- 1Deparrtment of Central Lab, Affiliated People’s Hospital of Jiangsu University, Zhenjiang, China
- 2Zhenjiang Clinical Research Center of Hematology, Affiliated People’s Hospital of Jiangsu University, Zhenjiang, China
- 3The Key Lab of Precision Diagnosis and Treatment in Hematologic Malignancies of Zhenjiang City, Affiliated People’s Hospital of Jiangsu University, Zhenjiang, China
- 4Deparrtment of Hematology, Affiliated People’s Hospital of Jiangsu University, Zhenjiang, China
Background: Various circular RNA (circRNA) molecules are abnormally expressed in acute myeloid leukemia (AML), and associated with disease occurrence and development, as well as patient prognosis. The roles of circ_0059706, a circRNA derived from ID1, in AML remain largely unclear.
Results: Here, we reported circ_0059706 expression in de novo AML and its association with prognosis. We found that circ_0059706 expression was significantly lower in AML patients than in controls (p < 0.001). Survival analysis of patients with AML divided into two groups according to high and low circ_0059706 expression showed that overall survival (OS) of patients with high circ_0059706 expression was significantly longer than that of those with low expression (p < 0.05). Further, female patients with AML and those aged >60 years old in the high circ_0059706 expression group had longer OS than male patients and those younger than 60 years. Multiple regression analysis showed that circ_0059706 was an independent factor-affecting prognosis of all patients with AML. To evaluate the prospects for application of circ_0059706 in machine learning predictions, we developed seven types of algorithm. The gradient boosting (GB) model exhibited higher performance in prediction of 1-year prognosis and 3-year prognosis, with AUROC 0.796 and 0.847. We analyzed the importance of variables and found that circ_0059706 expression level was the first important variables among all 26 factors included in the GB algorithm, suggesting the importance of circ_0059706 in prediction model. Further, overexpression of circ_0059706 inhibited cell growth and increased apoptosis of leukemia cells in vitro.
Conclusion: These results provide evidence that high expression of circ_0059706 is propitious for patient prognosis and suggest circ_0059706 as a potential new biomarker for diagnosis and prognosis evaluation in AML, with high predictive value and good prospects for application in machine learning algorithms.
Introduction
Acute myeloid leukemia (AML) is one of the most common hematological malignancies and the most frequent type of acute leukemia in adults (Haubner et al., 2019; Newell and Cook, 2021). In recent years, treatment approaches, including molecular targeted therapy and hematopoietic stem cell transplantation, among others, have led to great progress in improving patient outcomes; however, 5-year survival rates remain low (Huang et al., 2019; Burchert et al., 2020; Vetrie et al., 2020; Du et al., 2021; Pollyea et al., 2021; Zhang et al., 2021). Therefore, research into the molecular mechanisms underlying the occurrence and development of AML is crucial to inform discovery of new clinical markers and therapeutic targets.
Circular RNA (circRNA) is a type of non-coding RNA characterized by a closed ring structure and circRNA molecules are widely distributed in eukaryotes, where they perform complex biological functions (Kristensen et al., 2019; Zhou et al., 2020). As circRNAs do not have 5′ end cap or 3′-polyadenylation tail structures, they cannot easily be recognized and degraded by RNase enzymes (Huang et al., 2020). Hence, circRNAs have high stability, as well as specificity, which contribute to its good potential for application in the field of tumor biomarkers (Xu et al., 2019; Wang et al., 2021; Wei et al., 2020). Some circRNA molecules are abnormally expressed in AML and associated with patient prognosis (Chen et al., 2018; Sun et al., 2019; Lin et al., 2021); for example, circ-VIM is significantly up-regulated in AML, and its over-expression is an independent prognostic factor associated with duration of overall and leukemia-free survival of patients with AML (Yi et al., 2019).
Developments in big data and computer hardware and software technologies have led to widespread use of machine learning in medicine (Ngiam and Khor, 2019; Radakovich et al., 2020). Compared with traditional statistics, machine learning has more powerful predictive ability (Lewis and Kemp, 2021; Tran et al., 2021) and its value for application in assisting disease diagnosis and predicting clinical outcomes has also attracted the attention of scholars.
Our previous study has revealed ID1 transcript level significantly increased in AML and act as an independent risk factor in young non-M3 patients. Circ_0059706 is a circular RNA, formed by ID1 during its splicing. In this study, we investigated circ_0059706 expression in patients with AML, evaluated its clinical significance, and analyzed the predictive ability of circ_0059706 expression for AML prognosis using machine learning. The aim of the study was to explore the value of circ_0059706 as a new tumor marker for predicting AML prognosis.
Materials and methods
Patients
This study was approved by the Ethics Committee of the Affiliated People’s Hospital of Jiangsu University and included 100 patients newly-diagnosed with AML and 33 healthy controls (K-20190020-Y). All samples were from the sample bank at our hospital and all patients signed informed consent forms. AML was classified according to World Health Organization (WHO) criteria and French-American-British (FAB) classification. Mutations were detected by high-resolution melting analysis (Wen et al., 2015).
Cell culture and transfection
The K562 and THP-1 human leukemia cell line were purchased from ATCC. Cells were cultured in RPMI 1640 medium (Wisent, Nanjing, China) containing 10% fetal calf serum (FCS) (ExCell Bio, Shanghai, China) and 100 U/ml penicillin/streptomycin (Wisent, Nanjing, China) at 37°C in a 5% CO2 humidified atmosphere. Lentiviruses over-expressing circ_0059706 were purchased from Shanghai Jikai Biological Co., Ltd. (Shanghai, China) and cell transfection performed according to the manufacturer’s instructions.
Cell growth assays
Cells were seeded at 3 × 103 per well in 96-well plates. After culture for 0, 24, 48, and 72 h, 10 μl CCK-8 (Dojindo, Kumamoto, Japan) solution was added to each well. Optical density values were measured at 450 nm absorbance using a microplate reader.
Cell apoptosis assay
Cells (5 × 105) were seeded into 6-well plates containing complete 1640 culture solution, without FCS, for 48 h. Apoptosis rate was determined using an apoptosis detection kit (Annexin V PE/7-AAD, BD Biosciences, Franklin Lakes, NJ, United States), and analyzed by flow cytometry on a FACSCalibur platform (Becton Dickinson, San Jose, CA, Unied States).
RNA isolation, reverse transcription, and real-time quantitative PCR (RQ-PCR)
Mononuclear cells were extracted from bone marrow (BM) specimens using gradient centrifugation (TBD Sciences, China). RNA extraction and reverse transcription were conducted based on the instructions in miScript kits (Qiagen, Tilden, Germany). Reverse transcription and RQ-PCR were conducted as previously reported (Ma et al., 2014). The primers for circ_0059706 were 5′-TGGTAAACTCTCATTCCACGTTC-3' (forward) and 5′-CCACTGGCGACTTTCATGAT-3' (reverse). The primers used as controls were ABL and sequences were 5′- TCCTCCAGCTGTTATCTGGAAGA -3′ (forward) and 5′- TCCAACGAGCGGCTTCAC -3' (reverse). The primers for miR-326 were 5′-GTCGTATCCAGTGCAGGGTCCGAGGTATTCGCACTGGATACGA CCTGGAG-3' (forward) and 5′- GCCGAGCCTCTGGGCCCTTC-3' (reverse). The primers for U6 were 5′-CTCGCTTCGGCAGCACA-3' (forward) and 5′-AACGCTTCACGAATTTGCGT-3' (reverse). Relative circ_0059706 expression levels were calculated using 2−ΔΔCT method.
Statistical analysis
Data were analyzed using SPSS 20.0 software. Relative levels of circ_0059706 expression were calculated using the 2−ΔΔCT method. Categorical variables were analyzed using chi square tests and/or Fisher’s exact tests. The diagnostic capacity of circ_0059706 was analyzed using receiver operating characteristic (ROC) curves and area under the curve (AUC) values. Survival was analyzed using the Kaplan-Meier method. Univariate and multivariate Cox regression analyses were conducted. Differences in continuous variables between two groups were compared by Student’s t-test. Differences were considered statistically significant at two-tailed p < 0.05.
For machine learning, case and survival data, including 26 characteristic variables from 57 cases, were used. Twelve basic variables included in the analysis were: circ_0059706 expression level, sex, age, white blood cell (WBC) count, hemoglobin (HB) level, platelet (PLT) count, BM blasts, diagnosis, karyotype chromosome abnormalities, chromosome risk group, blast percentage, and granulocyte count. Mutations of nine genes (CEBPA, NPM1, FLT3, CKIT, RAS, IDH1/DH2, DNMT3A, SRSF2, and SETBP1) were also included as variables. Five derivative variables and the methods used to generate them are shown in Table 1. Data from all cases were randomly divided into a training set (75%) for model development and a test set (25%) for performance evaluation. We developed seven types of machine learning algorithm, including: logistic regression (LR), random forest (RF), gradient boosting (GB), neural network (NNK), support vector machine (SVM), k-NearestNeighor (KNN), and Gaussian naïve Bayes (GNB). Parameters of 1-year survival and 3-year survival are as follows: C = 0.01 in LR, n_estimators = 300, random_state = 10 in RF, C = 100 in SVM, MLPClassifier (80, 10), random_state = 100 and random_state = 200 in NNK separately, n_estimators = 500, random_state = 300 and n_estimators = 200, random_state = 280 in GB separately, neighbors = 9 in KNN. Area under the ROC curve (AUROC), sensitivity, and specificity values were used as performance evaluation indicators. Machine learning algorithms were developed using Python software (version 3.7.6.). The “LogisticRegression,” “randomforestclassfier,” “gradientbootingclassifier,” “MLP classifier,” “SVC,” “Kneighborsclassifier,” and “Gaussian NB” functions in the “Sklearn” package (version 1.0.2.) were used for machine learning algorithms, and the “matplotlib” package was applied for machine learning data display.

TABLE 1. Methods used to generate the five derivative variables included in machine learning models.
Results
Circ_0059706 expression and its capacity to distinguish between patients with AML and healthy controls
Levels of circ_0059706 expression in BM samples from 100 patients with AML and 33 healthy controls were detected by RQ-PCR. Median circ_0059706 expression levels in healthy controls and patients with AML were 4.581 and 0.153, respectively; Circ_0059706 expression was significantly lower in AML than in controls (p < 0.001) (Figure 1A). The AUC value of circ_0059706 was 0.925 in AML patients (95% confidence interval: 0.873–0.978, p < 0.001) (Figure 1B), indicating that circ_0059706 expression has potential as biomarker to distinguish AML from control samples.
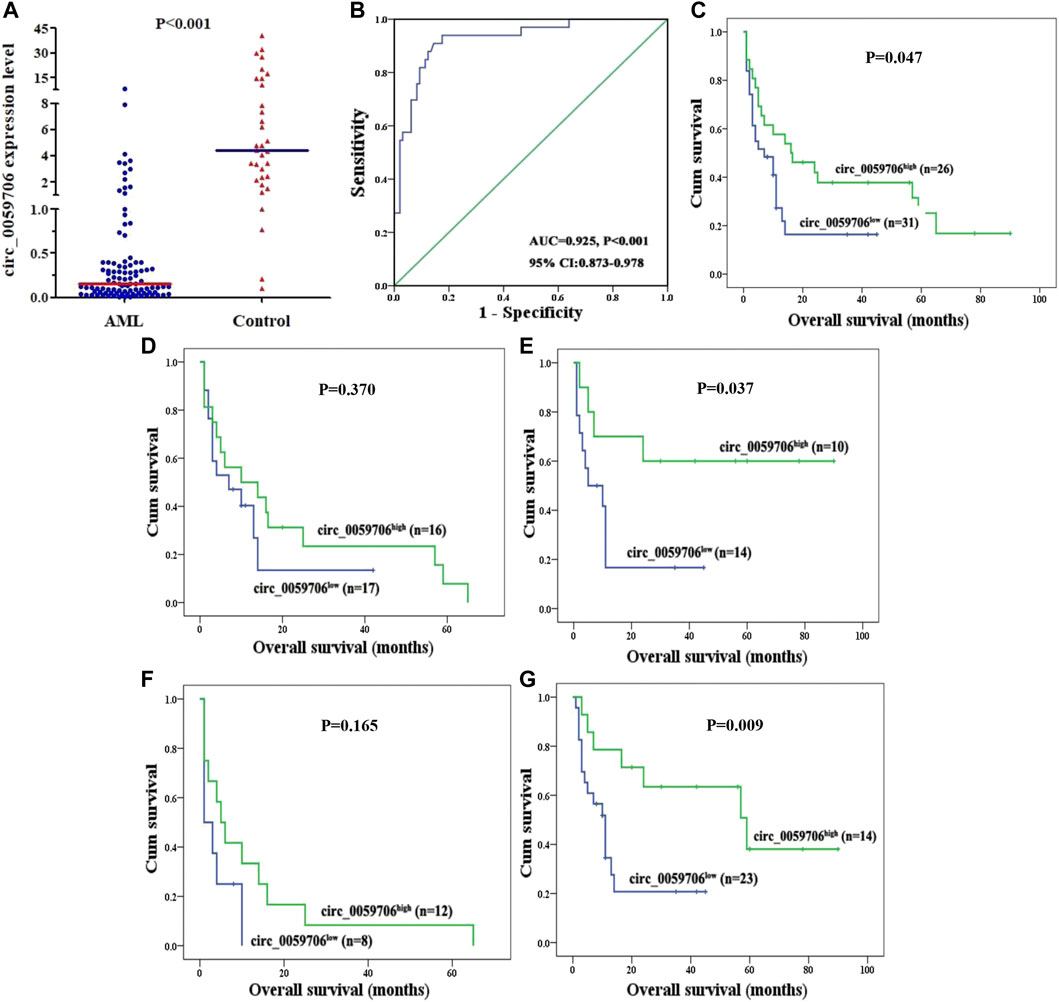
FIGURE 1. Expression of circ_0059706 in patients with AML and its impact on overall survival. (A) Level of circ_0059706 expression in controls and patients with AML detected by RQ-PCR. (B) Discriminative capacity of circ_0057606 expression in patients with AML determined by ROC curve analysis. (C–G) Impact of circ_0057606 expression on overall survival in: (C) total, (D) male, (E) female, (F) age >60 years, and (G) age ≤60 years, patients with AML.
Associations between Circ_0059706 expression and patient clinical characteristics
To investigate associations of circ_0059706 expression with AML clinical characteristics, the total patient group was divided into circ_0059706high and circ_0059706 low groups, according to median +1/16 standard deviation of circ_0059706 expression level, using a cutoff value of 0.254 (sensitivity, 91.3%; specificity, 85.6%). Then, clinical parameters were compared between the high and low expression groups (Table 2). No significant differences were detected between the high and low expression groups; however, HB level, WBC count, and platelet count were higher in peripheral blood from the circ_0059706high group than from the circ_0059706low group, and the proportion of BM blast cells in the circ_0059706high group was lower than that of the circ_0059706low group. No correlations between gene mutations and circ_0059706 expression were detected.
Association of Circ_0059706 expression level and prognosis of patients with AML
To explore association of circ_0059706 expression with patient prognosis, we analyzed survival data from 57 patients. Although circ_0059706 had no value for predicting complete remission (CR), patients in the circ_0059706high group had significantly longer overall survival (OS) than those in the circ_0059706low group (p = 0.047) (Figure 1C). Further, compared with male patients with AML, OS of circ_0059706high female patients was longer than that of circ_0059706low female patients (p = 0.037) (Figures 1D,E). Compared with older than 60 years, OS of those in the circ_0059706high group was significantly longer than that of those in the circ_0059706low group who were younger than 60-years-old AML (p = 0.009) (Figures 1F,G). Variables resulting in p < 0.1 in univariate analysis (age, WBC, risk classification, and circ_0059706 expression) were included in multivariate analysis, which demonstrated that circ_0059706 was an independent factor associated with poor prognosis in the total AML patients (p = 0.020) (Table 3).
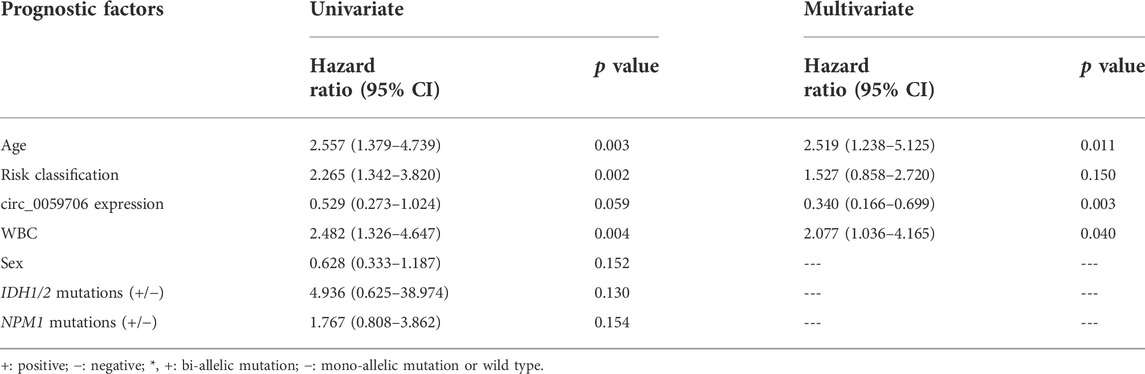
TABLE 3. Univariate and multivariate analyses of prognostic factors for overall survival in whole-cohort AML patients.
Evaluation of prediction ability of Circ_0059706 in machine learning algorithms
First, LR, RF, GB, NNK, SVM, KNN, and GNB 7 machine learning algorithms were developed using training set data, and their performance evaluated. As shown in Table 4, the GB model had better performance in predicting 1-year prognosis and 3-year prognosis, with AUROC 0.796 and 0.847, and sensitivity 0.615 and 0.923, specificity 0.75 and 1.
The selection of variables for machine learning algorithms is critical. Therefore, we analyzed the importance of variables included in the GB, LR, and RF algorithms, which had good modeling performance. Circ_0059706 expression level was the first important variable among all 26 features included in the GB and RF algorithms and was among the most important in the LR algorithm (Figure 2).
Circ_0059706 inhibits cell growth and increases apoptosis
To study the effect of circ_0059706 on leukemia cells, we over-expressed it in THP-1 and K562 cells (Figure 3A), and found that cell growth rate was inhibited by circ_0059706 over-expression (Figure 3B). Moreover, the apoptosis rate of circ_0059706-transfected THP-1 and K562 cells was significantly higher than that of the control group (p < 0.01) (Figure 3C).
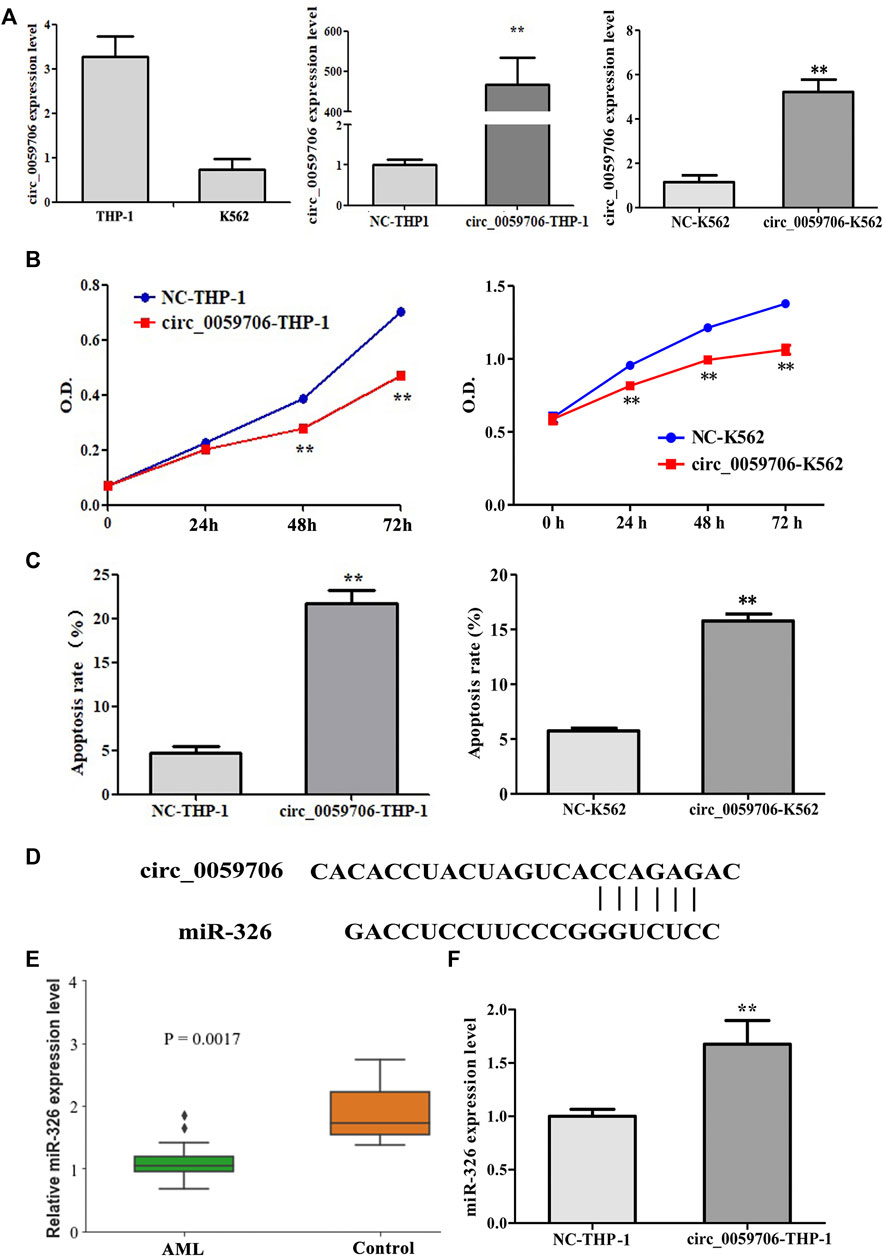
FIGURE 3. Effect of circ_0059706 on cell growth and apoptosis. (A) The level of circ_0059706 expression was detected by RQ-PCR. (B) Circ_0059706 inhibited cell growth in THP-1 and K562 cells detected by CCK8. (C) Circ_0059706 promoted cellular apoptosis in THP-1 and K562 cells, as detected by flow cytometry. (D) Bioinformatics analysis of the binding site between circ_0059706 and miR-326. (E) Expression levels of miR-326 in controls and AML patients were analyzed in the GSE51908 datasets. (F) The level of miR-326 was detected in circ_0059706 overexpressed cells by RQ-PCR. **, compared with the NC group, p < 0.01.
To determine the possible mechanism involved in the functionality of circ_0059706, we performed additional analysis. We analyzed the miRNAs that may bind to circ_0059706 by use of the circinteratome database (https://circinteractome.nia.nih.gov/index.html). Then, the expression levels of these miRNAs in AML patients were analyzed by the GEO database (Datasets: GSE51908). Bioinformatics analysis revealed that circ_0059706 contains a binding site for miR-326 (Figure 3D). The levels of miR-326 were lower in AML patients when compared with controls in GSE51908 datasets (Figure 3E). Finally, the expression levels of miR-326 were found to be up-regulated in cells that overexpressed circ_0059706 (Figure 3F).
Discussion
CircRNAs are non-coding RNAs that have recently emerged as a potential tumor biomarkers and drug targets, with good prospects for clinical application. CircPLXNB2 is a valuable predictor of prognosis in patients with AML (Lin, Wang, Bian, Sun, Guo, Kong, Zhao, Guo, Li, Wu, Wang, Wang and Li, 2021). Further, Liu et al. (2022) found that expression of hsa_circ_0004277 can be restored after chemotherapy in patients with AML, suggesting that its up-regulation is associated with successful treatment; hence, hsa_circ_0004277 is a potential diagnostic marker and treatment target in AML. Further, circRNF220 can distinguish AML from ALL and other hematological malignancies, while high circRNF220 expression is an unfavorable prognostic marker of recurrence, due to its role in sequestration of miR-30a, which increases MYSM1 and IER2 expression and is implicated in AML relapse (Liu et al., 2021).
Circ_0059706 derived from ID1. ID1 is a negative regulator of the HLH transcription factor that plays the role of an oncogene in promoting cell cycle, proliferation and inhibiting apoptosis (Yin et al., 2017; Chen et al., 2020). Our group previously reported that ID1 expression was up-regulated in AML patients, and the high expression associated with poor prognoses. Here, we found that circ_0059706 was down-regulated in AML. HB, WBC, and PLT levels were higher in peripheral blood from the circ_0059706high group than those in the group with low circ_0059706 expression. Further, the proportion of BM blast cells in the circ_0059706high group was lower than that in the circ_0059706low group. Hence, routine blood parameters appeared to be better in the high expression group than those of the low expression group. There were no correlations between gene mutations and circ_0059706 expression. Survival analysis showed that circ_0059706 has no value for prediction of CR; however, OS was significantly higher in patients with high circ_0059706 expression than those with low expression. Longer OS was observed in female patients and those >60-years-old with high circ_0059706 expression than in male patients <60-years-old. Furthermore, the total patient group was divided into circ_0059706high and circ_0059706low groups, according to circ_0059706 expression level at quartiles or tertiles. Patients in the circ_0059706high group had significantly longer OS than those in the circ_0059706low group (p = 0.038, p = 0.027). Multivariate analysis showed that circ_0059706 low expression was an independent factor associated with poor prognosis of all patients with AML, indicating that circ_0059706 has potential for application as a new biomarker for diagnosis and prognosis evaluation of AML.
Traditional statistics are generally used to infer relationships between variables, while machine learning models aim to make the most accurate predictions possible, and are increasingly being applied in medical prediction models. Gao et al. predicted a significant association between Luminal and HER2 breast cancer subtypes and estrogen/progesterone and HER2 receptor status, using the DeepCC method (Gao et al., 2019). Lee et al. comprehensively analyzed RNA-seq data and identified a potential role for machine learning in identifying categories of acute leukemia (Lee et al., 2021). Based on traditional statistical analysis, we found that Circ_0059706 level are closely related with survival, suggesting its potential as a biomarker in patients with AML. Different machine learning algorithms may be optimal for any data set; therefore, to assess the prospects for application of circ_0059706 levels in AML in machine learning algorithms, we developed seven types of machine learning algorithm, including LR, RF, GB, NNK, SVM, KNN, and GNB. The GB model had better performance in predicting 1-year prognosis and 3-year prognosis. We were unable to predict 5-year survival due to insufficient data. In recent years, scholars have established many risk assessment methods in various disease prognosis models using machine learning, which can provide guidance for the selection of treatment methods and prognosis assessment. For example, Heo applied an NNK algorithm to establish a prediction model for long-term prognosis in patients following ischemic stroke (Heo et al., 2019), while Tian established an early gastric cancer lymph node metastasis prediction model using a regularized dual averaging approach (Tian et al., 2021). Moreover, machine learning achieved acceptable prediction of central lymph node metastasis, with a GB model performing best, which may help to determine the optimal extent of initial surgical treatment for patients with T1–T2 stage, non-invasive, and clinically node-negative papillary thyroid cancer (Zhu et al., 2021).
Variables are crucial to the prediction results generated by machine learning; hence, the key roles of variables included in the machine learning models was also a focus of our attention. We analyzed the importance of variables in the GB, LR, and RF algorithms, which had good modeling performance. Circ_0059706 expression level was the first important variable among all 26 features included in the GB and RF algorithms, and it ranked highly in the LR algorithm. It indicated that circ_0059706 has a high predictive value and a good prospect for application in machine learning, supporting the potential of this circRNA as a new biomarker for diagnosis and prognosis evaluation in AML.
Furthermore, we analyzed the effect of circ_0059706 on cell growth and apoptosis in leukemia cells. The results showed that circ_0059706 overexpression inhibited cell growth and increased apoptosis, further supporting the hypothesis that the high expression of this circRNA is propitious for patient prognosis. To investigate the mechanisms, we analyzed miRNAs with common binding sites for circ_0059706 in the circinteratome database. The expression levels of miRNAs were analyzed by datasets GSE51908. Combined with literature reports, we focused miR-326, which was downregulated in GSE51908 datasets. P Cheng reported that expression of miR-326 was downregulated in AML patients compared with that in normal. Overexpression of miR-326 inhibited proliferation, promoted cell apoptosis and PMA-induced differentiation in AML cells (Cheng et al., 2020). Moreover, miR-326 down regulated in ALL patients and negative associated with its expression and MDR (Ghodousi and Rahgozar, 2018). These results suggested miR-326 maybe act as a tumor suppressor miRNA in leukemia and it was upregulated in circ_0059706 over-expressed cells. miR-326 was up-regulated in circ_0059706 overexpression cells, it may be a mechanism of inhibited growth and promoted cell apoptosis. However, more experiments needed to verify, such as luciferase reporter experiment, the effect of up/down-regulation of miR-326 expression on cell biological function, etc.
Conclusion
Taken together, our results indicate that down-regulation of circ_0059706 is a frequent event and predicts poor prognosis in patients with de novo AML. Circ_0059706 showed good predictive effects in machine learning models and was among the most important variables in the developed models. In addition, circ_0059706 overexpression could inhibit cell growth and increase apoptosis. These results demonstrated that circ_0059706 might act as a potential biomarker for prognosis in de novo AML.
Data availability statement
The original contributions presented in the study are included in the article/supplementary material, further inquiries can be directed to the corresponding authors.
Ethics statement
The studies involving human participants were reviewed and approved by Ethics Committee of the Affiliated People’s Hospital of Jiangsu University. Written informed consent to participate in this study was provided by the participants’ legal guardian/next of kin.
Author contributions
JM, XW, and ZX: performed the experiments. PX and YJ collected the data, performed data analyses. JL provided the resources. Jun Qian reviewed and edited the manuscript.
Funding
This study was supported by National Natural Science foundation of China (81900163,81970118), “Liu Ge Yi Gong Cheng” of Jiangsu Province (LGY2018024), China Postdoctoral Science Foundation funded project (2016M601748), youth medical talents project of “Ke Jiao Qiang Wei” project of Jiangsu province (QNRC2016450), Zhenjiang Clinical Research Center of Hematology (SS2018009). Social Development Foundation of Zhenjiang (SH2022065, SH2019067), Scientific Research Project of the Fifth 169 Project of Zhenjiang (21). Project of Zhenjiang First People’s Hospital (Y2021010-S).
Conflict of interest
The authors declare that the research was conducted in the absence of any commercial or financial relationships that could be construed as a potential conflict of interest.
Publisher’s note
All claims expressed in this article are solely those of the authors and do not necessarily represent those of their affiliated organizations, or those of the publisher, the editors and the reviewers. Any product that may be evaluated in this article, or claim that may be made by its manufacturer, is not guaranteed or endorsed by the publisher.
References
Burchert, A., Bug, G., Fritz, L. V., Finke, J., Stelljes, M., Rollig, C., et al. (2020). Sorafenib maintenance after allogeneic hematopoietic stem cell transplantation for acute myeloid leukemia with FLT3-internal tandem duplication mutation (SORMAIN). J. Clin. Oncol. 38 (26), 2993–3002. doi:10.1200/JCO.19.03345
Chen, H., Liu, T., Liu, J., Feng, Y., Wang, B., Wang, J., et al. (2018). Circ-ANAPC7 is upregulated in acute myeloid leukemia and appears to target the MiR-181 family. Cell. Physiol. biochem. 47 (5), 1998–2007. doi:10.1159/000491468
Chen, S. D., Yang, J. L., Lin, Y. C., Chao, A. C., and Yang, D. I. (2020). Emerging roles of inhibitor of differentiation-1 in alzheimer's disease: Cell cycle reentry and beyond. Cells 9 (7), E1746. doi:10.3390/cells9071746
Cheng, P., Lu, P., Guan, J., Zhou, Y., Zou, L., Yi, X., et al. (2020). LncRNA KCNQ1OT1 controls cell proliferation, differentiation and apoptosis by sponging miR-326 to regulate c-Myc expression in acute myeloid leukemia. Neoplasma 67 (2), 238–248. doi:10.4149/neo_2018_181215N972
Du, W., Xu, A., Huang, Y., Cao, J., Zhu, H., Yang, B., et al. (2021). The role of autophagy in targeted therapy for acute myeloid leukemia. Autophagy 17 (10), 2665–2679. doi:10.1080/15548627.2020.1822628
Gao, F., Wang, W., Tan, M., Zhu, L., Zhang, Y., Fessler, E., et al. (2019). DeepCC: A novel deep learning-based framework for cancer molecular subtype classification. Oncogenesis 8 (9), 44. doi:10.1038/s41389-019-0157-8
Ghodousi, E. S., and Rahgozar, S. (2018). MicroRNA-326 and microRNA-200c: Two novel biomarkers for diagnosis and prognosis of pediatric acute lymphoblastic leukemia. J. Cell. Biochem. 119 (7), 6024–6032. doi:10.1002/jcb.26800
Haubner, S., Perna, F., Kohnke, T., Schmidt, C., Berman, S., Augsberger, C., et al. (2019). Coexpression profile of leukemic stem cell markers for combinatorial targeted therapy in AML. Leukemia 33 (1), 64–74. doi:10.1038/s41375-018-0180-3
Heo, J., Yoon, J. G., Park, H., Kim, Y. D., Nam, H. S., and Heo, J. H. (2019). Machine learning-based model for prediction of outcomes in acute stroke. Stroke 50 (5), 1263–1265. doi:10.1161/STROKEAHA.118.024293
Huang, A., Zheng, H., Wu, Z., Chen, M., and Huang, Y. (2020). Circular RNA-protein interactions: Functions, mechanisms, and identification. Theranostics 10 (8), 3503–3517. doi:10.7150/thno.42174
Huang, Y., Su, R., Sheng, Y., Dong, L., Dong, Z., Xu, H., et al. (2019). Small-molecule targeting of oncogenic FTO demethylase in acute myeloid leukemia. Cancer Cell. 35 (4), 677–691. doi:10.1016/j.ccell.2019.03.006
Kristensen, L. S., Andersen, M. S., Stagsted, L. V. W., Ebbesen, K. K., Hansen, T. B., and Kjems, J. (2019). The biogenesis, biology and characterization of circular RNAs. Nat. Rev. Genet. 20 (11), 675–691. doi:10.1038/s41576-019-0158-7
Lee, J., Cho, S., Hong, S. E., Kang, D., Choi, H., Lee, J. M., et al. (2021). Integrative analysis of gene expression data by RNA sequencing for differential diagnosis of acute leukemia: Potential application of machine learning. Front. Oncol. 11, 717616. doi:10.3389/fonc.2021.717616
Lewis, J. E., and Kemp, M. L. (2021). Integration of machine learning and genome-scale metabolic modeling identifies multi-omics biomarkers for radiation resistance. Nat. Commun. 12 (1), 2700. doi:10.1038/s41467-021-22989-1
Lin, L., Wang, Y., Bian, S., Sun, L., Guo, Z., Kong, D., et al. (2021). A circular RNA derived from PLXNB2 as a valuable predictor of the prognosis of patients with acute myeloid leukaemia. J. Transl. Med. 19 (1), 123. doi:10.1186/s12967-021-02793-7
Liu, X., Liu, X., Cai, M., Luo, A., He, Y., Liu, S., et al. (2021). CircRNF220, not its linear cognate gene RNF220, regulates cell growth and is associated with relapse in pediatric acute myeloid leukemia. Mol. Cancer 20 (1), 139. doi:10.1186/s12943-021-01395-7
Liu, Y., Chen, X., Liu, J., Jin, Y., and Wang, W. (2022). Circular RNA circ_0004277 inhibits acute myeloid leukemia progression through MicroRNA-134-5p/single stranded DNA binding protein 2. Bioengineered 13 (4), 9662–9673. doi:10.1080/21655979.2022.2059609
Ma, J., Lin, J., Qian, J., Qian, W., Yin, J., Yang, B., et al. (2014). MiR-378 promotes the migration of liver cancer cells by down-regulating Fus expression. Cell. Physiol. biochem. 34 (6), 2266–2274. doi:10.1159/000369669
Newell, L. F., and Cook, R. J. (2021). Advances in acute myeloid leukemia. BMJ 375, n2026. doi:10.1136/bmj.n2026
Ngiam, K. Y., and Khor, I. W. (2019). Big data and machine learning algorithms for health-care delivery. Lancet. Oncol. 20 (5), e262–e273. doi:10.1016/S1470-2045(19)30149-4
Pollyea, D. A., Bixby, D., Perl, A., Bhatt, V. R., Altman, J. K., Appelbaum, F. R., et al. (2021). NCCN guidelines insights: Acute myeloid leukemia, version 2.2021. J. Natl. Compr. Canc. Netw. 19 (1), 16–27. doi:10.6004/jnccn.2021.0002
Radakovich, N., Nagy, M., and Nazha, A. (2020). Machine learning in haematological malignancies. Lancet. Haematol. 7 (7), e541–e550. doi:10.1016/S2352-3026(20)30121-6
Sun, Y. M., Wang, W. T., Zeng, Z. C., Chen, T. Q., Han, C., Pan, Q., et al. (2019). circMYBL2, a circRNA from MYBL2, regulates FLT3 translation by recruiting PTBP1 to promote FLT3-ITD AML progression. Blood 134 (18), 1533–1546. doi:10.1182/blood.2019000802
Tian, H., Ning, Z., Zong, Z., Liu, J., Hu, C., Ying, H., et al. (2021). Application of machine learning algorithms to predict lymph node metastasis in early gastric cancer. Front. Med. 8, 759013. doi:10.3389/fmed.2021.759013
Tran, K. A., Kondrashova, O., Bradley, A., Williams, E. D., Pearson, J. V., and Waddell, N. (2021). Deep learning in cancer diagnosis, prognosis and treatment selection. Genome Med. 13 (1), 152. doi:10.1186/s13073-021-00968-x
Vetrie, D., Helgason, G. V., and Copland, M. (2020). The leukaemia stem cell: Similarities, differences and clinical prospects in CML and AML. Nat. Rev. Cancer 20 (3), 158–173. doi:10.1038/s41568-019-0230-9
Wang, S., Zhang, K., Tan, S., Xin, J., Yuan, Q., Xu, H., et al. (2021). Circular RNAs in body fluids as cancer biomarkers: The new frontier of liquid biopsies. Mol. Cancer 20 (1), 13. doi:10.1186/s12943-020-01298-z
Wei, C. Y., Zhu, M. X., Lu, N. H., Liu, J. Q., Yang, Y. W., Zhang, Y., et al. (2020). Circular RNA circ_0020710 drives tumor progression and immune evasion by regulating the miR-370-3p/CXCL12 axis in melanoma. Mol. Cancer 19 (1), 84. doi:10.1186/s12943-020-01191-9
Wen, X. M., Hu, J. B., Yang, J., Qian, W., Yao, D. M., Deng, Z. Q., et al. (2015). CEBPA methylation and mutation in myelodysplastic syndrome. Med. Oncol. 32 (7), 192. doi:10.1007/s12032-015-0605-z
Xu, H., Wang, C., Song, H., Xu, Y., and Ji, G. (2019). RNA-Seq profiling of circular RNAs in human colorectal Cancer liver metastasis and the potential biomarkers. Mol. Cancer 18 (1), 8. doi:10.1186/s12943-018-0932-8
Yi, Y. Y., Yi, J., Zhu, X., Zhang, J., Zhou, J., Tang, X., et al. (2019). Circular RNA of vimentin expression as a valuable predictor for acute myeloid leukemia development and prognosis. J. Cell. Physiol. 234 (4), 3711–3719. doi:10.1002/jcp.27145
Yin, X., Tang, B., Li, J. H., Wang, Y., Zhang, L., Xie, X. Y., et al. (2017). ID1 promotes hepatocellular carcinoma proliferation and confers chemoresistance to oxaliplatin by activating pentose phosphate pathway. J. Exp. Clin. Cancer Res. 36 (1), 166. doi:10.1186/s13046-017-0637-7
Zhang, X. H., Chen, J., Han, M. Z., Huang, H., Jiang, E. L., Jiang, M., et al. (2021). The consensus from the Chinese society of Hematology on indications, conditioning regimens and donor selection for allogeneic hematopoietic stem cell transplantation: 2021 update. J. Hematol. Oncol. 14 (1), 145. doi:10.1186/s13045-021-01159-2
Zhou, W. Y., Cai, Z. R., Liu, J., Wang, D. S., Ju, H. Q., and Xu, R. H. (2020). Circular RNA: Metabolism, functions and interactions with proteins. Mol. Cancer 19 (1), 172. doi:10.1186/s12943-020-01286-3
Keywords: circ_0059706, acute myeloid leukemia, machine learning, prognosis, biomarker
Citation: Ma J, Wen X, Xu Z, Xia P, Jin Y, Lin J and Qian J (2022) Predicting the influence of Circ_0059706 expression on prognosis in patients with acute myeloid leukemia using classical statistics and machine learning. Front. Genet. 13:961142. doi: 10.3389/fgene.2022.961142
Received: 04 June 2022; Accepted: 10 October 2022;
Published: 21 October 2022.
Edited by:
Xiao Wang, Konge Larsen Aps, DenmarkReviewed by:
Jin-Yu Sun, Nanjing Medical University, ChinaDenggang Fu, Indiana University, United States
Ken Mills, Queen’s University Belfast, United Kingdom
Lin Fu, The Second Affiliated Hospital of Guangzhou Medical University, China
Copyright © 2022 Ma, Wen, Xu, Xia, Jin, Lin and Qian. This is an open-access article distributed under the terms of the Creative Commons Attribution License (CC BY). The use, distribution or reproduction in other forums is permitted, provided the original author(s) and the copyright owner(s) are credited and that the original publication in this journal is cited, in accordance with accepted academic practice. No use, distribution or reproduction is permitted which does not comply with these terms.
*Correspondence: Jiang Lin, 2651329493@qq.com; Jun Qian, qianjun0007@hotmail.com
†These authors have contributed equally to this work