myeloid leukemia (AML) tumor microenvironment (Wei et al., 2021). OTULIN, coding the M1-specific deubiquitinase, is essential for preventing TNF-associated systemic inflammation in humans and mice and critical for restraining life-threatening spontaneous inflammation and maintaining immune homeostasis (Damgaard et al., 2016; Hoste et al., 2021). The co-association between IGTB2 and OTULIN of Romboutsia genus and Ruminococcus gnavus might influence depressive symptoms through a key role in immunization and inflammation. We found less evidence that METTL8 and DCAF17 were involved in the depression and complex microbiota interaction. The low significance of some connections suggested that the association could only play a weaker effect, and we should pay much attention to the cumulative effect.
As for the associations between predicted pathways and genes, we would like to emphasize the linkage between CYP39A1 and PWY-5913. PWY-5913 was partial of the TCA cycle and commonly involved with energy utilization (Anderson et al., 2018; Linghu et al., 2022). Our results have shown no significant correlation between PWY-5913 and significantly different microbes, which indicated that this different pathway uncovered a whole-level difference. Meanwhile, CYP39A1 encoded a member of the cytochrome P450 superfamily of enzymes and played an important role in tumor progression (Khenjanta et al., 2014; Ji et al., 2022) and neurodegenerative disorder (Vishweswaraiah et al., 2022). CYP39A1 might affect a variety of microbes and finally show the difference in the PWY-5913 pathways. This association might indicate that a differentiated metabolism in MDD contributed to the depression symptom, which was consistent with the previous results (Zheng et al., 2016; Liu et al., 2021). It should be pointed out that these results were based on the prediction through 16S rRNA data, and the metabolism data needed further verification.
The specific connection of gut microbiota, genes, miRNAs, and depression or anxiety could be a new suggested way to improve symptoms. We found that SERPINA5 was a target gene of mmu-miR-3095-3p, which is associated with the anxiety-like behavior (Chen et al., 2017). The authors found that compared to SPF mice, the mmu-miR-3095-3p in the hippocampus of germ-free mice was significantly downregulated, and the changes could be reversed following colonization. We emphasized the association between beta diversity of gut microbiota and SERPINA5, and the reported changes in mmu-miR-3095-3p were also related to gut colonization. Furthermore, the linkage between SERPINA5 and mmu-miR-3095-3p was also reported. These findings construct a potential way that SERPINA5 might be affected by mmu-miR-3095-3p, in turn influencing the gut microbiome, as a result of depression or anxiety-like behavior. The similar pathogenesis could not be found in IFNL3.
Furthermore, the miRNA–gene interaction showed a potential connection associated with space distance. Restricted by the space distance, we screened eight genes that could be the target of mmu-miR-3095-3p, and all the genes could be at least a target of miRNAs, which were reported to be associated with microbiota and depression/anxiety (Rosa et al., 2022). The results suggested a potential mechanism that miRNAs affected by microbiota in depression/anxiety showed a spatial specific effect, which indicated that specific space areas of chromatin might co-effect microbiome-associated depression/anxiety.
In conclusion, we reported the replicable results of the difference between the MDD patients and the control group and constructed the specific association of genes, miRNAs, microbiome, and depression/anxiety. Accumulated studies have demonstrated that gut microbiota and genes can influence depression by a bidirectional path, while our results give some original points. The specificity of microbiota in depression could be associated with fixed genes, and the genes might show spatial proximity, which indicated new points in the pathway for the potential treatment of depression. It is important that the key genes and microbiota among the pathways support a probability of personalized treatment of depression and could help researchers better understand the specific therapeutic targets.
We used 51 cases and 30 controls to find out the distinction between the gut microbiome and utilized only 51 cases to construct associations between the gut microbiome and genetic heterogeneity, which is considered a limitation to this study. It is also limited by the scale of cases and the results need larger research on the East Asian people for further verification. Due to the lack of data on diet and exercise, we were unable to further determine what roles they played in microbiota and depression. The deep exploration of how depression or anxiety was influenced by the network of genes and microbiota and the genes and miRNAs still need further studies.
Data availability statement
The original contributions presented in the study are publicly available. These data can be found at: PRJNA647236.
Ethics statement
The studies involving human participants were reviewed and approved by the Ethics Committee of West China Hospital (WCH) of Sichuan University (approval number: 2019-268). The patients/participants provided their written informed consent to participate in this study.
Author contributions
KH conceived the hypothesis, developed the methodology, conducted the survey, collected the data, conducted the analysis, and drafted the manuscript. LJ participated in data collection and critically reviewed manuscript drafts. CW, YS, CC, LL, XW, FY, and MF participated in the study design and critically reviewed manuscript drafts. LL and YS reviewed the data analysis. SY, TY, ZD, and QX participated in survey conduction and data collection. LH and XL assessed the methodological quality of the included studies and critically reviewed manuscript drafts. GH conceptualized the research question, supervised KHs involvement, assessed the methodological quality, and critically reviewed manuscript drafts. All authors read and approved the final manuscript.
Funding
This project was supported by the National Key Research and Development Program (2016YFC0906400), the Innovation Funding in Shanghai (20JC1418600 and 18JC1413100), the National Natural Science Foundation of China (82071262 and 81671326), the Natural Science Foundation of Shanghai (20ZR1427200 and 20511101900), the Shanghai Municipal Science and Technology Major Project (2017SHZDZX01), the Shanghai Leading Academic Discipline Project (B205), the Technology Department of Sichuan Province (2022YFS0350), the Capacity Building Planning Program for Shanghai Women and Children’s Health Service, and the collaborative Innovation Center Project Construction for Shanghai Women and Children’s Health.
Conflict of interest
Author YS was employed by the company Asbios (Tianjin) Biotechnology Co., Ltd.
The remaining authors declare that the research was conducted in the absence of any commercial or financial relationships that could be construed as a potential conflict of interest.
Publisher’s note
All claims expressed in this article are solely those of the authors and do not necessarily represent those of their affiliated organizations, or those of the publisher, the editors, and the reviewers. Any product that may be evaluated in this article, or claim that may be made by its manufacturer, is not guaranteed or endorsed by the publisher.
Supplementary material
The Supplementary Material for this article can be found online at: https://www.frontiersin.org/articles/10.3389/fgene.2022.976814/full#supplementary-material
References
Aizawa, E., Tsuji, H., Asahara, T., Takahashi, T., Teraishi, T., Yoshida, S., et al. (2016). Possible association of Bifidobacterium and Lactobacillus in the gut microbiota of patients with major depressive disorder. J. Affect. Disord. 202, 254–257. doi:10.1016/j.jad.2016.05.038
Allen, L., and Dwivedi, Y. (2020). MicroRNA mediators of early life stress vulnerability to depression and suicidal behavior. Mol. Psychiatry 25 (2), 308–320. doi:10.1038/s41380-019-0597-8
Anderson, N. M., Mucka, P., Kern, J. G., and Feng, H. (2018). The emerging role and targetability of the TCA cycle in cancer metabolism. Protein Cell 9 (2), 216–237. doi:10.1007/s13238-017-0451-1
Bartram, A. K., Lynch, M. D. J., Stearns, J. C., Moreno-Hagelsieb, G., and Neufeld, J. D. (2011). Generation of multimillion-sequence 16S rRNA gene libraries from complex microbial communities by assembling paired-end Illumina reads. Appl. Environ. Microbiol. 77 (15), 5569. doi:10.1128/Aem.05896-11
Bijsmans, I. T. G. W., Smits, K. M., de Graeff, P., Wisman, G. B. A., van der Zee, A. G. J., Slangen, B. F., et al. (2011). Loss of SerpinA5 protein expression is associated with advanced-stage serous ovarian tumors. Mod. Pathol. 24 (3), 463–470. doi:10.1038/modpathol.2010.214
Bonder, M. J., Kurilshikov, A., Tigchelaar, E. F., Mujagic, Z., Imhann, F., Vila, A. V., et al. (2016). The effect of host genetics on the gut microbiome. Nat. Genet. 48 (11), 1407–1412. doi:10.1038/ng.3663
Cai, N., Choi, K. W., and Fried, E. I. (2020a). Reviewing the genetics of heterogeneity in depression: Operationalizations, manifestations and etiologies. Hum. Mol. Genet. 29 (R1), R10–R18. doi:10.1093/hmg/ddaa115
Cai, N., Revez, J. A., Adams, M. J., Andlauer, T. F. M., Breen, G., Byrne, E. M., et al. (2020b). Minimal phenotyping yields genome-wide association signals of low specificity for major depression. Nat. Genet. 52 (4), 437–447. doi:10.1038/s41588-020-0594-5
Carroll, V. A., Griffiths, M. R., Geiger, M., Merlo, C., Furlan, M., Lammle, B., et al. (1997). Plasma protein C inhibitor is elevated in survivors of myocardial infarction. Arterioscler. Thromb. Vasc. Biol. 17 (1), 114–118. doi:10.1161/01.atv.17.1.114
Cenit, M. C., Nuevo, I. C., Codoner-Franch, P., Dinan, T. G., and Sanz, Y. (2017). Gut microbiota and attention deficit hyperactivity disorder: New perspectives for a challenging condition. Eur. Child. Adolesc. Psychiatry 26 (9), 1081–1092. doi:10.1007/s00787-017-0969-z
Chahwan, B., Kwan, S., Isik, A., van Hemert, S., Burke, C., and Roberts, L. (2019). Gut feelings: A randomised, triple-blind, placebo-controlled trial of probiotics for depressive symptoms. J. Affect. Disord. 253, 317–326. doi:10.1016/j.jad.2019.04.097
Chen, J. J., Zeng, B. H., Li, W. W., Zhou, C. J., Fan, S. H., Cheng, K., et al. (2017). Effects of gut microbiota on the microRNA and mRNA expression in the hippocampus of mice. Behav. Brain Res. 322, 34–41. doi:10.1016/j.bbr.2017.01.021
Chen, J. J., Zheng, P., Liu, Y. Y., Zhong, X. G., Wang, H. Y., Guo, Y. J., et al. (2018). Sex differences in gut microbiota in patients with major depressive disorder. Neuropsychiatr. Dis. Treat. 14, 647–655. doi:10.2147/Ndt.S159322
Chen, Z., Li, J., Gui, S. W., Zhou, C. J., Chen, J. J., Yang, C. C., et al. (2018). Comparative metaproteomics analysis shows altered fecal microbiota signatures in patients with major depressive disorder. Neuroreport 29 (5), 417–425. doi:10.1097/Wnr.0000000000000985
Chung, Y. C. E., Chen, H. C., Chou, H. C. L., Chen, I. M., Lee, M. S., Chuang, L. C., et al. (2019). Exploration of microbiota targets for major depressive disorder and mood related traits. J. Psychiatric Res. 111, 74–82. doi:10.1016/j.jpsychires.2019.01.016
Crist, A. M., Hinkle, K. M., Wang, X., Moloney, C. M., Matchett, B. J., Labuzan, S. A., et al. (2021). Transcriptomic analysis to identify genes associated with selective hippocampal vulnerability in Alzheimer's disease. Nat. Commun. 12 (1), 2311. doi:10.1038/s41467-021-22399-3
Damgaard, R. B., Walker, J. A., Marco-Casanova, P., Morgan, N. V., Titheradge, H. L., Elliott, P. R., et al. (2016). The deubiquitinase OTULIN is an essential negative regulator of inflammation and autoimmunity. Cell 166(5), 1215–1230. doi:10.1016/j.cell.2016.07.019
Dinan, T. G., and Cryan, J. F. (2015). The impact of gut microbiota on brain and behaviour: Implications for psychiatry. Curr. Opin. Clin. Nutr. Metab. Care 18 (6), 552–558. doi:10.1097/MCO.0000000000000221
Dong, Z., Shen, X., Hao, Y., Li, J., Li, H., Xu, H., et al. (2021). Gut microbiome: A potential indicator for differential diagnosis of major depressive disorder and general anxiety disorder. Front. Psychiatry 12, 651536. doi:10.3389/fpsyt.2021.651536
Douglas, G. M., Maffei, V. J., Zaneveld, J. R., Yurgel, S. N., Brown, J. R., Taylor, C. M., et al. (2020). PICRUSt2 for prediction of metagenome functions. Nat. Biotechnol. 38 (6), 685–688. doi:10.1038/s41587-020-0548-6
Dubois, T., Reynaert, C., Jacques, D., Lepiece, B., and Zdanowicz, N. (2019). Role of gut microbiota in the interaction between immunity and psychiatry: a literature review. Psychiatr. Danub 31, 381–385.
Durbin, R. K., Kotenko, S. V., and Durbin, J. E. (2013). Interferon induction and function at the mucosal surface. Immunological Reviews 255(1), 25, 39. doi:10.1111/imr.12101
Elisen, M. G. L. M., vondemBorne, P. A. K., Bouma, B. N., and Meijers, J. C. M. (1998). Protein C inhibitor acts as a procoagulant by inhibiting the thrombomodulin induced activation of protein C in human plasma (vol 91, pg 1542, 1998). Blood 91 (8), 3091.
Espana, F., Berrettini, M., and Griffin, J. H. (1989). Purification and characterization of plasma protein C inhibitor. Thromb. Res. 55 (3), 369–384. doi:10.1016/0049-3848(89)90069-8
Evrensel, A., and Tarhan, K. N. (2021). Emerging role of Gut-microbiota-brain axis in depression and therapeutic implication. Prog. Neuro-Psychopharmacol. Biol. Psychiatry 106, 1110138. doi:10.1016/j.pnpbp.2020.110138
Flint, J., and Kendler, K. S. (2014). The genetics of major depression. Neuron 81 (5), 1214. doi:10.1016/j.neuron.2014.02.033
Gurzu, S., and Jung, I. (2021). Subcellular expression of maspin in colorectal cancer: Friend or foe. Cancers 13 (3), 366. doi:10.3390/cancers13030366
Hoste, E., Lecomte, K., Annusver, K., Vandamme, N., Roels, J., Maschalidi, S., et al. (2021). OTULIN maintains skin homeostasis by controlling keratinocyte death and stem cell identity. Nat. Commun. 12 (1), 5913. doi:10.1038/s41467-021-25944-2
Howard, D. M., Adams, M. J., Shirali, M., Clarke, T. K., Marioni, R. E., Davies, G., et al. (2018). Addendum: Genome-wide association study of depression phenotypes in UK Biobank identifies variants in excitatory synaptic pathways. Nat. Commun. 9, 3578. doi:10.1038/s41467-018-05310-5
Howard, D. M., Adams, M. J., Shirali, M., Clarke, T. K., Marioni, R. E., Davies, G., et al. (2018). Genome-wide association study of depression phenotypes in UK Biobank identifies variants in excitatory synaptic pathways. Nat. Commun. 12, 2012. doi:10.1038/s41467-021-22411-w
Hua, X., Song, L., Yu, G., Vogtmann, E., Goedert, J. J., Abnet, C. C., et al. (2022). MicrobiomeGWAS: A Tool for Identifying Host Genetic Variants Associated with Microbiome Composition. Genes (Basel) 13, 7. doi:10.3390/genes13071224
Huang, F., and Wu, X. J. (2021). Brain neurotransmitter modulation by gut microbiota in anxiety and depression. Front. Cell Dev. Biol. 9, 649103. doi:10.3389/fcell.2021.649103
Huang, Y. C., Shi, X., Li, Z. Y., Shen, Y., Shi, X. X., Wang, L. Y., et al. (2018). Possible association of Firmicutes in the gut microbiota of patients with major depressive disorder. Neuropsychiatr. Dis. Treat. 14, 3329–3337. doi:10.2147/Ndt.S188340
Huang, H. Y., Lin, Y. C., Cui, S., Huang, Y., Tang, Y., Xu, J., et al. (2022). miRTarBase update 2022: an informative resource for experimentally validated miRNA-target interactions. Nucleic Acids Res. 50 (D1), D222–D230. doi:10.1093/nar/gkab1079
Hurley, M. J., Durrenberger, P. F., Gentleman, S. M., Walls, A. F., and Dexter, D. T. (2015). Altered expression of brain proteinase-activated receptor-2, trypsin-2 and serpin proteinase inhibitors in Parkinson's disease. J. Mol. Neurosci. 57 (1), 48–62. doi:10.1007/s12031-015-0576-8
Ji, F. B., Zhang, J. J., Liu, N. Y., Gu, Y. Z., Zhang, Y., Huang, P. P., et al. (2022). Blocking hepatocarcinogenesis by a cytochrome P450 family member with female-preferential expression. Gut 71, 2313–2324. doi:10.1136/gutjnl-2021-326050
Jiang, H. Y., Ling, Z. X., Zhang, Y. H., Mao, H. J., Ma, Z. P., Yin, Y., et al. (2015). Altered fecal microbiota composition in patients with major depressive disorder. Brain Behav. Immun. 48, 186–194. doi:10.1016/j.bbi.2015.03.016
Jing, Y., Jia, D., Wong, C. M., Oi-Lin Ng, I., Zhang, Z., Liu, L., et al. (2014). SERPINA5 inhibits tumor cell migration by modulating the fibronectin-integrin β1 signaling pathway in hepatocellular carcinoma. Mol. Oncol. 8 (2), 366–377. doi:10.1016/j.molonc.2013.12.003
Khenjanta, C., Thanan, R., Jusakul, A., Techasen, A., Jamnongkan, W., Namwat, N., et al. (2014). Association of CYP39A1, RUNX2 and oxidized alpha-1 antitrypsin expression in relation to cholangiocarcinoma progression. Asian Pac. J. Cancer Prev. 15 (23), 10187–10192. doi:10.7314/Apjcp.2014.15.23.10187
Koopman, M., El Aidy, S., and Consortium, M. (2017). Depressed gut? The microbiota-diet-inflammation trialogue in depression. Curr. Opin. Psychiatry 30 (5), 369–377. doi:10.1097/Yco.0000000000000350
Kuczynski, J., Stombaugh, J., Walters, W. A., Gonzalez, A., Caporaso, J. G., and Knight, R. (2012). Using QIIME to analyze 16S rRNA gene sequences from microbial communities. Curr. Protoc. Microbiol. Chapter 10, 10.7.1–10.7.20. Unit 1E 5. doi:10.1002/9780471729259.mc01e05s27
Lai, W. T., Zhao, J., Xu, S. X., Deng, W. F., Xu, D., Wang, M. B., et al. (2021). Shotgun metagenomics reveals both taxonomic and tryptophan pathway differences of gut microbiota in bipolar disorder with current major depressive episode patients. J. Affect. Disord. 278, 311–319. doi:10.1016/j.jad.2020.09.010
Lasfar, A., Zloza, A., and Cohen-Solal, K. A. (2016). IFN-lambda therapy: current status and future perspectives. Drug Discovery Today 21(1), 167–171. doi:10.1016/j.drudis.2015.10.021
Lee, E. K., Chung, K. W., Yang, S. K., Park, M. J., Min, H. S., Kim, S. W., et al. (2013). DNA methylation of MAPK signal-inhibiting genes in papillary thyroid carcinoma. Anticancer Res. 33 (11), 4833–4839.
Lee, S. H., Ripke, S., Neale, B. M., Faraone, S. V., Purcell, S. M., Perlis, R. H., et al. (2013). Genetic relationship between five psychiatric disorders estimated from genome-wide SNPs. Nat. Genet. 45 (9), 984–994. doi:10.1038/ng.2711
Linghu, T., Zhao, Y. H., Wu, W. Z., Gao, Y., Tian, J. S., and Qin, X. M. (2022). Novel targets for ameliorating energy metabolism disorders in depression through stable isotope-resolved metabolomics. Biochim. Biophys. Acta-Bioenerg. 1863 (7), 148578. doi:10.1016/j.bbabio.2022.148578
Liu, Y. X., Zhang, L., Wang, X. Q., Wang, Z., Zhang, J. J., Jiang, R. H., et al. (2016). Similar fecal microbiota signatures in patients with diarrhea-predominant irritable bowel syndrome and patients with depression. Clin. Gastroenterol. Hepatol. 14 (11), 1602–1611. doi:10.1016/j.cgh.2016.05.033
Liu, Y. Y., Wang, H. Y., Gui, S. W., Zeng, B. H., Pu, J. C., Zheng, P., et al. (2021). Proteomics analysis of the gut-brain axis in a gut microbiota-dysbiosis model of depression. Transl. Psychiatry 11 (1), 568. doi:10.1038/s41398-021-01689-w
Luan, W. S., Hao, C. Z., Li, J. Q., Wei, Q., Gong, J. Y., Qiu, Y. L., et al. (2021). Biallelic loss-of-function ZFYVE19 mutations are associated with congenital hepatic fibrosis, sclerosing cholangiopathy and high-GGT cholestasis. J. Med. Genet. 58 (8), 514–525. doi:10.1136/jmedgenet-2019-106706
Mahlakoiv, T., Hernandez, P., Gronke, K., Diefenbach, A., and Staeheli, P. (2015). Leukocyte-Derived IFN-alpha/beta and Epithelial IFN-lambda Constitute a Compartmentalized Mucosal Defense System that Restricts Enteric Virus Infections. Plos Pathogens 11 4.
Malhi, G. S., and Mann, J. J. (2018). Depression. Lancet 392 (10161), 2299–2312. doi:10.1016/S0140-6736(18)31948-2
Malmstrom, E., Morgelin, M., Malmsten, M., Johansson, L., Norrby-Teglund, A., Shannon, O., et al. (2009). Protein C inhibitor--a novel antimicrobial agent. PLoS Pathog. 5 (12), e1000698. doi:10.1371/journal.ppat.1000698
Marlar, R. A., and Griffin, J. H. (1980). Deficiency of protein C inhibitor in combined factor V/VIII deficiency disease. J. Clin. Invest. 66 (5), 1186–1189. doi:10.1172/JCI109952
Martins-Silva, T., Salatino-Oliveira, A., Genro, J. P., Meyer, F. D. T., Li, Y., Rohde, L. A., et al. (2021). Host genetics influences the relationship between the gut microbiome and psychiatric disorders. Prog. Neuropsychopharmacol. Biol. Psychiatry 106, 110153. doi:10.1016/j.pnpbp.2020.110153
Mason, B. L., Li, Q. W., Minhajuddin, A., Czysz, A. H., Coughlin, L. A., Hussain, S. K., et al. (2020). Reduced anti-inflammatory gut microbiota are associated with depression and anhedonia. J. Affect. Disord. 266, 394–401. doi:10.1016/j.jad.2020.01.137
Meydan, C., Shenhar-Tsarfaty, S., and Soreq, H. (2016). MicroRNA regulators of anxiety and metabolic disorders. Trends Mol. Med. 22 (9), 798–812. doi:10.1016/j.molmed.2016.07.001
Moloney, G. M., O'Leary, O. F., Salvo-Romero, E., Desbonnet, L., Shanahan, F., Dinan, T. G., et al. (2017). Microbial regulation of hippocampal miRNA expression: Implications for transcription of kynurenine pathway enzymes. Behav. Brain Res. 334, 50–54. doi:10.1016/j.bbr.2017.07.026
Papareddy, P., Kalle, M., Bhongir, R. K., Morgelin, M., Malmsten, M., and Schmidtchen, A. (2014). Antimicrobial effects of helix D-derived peptides of human antithrombin III. J. Biol. Chem. 289 (43), 29790–29800. doi:10.1074/jbc.M114.570465
Penninx, B. W. J. H., and Lange, S. M. M. (2018). Metabolic syndrome in psychiatric patients: Overview, mechanisms, and implications. Dialogues Clin. Neurosci. 20 (1), 63–73. doi:10.31887/DCNS.2018.20.1/bpenninx
Qin, Y. W., Havulinna, A. S., Liu, Y., Jousilahti, P., Ritchie, S. C., Tokolyi, A., et al. (2022). Combined effects of host genetics and diet on human gut microbiota and incident disease in a single population cohort. Nat. Genet. 54 (2), 134–142. doi:10.1038/s41588-021-00991-z
Rogers, G. B., Keating, D. J., Young, R. L., Wong, M. L., Licinio, J., and Wesselingh, S. (2016). From gut dysbiosis to altered brain function and mental illness: mechanisms and pathways. Mol. Psychiatry 21 (6), 738–748. doi:10.1038/mp.2016.50
Rong, H., Xie, X. H., Zhao, J., Lai, W. T., Wang, M. B., Xu, D., et al. (2019). Similarly in depression, nuances of gut microbiota: Evidences from a shotgun metagenomics sequencing study on major depressive disorder versus bipolar disorder with current major depressive episode patients. J. Psychiatr. Res. 113, 90–99. doi:10.1016/j.jpsychires.2019.03.017
Rosa, J. M., Formolo, D. A., Yu, J., Lee, T. H., and Yau, S. Y. (2022). The role of MicroRNA and microbiota in depression and anxiety. Front. Behav. Neurosci. 16, 828258. doi:10.3389/fnbeh.2022.828258
Rothschild, D., Weissbrod, O., Barkan, E., Kurilshikov, A., Korem, T., Zeevi, D., et al. (2018). Environment dominates over host genetics in shaping human gut microbiota. Nature 555 (7695), 210–215. doi:10.1038/nature25973
Ruhlemann, M. C., Degenhardt, F., Thingholm, L. B., Wang, J., Skieceviciene, J., Rausch, P., et al. (2018). Application of the distance-based F test in an mGWAS investigating beta diversity of intestinal microbiota identifies variants in SLC9A8 (NHE8) and 3 other loci. Gut Microbes 9 (1), 68–75. doi:10.1080/19490976.2017.1356979
Shi, Y., Guo, Z., Su, X., Meng, L., Zhang, M., Sun, J., et al. (2020). DeepAntigen: a novel method for neoantigen prioritization via 3D genome and deep sparse learning. Bioinformatics 36 (19), 4894–4901. doi:10.1093/bioinformatics/btaa596
Simpson, C. A., Diaz-Arteche, C., Eliby, D., Schwartz, O. S., Simmons, J. G., and Cowan, C. S. M. (2021). The gut microbiota in anxiety and depression - a systematic review. Clin. Psychol. Rev. 83, 101943. doi:10.1016/j.cpr.2020.101943
Skalkidou, A., Poromaa, I. S., Iliadis, S. I., Huizink, A. C., Hellgren, C., Freyhult, E., et al. (2019). Stress-related genetic polymorphisms in association with peripartum depression symptoms and stress hormones: A longitudinal population-based study. Psychoneuroendocrinology 103, 296–305. doi:10.1016/j.psyneuen.2019.02.002
Stilling, R. M., Moloney, G. M., Ryan, F. J., Hoban, A. E., Bastiaanssen, T. F., Shanahan, F., et al. (2018). Social interaction-induced activation of RNA splicing in the amygdala of microbiome-deficient mice. Elife 7, e33070. doi:10.7554/eLife.33070
Suzuki, K., Nishioka, J., and Hashimoto, S. (1983). Protein C inhibitor. Purification from human plasma and characterization. J. Biol. Chem. 258 (1), 163–168. doi:10.1016/s0021-9258(18)33235-6
Uhrin, P., Dewerchin, M., Hilpert, M., Chrenek, P., Schofer, C., Zechmeister-Machhart, M., et al. (2000). Disruption of the protein C inhibitor gene results in impaired spermatogenesis and male infertility. J. Clin. Invest. 106 (12), 1531–1539. doi:10.1172/JCI10768
Vishweswaraiah, S., Akyol, S., Yilmaz, A., Ugur, Z., Gordevicius, J., Oh, K. J., et al. (2022). Methylated cytochrome P450 and the solute carrier family of genes correlate with perturbations in bile acid metabolism in Parkinson's disease. Front. Neurosci. 16, 804261. doi:10.3389/fnins.2022.804261
Waclawikova, B., and El Aidy, S. (2018). Role of microbiota and tryptophan metabolites in the remote effect of intestinal inflammation on brain and depression. Pharm. (Basel) 11 (3), 63. doi:10.3390/ph11030063
Wang, X., Sundquist, K., Hedelius, A., Palmer, K., Memon, A. A., and Sundquist, J. (2015). Circulating microRNA-144-5p is associated with depressive disorders. Clin. Epigen. 7, 69. doi:10.1186/s13148-015-0099-8
Wang, J., Thingholm, L. B., Skieceviciene, J., Rausch, P., Kummen, M., Hov, J. R., et al. (2016). Genome-wide association analysis identifies variation in vitamin D receptor and other host factors influencing the gut microbiota. Nat. Genet. 48 (11), 1396–1406. doi:10.1038/ng.3695
Wang, Z. X., Cheng, Y. P., Li, Y., Han, J. M., Yuan, Z. S., Li, Q. H., et al. (2022). The relationship between obesity and depression is partly dependent on metabolic health status: A nationwide inpatient sample database study. Front. Endocrinol. 13, 880230. doi:10.3389/fendo.2022.880230
Wei, J., Huang, X. J., Huang, Y., Xiong, M. Y., Yao, X. Y., Huang, Z. N., et al. (2021). Key immune-related gene ITGB2 as a prognostic signature for acute myeloid leukemia. Ann. Transl. Med. 9 (17), 1386. doi:10.21037/atm-21-3641
Weissbrod, O., Rothschild, D., Barkan, E., and Segal, E. (2018). Host genetics and microbiome associations through the lens of genome wide association studies. Curr. Opin. Microbiol. 44, 9–19. doi:10.1016/j.mib.2018.05.003
Wray, N. R., Ripke, S., Mattheisen, M., Trzaskowski, M., Byrne, E. M., Abdellaoui, A., et al. (2018). Genome-wide association analyses identify 44 risk variants and refine the genetic architecture of major depression. Nat. Genet. 50 (5), 668–681. doi:10.1038/s41588-018-0090-3
Xu, F., Fu, Y., Sun, T. Y., Jiang, Z., Miao, Z., Shuai, M., et al. (2020). The interplay between host genetics and the gut microbiome reveals common and distinct microbiome features for complex human diseases. Microbiome 8 (1), 145. doi:10.1186/s40168-020-00923-9
Yang, H. J., and Geiger, M. (2017). Cell penetrating SERPINA5 (Protein C inhibitor, PCI): More questions than answers. Seminars Cell Dev. Biol. 62, 187–193. doi:10.1016/j.semcdb.2016.10.007
Yi, Y., Liu, Y., Wu, K., Wu, W., and Zhang, W. (2019). The core genes involved in the promotion of depression in patients with ovarian cancer. Oncol. Lett. 18 (6), 5995–6007. doi:10.3892/ol.2019.10934
Keywords: gut microbiome, depression, SERPINA5, beta diversity, microRNA
Citation: Han K, Ji L, Wang C, Shao Y, Chen C, Liu L, Feng M, Yang F, Wu X, Li X, Xie Q, He L, Shi Y, He G, Dong Z and Yu T (2023) The host genetics affects gut microbiome diversity in Chinese depressed patients. Front. Genet. 13:976814. doi: 10.3389/fgene.2022.976814
Received: 23 June 2022; Accepted: 21 December 2022;
Published: 09 January 2023.
Edited by:
Yunjia Lai, Columbia University, United StatesReviewed by:
Claudia Pisanu, University of Cagliari, ItalyYunjia Lai, Columbia University, United States
Copyright © 2023 Han, Ji, Wang, Shao, Chen, Liu, Feng, Yang, Wu, Li, Xie, He, Shi, He, Dong and Yu. This is an open-access article distributed under the terms of the Creative Commons Attribution License (CC BY). The use, distribution or reproduction in other forums is permitted, provided the original author(s) and the copyright owner(s) are credited and that the original publication in this journal is cited, in accordance with accepted academic practice. No use, distribution or reproduction is permitted which does not comply with these terms.
*Correspondence: Guang He, aGVndWFuZ2Jpb3hAMTYzLmNvbQ==; Zaiquan Dong, cXVhbnphaWRvbmdAMTI2LmNvbQ==; Tao Yu, eXV0YW9Ac2p0dS5lZHUuY24=