- 1Department of Clinical Pathology, Faculty of Medicine, Mansoura University, El-Mansoura, Egypt
- 2Arthritis and Clinical Immunology Research Program, Oklahoma Medical Research Foundation, Oklahoma City, OK, United States
- 3Department of Neurosciences, Howard Hughes Medical Institute, University of California, San Diego, San Diego, CA, United States
- 4Division of Genetics, Lübeck Institute of Experimental Dermatology, University of Lübeck, Lübeck, Germany
- 5Institute of Global Health and Human Ecology, The American University in Cairo, New Cairo, Egypt
- 6Rheumatology and Clinical Immunology Unit, Department of Internal Medicine, Faculty of Medicine, Cairo University, Cairo, Egypt
- 7Department of Internal Medicine, Faculty of Medicine, Beni-Suef University, Beni Suef, Egypt
- 8Department of Internal Medicine, Faculty of Medicine, Mansoura University, El-Mansoura, Egypt
- 9Center for Genomics, Helmy Institute for Medical Sciences, Zewail City of Science and Technology, Giza, Egypt
- 10Department of Internal Medicine, Faculty of Medicine, Tanta University, Tanta, Egypt
- 11Department of Internal Medicine, Faculty of Medicine, Alexandria University, Bab Sharqi, Egypt
- 12Department of Clinical Pathology, Faculty of Medicine, Alexandria University, Bab Sharqi, Egypt
- 13Department of Internal Medicine, Faculty of Medicine, Al-Azhar University, Cairo, Egypt
- 14Department of Rheumatology and Immunology, Faculty of Medicine, Benha University Hospital, Benha, Egypt
- 15Department of Internal Medicine, Faculty of Medicine, South Valley University, Qena, Egypt
- 16Department of Clinical Pathology, Faculty of Medicine, South Valley University, Qena, Egypt
- 17Department of Internal Medicine, Faculty of Medicine, Menoufia University, Al Minufiyah, Egypt
- 18Rheumatology and Clinical Immunology Unit, Department of Internal Medicine, Faculty of Medicine, Ain Shams University, Cairo, Egypt
- 19Department of Internal Medicine, Faculty of Medicine, Assiut University, Asyut, Egypt
- 20Department of Clinical Pathology, Faculty of Medicine, Assiut University, Asyut, Egypt
- 21The Healthy Lifespan Institute, University of Sheffield, Sheffield, United Kingdom
- 22The Institute of Cancer Therapeutics, University of Bradford, Bradford, United Kingdom
Systemic lupus erythematosus (SLE) susceptibility has a strong genetic component. Genome-wide association studies (GWAS) across trans-ancestral populations show both common and distinct genetic variants of susceptibility across European and Asian ancestries, while many other ethnic populations remain underexplored. We conducted the first SLE GWAS on Egyptians–an admixed North African/Middle Eastern population–using 537 patients and 883 controls. To identify novel susceptibility loci and replicate previously known loci, we performed imputation-based association analysis with 6,382,276 SNPs while accounting for individual admixture. We validated the association analysis using adaptive permutation tests (n = 109). We identified a novel genome-wide significant locus near IRS1/miR-5702 (Pcorrected = 1.98 × 10−8) and eight novel suggestive loci (Pcorrected < 1.0 × 10−5). We also replicated (Pperm < 0.01) 97 previously known loci with at least one associated nearby SNP, with ITGAM, DEF6-PPARD and IRF5 the top three replicated loci. SNPs correlated (r2 > 0.8) with lead SNPs from four suggestive loci (ARMC9, DIAPH3, IFLDT1, and ENTPD3) were associated with differential gene expression (3.5 × 10−95 < p < 1.0 × 10−2) across diverse tissues. These loci are involved in cellular proliferation and invasion—pathways prominent in lupus and nephritis. Our study highlights the utility of GWAS in an admixed Egyptian population for delineating new genetic associations and for understanding SLE pathogenesis.
1 Introduction
Systemic lupus erythematosus (SLE) is a chronic, complex, multi-system autoimmune disease with substantial mortality and morbidity. Prevalence, severity, and sub-clinical manifestations vary significantly across ethnically diverse populations—with an outsized burden on individuals with African, Hispanic, and Asian backgrounds compared to Caucasians (Carter et al., 2016; Lewis and Jawad, 2017; Pisetsky et al., 2017). Additionally, SLE shows remarkable gender bias, affecting ∼9–10 times as many women as men.
In addition to epigenetic and environmental contributions, SLE has a very strong genetic component. This is evidenced by high heritability (∼66%), familial clustering (sibling recurrence risk ratio ∼30), twin studies (∼10 times higher concordance rate in monozygotic versus dizygotic twins) (Deapen et al., 1992; Block, 2006; Mak and Tay, 2014; Kuo et al., 2015), and by a growing number of susceptibility loci identified by genome-wide association studies (GWAS) and high-density candidate gene studies [e.g., ImmunoChip (Cortes and Brown, 2011)]. Despite the identification of ∼180 SLE susceptibility loci (Bentham et al., 2015; Sun et al., 2016; Langefeld et al., 2017; Molineros et al., 2017; Julia et al., 2018; Wang et al., 2018; Tangtanatakul et al., 2020; Ha et al., 2022), these account for only ∼30% of genetic heritability (Yin et al., 2020), indicating that many genes and pathways remain incompletely mapped or even undiscovered.
The statistical power and locus resolution of GWAS and candidate-gene studies can be increased in several ways: larger sample size, deeper genotyping of existing samples, improved imputation and statistical fine-mapping, and most importantly, introducing samples with different allele and haplotype usage than existing samples. Critically, most lupus studies—and most genetic studies in general—are built mainly upon individuals with European (and to a lesser extent Asian) ancestries (Ha et al., 2022). However, many alleles occur at low frequencies in these ethnicities, preventing statistically meaningful measurement of their effects. The use of underrepresented ethnicities with different allelic usage and recombination hotspots is the single most effective way to increase association power (Wojcik et al., 2019). In addition to providing sufficient power to resolve additional ancestry-independent signals, such studies also illuminate ancestry-specific signals (Goulielmos et al., 2018)—wherein a locus is risk only in a specific ethno-genetic background. Such studies are particularly important in autoimmune diseases like lupus, which afflict non-white ethnicities at much higher rates than whites.
Lack of diversity in the available human genomes deposited in public databases limits our understanding of the genetic underpinnings of complex traits, hinders precision medicine, and contributes to health disparities (Popejoy and Fullerton, 2016; Ben-Eghan et al., 2020). Africans and Middle Easterners are among the populations the most underrepresented in genetic association studies, despite comprising over 1.5 billion people and coming from the birthplace of humanity. Moreover, Africa is home to more genetic diversity than the rest of the world combined (Tishkoff et al., 2009) and as such constitutes a woefully underutilized pool for genetic discoveries. Meanwhile, the Middle East and North Africa were both the cradle of civilization and the largest crossroads of migration in the ancient world, bringing together Africa, Europe, and Asia as empires rose and fell. A recent population admixture study (Schuenemann et al., 2017) showed that Ancient Egyptians were more closely related to Middle Easterners and Europeans than to Africans, with African ancestry increasing after the fall of the Roman Empire. In SLE, striking racial/ethnic differences exist in incidence, disease course and clinical manifestations; genetic studies will be helpful to delineate these disparities. However, very few genetic association studies on Egyptians have been performed thus far—and most feature only a few SNPs from a handful of candidate genes (Elghzaly et al., 2015). Therefore, a large-scale genome-wide wide association study is required to understand the SLE genetic landscape in Egyptians—here, we perform the first such study. The major findings of our study are: 1) nine newly uncovered SLE susceptibility loci with one genome-wide significant, 2) replication of several previously known SLE loci, and 3) remarkable similarities in minor allele frequencies at risk loci between Egyptians and Europeans.
2 Materials and methods
2.1 Systemic lupus erythematosus patients and controls
The overview and design of the study is shown in Figure 1. Patients were informed of the nature of the study, and only those who gave their consent were included in the study. SLE was diagnosed according to the 1997 update of the Revised American College of Rheumatology (ACR) classification criteria for SLE (Hochberg, 1997) or the “Systemic Lupus International Collaborating Clinic” (SLICC) Criteria for Classification of Systemic Lupus Erythematosus (Petri et al., 2012). Our rheumatologists used both diagnostic criteria, because we had patients of long disease history with established diagnosis based on earlier criteria that preceded the SLICC standard. Patients who were identified as SLE positive after the introduction of the SLICC criteria were diagnosed accordingly and the two subsets, being included in each center’s registries, were recruited for the study as definite cases of SLE. Note that we lack ACR/SLICC information and some demographic data for 26 SLE patients who have left physicians’ care and are no longer available for verification. Patients’ recruitment, clinical examinations, diagnostic laboratory investigations, and data collection were all performed in their corresponding medical centers by expert rheumatology teams and in specialized university laboratories. Data was collected in an ad hoc unified Excel spreadsheet and reviewed by senior rheumatology experts. Exclusion criteria included the presence of overlap features or the presence of other autoimmune diseases except for Sjögren’s syndrome. The control group was selected from the same geographical location as the corresponding center. All participants provided informed consent, and the study was approved by the Institutional Review Boards from Oklahoma Medical Research Foundation (OMRF), Oklahoma City, United States (IRB approval number 14–16), and Mansoura University Ethics committee/IRB (MFM-IRB code number R/16.04.81). The recruiting hospitals/universities included Cairo University, Mansoura University, Tanta University, Alazhar University–females only, Beni-Suef University, Benha University, Menofia University, Alexandria University, Ain Shams University, South Valley University, and Assuit University.
Initially, 537 Egyptian SLE patients and 434 controls were recruited for the GWAS study, and DNA samples were extracted from 5 ml whole blood samples collected in EDTA-containing tubes and stored at −20°C. DNA extraction for all samples was done in Germany, according to manufacturer’s instructions using the Qiamp DNA Blood Mini Kit (QIAGEN, Hilden, Germany). To increase statistical power, 449 additional unaffected Egyptian controls [already genotyped in Germany for another GWAS study (Bejaoui et al., 2019)] were added to the study. These controls were recruited from the blood banks of Cairo and Mansoura Universities and approved by the IRBs of both Universities (as mentioned above).
2.2 Demographics
Gender and age distributions for SLE cases and controls (after quality control, described below) are shown in Supplementary Table S1. Overall, females constituted 90% and 64% of cases and controls, respectively. Average ages of cases and controls are comparable. The distribution of major ACR criteria within SLE patients are shown in Supplementary Table S1. Almost all (99%) SLE patients were positive for anti-nuclear antibodies (ANA).
2.3 Genotyping and quality control
Initial samples (537 SLE patients and 434 controls) were genotyped on the Illumina Multi-Ethnic Genotyping Array (MEGA) at the genotyping core facility of OMRF, Oklahoma City, United States. The 449 additional out-of-study controls were already genotyped on the Illumina Infinium Global Screening Array. The out-of-study controls have no genotyping data on the X-chromosome; thus we decided to focus only on autosomal loci. We hope to obtain this missing genotyping data for use in future studies on X-linked traits. We performed strict quality control (QC) on genotyped SNPs in each dataset separately (cases and two sets of controls) as follows: (a) we excluded SNPs with bad clusters due to poor genotyping calls, (b) we removed SNPs with missing genotype rate ≥ 0.05, and (c) we filtered out SNPs out of Hardy-Weinberg equilibrium (p < 0.0001) and/or minor allele frequency (MAF) < 0.5%. After QC, 295,981 autosomal (chromosomes 1–22) SNPs that overlapped both genotyping arrays remained for further analysis. Of the 537 cases and 883 controls, the GCTA algorithm (Yang et al., 2011) selected 500 cases and 815 controls as being unrelated from one another; the others were excluded. As a final QC step, 42 cases and 46 controls were removed from the cohort due to being outliers from principal components analysis—leaving 458 cases and 769 controls for this study. The inflation factor λ was calculated from the case-control association test on the final, clean cohort (458 cases and 769 controls).
2.4 Statistical power for detecting association
To estimate the ability of our GWAS cohort to support statistically significant associations, we performed a statistical power calculation. The Genetic Association Study Power Calculator server (Johnson and Abecasis, 2017), derived from the CaTS power calculator for two-stage association studies (Skol et al., 2006), was used. We used our final cohort (458 cases, 769 controls) to assess statistical power, while setting the parameter prevalence for SLE to 0.3% (Wang Y. F. et al., 2021).
2.5 Imputation for GWAS samples
We used the Michigan Imputation Server (Das et al., 2016) for imputation on the 22 autosomes. 1,000 Genomes Project data (Phase 3 Integrated Release Version 5 Haplotypes) was used as the reference panel. After imputation, we performed strict QC on post-imputed SNPs as described above (Hardy-Weinberg equilibrium p > 0.0001 in controls and/or MAF ≥ 0.5%). About 6.36 million post-imputed SNPs with high imputation quality (Rsq > 0.7 for MAF ≥ 3%) were used for downstream analysis.
2.6 Admixture-corrected association analysis with imputed data
To quantify individual ancestry proportion in our Egyptian cohort, we complemented our dataset with published samples from the ancient Near East (Lazaridis et al., 2016) (the historical Fertile Crescent and Levant regions, corresponding to modern-day Egypt, Turkey, Iran, and surrounding nations.) The Near East samples include 294 ancient and 2,068 modern individuals spanning a range of nationalities and ethnicities. In both the Egyptian dataset and Near East public dataset, we excluded SNPs with A/T or C/G alleles and identified ∼33,000 common unrelated SNPs (MAF > 5% and linkage disequilibrium, LD r2 < 0.2) for analysis. Principal components analysis was performed with GCTA (Yang et al., 2011), and genetic ancestry composition was estimated with ADMIXTURE (Alexander et al., 2009).
We performed imputation-based association analyses using mach2dat (Li et al., 2009) before and after admixture correction. (A/T and C/G alleles were recovered during imputation.) ADMIXTURE uses a maximum-likelihood approach to determine admixture proportions of individuals by assuming there are K hypothetical underlying populations among them. We selected K = 5 as the best model for the number of ancestral populations and took the first four ancestry proportions as covariates for admixture correction. The inflation factor (λ) was estimated using all admixture-corrected association results from QC-passed SNPs.
We performed multiple-testing correction using permutation (Gao, 2011)—considered the gold standard in GWAS studies. Note that we specifically avoided Bonferroni correction as this assumes independence, which is failed when any SNPs are in linkage disequilibrium—thus producing unacceptably conservative associations (Johnson et al., 2010). Besides, Bonferroni correction suffers several shortcomings, most notably low statistical power for rejecting the null hypothesis (Cohen, 1990, 1994; Perneger, 1998; Nakagawa, 2004) and also performing large amounts of unnecessary testing (Fadista et al., 2016). Critically, permutation analysis controls for low sample-size effects, as all permutations have identical n as the proposal—comparisons are between permutations with identical statistical power a priori to produce an association. Thus, we do not fear spurious associations despite our relatively small sample sizes.
2.7 Replication of previously known variants and loci
To replicate previously reported SLE susceptibility loci, we followed a recent study (Ha et al., 2022) that collated 179 statistically-independent non-human leukocyte antigen (HLA) loci as underlying SLE susceptibility. At each risk locus, we defined a boundary as ± 150 kb around the nominated SNP and collated the full set of SNPs in these intervals. Then, we compared this (enlarged) SNP set with the admixture-corrected association results. Using an ancestry-corrected permutation-based analysis, we set the threshold for replication at Pperm < 0.01—stricter than the more commonly used 0.05 threshold, to better exclude false positive associations.
2.8 SNP functional annotation
Functional interpretation of the associated SNP under any GWAS peak is of critical importance in follow-up analysis. Like most other GWAS studies, many of our identified SNPs concentrate in non-coding DNA, e.g., transcriptional regulatory regions (promoters, enhancers) and non-coding RNA. Therefore, analyzing tissue-specific effects of expression quantitative trait loci (eQTLs) is a promising approach. We used Qtlizer (Munz et al., 2020) for the top SNPs in the novel loci to obtain their eQTLs from several public databases, including GTEx v7 (Consortium, 2013), GEUVADIS (Lappalainen et al., 2013), GRASP (Leslie et al., 2014), Haploreg (Ward and Kellis, 2012), SCAN (Gamazon et al., 2009), seeQTL (Xia et al., 2011), Blood eQTL Browser (Westra et al., 2013), pGWAS (Suhre et al., 2017), ExSNP (Yu et al., 2016), and BRAINEAC (Ramasamy et al., 2014). Qtlizer allowed exploration of QTL data for a given list of variants (indels and SNPs) in a fast and efficient manner by integrating many QTL datasets.
2.9 Annotation of long non-coding RNA targets and microRNAs
Target sites of lncRNAs were predicted using the LncRRIsearch server (http://rtools.cbrc.jp/LncRRIsearch) (Fukunaga et al., 2019), built upon RIblast (Fukunaga and Hamada, 2017), which computationally identifies lncRNA-mRNA interactions through a seed-and-extension approach. For miRNAs, we identified similar sequences in the human genome (GRCh37/hg19) with BLAT on the UCSC Genome Browser (GRCh37/hg19).
3 Results
3.1 Study population and statistical power
After rigorous QC, 458 cases and 769 controls were identified by GCTA (Yang et al., 2011) as unrelated samples and were used for association (Figure 1, Section 2). Due to the small sample size, our study had moderate power (68%) for detection of significant (p < 1 × 10−5) variants with odds ratio (OR) < 1.5 (Supplementary Figure S1). However, for common (minor allele frequency, MAF ≥ 10%) SNPs with large effect (OR ≥ 1.6), our study had high (up to 90%) power. The inflation factor (λ) between cases and controls was 1.06; the QQ plot is shown in Supplementary Figure S2.
3.2 Genome-wide association of SLE
To discover novel SLE susceptibility loci and to replicate previously known SLE risk loci in our Egyptian cohort, we performed a genome-wide imputation-based association study with 6,382,276 SNPs while adjusting for individual admixture (see Section 2). The Manhattan plot showing -log10 p values for SNP associations for our GWAS is shown in Figure 2.
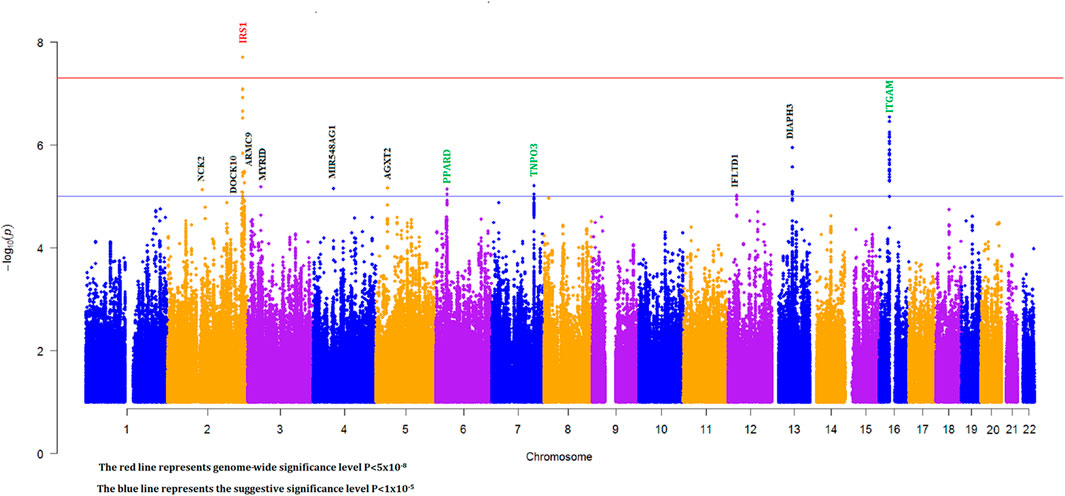
FIGURE 2. Manhattan plot for imputation-based association with individual ancestry correction (green, black, and red gene names represent known, novel suggestive, and novel genome-wide significant loci, respectively).
3.2.1 Discovery of novel SLE loci
We identified 28 SNPs in nine novel loci with Pcorrected < 1 × 10−5 (Table 1; Supplementary Table S2; Figure 3). The most significant signal, which passed genome-wise significance (rs5839171, Pcorrected = 1.98 × 10−8, Pperm = 3.30 × 10−8), is downstream of the Insulin receptor substrate 1 gene (IRS1) and miR-5702, a microRNA involved in cellular proliferation and cancer risk (Li K. et al., 2019). IRS1 is a signaling adapter protein linking activity of insulin and insulin-like growth factor receptors to intracellular signaling cascades, most notably the PI3K/Akt and Erk MAP kinase pathways (Leslie et al., 2014). IRS1 has been flagged as a risk locus for insulin resistance (Rung et al., 2009) and type II diabetes (Rung et al., 2009; Voight et al., 2010), and also several cancers (Slattery et al., 2004). The best characterized target of miR-5702 is Zinc finger E-box-binding homeobox 1 (ZEB1) (Zhang et al., 2018), a transcription factor critically involved in T-lymphocyte differentiation and interleukin-2 signaling (Guan et al., 2018). At this locus, another nearby (1.7 kb) SNP, rs10804331 (Pcorrected = 8.5 × 10−8), is a significant eQTL for IRS1 in CD4+ naïve T-cells (p = 0.002) and CD16+ neutrophils (p = 0.01) (Supplementary Table S3). This SNP is also a significant mQTL for methylated CpG cg07514207 in the IRS1 promoter (Chen et al., 2016), potentially modulating transcription.
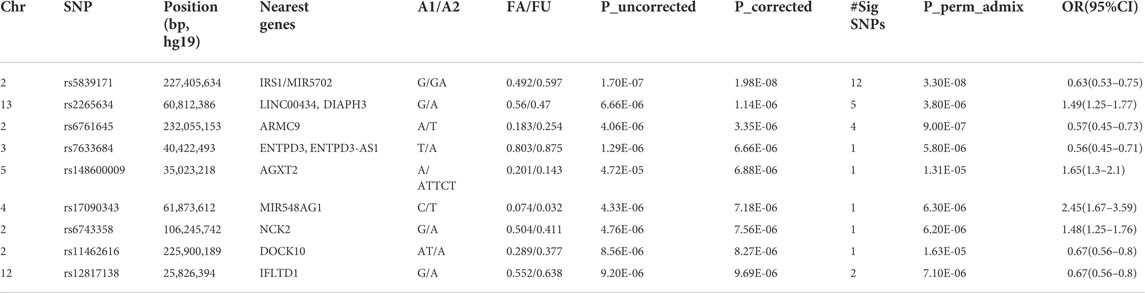
TABLE 1. Association (genome-wide significant and suggestive) signals at the lead SNPs with p < 1.0x10−5.
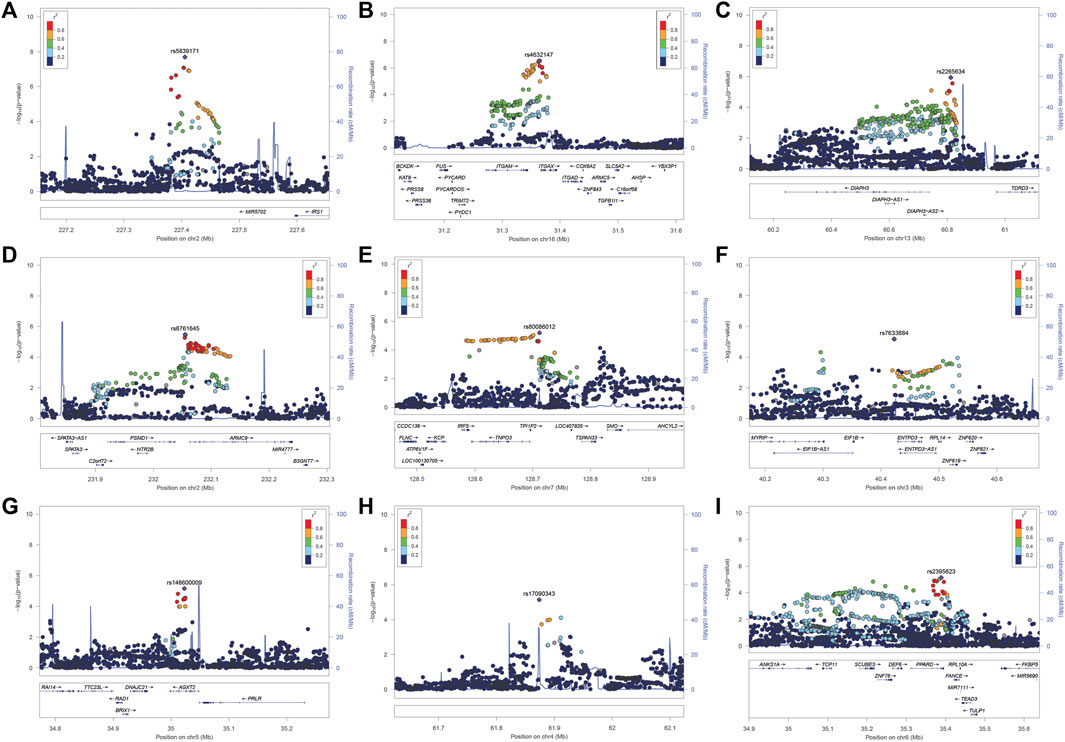
FIGURE 3. (A–I). LocusZoom plot near the IRS1/miR-5702 locus, which reached genome-wide significance, and 8 suggestive loci.
The second signal (rs2265634; Pcorrected = 1.14 × 10−6) is between Diaphanous homolog 1 (DIAPH3), which modulates mTOR signaling and cellular proliferation (Wan et al., 2021), and Tudor domain-containing protein 3 (TDRD3), a chromatin modulator also involved in proliferation (Morettin et al., 2017); it is also close to long non-coding RNA LINC00434. LINC00434 appears to target several genes involved in cancer and autoimmunity (Supplementary Table S4). This SNP is a significant (p = 4.78 × 10−8) expression quantitative trait locus (eQTL) for DIAPH3, which was a nominally significant (p = 7.23 × 10−3) SLE risk locus in a previous GWAS study (Lee et al., 2014).
The third signal (rs6761645; Pcorrected = 3.35 × 10−6) is upstream of Armadillo repeat containing 9 [ARMC9, encoding KU-MEL-1 (Kiniwa et al., 2001)], a ciliary basal body protein, mutations of which underlie the systemic inflammatory disorder Vogt-Koyanagi-Harada disease (VKH) (Ohno et al., 2019) and the neurodevelopmental disorder Joubert syndrome (Van De Weghe et al., 2017). KU-MEL-1, over-expressed in melanocytes, appears to be a primary autoantigen in VKH (Otani et al., 2006) and is also associated with vitiligo and autoimmune uveitis. These top three hits were all located in active chromatin, with rs6761645, in particular, overlapping promoter signals in 17 tissues and enhancers in 12 tissues (Ward and Kellis, 2012); having 14 significant eQTL hits (Supplementary Table S3); and being associated with transcription factor binding sites for Hypoxia-inducible factor 1 (HIF1), a critical regulator of inflammation and the DNA damage response (Dehne and Brüne, 2009; Ramachandran et al., 2021). rs6761645 is an eQTL for PSMD1 (26S proteasome non-ATPase regulatory subunit 1) (Supplementary Table S3), another proliferation marker (Okumura et al., 2017).
The five other novel signals localized to ENTPD3/ENTPD3-AS1 (a tumor suppressor (Li M. et al., 2019) that strongly signals through HIF1 (Wang J. et al., 2021) and its associated antisense RNA), NCK2 [a critical immune adaptor protein linking B-cell receptor activation to PI3K signaling (Cannons et al., 2013)], IFLTD1 [a ciliary organization protein and cancer risk gene (Wang et al., 2005), also known as LMNTD1 and PAS1C1], AGXT2 [a risk locus of premature myocardial infarct (Yuanfeng et al., 2016)], DOCK10 [critical in B-cell activation and proliferation (Yelo et al., 2008)], and the microRNA MIR548AG1.
Given the prominent role played by microRNAs in cancer (Calin and Croce, 2006) and autoimmunity (Xiao and Rajewsky, 2009), and the fact that miR-548ag1 had the largest effect size of our novel loci [odds ratio = 2.45 (95% confidence interval 1.67–3.59)], we closely examined the apparent involvement of this miRNA in SLE. The miR-548 family was first discovered in colorectal expression arrays (Cummins et al., 2006) and was expanded through bioinformatic searches for miRNAs overlapping repetitive elements (Piriyapongsa and Jordan, 2007). Both the microRNA family itself and putative target sites derive from the Made1 repetitive element; these sequences form very stable hairpin structures of ∼37 bp in length. miR-548ag1 was discovered from a B-cell tumor line (Jima et al., 2010), suggesting immune involvement. Many other family members are implicated in cancer (Shi et al., 2015; Ke et al., 2016) and autoimmunity (Yu et al., 2017; Cakmak Genc et al., 2018; Li et al., 2018). To further elucidate the biological roles of miR-548ag1, we searched the human genome for miR-548ag1-like sequences (see Section 2), restricting ourselves to loci exhibiting primate-specific insertions [consistent with other verified miR-548 family members (Piriyapongsa and Jordan, 2007)]. We found closely related sequences in UTRs and introns of ten genes—usually overlapping enhancers and/or transcription factor binding sites (Supplementary Table S5). Strikingly, all ten genes have clear immune involvement (Supplementary Table S5). Five of the ten hits are expressed sequence tags (ESTs) of ∼90 bp–100 bp and almost certainly represent previously unannotated members of the miR-548 family. Of the remaining five, it is not immediately clear which are microRNAs themselves and which are potential miRNA target sites. Together, these results emphasize the immune system relevance of our SLE risk locus miR-548ag1 and related miR-548 family members.
3.2.2 Replication of known SLE loci
We compared our association results with 179 SLE known loci (see Section 2) and found 97 known loci replicated with at least one significantly (Pperm < 0.01) associated SNP. Whenever available, we showed LD with the top published variant to identify independent associations at known loci (Table 2). The most significant SNPs at each locus are listed in Table 2. Among them, prominent (Pperm < 5.0 × 10−5) signals include ITGAM-ITGAX (rs71391210; Pperm = 2.0 × 10−7), IRF5 (rs80086012; Pperm = 3.5 × 10−6), DEF6-PPARD (rs2395623; Pperm = 4.70 × 10−6), XKR6 (rs2409660; Pperm = 4.55 × 10−5), IRF1 (rs10586626; Pperm = 5.43 × 10−5), and TYK2 (rs77389625; Pperm = 1.91 × 10−4). All replicated (Pperm < 0.01) SNPs and their nearest genes are shown in Table 2.
3.2.3 Minor allele frequency of associated SNPs and ethnicity
Allelic abundance—most often reported as minor allele frequency (MAF)—is an important measure both to estimate the effect of given SNPs on specific populations and more broadly to compare ancestry and population stratification between diverse populations. Using the 97 total replicated lead SNPs, we compared MAFs in our Egyptian cohort with those of Africans, Europeans, and Asians. We found a remarkable similarity between the MAFs of Egyptians and Europeans (R2 = 93%)—i.e., the MAF variance in Egyptians was well explained by the MAF variance in Europeans. Conversely, correlation with Africans (R2 = 51%) and Asians (R2 = 59%) was markedly lower (Figure 4). Although surprising prima facie, these results were supported by our admixture analysis (Supplementary Figure S3).
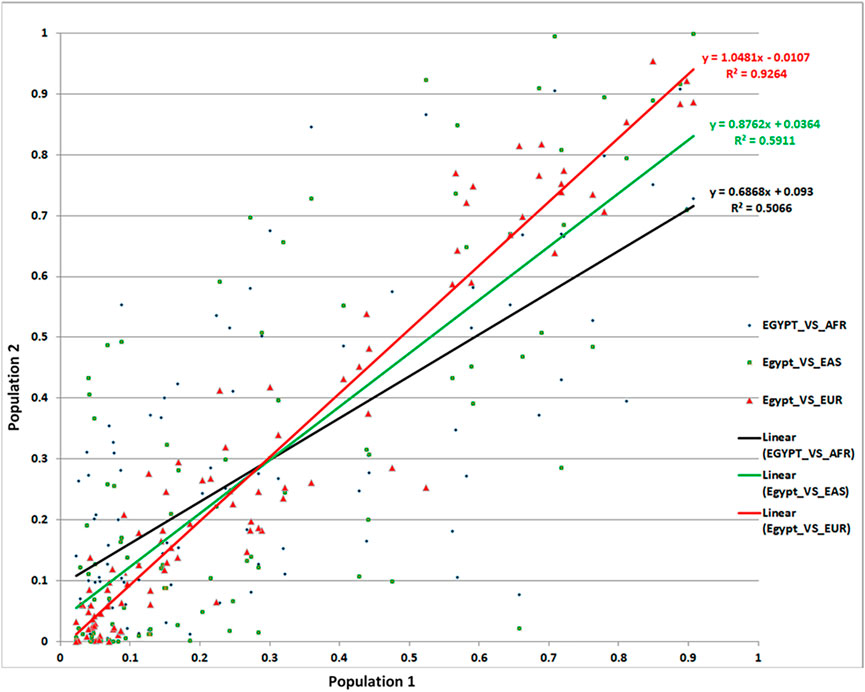
FIGURE 4. Comparison of MAFs for the associated SNPs from our Egyptian controls and 1,000 Genomes data.
3.2.4 Functional SNPs from some notable loci
Having identified several new SLE-associated loci, most of which have known roles in both immune homeostasis and other autoimmune disorders, we sought to better establish possible mechanisms for both the loci and the associated SNPs. For our nine novel loci, we first looked for significant (P < 1 × 10−5) eQTLs for both the top SNPs and the larger set of SNPs in linkage disequilibrium (r2 > 0.8) with them. We found several significant (3.5 × 10−95 < P < 1 × 10−2) eQTLs from our new loci, particularly from ARMC9 (43 SNPs; 25 tissues), IFLTD1 (5 SNPs; 4 tissues), DIAPH3 (6 SNPs; 1 tissue), and ENTPD3 (1 SNP; 10 tissues) (Figure 5A). IFLTD1 is enriched in the brain, sex organs, and digestive tract (https://www.proteinatlas.org), and most eQTL signals came from these tissues. ARMC9 is very strongly expressed in natural killer (NK) cells (https://www.proteinatlas.org) and melanocytes (Otani et al., 2006); interestingly, the strongest eQTL signals arose from vascular endothelium and thyroid. Known loci also contained many eQTL SNPs; for example, IRF1 (58 SNPs; 23 tissues) showed particularly strong eQTL signals. IRF1 is quite immune cell-specific, and accordingly, the strongest eQTL signals came from blood (Figure 5B).
4 Discussion
Our study represents the first lupus GWAS in North Africans, an underrepresented population in human genetic studies. Previous studies indicated that Egyptians are highly admixed, with substantial ancestry arising from the Middle East, Europe, and Africa—with the extent of European and Caucasus hunter-gatherer (Lazaridis et al., 2016) contribution being higher, and the African contribution being lower, than might be expected from geography. Greater European/Middle Eastern than African ancestry is also supported by whole-genome sequencing (ElHefnawi et al., 2021). Importantly, modern Egyptians are quite divergent from ancient populations. The inclusion of more diverse participants in genomics studies has been shown to significantly increase fine-mapping resolution (Zaitlen et al., 2010; Asimit et al., 2016). Further studies in Egyptians (and other North African and Middle Eastern populations) could potentially reveal more genetic associations, with implications for understanding, diagnosing, and treating disease for Egyptians and all humans.
In this study, we identified nine novel loci; one is genome-wide significant for lupus susceptibility and eight are suggestive. Among 179 known non-HLA SLE susceptibility loci, 97 were successfully replicated—indicating that most lupus susceptibility in Egyptians is ancestry-independent. We also found several loci not previously reported in any populations. Intriguingly, the novel loci were substantially enriched in proteins involved in cellular proliferation and cancer risk. Specifically, Insulin receptor substrate 1 (IRS1), the microRNA miR-5702, Diaphanous homolog 1 (DIAPH3), Armadillo repeat containing 9 (ARMC9, a.k.a. KU-MEL-1), Intermediate filament tail domain containing 1 (IFLTD1, a.k.a. LMNTD1), immune adaptor protein NCK2 (Labelle-Côté et al., 2011), and Ectonucleoside triphosphate diphosphohydrolase 3 (ENTPD3) (Wang J. et al., 2021) all have well-documented roles in cellular proliferation and tumor invasion. Proliferation factors play a prominent role in lupus, particularly in invasion of specific organs in sub-clinical phenotypes, most notably lupus nephritis (Balomenos et al., 2000; Treamtrakanpon et al., 2012).
The novel and replicated loci in this study share other features in addition to association with cellular proliferation—for instance, regulation of and by microRNAs, another hallmark of SLE (Dai et al., 2007; Carlsen et al., 2013). Notably, both IRS1 and ARMC9 (along with LRIG2, PSPH, and SKP2) are direct targets down-regulated by miRNA-150 (Zhang et al., 2021). miRNA-150 is a critical immune regulator, for instance driving expression of the inflammatory receptor TREM-1 (Triggering receptor expressed on myeloid cells 1) in dendritic cells and contributing to lupus-like symptoms in mice (Gao et al., 2017). More recently, miRNA-150 (along with miRNA-148b) has been proposed as a specific biomarker for lupus nephritis (Alkhateeb and Altamemi, 2021). Two of our novel risk loci even interact at the protein level: NCK2 directly binds to IRS1 to create an intracellular signal transduction mechanism for signaling through insulin and insulin-like growth factor receptors (Tu et al., 1998; Tu et al., 2001). We performed a sequence search with our novel risk miRNA miR548AG1 and discovered ten closely related sequences, all of them in introns or UTRs of immune-critical genes (Supplementary Table S5) –many overlapping enhancers and/or transcription factor binding sites. Furthermore, five of these ten hits are ESTs of ∼90 bp–100 bp and almost certainly constitute previously unannotated members of the miR-548 family. The clear immune association of all ten closely related sequences further confirms the relevance of our SLE risk locus miR548AG1.
Our study very carefully controlled for admixture in estimating effect size and significance level. This was a critical step, as multiple loci became more significant or less significant once controlled for admixture (Tables 1, 2; Supplementary Table S1). The most substantive change was at the novel locus IRS1/miR-5702, where the top SNP rs5839171 achieved genome-wide significance after admixture correction (Puncorrected = 1.70 × 10−7, Pcorrected = 1.98 × 10−8). This remains significant (Ppermuted = 3.3 × 10−8) after 109 permutations. This emphasizes the utility of admixed populations, like Egyptians, in studying novel gene discovery.
To assess the replication of previously known loci [Table 1 from (Ha et al., 2022)], we implemented a very large (107–109 combinations) ancestry-adjusted permutation analysis on our data. Critically, permutation analysis controls for low sample-size effects, as all permutations have identical n as the proposal—comparisons are between permutations with identical statistical power a priori to produce an association. Using the ancestry-corrected permutation-based analysis, we set threshold for replication at p < 0.01. Here we further explain our reasoning behind the robustness of our permutation analysis and some of its findings.
First, a recent large-scale (n > 208,000) SLE GWAS meta-analysis on East-Asian populations identified rs956237 (GWAS lead variant; intron of LEF1, Lymphoid enhancer-binding factor 1—a leukocyte transcription factor) at 4q25 as a novel susceptibility locus (Yin et al., 2020; p < 4.0 × 10−11). Reassuringly, a companion transcriptome-wide association study (TWAS) using data from Asian samples also strongly indicated LEF1 as the primary gene underlying 4q25 association (Yin et al., 2020; p < 1.3 × 10−10). However, our primary reference (Table 1 from Ha et al., 2022) nominated rs58107865 instead of rs956237 as lead SNP. In our study, we found that rs58107865 does not exist in the African/Egyptian population. Instead, we discovered an association with another intronic LEF1 SNP (rs10516550, p = 0.008), common in African/European/Hispanic populations (but not Asian). Of course, this SNP needs to be independently replicated in a population with similar ethnic background. But we believe that it’s appropriate to cautiously interpret this in the replication category.
Second, NCF2 is a very well-known SLE locus, where multiple studies (Cunninghame Graham et al., 2011; Yin et al., 2020) including ours (Kim-Howard et al., 2014) have reported non-coding and at least 3 independent ethnicity-specific coding variants within NCF2 passing genome-wide significance in Asian/Hispanic (rs13306575), European/African-American (rs17849502) and African-American (rs35937854) populations. This locus was represented by rs13306575 in our reference data (Table 1 from Ha et al., 2022). In our data, we found evidence of association (ancestry-adjusted permutation p = 0.004) with nearby (< 150 bp) rs17849502. Thus, we have found essentially the same result as the prior studies; however, this real association falls below the strict threshold set by the Bonferroni correction (p = 0.05/179 = 0.00027). Our non-parametric permutation analysis, however, detects this locus as a significant association, which we believe is supported by the preponderance of evidence.
Although the sample sizes in the current study are not overly large, we had the power to attain multiple noteworthy discoveries for several reasons: 1) The highly admixed Egyptian population gave us the power to discover nine novel loci, one of which achieved genome-wide significance. 2) Our data attained a remarkable replication rate (97 out of 179 loci; 54%)—increasing confidence in both known and novel loci, and supporting the notion that despite different genetic backgrounds, underlying loci and risk alleles are broadly conserved across ethnicities (Marigorta and Navarro, 2013). Over 80% of our replicated loci arose from GWASs on Europeans, indicating broad concordance of genetic risk. However, it is important to note that the studies included in Ha et al. (2022) were performed on populations with large sample sizes and statistical power. Egyptians have a stronger contribution from Middle Eastern ancestries than European (Wohlers et al., 2020), and their population history and admixture proportions are different. Moreover, our sample size was relatively small. Future studies will boost sample sizes and further address the other aspects. 3) We found several common pathways shared by the known and new loci, increasing the robustness of the observations, and laying out the foundation for further experimental studies to establish a clear mechanistic basis for these loci contributing to SLE risk and progression.
However, we also acknowledge that lack of an independent replication, especially for the novel associations, is a limitation of this study. Therefore, in addition to strengthening the observations and establishing mechanisms, further studies with larger sample sizes will clearly delineate the ancestry-independent and ancestry-specific components of SLE association.
Institutional Review Board Statement: The study was conducted in accordance with the Declaration of Helsinki and approved by the Institutional Review Board of the Oklahoma Medical Research Foundation (IRB approval number 14–16), and Mansoura University (Egypt) Ethics committee/IRB: MFM-IRB code number R/16.04.81.
Informed Consent Statement: Informed consent was obtained from all subjects involved in the study.
Data availability statement
The original contributions presented in the study are included in the article/Supplementary Materials, further inquiries can be directed to the corresponding authors.
Ethics statement
The studies involving human participants were reviewed and approved by Institutional Review Board of the Oklahoma Medical Research Foundation And Mansoura University Ethics Committee/Institutional Review Board. The patients/participants provided their written informed consent to participate in this study.
Author contributions
Conceptualization, GR and SN; Data curation, AAE, CS, LL and MTH; Formal analysis, CS and SN; Funding acquisition, SI and SN; Investigation, ZT, MA, WA, IE-S, ME, AnA, SH, NS, AH and ENA; Methodology, CS, LL and SN; Resources, NMK, MB, MTH, MAH, MNS, EE, ZT, MA, WA, IE-S, ME, AnA, SH, NS, AS, AmE, AmH, KR, HE-T, AY, FE, AAH, AsA, EmA, DF, SE-B, ME, ENA, and SN; Software, CS, LL, NK, MB and SN; Supervision, NK, SI, GR and SN; Validation, AAE, CS, MAH, MNS and SI; Visualization, AAE, SI, GR and SN; Writing–review and editing, AAE, LL, CS, SE-K, GR and SN. All authors have read and agreed to the published version of the manuscript.
Funding
This research was funded by the University of Lübeck and by the National Institutes of Health, grant numbers MD007909 and AR060366.
Acknowledgments
We thank all patients with SLE and healthy controls who participated in this study. We also thank the research assistants, coordinators, and physicians who helped in the recruitment of subjects for this project.
Conflict of interest
The authors declare that the research was conducted in the absence of any commercial or financial relationships that could be construed as a potential conflict of interest.
Publisher’s note
All claims expressed in this article are solely those of the authors and do not necessarily represent those of their affiliated organizations, or those of the publisher, the editors and the reviewers. Any product that may be evaluated in this article, or claim that may be made by its manufacturer, is not guaranteed or endorsed by the publisher.
Supplementary material
The Supplementary Material for this article can be found online at: https://www.frontiersin.org/articles/10.3389/fgene.2022.948505/full#supplementary-material.
References
Alexander, D. H., Novembre, J., and Lange, K. (2009). Fast model-based estimation of ancestry in unrelated individuals. Genome Res. 19 (9), 1655–1664. doi:10.1101/gr.094052.109
Alkhateeb, D. M., and Altamemi, A. (2021). Circulating microrna (148b and 150) as potential biomarker in iga nephropathy and lupus nephritis. Ann. Romanian Soc. Cell Biol. 25 (6), 6294–6309.
Asimit, J. L., Hatzikotoulas, K., McCarthy, M., Morris, A. P., and Zeggini, E. (2016). Trans-ethnic study design approaches for fine-mapping. Eur. J. Hum. Genet. 24 (9), 1330–1336. doi:10.1038/ejhg.2016.1
Balomenos, D., Martín-Caballero, J., García, M. I., Prieto, I., Flores, J. M., Serrano, M., et al. (2000). The cell cycle inhibitor p21 controls T-cell proliferation and sex-linked lupus development. Nat. Med. 6 (2), 171–176. doi:10.1038/72272
Bejaoui, Y., Witte, M., Abdelhady, M., Eldarouti, M., Abdallah, N. M. A., Elghzaly, A. A., et al. (2019). Genome-wide association study of psoriasis in an Egyptian population. Exp. Dermatol. 28 (5), 623–627. doi:10.1111/exd.13926
Ben-Eghan, C., Sun, R., Hleap, J. S., Diaz-Papkovich, A., Munter, H. M., Grant, A. V., et al. (2020). Don't ignore genetic data from minority populations. Nature 585 (7824), 184–186. doi:10.1038/d41586-020-02547-3
Bentham, J., Morris, D. L., Cunninghame Graham, D. S., Pinder, C. L., Tombleson, P., Behrens, T. W., et al. (2015). Genetic association analyses implicate aberrant regulation of innate and adaptive immunity genes in the pathogenesis of systemic lupus erythematosus. Nat. Genet. 47 (12), 1457–1464. doi:10.1038/ng.3434
Cakmak Genc, G., Dursun, A., Karakas Celik, S., Calik, M., Kokturk, F., and Piskin, I. E. (2018). IL28B, IL29 and micro-RNA 548 in subacute sclerosing panencephalitis as a rare disease. Gene 678, 73–78. doi:10.1016/j.gene.2018.07.062
Calin, G. A., and Croce, C. M. (2006). MicroRNA-cancer connection: The beginning of a new tale. Cancer Res. 66 (15), 7390–7394. doi:10.1158/0008-5472.Can-06-0800
Cannons, J. L., Zhao, F., and Schwartzberg, P. L. (2013). Ncking BCR-mediated PI3K activation. EMBO Rep. 14 (10), 852–853. doi:10.1038/embor.2013.133
Carlsen, A. L., Schetter, A. J., Nielsen, C. T., Lood, C., Knudsen, S., Voss, A., et al. (2013). Circulating MicroRNA expression profiles associated with systemic lupus erythematosus. Arthritis Rheum. 65 (5), 1324–1334. doi:10.1002/art.37890
Carter, E. E., Barr, S. G., and Clarke, A. E. (2016). The global burden of SLE: Prevalence, health disparities and socioeconomic impact. Nat. Rev. Rheumatol. 12 (10), 605–620. doi:10.1038/nrrheum.2016.137
Chen, L., Ge, B., Casale, F. P., Vasquez, L., Kwan, T., Garrido-Martin, D., et al. (2016). Genetic drivers of epigenetic and transcriptional variation in human immune cells. Cell 167 (5), 1398–1414. doi:10.1016/j.cell.2016.10.026
Cohen, J. (1994). The Earth is round (p < .05). Am. Psychol. 49, 997–1003. doi:10.1037/0003-066X.49.12.997
Cohen, J. (1990). Things I have learned (so far). Am. Psychol. 45, 1304–1312. doi:10.1037/0003-066X.45.12.1304
Consortium, G. T. (2013). The genotype-tissue expression (GTEx) project. Nat. Genet. 45 (6), 580–585. doi:10.1038/ng.2653
Cortes, A., and Brown, M. A. (2011). Promise and pitfalls of the immunochip. Arthritis Res. Ther. 13 (1), 101. doi:10.1186/ar3204
Cummins, J. M., He, Y., Leary, R. J., Pagliarini, R., Diaz, L. A., Sjoblom, T., et al. (2006). The colorectal microRNAome. Proc. Natl. Acad. Sci. U. S. A. 103 (10), 3687–3692. doi:10.1073/pnas.0511155103
Cunninghame Graham, D. S., Morris, D. L., Bhangale, T. R., Criswell, L. A., Syvanen, A. C., Ronnblom, L., et al. (2011). Association of NCF2, IKZF1, IRF8, IFIH1, and TYK2 with systemic lupus erythematosus. PLoS Genet. 7 (10), e1002341. doi:10.1371/journal.pgen.1002341
Dai, Y., Huang, Y. S., Tang, M., Lv, T. Y., Hu, C. X., Tan, Y. H., et al. (2007). Microarray analysis of microRNA expression in peripheral blood cells of systemic lupus erythematosus patients. Lupus 16 (12), 939–946. doi:10.1177/0961203307084158
Das, S., Forer, L., Schönherr, S., Sidore, C., Locke, A. E., Kwong, A., et al. (2016). Next-generation genotype imputation service and methods. Nat. Genet. 48 (10), 1284–1287. doi:10.1038/ng.3656
Deapen, D., Escalante, A., Weinrib, L., Horwitz, D., Bachman, B., Roy-Burman, P., et al. (1992). A revised estimate of twin concordance in systemic lupus erythematosus. Arthritis Rheum. 35 (3), 311–318. doi:10.1002/art.1780350310
Dehne, N., and Brüne, B. (2009). HIF-1 in the inflammatory microenvironment. Exp. Cell Res. 315 (11), 1791–1797. doi:10.1016/j.yexcr.2009.03.019
Elghzaly, A. A., Metwally, S. S., El-Chennawi, F. A., Elgayaar, M. A., Mosaad, Y. M., El-Toraby, E. E., et al. (2015). IRF5, PTPN22, CD28, IL2RA, KIF5A, BLK and TNFAIP3 genes polymorphisms and lupus susceptibility in a cohort from the Egypt Delta; relation to other ethnic groups. Hum. Immunol. 76 (7), 525–531. Epub 2015 Jun 17. doi:10.1016/j.humimm.2015.06.001
ElHefnawi, M., Hegazy, E., Elfiky, A., Jeon, Y., Jeon, S., Bhak, J., et al. (2021). Complete genome sequence and bioinformatics analysis of nine Egyptian females with clinical information from different geographic regions in Egypt. Gene 769, 145237. doi:10.1016/j.gene.2020.145237
Fadista, J., Manning, A. K., Florez, J. C., and Groop, L. (2016). The (in)famous GWAS P-value threshold revisited and updated for low-frequency variants. Eur. J. Hum. Genet. 24 (8), 1202–1205. doi:10.1038/ejhg.2015.269
Fukunaga, T., and Hamada, M. (2017). RIblast: An ultrafast RNA-RNA interaction prediction system based on a seed-and-extension approach. Bioinformatics 33 (17), 2666–2674. doi:10.1093/bioinformatics/btx287
Fukunaga, T., Iwakiri, J., Ono, Y., and Hamada, M. (2019). LncRRIsearch: A web server for lncRNA-RNA interaction prediction integrated with tissue-specific expression and subcellular localization data. Front. Genet. 10, 462. doi:10.3389/fgene.2019.00462
Gamazon, E. R., Zhang, W., Konkashbaev, A., Duan, S., Kistner, E. O., Nicolae, D. L., et al. (2009). Scan: SNP and copy number annotation. Bioinformatics 26 (2), 259–262. doi:10.1093/bioinformatics/btp644
Gao, S., Yuan, L., Wang, Y., and Hua, C. (2017). Enhanced expression of TREM-1 in splenic cDCs in lupus prone mice and it was modulated by miRNA-150. Mol. Immunol. 81, 127–134. doi:10.1016/j.molimm.2016.12.006
Gao, X. (2011). Multiple testing corrections for imputed SNPs. Genet. Epidemiol. 35 (3), 154–158. doi:10.1002/gepi.20563
Goulielmos, G. N., Zervou, M. I., Vazgiourakis, V. M., Ghodke-Puranik, Y., Garyfallos, A., and Niewold, T. B. (2018). The genetics and molecular pathogenesis of systemic lupus erythematosus (SLE) in populations of different ancestry. Gene 668, 59–72. doi:10.1016/j.gene.2018.05.041
Guan, T., Dominguez, C. X., Amezquita, R. A., Laidlaw, B. J., Cheng, J., Henao-Mejia, J., et al. (2018). ZEB1, ZEB2, and the miR-200 family form a counterregulatory network to regulate CD8+ T cell fates. J. Exp. Med. 215 (4), 1153–1168. doi:10.1084/jem.20171352
Ha, E., Bae, S. C., and Kim, K. (2022). Recent advances in understanding the genetic basis of systemic lupus erythematosus. Semin. Immunopathol. 44 (1), 29–46. doi:10.1007/s00281-021-00900-w
Hochberg, M. C. (1997). Updating the American College of Rheumatology revised criteria for the classification of systemic lupus erythematosus. Arthritis Rheum. 40 (9), 1725. doi:10.1002/art.1780400928
Jima, D. D., Zhang, J., Jacobs, C., Richards, K. L., Dunphy, C. H., Choi, W. W. L., et al. (2010). Deep sequencing of the small RNA transcriptome of normal and malignant human B cells identifies hundreds of novel microRNAs. Blood 116 (23), e118–e127. doi:10.1182/blood-2010-05-285403
Johnson, J. L., and Abecasis, G. R. (2017). GAS power calculator: Web-based power calculator for genetic association studies. Cold Spring Harbor, NY: bioRxiv, 164343. doi:10.1101/164343
Johnson, R. C., Nelson, G. W., Troyer, J. L., Lautenberger, J. A., Kessing, B. D., Winkler, C. A., et al. (2010). Accounting for multiple comparisons in a genome-wide association study (GWAS). BMC Genomics 11 (1), 724. doi:10.1186/1471-2164-11-724
Julia, A., Lopez-Longo, F. J., Perez Venegas, J. J., Bonas-Guarch, S., Olive, A., Andreu, J. L., et al. (2018). Genome-wide association study meta-analysis identifies five new loci for systemic lupus erythematosus. Arthritis Res. Ther. 20 (1), 100. doi:10.1186/s13075-018-1604-1
Ke, H., Zhao, L., Feng, X., Xu, H., Zou, L., Yang, Q., et al. (2016). NEAT1 is required for survival of breast cancer cells through FUS and miR-548. Gene Regul. Syst. Bio.GRSB 10s1, 11–17. doi:10.4137/grsb.S29414
Kim-Howard, X., Sun, C., Molineros, J. E., Maiti, A. K., Chandru, H., Adler, A., et al. (2014). Allelic heterogeneity in NCF2 associated with systemic lupus erythematosus (SLE) susceptibility across four ethnic populations. Hum. Mol. Genet. 23 (6), 1656–1668. doi:10.1093/hmg/ddt532
Kiniwa, Y., Fujita, T., Akada, M., Ito, K., Shofuda, T., Suzuki, Y., et al. (2001). Tumor antigens isolated from a patient with vitiligo and T-cell-infiltrated melanoma. Cancer Res. 61 (21), 7900–7907.
Kuo, C. F., Grainge, M. J., Valdes, A. M., See, L. C., Luo, S. F., Yu, K. H., et al. (2015). Familial aggregation of systemic lupus erythematosus and coaggregation of autoimmune diseases in affected families. JAMA Intern. Med. 175 (9), 1518–1526. doi:10.1001/jamainternmed.2015.3528
Labelle-Côté, M., Dusseault, J., Ismaïl, S., Picard-Cloutier, A., Siegel, P. M., and Larose, L. (2011). Nck2 promotes human melanoma cell proliferation, migration and invasion in vitro and primary melanoma-derived tumor growth in vivo. BMC Cancer 11 (1), 443. doi:10.1186/1471-2407-11-443
Langefeld, C. D., Ainsworth, H. C., Cunninghame Graham, D. S., Kelly, J. A., Comeau, M. E., Marion, M. C., et al. (2017). Transancestral mapping and genetic load in systemic lupus erythematosus. Nat. Commun. 8, 16021. doi:10.1038/ncomms16021
Lappalainen, T., Sammeth, M., Friedlander, M. R., t Hoen, P. A., Monlong, J., Rivas, M. A., et al. (2013). Transcriptome and genome sequencing uncovers functional variation in humans. Nature 501 (7468), 506–511. doi:10.1038/nature12531
Lazaridis, I., Nadel, D., Rollefson, G., Merrett, D. C., Rohland, N., Mallick, S., et al. (2016). Genomic insights into the origin of farming in the ancient Near East. Nature 536 (7617), 419–424. doi:10.1038/nature19310
Lee, H. S., Kim, T., Bang, S. Y., Na, Y. J., Kim, I., Kim, K., et al. (2014). Ethnic specificity of lupus-associated loci identified in a genome-wide association study in Korean women. Ann. Rheum. Dis. 73 (6), 1240–1245. Epub 2013 Jun 5. doi:10.1136/annrheumdis-2012-202675
Leslie, R., O'Donnell, C. J., and Johnson, A. D. (2014). Grasp: Analysis of genotype-phenotype results from 1390 genome-wide association studies and corresponding open access database. Bioinformatics 30 (12), i185–i194. doi:10.1093/bioinformatics/btu273
Lewis, M. J., and Jawad, A. S. (2017). The effect of ethnicity and genetic ancestry on the epidemiology, clinical features and outcome of systemic lupus erythematosus. Rheumatol. Oxf. 56 (1), i67–i77. doi:10.1093/rheumatology/kew399
Li, J., Qiu, D., Chen, Z., Du, W., Liu, J., and Mo, X. (2018). miR-548k regulates CXCL13 expression in myasthenia gravis patients with thymic hyperplasia and in Jurkat cells. J. Neuroimmunol. 320, 125–132. doi:10.1016/j.jneuroim.2018.03.021
Li, K., Xu, Y., and Yuan, L. N. (2019a). Down-regulation of miR-5702 is associated with clinical progression and poor prognosis in patients with non-small-cell lung cancer. Eur. Rev. Med. Pharmacol. Sci. 23 (5), 2047–2052. doi:10.26355/eurrev_201903_17245
Li, M., Qi, Y., Chen, M., Wang, Z., Zeng, D., Xiao, Y., et al. (2019b). GATA binding protein 3 boosts extracellular ATP hydrolysis and inhibits metastasis of breast cancer by up-regulating Ectonucleoside triphosphate diphosphohydrolase 3. Int. J. Biol. Sci. 15 (12), 2522–2537. doi:10.7150/ijbs.35563
Li, Y., Willer, C., Sanna, S., and Abecasis, G. (2009). Genotype imputation. Annu. Rev. Genomics Hum. Genet. 10, 387–406. doi:10.1146/annurev.genom.9.081307.164242
Mak, A., and Tay, S. H. (2014). Environmental factors, toxicants and systemic lupus erythematosus. Int. J. Mol. Sci. 15 (9), 16043–16056. doi:10.3390/ijms150916043
Marigorta, U. M., and Navarro, A. (2013). High trans-ethnic replicability of GWAS results implies common causal variants. PLoS Genet. 9 (6), e1003566. doi:10.1371/journal.pgen.1003566
Molineros, J. E., Yang, W., Zhou, X. J., Sun, C., Okada, Y., Zhang, H., et al. (2017). Confirmation of five novel susceptibility loci for systemic lupus erythematosus (SLE) and integrated network analysis of 82 SLE susceptibility loci. Hum. Mol. Genet. 26 (6), 1205–1216. doi:10.1093/hmg/ddx026
Morettin, A., Paris, G., Bouzid, Y., Baldwin, R. M., Falls, T. J., Bell, J. C., et al. (2017). Tudor domain containing protein 3 promotes tumorigenesis and invasive capacity of breast cancer cells. Sci. Rep. 7 (1), 5153. doi:10.1038/s41598-017-04955-4
Munz, M., Wohlers, I., Simon, E., Reinberger, T., Busch, H., Schaefer, A. S., et al. (2020). Qtlizer: Comprehensive QTL annotation of GWAS results. Sci. Rep. 10 (1), 20417. doi:10.1038/s41598-020-75770-7
Nakagawa, S. (2004). A farewell to Bonferroni: The problems of low statistical power and publication bias. Behav. Ecol. 15 (6), 1044–1045. doi:10.1093/beheco/arh107
Ohno, T., Meguro, A., Takeuchi, M., Yamane, T., Teshigawara, T., Kitaichi, N., et al. (2019). Association study of ARMC9 gene variants with vogt-koyanagi-harada disease in Japanese patients. Ocul. Immunol. Inflamm. 27 (5), 699–705. doi:10.1080/09273948.2018.1523438
Okumura, T., Ikeda, K., Ujihira, T., Okamoto, K., Horie-Inoue, K., Takeda, S., et al. (2017). Proteasome 26S subunit PSMD1 regulates breast cancer cell growth through p53 protein degradation. J. Biochem. 163 (1), 19–29. doi:10.1093/jb/mvx053
Otani, S., Sakurai, T., Yamamoto, K., Fujita, T., Matsuzaki, Y., Goto, Y., et al. (2006). Frequent immune response to a melanocyte specific protein KU-MEL-1 in patients with Vogt-Koyanagi-Harada disease. Br. J. Ophthalmol. 90 (6), 773–777. doi:10.1136/bjo.2005.086520
Perneger, T. V. (1998). What's wrong with Bonferroni adjustments. BMJ 316 (7139), 1236–1238. doi:10.1136/bmj.316.7139.1236
Petri, M., Orbai, A. M., Alarcón, G. S., Gordon, C., Merrill, J. T., Fortin, P. R., et al. (2012). Derivation and validation of the Systemic Lupus International Collaborating Clinics classification criteria for systemic lupus erythematosus. Arthritis Rheum. 64 (8), 2677–2686. doi:10.1002/art.34473
Piriyapongsa, J., and Jordan, I. K. (2007). A family of human MicroRNA genes from miniature inverted-repeat transposable elements. PLOS ONE 2 (2), e203. doi:10.1371/journal.pone.0000203
Pisetsky, D. S., Rovin, B. H., and Lipsky, P. E. (2017). New perspectives in rheumatology: Biomarkers as entry criteria for clinical trials of new therapies for systemic lupus erythematosus: The example of antinuclear antibodies and anti-DNA. Arthritis Rheumatol. 69 (3), 487–493. doi:10.1002/art.40008
Popejoy, A. B., and Fullerton, S. M. (2016). Genomics is failing on diversity. Nature 538 (7624), 161–164. doi:10.1038/538161a
Ramachandran, S., Ma, T. S., Griffin, J., Ng, N., Foskolou, I. P., Hwang, M. S., et al. (2021). Hypoxia-induced SETX links replication stress with the unfolded protein response. Nat. Commun. 12 (1), 3686. doi:10.1038/s41467-021-24066-z
Ramasamy, A., Trabzuni, D., Guelfi, S., Varghese, V., Smith, C., Walker, R., et al. (2014). Genetic variability in the regulation of gene expression in ten regions of the human brain. Nat. Neurosci. 17 (10), 1418–1428. doi:10.1038/nn.3801
Rung, J., Cauchi, S., Albrechtsen, A., Shen, L., Rocheleau, G., Cavalcanti-Proença, C., et al. (2009). Genetic variant near IRS1 is associated with type 2 diabetes, insulin resistance and hyperinsulinemia. Nat. Genet. 41 (10), 1110–1115. doi:10.1038/ng.443
Schuenemann, V. J., Peltzer, A., Welte, B., van Pelt, W. P., Molak, M., Wang, C. C., et al. (2017). Ancient Egyptian mummy genomes suggest an increase of Sub-Saharan African ancestry in post-Roman periods. Nat. Commun. 8, 15694. doi:10.1038/ncomms15694
Shi, Y., Qiu, M., Wu, Y., and Hai, L. (2015). MiR-548-3p functions as an anti-oncogenic regulator in breast cancer. Biomed. Pharmacother. 75, 111–116. doi:10.1016/j.biopha.2015.07.027
Skol, A. D., Scott, L. J., Abecasis, G. R., and Boehnke, M. (2006). Joint analysis is more efficient than replication-based analysis for two-stage genome-wide association studies. Nat. Genet. 38 (2), 209–213. doi:10.1038/ng1706
Slattery, M. L., Samowitz, W., Curtin, K., Ma, K. N., Hoffman, M., Caan, B., et al. (2004). Associations among IRS1, IRS2, IGF1, and IGFBP3 genetic polymorphisms and colorectal cancer. Cancer Epidemiol. Biomarkers Prev. 13 (7), 1206–1214. doi:10.1158/1055-9965.1206.13.7
Suhre, K., Arnold, M., Bhagwat, A. M., Cotton, R. J., Engelke, R., Raffler, J., et al. (2017). Connecting genetic risk to disease end points through the human blood plasma proteome. Nat. Commun. 8 (1), 14357. doi:10.1038/ncomms14357
Sun, C., Molineros, J. E., Looger, L. L., Zhou, X. J., Kim, K., Okada, Y., et al. (2016). High-density genotyping of immune-related loci identifies new SLE risk variants in individuals with Asian ancestry. Nat. Genet. 48, 323–330. doi:10.1038/ng.3496
Tangtanatakul, P., Thumarat, C., Satproedprai, N., Kunhapan, P., Chaiyasung, T., Klinchanhom, S., et al. (2020). Meta-analysis of genome-wide association study identifies FBN2 as a novel locus associated with systemic lupus erythematosus in Thai population. Arthritis Res. Ther. 22 (1), 185. doi:10.1186/s13075-020-02276-y
Tishkoff, S. A., Reed, F. A., Friedlaender, F. R., Ehret, C., Ranciaro, A., Froment, A., et al. (2009). The genetic structure and history of Africans and african Americans. Science 324(5930), 1035–1044. doi:10.1126/science.1172257
Treamtrakanpon, W., Tantivitayakul, P., Benjachat, T., Somparn, P., Kittikowit, W., Eiam-ong, S., et al. (2012). APRIL, a proliferation-inducing ligand, as a potential marker of lupus nephritis. Arthritis Res. Ther. 14 (6), R252. doi:10.1186/ar4095
Tu, Y., Li, F., and Wu, C. (1998). Nck-2, a novel src homology2/3-containing adaptor protein that interacts with the LIM-only protein PINCH and components of growth factor receptor kinase-signaling pathways. Mol. Biol. Cell 9 (12), 3367–3382. doi:10.1091/mbc.9.12.3367
Tu, Y., Liang, L., Frank, S. J., and Wu, C. (2001). Src homology 3 domain-dependent interaction of Nck-2 with insulin receptor substrate-1. Biochem. J. 354 (2), 315–322. doi:10.1042/0264-6021:3540315
Van De Weghe, J. C., Rusterholz, T. D. S., Latour, B., Grout, M. E., Aldinger, K. A., Shaheen, R., et al. (2017). Mutations in ARMC9, which encodes a basal body protein, cause Joubert syndrome in humans and ciliopathy phenotypes in zebrafish. Am. J. Hum. Genet. 101 (1), 23–36. doi:10.1016/j.ajhg.2017.05.010
Voight, B. F., Scott, L. J., Steinthorsdottir, V., Morris, A. P., Dina, C., Welch, R. P., et al. (2010). Twelve type 2 diabetes susceptibility loci identified through large-scale association analysis. Nat. Genet. 42 (7), 579–589. doi:10.1038/ng.609
Wan, L., Zhu, J., and Wu, Q. (2021). Knockdown of DIAPH3 inhibits the proliferation of cervical cancer cells through inactivating mTOR signaling pathway. J. Oncol. 2021, 4228241. doi:10.1155/2021/4228241
Wang, J., Zou, Y., Du, B., Li, W., Yu, G., Li, L., et al. (2021a). SNP-mediated lncRNA-ENTPD3-AS1 upregulation suppresses renal cell carcinoma via miR-155/HIF-1α signaling. Cell Death Dis. 12 (7), 672. doi:10.1038/s41419-021-03958-4
Wang, M., Futamura, M., Wang, Y., and You, M. (2005). Pas1c1 is a candidate for the mouse pulmonary adenoma susceptibility 1 locus. Oncogene 24 (11), 1958–1963. doi:10.1038/sj.onc.1208295
Wang, Y. F., Zhang, Y., Lin, Z., Zhang, H., Wang, T. Y., Cao, Y., et al. (2021b). Identification of 38 novel loci for systemic lupus erythematosus and genetic heterogeneity between ancestral groups. Nat. Commun. 12 (1), 772. doi:10.1038/s41467-021-21049-y
Wang, Y. F., Zhang, Y., Zhu, Z., Wang, T. Y., Morris, D. L., Shen, J. J., et al. (2018). Identification of ST3AGL4, MFHAS1, CSNK2A2 and CD226 as loci associated with systemic lupus erythematosus (SLE) and evaluation of SLE genetics in drug repositioning. Ann. Rheum. Dis. 77 (7), 1078–1084. doi:10.1136/annrheumdis-2018-213093
Ward, L. D., and Kellis, M. (2012). HaploReg: A resource for exploring chromatin states, conservation, and regulatory motif alterations within sets of genetically linked variants. Nucleic Acids Res. 40, D930–D934. doi:10.1093/nar/gkr917
Westra, H. J., Peters, M. J., Esko, T., Yaghootkar, H., Schurmann, C., Kettunen, J., et al. (2013). Systematic identification of trans eQTLs as putative drivers of known disease associations. Nat. Genet. 45 (10), 1238–1243. doi:10.1038/ng.2756
Wohlers, I., Künstner, A., Munz, M., Olbrich, M., Fähnrich, A., Calonga-Solís, V., et al. (2020). An integrated personal and population-based Egyptian genome reference. Nat. Commun. 11 (1), 4719. doi:10.1038/s41467-020-17964-1
Wojcik, G. L., Graff, M., Nishimura, K. K., Tao, R., Haessler, J., Gignoux, C. R., et al. (2019). Genetic analyses of diverse populations improves discovery for complex traits. Nature 570 (7762), 514–518. doi:10.1038/s41586-019-1310-4
Xia, K., Shabalin, A. A., Huang, S., Madar, V., Zhou, Y.-H., Wang, W., et al. (2011). seeQTL: a searchable database for human eQTLs. Bioinformatics 28 (3), 451–452. doi:10.1093/bioinformatics/btr678
Xiao, C., and Rajewsky, K. (2009). MicroRNA control in the immune system: Basic principles. Cell 136 (1), 26–36. doi:10.1016/j.cell.2008.12.027
Yang, J., Lee, S. H., Goddard, M. E., and Visscher, P. M. (2011). Gcta: A tool for genome-wide complex trait analysis. Am. J. Hum. Genet. 88 (1), 76–82. Epub 2010 Dec 17. doi:10.1016/j.ajhg.2010.11.011
Yelo, E., Bernardo, M. V., Gimeno, L., Alcaraz-García, M. J., Majado, M. J., and Parrado, A. (2008). Dock10, a novel CZH protein selectively induced by interleukin-4 in human B lymphocytes. Mol. Immunol. 45 (12), 3411–3418. doi:10.1016/j.molimm.2008.04.003
Yin, X., Kim, K., Suetsugu, H., Bang, S. Y., Wen, L., Koido, M., et al. (2020). Meta-analysis of 208370 East Asians identifies 113 susceptibility loci for systemic lupus erythematosus. Ann. Rheum. Dis. 80, 632–640. doi:10.1136/annrheumdis-2020-219209
Yu, C.-H., Pal, L. R., and Moult, J. (2016). Consensus genome-wide expression quantitative trait loci and their relationship with human complex trait disease. OMICS A J. Integr. Biol. 20 (7), 400–414. doi:10.1089/omi.2016.0063
Yu, K., Li, Q., Cheng, Q., Huang, C., Zheng, J., Chen, S., et al. (2017). MicroRNA-548j inhibits type I interferon production by targeting ZBTB11 in patients with chronic Hepatitis B. Biochem. Biophys. Res. Commun. 488 (4), 628–633. doi:10.1016/j.bbrc.2017.03.109
Yuanfeng, G., Song, J., Li, S., Cui, Y., Lee, C., and Chen, H. (2016). GW27-e0470 mutations in SCAP and AGXT2 revealed by exome sequencing in a pedigree with premature myocardial infarction. J. Am. Coll. Cardiol. 68(16_Supplement), C19–C20. doi:10.1016/j.jacc.2016.07.073
Zaitlen, N., Paşaniuc, B., Gur, T., Ziv, E., and Halperin, E. (2010). Leveraging genetic variability across populations for the identification of causal variants. Am. J. Hum. Genet. 86 (1), 23–33. doi:10.1016/j.ajhg.2009.11.016
Zhang, C., Xue, Q., Xu, Z., and Lu, C. (2018). MiR-5702 suppresses proliferation and invasion in non-small-cell lung cancer cells via posttranscriptional suppression of ZEB1. J. Biochem. Mol. Toxicol. 32 (7), e22163. doi:10.1002/jbt.22163
Keywords: GWAS, lupus, admixture, Egypt, imputation
Citation: Elghzaly AA, Sun C, Looger LL, Hirose M, Salama M, Khalil NM, Behiry ME, Hegazy MT, Hussein MA, Salem MN, Eltoraby E, Tawhid Z, Alwasefy M, Allam W, El-Shiekh I, Elserafy M, Abdelnaser A, Hashish S, Shebl N, Shahba AA, Elgirby A, Hassab A, Refay K, El-Touchy HM, Youssef A, Shabacy F, Hashim AA, Abdelzaher A, Alshebini E, Fayez D, El-Bakry SA, Elzohri MH, Abdelsalam EN, El-Khamisy SF, Ibrahim S, Ragab G and Nath SK (2022) Genome-wide association study for systemic lupus erythematosus in an egyptian population. Front. Genet. 13:948505. doi: 10.3389/fgene.2022.948505
Received: 19 May 2022; Accepted: 30 September 2022;
Published: 17 October 2022.
Edited by:
Xianyong Yin, Department of Biostatistics, University of Michigan, United StatesReviewed by:
Xu-jie Zhou, First Hospital, Peking University, ChinaAshish Kapoor, University of Texas Health Science Center at Houston, United States
Copyright © 2022 Elghzaly, Sun, Looger, Hirose, Salama, Khalil, Behiry, Hegazy, Hussein, Salem, Eltoraby, Tawhid, Alwasefy, Allam, El-Shiekh, Elserafy, Abdelnaser, Hashish, Shebl, Shahba, Elgirby, Hassab, Refay, El-Touchy, Youssef, Shabacy, Hashim, Abdelzaher, Alshebini, Fayez, El-Bakry, Elzohri, Abdelsalam, El-Khamisy, Ibrahim, Ragab and Nath. This is an open-access article distributed under the terms of the Creative Commons Attribution License (CC BY). The use, distribution or reproduction in other forums is permitted, provided the original author(s) and the copyright owner(s) are credited and that the original publication in this journal is cited, in accordance with accepted academic practice. No use, distribution or reproduction is permitted which does not comply with these terms.
*Correspondence: Gaafar Ragab, GaafarR@gmail.com; Swapan K. Nath, Swapan-Nath@omrf.org
§These authors co-directed the project
†These authors have contributed equally to this work