- School of Science, Shanghai Institute of Technology, Shanghai, China
Colon cancer (CC) is a common malignant tumor worldwide, and ferroptosis plays a vital role in the pathology and progression of CC. Effective prognostic tools are required to guide clinical decision-making in CC. In our study, gene expression and clinical data of CC were downloaded from The Cancer Genome Atlas (TCGA) and Gene Expression Omnibus (GEO) databases. We identified the differentially expressed ferroptosis-related lncRNAs using the differential expression and gene co-expression analysis. Then, univariate and multivariate Cox regression analyses were used to identify the effective ferroptosis-related lncRNAs for constructing the prognostic model for CC. Gene set enrichment analysis (GSEA) was conducted to explore the functional enrichment analysis. CIBERSORT and single-sample GSEA were performed to investigate the association between our model and the immune microenvironment. Finally, three ferroptosis-related lncRNAs (XXbac-B476C20.9, TP73-AS1, and SNHG15) were identified to construct the prognostic model. The results of the validation showed that our model was effective in predicting the prognosis of CC patients, which also was an independent prognostic factor for CC. The GSEA analysis showed that several ferroptosis-related pathways were significantly enriched in the low-risk group. Immune infiltration analysis suggested that the level of immune cell infiltration was significantly higher in the high-risk group than that in the low-risk group. In summary, we established a prognostic model based on the ferroptosis-related lncRNAs, which could provide clinical guidance for future laboratory and clinical research on CC.
Introduction
Colon cancer (CC) has the third most incidences among malignancies, and it is the second most common cause of cancer death in men and women combined (Siegel et al., 2022). The malignant transformation of CC is a multistep process that takes approximately ten years from small clumps to CC (Jemal et al., 2011). Therefore, early diagnosis is essential for improving the prognosis of CC patients. However, the survival of CC patients is poor because of the complexity of the disease, late disease detection, and lack of reliable risk-assessment biomarkers (Lin et al., 2020; Yang C. et al., 2021). Even after treatment, the risk of recurrence and metastasis in CC patients is still high (Chang et al., 2020; Jin et al., 2020). In recent years, more studies have suggested that it is promising to solve the problem by integrating computational techniques with big biomedical data involving multiple types of biomarkers including epigenetic, genetic, and gene expression profiles (Yang Y. et al., 2021; Liu et al., 2021). Therefore, identifying effective biomarkers to establish a prognostic model for survival prediction is gaining increasing attention.
lncRNAs are non-protein coding transcripts over 200 nucleotides in length (Mercer et al., 2009). There are more than 50,000 lncRNA genes annotated in the human genome (Borkiewicz et al., 2021). Studies have shown that lncRNAs are often dysregulated during tumorigenesis, which might cause tumor development (Prensner and Chinnaiyan, 2011; Schmitt and Chang, 2016). Therefore, they are used as molecular biomarkers to diagnose and treat many diseases, including CC. For example, Zhou et al. (2019) revealed that lncRNA XIRP2-AS1 has a favorable impact on the overall survival of patients with colon cancer. Tsai et al. (2018) found that lncRNA Linc00659 expression knockdown could accelerate cell apoptosis in CC cells treated with chemotherapy drugs.
Ferroptosis is a newly discovered form of programmed cell death characterized by iron-dependent accumulation of lethal lipid peroxidation (Tang et al., 2018; Mou et al., 2019). Cancer cells are vulnerable to ferroptosis because of their high iron uptake to support fast proliferation (Hassannia et al., 2019). Recently, studies have demonstrated that ferroptosis plays a crucial role in tumorigenesis and cancer therapeutics. Wang et al. (2021) constructed a ferroptosis-related prognostic signature for LUAD and suggested that ferroptosis is a functional and therapeutic target in LUAD. He et al. (2021) have constructed a prognostic risk model based on 10 genes related to ferroptosis and identified potential novel therapeutic targets which improve the individualized treatment of patients with HNSCC. Moreover, considering the critical role of ferroptosis in cancer, many studies proposed ferroptosis-based strategies to identify potential lncRNA biomarkers associated with various cancers. For example, Guo et al. (2021) revealed that ferroptosis-related lncRNAs have the potential to inform immunological research and treatment. Wei et al. (2021) identified that ferroptosis-related lncRNAs have an important prognostic value in gastric cancer. Feng et al. (2022) suggested that ferroptosis and iron metabolism–related lncRNAs can independently predict the overall survival and therapeutic effect in patients with ovarian cancer. Currently, many prognostic models have been proposed based on the ferroptosis-related lncRNAs for colon cancer (Cai et al., 2021; Zhang et al., 2021). However, the functional mechanisms of the ferroptosis-related lncRNAs and the relationship between the prognostic model and the tumor immune microenvironment require further investigation for CC patients.
In this study, three ferroptosis-related lncRNAs were identified as the prognostic biomarkers for CC. The prognostic model based on the ferroptosis-related lncRNAs was constructed for predicting the overall survival of CC patients, which would provide prognostic insights into anticancer therapies and a novel source for immune therapies. The workflow of this study is shown in Figure 1.
Materials and Methods
Data Collection
In this study, we selected four independent datasets from two different high-throughput platforms, including 458 colon adenocarcinoma (COAD) samples and 41 normal samples from TCGA (https://portal.gdc.cancer.gov/); 111 CC samples, 34 normal samples (GSE20916), 124 colorectal cancer samples (GSE72970), and 177 CC samples (GSE17536) from the GEO (https://www.ncbi.nlm.nih.gov/geo/). The gene expression profiling of the three datasets (GSE20916, GSE72970, and GSE17536) was based on the GPL570 platform. Patients with a survival time of more than 30 days were used for the survival analysis. The detailed clinical characteristics of the patients are shown in Table 1. We downloaded 259 ferroptosis-related genes from the FerrDb database (Zhou and Bao, 2020), including 108 driver genes, 69 suppressor genes, and 111 marker genes (Supplementary Table S1).
Identification of Differentially Expressed Ferroptosis-Related lncRNAs
In this study, we identified mRNAs and lncRNAs using the Ensembl database (http://ensemblgenomes.org). The expression profile of mRNAs and lncRNAs was extracted from RNA-seq count data, which was normalized using the edgeR package (version 3.32.1). Differentially expressed mRNAs and lncRNAs shared by TCGA-COAD and GSE20916 were identified using the edgeR and limma R packages [
Construction of a DEFR-lncRNA Prognostic Model
Univariate Cox regression analysis was first performed by integrating the gene expression matrix of the DEFR-lncRNAs and the survival data in TCGA-COAD to identify the DEFR-lncRNAs with prognostic relevance for the overall survival (OS). Statistically significant value was set at
where
Enrichment Analysis
Gene set enrichment analysis (GSEA) (http://www.broad.mit.edu/gsea/) is a computational method used to identify whether a pre-defined set of genes shows significant differences between two biological states (Subramanian et al., 2005). GSEA was performed by GSEA software (version 4.2.3). The Kyoto Encyclopedia of Genes and Genomes (KEGG) pathway and Hallmark pathways were used to explore the potential pathways and gene sets associated with the model. They were visualized using the ggplot2 R package.
Immunity Analysis
CIBERSORT (https://cibersort.stanford.edu/) is an established computational resource to estimate the abundance of member cell types in a mixed cell population (Newman et al., 2015). In our study, we applied the CIBERSORT algorithm to assess the tumor infiltration levels of 22 immune cell types from the CC patients in TCGA-COAD. It was run using the LM22 signature with 1,000 permutations to estimate the relative fractions of the 22 immune cell types. Moreover, the single-sample gene set enrichment analysis (ssGSEA) was also performed, and 28 immune cell types that are over-represented in the tumor microenvironment were analyzed to understand the association between the prognostic model and immune infiltration (Charoentong et al., 2017).
Statistical Analysis
All statistical analyses were conducted by R software (Version 4.0.2). Univariate Cox regression analysis, LASSO regression analysis, and multivariate Cox regression analysis were performed to identify the DEFR-lncRNAs associated with the prognosis of CC patients. The Kaplan–Meier survival analysis and log-rank test were used to conduct survival analysis. The timeROC R package was used to draw receiver operating characteristic (ROC) curves and quantify the area under the curve (AUC) values. The GSVA R package was used for the ssGSEA.
Results
Identification of Differentially Expressed Ferroptosis-Related lncRNAs
In our study, using the gene type data reported for the genome GRCh38.p13, 19,674 mRNAs and 14,826 lncRNAs were downloaded from TCGA-COAD, and 12,001 mRNAs and 370 lncRNAs were downloaded from GSE20916. The differential expression analysis showed that 4,876 mRNAs and 1,671 lncRNAs were differentially expressed in TCGA-COAD, and 1,370 mRNAs and 44 lncRNAs were differentially expressed in GSE20916. The volcano plots of DEmRNAs and DElncRNAs of TCGA-COAD and GSE20916 are shown in Figures 2A,B, respectively. Moreover, 1,157 DEmRNAs and 34 DElncRNAs shared by the two databases were obtained (Figure 2C). Then, 30 DEFR-mRNAs were obtained after intersecting 1,157 DEmRNAs and 259 ferroptosis-related genes (Figure 2D). Finally, 29 DEFR-lncRNAs were identified using the co-expression analysis, which was shown in the co-expression network (Figure 2E).
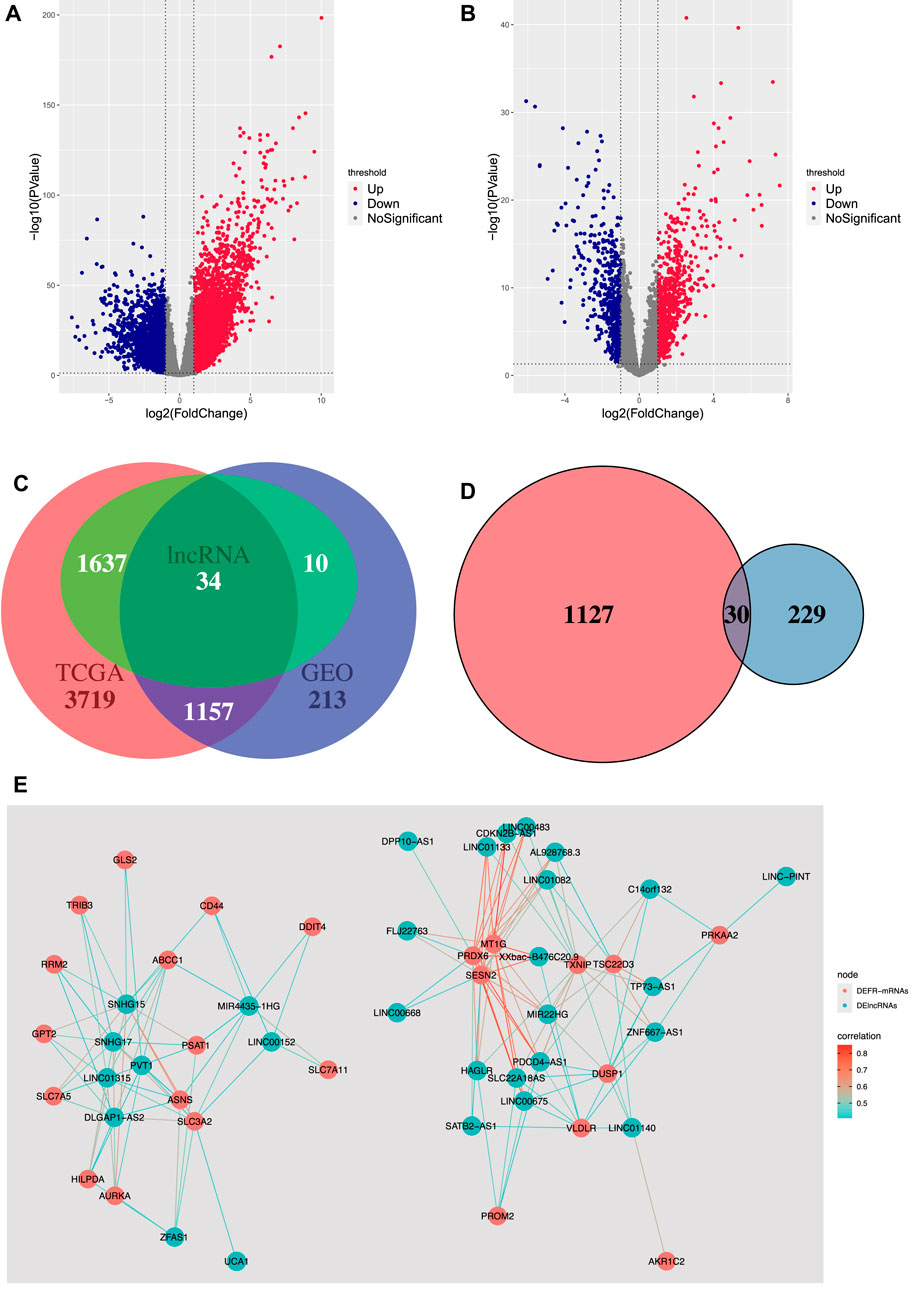
FIGURE 2. Identification of DEFR-lncRNAs. (A) Volcano plot of DEmRNAs and DElncRNAs in TCGA-COAD. (B) Volcano plot of DEmRNAs and DElncRNAs in GSE20916. (C) Venn diagram of DEmRNAs and DElncRNAs in TCGA-COAD and GSE20916. (D) Venn diagram of the shared DEmRNAs and ferroptosis-related genes. Red represents the shared DEmRNAs between TCGA-COAD and GSE20916, and blue represents the ferroptosis-related genes. (E) Co-expression network between DEFR-mRNAs and DElncRNAs.
Construction of a Prognostic Model Based on DEFR-lncRNAs
Based on the 29 DEFR-lncRNAs, we identified five DEFR-lncRNAs (SNHG17, XXbac-B476C20.9, TP73-AS1, SNHG15, and PVT1) that were statistically related to the OS of CC patients using the univariate Cox regression analysis (
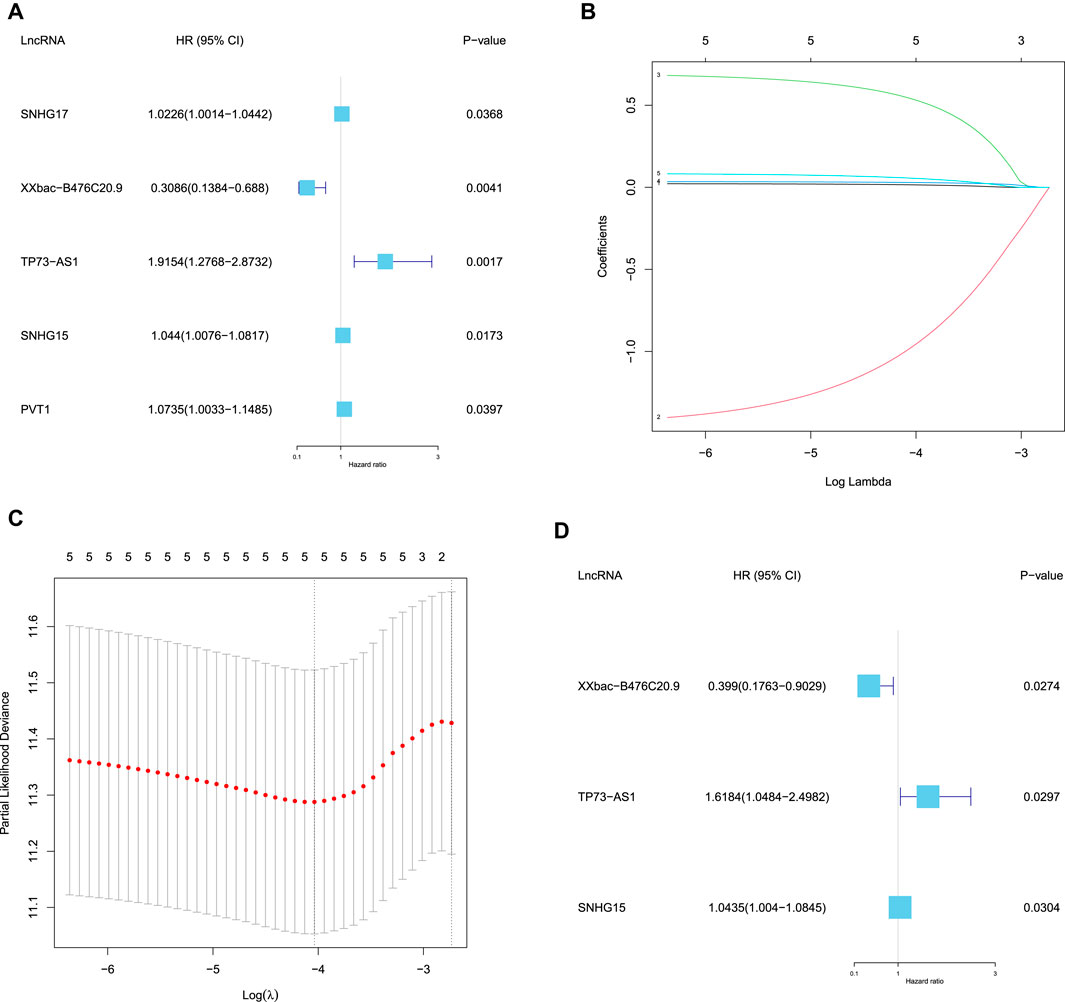
FIGURE 3. Identification of the prognostic DEFR-lncRNAs. (A) Forest map of five DEFR-lncRNAs identified by univariate Cox regression analysis. (B) LASSO coefficient profile of the five DEFR-lncRNAs. (C) Plots of the cross-validation error rates. (D) Forest map of three DEFR-lncRNAs identified by the multivariate Cox regression analysis.
After filtering patients with incomplete gene expression data and clinical information, 370 patients in TCGA-COAD remained in our study, who were divided randomly into the training set and the test set in a 1:1 ratio. The prognostic model was constructed based on the three prognostic DEFR-lncRNAs in the training set. The RS was calculated for each patient using the following equation:
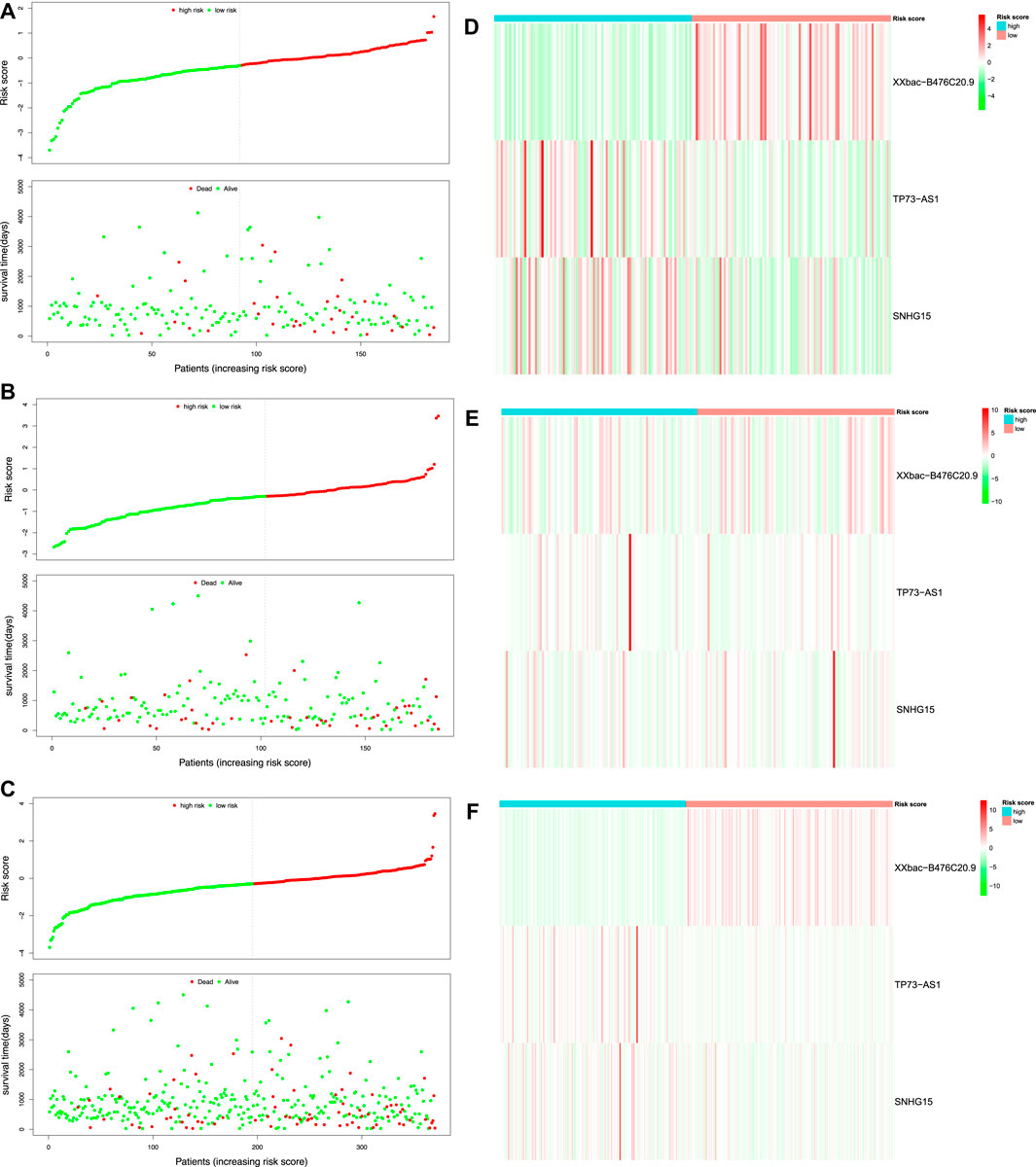
FIGURE 4. Risk score analysis of the prognostic model in TCGA-COAD. Risk score distribution and survival status of the patients in the training set (A), test set (B), and whole set (C). Expression heatmap of three DEFR-lncRNAs in the training set (D), test set (E), and whole set (F).
Kaplan–Meier survival curves were plotted to compare the difference in the OS between the high-risk and low-risk groups, which indicated that the patients in the low-risk group had better OS than those in the high-risk group in the training, test, and whole sets (Figures 5A–C). Moreover, time-dependent ROC curves were plotted to assess the sensitivity and specificity of the 1-, 3-, and 5-year survival predictions of CC patients using the timeROC R package. In the training set, the AUCs used for 1-, 3-, and 5-year OS predictions were 0.72, 0.69, and 0.73, respectively (Figure 5D). In the test set, the AUCs used for 1-, 3-, and 5-year OS predictions were 0.63, 0.6, and 0.66, respectively (Figure 5E). In the whole set, the AUCs used for 1-, 3-, and 5-year OS predictions were 0.64, 0.63, and 0.66, respectively (Figure 5F).
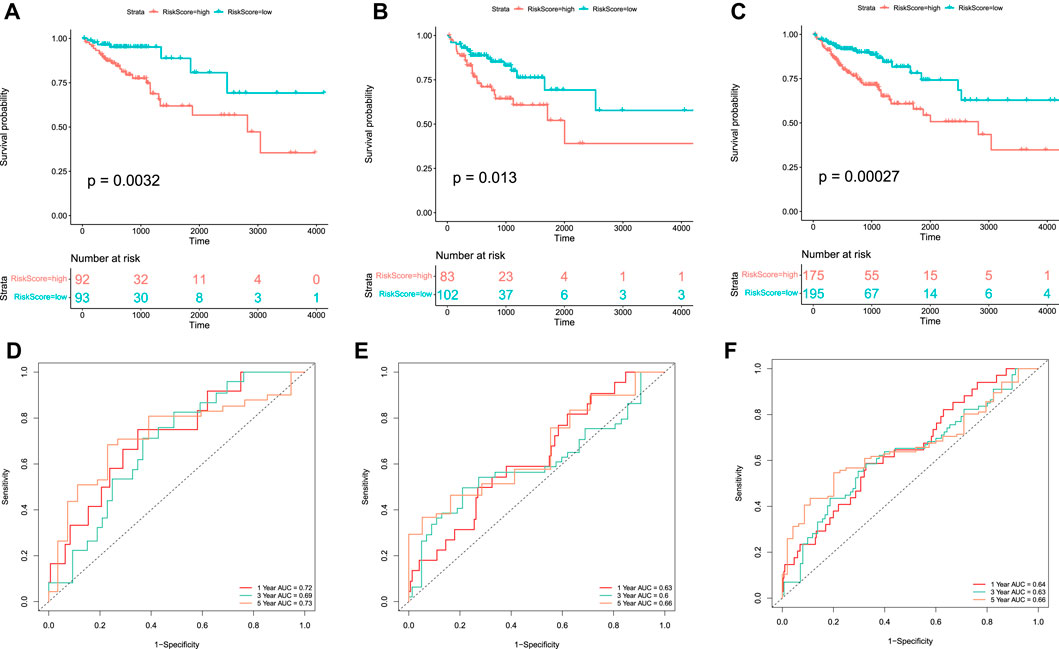
FIGURE 5. Kaplan–Meier curve and ROC curve of the model in TCGA-COAD. Kaplan–Meier curves of the OS of patients between high-risk and low-risk groups in the training set (A), test set (B), and whole set (C). Time-dependent ROC curve analysis of the RS in the training set (D), test set (E), and whole set (F).
Furthermore, the univariate and multivariate Cox regression analyses were performed to validate the independent predictive power of the prognostic RS model for CC patients in the training, test, and whole sets, and the variables (age, gender, T stage, N stage, M stage, AJCC stage, and RS) were used as the possible risk factors. These results revealed that the prognostic model proposed in our study can be used as an independent prognostic factor for CC patients (Supplementary Table S2). In the whole set, we found that age, M stage, AJCC stage, and RS were the independent risk factors for CC patients (
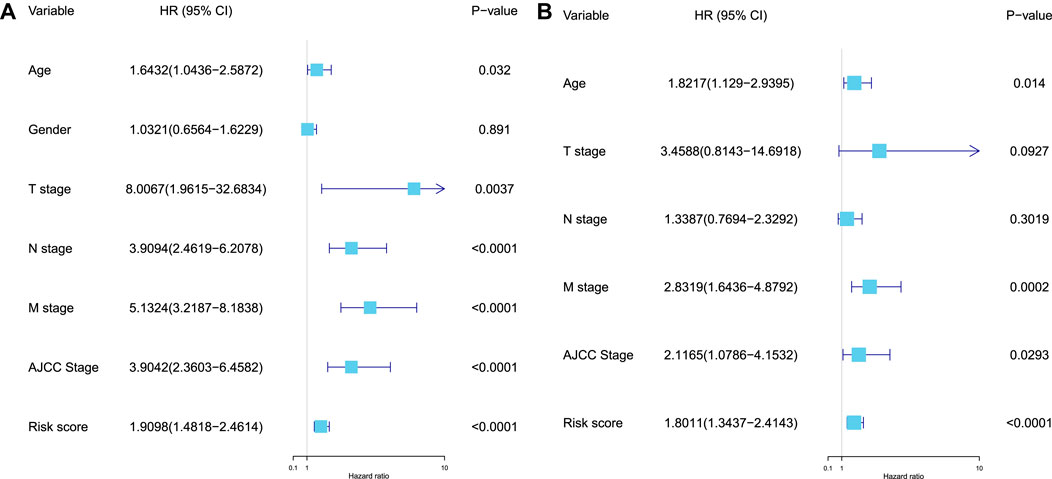
FIGURE 6. Validation of the independent predictive power of the model. (A) Univariate Cox regression analysis of the prognostic variables in the whole set. (B) Multivariate Cox regression analysis of the prognostic variables (age, T stage, N stage, M stage, AJCC stage, and RS) in the whole set.
Verification of the Prognostic Model in the Validation Set
We merged GSE72970 and GSE17536 to form the validation set, which contained 301 tumor samples. We calculated the RS of each patient in the validation set based on the formula of the prognostic RS model. The patients in the validation set were classified into high-risk (n = 136) and low-risk groups (n = 165) according to the optimal cut-off value (
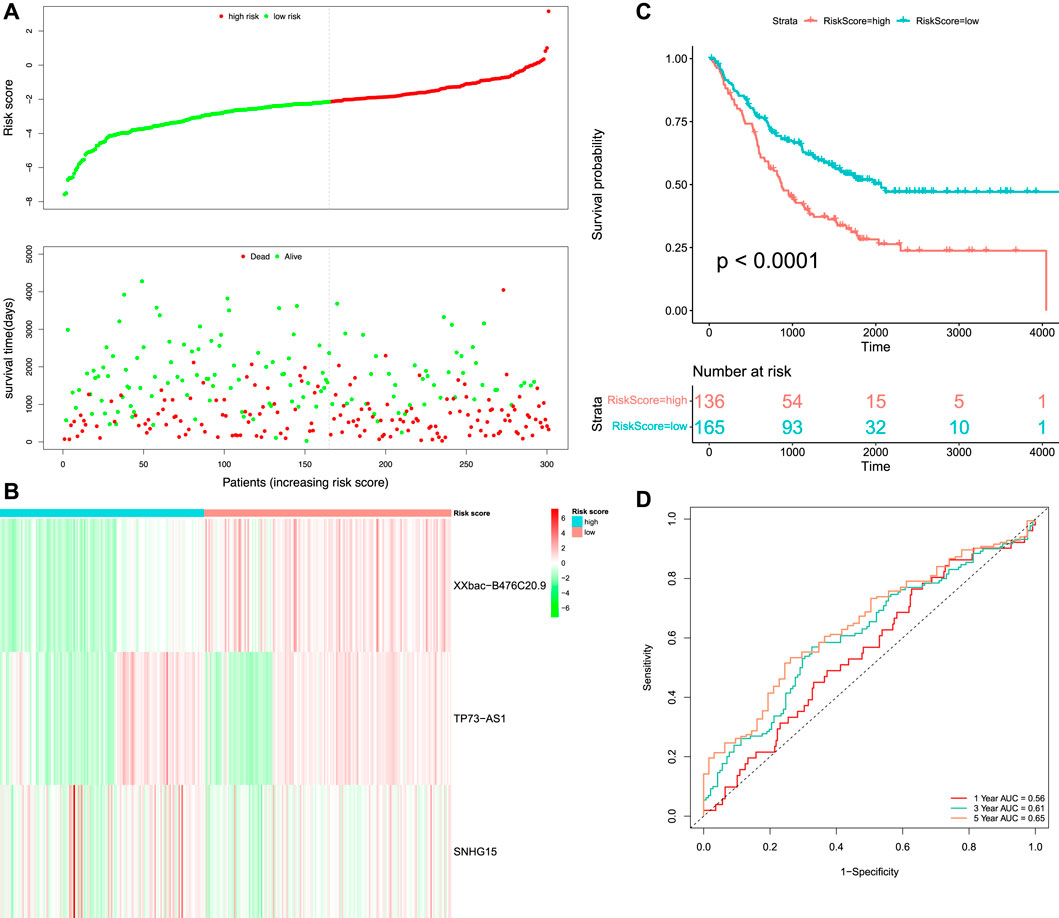
FIGURE 7. Survival and time-dependent ROC curve analysis of the prognostic model in the validation set. (A) Risk score distribution and survival status of the patients. (B) Heatmap of three DEFR-lncRNAs. (C) Kaplan–Meier curve analysis of the OS between high-risk and low-risk groups. (D) Time-dependent ROC curve analysis of the RS.
Functional Enrichment Analysis
The GSEA was performed to investigate the potential pathways and functions connected with high-risk and low-risk groups, and the terms
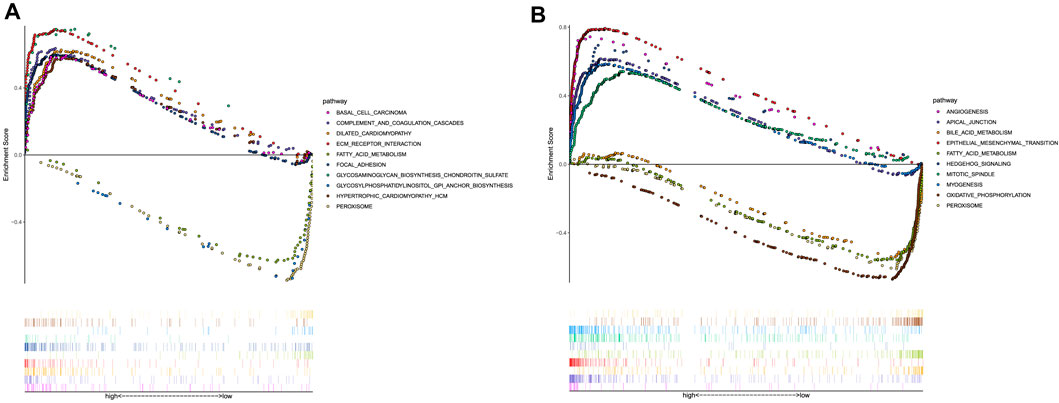
FIGURE 8. Functional enrichment analysis. (A) KEGG pathways with the top 10 NES. (B) Hallmark pathways with the top 10 NES.
Immune Infiltration Analysis
After the filtration of samples with
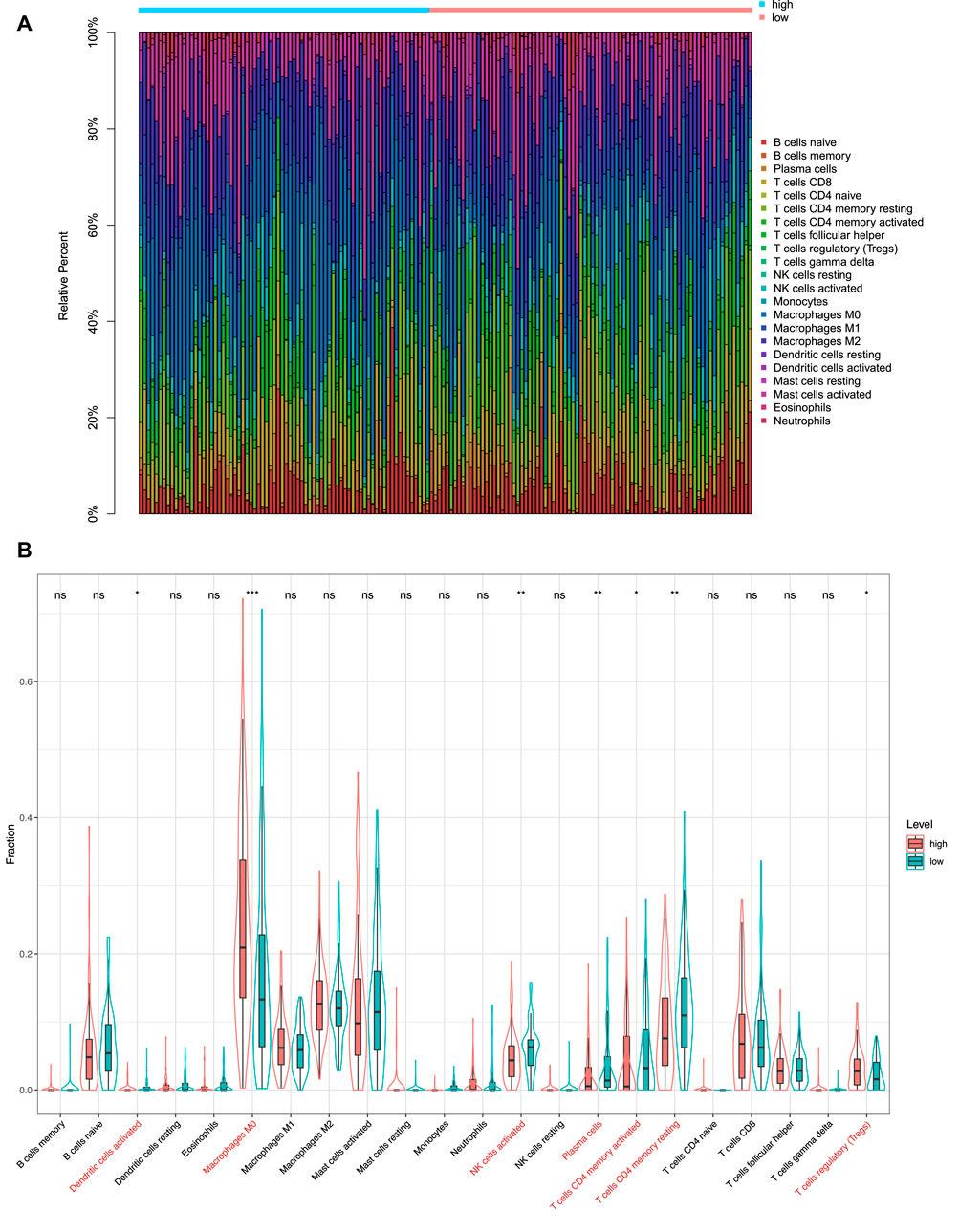
FIGURE 9. Immunity analysis via CIBERSORT. (A) Bar graph showing the proportion of 22 immune cell types in CC patients of TCGA-COAD. Column names of the plot are the sample ID. (B) Difference in the proportions of 22 immune cell types between patients in the high-risk and low-risk groups. *p < 0.05; **p < 0.01; ***p < 0.001; and ****p < 0.0001; ns, not significant.
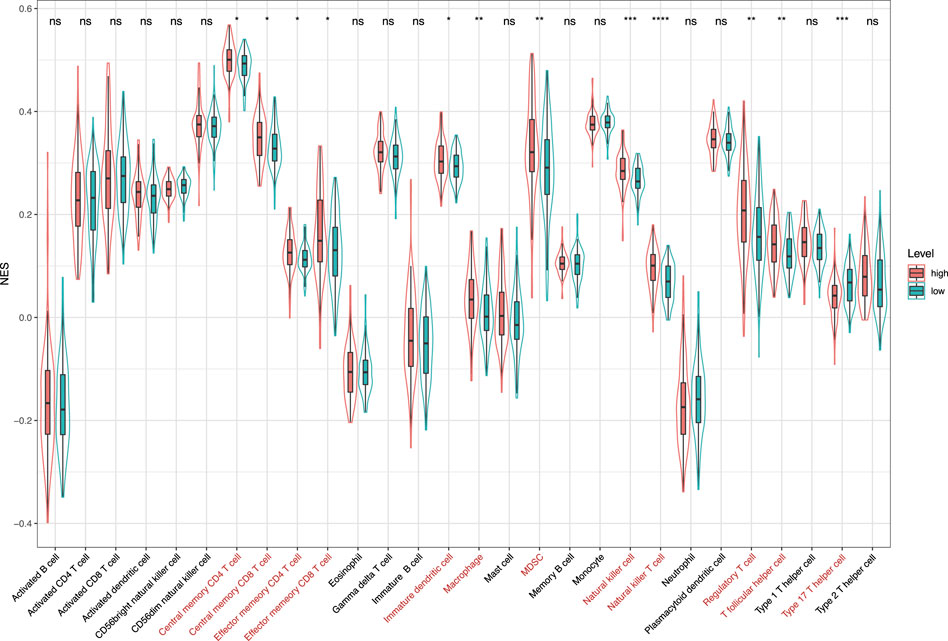
FIGURE 10. Normalized enrichment scores of 28 kinds of immune cells in the high-risk and low-risk groups. *p < 0.05; **p < 0.01; ***p < 0.001; and ****p < 0.0001; ns, not significant.
Discussion
With the rapid development of next-generation sequencing technologies, computational tools are used to identify biomarkers and study cancer disease, which is an emergent field in cancer systems biology (Yang J. et al., 2020; Xu et al., 2020). CC is a high-incidence malignant tumor with a poor prognosis. Although targeted drugs can improve the prognosis of patients with CC, the mortality rate among patients remains high (Zhou and Ma, 2019). Therefore, reliable biomarkers must be identified for constructing a prognostic model to assess the prognosis and survival of CC patients.
Ferroptosis is morphologically, biochemically, and genetically distinct from other forms of cell death (Dixon et al., 2012). Previous studies have demonstrated that ferroptosis is involved in tumor immunization and cancer immunotherapy (Wang W. et al., 2019; Xu et al., 2021). Ferroptosis and iron metabolism play important roles in the pathogenesis of cancer. Moreover, ferroptosis-related lncRNA has also attracted attention (Mao et al., 2018; Wang M. et al., 2019; Yang Y. et al., 2020).
In this study, we constructed a prognostic model of three ferroptosis-related lncRNAs (XXbac-B476C20.9, TP73-AS1, and SNHG15) and showed that it had a good predictive ability for the overall survival of CC patients. Interestingly, literature mining revealed that three lncRNAs (XXbac-B476C20.9, TP73-AS1, and SNHG15) had been confirmed to be significantly associated with cancer. For example, the lncRNA XXbac-B476C20.9 was identified as a potential biomarker closely related to the prognosis of CC patients (Huang et al., 2019), which was consistent with our results. The overexpression of lncRNA TP73-AS1 was not only associated with metastasis and advanced clinical stages in colorectal cancer patients (Cai et al., 2018) but also promoted colorectal cancer cell migration and invasion (Li et al., 2019). Patients with high expression of lncRNA SNHG15 displayed a significantly shorter overall survival in COAD (Jiang et al., 2018). Moreover, the deregulation of the lncRNA SNHG15 strongly affected the proliferation, invasion, and tumor formation abilities of colorectal cancer cells (Saeinasab et al., 2019). The aforementioned previous studies further corroborated the results of our study.
We also investigated the underlying molecular mechanism by which the prognostic model is involved in the occurrence and development of CC through the GSEA analysis. Previous studies have also shown that GPI anchor biosynthesis, complement and coagulation cascades, and focal adhesion could play an important role in the progression of colorectal cancer (Cubiella et al., 2018; Gu et al., 2018; Xing et al., 2020). ECM receptor interaction, focal adhesion, and glycosaminoglycan biosynthesis chondroitin sulfate enriched in the high-risk group were related to cell motility, cell proliferation, and cell differentiation, which play a crucial role in the invasion of cancer cells (Han et al., 2021). Moreover, the Hallmark pathway analysis showed that epithelial-mesenchymal transition, apical junction, angiogenesis, and hedgehog signaling were enriched in the high-risk group, which was consistent with a previous study on CC (Yang et al., 2022). It was revealed that the mitotic spindle might lead to tumor formation in multiple tissues including colon cancer (Pussila et al., 2018). Bile acid metabolism was found to impact the microbial composition in colon cancer (Kennedy and Chang, 2020). Therefore, it is plausible that the prognostic model based on the three ferroptosis-related lncRNAs is highly correlated with CC.
Notably, our study found that the infiltration levels of macrophages M0, macrophages M2, and mast cells activated were significantly higher in the high-risk group. It has been shown that macrophages M0 were associated with the survival risk of CC, and the relative fraction of macrophages M0 was significantly increased in CC tissues compared with healthy bowel tissues (Wu et al., 2020). In addition, macrophages M2 induce the epithelial-mesenchymal transition phenotype in CC cells (Lee et al., 2020). The mast cells activated were C3-associated immune cells, where the C3 gene can predict the prognosis of colorectal adenocarcinoma (Liu and Wang, 2021). After analyzing the 28 kinds of immune cells that are over-represented in the tumor microenvironment, we also found that 12 kinds of immune infiltration cells are significantly different between the high-risk and low-risk groups, especially natural killer cells and natural killer T cells. El-Deeb et al. (2022) have found that the natural killer cells activated by the alginate/κ-carrageenan oral microcapsules lead to apoptosis in the colon cancer Caco-2 cells. Yoshioka et al. (2012) showed that the number of colon tumors and natural killer T cells significantly decreased in the mice in the treated group. In summary, the results indicated that the prognostic model was associated with immune infiltration of CC and might provide a reference for the immunotherapy of CC.
Conclusion
In conclusion, we analyzed the lncRNA expression and clinical profiles in TCGA-COAD and GEO databases. Three differentially expressed ferroptosis-related lncRNAs (XXbac-B476C20.9, TP73-AS1, and SNHG15) were identified as biomarkers to establish a prognostic model for CC patients. The limitation to our study is that the prognostic model was constructed and validated on the database publicly available online. Future prospective clinical trials are required to further consolidate the effectiveness of the prognostic model.
Data Availability Statement
The original contributions presented in the study are included in the article/Supplementary Material; further inquiries can be directed to the corresponding author.
Author Contributions
JL performed data analysis and drafted the manuscript; JT assisted in manuscript writing; and XY designed the study and revised the manuscript. All authors read and approved the final version of the manuscript.
Funding
This work was supported by the National Natural Science Foundation of China (No. 11701379).
Conflict of Interest
The authors declare that the research was conducted in the absence of any commercial or financial relationships that could be construed as a potential conflict of interest.
Publisher’s Note
All claims expressed in this article are solely those of the authors and do not necessarily represent those of their affiliated organizations, or those of the publisher, the editors, and the reviewers. Any product that may be evaluated in this article, or claim that may be made by its manufacturer, is not guaranteed or endorsed by the publisher.
Supplementary Material
The Supplementary Material for this article can be found online at: https://www.frontiersin.org/articles/10.3389/fgene.2022.934196/full#supplementary-material
References
Borkiewicz, L., Kalafut, J., Dudziak, K., Przybyszewska-Podstawka, A., and Telejko, I. (2021). Decoding LncRNAs. Cancers 13 (11), 2643. doi:10.3390/cancers13112643
Cai, H.-j., Zhuang, Z.-c., Wu, Y., Zhang, Y.-y., Liu, X., Zhuang, J.-f., et al. (2021). Development and Validation of a Ferroptosis-Related lncRNAs Prognosis Signature in Colon Cancer. Bosn J Basic Med Sci 21 (5), 569–576. doi:10.17305/bjbms.2020.5617
Cai, Y., Yan, P., Zhang, G., Yang, W., Wang, H., and Cheng, X. (2018). Long Non-coding RNA TP73-AS1 Sponges miR-194 to Promote Colorectal Cancer Cell Proliferation, Migration and Invasion via Up-Regulating TGFα. Cbm 23 (1), 145–156. doi:10.3233/CBM-181503
Chang, Z., Huang, R., Fu, W., Li, J., Ji, G., Huang, J., et al. (2020). The Construction and Analysis of ceRNA Network and Patterns of Immune Infiltration in Colon Adenocarcinoma Metastasis. Front. Cell. Dev. Biol. 8, 688. doi:10.3389/fcell.2020.00688
Charoentong, P., Finotello, F., Angelova, M., Mayer, C., Efremova, M., Rieder, D., et al. (2017). Pan-cancer Immunogenomic Analyses Reveal Genotype-Immunophenotype Relationships and Predictors of Response to Checkpoint Blockade. Cell. Rep. 18 (1), 248–262. doi:10.1016/j.celrep.2016.12.019
Cubiella, J., Clos-Garcia, M., Alonso, C., Martinez-Arranz, I., Perez-Cormenzana, M., Barrenetxea, Z., et al. (2018). Targeted UPLC-MS Metabolic Analysis of Human Faeces Reveals Novel Low-Invasive Candidate Markers for Colorectal Cancer. Cancers 10 (9), 300. doi:10.3390/cancers10090300
Dixon, S. J., Lemberg, K. M., Lamprecht, M. R., Skouta, R., Zaitsev, E. M., Gleason, C. E., et al. (2012). Ferroptosis: an Iron-dependent Form of Nonapoptotic Cell Death. Cell. 149 (5), 1060–1072. doi:10.1016/j.cell.2012.03.042
El-Deeb, N. M., Ibrahim, O. M., Mohamed, M. A., Farag, M. M. S., Farrag, A. A., and El-Aassar, M. R. (2022). Alginate/κ-carrageenan Oral Microcapsules Loaded with Agaricus Bisporus Polysaccharides MH751906 for Natural Killer Cells Mediated Colon Cancer Immunotherapy. Int. J. Biol. Macromol. 205, 385–395. doi:10.1016/j.ijbiomac.2022.02.058
Feng, S., Yin, H., Zhang, K., Shan, M., Ji, X., Luo, S., et al. (2022). Integrated Clinical Characteristics and Omics Analysis Identifies a Ferroptosis and Iron-Metabolism-Related lncRNA Signature for Predicting Prognosis and Therapeutic Responses in Ovarian Cancer. J. Ovarian Res. 15 (1), 10. doi:10.1186/s13048-022-00944-y
Gu, C., Wang, X., Long, T., Wang, X., Zhong, Y., Ma, Y., et al. (2018). FSTL1 Interacts with VIM and Promotes Colorectal Cancer Metastasis via Activating the Focal Adhesion Signalling Pathway. Cell. Death Dis. 9 (6), 654. doi:10.1038/s41419-018-0695-6
Guo, Y., Qu, Z., Li, D., Bai, F., Xing, J., Ding, Q., et al. (2021). Identification of a Prognostic Ferroptosis-Related lncRNA Signature in the Tumor Microenvironment of Lung Adenocarcinoma. Cell. Death Discov. 7 (1), 190. doi:10.1038/s41420-021-00576-z
Han, N., Zhang, Y.-Y., Zhang, Z.-M., Zhang, F., Zeng, T.-Y., Zhang, Y.-B., et al. (2021). High Expression of PDGFA Predicts Poor Prognosis of Esophageal Squamous Cell Carcinoma. Med. Baltim. 100 (20), e25932. doi:10.1097/MD.0000000000025932
Hassannia, B., Vandenabeele, P., and Vanden Berghe, T. (2019). Targeting Ferroptosis to Iron Out Cancer. Cancer Cell. 35 (6), 830–849. doi:10.1016/j.ccell.2019.04.002
He, D., Liao, S., Xiao, L., Cai, L., You, M., He, L., et al. (2021). Prognostic Value of a Ferroptosis-Related Gene Signature in Patients with Head and Neck Squamous Cell Carcinoma. Front. Cell. Dev. Biol. 9, 739011. doi:10.3389/fcell.2021.739011
Huang, W., Liu, Z., Li, Y., Liu, L., and Mai, G. (2019). Identification of Long Noncoding RNAs Biomarkers for Diagnosis and Prognosis in Patients with Colon Adenocarcinoma. J Cell. Biochem. 120 (3), 4121–4131. doi:10.1002/jcb.27697
Jemal, A., Bray, F., Center, M. M., Ferlay, J., Ward, E., and Forman, D. (2011). Global Cancer Statistics. CA A Cancer J. Clin. 61 (2), 69–90. doi:10.3322/caac.20107
Jiang, H., Li, T., Qu, Y., Wang, X., Li, B., Song, J., et al. (2018). Long Non-coding RNA SNHG15 Interacts with and Stabilizes Transcription Factor Slug and Promotes Colon Cancer Progression. Cancer Lett. 425, 78–87. doi:10.1016/j.canlet.2018.03.038
Jin, L., Li, C., Liu, T., and Wang, L. (2020). A Potential Prognostic Prediction Model of Colon Adenocarcinoma with Recurrence Based on Prognostic lncRNA Signatures. Hum. Genomics 14 (1), 24. doi:10.1186/s40246-020-00270-8
Kennedy, M. S., and Chang, E. B. (2020). The Microbiome: Composition and Locations. Prog. Mol. Biol. Transl. Sci. 176, 1–42. doi:10.1016/bs.pmbts.2020.08.013
Lee, Y. S., Song, S. J., Hong, H. K., Oh, B. Y., Lee, W. Y., and Cho, Y. B. (2020). The FBW7-MCL-1 axis Is Key in M1 and M2 Macrophage-Related Colon Cancer Cell Progression: Validating the Immunotherapeutic Value of Targeting PI3Kγ. Exp. Mol. Med. 52 (5), 815–831. doi:10.1038/s12276-020-0436-7
Li, M., Jin, Y., and Li, Y. (2019). LncRNA TP73-AS1 Activates TGF-Β1 to Promote the Migration and Invasion of Colorectal Cancer Cell. Cmar Vol. 11, 10523–10529. doi:10.2147/CMAR.S228490
Lin, A., Zhang, J., and Luo, P. (2020). Crosstalk between the MSI Status and Tumor Microenvironment in Colorectal Cancer. Front. Immunol. 11, 2039. doi:10.3389/fimmu.2020.02039
Liu, H., Qiu, C., Wang, B., Bing, P., Tian, G., Zhang, X., et al. (2021). Evaluating DNA Methylation, Gene Expression, Somatic Mutation, and Their Combinations in Inferring Tumor Tissue-Of-Origin. Front. Cell. Dev. Biol. 9, 619330. doi:10.3389/fcell.2021.619330
Liu, Y., and Wang, X. (2021). Tumor Microenvironment-Associated Gene C3 Can Predict the Prognosis of Colorectal Adenocarcinoma: a Study Based on TCGA. Clin. Transl. Oncol. 23 (9), 1923–1933. doi:10.1007/s12094-021-02602-z
Ma, T.-L., Zhou, Y., Wang, C., Wang, L., Chen, J.-X., Yang, H.-H., et al. (2021). Targeting Ferroptosis for Lung Diseases: Exploring Novel Strategies in Ferroptosis-Associated Mechanisms. Oxidative Med. Cell. Longev. 2021, 1–21. doi:10.1155/2021/1098970
Mao, C., Wang, X., Liu, Y., Wang, M., Yan, B., Jiang, Y., et al. (2018). A G3BP1-Interacting lncRNA Promotes Ferroptosis and Apoptosis in Cancer via Nuclear Sequestration of P53. Cancer Res. 78 (13), canres.3454.2017–3496. doi:10.1158/0008-5472.CAN-17-3454
Mercer, T. R., Dinger, M. E., and Mattick, J. S. (2009). Long Non-coding RNAs: Insights into Functions. Nat. Rev. Genet. 10 (3), 155–159. doi:10.1038/nrg2521
Mou, Y., Wang, J., Wu, J., He, D., Zhang, C., Duan, C., et al. (2019). Ferroptosis, a New Form of Cell Death: Opportunities and Challenges in Cancer. J. Hematol. Oncol. 12 (1), 34. doi:10.1186/s13045-019-0720-y
Newman, A. M., Liu, C. L., Green, M. R., Gentles, A. J., Feng, W., Xu, Y., et al. (2015). Robust Enumeration of Cell Subsets from Tissue Expression Profiles. Nat. Methods 12 (5), 453–457. doi:10.1038/nmeth.3337
Prensner, J. R., and Chinnaiyan, A. M. (2011). The Emergence of lncRNAs in Cancer Biology. Cancer Discov. 1 (5), 391–407. doi:10.1158/2159-8290.CD-11-0209
Pussila, M., Törönen, P., Einarsdottir, E., Katayama, S., Krjutškov, K., Holm, L., et al. (2018). Mlh1 Deficiency in Normal Mouse Colon Mucosa Associates with Chromosomally Unstable Colon Cancer. Carcinogenesis 39 (6), 788–797. doi:10.1093/carcin/bgy056
Saeinasab, M., Bahrami, A. R., González, J., Marchese, F. P., Martinez, D., Mowla, S. J., et al. (2019). SNHG15 Is a Bifunctional MYC-Regulated Noncoding Locus Encoding a lncRNA that Promotes Cell Proliferation, Invasion and Drug Resistance in Colorectal Cancer by Interacting with AIF. J. Exp. Clin. Cancer Res. 38 (1), 172. doi:10.1186/s13046-019-1169-0
Schmitt, A. M., and Chang, H. Y. (2016). Long Noncoding RNAs in Cancer Pathways. Cancer Cell. 29 (4), 452–463. doi:10.1016/j.ccell.2016.03.010
Siegel, R. L., Miller, K. D., Fuchs, H. E., and Jemal, A. (2022). Cancer Statistics, 2022. CA A Cancer J. Clin. 72 (1), 7–33. doi:10.3322/caac.21708
Stockwell, B. R., Friedmann Angeli, J. P., Bayir, H., Bush, A. I., Conrad, M., Dixon, S. J., et al. (2017). Ferroptosis: A Regulated Cell Death Nexus Linking Metabolism, Redox Biology, and Disease. Cell. 171 (2), 273–285. doi:10.1016/j.cell.2017.09.021
Subramanian, A., Tamayo, P., Mootha, V. K., Mukherjee, S., Ebert, B. L., Gillette, M. A., et al. (2005). Gene Set Enrichment Analysis: a Knowledge-Based Approach for Interpreting Genome-wide Expression Profiles. Proc. Natl. Acad. Sci. U.S.A. 102 (43), 15545–15550. doi:10.1073/pnas.0506580102
Tang, D., and Kroemer, G. (2020). Peroxisome: the New Player in Ferroptosis. Sig Transduct. Target Ther. 5 (1), 273. doi:10.1038/s41392-020-00404-3
Tang, M., Chen, Z., Wu, D., and Chen, L. (2018). Ferritinophagy/ferroptosis: Iron‐related Newcomers in Human Diseases. J. Cell. Physiology 233 (12), 9179–9190. doi:10.1002/jcp.26954
Tsai, K.-W., Lo, Y.-H., Liu, H., Yeh, C.-Y., Chen, Y.-Z., Hsu, C.-W., et al. (2018). Linc00659, a Long Noncoding RNA, Acts as Novel Oncogene in Regulating Cancer Cell Growth in Colorectal Cancer. Mol. Cancer 17 (1), 72. doi:10.1186/s12943-018-0821-1
Wang, M., Mao, C., Ouyang, L., Liu, Y., Lai, W., Liu, N., et al. (2019a). Long Noncoding RNA LINC00336 Inhibits Ferroptosis in Lung Cancer by Functioning as a Competing Endogenous RNA. Cell. Death Differ. 26 (11), 2329–2343. doi:10.1038/s41418-019-0304-y
Wang, W., Green, M., Choi, J. E., Gijón, M., Kennedy, P. D., Johnson, J. K., et al. (2019b). CD8+ T Cells Regulate Tumour Ferroptosis during Cancer Immunotherapy. Nature 569 (7755), 270–274. doi:10.1038/s41586-019-1170-y
Wang, Z., Diao, J., Zhao, X., Xu, Z., and Zhang, X. (2021). Clinical and Functional Significance of a Novel Ferroptosis‐related Prognosis Signature in Lung Adenocarcinoma. Clin. Transl. Med. 11 (3), e364. doi:10.1002/ctm2.364
Wei, J., Zeng, Y., Gao, X., and Liu, T. (2021). A Novel Ferroptosis-Related lncRNA Signature for Prognosis Prediction in Gastric Cancer. BMC Cancer 21 (1), 1221. doi:10.1186/s12885-021-08975-2
Wu, D., Ding, Y., Wang, T., Cui, P., Huang, L., Min, Z., et al. (2020). Significance of Tumor-Infiltrating Immune Cells in the Prognosis of Colon Cancer. Ott Vol. 13, 4581–4589. doi:10.2147/OTT.S250416
Xing, S., Wang, Y., Hu, K., Wang, F., Sun, T., and Li, Q. (2020). WGCNA Reveals Key Gene Modules Regulated by the Combined Treatment of Colon Cancer with PHY906 and CPT11. Biosci. Rep. 40 (9), BSR20200935. doi:10.1042/BSR20200935
Xu, H., Ye, D., Ren, M., Zhang, H., and Bi, F. (2021). Ferroptosis in the Tumor Microenvironment: Perspectives for Immunotherapy. Trends Mol. Med. 27 (9), 856–867. doi:10.1016/j.molmed.2021.06.014
Xu, J., Cai, L., Liao, B., Zhu, W., and Yang, J. (2020). CMF-impute: an Accurate Imputation Tool for Single-Cell RNA-Seq Data. Bioinformatics 36 (10), 3139–3147. doi:10.1093/bioinformatics/btaa109
Yang, C., Huang, S., Cao, F., and Zheng, Y. (2021a). A Lipid Metabolism-Related Genes Prognosis Biomarker Associated with the Tumor Immune Microenvironment in Colorectal Carcinoma. BMC Cancer 21 (1), 1182. doi:10.1186/s12885-021-08902-5
Yang, J., Ju, J., Guo, L., Ji, B., Shi, S., Yang, Z., et al. (2022). Prediction of HER2-Positive Breast Cancer Recurrence and Metastasis Risk from Histopathological Images and Clinical Information via Multimodal Deep Learning. Comput. Struct. Biotechnol. J. 20, 333–342. doi:10.1016/j.csbj.2021.12.028
Yang, J., Peng, S., Zhang, B., Houten, S., Schadt, E., Zhu, J., et al. (2020a). Human Geroprotector Discovery by Targeting the Converging Subnetworks of Aging and Age-Related Diseases. GeroScience 42 (1), 353–372. doi:10.1007/s11357-019-00106-x
Yang, Y., Tai, W., Lu, N., Li, T., Liu, Y., Wu, W., et al. (2020b). lncRNA ZFAS1 Promotes Lung Fibroblast-To-Myofibroblast Transition and Ferroptosis via Functioning as a ceRNA through miR-150-5p/SLC38A1 axis. Aging 12 (10), 9085–9102. doi:10.18632/aging.103176
Yang, Y., Yan, X., Li, X., Ma, Y., and Goel, A. (2021b). Long Non-coding RNAs in Colorectal Cancer: Novel Oncogenic Mechanisms and Promising Clinical Applications. Cancer Lett. 504, 67–80. doi:10.1016/j.canlet.2021.01.009
Yoshioka, K., Ueno, Y., Tanaka, S., Nagai, K., Onitake, T., Hanaoka, R., et al. (2012). Role of Natural Killer T Cells in the Mouse Colitis-Associated Colon Cancer Model. Scand. J. Immunol. 75 (1), 16–26. doi:10.1111/j.1365-3083.2011.02607.x
Zhang, W., Fang, D., Li, S., Bao, X., Jiang, L., and Sun, X. (2021). Construction and Validation of a Novel Ferroptosis-Related lncRNA Signature to Predict Prognosis in Colorectal Cancer Patients. Front. Genet. 12, 709329. doi:10.3389/fgene.2021.709329
Zhou, F., Shen, F., Zheng, Z., and Ruan, J. (2019). The LncRNA XIRP2-AS1 Predicts Favorable Prognosis in Colon Cancer. Ott Vol. 12, 5767–5778. doi:10.2147/OTT.S215419
Zhou, N., and Bao, J. (2020). FerrDb: a Manually Curated Resource for Regulators and Markers of Ferroptosis and Ferroptosis-Disease Associations. Database (Oxford) 2020, baaa021. doi:10.1093/database/baaa021
Keywords: colon cancer, ferroptosis, long non-coding RNA, prognostic model, immune microenvironment
Citation: Lu J, Tan J and Yu X (2022) A Prognostic Ferroptosis-Related lncRNA Model Associated With Immune Infiltration in Colon Cancer. Front. Genet. 13:934196. doi: 10.3389/fgene.2022.934196
Received: 02 May 2022; Accepted: 13 June 2022;
Published: 31 August 2022.
Edited by:
Jialiang Yang, Geneis (Beijing) Co. Ltd., ChinaReviewed by:
Weiwei Zhang, East China University of Technology, ChinaYan Yang, Beijing Genomics Institute (BGI), China
Copyright © 2022 Lu, Tan and Yu. This is an open-access article distributed under the terms of the Creative Commons Attribution License (CC BY). The use, distribution or reproduction in other forums is permitted, provided the original author(s) and the copyright owner(s) are credited and that the original publication in this journal is cited, in accordance with accepted academic practice. No use, distribution or reproduction is permitted which does not comply with these terms.
*Correspondence: Xiaoqing Yu, eHF5dUBzaXQuZWR1LmNu