- 1Department of Pharmacy, Shenzhen Longhua District Central Hospital, Shenzhen, China
- 2Department of Clinical Medicine, School of the Second Clinical Medicine, Anhui Medical University, Hefei, China
- 3Shenzhen Key Laboratory of Respiratory Diseases, Shenzhen People’s Hospital (The First Affiliated Hospital, Southern University of Science and Technology), Shenzhen, China
Objective: Based on TCGA database, a prediction model for 1-, 3-, and 5-year overall survival rates of gastric cancer (GC) patients was constructed by analyzing the critical risk factors affecting the prognosis of gastric cancer patients.
Method: Clinicopathological features as well as gene signature of GC patients were obtained from TCGA database. Patients were randomly divided into a training cohort and an internal validation cohort. Independent predictors of GC prognosis were analyzed by univariate and multivariate Cox analyses to construct nomogram. The accuracy and reliability of the model was further validated by calibration curves, ROC curves, and C-indexes, and the clinical utility of the model was analyzed by decision analysis curves.
Result: Age, sex, N stage, M stage, METTL16, RBM15, FMR1, IGFBP1, and FTO were significantly associated with the prognosis of GC patients, and these predictors were further included in the construction of nomogram. The C-indexes for the training cohort and validation set were 0.735 and 0.688, respectively. The results of the ROC curve analysis indicated that the area under the curve (AUC) exceeded 0.6 in training and validation sets at 1, 3, and 5 years.
Conclusion: We have constructed and validated a nomogram that provides individual survival condition prediction for GC patients. The prognostic model integrating gene signatures and clinicopathological characteristics would help clinicians determine the prognosis of patients with GC and develop individualized treatment plans.
Introduction
Gastric cancer (GC) is one of the most common, aggressive malignancies worldwide, with over 1 million newly diagnosed cases annually, and persists to be the third leading cause of cancer-related mortality (Bray et al., 2018; Siegel et al., 2019). Accordingly, early diagnosis of gastric cancer directly determines the clinical outcomes of patients with GC. It is reported that less than 30% of patients with a diagnosis of gastric cancer survived more than 5 years (Ferlay et al., 2015). However, owing to the silent and occult characteristics of early-stage GC, patients are frequently diagnosed at the advanced stage. Several risk factors contribute to GC, such as age, high salt intake, low fruit and vegetable consumption, and Helicobacter pylori infection (Tsugane and Sasazuki, 2007). Among factors mentioned earlier, H. pylori plays a pivotal role in predisposing one to chronic gastritis, atrophic gastritis, intestinal metaplasia, dysplasia, and eventually gastric cancer (den Hoed and Kuipers, 2016). Up to now, surgical resection is the best treatment option for patients with gastric cancer. Although there were advances in surgical techniques, radiotherapy, chemotherapy, and neoadjuvant therapy, GC’s overall disease and death burden unacceptably continued to increase (Tan, 2019). Therefore, there is an urgent need to develop an effective strategy for the early diagnosis and treatment of GC.
RNA modification plays an indispensable role in the post-transcriptional regulation of gene expression (He, 2010). Numerous studies have shown that dysregulation of m6A regulator expression and genetic changes are associated with the disruption of a variety of biological processes, including dysregulation of cell death and proliferation, developmental defects, malignancy progression, and abnormal immune regulation (Fu et al., 2014; Pinello et al., 2018; Tong et al., 2018). In addition, a growing number of research suggest that m6A modification is responsible for a variety of human cancers, particularly gastric cancer (Jaffrey and Kharas, 2017). Zhang et al. (2020a) recently found that m6A modifications play an integral role in the development of tumor microenvironment (TME) diversity and complexity, and evaluating the pattern of m6A modifications in individual tumors will help guide more effective immunotherapeutic strategies. Both Wang et al. (2020) and Liu et al. (2022) demonstrated that m6A RNA modification mediated by methyltransferase 3 (METTL3) promoted the growth of gastric cancer. As such, we assessed the clinical correlation of m6A modification and identified the pathways and phenotypes regulated by m6A modification to explore the mechanism of m6A in gastric cancer in this study.
The tumor lymph node metastasis (TNM) staging system, proposed by the American Joint Committee on Cancer (AJCC) and the International Union Against Cancer (UICC), is widely used to evaluate tumor staging and predict the prognosis of cancer patients (Sano et al., 2017). Nevertheless, the TNM staging system is not adequate to precisely predict survival outcomes for individual patients, as other clinicopathological parameters such as age, gender, and ethnicity also affect the ultimate survival rate of GC patients (Ashktorab et al., 2017; Yao et al., 2020). In addition, Huo et al. (2021) recently presented a study investigating the prognostic significance of several genes for GC. Although the study was substantial and the data were analyzed retrospectively, clinical data that play an indispensable role in predicting the prognosis of GC were not covered in their study. Therefore, the nomogram has emerged as a more advanced method due to its ability to predict the survival outcome of an individual based on more comprehensive set of patient characteristics.
In this study, we obtained the clinical data and m6A-related gene expression data provided by TCGA database. We retrospectively analyzed all of them for potential risk factors for GC and then constructed a predictive nomogram to assess the probability of survival at 1, 3, and 5 years.
Materials and methods
Data collection
This study investigated the prognosis of GC patients using the Cancer Genome Atlas (TCGA) database (https://portal.gdc.cancer.gov/). TCGA database is a collaboration between the National Cancer Institute (NCI) and the National Human Genome Research Institute (NHGRI) of the United States project to provide a large, free reference for cancer research by collecting and organizing a variety of histological data related to cancer. In this study, the transcriptome profiling for the GC project was selected in the format of HTSeq-FPKM, which included 32 normal tissue samples and 375 cancer tissue samples. Clinical data for the “bcr xml” workflow type were also downloaded for 443 GC patients. Patients with missing clinical data will be excluded from subsequent analyses. Figure 1A illustrated the screening process for this study.
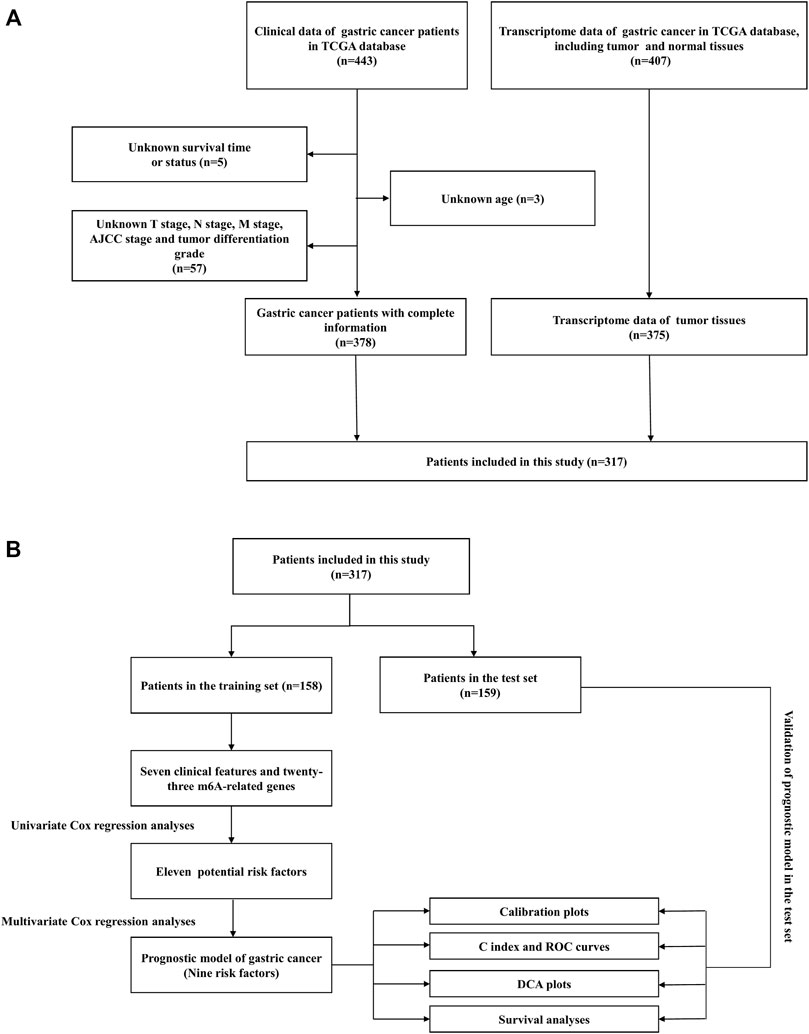
FIGURE 1. (A) Flowchart of the patient screening process. (B) Flowchart of the research process of this article.
Variables and outcomes
The variables initially included in this study were divided into two categories, including 23 m6A-related genes and several clinical variables. Based on previous studies, m6A-associated genes were classified into three categories, involving writes (METTL3, METTL14, METTL16, WTAP, VIRMA, ZC3H13, RBM15, and RBM15B), readers (YTHDC1, YTHDC2, YTHDF1, YTHDF2, YTHDF3, HNRNPC, FMR1, LRPPRC, HNRNPA2B1, IGFBP1, IGFBP2, IGFBP3, and RBMX), and erasers (FTO and ALKBH5). Clinical variables extracted from TCGA database were listed as follows: age, sex, tumor differentiation grade, AJCC stage, T stage, M stage, N stage, survival time, and survival status. The outcome was overall survival (OS), which was defined as the time from initiation to death due to any cause. For subjects lost to follow-up before death, the time of the last follow-up visit was usually considered as the time of death.
Statistical analysis
After setting the seed number, patients were divided into training set and validation set at a ratio of 1:1.
Univariate Cox analysis and multivariate Cox regression for independent prognostic factors
Univariate Cox analysis was performed to determine the association between survival outcomes and variables. These variables included demographic characteristics (age and gender), tumor characteristics (grade, AJCC stage, T stage, M stage, and N stage), and 23 m6A-related genes. Statistically significant potential risk factors were further analyzed by multivariate Cox regression, and hazard ratios (HRs) with 95% confidence intervals (CIs) were calculated. Considering that the AJCC stage is a comprehensive result of T, M, and N stages, the AJCC stage is not considered as one of the variables in the multivariate regression analysis.
Construction of the prognostic nomogram
According to the results of multivariate regression analyses, clinical characteristics and m6A-related genes were integrated through the “rms,” “foreign,” “survival,” and “regplot” packages of R software to construct prognostic nomogram. Scores for each risk factor listed in the nomogram were summed to predict OS at 1, 3, and 5 years for individual GC patients. Simultaneously, we grouped GC patients into low-risk groups and high-risk groups according to median scores. Survival analyses were performed between the high-risk and low-risk groups.
Validation of the prognostic nomogram
Discrimination is measured by the area under the curve (AUC) of the ROC curve and the concordance index (C-index). Ranging from 0.5 to 1, excellent discrimination is represented by values of 0.6 or more for both AUC and C-index. Furthermore, we also used the calibration plot to measure the extent of proximity between predicted risk and actual risk. Decision curve analysis (DCA) also was used to determine whether the predictive nomogram can improve the prognosis of GC patients in the clinical decisions.
Results
Characteristics of the gastric cancer patients
A total of 317 patients with GC were obtained from TCGA database. Fifty percent of the patients from TCGA database were used as the training cohort and the remaining patients were treated as the internal validation cohort. The detailed selection process and study process are presented in Figure 1. In the training set, men accounted for 70% of the total number of patients, 6.94% patients were in M1 stage, and 20.8% patients were in N3 stage, while in the validation cohort, 61.1% were men, 4.21% patients were in M1 stage, and 17.9% patients were in N3 stage. The detailed clinicopathological characteristics of the patients are displayed in Table 1.
Construction of the prognostic nomogram
At a p-value cutoff of 0.2 for univariate Cox analysis and multivariate Cox analysis, the potential risk factors were listed as follows: age, gender, M stage, N stage, and five m6A-related genes (METTL16, RBM15, FMR1, IGFBP1, and FTO) (Figure 2). We performed a nomogram using independent variables associated with OS identified in the training set. The nomogram sums the scores for each risk factor identified on the scale, and by adding up the scores for individual items in the nomogram, the 1-, 3-, and 5-year OS for an individual patient can be predicted from the total scores shown at the bottom of the graph (Figure 3). The results of univariate and multivariate Cox analyses are presented as HRs and corresponding 95% CIs in Table 2.
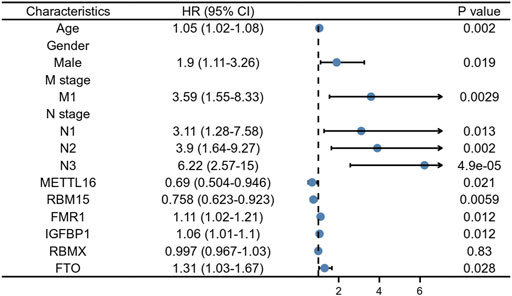
FIGURE 2. Forest plot for multivariate Cox regression analysis of GC patients in the training cohort.
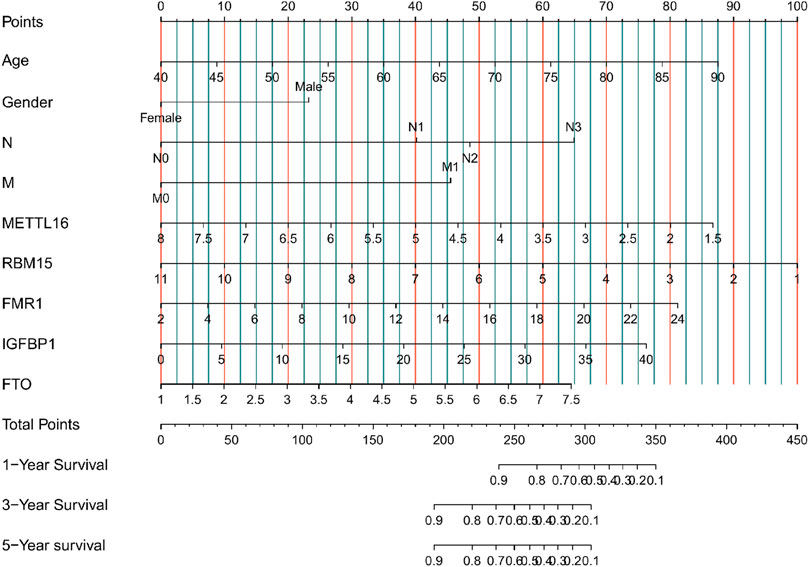
FIGURE 3. Nomogram for predicting 1-, 3-, and 5-year overall survival (OS) for GC patients in the training cohort.
Calibration and validation of the prognostic nomogram
Calibration curves illustrated that there is a strong agreement between predicted survival probabilities and actual survival outcomes (Figure 4). The C-index values for GC patients were 0.735 (95% CI = 0.668–0.802) and 0.688 (95% CI = 0.614–0.762) for training cohort and internal validation cohort, respectively, which demonstrated excellent discrimination. We also plotted the ROC curves and calculated the corresponding AUC values. The AUC values for predicting OS at 1, 3, and 5 years were 0.76, 0.79, and 0.82 in the training cohort and 0.67, 0.67, and 0.64 in the validation cohort, respectively (Figure 5). The AUC values were greater than 0.6, which revealed an excellent accuracy of this predictive model. In addition, as presented in Figure 6, the DCA also indicated excellent clinical applicability for predicting the overall survival of GC patients.
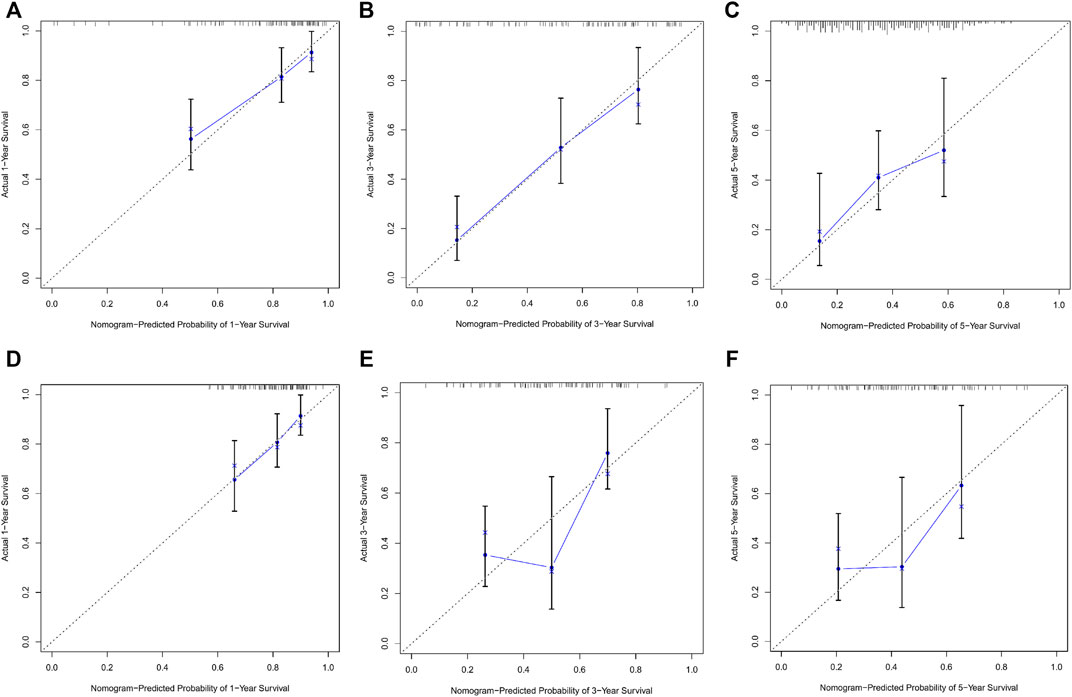
FIGURE 4. (A–C) Nomogram calibration plots to predict 1-, 3-, and 5-year overall survival (OS) in the training cohort. (D–F) Nomogram calibration plots to predict 1-, 3-, and 5-year overall survival (OS) in the validation cohort.
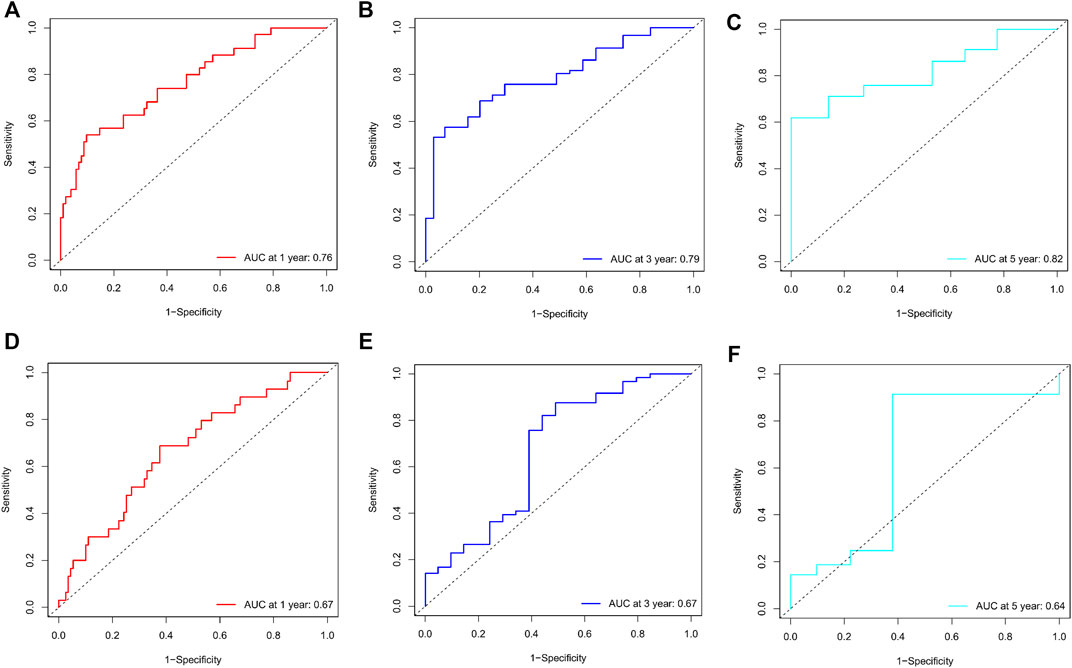
FIGURE 5. (A–C) Nomogram ROC curves to predict 1-, 3-, and 5-year overall survival (OS) in the training cohort. (D–F) Nomogram ROC curves to predict 1-, 3-, and 5-year overall survival (OS) in the validation cohort.
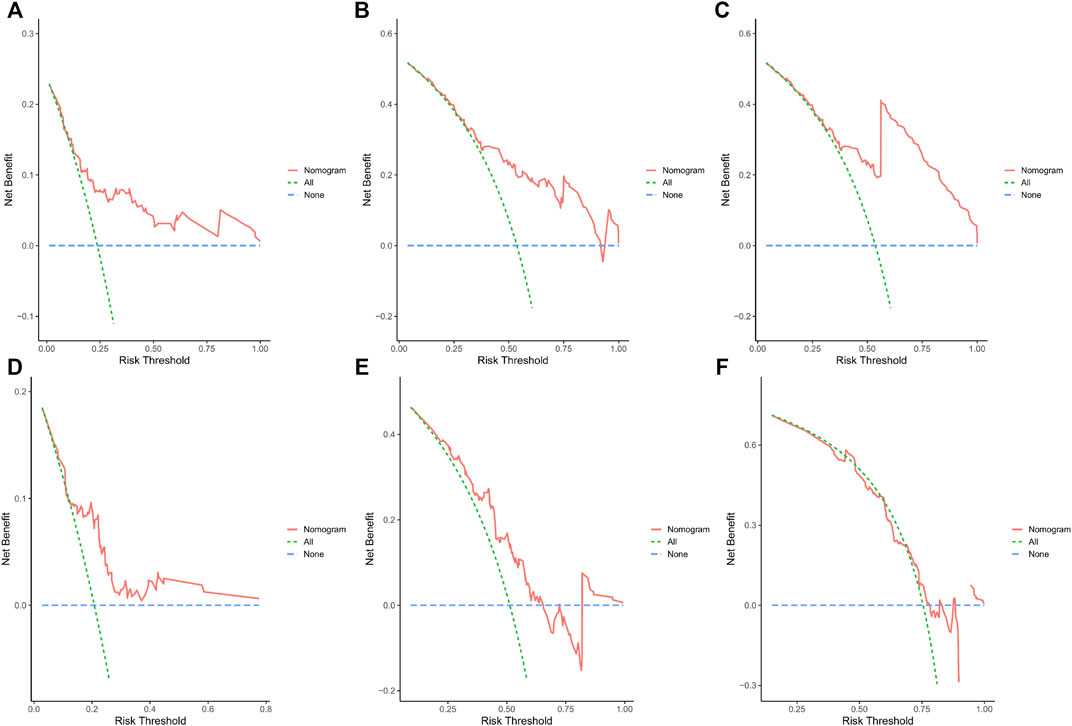
FIGURE 6. (A–C) DCA predicting 1-, 3-, and 5-year OS in the training cohort. (D–F) DCA analysis predicting 1-, 3-, and 5-year OS in the validation cohort.
Incorporating the results of the DCA curve, C-index, ROC curve, and calibration curve, we found that the prediction model constructed based on the abovementioned factors had a significant predictive value for OS in GC patients with high accuracy and clinical applicability.
Survival analyses
After summing the risk scores of all independent risk factors, patients were divided into low-risk group and high-risk group according to the median score. Considering all prognostic factors and the different risk groups, survival analyses were performed by Kaplan–Meier plots for both the training and internal validation groups (Figure 7). The prognosis was better in the low-risk group than in the high-risk group (p < 0.05).
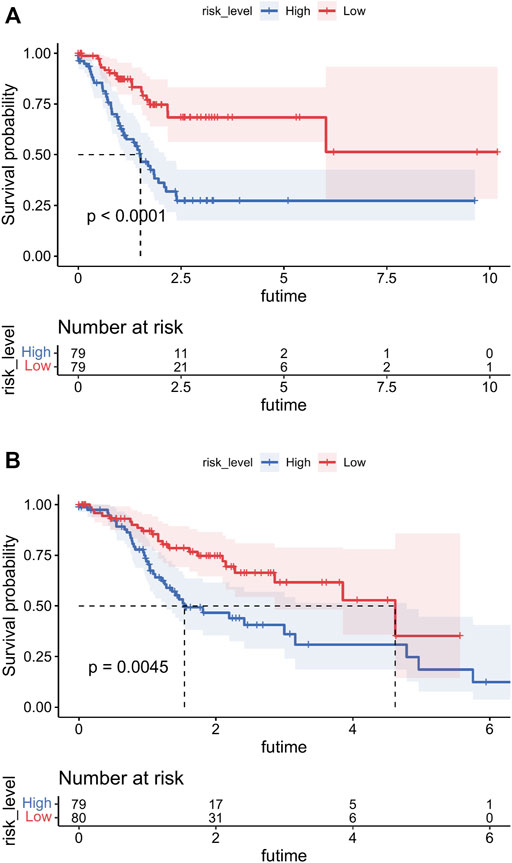
FIGURE 7. Overall survival (OS) Kaplan–Meier curves for patients in the low- and high-risk groups. (A) Training cohort. (B) Internal validation cohort.
Discussion
Gastric cancer represents the second most common cause of cancer (Joshi and Badgwell, 2021). GC is a multifactorial disease, with both environmental and genetic factors influencing its onset and development (Machlowska et al., 2020). Therefore, the prognosis of GC is of great importance to patients. Currently, although there is a large amount of constructed models to predict the prognosis of GC patients, the variables included were confined, especially the m6A-related genes they incorporated were limited, and other important clinicopathological characteristics such as age, gender, and staging were ignored (Su et al., 2019; Zhang B. et al., 2020; Li et al., 2021; Yue et al., 2022). The AJCC staging system is still the most widely used method to assess the prognosis of patients with GC based on factors such as the location of the primary lesion, tumor size, and the presence of distant metastases (Kumagai and Sano, 2021). However, it has some drawbacks that should not be overlooked. It ignores other important clinicopathological characteristics of patients that can affect survival, such as gender, age, ethnicity, as well as cancer genes involved in the formation and development of tumor cells. Genes have been shown to play a remarkably significant role in the initiation, growth, progression, metastasis, immune evasion, and suppression of many kinds of cancer (Dhanasekaran et al., 2022; Luo et al., 2022). A number of gene-specific studies have been carried out to predict the prognosis of tumors, particularly aggressive malignancies, focusing on ferroptosis, pyroptosis, and m6A-related genes (Ghosh et al., 2022; Liang et al., 2021; Zhou Z. et al., 2022). N6-methyladenosine (m6A) RNA modification has emerged as a novel regulatory mechanism for controlling eukaryotic gene expression. m6A is the most abundant internal RNA modification in eukaryotic cells and has received increasing attention in recent years (Sun et al., 2019; Huang et al., 2020). m6A regulates gene expression and cellular processes, including cellular self-renewal, differentiation, invasion, and apoptosis (He et al., 2019). The mechanisms of m6A RNA modification under physiological and pathological conditions have also been revealed. m6A modifications are modified by m6A methyltransferases or writers, such as METTL3/14/16, RBM15/15B, ZC3H3, VIRMA, CBLL1, WTAP, and KIAA1429, and removed by demethylases or erasers, including FTO and ALKBH5. It is recognized by the m6A binding proteins YTHDF1/2/3, YTHDC1/2, IGF2BP1/2/3, and HNRNPA2B1, which are also known as “readers” (Jiang et al., 2021). Most studies have shown that m6A modifications can influence the complexity of cancer progression by regulating biological functions associated with cancer (Ma et al., 2019). The role of m6A modifications in the development, metastasis, and invasion of gastric cancer has been gradually elucidated as potential mechanisms have been revealed. As such, m6A and its regulators contribute to the prognosis of GC, and are expected to be targeted for cancer diagnosis and treatment (Zhao et al., 2021; Hu et al., 2022). Currently, many studies have constructed prognostic models for patients with gastric cancer-targeting m6A modifications (Athauda et al., 2020; Wang et al., 2022). However, a failure to integrate m6A-related genes with other critical clinicopathological characteristics implied that their predictive models had unmitigated limitations.
This study was based on TCGA database, and screened for genetic and detailed clinicopathological characteristics in the tissues of 317 GC patients. Fifty percent of GC patients were used for modeling, with the remaining patients in the internal validation cohort. After univariate Cox regression and multivariate Cox regression, we derived that patient age, sex, N stage, M stage, METTL16, RBM15, FMR1, IGFBP1, and FTO were independent prognostic factors for OS in GC patients and constructed predictive models. The results of our study are consistent with the AJCC grading results. Patients with GC have a worse prognosis when the extent of their primary tumor is more extensive and when there are lymph node metastases and distant metastases. Notably, the extent of the primary tumor and depth of invasion were statistically significantly associated with adverse prognosis for patients with gastric cancer (N1: HR = 3.15, 95% CI 1.28–7.73; N2: HR = 4.05, 95% CI 1.71–9.95; N3: HR = 6.58, 95% CI 2.74–15.8). Our results showed that men had a worse prognosis than women. In line with our findings, Athauda et al. (2020) showed that women with gastric cancer had a more favorable clinical outcome than men. Wang et al. (2021) recently revealed that METTL16-mediated m6A methylation promotes GC cell proliferation through enhanced cyclin D1 expression, and both in vitro and in vivo experiments confirmed that METTL16 promotes the growth of the tumor by GC cell proliferation. Consistent with our study, other studies have similarly shown that RBM15 is associated with the prediction of gastric cancer. However, the precise mechanism has not been fully elucidated and requires subsequent research (Zhang J. et al., 2020b; Jing et al., 2021). Luo and her colleagues demonstrated that IGFBP-1 inhibited the migration of BGC-823 cells and played a protective role in H. pylori-induced gastric cancer, and HK and his colleagues’ data suggested that the IGF-IGFBP system may play an important role in the initiation, progression, and metastasis of gastric cancer. Since data are still lacking, future study is still urgently needed (Yi et al., 2001; Luo et al., 2017). Li et al. (2019b) elucidated that the aberrant expression of FTO has a significant prognostic value in GC patients, suggesting that FTO may play an essential role in gastric cancer progression and metastasis, and Zhou Y. et al. (2022) elucidated that FTO promotes growth and metastasis of gastric cancer via m6A modification of caveolin-1 and metabolic regulation of mitochondrial dynamics. Although no studies have yet elucidated the mechanism of FMR1 and RBMX in gastric cancer, many articles have demonstrated that FMR1 and RBMX were associated with the proliferation or metastasis in many kinds of cancers such as esophageal carcinoma and bladder cancer (Li et al., 2019a; Yan et al., 2021).
There are some inevitable constraints in this cohort study. First, retrospective studies may be subject to selection bias in the selection of patients. Second, due to the limited clinical information available on patients in TCGA database, more valuable clinical factors were not considered in the analysis. In addition, we did not consider other genes, particularly immune genes, which have been shown to be strongly correlated with the development and proliferation of cancer. Despite these drawbacks, our study has some notable strengths. As far as we are concerned, it is the first nomogram that integrates clinicopathological features and m6A-related genes to predict the prognosis of GC patients, which makes the predictive model more comprehensive and able to predict the survival time of each patient more accurately. Second, in addition to building the model, this study also verified the model, and the validation results showed the stability and reliability of the model.
In summary, based on the clinical and genetic data of GC patients extracted from TCGA database, we have established a relatively effective prediction model to assess the survival of GC patients at 1, 3, and 5 years, and the m6A-related genes may subsequently be targeted for the treatment of GC. The accuracy and usefulness of the nomogram still need to be further validated in subsequent clinical work.
Data availability statement
The original contributions presented in the study are included in the article/Supplementary Material; further inquiries can be directed to the corresponding author.
Author contributions
XW designed the whole research study. XW and KX performed research. XW contributed to drafting the manuscript. XL, JG, and JR reviewed and revised the manuscript. All authors have read and approved the final submitted manuscript.
Funding
This work was supported by the Project of Guangdong Administration of Traditional Chinese Medicine (No. 20221273) and research grants from the Shenzhen Longhua District Science and Technology Innovation Fund Projects (Nos. 2022045, 2020036, and 2020152).
Acknowledgments
We thank all the researchers who participated in the whole experimental study and appreciate all the reviewers for their helpful comments on this article.
Conflict of interest
The authors declare that the research was conducted in the absence of any commercial or financial relationships that could be construed as a potential conflict of interest.
Publisher’s note
All claims expressed in this article are solely those of the authors and do not necessarily represent those of their affiliated organizations, or those of the publisher, the editors, and the reviewers. Any product that may be evaluated in this article, or claim that may be made by its manufacturer, is not guaranteed or endorsed by the publisher.
Reference
Ashktorab, H., Kupfer, S. S., Brim, H., and Carethers, J. M. (2017). Racial disparity in gastrointestinal cancer risk. Gastroenterology 153, 910–923. doi:10.1053/j.gastro.2017.08.018
Athauda, A., Nankivell, M., Langley, R. E., Alderson, D., Allum, W., Grabsch, H. I., et al. (2020). Impact of sex and age on chemotherapy efficacy, toxicity and survival in localised oesophagogastric cancer: A pooled analysis of 3265 individual patient data from four large randomised trials (OE02, OE05, magic and ST03). Eur. J. Cancer 137, 45–56. doi:10.1016/j.ejca.2020.06.005
Bray, F., Ferlay, J., Soerjomataram, I., Siegel, R. L., Torre, L. A., Jemal, A., et al. (2018). Global cancer statistics 2018: GLOBOCAN estimates of incidence and mortality worldwide for 36 cancers in 185 countries. Ca. Cancer J. Clin. 68, 394–424. doi:10.3322/caac.21492
den Hoed, C. M., and Kuipers, E. J. (2016). Gastric cancer: How can we reduce the incidence of this disease? Curr. Gastroenterol. Rep. 18, 34. doi:10.1007/s11894-016-0506-0
Dhanasekaran, R., Deutzmann, A., Mahauad-Fernandez, W. D., Hansen, A. S., Gouw, A. M., Felsher, D. W., et al. (2022). The MYC oncogene - the grand orchestrator of cancer growth and immune evasion. Nat. Rev. Clin. Oncol. 19, 23–36. doi:10.1038/s41571-021-00549-2
Ferlay, J., Soerjomataram, I., Dikshit, R., Eser, S., Mathers, C., Rebelo, M., et al. (2015). Cancer incidence and mortality worldwide: Sources, methods and major patterns in GLOBOCAN 2012. Int. J. Cancer 136, E359–E386. doi:10.1002/ijc.29210
Fu, Y., Dominissini, D., Rechavi, G., and He, C. (2014). Gene expression regulation mediated through reversible m⁶A RNA methylation. Nat. Rev. Genet. 15, 293–306. doi:10.1038/nrg3724
Ghosh, A., Das, C., Ghose, S., Maitra, A., Roy, B., Majumder, P. P., et al. (2022). Integrative analysis of genomic and transcriptomic data of normal, tumour, and co-occurring leukoplakia tissue triads drawn from patients with gingivobuccal oral cancer identifies signatures of tumour initiation and progression. J. Pathology. Online Ahead of Print . doi:10.1002/path.5900
He, C. (2010). Grand challenge commentary: RNA epigenetics? Nat. Chem. Biol. 6, 863–865. doi:10.1038/nchembio.482
He, L., Li, H., Wu, A., Peng, Y., Shu, G., Yin, G., et al. (2019). Functions of N6-methyladenosine and its role in cancer. Mol. Cancer 18, 176. doi:10.1186/s12943-019-1109-9
Hu, Y., Gong, C., Li, Z., Liu, J., Chen, Y., Huang, Y., et al. (2022). Demethylase ALKBH5 suppresses invasion of gastric cancer via PKMYT1 m6A modification. Mol. Cancer 21, 34. doi:10.1186/s12943-022-01522-y
Huang, H., Weng, H., and Chen, J. (2020). m6A modification in coding and non-coding RNAs: Roles and therapeutic implications in cancer. Cancer Cell 37, 270–288. doi:10.1016/j.ccell.2020.02.004
Huo, J., Wu, L., and Zang, Y. (2021). Eight-gene prognostic signature associated with hypoxia and ferroptosis for gastric cancer with general applicability. Epigenomics 13, 875–890. doi:10.2217/epi-2020-0411
Jaffrey, S. R., and Kharas, M. G. (2017). Emerging links between m6A and misregulated mRNA methylation in cancer. Genome Med. 9, 2. doi:10.1186/s13073-016-0395-8
Jiang, X., Liu, B., Nie, Z., Duan, L., Xiong, Q., Jin, Z., et al. (2021). The role of m6A modification in the biological functions and diseases. Signal Transduct. Target. Ther. 6, 74. doi:10.1038/s41392-020-00450-x
Jing, J.-J., Zhao, X., Li, H., Sun, L.-P., and Yuan, Y. (2021). Expression profiles and prognostic roles of m6A writers, erasers and readers in gastric cancer. Future Oncol. 17, 2605–2620. doi:10.2217/fon-2020-0630
Joshi, S. S., and Badgwell, B. D. (2021). Current treatment and recent progress in gastric cancer. Ca. Cancer J. Clin. 71, 264–279. doi:10.3322/caac.21657
Kumagai, K., and Sano, T. (2021). Revised points and disputed matters in the eighth edition of the TNM staging system for gastric cancer. Jpn. J. Clin. Oncol. 51, 1024–1027. doi:10.1093/jjco/hyab069
Li, J., Zuo, Z., Lai, S., Zheng, Z., Liu, B., Wei, Y., et al. (2021). Differential analysis of RNA methylation regulators in gastric cancer based on TCGA data set and construction of a prognostic model. J. Gastrointest. Oncol. 12 (4), 1384–1397. doi:10.21037/jgo-21-325
Li, W., Zhang, L., Guo, B., Deng, J., Wu, S., Li, F., et al. (2019a). Exosomal FMR1-AS1 facilitates maintaining cancer stem-like cell dynamic equilibrium via TLR7/NFκB/c-Myc signaling in female esophageal carcinoma. Mol. Cancer 18, 22. doi:10.1186/s12943-019-0949-7
Li, Y., Zheng, D., Wang, F., Xu, Y., Yu, H., Zhang, H., et al. (2019b). Expression of demethylase genes, FTO and ALKBH1, is associated with prognosis of gastric cancer. Dig. Dis. Sci. 64, 1503–1513. doi:10.1007/s10620-018-5452-2
Liang, C., Fan, J., Liang, C., and Guo, J. (2021). Identification and validation of a pyroptosis-related prognostic model for gastric cancer. Front. Genet. 12, 699503. doi:10.3389/fgene.2021.699503
Liu, Y., Zhai, E., Chen, J., Qian, Y., Zhao, R., Ma, Y., et al. (2022). m6 A-mediated regulation of PBX1-GCH1 axis promotes gastric cancer proliferation and metastasis by elevating tetrahydrobiopterin levels. Cancer Commun. 42, 327–344. doi:10.1002/cac2.12281
Luo, C., Sun, F., Zhu, H., Ni, Y., Fang, J., Liu, Y., et al. (2017). Insulin-like growth factor binding protein-1 (IGFBP-1) upregulated by Helicobacter pylori and is associated with gastric cancer cells migration. Pathol. Res. Pract. 213, 1029–1036. doi:10.1016/j.prp.2017.08.009
Luo, L., Santos, A., Konganti, K., Hillhouse, A., Lambertz, I. U., Zheng, Y., et al. (2022). Overexpression of IGF-1 during early development expands the number of mammary stem cells and primes them for transformation. Stem Cells 40, 273–289. doi:10.1093/stmcls/sxab018
Ma, S., Chen, C., Ji, X., Liu, J., Zhou, Q., Wang, G., et al. (2019). The interplay between m6A RNA methylation and noncoding RNA in cancer. J. Hematol. Oncol. 12, 121. doi:10.1186/s13045-019-0805-7
Machlowska, J., Baj, J., Sitarz, M., Maciejewski, R., and Sitarz, R. (2020). Gastric cancer: Epidemiology, risk factors, classification, genomic characteristics and treatment strategies. Int. J. Mol. Sci. 21, E4012. doi:10.3390/ijms21114012
Pinello, N., Sun, S., and Wong, J. J.-L. (2018). Aberrant expression of enzymes regulating m6A mRNA methylation: Implication in cancer. Cancer Biol. Med. 15, 323–334. doi:10.20892/j.issn.2095-3941.2018.0365
Sano, T., Coit, D. G., Kim, H. H., Roviello, F., Kassab, P., Wittekind, C., et al. (2017). Proposal of a new stage grouping of gastric cancer for TNM classification: International Gastric Cancer Association staging project. Gastric Cancer 20, 217–225. doi:10.1007/s10120-016-0601-9
Siegel, R. L., Miller, K. D., and Jemal, A. (2019). Cancer statistics, 2019. Ca. Cancer J. Clin. 69, 7–34. doi:10.3322/caac.21551
Su, Y., Huang, J., and Hu, J. (2019). m6A RNA methylation regulators contribute to malignant progression and have clinical prognostic impact in gastric cancer. Front. Oncol. 9, 1038. doi:10.3389/fonc.2019.01038
Sun, T., Wu, R., and Ming, L. (2019). The role of m6A RNA methylation in cancer. Biomed. Pharmacother. 112, 108613. doi:10.1016/j.biopha.2019.108613
Tan, Z. (2019). Recent advances in the surgical treatment of advanced gastric cancer: A review. Med. Sci. Monit. 25, 3537–3541. doi:10.12659/MSM.916475
Tong, J., Cao, G., Zhang, T., Sefik, E., Amezcua Vesely, M. C., Broughton, J. P., et al. (2018). m6A mRNA methylation sustains Treg suppressive functions. Cell Res. 28, 253–256. doi:10.1038/cr.2018.7
Tsugane, S., and Sasazuki, S. (2007). Diet and the risk of gastric cancer: Review of epidemiological evidence. Gastric Cancer 10, 75–83. doi:10.1007/s10120-007-0420-0
Wang, Q., Chen, C., Ding, Q., Zhao, Y., Wang, Z., Chen, J., et al. (2020). METTL3-mediated m6A modification of HDGF mRNA promotes gastric cancer progression and has prognostic significance. Gut 69, 1193–1205. doi:10.1136/gutjnl-2019-319639
Wang, X.-K., Zhang, Y.-W., Wang, C.-M., Li, B., Zhang, T.-Z., Zhou, W.-J., et al. (2021). METTL16 promotes cell proliferation by up-regulating cyclin D1 expression in gastric cancer. J. Cell. Mol. Med. 25, 6602–6617. doi:10.1111/jcmm.16664
Wang, Y., Zhu, G.-Q., Tian, D., Zhou, C.-W., Li, N., Feng, Y., et al. (2022). Comprehensive analysis of tumor immune microenvironment and prognosis of m6A-related lncRNAs in gastric cancer. BMC Cancer 22, 316. doi:10.1186/s12885-022-09377-8
Yan, Q., Zeng, P., Zhou, X., Zhao, X., Chen, R., Qiao, J., et al. (2021). RBMX suppresses tumorigenicity and progression of bladder cancer by interacting with the hnRNP A1 protein to regulate PKM alternative splicing. Oncogene 40, 2635–2650. doi:10.1038/s41388-021-01666-z
Yao, Q., Qi, X., and Xie, S.-H. (2020). Sex difference in the incidence of cardia and non-cardia gastric cancer in the United States, 1992-2014. BMC Gastroenterol. 20, 418. doi:10.1186/s12876-020-01551-1
Yi, H. K., Hwang, P. H., Yang, D. H., Kang, C. W., and Lee, D. Y. (20011990)., 37. Oxford, England, 2257–2263. doi:10.1016/s0959-8049(01)00269-6Expression of the insulin-like growth factors (IGFs) and the IGF-binding proteins (IGFBPs) in human gastric cancer cellsEur. J. Cancer17
Yue, Q., Zhang, Y., Bai, J., Duan, X., and Wang, H. (2022). Identification of five N6-methylandenosine-related ncRNA signatures to predict the overall survival of patients with gastric cancer. Dis. Markers 2022, 7765900. doi:10.1155/2022/7765900
Zhang, B., Wu, Q., Li, B., Wang, D., Wang, L., Zhou, Y. L., et al. (2020a). m6A regulator-mediated methylation modification patterns and tumor microenvironment infiltration characterization in gastric cancer. Mol. Cancer 19, 53. doi:10.1186/s12943-020-01170-0
Zhang, J., Piao, H.-Y., Wang, Y., Meng, X.-Y., Yang, D., Zhao, Y., et al. (2020b). To develop and validate the combination of RNA methylation regulators for the prognosis of patients with gastric cancer. Onco. Targets. Ther. 13, 10785–10795. doi:10.2147/OTT.S276239
Zhao, Y., Yan, X., Wang, Y., Zhou, J., and Yu, Y. (2021). N6-Methyladenosine regulators promote malignant progression of gastric adenocarcinoma. Front. Oncol. 11, 726018. doi:10.3389/fonc.2021.726018
Zhou Y, Y., Wang, Q., Deng, H., Xu, B., Zhou, Y., Liu, J., et al. (2022). N6-methyladenosine demethylase FTO promotes growth and metastasis of gastric cancer via m6A modification of caveolin-1 and metabolic regulation of mitochondrial dynamics. Cell Death Dis. 13 (1), 72. doi:10.1038/s41419-022-04503-7
Zhou Z, Z., Yang, Z., Cui, Y., Lu, S., Huang, Y., Che, X., et al. (2022). Identification and validation of a ferroptosis-related long non-coding RNA (FRlncRNA) signature to predict survival outcomes and the immune microenvironment in patients with clear cell renal cell carcinoma. Front. Genet. 13, 787884. doi:10.3389/fgene.2022.787884
Keywords: gastric cancer, m6A, prognosis, nomogram, TCGA
Citation: Wang X, Xu K, Liao X, Rao J, Huang K, Gao J, Xu G and Wang D (2022) Construction of a survival nomogram for gastric cancer based on the cancer genome atlas of m6A-related genes. Front. Genet. 13:936658. doi: 10.3389/fgene.2022.936658
Received: 11 May 2022; Accepted: 04 July 2022;
Published: 05 August 2022.
Edited by:
Zhenjian Zhuo, Guangzhou Medical University, ChinaCopyright © 2022 Wang, Xu, Liao, Rao, Huang, Gao, Xu and Wang. This is an open-access article distributed under the terms of the Creative Commons Attribution License (CC BY). The use, distribution or reproduction in other forums is permitted, provided the original author(s) and the copyright owner(s) are credited and that the original publication in this journal is cited, in accordance with accepted academic practice. No use, distribution or reproduction is permitted which does not comply with these terms.
*Correspondence: Xiaokang Wang, kangtae_won@i.smu.edu.cn