- 1Department of Clinical Laboratory, The Third Xiangya Hospital, Central South University, Changsha, Hunan, China
- 2Clinical Laboratory of Hunan Cancer Hospital, The Affiliated Cancer Hospital of Xiangya School of Medicine, Central South University, Hunan Key Laboratory of Oncotarget Gene, Changsha, Hunan, China
Immunotherapy is an individualized therapeutic strategy for nasopharyngeal carcinoma (NPC). However, few molecular targets are clinically satisfactory. This work aimed to integrate bulk and single-cell RNA sequencing data to identify novel biomarkers involved in NPC. We performed differentially expressed gene (DEG) analysis, Gene Ontology (GO) enrichment, Kyoto Encyclopedia of Genes and Genomes (KEGG) pathway analysis, and immune cell infiltration analysis prior to correlation analysis of the identified genes and immune cells and further assessed the prognostic effects of the biomarkers and immune cells in NPC. As a result, PKP1, a potential molecular biomarker associated with immune infiltration, and tumor-infiltrating lymphocyte-B cells (TIL-Bs) were identified as promising therapeutic targets for NPC. Importantly, immunohistochemistry (IHC) validated that PKP1 protein expression was mainly found in NPC cells rather than noncancerous cells. In addition, the tumor microenvironment (TME) of NPC was characterized by the infiltration of more dendritic cells (DCs) and γδT cells but fewer B cells. Our results suggest that the interaction of PKP1 and TIL-B cells is involved in NPC development. It is possible that TIL-B cells produce immunoglobulin G (IgG) to tumor antigens, such as PKP1, or viral antigens, including EBV and HPV, to execute antitumor ability through DC and T cells. In response, NPC cells express proteins such as PKP1 (absent in normal nasopharynx) to induce myeloid-derived suppressor cell (MDSC) expansion, which subsequently impairs the proliferation of B cells and results in B-cell death by generating iNOS and NOX2. In summary, our findings provide a potential therapeutic strategy for NPC by disrupting the interaction of PKP1 and TIL-Bs in the TME.
Introduction
Nasopharyngeal carcinoma (NPC) is a unique head and neck malignancy that originates from the nasopharynx. NPC exhibits a distinct geographic distribution in which >70% of new cases originate in southern China and southeast Asia (Chen et al., 2019; Wong et al., 2021). Since the location of NPC is anatomically hidden and patients often exhibit no precursors or specific clinical symptoms, the discovery of the disease is usually delayed until it has reached an advanced stage, accompanied by cervical lymph nodes with or without distant metastasis (Bossi et al., 2021; Siak et al., 2021; Xie et al., 2022). Therefore, there is an urgent need for a highly sensitive and specific molecular biomarker for the early diagnosis of disease, prognosis, and prediction of recurrence and metastasis. Despite the efforts of scientists and doctors toward this goal through technologies such as genomics, transcriptomics, proteomics and metabolomics (Zhang et al., 2021; Siak et al., 2021), no such satisfactory biomarkers are currently available.
Gene expression profiling of tumor tissue is an important resource for simulating tumor microenvironment (TME) conditions and identifying potential biomarkers. However, NPC tissues are difficult to obtain. Sometimes, the primary tumor is not visible at endoscopy (King et al., 2019). Fortunately, with the high throughput and affordable price of sequencing technology, a large amount of sequencing data based on various kinds of samples have been generated. Most of these data are shared by researchers on datasets with free access such as Gene Expression Omnibus (GEO). One of the advantages of using these public data is that we can combine homogeneous sample data from different sources. In this way, the sample size is enlarged, and correspondingly, the reliability of the conclusion is enhanced. Here, we utilized bulk RNA sequencing (bulk RNA-seq) data from GEO and single-cell RNA sequencing (scRNA-seq) results from a web portal to investigate the molecular biomarkers and TME of NPC and to explore the underlying mechanism.
Materials and methods
The design of this study is shown by a flow chart (Supplementary Figure S1).
Information on nasopharyngeal carcinoma sequencing data from Gene Expression Omnibus
We retrieved the expression profiles of NPC samples produced by high-throughput sequencing from the GEO database (http://www.ncbi.nlm.nih.gov/geo/). Then, three datasets (GSE68799, GSE118719 and GSE102349) were obtained (Table 1). Because GSE68799 and GSE118719 included both tumor and nontumor tissues, we downloaded their raw clean data to probe the differentially expressed genes (DEGs) and the hub genes. We also downloaded the normalized (CPM and TMM) data of GSE102349 submitted to GEO by researchers. Considering that GSE102349 introduced relatively complete clinical information, including disease progression, we used it for survival and diagnostic analysis.
RNA-sequencing raw data analysis
RNA sequencing raw data were downloaded by sratoolkit. Quality control was carried out by fastaqc software, a quality control tool for high-throughput sequencing data (https://www.bioinformatics.babraham.ac.uk/projects/fastqc/). The raw sequence reads were aligned to the human genome GRCh38.103 by the star aligner (star-2.7.8a) (Dobin et al., 2012). Then, the gene expression matrix of read counts was quantified by merging from ReadsPerGene text generated by star.
This work was carried out and relied on computing resources from the High-Performance Computing Center of Central South University, China (CentOS Linux release 7.5.1804).
Screening of the common differentially expressed genes
The raw read count matrix from the previous step was normalized and used to perform differential gene analysis by the R package DESeq2 (https://bioconductor.org/packages/release/bioc/html/DESeq2.html). Principal component analysis (PCA) was implemented to detect the batch effect. Genes with an adjusted p value (FDR) < 0.05 and | log2FC | > 2 were considered significant. Volcano maps of all significant genes were plotted by the easy-to-use data visualization web server ImageGP (Chen et al., 2022). The online web tool Venny 2.1 was employed to overlap the upregulated and downregulated DEGs of the two datasets.
Gene annotation and enrichment analysis of the differentially expressed genes and identification of the hub genes
Gene Ontology (GO) functional and Kyoto Encyclopedia of Genes and Genomes (KEGG) pathway analyses of the common DEGs were carried out by the R package clusterProfiler. FDR <0.05 was considered to indicate a significant difference. The GO analysis consisted of biological process (BP), cellular component (CC) and molecular function (MF).
Metascape integrates more than 40 bioinformatics databases that allow biologists to easily analyze comprehensive data through a simple one-click interface. It provides enrichment analysis of biological pathways, structure analysis of protein‒protein interaction (PPI) networks and abundant gene annotation functions (Zhou et al., 2019). The PPI network was constructed by metascape, which utilizes physical protein‒protein interactions from BioGrid as the main data source, integrating interactome datasets, including InWeb_IM and OmniPath. In addition, an MCODE identification algorithm was adopted by Metascape to automatically extract the protein complexes and obtain the core genes.
Prognostic significance analysis
The GSE102349 dataset was exploited for prognostic significance analysis in this study due to the availability of survival information. The survminer package was used to identify the recent survival analysis threshold. Then, the progression-free survival (PFS) of NPC patients was evaluated via the R package survival by the two-sided log-rank test, and p values < 0.05 were considered statistically significant.
Expression and location analysis
It was unclear what types of cells possessed the genes that could predict progression of NPC, so we applied a website portal (db.cngb.org/npcatlas) based on Chen and his colleagues’ study on NPC where researchers can analyze and visualize the single-cell data easily and for free (Chen et al., 2020). We input the gene names to identify whether they were located in epithelial cells or immune cells.
Furthermore, immunohistochemistry (IHC) was performed to validate the expression and location of PKP1 protein in ten primary NPC specimens. The study was approved by the Medical Ethics Committee of Xiangya Hospital Central South University (No: 202207389). Briefly, 4-μm formalin-fixed, paraffin-embedded (FFPE) NPC tissue sections were deparaffinized in xylene and then rehydrated in sequentially increasing dilutions of alcohol. Antigen retrieval was conducted in EGTA (pH 9.0, AR-0541, Dingguo Changsheng, Beijing, China) at 95°C using a water bath. After that, the sections were blocked with 5% BSA at 37°C for 30 min followed by incubation overnight at 4°C with rabbit anti-PKP1 antibody (1:250, ab154622, Abcam, Cambridge, MA, United States). Next, the sections were blocked using an endogenous peroxidase blocker and subsequently incubated with a mouse/rabbit enhanced polymer detection system (PV-9000, ZSGB-BIO, Beijing, China) as described in the instruction manual. After that, the sections were treated with chromogen (DAB) for 5 min at room temperature. The sections were dried and prepared for optical microscope observation.
Immune cell infiltration analysis
The outstanding platform of Immune Cell Abundance Identifier (ImmuCellAI, http://bioinfo.life.hust.edu.cn/ImmuCellAI#!/) was utilized to predict the abundance of 18 T-cell subtypes and 6 other immune cells, B cells, NK cells, dendritic cells (DCs), macrophage cells, monocyte cells and neutrophil cells, with the given gene expression data, including bulk RNA-Seq and microarray data (Miao et al., 2020). We merged the GSE68799 and GSE118719 datasets and assayed their batch effects. Then, ImmuCellAI was implemented to estimate the proportion of immune cells infiltrated in NPC tissues from this merged dataset as well as the GSE102349 dataset. Then, we compared the differences in immune cell infiltration between the NPC and nontumor samples from the merged data by the Kruskal‒Wallis test. Meanwhile, box plots were introduced to visualize the data distribution. A p value < 0.05 was considered statistically significant. Survival analysis of different cell types was also performed on the GSE102349 dataset. A p value < 0.1 was considered statistically significant.
Genes and immune cells correlation analysis
The R package ggcorrplot was adopted to disclose the relationships between the expression levels of the selected genes and the immune marker sets that constituted the first layer of the immune cells defined by ImmuCellAI, including DC cells, B cells, monocyte cells, macrophage cells, NK cells, neutrophil cells, CD4+ T cells, CD8+ T cells, NKT cells, and gamma delta + T cells (γδT). p values were determined by Spearman’s rank correlation test with a statistical significance of p < 0.05.
Results
Identification of differentially expressed genes in nasopharyngeal carcinoma
PCA showed that data from GSE68799 and GSE118719, after normalization, were mainly classified into the tumor cluster and the control cluster, indicating that they were appropriate for further analysis (Figures 1A,B). With the criteria of FDR<0.05 and | log2 FC | > 2, DEGs in the GSE68799 dataset were identified, which consisted of 3,878 upregulated and 1,117 downregulated genes, as displayed by a volcano plot in Figure 1C. In the GSE118719 dataset, 1,665 genes were found to be upregulated and 801genes were downregulated (Figure 1D). Then, we overlapped the upregulated and downregulated DEGs from the two datasets by the Venn tool and obtained 1026 upregulated and 273 downregulated genes (Figures 1E,F).
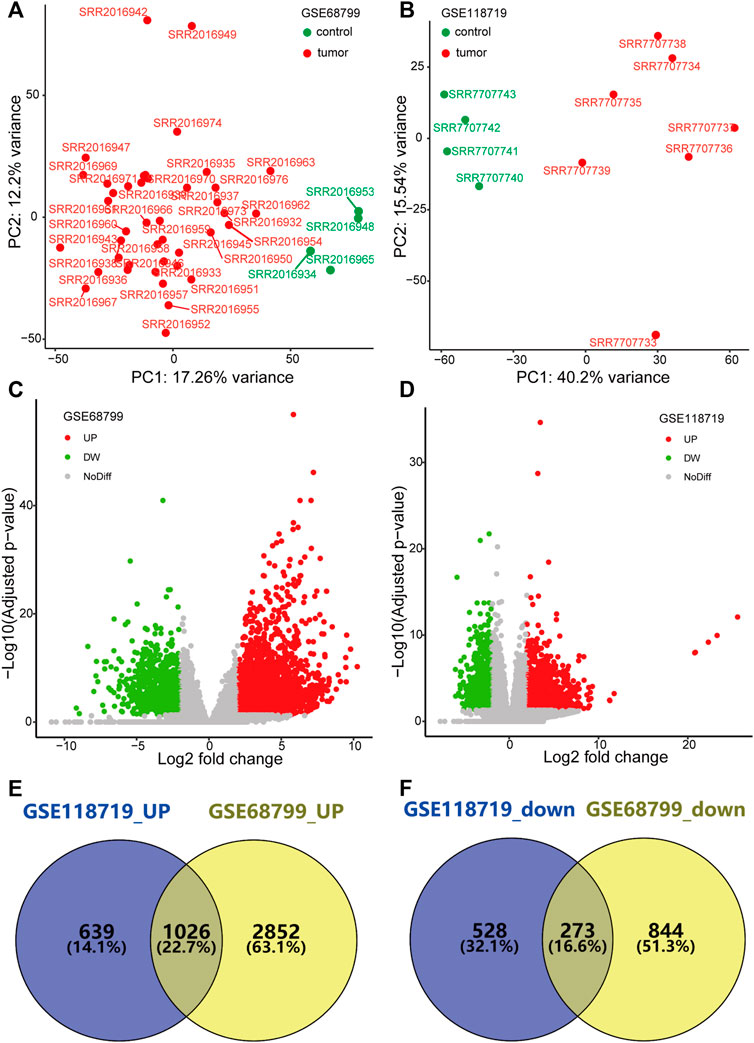
FIGURE 1. Identification of differentially expressed genes (DEGs). (A,B) PCA plots show that genes from GSE68799 and GSE118719 were mainly classified into the tumor cluster and the control cluster. (C,D) Volcano plots present the distribution of DEGs with thresholds of FDR<0.05 and |log2 FC| > 2 in GEO datasets GSE68799 and GSE118719. (E,F) Venn diagrams show the common genes of upregulated and downregulated DEGs from the two datasets.
Enrichment analysis of the differentially expressed genes and identification of the hub genes
GO analysis revealed that the upregulated DEGs relevant to BP were preferentially enriched in skin development, epidermis development, extracellular matrix organization, extracellular structure organization and hair follicle development (Figure 2A). The upregulated DEGs involved in CC were markedly enriched in cell‒cell junctions, collagen-containing extracellular matrix, keratin filaments, collagen trimers and intermediate filaments (Figure 2B). The upregulated DEGs associated with MF were predominantly clustered in extracellular matrix structural constituents, extracellular matrix structural constituents conferring tensile strength, integrin binding, cell adhesion mediator activity and cell‒cell adhesion mediator activity (Figure 2C). KEGG analysis revealed that the PI3K-Akt signaling pathway, human papillomavirus infection, MAPK signaling pathway, calcium signaling pathway, and ECM-receptor interaction were the primary enriched signaling pathways (Figure 2D).
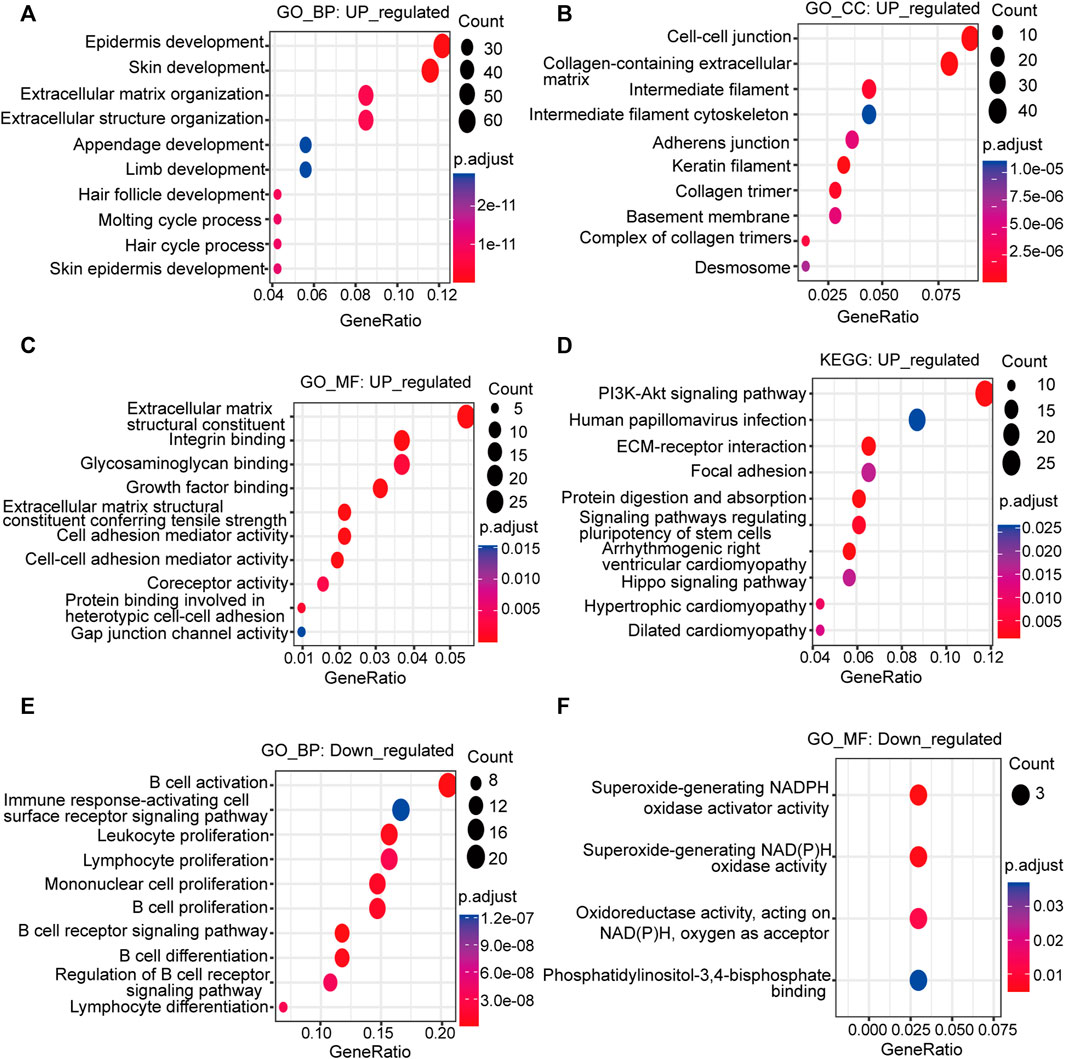
FIGURE 2. Functional analysis of the upregulated and downregulated DEGs. Bubble plots depict the GO and KEGG signaling pathway enrichment analysis. The size of the bubble represents the gene count, and the color of the dot represents the adjusted p value. Upregulated: (A) BP; (B) CC; (C) MF; (D) KEGG pathway analysis; downregulated: (E) BP; (F) MF; GO: Gene Ontology; KEGG: Kyoto Encyclopedia of Genes and Genomes; BP: biological process; CC: cellular component; MF: molecular function.
The downregulated DEGs related to BP were enriched in B-cell activation, B-cell proliferation, the B-cell receptor signaling pathway, leukocyte proliferation, lymphocyte proliferation and mononuclear cell proliferation (Figure 2E). It could be inferred from the BP of the downregulated DEGs that they were mainly concentrated in the activation and proliferation of immune cells, especially B cells. The main MF involved was NADPH oxidase activity (Figure 2F). The CC was the external side of the plasma membrane. The KEGG pathway was the B-cell receptor signaling pathway.
Construction of a protein‒protein interaction network and module analysis
The PPI network of the upregulated DEGs was constructed by the Metascape web server (Figure 3A; Supplementary Table S1). The significant modules of the DEGs were also automatically extracted by using the molecular complex detection (MCODE) identification algorithm. We then chose the first-presented module that contained most of the genes (13 genes) and 223 edges for further analysis (Figure 3B).
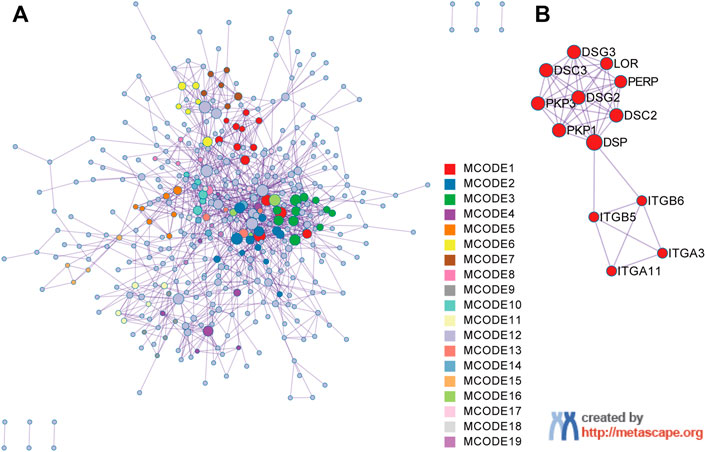
FIGURE 3. Protein‒protein interaction (PPI) network construction and MCODE component identification by Metascape. (A) PPI network constructed with the 1026 upregulated DEGs. (B) The first module identified from the PPI network using the molecular complex detection (MCODE) method with a score of 3.31. Each color represents a unique MCODE module.
Identification of prognostic markers for nasopharyngeal carcinoma
We used the calculated cut point for PFS analysis (cut points are shown in Supplementary Table S2).
The high expression of ITGA11 indicated good prognosis in NPC patients, while the high expression of PKP1, PERP, LOR and ITGB5 showed poor PFS (Figures 4A–E). Nevertheless, there was no significant correlation between DSG3, ITGA3, PKP3, DSG2, DSC2, DSC3, DSP, and ITGB6 expression and survival (Figure 4F, only shows DSG3 as representative).
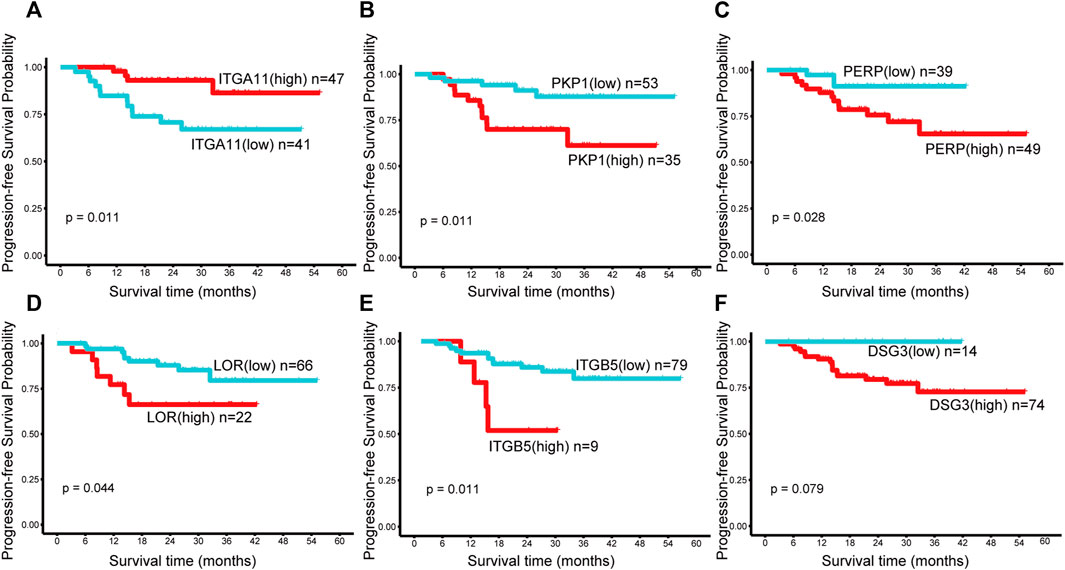
FIGURE 4. Kaplan–Meier survival curves of the genes in the first module in NPC. Progression-free survival (PFS) by high and low (A) ITGA11, (B) PKP1, (C) PERP, (D) LOR, (E) ITGB5, (F) DSG3 mRNA expression. A p value < 0.05 was considered statistically significant. The best cut-off values which were determined by the R package Survminer were selected to divide the cases into high and low expression groups.
Gene location analysis
The t-SNE cell plot displayed five cell types with each cell color coded, including B cells, cancer associated fibroblasts (CAFs), epithelial cells, myeloid cells, and T cells (Figure 5A). PKP1 was predominantly found in epithelial cells (Figure 5B). PERP was mainly distributed in epithelial cells at a relatively high level. In contrast, the expression of ITGA11, LOR, and ITGB5 was very low in each cell type. Then, we further confirmed the protein expression of PKP1. As a result, IHC validated the distribution and expression of PKP1 protein in NPC tissues. Weak to strong cytoplasmic positivity was observed mainly in NPC cells rather than in noncancerous cells (Figure 5C).
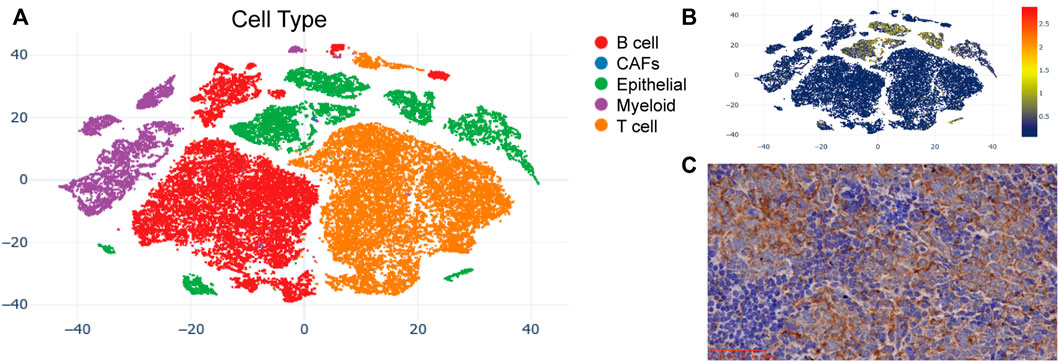
FIGURE 5. PKP1 expression analysis in NPC patients. (A) The t-SNE plots of cells with each cell color-coded to indicate the different cell types; (B) The mRNA expression of PKP1 in cells defined above; (C) Immunohistochemical staining of PKP1 protein was performed in 10 NPC tissues. A representative image is shown. Scale bars are shown in red.
Immune cell infiltration and prognostic significance analysis
Among the 24 cell types, B cells, Tfh cells, and Th17 cells achieved higher proportions in nontumor tissues than in NPC tissues. More naïve CD8+ cells, DCs, γδT cells, MAIT cells, and Th2 cells infiltrated NPC (Figure 6A). Cells infiltrated in tissues were mainly in layer 1, including DCs, B cells, monocytes, macrophages, NK cells, neutrophils, CD4+ T cells, CD8+ T cells, NKT cells, and γδT cells (Figure 6A). With respect to the extremely low proportion of the cells in layer 2 (maximum 2.4%, maximum median 1.025%), analysis was not conducted to avoid statistical errors.
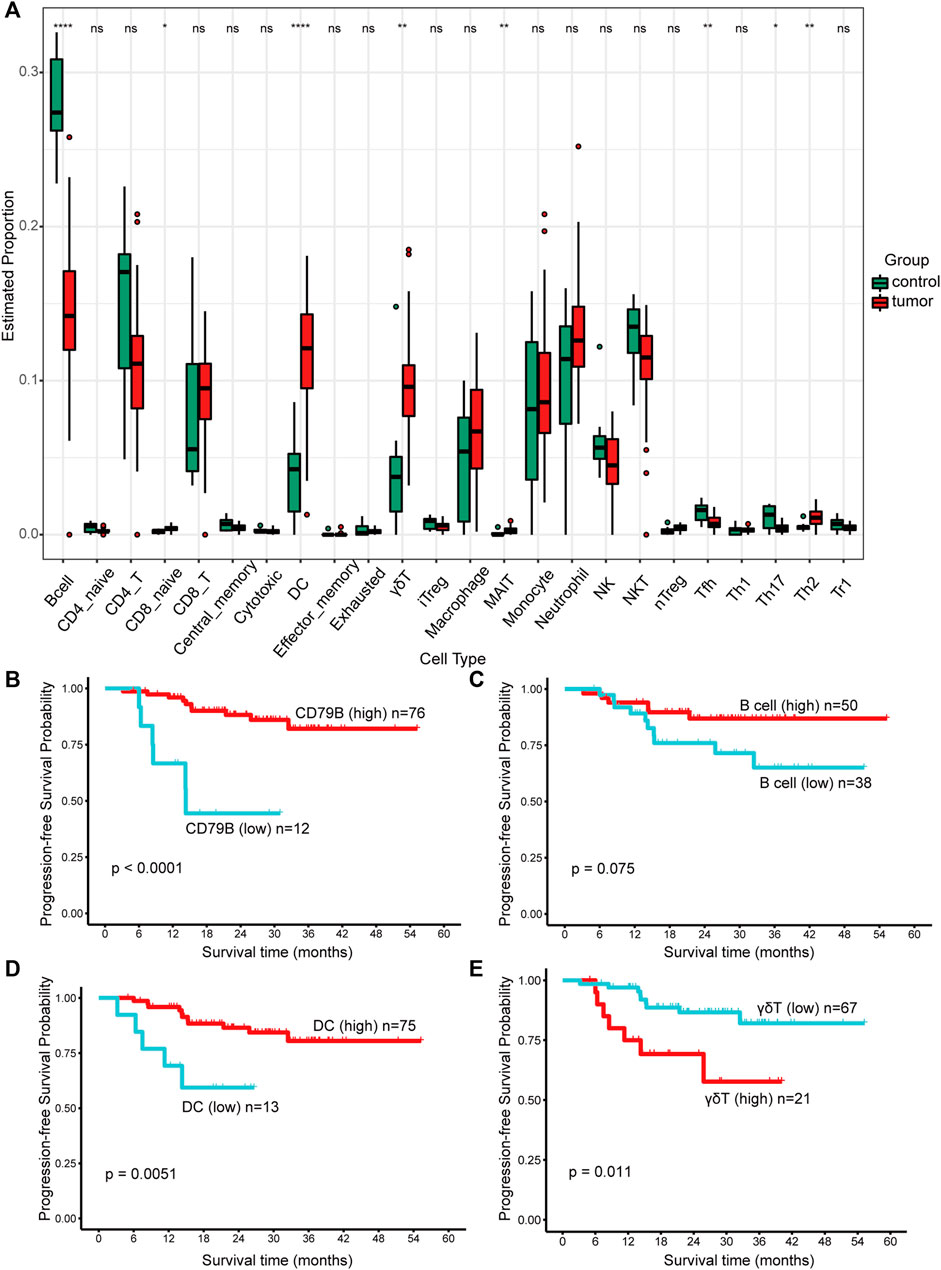
FIGURE 6. The proportions of immune cells and their prognostic significance analysis. (A) The proportions of immune cells infiltrated in control and tumor tissues. Data were analyzed using the Kruskal‒Wallis test. A p value > 0.05 was considered not statistically significant and represented as ns, while a p value ≤ 0.05 was represented as *, ≤0.01 as **, ≤0.001 as ***, and ≤0.0001 as ****. (B–E) Kaplan–Meier survival analysis of progression-free survival (PFS). The best cut-off values which were determined by the R package Survminer were selected to divide the cases into high and low expression groups. A p value < 0.1 was considered statistically significant.
Significantly, the infiltration condition of the B cells was consistent with the DEG results, in which CD79B was downregulated in NPC. Further analysis revealed that both high expression of CD79B (p < 0.0001, Figure 6B) and B cells (p = 0.075, Figure 6C) could predict favorable prognosis in NPC. Of note, a longer progression time was observed in patients with higher DC infiltration (p = 0.0051, Figure 6D). Patients with higher γδT cell infiltration were more likely to progress (p = 0.011, Figure 6E). However, monocytes, macrophages, NK cells, neutrophils, CD4+ T cells, CD8+ T cells, and NKT cells were not significantly associated with PFS (figures not shown).
Correlations between the genes and immune cells
The expression of relative PKP1 mRNA was significantly correlated with B cells (r = −0.667, p = 0), DCs (r = 0.579, p = 0), macrophages (r = 0.335, p = 0.011), γδTs (r = 0.449, p = 0), and CD4+ T cells (r = −0.402, p = 0.002) (Figures 7A–E). However, there was no significant correlation between PKP1 and CD8+ T cells, monocytes, NK cells, neutrophils, and NKT cells (Figure 7F as a representative).
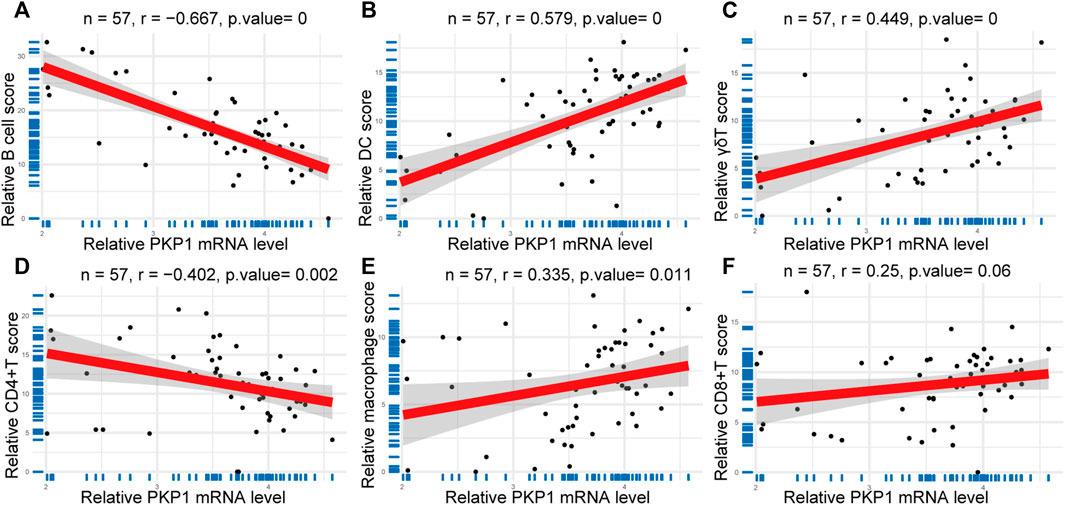
FIGURE 7. Correlations between PKP1 and immune cells. (A–E) The expression of relative PKP1 mRNA was significantly correlated with B cells, DCs, γδTs, CD4+ T cells, and macrophages. (F) A representative that the expression of relative PKP1 mRNA had no significant correlation with CD8+ T cells, monocytes, NK cells, neutrophils, and NKT cells. p values were calculated by Spearman’s rank correlation test with a statistical significance of p < 0.05.
Discussion
High-throughput bulk RNA-Seq and scRNA-Seq are revolutionary approaches to cancer research that contribute to revealing the heterogeneity between tumors (Levy and Boone, 2019; Zhang et al., 2021). scRNA-Seq analyzes a single-cell suspension, which is, on the downside, an obstacle for this technology due to the difficulty of obtaining a single-cell sample and the loss of certain cell types (Mints and Tirosh, 2020). Whereas bulk RNA-seq, just as its name implies, deals with a population of cells in bulk samples, it is incapable of uncovering the diversity of the cells within a tumor like scRNA-Seq. The sensitivity of this technology is greatly improved as the yield of cells increases, together with a boost in data generation. To make full use of these data, several excellent algorithms have been developed to estimate the proportions of the cell types in a mixed cell population based on gene expression datasets, such as bulk RNA-Seq and microarray data. Among them, ImmuCellAI, CIBERSORT, and TIMER are outstanding representatives (Newman et al., 2019; Li et al., 2020; Miao et al., 2020). NPC is characterized by massive lymphocyte infiltration, which is significantly related to the progression of the cancer and the effect of immunotherapy (Chen et al., 2020). However, the mechanism of immune cell infiltration and the genes capable of assessing prognosis and immune cell infiltration in NPC remain unknown. Our study intended to integrate bulk RNA-seq data with scRNA-Seq information to identify biomarkers and assess immune cell infiltration in NPC.
Our GO and KEGG pathway analysis results demonstrated that the upregulated DEGs were preferentially present in skin and epidermal development, which is consistent with their epithelial characteristics (Chen et al., 2019). Moreover, the results of GO and KEGG analyses of downregulated DEGs and the proportions of infiltrating immune cells also revealed that B cells were significantly less infiltrated in the NPC microenvironment than in normal tissues. The above results reflected that the tumor tissues used in this study acquired from the two gene sets GSE68799 and GSE118719, on the one hand, were of good quality, suggesting that the results obtained from these datasets would be reliable; on the other hand, the reduced infiltration of B cells was indicative of the features of the TME in NPC. Combined with the immune cell infiltration analysis, we initially depicted the TME landscape of NPC tissues, which were infiltrated with more DC and γδT cells but fewer B cells in the first layer, while in the second layer, there were more CD8 naïve cells, MAITs, and Th2 cells but fewer Tfh and Th17 cells. Furthermore, survival analysis indicated that B-cell and DC infiltration were protective factors for NPC patients, whereas infiltration of γδT cells was a risk factor.
To date, cancer immunotherapy has focused on the T-cell compartment. Although, apart from T cells, other immune subsets have not been widely studied, several reports have concluded that B cells in the TME contribute to antitumor responses (Cabrita et al., 2020; Helmink et al., 2020; Petitprez et al., 2020; Dyugay et al., 2022). In NPC patients, B cells in tertiary lymphoid structures (TLSs) could be recruited to improve survival (Li et al., 2021). In addition, NPC has a strong etiological association with EBV infection (Baloche et al., 2020; Jin et al., 2020; Tsang et al., 2020). Since B cells are the major host of EBVs, which can transmit through oral secretions and colonize epithelial cells in the oropharynx, the virus shuttles between B cells and epithelial cells (Houen and Trier, 2020). This finding reminds us that B cells are indeed important in the development of NPC. Intratumoral B cells can produce immunoglobulin G (IgG) antibodies to enhance the internalization of tumor antigens presented by DCs, and subsequently activate tumor-reactive T cells. This mechanism is believed to have potent antitumor immunity, with evidence that tumors in mice have been successfully eradicated (Carmi et al., 2015). In addition, human papillomavirus (HPV) expression has been reported to be linked to NPC (Huang et al., 2018), which is supported by our KEGG results. There is evidence of the infiltration of HPV-specific B cells in the TME of head and neck cancer, which can secrete HPV-specific IgG antibodies, contributing to antitumor immunity through indirect effects such as enhancing antigen cross-presentation or the generation and maintenance of HPV-specific T-cell responses (Wieland et al., 2021). In light of our TME landscape of NPC, we boldly speculate that tumor-infiltrating B cells in NPC produce specific antibodies and then exert antitumor effects through DCs and T cells.
Correspondingly, tumor cells struggle to resist attacks from the immune system in a variety of ways, such as the overexpression of some molecules as weapons. For example, latent membrane protein 1 (LMP1), the EBV principal oncoprotein and essential for cell malignant transformation and immortalization, induces myeloid-derived suppressor cell (MDSC) expansion to suppress immune responses (Kieser and Sterz, 2015; Cai et al., 2017). MDSCs are the major cause of tumor immunosuppression, so they come naturally to us. Many studies have proven that MDSCs are propagated in the TME of various tumors, including NPC (Li et al., 2015; Pyzer et al., 2016; Zhang et al., 2019; Yang et al., 2020). Moreover, MDSCs can restrain the proliferation of B cells and induce B-cell death by expressing iNOS and NADPH oxidase (NOX2), which are relevant to the production of nitric oxide (NO) and reactive oxygen species (ROS), respectively (Gabrilovich and Nagaraj, 2009; Raffaghello and Bianchi, 2014; Lelis et al., 2017). Furthermore, Wang et al. confirmed that MDSCs could suppress B-cell proliferation and impair B-cell responses in lung cancer (Wang et al., 2018). Interestingly, our study found that the activity of NADPH oxidase was the predominantly enriched MF of the downregulated genes and that B cells were the markedly reduced cell type. Therefore, it is reasonable for us to surmise that NPC cells can recruit MDSCs to kill B cells through the expression of molecules such as LMP or the potential biomarker PKP1, which was determined in our analysis and subsequently discussed.
PKP1 is an important accessory desmosomal plaque protein that participates in desmoglein (DSG) membranous availability (Fuchs et al., 2019). It is involved in cell growth, motility, migration, and apoptosis (Weiske et al., 2001; Sobolik-Delmaire et al., 2007; Wolf et al., 2010). PKP1 has been shown to be downregulated in lung cancer and predict favorable clinical outcomes (Haase et al., 2019). While PKP1 is rarely expressed in the nasopharynx, as reported by integrated proteomics in GeneCards: Protein expression in normal tissues and cell lines from ProteomicsDB, MaxQB, and MOPED. Indeed, our study found that it was upregulated and was predominantly present in NPC cells but not in noncancerous cells. Our analysis further revealed that the mRNA levels of PKP1 predicted poor prognosis of NPC and were positively correlated with the infiltration levels of DCs, γδTs, and macrophages and negatively correlated with B cells and CD4+ T cells in NPC.
Overall, we depicted a possible mechanism diagram as follows (Figure 8): EBV and/or HPV infect nasopharyngeal epithelial cells and then lead to NPC for undefined reasons (Figure 8A). Tumor-infiltrating lymphocyte-B cells (TIL-Bs) produce IgG to tumor antigens such as PKP1 to assist dendritic cell internalization of tumor antigens, which subsequently activates T cells in response to tumor cells; alternatively, HPV-specific IgG may be generated to enhance antigen cross-presentation or provoke and maintain the HPV-specific T-cell reaction. In response, NPC cells struggle to resist attacks from the immune system. NPC cells express proteins such as PKP1 (absent in the normal nasopharynx) to induce MDSCs expansion, which subsequently impairs the proliferation of B cells and results in B-cell death by expressing iNOS and NOX2 (Figure 8B). In summary, our results provide a potential therapeutic strategy for NPC by disrupting the interaction of PKP1 and TIL-B cells in the TME and a theoretical basis for better understanding the mechanism of NPC development.
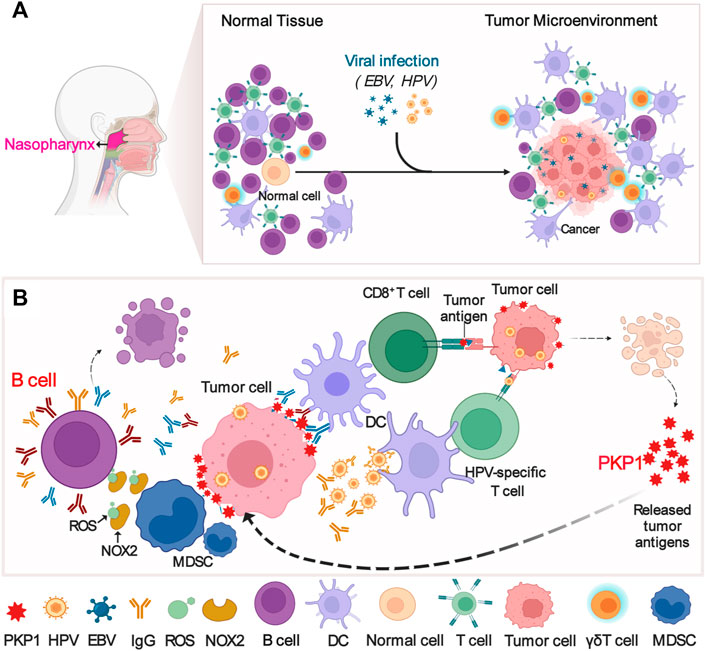
FIGURE 8. Possible mechanism diagram of the interaction between PKP1 and TIL-Bs in NPC microenvironments. (A) EBV and HPV infection results in NPC. (B) The interaction between PKP1 and TIL-Bs. TIL-Bs produce IgG to kill tumors, and NPC cells fight back. TIL-Bs: tumor-infiltrating lymphocyte-B cells. This diagram is only a proposal of the mechanism that warrants further validation. Created with BioRender.com.
Conclusion
In summary, this work indicates that PKP1 can be a candidate gene for assessing the immune infiltration levels in NPC and a potential therapeutic target for NPC. More importantly, inducing TIL-Bs in the TME might be an effective anti-NPC approach. This paper also provides a theoretical basis as well as a new perspective to help elucidate the underlying mechanism of NPC development and progression.
Data availability statement
The datasets presented in this study can be found in online repositories. The names of the repository/repositories and accession number(s) can be found in the article/Supplementary Material.
Ethics statement
The studies involving human participants were reviewed and approved by Medical Ethics Committee of Xiangya Hospital Central South University. Written informed consent for participation was not required for this study in accordance with the national legislation and the institutional requirements.
Author contributions
Y-MH designed the present study. Y-MH and L-QW wrote the manuscript. Y-MH and YL drew and organized the illustrations. Y-MH and YL performed the IHC. W-LZ confirmed the IHC results. W-LZ and F-QT revised the paper. All authors read and approved the final manuscript.
Funding
This work was supported by research funding from the Natural Science Foundation of Hunan Province, China [grant number: 2019JJ50359 and 2020JJ4780]; the Scientific Research Project of Hunan Provincial Health Commission [grant number: 20201748]; the National Natural Science Foundation of China (No. 81672688); and the Graduate education reform project of Central South University [grant number: 2021JGB080].
Acknowledgments
We thank Yibo Hu, The Third Xiangya Hospital, Central South University, China, for scientific advice on this work. We thank Jianming Zeng (University of Macau), and all the members of his bioinformatics team, biotrainee, and Dr Tong Chen, EHBIO Gene Technology (Beijing) Co., Ltd, and Biomamba and his wechat public account team, Helixlife for generously sharing their experience and codes. We thank the Hunan Key Laboratory of Oncotarget Gene at Hunan Cancer Hospital and the affiliated Cancer Hospital of Xiangya School of Medicine, Central South University, Changsha, China; and the High-Performance Computing Center at Central South University, China.
Conflict of interest
The authors declare that the research was conducted in the absence of any commercial or financial relationships that could be construed as a potential conflict of interest.
Publisher’s note
All claims expressed in this article are solely those of the authors and do not necessarily represent those of their affiliated organizations, or those of the publisher, the editors and the reviewers. Any product that may be evaluated in this article, or claim that may be made by its manufacturer, is not guaranteed or endorsed by the publisher.
Supplementary material
The Supplementary Material for this article can be found online at: https://www.frontiersin.org/articles/10.3389/fgene.2022.935749/full#supplementary-material
References
Baloche, V., Ferrand, F. R., Makowska, A., Even, C., Kontny, U., and Busson, P. (2020). Emerging therapeutic targets for nasopharyngeal carcinoma: Opportunities and challenges. Expert Opin. Ther. Targets 24 (6), 545–558. doi:10.1080/14728222.2020.1751820
Bossi, P., Chan, A. T., Licitra, L., Trama, A., Orlandi, E., Hui, E. P., et al. (2021). Nasopharyngeal carcinoma: ESMO-EURACAN clinical practice guidelines for diagnosis, treatment and follow-up(†). Ann. Oncol. 32 (4), 452–465. doi:10.1016/j.annonc.2020.12.007
Cabrita, R., Lauss, M., Sanna, A., Donia, M., Skaarup Larsen, M., Mitra, S., et al. (2020). Tertiary lymphoid structures improve immunotherapy and survival in melanoma. Nature 577 (7791), 561–565. doi:10.1038/s41586-019-1914-8
Cai, T. T., Ye, S. B., Liu, Y. N., He, J., Chen, Q. Y., Mai, H. Q., et al. (2017). LMP1-mediated glycolysis induces myeloid-derived suppressor cell expansion in nasopharyngeal carcinoma. PLoS Pathog. 13 (7), e1006503. doi:10.1371/journal.ppat.1006503
Carmi, Y., Spitzer, M. H., Linde, I. L., Burt, B. M., Prestwood, T. R., Perlman, N., et al. (2015). Allogeneic IgG combined with dendritic cell stimuli induce antitumour T-cell immunity. Nature 521 (7550), 99–104. doi:10.1038/nature14424
Chen, T., Liu, Y.-X., and Huang, L. (2022). ImageGP: An easy-to-use data visualization web server for scientific researchers. iMeta 1 (1), e5. doi:10.1002/imt2.5
Chen, Y. P., Chan, A. T. C., Le, Q. T., Blanchard, P., Sun, Y., and Ma, J. (2019). Nasopharyngeal carcinoma. Lancet 394 (10192), 64–80. doi:10.1016/s0140-6736(19)30956-0
Chen, Y. P., Yin, J. H., Li, W. F., Li, H. J., Chen, D. P., Zhang, C. J., et al. (2020). Single-cell transcriptomics reveals regulators underlying immune cell diversity and immune subtypes associated with prognosis in nasopharyngeal carcinoma. Cell Res. 30 (11), 1024–1042. doi:10.1038/s41422-020-0374-x
Dobin, A., Davis, C. A., Schlesinger, F., Drenkow, J., Zaleski, C., Jha, S., et al. (2012). Star: Ultrafast universal RNA-seq aligner. Bioinformatics 29 (1), 15–21. doi:10.1093/bioinformatics/bts635
Dyugay, I. A., Lukyanov, D. K., Turchaninova, M. A., Serebrovskaya, E. O., Bryushkova, E. A., Zaretsky, A. R., et al. (2022). Accounting for B-cell behavior and sampling bias predicts anti-PD-L1 response in bladder cancer. Cancer Immunol. Res. 10 (3), 343–353. doi:10.1158/2326-6066.CIR-21-0489
Fuchs, M., Foresti, M., Radeva, M. Y., Kugelmann, D., Keil, R., Hatzfeld, M., et al. (2019). Plakophilin 1 but not plakophilin 3 regulates desmoglein clustering. Cell. Mol. Life Sci. 76 (17), 3465–3476. doi:10.1007/s00018-019-03083-8
Gabrilovich, D. I., and Nagaraj, S. (2009). Myeloid-derived suppressor cells as regulators of the immune system. Nat. Rev. Immunol. 9 (3), 162–174. doi:10.1038/nri2506
Haase, D., Cui, T., Yang, L., Ma, Y., Liu, H., Theis, B., et al. (2019). Plakophilin 1 is methylated and has a tumor suppressive activity in human lung cancer. Exp. Mol. Pathol. 108, 73–79. doi:10.1016/j.yexmp.2019.04.001
Helmink, B. A., Reddy, S. M., Gao, J., Zhang, S., Basar, R., Thakur, R., et al. (2020). B cells and tertiary lymphoid structures promote immunotherapy response. Nature 577 (7791), 549–555. doi:10.1038/s41586-019-1922-8
Houen, G., and Trier, N. H. (2020). Epstein-barr virus and systemic autoimmune diseases. Front. Immunol. 11, 587380. doi:10.3389/fimmu.2020.587380
Huang, W. B., Chan, J. Y. W., and Liu, D. L. (2018). Human papillomavirus and World Health Organization type III nasopharyngeal carcinoma: Multicenter study from an endemic area in Southern China. Cancer 124 (3), 530–536. doi:10.1002/cncr.31031
Jin, S., Li, R., Chen, M. Y., Yu, C., Tang, L. Q., Liu, Y. M., et al. (2020). Single-cell transcriptomic analysis defines the interplay between tumor cells, viral infection, and the microenvironment in nasopharyngeal carcinoma. Cell Res. 30 (11), 950–965. doi:10.1038/s41422-020-00402-8
Kieser, A., and Sterz, K. R. (2015). The latent membrane protein 1 (LMP1). Curr. Top. Microbiol. Immunol. 391, 119–149. doi:10.1007/978-3-319-22834-1_4
King, A. D., Woo, J. K. S., Ai, Q. Y., Chan, J. S. M., Lam, W. K. J., Tse, I. O. L., et al. (2019). Complementary roles of MRI and endoscopic examination in the early detection of nasopharyngeal carcinoma. Ann. Oncol. 30 (6), 977–982. doi:10.1093/annonc/mdz106
Lelis, F. J. N., Jaufmann, J., Singh, A., Fromm, K., Teschner, A. C., Pöschel, S., et al. (2017). Myeloid-derived suppressor cells modulate B-cell responses. Immunol. Lett. 188, 108–115. doi:10.1016/j.imlet.2017.07.003
Levy, S. E., and Boone, B. E. (2019). Next-generation sequencing strategies. Cold Spring Harb. Perspect. Med. 9 (7), a025791. doi:10.1101/cshperspect.a025791
Li, J. P., Wu, C. Y., Chen, M. Y., Liu, S. X., Yan, S. M., Kang, Y. F., et al. (2021). PD-1(+)CXCR5(-)CD4(+) Th-CXCL13 cell subset drives B cells into tertiary lymphoid structures of nasopharyngeal carcinoma. J. Immunother. Cancer 9 (7), e002101. doi:10.1136/jitc-2020-002101
Li, T., Fu, J., Zeng, Z., Cohen, D., Li, J., Chen, Q., et al. (2020). TIMER2.0 for analysis of tumor-infiltrating immune cells. Nucleic Acids Res. 48 (W1), W509–w514. doi:10.1093/nar/gkaa407
Li, Z. L., Ye, S. B., OuYang, L. Y., Zhang, H., Chen, Y. S., He, J., et al. (2015). COX-2 promotes metastasis in nasopharyngeal carcinoma by mediating interactions between cancer cells and myeloid-derived suppressor cells. Oncoimmunology 4 (11), e1044712. doi:10.1080/2162402x.2015.1044712
Miao, Y.-R., Zhang, Q., Lei, Q., Luo, M., Xie, G.-Y., Wang, H., et al. (2020). ImmuCellAI: A unique method for comprehensive T-cell subsets abundance prediction and its application in cancer immunotherapy. Adv. Sci. 7 (7), 1902880. doi:10.1002/advs.201902880
Mints, M., and Tirosh, I. (2020). Nasopharyngeal carcinoma joins the single-cell party. Cancer Commun. 40 (9), 453–455. doi:10.1002/cac2.12091
Newman, A. M., Steen, C. B., Liu, C. L., Gentles, A. J., Chaudhuri, A. A., Scherer, F., et al. (2019). Determining cell type abundance and expression from bulk tissues with digital cytometry. Nat. Biotechnol. 37 (7), 773–782. doi:10.1038/s41587-019-0114-2
Petitprez, F., de Reyniès, A., Keung, E. Z., Chen, T. W.-W., Sun, C.-M., Calderaro, J., et al. (2020). B cells are associated with survival and immunotherapy response in sarcoma. Nature 577 (7791), 556–560. doi:10.1038/s41586-019-1906-8
Pyzer, A. R., Cole, L., Rosenblatt, J., and Avigan, D. E. (2016). Myeloid-derived suppressor cells as effectors of immune suppression in cancer. Int. J. Cancer 139 (9), 1915–1926. doi:10.1002/ijc.30232
Raffaghello, L., and Bianchi, G. (2014). “Myeloid-derived suppressor cells and tumor growth,” in Interaction of immune and cancer cells. Editor M. Klink (Poland: Springer), 91
Siak, P. Y., Khoo, A. S., Leong, C. O., Hoh, B. P., and Cheah, S. C. (2021). Current status and future perspectives about molecular biomarkers of nasopharyngeal carcinoma. Cancers (Basel) 13 (14), 3490. doi:10.3390/cancers13143490
Sobolik-Delmaire, T., Katafiasz, D., Keim, S. A., Mahoney, M. G., and Wahl, J. K. (2007). Decreased plakophilin-1 expression promotes increased motility in head and neck squamous cell carcinoma cells. Cell Commun. Adhes. 14 (2-3), 99–109. doi:10.1080/15419060701463082
Tsang, C. M., Lui, V. W. Y., Bruce, J. P., Pugh, T. J., and Lo, K. W. (2020). Translational genomics of nasopharyngeal cancer. Semin. Cancer Biol. 61, 84–100. doi:10.1016/j.semcancer.2019.09.006
Wang, Y., Schafer, C. C., Hough, K. P., Tousif, S., Duncan, S. R., Kearney, J. F., et al. (2018). Myeloid-derived suppressor cells impair B cell responses in lung cancer through IL-7 and STAT5. J. Immunol. 201 (1), 278–295. doi:10.4049/jimmunol.1701069
Weiske, J., Schöneberg, T., Schröder, W., Hatzfeld, M., Tauber, R., and Huber, O. (2001). The fate of desmosomal proteins in apoptotic cells. J. Biol. Chem. 276 (44), 41175–41181. doi:10.1074/jbc.M105769200
Wieland, A., Patel, M. R., Cardenas, M. A., Eberhardt, C. S., Hudson, W. H., Obeng, R. C., et al. (2021). Defining HPV-specific B cell responses in patients with head and neck cancer. Nature 597 (7875), 274–278. doi:10.1038/s41586-020-2931-3
Wolf, A., Krause-Gruszczynska, M., Birkenmeier, O., Ostareck-Lederer, A., Hüttelmaier, S., and Hatzfeld, M. (2010). Plakophilin 1 stimulates translation by promoting eIF4A1 activity. J. Cell Biol. 188 (4), 463–471. doi:10.1083/jcb.200908135
Wong, K. C. W., Hui, E. P., Lo, K.-W., Lam, W. K. J., Johnson, D., Li, L., et al. (2021). Nasopharyngeal carcinoma: An evolving paradigm. Nat. Rev. Clin. Oncol. 18 (11), 679–695. doi:10.1038/s41571-021-00524-x
Xie, S.-H., Huang, R.-Q., Liu, Y.-L., Cao, S.-M., and Qian, C.-N. (2022). An increase in early cancer detection rates at a single cancer center: Experiences from Sun Yat-sen University Cancer Center. Vis. Cancer Med. 3, 1. doi:10.1051/vcm/2022001
Yang, Y., Li, C., Liu, T., Dai, X., and Bazhin, A. V. (2020). Myeloid-derived suppressor cells in tumors: From mechanisms to antigen specificity and microenvironmental regulation. Front. Immunol. 11, 1371. doi:10.3389/fimmu.2020.01371
Zhang, C. X., Ye, S. B., Ni, J. J., Cai, T. T., Liu, Y. N., Huang, D. J., et al. (2019). STING signaling remodels the tumor microenvironment by antagonizing myeloid-derived suppressor cell expansion. Cell Death Differ. 26 (11), 2314–2328. doi:10.1038/s41418-019-0302-0
Zhang, S. Q., Pan, S. M., Liang, S. X., Han, Y. S., Chen, H. B., and Li, J. C. (2021a). Research status and prospects of biomarkers for nasopharyngeal carcinoma in the era of high-throughput omics (Review). Int. J. Oncol. 58 (4), 9. doi:10.3892/ijo.2021.5188
Zhang, Y., Wang, D., Peng, M., Tang, L., Ouyang, J., Xiong, F., et al. (2021b). Single-cell RNA sequencing in cancer research. J. Exp. Clin. Cancer Res. 40 (1), 81. doi:10.1186/s13046-021-01874-1
Keywords: PKP1, tumor-infiltrating lymphocyte-B cells, bulk RNA sequencing, single-cell RNA sequencing, nasopharyngeal carcinoma
Citation: Huang Y-M, Wang L-Q, Liu Y, Tang F-Q and Zhang W-L (2022) Integrated analysis of bulk and single-cell RNA sequencing reveals the interaction of PKP1 and tumor-infiltrating B cells and their therapeutic potential for nasopharyngeal carcinoma. Front. Genet. 13:935749. doi: 10.3389/fgene.2022.935749
Received: 04 May 2022; Accepted: 23 August 2022;
Published: 14 September 2022.
Edited by:
Guoxian Yu, Shandong University, ChinaReviewed by:
Chao-Nan Qian, Guangzhou Concord Cancer Center, ChinaJin-Xing Liu, School of Computer Science, China
Li Xun, Lanzhou University Medical College, China
Copyright © 2022 Huang, Wang, Liu, Tang and Zhang. This is an open-access article distributed under the terms of the Creative Commons Attribution License (CC BY). The use, distribution or reproduction in other forums is permitted, provided the original author(s) and the copyright owner(s) are credited and that the original publication in this journal is cited, in accordance with accepted academic practice. No use, distribution or reproduction is permitted which does not comply with these terms.
*Correspondence: Wen-Ling Zhang, emhhbmd3ZW5saW5nNzNAMTI2LmNvbQ==