- 1Acupuncture and Tuina College, Chengdu University of Traditional Chinese Medicine, Chengdu, China
- 2Acupuncture and Chronobiology Key Laboratory of Sichuan Province, Chengdu, China
Background: Ulcerative colitis (UC) is a common chronic disease of the digestive system. Recently, competitive endogenous RNAs (ceRNAs) have been increasingly used to reveal key mechanisms for the pathogenesis and treatment of UC. However, the role of ceRNA in UC pathogenesis has not been fully clarified. This study aimed to explore the mechanism of the lncRNA-miRNA-mRNA ceRNA network in UC and identify potential biomarkers and therapeutic targets.
Materials and Methods: An integrative analysis of mRNA, microRNA (miRNA), and long non-coding RNA (lncRNA) files downloaded from the Gene Expression Omnibus (GEO) was performed. Differentially expressed mRNA (DE-mRNAs), miRNA (DE-miRNAs), and lncRNA (DE-lncRNAs) were investigated between the normal and UC groups by the limma package. A weighted correlation network analysis (WGCNA) was used to identify the relative model for constructing the ceRNA network, and, concurrently, miRWalk and DIANA-LncBase databases were used for target prediction. Consecutively, the Gene Ontology (GO), Kyoto encyclopedia of genes and genomes (KEGG) pathway, and Reactome pathway enrichment analyses, protein-protein interaction (PPI) network, Cytohubba, and ClueGO were performed to identify hub genes. Additionally, we examined the immune infiltration characteristics of UC and the correlation between hub genes and immune cells using the immuCellAI database. Finally, the expression of potential biomarkers of ceRNA was validated via qRT-PCR in an experimental UC model induced by dextran sulfate sodium (DSS).
Result: The ceRNA network was constructed by combining four mRNAs, two miRNAs, and two lncRNAs, and the receiver operating characteristic (ROC) analysis showed that two mRNAs (CTLA4 and STAT1) had high diagnostic accuracy (area under the curve [AUC] > 0.9). Furthermore, CTLA4 up-regulation was positively correlated with the infiltration of immune cells. Finally, as a result of this DSS-induced experimental UC model, CTLA4, MIAT, and several associate genes expression were consistent with the results of previous bioinformatics analysis, which proved our hypothesis.
Conclusion: The investigation of the ceRNA network in this study could provide insight into UC pathogenesis. CTLA4, which has immune-related properties, can be a potential biomarker in UC, and MIAT/miR-422a/CTLA4 ceRNA networks may play important roles in UC.
Introduction
Inflammatory bowel disease (IBD), which includes Crohn’s disease (CD) and ulcerative colitis (UC), is a chronic inflammatory disorder of the gastrointestinal (GI) tract characterized by aberrant GI activation (Franzosa et al., 2019; Sun et al., 2019). UC is further characterized by an overactive immune response and destruction of the colorectal epithelium involving many complicated pathological factors (Yu et al., 2021). Pharmacologic therapy can relieve the clinical picture of UC, which mainly comprises abdominal pain, bloody stool, and diarrhea (Kornbluth and Sachar, 2010). Still, modern treatment has certain side effects, and tolerance to treatment may develop among UC patients (Marius et al., 2020; Wang Z et al., 2021). Over the last few decades, UC has become a global disease, with prevalence on every continent rising, increasing a greater economic burden on society (Ng et al., 2017). Unfortunately, the exact pathogenesis of UC remains unclear (Sarvestani et al., 2021).
In recent years, the competing endogenous RNA (ceRNA) hypothesis has proposed the synergistic regulation of different RNAs, including long non-coding RNA (lncRNA), microRNA (miRNA), transcribable pseudogenes, and circular RNAs (Salmena et al., 2011; Thomson and Dinger, 2016). There is increasing evidence that lncRNAs play critical roles in gene regulation, cell biology, human development, and several diseases (Mercer et al., 2022). LncRNAs, miRNA molecular “sponges,” can indirectly modulate gene expression through their ability to compete and sequester miRNAs, thereby inhibiting the miRNA-mediated suppression of target mRNAs (Salmena et al., 2011). In the current research, ceRNA has been closely related to immune diseases, such as rheumatoid arthritis (RA), Sjögren’s syndrome (SS), systemic lupus erythematosus (SLE), and systemic sclerosis (SSc) (Chen et al., 2021; Song et al., 2021; Wu et al., 2021; Zhang Y et al., 2020). Similarly, ceRNA has also been recently identified as one of the key mechanisms involved in the development of IBD (Nie and Zhao, 2020; Liu et al., 2021).
In the present study, we obtained the original data from the NCBI Gene Expression Omnibus (GEO) DataSets database, including the UC group and the normal control group. Then, we obtained differentially expressed (DE)-lncRNAs, DE-miRNAs, and DE-mRNAs through the gene differential expression analysis. We aimed to construct and explore a complete lncRNA-miRNA-mRNA network to determine the specific immune infiltration characteristics of UC. Finally, we validated the bioinformatics analysis results using the UC model induced by dextran sulfate sodium (DSS) to provide a basis for further understanding of UC molecular mechanism and identifying potential biomarkers.
Materials and methods
GEO dataset selection
A flowchart of the study design is depicted in Figure 1. The datasets of mRNAs, miRNAs, and lncRNAs were downloaded from the GEO database (http://www.ncbi.nlm.nih.gov/geo). For a better understanding of the ceRNA mechanism in UC, the independent datasets of GSE87466 for mRNAs, GSE48957 for miRNAs, and GSE67106 for lncRNAs were selected, including 87 UC tissues and 21 normal tissues, 10 UC tissues and 10 normal tissues, and 21 UC tissues and 22 normal tissues from intestinal biopsies, respectively. Table 1 lists the basic details of these three datasets.
Differential expression analysis and weighted correlation network analysis (WGCNA)
The limma package was used for differential expression analysis with a p-value of <0.05 and |log2 (fold change) | > 1 to define DE-mRNAs, DE-miRNAs, and DE-lncRNAs (Davis and Meltzer, 2007). The relative importance of mRNAs, lncRNAs, and their module membership was assessed using the WGCNA package (Langfelder and Horvath, 2008). The minimum number of genes per module was set to 30. Clustering by hierarchical analysis identified gene co-expression modules, which were then colored arbitrarily for identification.
Functional enrichment analysis and protein-protein interaction (PPI) network construction
DAVID (http://david.abcc.ncifcrf.gov) was used to analyze the Kyoto encyclopedia of genes and genomes (KEGG) and Gene Ontology (GO) terms (Yu et al., 2012). A high-confidence set of human PPI networks was constructed by the STRING database (https://string-db.org, version 11.5) (Szklarczyk et al., 2021). A CytoHubba plug-in (http://apps.cytoscape.org/apps/cytohubba) in Cytoscape (version 3.9.1) was applied to identify the top 10 hub genes in PPI networks through the degree algorithm (Chin et al., 2014; Otasek et al., 2019). In ClueGo (http://apps.cytoscape.org/apps/cluego), kappa statistics were used to construct and compare networks of functionally related GO terms and Reactome pathways (Bindea et al., 2009; Croft et al., 2014; Szklarczyk et al., 2021).
ceRNA network construction
We used the miRWalk database (http://mirwalk.umm.uni-heidelberg.de) to predict the interaction between miRNA and mRNA (Dweep and Gretz, 2015). We predicted lncRNA-miRNA interactions via the DIANA-LncBase v2 database (http://www.microrna.gr/LncBase) (Paraskevopoulou et al., 2016). DE-mRNA, DE-lncRNA, DE-miRNA, WGCNA module, predict lncRNA, and predict mRNA were selected to construct the ceRNA network. A visualization of the ceRNA network was performed by Cytoscape (Otasek et al., 2019).
Immune infiltration analysis
The immuCellAI database (http://bioinfo.life.hust.edu.cn/web/ImmuCellAI) was used to estimate differential immune cell infiltration between the normal and UC groups (Miao et al., 2020).
DSS-induced UC mice model
Male C57BL/6J mice (26 ± 2 g) were obtained from GemPharmatech Co., Ltd. (Chengdu, China). The animals were fed standard rodent chow and water ad libitum under ambient temperature (23 ± 1 °C) and a 12-h light/dark cycle in their pathogen-free enclosure. The mice were randomized into the normal and UC groups. In the UC group, mice were administered 2.5% DSS (MP Biomedicals, CA, United States ) for 7 days, while the normal group only received distilled water. Daily measurements of food intake, body weight, stool consistency, and fecal bleeding were taken.
Disease activity index (DAI)
DAI was calculated by combining body weight loss, feces status, and occult levels. The scores for each subscale are shown in Supplementary Table S1.
Enzyme-linked immunosorbent assay (ELISA)
Centrifugation at 3,000 rpm for 20 min was used to collect supernatants from the different groups. In accordance with the manufacturer’s protocols, ELISA kits (Elabscience, Wuhan, China) were used to detect tumor necrosis factor (TNF)-α and interferon (IFN)-γ levels in the supernatant. Then, the absorption coefficients were applied to calculate TNF-α and IFN-γ concentrations.
Hematoxylin and eosin (HE) staining
In the normal and UC groups, the colon tissues were fixed in buffered 4% formalin for 2 days. The samples were sectioned at a thickness of 5 mm and stained with HE for histopathological examination using a light microscope (Olympus Corporation, Tokyo, Japan). Supplementary Table S2 presents the scores for each subscale.
Real-time quantitative PCR (qRT-PCR)
MolPure® TRIeasyTM Plus Total RNA Kit (YEASEN, Shanghai, China) was used to isolate total mRNA from colon tissues, and cDNA was prepared using Hifair®III first Strand cDNA Synthesis SuperMix for qPCR (YEASEN, Shanghai, China) according to the manufacturer’s instructions. We then analyzed the resulting cDNA by RT-qPCR using Hieff UNICON universal Blue qPCR SYBR Green Master Mix (YEASEN, Shanghai, China). The primer sequences are seen in Supplementary Table S3. Target mRNA expression levels were analyzed using the comparative 2-Ct method by normalizing to levels of β-actin.
Western blot (WB)
Western blot analysis was performed as previously described (Sun et al., 2017). The protein extracts of mice colon were prepared using a lysis buffer supplemented with ethylenediaminetetraacetic acid (EDTA)-free complete protease inhibitors. We extracted proteins and subjected them to 10% sodium dodecyl sulfate-polyacrylamide gels (SDS-PAGE). Then, a polyvinylidene difluoride membrane was used to transfer the bands. After blocking with 5% nonfat dry milk in Tris-buffered saline supplemented with 0.1% Tween 20 for 2 h at room temperature, the membranes were incubated overnight at 4 °C with the anti-CTLA4 antibody (ab237712, 1:1000 Abcam, MA, United States ). The membranes were then incubated with a secondary antibody (HS101-01, 1:5000, TRAN, Beijing, China) at 37 °C for 2 h. Western blotting of the same membranes with an anti-β-actin antibody (20536-1-AP, 1:1000, Proteintech, Wuhan, China) was used to normalize the results.
Immunofluorescence (IF)
Sections of samples were deparaffinized in xylene and rehydrated in alcohol series with decreasing concentrations. We washed sections three times in PBS for 5 min, then blotted them with 5% goat serum for 1 h at 37°C. Slices were incubated with the Anti-CTLA4 antibody (ab237712, 1:200, Abcam, MA, United States ) overnight at 4 °C. These sections were washed three times in phosphate-buffered saline (PBS) and then incubated with Cy3–conjugated Affinipure Goat Anti-Rabbit IgG (SA00009-2, 1:100, Proteintech, Wuhan, China) at 37°C for 2 h. Then, the sections were rinsed three more times and stained for 5 minutes with DAPI (Biyuntian, Shanghai, China). The results were imaged by Pannoramic 250FLASH (3DHISTECH, Budapest, Hungary).
Statistical analysis
Statistical analysis was performed using SPSS software (version 24.0, SPSS Inc., Chicago, United States ), and pictures were drawn using GraphPad Prism software (version 8.0, GraphPad Prism Software Inc., CA, United States ). PPI network construction and analysis were performed according to the required confidence threshold set (combined score) of >0.9. Spearman correlation analysis was used to analyze the correlation between hub genes and immune cells. The receiver operating characteristic (ROC) curves were generated with the R package pROC, and the corresponding area under the curve (AUC) values were calculated. The negatively related mRNA-lncRNA co-expression interactions and positively related mRNA-miRNA and lncRNA-miRNA were removed to construct the lncRNA-miRNA-mRNA ceRNA network construction. The qRT-PCR and WB results were analyzed by Student’s t tests. All performed statistical tests were two-sided, and a P of >0.05 was chosen as the threshold of statistical significance. The results were presented as mean ± standard deviation (SD).
Results
Identification of DE-miRNAs
In this study, the expression levels of GSE48957 miRNAs, which were obtained from the GEO database, were explored. As shown in the volcano plot and the heatmap, we found that 7 DE-miRNAs were significantly increased, and 12 DE-miRNAs were decreased in the UC group compared with the normal group (Figure 2 and Table 2).
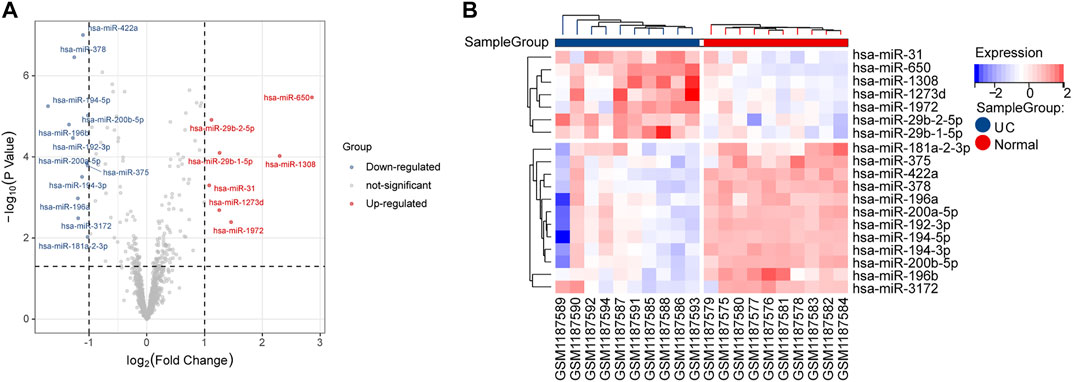
FIGURE 2. DE-miRNA screening. (A) Volcano plots of DE-miRNAs from GSE48957. (B) Heatmap of DE-miRNAs from GSE48957.
Enrichment analysis of DE-mRNAs
We investigated the expression levels of mRNAs related to GSE87466, which were obtained from the GEO database. As shown in the volcano plot and the heatmap, we found that 537 DE-mRNAs were significantly increased, and 270 DE-mRNAs were decreased in the UC group compared with the normal group (Figures 3A, B). Then, a functional enrichment analysis of GO and KEGG was performed to explore the potential function of 807 DE-mRNAs. Among them, the top five BP, top 5 MF, and top 5 CC GO terms are shown in Figure 3C. “Leukocyte migration,” “humoral immune response,” “response to molecule of bacterial origin,” “leukocyte chemotaxis,” and “response to lipopolysaccharide” were the top five enriched terms of BP. The top five GO terms on CC were “external side of plasma membrane,” “collagen-containing extracellular matrix,” “secretory granule membrane,” “membrane microdomain,” and “membrane region.” In categories of MF, “chemokine receptor binding,” “chemokine activity,” “glycosaminoglycan binding,” “G protein-coupled receptor binding,” and “cytokine activity” were major terms. KEGG analysis showed that obvious DE-mRNAs were significantly enriched in 37 KEGG pathways, such as “viral protein interaction with cytokine and cytokine receptor,” “IL-17 signaling pathway,” and “cytokine-cytokine receptor interaction,” as shown in Figure 3D.
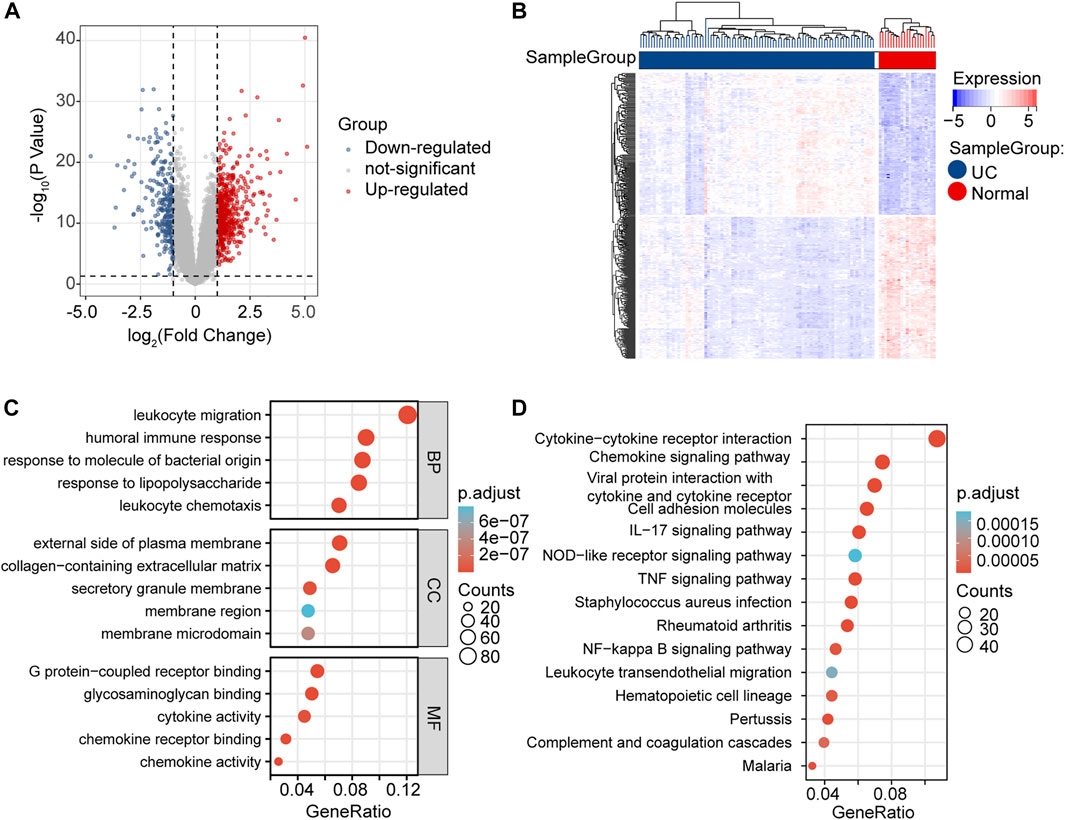
FIGURE 3. DE-mRNAs screening and enrichment analysis. (A) Volcano plots of DE-mRNAs from GSE87466. (B) Heatmap of DE-mRNAs from GSE87466. (C) BP, CC, and MF presented the top five significant terms of GO enrichment analysis. (D) Top 15 significant terms of KEGG enrichment analysis.
Identification of mRNAs co-expression modules via WGCNA
We analyzed the gene co-expression networks and identified gene modules using WGCNA to further confirm the key mRNAs in DE-mRNAs. When β was 26 (a soft threshold), the scale-free R2 was 0.85 to obtain a higher average connectivity degree (Figure 4A). Ten different gene co-expression modules were determined in DE-mRNAs after the insignificant gray module was excluded (Figure 4B). As shown in Figure 4C, lightcyan modules showed the most positive correlation (module trait correlation = 0.69), and lightgreen modules exhibited a negative correlation (module trait correlation = −0.62) with the UC group.
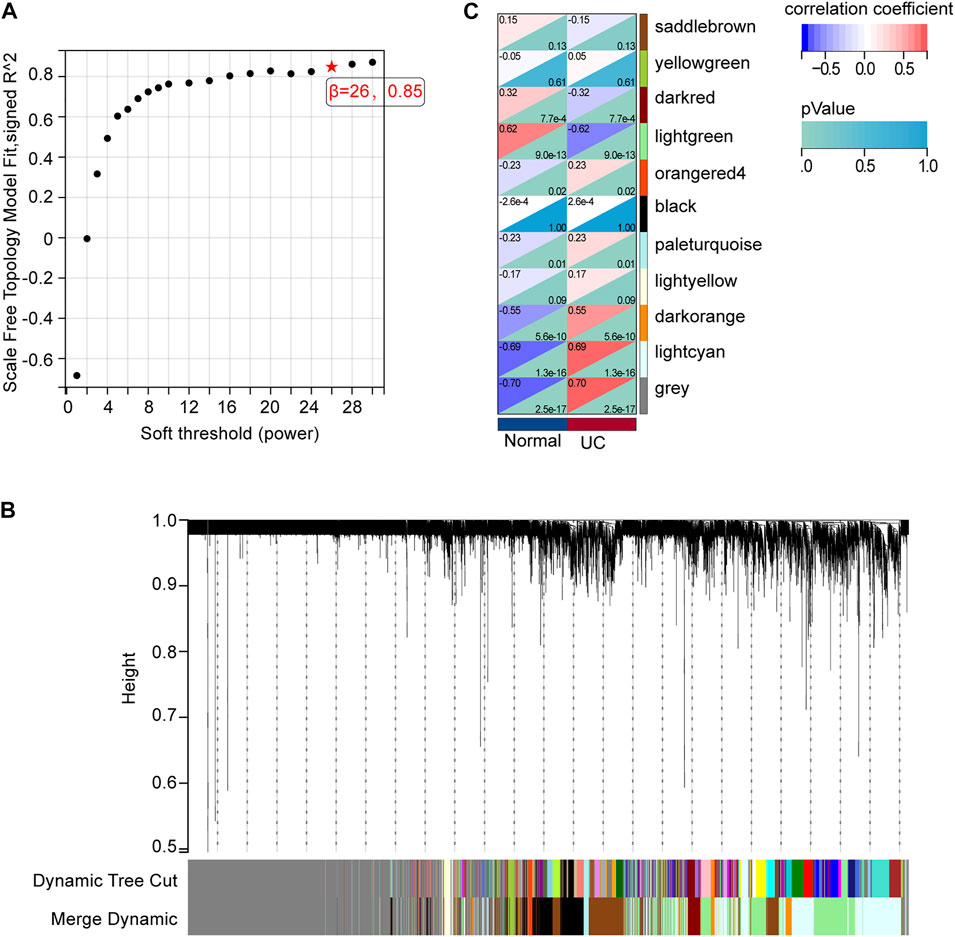
FIGURE 4. mRNA modules analyzed by WGCNA. (A) Determination of soft-thresholding power. (B) Clustering dendrogram. (C) The module-trait relationships.
Enrichment analysis of interacted DE-mRNAs and screened hub DE-mRNAs
To further define the DE-mRNAs of UC, the mRNAs targeted by DE-miRNAs were predicted by using the miRwalk database, and 330 DE-mRNAs were defined in the intersection between DE-mRNAs, WGCNA modules, and DE-miRNA-predict-mRNA (Figure 5A). To further understand the biological functions of these mRNAs, GO functional enrichment and Reactome pathway enrichment were conducted by ClueGO of Cytoscape. As can be seen in Figure 5B and Supplementary Figure S1A, within the BP category, “mononuclear cell differentiation” (29.03%) was the most dominant group, followed by “regulation of B cell proliferation” (12.9%), “peptidyl-tyrosine phosphorylation” (9.68%), “angiogenesis” (9.68%), and “regulation of tumor necrosis factor production” (9.68%). The top CC categories were “cell projection membrane” (23.08%), “basal plasma membrane” (15.38%), “membrane raft” (15.38%), and “external side of plasma membrane” (15.38%), as indicated in Figure 5C and Supplementary Figure S1B. MF mainly involved “CXCR chemokine receptor binding” (21.05%), “non-membrane spanning protein tyrosine kinase activity” (10.53%), “immune receptor activity” (10.53%), and “growth factor receptor binding” (10.53%) (Figure 5D and Supplementary Figure S1C). Meanwhile, we found that “immune system” (13.64%), “DAP12 interactions” (13.64%), “signaling by interleukins” (9.09%), and “chemokine receptors bind chemokines” (9.09%) were significantly enriched in Reactome pathway terms, as shown in Figure 5E and Supplementary Figure S1D.
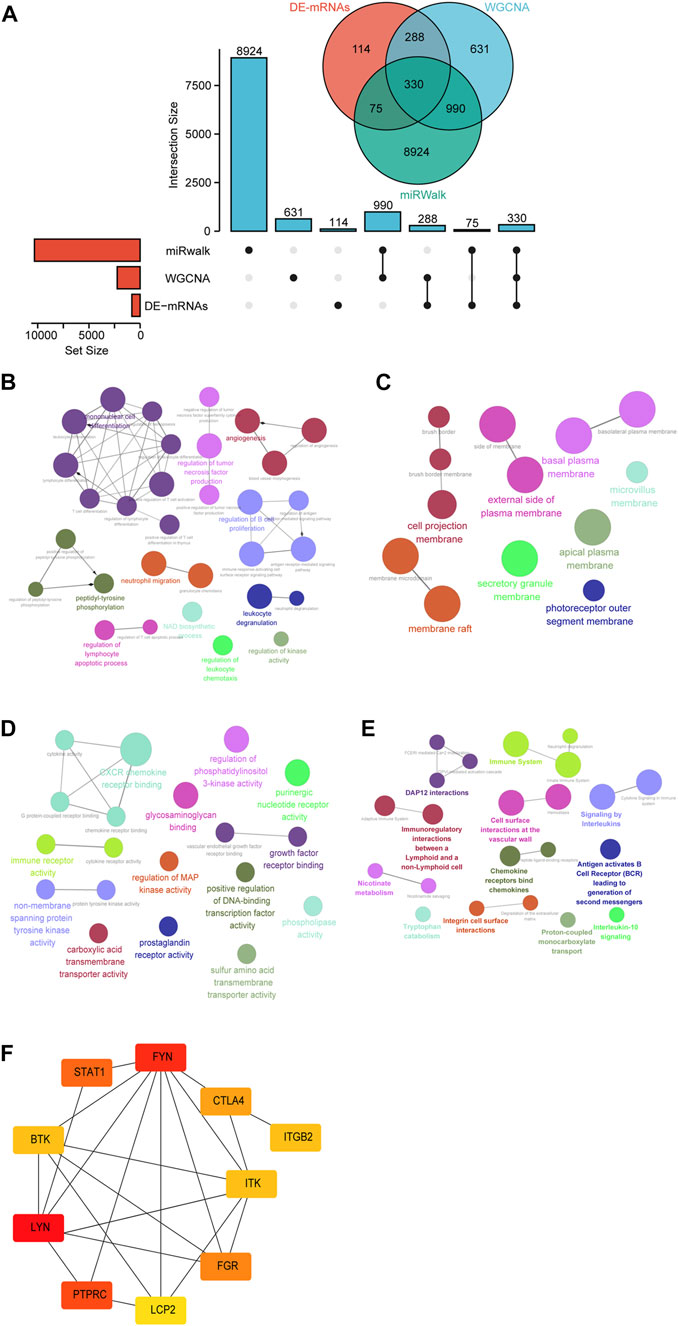
FIGURE 5. Identification of candidate overlapping mRNA and function annotation. (A) The intersection of DE-mRNAs, WGCNA modules, and miRwalk-predict. (B) BP of overlapping mRNA visualized by ClueGO. (C) CC of overlapping mRNA visualized by ClueGO. (D) MF of overlapping mRNA visualized by ClueGO. (E) Reactome pathway of overlapping mRNA visualized by ClueGO. (F) Hub genes were identified in the overlapping mRNA via cytohubba.
To further investigate the functions of these mRNAs, we performed a PPI network analysis on the String database and selected mRNAs with the highest confidence. A total of 103 DE-mRNAs were filtered into the DE-mRNAs’ PPI network complex containing 103 nodes and 142 edges (Supplementary Figure S2). Subsequently, CytoHubba was used to identify the hub mRNAs with the highest connectivity in the PPI network. The top 10 hub mRNAs, such as LYN, FYN, PTPRC, STAT1, FGR, CTLA4, BTK, ITK, ITGB2, and LCP2, are shown in Figure 5F.
Identifying interacted lncRNAs via DE-lncRNAs, WGCNA, and predicted lncRNAs
To search for the lncRNAs involved in our study, we analyzed the expression of GSE67106 lncRNAs from the GEO dataset. 637 DE-lncRNAs were obtained, with 441 up-regulated and 196 down-regulated DE-lncRNAs (Figures 6A, B). In WGCNA analysis, the soft threshold power was selected as eight to ensure a scale-free network distribution (scale-free index R2 = 0.87) (Figure 6C). The lncRNAs co-expression modules and the corresponding hierarchical clustering dendrograms of these lncRNAs are shown in Figure 6D. As shown in Figure 6E, blue modules showed the most positive correlation (module trait correlation = 0.89), and purple modules exhibited a negative correlation (module trait correlation = −0.68) with the UC group. The DE-lncRNAs interacted with WGCNA modules, and predicted lncRNAs, as shown in Figure 6F, were used to construct the ceRNA network in the next step.
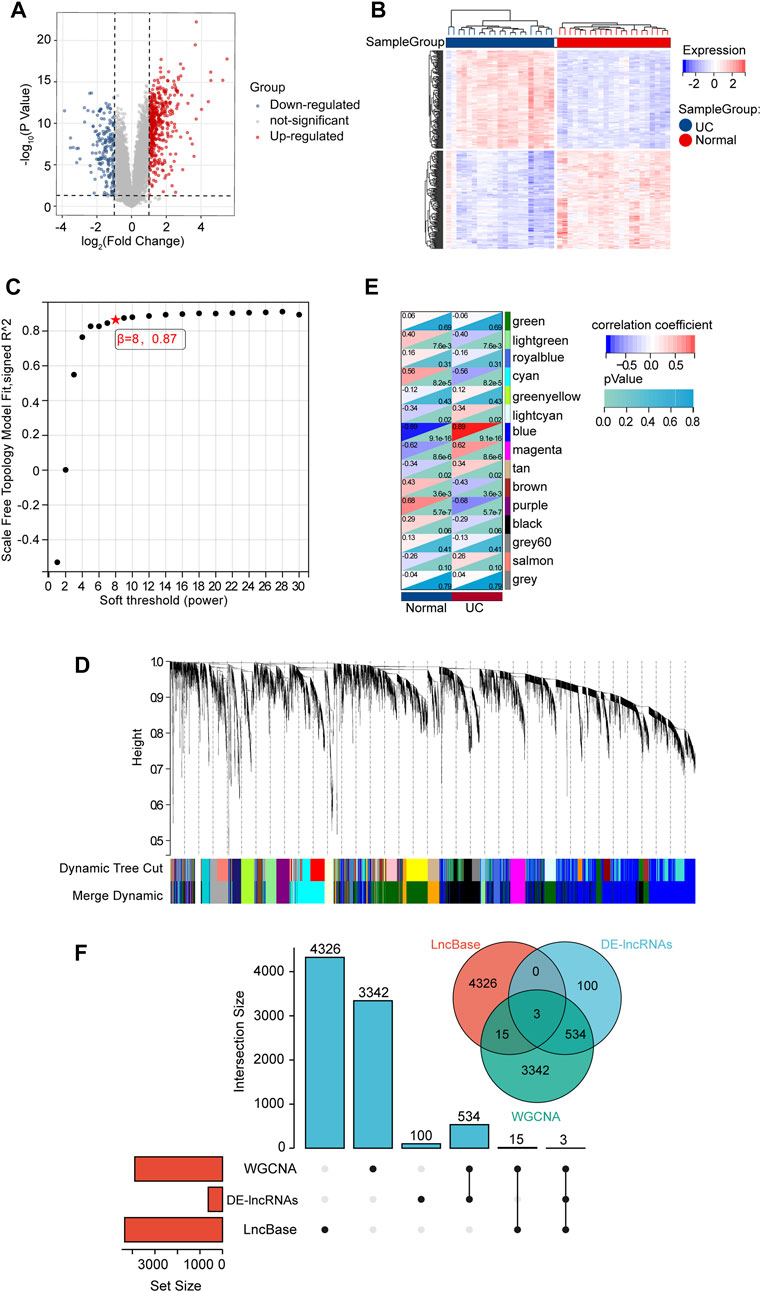
FIGURE 6. DE-lncRNA screening and identification of candidate overlapping DE-lncRNA. (A) Volcano plots of DE-mRNAs from GSE67106. (B) Heatmap of DE-mRNAs from GSE67106. (C) Determination of soft-thresholding power. (D) Clustering dendrogram. (E) The module-trait relationships. (F) The intersection of DE-lncRNAs, WGCNA modules, and LncBase-predict.
CeRNA network constructed
The above-described interactions between lncRNA-miRNA, miRNA-mRNA, and lncRNA-mRNA were used to establish a lncRNA-miRNA-mRNA network via Cytoscape. We constructed a ceRNA network based on eight miRNA nodes, 10 mRNA nodes, and three lncRNA nodes, as shown in Figure 7A. We finally identified the ceRNA network among four mRNAs, two miRNAs, and two lncRNAs (Figure 7B).
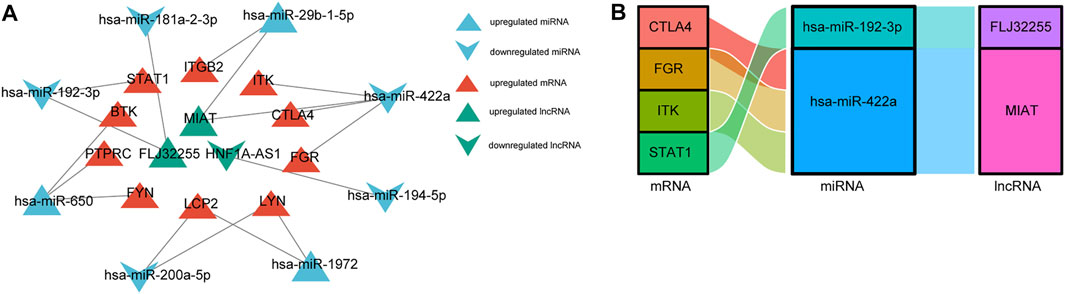
FIGURE 7. The construction of (A) ceRNA network. The triangle represents up-regulate, and v represents down-regulate. Red color represents mRNAs, blue color represents miRNAs, and green color represents lncRNAs. (B) Sankey diagram of final ceRNA network. The squareness represents lncRNAs, miRNAs, and mRNAs, and the size indicates their degree of connection.
ROC analysis and validation
Representative ROC curves for the finally identified ceRNA network mRNAs (ITK, CTLA4, FGR, and STAT1) are shown in Figure 8A. We found that except for ITK and FGR, the AUCs for the responsiveness of CTLA4 and STAT1 were >0.90, which demonstrated excellent responsiveness to predict UC.
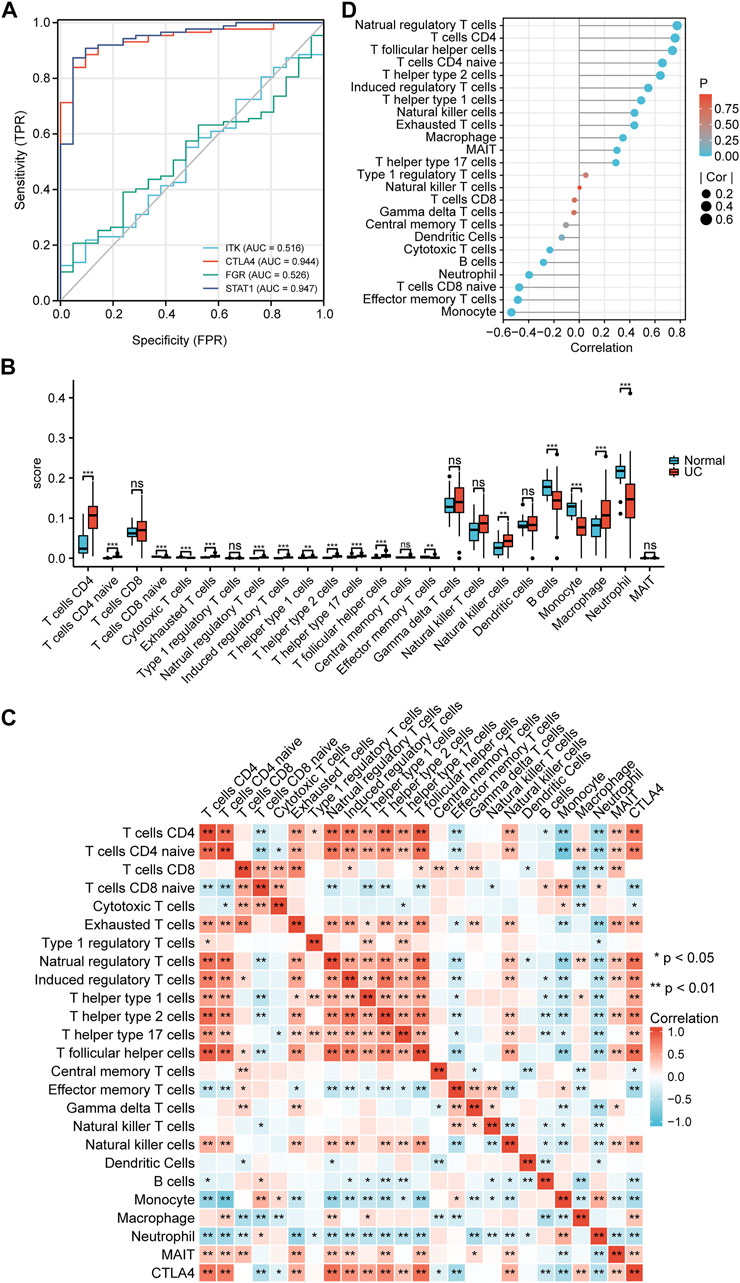
FIGURE 8. Validation and immune infiltration analysis. (A) ROC of mRNAs in the ceRNA network. (B) The differential of immune infiltration between the UC and normal groups. (C) Correlation matrix of immune cell proportions and CTLA4. (D) Lollipop chart of the correlation between immune cells and CTLA4. *p < 0.05, **p < 0.01.
Immune infiltration analysis
To further test our hypothesis, the immune cell abundance between the normal and UC groups was calculated using the immuCellAI database. As shown in Figure 8B, the fraction of CD4+ T cells, CD4 naïve T cells, exhausted T cells, natural regulatory T cells, induced regulatory T cells, T helper type 1 cells, T helper type 2 cells, T helper type 17 cells, T follicular helper cells, natural killer cells, and macrophages in the UC group were higher than in the normal group while that of CD8 naïve T cells, cytotoxic T cells, effector memory T cells, monocytes, and neutrophils was lower. CTLA4 expression levels correlated well with natural regulatory T follicular helper cells, CD4+ T cells, natural regulatory T cells, CD4 naïve T cells, T helper type 2 cells, induced regulatory T cells, and monocytes (Pearsons correlation coefficient >0.5, p < 0.01) (Figures 8C, D).
DSS-induced mice model validation
After the DSS-induced mice model establishment (Supplementary Figure S3), we examined the mRNA expression levels of CTLA4 and three strongly correlated mRNAs (FYN, ITK, and IGTB2) in the top 10 hub mRNAs (Figure 9A) and the lncRNA expression levels of myocardial infarction-associated transcript (MIAT) with qRT-PCR to verify the dataset’ reliability. Compared with the normal group, the expression levels of CTLA4, FYN, ITK, ITGB2, and MIAT in the UC group were significantly increased (Figures 9B–F). Additionally, IF and WB confirmed that the critical hub gene CTLA4 was significantly elevated at the protein level compared to the normal group (Figures 9G–I).
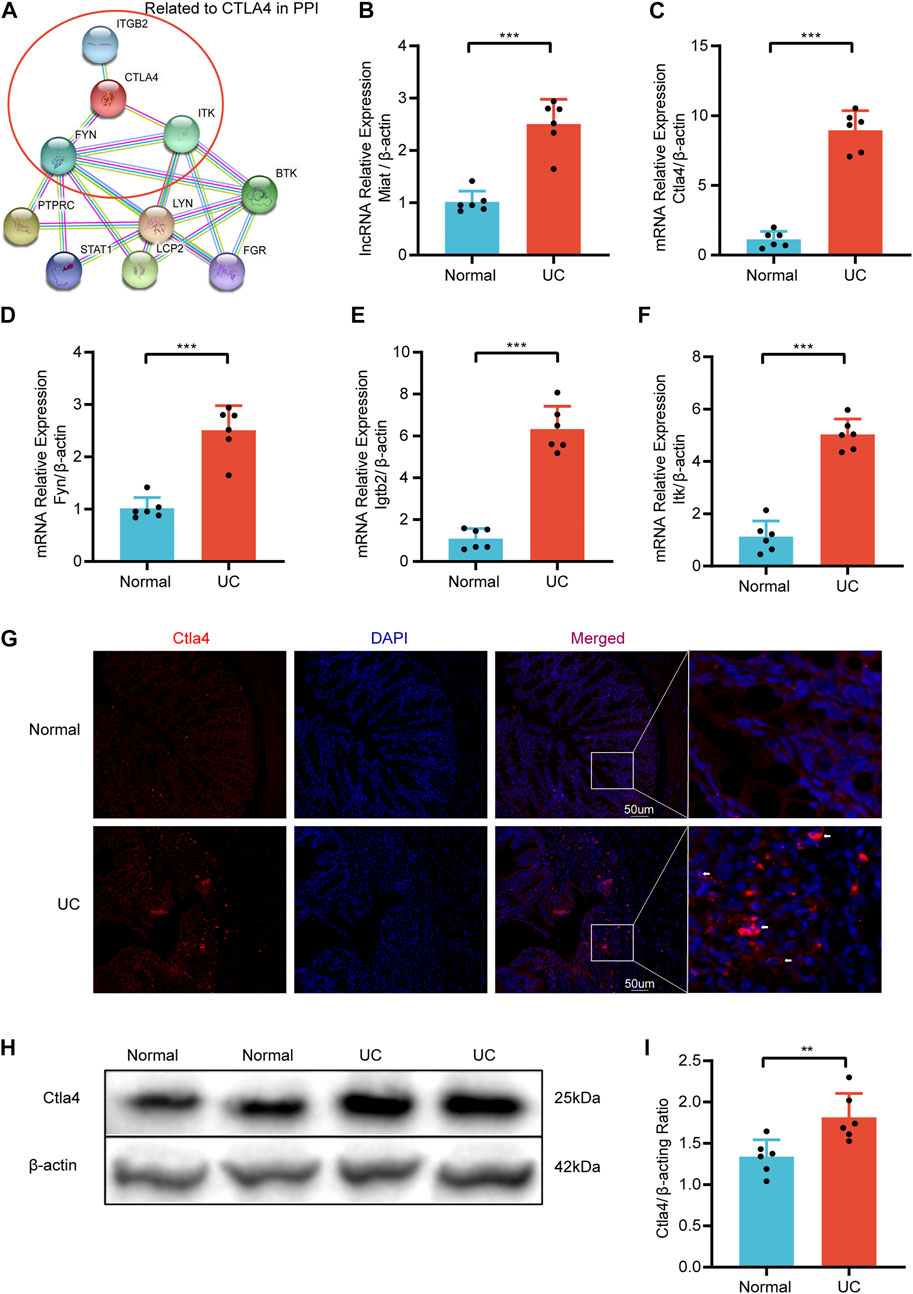
FIGURE 9. The relative expression was validated via qRT-PCR. (A) Hub mRNAs Related to CTLA4 in PPI. The relative expression of Miat (B), Clta4(C), Fyn (D), Igtb2 (E), and Itk (F) in DSS-induced mice and Normal mice. (G) Representative immunofluorescence image of CTLA4 between DSS-induced mice and Normal mice. Red, Ctla4; blue, DAPI nuclear staining (n = 6/group). (H) Representative western blot image of Ctla4 between DSS-induced mice and Normal mice. (I) Quantification of western blot analysis. Dates are shown as mean ± SD. **p < 0.01, ***p < 0.001.
Discussion
UC is a chronic inflammatory disease characterized by dysregulated immune responses in the colon with a rising incidence worldwide (Ungaro et al., 2017). Due to the complex pathogenesis and unclear pathophysiological mechanism of UC, the current clinical treatment has mainly focused on alleviating the symptoms (Sarvestani et al., 2021; Ungaro et al., 2017). CeRNA cross-talk, mediated by miRNA in complex ceRNA networks, and the interactions between miRNA and lncRNA have been shown to play critical roles in the pathogenesis of various immune disorders (Chen et al., 2021; Salmena et al., 2011; Song et al., 2021; Thomson and Dinger, 2016; Wu et al., 2021; Zhang Y et al., 2020). Studies have shown that lncRNA-ITSN1-2 acts as a competing endogenous RNA for IL-23R via sponging miR-125a, and lncRNA meg3 serves as a competing RNA for IL-10 via sponging miR-98-5p in IBD (Nie and Zhao, 2020; Wang Y et al., 2021). In UC-associated colon cancer, CXCR4 promotes the progression of the disease by recruiting immune cells and enhancing cytoskeletal remodeling through the lncRNA XIST/miR-133a-3p/RhoA signaling (Yu et al., 2019). Therefore, the construction of the ceRNA is essential to understanding the immune-regulation mechanisms and exploring potential biomarkers in UC.
In the present study, DE-mRNAs, DE-miRNAs, and DE-lncRNAs were identified in UC and normal colon tissues from GSE87466, GSE48957, and GSE67106, respectively. Next, UC-related modules were selected by WGCNA. Additionally, we used DE-miRNAs to predict interacted mRNAs and lncRNAs through the miRwalk and LncBase databases. Intersection-mRNAs were identified at the intersection of DE-mRNAs, mRNAs in the WGCNA module, and predicted mRNAs. Similarly, intersection-lncRNAs were also obtained by the above-mentioned method. Thus, we proposed a regulatory network of ceRNA relying on intersection-mRNAs, intersection-lncRNAs, and DE-miRNAs. Finally, according to the competition rules of ceRNA, we further established a ceRNA regulatory network including four mRNAs, two lncRNAs, and two miRNAs. GSE87466 from colon tissues was validated by the ROC analysis to determine whether eight mRNAs from ceRNA were used as UC biomarkers. The results showed that AUCs of CTLA4 and STAT1 exceeded 0.9, suggesting that these genes could be potential biomarkers of UC.
CTLA4, predominantly found in intracellular vesicles in FoxP3+ T regulatory cells or activated conventional T cells, is a key regulator of autoimmunity and acts as an immune checkpoint (Hodi et al., 2014; Rowshanravan et al., 2018; Wang et al., 2015). With the wide application of immune checkpoint inhibitors (ICIs) in treating various diseases, colitis, as one of the side effects of ICIs, has also received more and more attention (Ouyang et al., 2021). For example, an observational study focusing on gastrointestinal immune-related adverse events of ICIs has suggested that patients treated with anti-CTLA4 antibodies show a higher incidence of events and more severe symptoms (Yamada et al., 2021). Furthermore, compared with non-inflamed individuals, CTLA4 is increased in biopsies from UC (Lushnikova et al., 2021). In fact, as shown by the results of immune infiltration analysis, the expression level of CTLA4 is strongly associated with many types of immune cells in UC. Studies have confirmed that CTLA4 may affect the progression of UC by secreting IL-10 from regulatory T cells (Coquerelle et al., 2009; Liu et al., 2003). Moreover, CTLA4 can elicit a CD4+ T cell response, blocking T cell homing and increasing UC severity (Zhang S et al., 2020). CTLA-4 has a particularly important role in the microbe-rich barriers of the intestine (Rowshanravan et al., 2018). Patients treated with anti-CTLA-4 have shown fluctuating levels of antibodies against gastrointestinal commensals, suggesting dysregulated mucosal immunity with CTLA-4 blockade (Berman et al., 2010). These results suggest that CTLA4 is likely to be an immune-related biomarker candidate for UC.
In this study, we confirmed that CTLA4 expression was regulated via the MIAT/miR-422a/CTLA4 ceRNA network. In the previous study, lncRNA MIAT, as a genetic risk factor for myocardial infarction, has become the focus of extensive studies (Yang et al., 2021). With the deepening of research, it has been found that MIAT is also closely related to the occurrence and development of inflammation (Meydan et al., 2018; Qu et al., 2021). Interestingly, MIAT has been positively correlated with the number of immune cells in various diseases, such as B cells, T lymphocytes, and macrophages, and the expression of CTLA4 in the ceRNA network constructed in this study (Peng et al., 2020; Ye et al., 2021). Furthermore, MIAT was up-regulated in a time-dependent manner during the differentiation of bone marrow mesenchymal stem cells into endothelial cells (Wang et al., 2018). MIAT may also function through a feedback loop with AKT to regulate human lens epithelial cell function by increasing oxidative stress and inflammation factors (Shen et al., 2016). For those reasons, we considered that the role of MIAT in UC is not only in regulating inflammatory immunity but also in regulating the function of intestinal epithelial cells and vascular endothelial cells. MIAT acts as a post-transcriptional regulator by interacting with miR-422a, which has been reported as a kind of important molecule to participate in the formation of the immune landscape (Peng et al., 2020). Research has indicated that miR-422a may affect the inflammatory response in UC colon tissue by regulating the expression level of IL-33, which has been shown to cause a T helper 2-like cytokine response in immune cells (Dubois-Camacho et al., 2019; Kobori et al., 2010). Long-standing IBD has an approximately 2–3-fold increased risk of colorectal cancer (CRC) (Shah and Itzkowitz, 2022). Interestingly, it has been found that miR-422a also regulates the expression of AKT1, MAPK1, MAPKK6, and other inflammatory-related factors in CRC, thereby affecting the proliferation and apoptosis of CRC cells (Li et al., 2018; Liang et al., 2021; Wei et al., 2017). Additionally, studies on the expression profile of miRNAs, which were not only in CRC tissue but also in the serum, have suggested that miR-422a is highly dysregulated in CRC patients compared to healthy individuals (Faltejskova et al., 2012; Qin et al., 2015; Zheng et al., 2014). Therefore, we believe that miR-422a plays a vital role in both UC and CRC and is an essential biomarker for the prognosis of colitis.
The DSS-induced UC model is widely used due to its simplicity, reliability, and many similarities to human UC (Chassaing et al., 2014). Therefore, we established DSS-induced UC in mice and validated the expression of Miat, Ctla4, and associated mRNAs, such as Fyn, Itk and Itgb2, in this model. The results were consistent with the previous finding and revealed that the expression level of the RNA expression levels of Miat, Ctla4, Fyn, Itk, and Itgb2 were obviously increased in the UC model by qRT-PCR. Moreover, IF and WB verified that the changing trend of CTLA4 was consistent with mRNA at the protein level, which further confirmed our hypothesis. Thus, this study shows that the MIAT/miR-422a/CTLA4 ceRNA network may play an essential role in the immune responses in UC.
Conclusion
In summary, we constructed a MIAT/miR-422a/CTLA4 ceRNA network in UC. We found an exact relationship between CTLA4 and MIAT, which is a novel insight into the molecular mechanism of UC. CTLA4 was significantly positively correlated with up-regulated immune cells, and MIAT was lncRNA closely related to vascular lesions. Our results help improve understanding of the UC mechanism and provide future potential biomarkers for UC diagnosis and prognosis.
Data availability statement
The datasets presented in this study can be found in online repositories. The names of the repository/repositories and accession number(s) can be found below: Gene Expression Omnibus, accession number GSE87466, GSE48957, and GSE67106.
Ethics statement
The animal study was reviewed and approved by the The Animal Welfare Officer of the Chengdu University of Traditional Chinese Medicine (Ethics approval number: 2019-04).
Author contributions
LD drafted the manuscript and analyzed the data. RZ performed most of the experiments. RZ and QH performed animal breeding and part of the animal experiments. QW and SY performed and supervised the study. YS and HL provided suggestions for this study and revised the article. All authors contributed to the article and approved the submitted version.
Funding
This work was supported by the National Key R&D Program of China (No.2019YFC1709001), the National Natural Science Foundation of China (Nos. 82174512 and 81873383), the Innovation Team and Talents Cultivation Program of National Administration of Traditional Chinese Medicine (No. ZYYCXTD-D-202003), and the Fund of Science and Technology Department of Sichuan Province, China (Nos. 2021ZYD0081 and 2022ZDZX0033).
Acknowledgments
We are grateful to the technical team of Acupuncture and Tuina School at Chengdu University of Traditional Chinese Medicine.
Conflict of interest
The authors declare that the research was conducted in the absence of any commercial or financial relationships that could be construed as a potential conflict of interest.
Publisher’s note
All claims expressed in this article are solely those of the authors and do not necessarily represent those of their affiliated organizations, or those of the publisher, the editors and the reviewers. Any product that may be evaluated in this article, or claim that may be made by its manufacturer, is not guaranteed or endorsed by the publisher.
Supplementary material
The Supplementary Material for this article can be found online at: https://www.frontiersin.org/articles/10.3389/fgene.2022.951243/full#supplementary-material
References
Berman, D., Parker, S. M., Siegel, J., Chasalow, S. D., Weber, J., Galbraith, S., et al. (2010). Blockade of cytotoxic t-lymphocyte antigen-4 by ipilimumab results in dysregulation of gastrointestinal immunity in patients with advanced melanoma. [Clinical Trial, Phase II; Journal Article; Randomized Controlled Trial; Research Support, Non-U.S. Gov't]. Cancer Immun. 10, 11.
Bindea, G., Mlecnik, B., Hackl, H., Charoentong, P., Tosolini, M., Kirilovsky, A., et al. (2009). ClueGO: A Cytoscape plug-in to decipher functionally grouped gene ontology and pathway annotation networks.Research support, non-U.S. Gov't]. J. Artic. 25 (8), 1091–1093. doi:10.1093/bioinformatics/btp101
Chassaing, B., Aitken, J. D., Malleshappa, M., and Vijay-Kumar, M. (2014). Dextran sulfate sodium (dss)-induced colitis in mice. [Journal article; research support, N.I.H., extramural; research support, non-U.S. Gov't]. Curr. Protoc. Immunol. 104, 15–25. doi:10.1002/0471142735.im1525s104
Chen, X., Cheng, Q., Du, Y., Liu, L., and Wu, H. (2021). Differential long non-coding RNA expression profile and function analysis in primary Sjogren's syndrome. BMC Immunol. 22 (1), 47. doi:10.1186/s12865-021-00439-3
Chin, C. H., Chen, S. H., Wu, H. H., Ho, C. W., Ko, M. T., Lin, C. Y., et al. (2014). cytoHubba: identifying hub objects and sub-networks from complex interactome.Research Support, Non-U.S. Gov't]. J. Artic. Syst. Biol. 8, 1. doi:10.1186/1752-0509-8-S4-S11
Coquerelle, C., Oldenhove, G., Acolty, V., Denoeud, J., Vansanten, G., Verdebout, J. M., et al. (2009). Anti-CTLA-4 treatment induces IL-10-producing ICOS+ regulatory T cells displaying Ido-dependent anti-inflammatory properties in a mouse model of colitis. Gut 58 (10), 1363–1373. doi:10.1136/gut.2008.162842
Croft, D., Mundo, A. F., Haw, R., Milacic, M., Weiser, J., Wu, G., et al. (2014). The reactome pathway knowledgebase. [Journal article; research support, N.I.H., extramural; research support, non-U.S. Gov't]. Nucleic Acids Res. 42, D472–D477. Database issue). doi:10.1093/nar/gkt1102
Davis, S., and Meltzer, P. S. (2007). GEOquery: A bridge between the gene expression Omnibus (GEO) and BioConductor. Bioinformatics 23 (14), 1846–1847. doi:10.1093/bioinformatics/btm254
Dubois-Camacho, K., Diaz-Jimenez, D., De la Fuente, M., Quera, R., Simian, D., Martinez, M., et al. (2019). Inhibition of miR-378a-3p by inflammation enhances IL-33 levels: A novel mechanism of alarmin modulation in ulcerative colitis. Front. Immunol. 10, 2449. doi:10.3389/fimmu.2019.02449
Dweep, H., and Gretz, N. (2015). miRWalk2.0: a comprehensive atlas of microRNA-target interactions. Nat. Methods 12 (8), 697. doi:10.1038/nmeth.3485
Faltejskova, P., Svoboda, M., Srutova, K., Mlcochova, J., Besse, A., Nekvindova, J., et al. (2012). Identification and functional screening of microRNAs highly deregulated in colorectal cancer. J. Cell. Mol. Med. 16 (11), 2655–2666. doi:10.1111/j.1582-4934.2012.01579.x
Franzosa, E. A., Sirota-Madi, A., Avila-Pacheco, J., Fornelos, N., Haiser, H. J., Reinker, S., et al. (2019). Gut microbiome structure and metabolic activity in inflammatory bowel disease. Nat. Microbiol. 4 (2), 293–305. doi:10.1038/s41564-018-0306-4
Hodi, F. S., Lee, S., McDermott, D. F., Rao, U. N., Butterfield, L. H., Tarhini, A. A., et al. (2014). Ipilimumab plus sargramostim vs ipilimumab alone for treatment of metastatic melanoma: A randomized clinical trial. JAMA 312 (17), 1744–1753. doi:10.1001/jama.2014.13943
Kobori, A., Yagi, Y., Imaeda, H., Ban, H., Bamba, S., Tsujikawa, T., et al. (2010). Interleukin-33 expression is specifically enhanced in inflamed mucosa of ulcerative colitis. J. Gastroenterol. 45 (10), 999–1007. doi:10.1007/s00535-010-0245-1
Kornbluth, A., and Sachar, D. B. (2010). Ulcerative colitis practice guidelines in adults: American college of gastroenterology, practice parameters committee. Am. J. Gastroenterol. 105 (3), 501–523. doi:10.1038/ajg.2009.727
Langfelder, P., and Horvath, S. (2008). Wgcna: an R package for weighted correlation network analysis. BMC Bioinforma. 9, 559. doi:10.1186/1471-2105-9-559
Li, P., Li, Q., Zhang, Y., Sun, S., Liu, S., Lu, Z., et al. (2018). Mir-422a targets mapkk6 and regulates cell growth and apoptosis in colorectal cancer cells. Biomed. Pharmacother. 104, 832–840. doi:10.1016/j.biopha.2018.03.013
Liang, H., Zhao, Q., Zhu, Z., Zhang, C., and Zhang, H. (2021). Long noncoding RNA LINC00958 suppresses apoptosis and radiosensitivity of colorectal cancer through targeting miR-422a. Cancer Cell Int. 21 (1), 477. doi:10.1186/s12935-021-02188-0
Liu, H., Hu, B., Xu, D., and Liew, F. Y. (2003). CD4+CD25+ regulatory T cells cure murine colitis: The role of IL-10, TGF-beta, and CTLA4. J. Immunol. 171 (10), 5012–5017. doi:10.4049/jimmunol.171.10.5012
Liu, H., Li, T., Zhong, S., Yu, M., and Huang, W. (2021). Intestinal epithelial cells related lncRNA and mRNA expression profiles in dextran sulphate sodium-induced colitis. J. Cell. Mol. Med. 25 (2), 1060–1073. doi:10.1111/jcmm.16174
Lushnikova, A., Bohr, J., Wickbom, A., Munch, A., Sjoberg, K., Hultgren, O., et al. (2021). Patients with microscopic colitis have altered levels of inhibitory and stimulatory biomarkers in colon biopsies and sera compared to non-inflamed controls. Front. Med. 8, 727412. doi:10.3389/fmed.2021.727412
Marius, M., Amadou, D., Donatien, A. A., Gilbert, A., William, Y. N., Rauf, K., et al. (2020). In vitro antioxidant, anti-inflammatory, and in vivo anticolitis effects of combretin A and combretin B on dextran sodium sulfate-induced ulcerative colitis in mice. Gastroenterol. Res. Pract. 2020, 4253174. doi:10.1155/2020/4253174
Mercer, T. R., Munro, T., and Mattick, J. S. (2022). The potential of long noncoding RNA therapies. Trends Pharmacol. Sci. 43 (4), 269–280. doi:10.1016/j.tips.2022.01.008
Meydan, C., Bekenstein, U., and Soreq, H. (2018). Molecular regulatory pathways link sepsis with metabolic syndrome: Non-coding RNA elements underlying the sepsis/metabolic cross-talk. Front. Mol. Neurosci. 11, 189. doi:10.3389/fnmol.2018.00189
Miao, Y. R., Zhang, Q., Lei, Q., Luo, M., Xie, G. Y., Wang, H., et al. (2020). ImmuCellAI: A unique method for comprehensive T-cell subsets abundance prediction and its application in cancer immunotherapy. Adv. Sci. 7 (7), 1902880. doi:10.1002/advs.201902880
Ng, S. C., Shi, H. Y., Hamidi, N., Underwood, F. E., Tang, W., Benchimol, E. I., et al. (2017). Worldwide incidence and prevalence of inflammatory bowel disease in the 21st century: A systematic review of population-based studies. Lancet 390 (10114), 2769–2778. doi:10.1016/S0140-6736(17)32448-0
Nie, J., and Zhao, Q. (2020). Lnc-ITSN1-2, derived from RNA sequencing, correlates with increased disease risk, activity and promotes CD4+ T cell activation, proliferation and Th1/Th17 cell differentiation by serving as a ceRNA for IL-23r via sponging miR-125a in inflammatory bowel disease. Front. Immunol. 11, 852. doi:10.3389/fimmu.2020.00852
Otasek, D., Morris, J. H., Boucas, J., Pico, A. R., and Demchak, B. (2019). Cytoscape automation: Empowering workflow-based network analysis. Genome Biol. 20 (1), 185. doi:10.1186/s13059-019-1758-4
Ouyang, T., Cao, Y., Kan, X., Chen, L., Ren, Y., Sun, T., et al. (2021). Treatment-related serious adverse events of immune checkpoint inhibitors in clinical trials: A systematic review. Front. Oncol. 11, 621639. doi:10.3389/fonc.2021.621639
Paraskevopoulou, M. D., Vlachos, I. S., Karagkouni, D., Georgakilas, G., Kanellos, I., Vergoulis, T., et al. (2016). DIANA-LncBase v2: Indexing microRNA targets on non-coding transcripts.Research support, non-U.S. Gov't]. Nucleic Acids Res.Nucleic Acids Res. 44 (D1), D231–D238. doi:10.1093/nar/gkv1270
Peng, L., Chen, Y., Ou, Q., Wang, X., and Tang, N. (2020). LncRNA MIAT correlates with immune infiltrates and drug reactions in hepatocellular carcinoma. Int. Immunopharmacol. 89 (Pt A), 107071. doi:10.1016/j.intimp.2020.107071
Qin, Y. Z., Xie, X. C., Liu, H. Z., Lai, H., Qiu, H., Ge, L. Y., et al. (2015). Screening and preliminary validation of miRNAs with the regulation of hTERT in colorectal cancer. Oncol. Rep. 33 (6), 2728–2736. doi:10.3892/or.2015.3892
Qu, S. L., Chen, L., Wen, X. S., Zuo, J. P., Wang, X. Y., Lu, Z. J., et al. (2021). Suppression of th17 cell differentiation via sphingosine-1-phosphate receptor 2 by cinnamaldehyde can ameliorate ulcerative colitis. J. Artic. Biomed. Pharmacother. 134, 111116. doi:10.1016/j.biopha.2020.111116
Rowshanravan, B., Halliday, N., and Sansom, D. M. (2018). Ctla-4: A moving target in immunotherapy. Blood 131 (1), 58–67. doi:10.1182/blood-2017-06-741033
Salmena, L., Poliseno, L., Tay, Y., Kats, L., and Pandolfi, P. P. (2011). A cerna hypothesis: The rosetta stone of a hidden rna language? Cell 146 (3), 353–358. doi:10.1016/j.cell.2011.07.014
Sarvestani, S. K., Signs, S., Hu, B., Yeu, Y., Feng, H., Ni, Y., et al. (2021). Induced organoids derived from patients with ulcerative colitis recapitulate colitic reactivity. Nat. Commun. 12 (1), 262. doi:10.1038/s41467-020-20351-5
Shah, S. C., and Itzkowitz, S. H. (2022). Colorectal cancer in inflammatory bowel disease: Mechanisms and management. Gastroenterology 162 (3), 715–730. e3. doi:10.1053/j.gastro.2021.10.035
Shen, Y., Dong, L. F., Zhou, R. M., Yao, J., Song, Y. C., Yang, H., et al. (2016). Role of long non-coding RNA MIAT in proliferation, apoptosis and migration of lens epithelial cells: A clinical and in vitro study. J. Cell. Mol. Med. 20 (3), 537–548. doi:10.1111/jcmm.12755
Song, W., Qiu, J., Yin, L., Hong, X., Dai, W., Tang, D., et al. (2021). Integrated analysis of competing endogenous RNA networks in peripheral blood mononuclear cells of systemic lupus erythematosus. J. Transl. Med. 19 (1), 362. doi:10.1186/s12967-021-03033-8
Sun, J., Zhang, H., Wang, C., Yang, M., Chang, S., Geng, Y., et al. (2017). Regulating the balance of th17/treg via electroacupuncture and moxibustion: An ulcerative colitis mice model based study. Evid. Based Complement. Altern. Med. 2017, 7296353. doi:10.1155/2017/7296353
Sun, X., Guo, C., Zhao, F., Zhu, J., Xu, Y., Liu, Z. Q., et al. (2019). Vasoactive intestinal peptide stabilizes intestinal immune homeostasis through maintaining interleukin-10 expression in regulatory B cells. Theranostics 9 (10), 2800–2811. doi:10.7150/thno.34414
Szklarczyk, D., Gable, A. L., Nastou, K. C., Lyon, D., Kirsch, R., Pyysalo, S., et al. (2021). Correction to 'the STRING database in 2021: Customizable protein-protein networks, and functional characterization of user-uploaded gene/measurement sets'. Nucleic Acids Res. 49 (18), 10800. doi:10.1093/nar/gkab835
Thomson, D. W., and Dinger, M. E. (2016). Endogenous microRNA sponges: Evidence and controversy. Nat. Rev. Genet. 17 (5), 272–283. doi:10.1038/nrg.2016.20
Ungaro, R., Mehandru, S., Allen, P. B., Peyrin-Biroulet, L., and Colombel, J. F. (2017). Ulcerative colitis. Lancet 389 (10080), 1756–1770. doi:10.1016/S0140-6736(16)32126-2
Wang, C. J., Heuts, F., Ovcinnikovs, V., Wardzinski, L., Bowers, C., Schmidt, E. M., et al. (2015). CTLA-4 controls follicular helper T-cell differentiation by regulating the strength of CD28 engagement. Proc. Natl. Acad. Sci. U. S. A. 112 (2), 524–529. doi:10.1073/pnas.1414576112
Wang, H., Ding, X. G., Yang, J. J., Li, S. W., Zheng, H., Gu, C. H., et al. (2018). LncRNA MIAT facilitated BM-MSCs differentiation into endothelial cells and restored erectile dysfunction via targeting miR-200a in a rat model of erectile dysfunction. Eur. J. Cell Biol. 97 (3), 180–189. doi:10.1016/j.ejcb.2018.02.001
Wang, Y., Wang, N., Cui, L., Li, Y., Cao, Z., Wu, X., et al. (2021). Long non-coding rna meg3 alleviated ulcerative colitis through upregulating mir-98-5p-sponged il-10. Inflammation 44 (3), 1049–1059. doi:10.1007/s10753-020-01400-z
Wang, Z., Chen, J., Chen, Z., Xie, L., and Wang, W. (2021). Clinical effects of ursodeoxycholic acid on patients with ulcerative colitis may improve via the regulation of IL-23-IL-17 axis and the changes of the proportion of intestinal microflora. Saudi J. Gastroenterol. 27 (3), 149–157. doi:10.4103/sjg.SJG_462_20
Wei, W. T., Nian, X. X., Wang, S. Y., Jiao, H. L., Wang, Y. X., Xiao, Z. Y., et al. (2017). miR-422a inhibits cell proliferation in colorectal cancer by targeting AKT1 and MAPK. Cancer Cell Int. 17, 91. doi:10.1186/s12935-017-0461-3
Wu, Q., Liu, Y., Xie, Y., Wei, S., and Liu, Y. (2021). Identification of potential ceRNA network and patterns of immune cell infiltration in systemic sclerosis-associated interstitial lung disease. Front. Cell Dev. Biol. 9, 622021. doi:10.3389/fcell.2021.622021
Yamada, K., Sawada, T., Nakamura, M., Yamamura, T., Maeda, K., Ishikawa, E., et al. (2021). Clinical characteristics of gastrointestinal immune-related adverse events of immune checkpoint inhibitors and their association with survival. World J. Gastroenterol. 27 (41), 7190–7206. doi:10.3748/wjg.v27.i41.7190
Yang, C., Zhang, Y., and Yang, B. (2021). MIAT, a potent CVD-promoting lncRNA. Cell. Mol. Life Sci. 79 (1), 43. doi:10.1007/s00018-021-04046-8
Ye, T., Feng, J., Cui, M., Yang, J., Wan, X., Xie, D., et al. (2021). LncRNA MIAT services as a noninvasive biomarker for diagnosis and correlated with immune infiltrates in breast cancer. Int. J. Womens Health 13, 991–1004. doi:10.2147/IJWH.S312714
Yu, G., Wang, L. G., Han, Y., and He, Q. Y. (2012). clusterProfiler: an R package for comparing biological themes among gene clusters.Research Support, Non-U.S. Gov't]. J. Artic. 16 (5), 284–287. doi:10.1089/omi.2011.0118
Yu, W., Cheng, H., Zhu, B., and Yan, J. (2021). Network pharmacology-based validation of the efficacy of huiyangjiuji decoction in the treatment of experimental colitis. Front. Pharmacol. 12, 666432. doi:10.3389/fphar.2021.666432
Yu, X., Wang, D., Wang, X., Sun, S., Zhang, Y., Wang, S., et al. (2019). CXCL12/CXCR4 promotes inflammation-driven colorectal cancer progression through activation of RhoA signaling by sponging miR-133a-3p. J. Exp. Clin. Cancer Res. 38 (1), 32. doi:10.1186/s13046-018-1014-x
Zhang S, S., Liang, W., Luo, L., Sun, S., and Wang, F. (2020). The role of T cell trafficking in CTLA-4 blockade-induced gut immunopathology. BMC Biol. 18 (1), 29. doi:10.1186/s12915-020-00765-9
Zhang Y, Y., Wang, X., Li, W., Wang, H., Yin, X., Jiang, F., et al. (2020). Inferences of individual differences in response to tripterysium glycosides across patients with Rheumatoid arthritis using a novel ceRNA regulatory axis. Clin. Transl. Med. 10 (6), e185. doi:10.1002/ctm2.185
Keywords: ulcerative colitis, ceRNA network, WGCNA, bioinformatics, qRT-PCR, immune infiltration
Citation: Dong L, Zhang R, Huang Q, Shen Y, Li H, Yu S and Wu Q (2022) Construction, bioinformatics analysis, and validation of competitive endogenous RNA networks in ulcerative colitis. Front. Genet. 13:951243. doi: 10.3389/fgene.2022.951243
Received: 23 May 2022; Accepted: 06 July 2022;
Published: 17 August 2022.
Edited by:
Mandeep Kaur, University of the Witwatersrand, South AfricaReviewed by:
Bo Chen, Shengjing Hospital of China Medical University, ChinaJavier P Gisbert, Princess University Hospital, Spain
Copyright © 2022 Dong, Zhang, Huang, Shen, Li, Yu and Wu. This is an open-access article distributed under the terms of the Creative Commons Attribution License (CC BY). The use, distribution or reproduction in other forums is permitted, provided the original author(s) and the copyright owner(s) are credited and that the original publication in this journal is cited, in accordance with accepted academic practice. No use, distribution or reproduction is permitted which does not comply with these terms.
*Correspondence: Qiaofeng Wu, d3VxaWFvZmVuZ0BjZHV0Y20uZWR1LmNu
†These authors have contributed equally to this work and share the first authorship
‡ORCID: Qiaofeng Wu, orcid.org/0000-0002-8308-2843