- 1Department of Surgery, James D. Eason Transplant Institute, College of Medicine, University of Tennessee Health Science Center, Memphis, TN, United States
- 2Center for Biomedical Informatics, College of Medicine, University of Tennessee Health Science Center, Memphis, TN, United States
Invasive fungal infections are a significant reason for morbidity and mortality among organ transplant recipients. Therefore, it is critical to investigate the host and candida niches to understand the epidemiology of fungal infections in transplantation. Candida albicans is an opportunistic fungal pathogen that causes fatal invasive mucosal infections, particularly in solid organ transplant patients. Therefore, identifying and characterizing these genes would play a vital role in understanding the complex regulation of host-pathogen interactions. Using 32 RNA-sequencing samples of human cells infected with C. albicans, we developed WGCNA coexpression networks and performed DESeq2 differential gene expression analysis to identify the genes that positively correlate with human candida infection. Using hierarchical clustering, we identified 5 distinct modules. We studied the inter- and intramodular gene network properties in the context of sample status traits and identified the highly enriched genes in the correlated modules. We identified 52 genes that were common in the most significant WGCNA turquoise module and differentially expressed genes in human endothelial cells (HUVEC) infection vs. control samples. As a validation step, we identified the differentially expressed genes from the independent Candida-infected human oral keratinocytes (OKF6) samples and validated 30 of the 52 common genes. We then performed the functional enrichment analysis using KEGG and GO. Finally, we performed protein-protein interaction (PPI) analysis using STRING and CytoHubba from 30 validated genes. We identified 8 hub genes (JUN, ATF3, VEGFA, SLC2A1, HK2, PTGS2, PFKFB3, and KLF6) that were enriched in response to hypoxia, angiogenesis, vasculogenesis, hypoxia-induced signaling, cancer, diabetes, and transplant-related disease pathways. The discovery of genes and functional pathways related to the immune system and gene coexpression and differential gene expression analyses may serve as novel diagnostic markers and potential therapeutic targets.
Introduction
Solid organ transplant (SOT) patients are exposed to various complications, e.g., invasive fungal infection and organ failure, which are the major challenge in SOT and affect the morbidity and mortality in transplant patients. The most prevalent invasive fungal infection in SOT is Candidiasis, which includes about 60% of infections, followed by aspergillosis accounts for up to 25% of fungal infections (Shoham and Marr, 2012).
An opportunistic fungal pathogen, Candida albicans, is part of healthy human gut microbiota. However, when immunity is compromised or suppressed, particularly in organ transplant individuals, AIDS patients, chemotherapy-treated patients, and neonates, the mucosal layer becomes more susceptible to fatal invasive C. albicans infections such as candidiasis (Sangeorzan et al., 1994; Rhodus et al., 1997; Revankar et al., 1998; Redding et al., 1999; Willis et al., 1999), (Sobel, 1985). C. albicans can switch from an avirulent commensal yeast form to a virulent invasive hyphal form in which hyphae invade through the mucosal layer and disseminate/propagate through the blood, infecting other organs as well as developing multidrug resistance (Klepser, 2006; Cowen et al., 2015; Arendrup and Patterson, 2017; Pendleton et al., 2017; Nishimoto et al., 2020). In the process of C. albicans infection, the first site of host-pathogen interactions is epithelial and endothelial cells (Barker et al., 2008; Liu et al., 2015a). The development of invasive fungal diseases relies on the synergy between the host immune response and fungal virulence. Comprehensive network analysis is vital to understanding the regulatory network and rewiring to respond to these infections.
Recent efforts have been made for the functional and molecular characterization of C. albicans genes using RNA sequencing (Wu et al., 2016; Brown et al., 2019; Romo et al., 2019; Zhang et al., 2019; de Vries et al., 2020; Thomas et al., 2020; Xu et al., 2020). Numerous studies suggest gene biomarkers as potential therapeutic targets and diagnostic markers in various fungal infections (Dix et al., 2015; Huppler et al., 2017; Díez et al., 2021; Hamam et al., 2021). Weighted gene correlation network analysis (WGCNA) has been widely used in disease diagnosis (Liu et al., 2017; Liang et al., 2018; Tang et al., 2018; Li et al., 2019; Yin et al., 2019), physiology (Kadarmideen et al., 2011; Zuo et al., 2018; Chen et al., 2019), drug targets (Puniya et al., 2013; Maertens et al., 2018), and cross-species (Mueller et al., 2017) but has never been applied in the context of candida pathogenesis (Thomas et al., 2020). Therefore, we developed a novel approach to identify host-pathogen interactions in C. albicans and humans.
In this work, we applied WGCNA to analyze 32 RNA-seq samples from in vitro infection of C. albicans on human endothelial and oral epithelial cells after 1.5, 5, and 8-h of infection and controls. We identified 5 modules in human endothelial cells (HUVEC) human cell lines in infection vs. control status and separately identified differentially expressed genes (DEG). We reported the common genes across the two methods (WGCNA and DEG). We then validated a subset of genes using differential gene expression analysis of candida-infected human cell lines OKF6. Finally, we performed protein-protein interaction network analysis and identified hub genes that could be novel targets to investigate C. albicans infection in humans. Through these central genes’ biological and molecular functions, we gained insights into the signaling pathways previously not correlated with the fungal pathogen-host response and other diseases.
Materials and methods
Data collection
All processed gene expression datasets were collected from publicly available NCBI Gene Expression Omnibus GSE56093 (Liu et al., 2015a). The raw sequence data was aligned to the human and candida reference genomes separately by Liu et al. (Liu et al., 2015a), and the resultant count matrices were utilized for the WGCNA and DEG analyses. This dataset was comprised of 88 samples from in vitro and in vivo experiments. Of those, we only utilized 32 in vitro samples of human cell lines (endothelial and epithelial) infected with C. albicans (SC5314 and WO1 strains) and their controls at three different time points. More information is given in Supplementary Table S1. The overall methodology steps are shown in Figure 1.
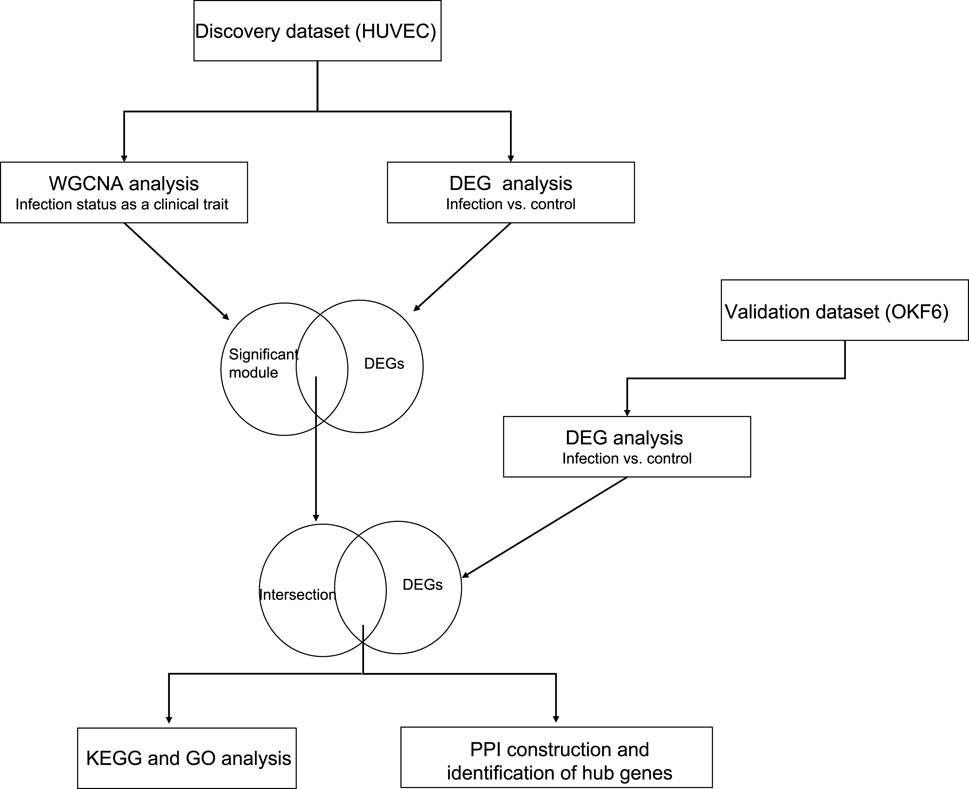
FIGURE 1. The schematic representation of the overall methodology: The discovery dataset was analyzed using two independent methods (WGCNA and DESeq2). Their intersecting genes were overlapped with the DEG list from the independent validation set to build the PPI network and identify the hub genes.
Data normalization and transformation
We performed normalization on the RPKM (reads per kilobase of transcript, per million mapped reads) values using the GCRMA limma package (Gautier et al., 2004) by first removing features with counts <10 in 90% of the samples, as these could be a potential cause of the noise. Then, we performed and compared three data transformation techniques, logarithmic, regularized logarithmic, and variance stabilizing transformation (Lin et al., 2008), to stabilize the variance across sample mean values. We chose the regularized log transformation due to stability (Supplementary Figure S1).
Weighted gene coexpression network framework
We constructed the weighted gene coexpression network using the R WGCNA package (Langfelder and Horvath, 2008). The normalized data were used as input for network construction and gene module detection. It uses correlation to find functional modules of the highly correlated gene networks. First, we evaluated the soft threshold power (β) to convert coexpression into weight with a scale-free topology index of 0.9. We chose soft threshold powers of 8 to calculate the correlations between the adjacent genes (Supplementary Figure S2). Pearson correlations between each gene pair were calculated. We then converted this adjacency matrix into a topological overlap matrix (TOM) to define gene clusters that show the amount of overlap in shared neighbors of the gene network. The dissimilarity measure was determined for hierarchical clustering and module detection. Modules of clusters of genes with high topological overlap were selected using a dynamic tree-cut algorithm. Several modules were identified, and the modules with similar expression levels were merged by calculating their eigengenes corresponding to their correlations. We further determined the association of these modules with the external traits. We identified the genes with high gene significance (GS) and module membership (MM) in the turquoise and blue modules in HUVEC data. Last, intramodular connectivity was analyzed in human modules using MTR>0.35 and p-value < 0.05. All the categorical variables were binarized for the analyses.
Identification of differentially expressed genes
Differentially expressed genes were identified using DESeq2 R Bioconductor package (Love et al., 2014). We used raw counts that were fed to the DESeq2 since it corrects for library size. The variance stabilizing transformations (VST) function estimated the sample differences (Lin et al., 2008). The statistical significance for the differentially expressed genes was set to q-value < 0.05 and log2 fold change (log2FC) > 1.
Functional enrichment analysis of genes
We performed Gene Ontology (GO) and Kyoto Encyclopedia of Genes and Genomes (KEGG) enrichment analyses to study the role of the genes and identify their biological functions and pathways. Gene Ontology analysis was performed to determine the biological process. We considered an adjusted p-value threshold of ≤ 0.05 and a minimum gene count of 3 for the KEGG pathways and GO functional terms. As the contribution of all the genes is not the same, we identified hub genes and further investigated their function.
Statistical analysis and data visualization
The R programming language (Horgan, 2012) was used to normalize the RNA-seq data. We conducted Fisher’s exact tests to identify the statistically significant Gene Ontology terms and functional classes. Enrichment analysis based on a hypergeometric test was implemented, and Benjamini Hochberg multiple testing was used to correct the p-value. Data visualization to show differentially expressed genes between infected and uninfected groups for top selected genes was plotted using the complex Heatmap function in R. The data visualization was performed using the cluster profiler package in R (Yu et al., 2012).
Protein-protein interaction network analysis
The validated genes are uploaded into the STRING database, and high confidence interaction score ≥ 0.7 was used to reduce false-positive interactions (Bozhilova et al., 2019). The resultant network output was loaded into Cytoscape. CytoHubba (Chin et al., 2014) was used with the Maximal Clique Centrality (MCC) algorithm to discover the hub genes in the PPI network (Li and Xu, 2019).
Results
Network construction and module identification
Weighted Gene Correlation Network Analysis was conducted on HUVEC data. We performed hierarchical clustering of genes using a topological overlap matrix and merged modules with similar expression profiles (Figure 2A). Each leaf corresponds to a gene, and branches correspond to the cluster of highly coexpressed genes. After cutting tree branches, we identified five different modules, turquoise, yellow, black, blue, and green, with 1,365, 459, 261, 1829, and 755 genes in HUVEC (Supplementary Table S2). A total of 4,669 genes were identified from the HUVEC data set, and in each module, the number of genes ranged between 261 and 1829.
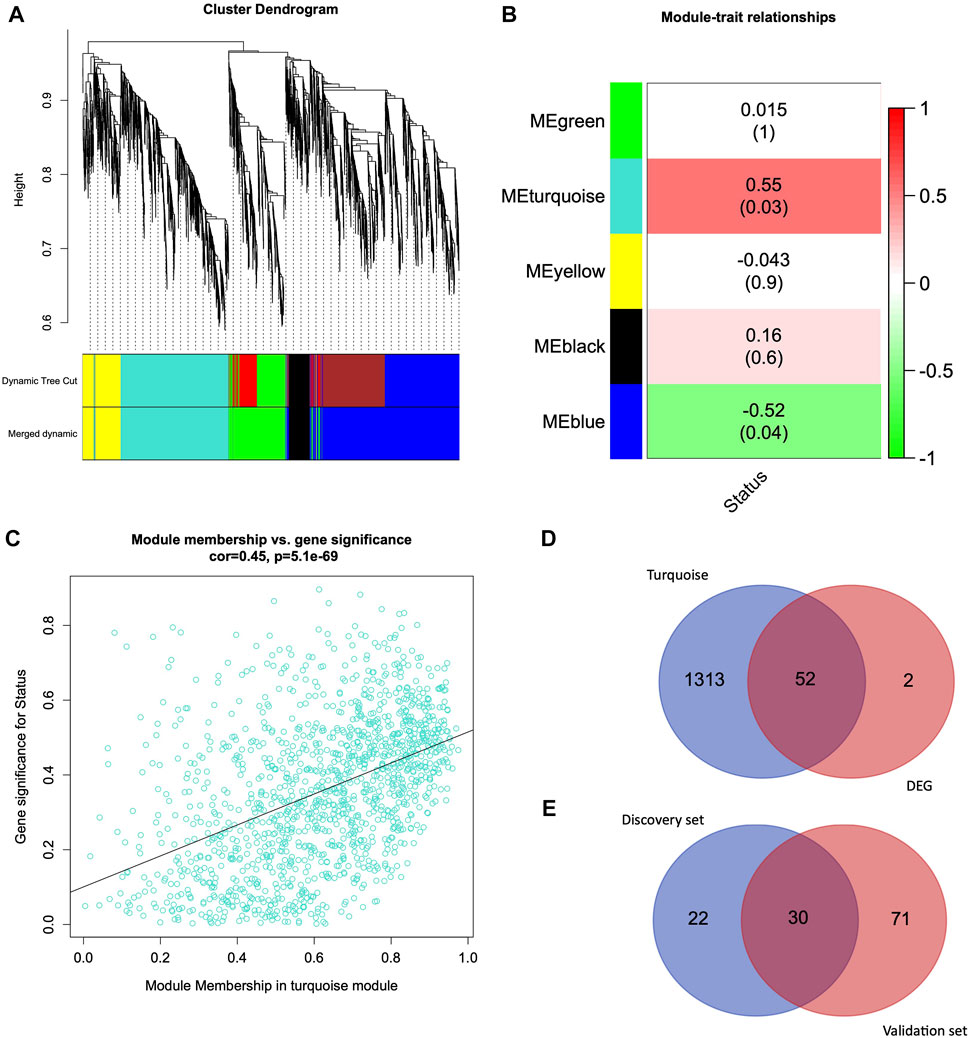
FIGURE 2. Weighted Gene Coexpression Network Analysis and Venn Diagram (A) Hierarchical clustering of 4,669 genes from HUVEC discovery dataset (B) Module-trait relationship exhibiting associations of module eigengenes with the clinical trait (infection status). (C) Relationship between turquoise module membership (MM) and gene significance (GS). (D) Venn diagram representing the overlapping genes from the turquoise module genes and differentially expressed genes. (E) Venn diagram representing the common genes between the discovery set genes (overlapping genes from WGCNA turquoise module and DEG genes) and differentially expressed genes from the validation set.
Module association with external traits
We further analyzed the module trait relationship (MTR) between the module eigengene and clinical traits, where each cell represents the correlation strength (red is positively correlated, and green is negatively correlated) with their corresponding p-value (Figure 2B). We demonstrate that some module eigengenes are highly correlated with infection (status traits). We observed that the turquoise (r = 0.55, p = 0.03) and blue (r = −0.52, p = 0.04) modules were highly correlated with the infection status in HUVEC cells. Since the turquoise module, with 1,365 genes, is the most significantly correlated with the clinical trait, we focused on this module for further analysis.
Intramodular connectivity using gene significance and module membership
We quantified genes with high significance for the trait status of HUVEC and high module membership by comparing their similarities in every module. There was a highly significant correlation between gene significance and module membership in the turquoise module. Figure 2C represents the correlation between turquoise module membership and gene significance (r = 0.45, p = 5.1e-69).
Differentially expressed genes and intersection with WGCNA
We used DESeq2 as a second independent method on the entire HUVEC dataset to identify 54 genes that were differentially expressed in the HUVEC (infection vs. control) samples (q-value < 0.05 and log2FC > 1). From the WGCNA analysis, we identified 1,365 genes in the most significantly correlated turquoise module (Figure 2B). When we further investigated the intersection of WGCNA and DEGs, 52 genes were common between the turquoise module and the DEG list (Figure 2D). The list of turquoise module genes, DEGs, and intersecting genes is given in Supplementary Table S4.
Validation of candidate genes
In order to validate these 52 common genes, we utilized a validation dataset comprised of candida-infected human oral keratinocytes (OKF6 cell line). We performed the differential gene expression analysis on infection vs. control and identified 101 DEGs. When we overlapped these 101 genes with 52 common genes from the discovery dataset, we found 30 genes that were differentially expressed in the OKF6 validation dataset (Figure 2E and Supplementary Table S3). The following are the 30 validated genes: SLC2A1, ATF3, JUN, KDM7A, DUSP1, PTGS2, NAB2, PIM1, MAFF, ADM, PFKFB3, KLF6, BNIP3, CSRNP1, VEGFA, ENO2, ANKRD37, PPP1R15A, KDM3A, ANGPTL4, BHLHE40, ARRDC3, SLC2A3, KLF7, DDIT4, ERRFI1, KLF4, FOSL2, EFNA1, and HK2. The list of genes from the discovery set, validation set, and intersecting genes are given in Supplementary Table S4.
Integrating network analysis with functional enrichment analyses
To understand the biological roles of these 30 validated genes, we performed GO and KEGG pathway analyses to identify the biological pathways that were significantly enriched (FDR ≤ 0.05) in these modules.
KEGG analyses revealed that the genes were highly enriched in the HIF-1 signaling pathway, microRNAs in cancer, renal cell carcinoma, and AGE-RAGE signaling pathway in diabetes complications, as shown in Figure 3A (Detailed information is provided in Supplementary Table S3). Gene Ontology analyses elucidated that these genes were enriched in response to hypoxia, monosaccharide metabolic process, angiogenesis regulation, vasculature development, reproductive process, and epidermis development, as shown in Figure 3B. Additional details are given in Supplementary Table S3.
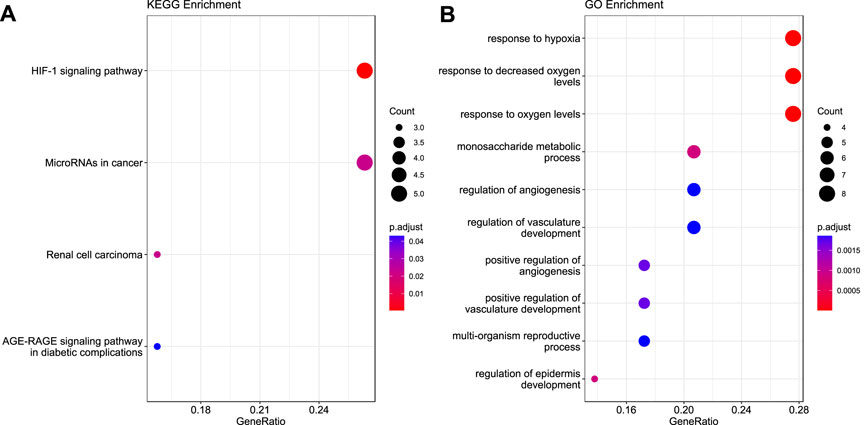
FIGURE 3. Functional enrichment analysis for the 30 validated genes. (A) KEGG pathway (B) Gene Ontology.
Protein-protein interaction network analysis
From the 30 validated genes, we first performed the protein-protein interaction (PPI) analysis using the STRING database (Figure 4A). The resultant data is then imported to the Cytoscape plugin CytoHubba, and the top 8 genes with the highest Maximal Clique Centrality (MCC) score were considered hub genes: JUN, ATF3, VEGFA, SLC2A1, HK2, PTGS2, PFKFB3, and KLF6 (Figure 4B and Supplementary Table S3).
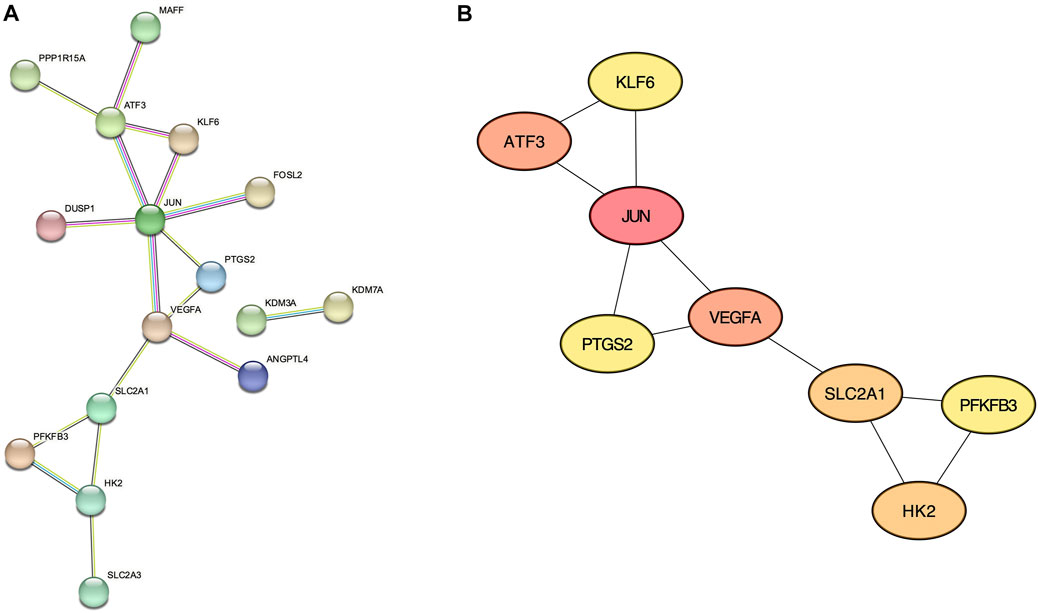
FIGURE 4. Protein-Protein interaction networks. (A) STRING analysis of validated genes. (B) CytoHubba with Maximal Clique Centrality analysis showing 8 hub genes.
Discussion
The interaction between host cells and Candida is central to the immunopathology of candidiasis in transplant patients; a comprehensive understanding of this synergy will identify new treatment strategies. Here, we investigate how human epithelial and endothelial cells communicate with different Candida species during infection. In this study, we constructed a weighted gene correlation network and performed differential gene expression analysis to identify genes that are important in host‒candida interactions.
Comparative network analysis could rank genes for further investigation of their connectivity (Schadt et al., 2005). A distinct advantage of WGCNA is that it considers modules or gene clusters for constructing interactions, and the genes in a module are likely to be connected by the same regulatory pathways. Therefore, in this study, we aim to discover novel genes and molecular pathways in human-candida infection and to understand the regulation due to cell dynamics using the WGCNA and DESeq2 algorithms. Network depictions provided immediate insight into the relationships between the correlated modules. The construction of a gene coexpression network and differential gene expression analysis of the discovery and validation data set facilitated the identification of genes with similar biological functions by GO and KEGG analyses.
According to the results of functional enrichment analysis, the top 3 GO terms and topmost KEGG pathway were a response to hypoxia, response to decreased oxygen, response to oxygen levels (Figure 3B), and hypoxia-inducible factor 1 (HIF-1) signaling pathway (Figure 3A). HIF-1 is a transcription factor that functions as a master regulator of oxygen homeostasis. It has been shown that suppressing HIF-1 helps treat cancer and ischemia (Ziello et al., 2007). All organs during the process of transplantation undergo hypoxic and ischemic injury. Low oxygen levels trigger the colonization of candida infection in the human host, resulting in complications like allograft rejection in SOT patients (Akhtar et al., 2014). We identified eight hub genes using PPI network analysis. Four hub genes (HK2, PFKFB3, SLC2A1, and VEGFA) are involved in the HIF-1 signaling pathway. The hexokinase isoenzyme (HK2) elevates innate immunity in hepatocellular carcinoma (Perrin-Cocon et al., 2021). HK2 and PFKFB3 are involved in glycolysis which affects the immune response against fungal infection (Perrin-Cocon et al., 2021); specifically, after transplantation, the PFKFB3 gene increase the risk of invasive pulmonary aspergillosis (Gonçalves et al., 2021). Huang et al. showed in their omics analysis that SLC2A1 is involved in ischemic reperfusion injury in liver transplant patients and forms the core gene network (Huang et al., 2019a). Vascular Endothelial Growth Factor A (VEGFA) is associated with an increased risk of chronic kidney disease (Anderson et al., 2018) but induces vasculogenesis. Kidney vasculature comprises vascular smooth muscle and endothelial cells (Udan et al., 2012). One of the most challenging components to handle during a kidney transplant is through vasculogenesis and angiogenesis processes (Munro and Davies, 2018; Lebedenko and Banerjee, 2021). HIF-1 stimulates the VEGFA to maintain oxygen delivery and protect the kidney (Hunga et al., 2013).
The other top enriched KEGG pathways in our analysis were microRNAs in cancer (hsa05211) and renal cell carcinoma (hsa05206). MicroRNAs play a diverse role in cancer and infections (Yong and Dutta, 2009). Recent advances in microRNA therapeutics have shown the extensive use of microRNAs for cancer and infections (Rupaimoole and Slack, 2017). There has been increased support for microRNA therapeutics in solid organ transplantation, including kidney (Wilflingseder et al., 2015; Jin et al., 2017; Ledeganck et al., 2019), lung (Benazzo et al., 2022), and heart transplantation (Hamdorf et al., 2017). Candida albicans have been linked to cancerous processes by taking advantage of the compromised immune system (Ramirez-Garcia et al., 2016; Chung et al., 2017; Sultan et al., 2022). Our PPI network analysis identified four hub genes (SLC2A1, VEGFA, JUN, and PTGS2) enriched in the cancer-related pathways. SLC2A1 belongs to a glucose transporter family and has been reported to be associated with HCC (Kim et al., 2017). SLC2A1 is also essential to IRI during liver transplantation (Huang et al., 2019b) and a diagnostic biomarker for colorectal cancer (CRC) (Liu et al., 2022). In CRC, the SLC2A1 gene infiltrates the CD4+ T cell, neutrophil, dendritic cells, and B cells (Liu et al., 2022). Candidiasis is one of the risk factors for Oral squamous cell carcinoma (OSCC). The transcriptomics data analysis revealed that VEGFA and JUN are highly regulated in OSCC invasion and metastasis (Vadovics et al., 2022). JUN is a member of the activator protein-1 family of oncogenic transcription factors, which is involved in various cancer-related and cell signaling pathways such as tumorigenesis, cell differentiation, and angiogenesis (Brennan et al., 2020). Post renal transplantation, the activation of c-JUN affects acute humoral rejection and acute T-cell-mediated rejection (Kobayashi et al., 2010). c-JUN is also associated with reduced graft function and plays an important role in renal pathophysiological events (Kobayashi et al., 2010). Prostaglandin E2 (PGE2) is an inflammatory mediator produced by the Prostaglandin-endoperoxide synthase (PTGS2) enzyme, and PGE2 promotes candida morphogenesis. In response to candida infection, PTGS2 activation promotes NF-kB and MAPK signaling pathways (Deva et al., 2003). In OSCC, PTGS2 involves an inflammatory response to infection by promoting tumorigenesis (Cacina et al., 2018) and activating transcription factor 3 (ATF3), one of the 8 hub genes that regulate the PTGS2 during acute inflammation (Hellmann et al., 2015) and helps in the homeostasis of the metabolism and immune system (Sha et al., 2017). Zhu et al. also showed that ATF3 is one of the top hub genes in samples infected with 4 different candida species (Zhu et al., 2022). Using bioinformatics omics analysis, ATF3 and Kruppel-like factor 6 (KLF6, hub gene) are shown to be the central players in ischemic reperfusion injury in liver transplant patients (Huang et al., 2019b). KLF6 promotes inflammation and oxidative stress by regulating HIF-1 expression in macrophages (Kim et al., 2020).
Another enriched KEGG pathway was the AGE-RAGE signaling in diabetes complications (hsa04933). Endoplasmic reticulum stress due to AGE-RAGE plays an essential role in renal inflammation, diabetic nephropathy (Pathomthongtaweechai and Chutipongtanate, 2020) and early-stage renal disease (Meerwaldt et al., 2009). Advanced glycation end products (AGEs) may also play a role in the hardening of arteries after renal transplantation (Liu et al., 2015b). Our two hub genes, JUN and VEGFA, showed enrichment in the AGE-RAGE signaling pathway in diabetes complications. Poorly controlled diabetes increases the risk of fungal infections (Rodrigues et al., 2019). Some diabetes-related complications include cardiovascular disease, kidney disease, neuropathy, hearing loss, vision loss, Alzheimer’s, liver disease, etc. (Deshpande et al., 2008; Prasad et al., 2016). VEGFA and JUN were identified as the central players in diabetic nephropathy (Oltean et al., 2015; Wang et al., 2021) and Alzheimer’s disease (Zu et al., 2021) whereas, VEGFA was associated with diabetic retinopathy (Bucolo et al., 2021), cardiac autonomic neuropathy (Ravichandran et al., 2019), and non-alcoholic fatty liver disease-hepatocellular carcinoma (Shen et al., 2022). Each hub gene plays a vital and diverse role in the pathways and biological processes. Therefore, more research is warranted on the divergent roles of these genes’ signaling and regulatory mechanisms during infection, cancer, and transplantation.
Limitations
WGCNA lacks resolution as it decomposes a group of genes into a single eigenvalue that may not correctly represent a single gene’s expression profile or pathway changes. Further analysis may be needed to detect changes in the expression of individual processes. Another limitation of the study is the small sample size; therefore, we present this study as a proof of concept to be validated in a larger cohort. The current study used cell lines from epithelial and endothelial cells; thus, the identified gene markers should be validated from the peripheral blood transcriptome of candidiasis patients for non-invasive clinical relevance.
Data availability statement
Publicly available datasets were analyzed in this study. This data can be found here: https://www.ncbi.nlm.nih.gov/geo/query/acc.cgi?acc=GSE56093.
Author contributions
AM conceived the study design. SN developed the methodology and performed data analysis, and wrote the manuscript. SN and AM revised and edited the manuscript.
Acknowledgments
We would like to acknowledge Liu Yaoping for providing the data. We would also like to thank the office of scientific writing at the University of Tennessee Health Science Center for copy editing and proofreading the manuscript. We also thank the reviewers for their constructive feedback on improving the manuscript, including the experimental design and analysis suggestions. The publication costs for this article have been covered by the Center for Biomedical Informatics at the University of Tennessee Health Science Center.
Conflict of interest
The authors declare that the research was conducted in the absence of any commercial or financial relationships that could be construed as a potential conflict of interest.
Publisher’s note
All claims expressed in this article are solely those of the authors and do not necessarily represent those of their affiliated organizations, or those of the publisher, the editors and the reviewers. Any product that may be evaluated in this article, or claim that may be made by its manufacturer, is not guaranteed or endorsed by the publisher.
Supplementary material
The Supplementary Material for this article can be found online at: https://www.frontiersin.org/articles/10.3389/fgene.2022.917636/full#supplementary-material
References
Akhtar, M. Z., Sutherland, A. I., Huang, H., Ploeg, R. J., and Pugh, C. W. (2014). The role of hypoxia-inducible factors in organ donation and transplantation: The current perspective and future opportunities. Am. J. Transpl. 14, 1481–1487. doi:10.1111/ajt.12737
Anderson, C. E., Hamm, L. L., Batuman, G., Kumbala, D. R., Chen, C. S., Kallu, S. G., et al. (2018). The association of angiogenic factors and chronic kidney disease. BMC Nephrol. 19, 117–118. doi:10.1186/s12882-018-0909-2
Arendrup, M. C., and Patterson, T. F. (2017). Multidrug-resistant candida: Epidemiology, molecular mechanisms, and treatment. J. Infect. Dis. 216, S445–S451. doi:10.1093/infdis/jix131
Barker, K. S., Park, H., Phan, Q. T., Xu, L., Homayouni, R., Rogers, P. D., et al. (2008). Transcriptome profile of the vascular endothelial cell response to Candida albicans. J. Infect. Dis. 198, 193–202. doi:10.1086/589516
Benazzo, A., Bozzini, S., Auner, S., Berezhinskiy, H. O., Watzenboeck, M. L., Schwarz, S., et al. (2022). Differential expression of circulating miRNAs after alemtuzumab induction therapy in lung transplantation. Sci. Rep. 121 12, 7072–7113. doi:10.1038/s41598-022-10866-w
Bozhilova, L. V., Whitmore, A. V., Wray, J., Reinert, G., and Deane, C. M. (2019). Measuring rank robustness in scored protein interaction networks. BMC Bioinforma. 20, 446. doi:10.1186/s12859-019-3036-6
Brennan, A., Leech, J. T., Kad, N. M., and Mason, J. M. (2020). Selective antagonism of cJun for cancer therapy. J. Exp. Clin. Cancer Res. 39, 184–216. doi:10.1186/s13046-020-01686-9
Brown, S. E., Schwartz, J. A., Robinson, C. K., OʼHanlon, D. E., Bradford, L. L., He, X., et al. (2019). The vaginal microbiota and behavioral factors associated with genital Candida albicans detection in reproductive-age women. Sex. Transm. Dis. 46, 753–758. doi:10.1097/OLQ.0000000000001066
Bucolo, C., Barbieri, A., Vigano, I., Marchesi, N., Bandello, F., Drago, F., et al. (2021). Short-and long-term expression of vegf: A temporal regulation of a key factor in diabetic retinopathy. Front. Pharmacol. 12, 2164. doi:10.3389/fphar.2021.707909
Cacina, C., Kaşarci, G., Bektaş, K., Unur, M., and Cakmakoglu, B. (2018). The COX2 genetic variants in oral squamous cell carcinoma in Turkish population. Cell. Mol. Biol. 64, 96–100. doi:10.14715/cmb/2018.64.14.16
Chen, R., Ge, T., Jiang, W., Huo, J., Chang, Q., Geng, J., et al. (2019). Identification of biomarkers correlated with hypertrophic cardiomyopathy with co-expression analysis. J. Cell. Physiol. 234, 21999–22008. doi:10.1002/jcp.28762
Chin, C. H., Chen, S. H., Wu, H. H., Ho, C. W., Ko, M. T., and Lin, C. Y. (2014). cytoHubba: Identifying hub objects and sub-networks from complex interactome. BMC Syst. Biol. 8, S11–S17. doi:10.1186/1752-0509-8-S4-S11
Chung, L. M., Liang, J. A., Lin, C. L., Sun, L. M., and Kao, C. H. (2017). Cancer risk in patients with candidiasis: A nationwide population-based cohort study. Oncotarget 8, 63562–63573. doi:10.18632/oncotarget.18855
Cowen, L. E., Sanglard, D., Howard, S. J., Rogers, P. D., and Perlin, D. S. (2015). Mechanisms of antifungal drug resistance. Cold Spring Harb. Perspect. Med. 5, a019752. doi:10.1101/cshperspect.a019752
de Vries, D. H., Matzaraki, V., Bakker, O. B., Brugge, H., Westra, H. J., Netea, M. G., et al. (2020). Integrating GWAS with bulk and single-cell RNA-sequencing reveals a role for LY86 in the anti-Candida host response. PLoS Pathog. 16, e1008408. doi:10.1371/journal.ppat.1008408
Deshpande, A. D., Harris-Hayes, M., and Schootman, M. (2008). Epidemiology of diabetes and diabetes-related complications. Phys. Ther. 88, 1254–1264. doi:10.2522/ptj.20080020
Deva, R., Shankaranarayanan, P., Ciccoli, R., and Nigam, S. (2003). Candida albicans induces selectively transcriptional activation of cyclooxygenase-2 in HeLa cells: Pivotal roles of toll-like receptors, p38 mitogen-activated protein kinase, and NF-κB. J. Immunol. 171, 3047–3055. doi:10.4049/jimmunol.171.6.3047
Díez, A., Carrano, G., Bregon-Villahoz, M., Cuetara, M. S., Garcia-Ruiz, J. C., Fernandez-de-Larrinoa, I., et al. (2021). Biomarkers for the diagnosis of invasive candidiasis in immunocompetent and immunocompromised patients. Diagn. Microbiol. Infect. Dis. 101, 115509. doi:10.1016/j.diagmicrobio.2021.115509
Dix, A., Hunniger, K., Weber, M., Guthke, R., Kurzai, O., and Linde, J. (2015). Biomarker-based classification of bacterial and fungal whole-blood infections in a genome-wide expression study. Front. Microbiol. 6, 171. doi:10.3389/fmicb.2015.00171
Gautier, L., Cope, L., Bolstad, B. M., and Irizarry, R. A. (2004). Affy - analysis of affymetrix GeneChip data at the probe level. Bioinformatics 20, 307–315. doi:10.1093/bioinformatics/btg405
Gonçalves, S. M., Antunes, D., Leite, L., Mercier, T., Horst, R. T., Vieira, J., et al. (2021). Genetic variation in PFKFB3 impairs antifungal immunometabolic responses and predisposes to invasive pulmonary aspergillosis. MBio 12, e0036921. doi:10.1128/mBio.00369-21
Hamam, J., Navellou, J. C., Bellanger, A. P., Bretagne, S., Winiszewski, H., Scherer, E., et al. (2021). New clinical algorithm including fungal biomarkers to better diagnose probable invasive pulmonary aspergillosis in ICU. Ann. Intensive Care 11, 41–49. doi:10.1186/s13613-021-00827-3
Hamdorf, M., Kawakita, S., and Everly, M. (2017). The potential of MicroRNAs as novel biomarkers for transplant rejection. J. Immunol. Res. 2017, 4072364. doi:10.1155/2017/4072364
Hellmann, J., Tang, Y., Zhang, M. J., Hai, T., Bhatnagar, A., Srivastava, S., et al. (2015). Atf3 negatively regulates Ptgs2/Cox2 expression during acute inflammation. Prostagl. Other Lipid Mediat. 0, 49–56. doi:10.1016/j.prostaglandins.2015.01.001
Huang, S., Ju, W., Zhu, Z., Han, M., Sun, C., Tang, Y., et al. (2019). Comprehensive and combined omics analysis reveals factors of ischemia-reperfusion injury in liver transplantation. Epigenomics 11, 527–542. doi:10.2217/epi-2018-0189
Hunga, T. W., Liou, J. H., Yeh, K. T., Tsai, J. P., Wu, S. W., Tai, H. C., et al. (2013). Renal expression of hypoxia inducible factor-1α in patients with chronic kidney disease: A clinicopathologic study from nephrectomized kidneys. Indian J. Med. Res. 137, 102–110.
Huppler, A. R., Fisher, B. T., Lehrnbecher, T., Walsh, T. J., and Steinbach, W. J. (2017). Role of molecular biomarkers in the diagnosis of invasive fungal diseases in children. J. Pediatr. Infect. Dis. Soc. 6, S32–S44. doi:10.1093/jpids/pix054
Jin, P., Chen, H., Xie, J., Zhou, C., and Zhu, X. (2017). Essential role of microRNA-650 in the regulation of B-cell CLL/lymphoma 11B gene expression following transplantation: A novel mechanism behind the acute rejection of renal allografts. Int. J. Mol. Med. 40, 1840–1850. doi:10.3892/ijmm.2017.3194
Kadarmideen, H. N., Watson-Haigh, N. S., and Andronicos, N. M. (2011). Systems biology of ovine intestinal parasite resistance: Disease gene modules and biomarkers. Mol. Biosyst. 7, 235–246. doi:10.1039/c0mb00190b
Kim, G. D., Ng, H. P., Chan, E. R., and Mahabeleshwar, G. H. (2020). Kruppel-like factor 6 promotes macrophage inflammatory and hypoxia response. FASEB J. 34, 3209–3223. doi:10.1096/fj.201902221R
Kim, Y. H., Jeong, D. C., Pak, K., Han, M. E., Kim, J. Y., Liangwen, L., et al. (2017). SLC2A2 (GLUT2) as a novel prognostic factor for hepatocellular carcinoma. Oncotarget 8, 68381–68392. doi:10.18632/oncotarget.20266
Klepser, M. E. (2006). Candida resistance and its clinical relevance. Pharmacotherapy 26, 68S–75S. doi:10.1592/phco.26.6part2.68S
Kobayashi, A., Takahashi, T., Horita, S., Yamamoto, I., Yamamoto, H., Teraoka, S., et al. (2010). Activation of the transcription factor c-Jun in acute cellular and antibody-mediated rejection after kidney transplantation. Hum. Pathol. 41, 1682–1693. doi:10.1016/j.humpath.2010.04.016
Langfelder, P., and Horvath, S. W. G. C. N. A. (2008). Wgcna: an R package for weighted correlation network analysis. BMC Bioinforma. 9, 559. doi:10.1186/1471-2105-9-559
Lebedenko, C. G., and Banerjee, I. A. (2021). Enhancing kidney vasculature in tissue engineering—current trends and approaches: A review. Biomimetics 6, 40. doi:10.3390/biomimetics6020040
Ledeganck, K. J., Gielis, E. M., Abramowicz, D., Stenvinkel, P., Shiels, P. G., and Van Craenenbroeck, A. H. (2019). MicroRNAs in AKI and kidney transplantation. Clin. J. Am. Soc. Nephrol. 14, 454–468. doi:10.2215/CJN.08020718
Li, B., Pu, K., and Wu, X. (2019). Identifying novel biomarkers in hepatocellular carcinoma by weighted gene co-expression network analysis. J. Cell. Biochem. 120, 11418–11431. doi:10.1002/jcb.28420
Li, C., and Xu, (2019). Feature selection with the Fisher score followed by the Maximal Clique Centrality algorithm can accurately identify the hub genes of hepatocellular carcinoma. Sci. Rep. 91 9, 17283–17311. doi:10.1038/s41598-019-53471-0
Liang, J. W., Fang, Z. Y., Huang, Y., Liuyang, Z. Y., Zhang, X. L., Wang, J. L., et al. (2018). Application of weighted gene Co-expression network analysis to explore the key genes in alzheimer’s disease. J. Alzheimer’s Dis. 65, 1353–1364. doi:10.3233/JAD-180400
Lin, S. M., Du, P., Huber, W., and Kibbe, W. A. (2008). Model-based variance-stabilizing transformation for Illumina microarray data. Nucleic Acids Res. 36, e11. doi:10.1093/nar/gkm1075
Liu, X., Hu, A. X., Zhao, J. L., and Chen, F. L. (2017). Identification of key gene modules in human osteosarcoma by Co-expression analysis weighted gene Co-expression network analysis (WGCNA). J. Cell. Biochem. 118, 3953–3959. doi:10.1002/jcb.26050
Liu, X., Liu, K., Wang, Z., Liu, C., Han, Z., Tao, J., et al. (2015). Advanced glycation end products accelerate arteriosclerosis after renal transplantation through the AGE/RAGE/ILK pathway. Exp. Mol. Pathol. 99, 312–319. doi:10.1016/j.yexmp.2015.07.009
Liu, X. S., Yang, J. W., Zeng, J., Chen, X. Q., Gao, Y., Kui, X. Y., et al. (2022). SLC2A1 is a diagnostic biomarker involved in immune infiltration of colorectal cancer and associated with m6A modification and ceRNA. Front. Cell. Dev. Biol. 10, 853596. doi:10.3389/fcell.2022.853596
Liu, Y., Shetty, A. C., Schwartz, J. A., Bradford, L. L., Xu, W., Phan, Q. T., et al. (2015). New signaling pathways govern the host response to C. albicans infection in various niches. Genome Res. 125, 679–689. doi:10.1101/gr.187427.114
Love, M. I., Huber, W., and Anders, S. (2014). Moderated estimation of fold change and dispersion for RNA-seq data with DESeq2. Genome Biol. 15, 550–621. doi:10.1186/s13059-014-0550-8
Maertens, A., Tran, V., Kleensang, A., and Hartung, T. (2018). Weighted gene correlation network analysis (WGCNA) reveals novel transcription factors associated with bisphenol A dose-response. Front. Genet. 9, 508. doi:10.3389/fgene.2018.00508
Meerwaldt, R., Zeebregts, C. J., Navis, G., Hillebrands, J. L., Lefrandt, J. D., and Smit, A. J. (2009). Accumulation of advanced glycation end products and chronic complications in ESRD treated by dialysis. Am. J. Kidney Dis. 53, 138–150. doi:10.1053/j.ajkd.2008.08.031
Mueller, A. J., Canty-Laird, E. G., Clegg, P. D., and Tew, S. R. (2017). Cross-species gene modules emerge from a systems biology approach to osteoarthritis. npj Syst. Biol. Appl. 3, 13–14. doi:10.1038/s41540-017-0014-3
Munro, D. A. D., and Davies, J. A. (2018). Vascularizing the kidney in the embryo and organoid: Questioning assumptions about renal vasculogenesis. J. Am. Soc. Nephrol. 29, 1593–1595. doi:10.1681/ASN.2018020179
Nishimoto, A. T., Sharma, C., and Rogers, P. D. (2020). Molecular and genetic basis of azole antifungal resistance in the opportunistic pathogenic fungus candida albicans. J. Antimicrob. Chemother. 75, 257–270. doi:10.1093/jac/dkz400
Oltean, S., Qiu, Y., Ferguson, J. K., Stevens, M., Neal, C., Russell, A., et al. (2015). Vascular endothelial growth factor-A165b is protective and restores endothelial glycocalyx in diabetic nephropathy. J. Am. Soc. Nephrol. 26, 1889–1904. doi:10.1681/ASN.2014040350
Pathomthongtaweechai, N., and Chutipongtanate, S. (2020). AGE/RAGE signaling-mediated endoplasmic reticulum stress and future prospects in non-coding RNA therapeutics for diabetic nephropathy. Biomed. Pharmacother. 131, 110655. doi:10.1016/j.biopha.2020.110655
Pendleton, K. M., Huffnagle, G. B., and Dickson, R. P. (2017). The significance of Candida in the human respiratory tract: Our evolving understanding. Pathog. Dis. 75, 29. doi:10.1093/femspd/ftx029
Perrin-Cocon, L., Vidalain, P. O., Jacquemin, C., Aublin-Gex, A., Olmstead, K., Panthu, B., et al. (2021). A hexokinase isoenzyme switch in human liver cancer cells promotes lipogenesis and enhances innate immunity. Commun. Biol. 41 4, 217–315. doi:10.1038/s42003-021-01749-3
Prasad, K., Dhar, I., Zhou, Q., Elmoselhi, H., Shoker, M., and Shoker, A. (2016). AGEs/sRAGE, a novel risk factor in the pathogenesis of end-stage renal disease. Mol. Cell. Biochem. 423, 105–114. doi:10.1007/s11010-016-2829-4
Puniya, B. L., Kulshreshtha, D., Verma, S. P., Kumar, S., and Ramachandran, S. (2013). Integrated gene co-expression network analysis in the growth phase of Mycobacterium tuberculosis reveals new potential drug targets. Mol. Biosyst. 9, 2798–2815. doi:10.1039/c3mb70278b
Ramirez-Garcia, A., Rementeria, A., Aguirre-Urizar, J. M., Moragues, M. D., Antoran, A., Pellon, A., et al. (2016). Candida albicans and cancer: Can this yeast induce cancer development or progression? Crit. Rev. Microbiol. 42, 181–193. doi:10.3109/1040841X.2014.913004
Ravichandran, S., Srivastav, S., Kamble, P. H., Chambial, S., Shukla, R., Sharma, P., et al. (2019). VEGF-A and cardiac autonomic function in newly diagnosed type 2 diabetes mellitus: A cross-sectional study at a tertiary care center. J. Fam. Med. Prim. Care 8, 3185–3190. doi:10.4103/jfmpc.jfmpc_537_19
Redding, S. W., Zellars, R. C., Kirkpatrick, W. R., McAtee, R. K., Caceres, M. A., Fothergill, A. W., et al. (1999). Epidemiology of oropharyngeal Candida colonization and infection in patients receiving radiation for head and neck cancer. J. Clin. Microbiol. 37, 3896–3900. doi:10.1128/JCM.37.12.3896-3900.1999
Revankar, S. G., Sanche, S. E., Dib, O. P., Caceres, M., and Patterson, T. F. (1998). Effect of highly active antiretroviral therapy on recurrent oropharyngeal candidiasis in HIV-infected patients. Aids 12, 2511–2513.
Rhodus, N. L., Bloomquist, C., Liljemark, W., and Bereuter, J. (1997). Prevalence, density, and manifestations of oral Candida albicans in patients with Sjogren’s syndrome. J. Otolaryngol. 26, 300–305.
Rodrigues, C. F., Rodrigues, M. E., and Henriques, M. (2019). Candida sp. infections in patients with diabetes mellitus. J. Clin. Med. 8, E76. doi:10.3390/jcm8010076
Romo, J. A., Zhang, H., Cai, H., Kadosh, D., Koehler, J. R., Saville, S. P., et al. (2019). Global transcriptomic analysis of the Candida albicans response to treatment with a novel inhibitor of filamentation. mSphere 4, 006200-e719. doi:10.1128/mSphere.00620-19
Rupaimoole, R., and Slack, F. J. (2017). MicroRNA therapeutics: Towards a new era for the management of cancer and other diseases. Nat. Rev. Drug Discov. 163 16, 203–222. doi:10.1038/nrd.2016.246
Sangeorzan, J. A., Bradley, S. F., He, X., Zarins, L. T., Ridenour, G. L., Tiballi, R. N., et al. (1994). Epidemiology of oral candidiasis in HIV-infected patients: Colonization, infection, treatment, and emergence of fluconazole resistance. Am. J. Med. 97, 339–346. doi:10.1016/0002-9343(94)90300-x
Schadt, E. E., Lamb, J., Yang, X., Zhu, J., Edwards, S., Guhathakurta, D., et al. (2005). An integrative genomics approach to infer causal associations between gene expression and disease. Nat. Genet. 37, 710–717. doi:10.1038/ng1589
Sha, H., Zhang, D., Zhang, Y., Wen, Y., and Wang, Y. (2017). ATF3 promotes migration and M1/M2 polarization of macrophages by activating tenascin-C via Wnt/β-catenin pathway. Mol. Med. Rep. 16, 3641–3647. doi:10.3892/mmr.2017.6992
Shen, H., Yu, H., Li, Q. Y., Wei, Y. T., Fu, J., Dong, H., et al. (2022). Hepatocyte-derived VEGFA accelerates the progression of non-alcoholic fatty liver disease to hepatocellular carcinoma via activating hepatic stellate cells. Acta Pharmacol. Sin. 43, 2917–2928. doi:10.1038/S41401-022-00907-5
Shoham, S., and Marr, K. A. (2012). Invasive fungal infections in solid organ transplant recipients. Future Microbiol. 7, 639–655. doi:10.2217/fmb.12.28
Sobel, J. D. (1985). Epidemiology and pathogenesis of recurrent vulvovaginal candidiasis. Am. J. Obstet. Gynecol. 152, 924–935. doi:10.1016/s0002-9378(85)80003-x
Sultan, A. S., Theofilou, V. I., Alfaifi, A., Montelongo-Jauregui, D., and Jabra-Rizk, M. A. (2022). Is Candida albicans an opportunistic oncogenic pathogen? PLoS Pathog. 18, e1010413. doi:10.1371/journal.ppat.1010413
Tang, J., Kong, D., Cui, Q., Wang, K., Zhang, D., Gong, Y., et al. (2018). Prognostic genes of breast cancer identified by gene co-expression network analysis. Front. Oncol. 8, 374. doi:10.3389/fonc.2018.00374
Thomas, G., Bain, J. M., Budge, S., Brown, A. J. P., and Ames, R. M. (2020). Identifying Candida albicans gene networks involved in pathogenicity. Front. Genet. 11, 375–412. doi:10.3389/fgene.2020.00375
Udan, R. S., Culver, J. C., and Dickinson, M. E. (2012). Understanding vascular development: WIRE developmental biology. Wiley Interdiscip. Rev. Dev. Biol. 2, 327–346. doi:10.1002/wdev.91
Vadovics, M., Ho, J., Igaz, N., Alfoldi, R., Rakk, D., Veres, E., et al. (2022). Candida albicans enhances the progression of oral squamous cell carcinoma in vitro and in vivo. MBio 13, e0314421. doi:10.1128/mBio.03144-21
Wang, G., Zeng, L., Huang, Q., Lu, Z., Sui, R., Liu, D., et al. (2021). Exploring the molecular mechanism of liuwei dihuang pills for treating diabetic nephropathy by combined network pharmacology and molecular docking. Evid. Based. Complement. Altern. Med. 2021, 7262208. doi:10.1155/2021/7262208
Wilflingseder, J., Reindl-Schwaighofer, R., Sunzenauer, J., Kainz, A., Heinzel, A., Mayer, B., et al. (2015). MicroRNAs in kidney transplantation. Nephrol. Dial. Transpl. 30, 910–917. doi:10.1093/ndt/gfu280
Willis, A. M., Coulter, W. A., Fulton, C. R., Hayes, J. R., Bell, P. M., and Lamey, P. J. (1999). Oral candidal carriage and infection in insulin-treated diabetic patients. Diabet. Med. 16, 675–679. doi:10.1046/j.1464-5491.1999.00134.x
Wu, Y., Li, Y. H., Yu, S. B., Li, W. G., Liu, X. S., Zhao, L., et al. (2016). A genome-wide transcriptional analysis of yeast-hyphal transition in Candida tropicalis by RNA-Seq. PLoS One 11, e0166645. doi:10.1371/journal.pone.0166645
Xu, H., Fang, T., Omran, R. P., Whiteway, M., and Jiang, L. (2020). RNA sequencing reveals an additional Crz1-binding motif in promoters of its target genes in the human fungal pathogen Candida albicans. Cell. Commun. Signal. 18, 1. doi:10.1186/s12964-019-0473-9
Yin, K., Zhang, Y., Zhang, S., Bao, Y., Guo, J., Zhang, G., et al. (2019). Using weighted gene co-expression network analysis to identify key modules and hub genes in tongue squamous cell carcinoma. Medicine 98, e17100. doi:10.1097/MD.0000000000017100
Yong, S. L., and Dutta, A. (2009). MicroRNAs in cancer. Annu. Rev. Pathol. 4, 199–227. doi:10.1146/annurev.pathol.4.110807.092222
Yu, G., Wang, L. G., Han, Y., and HeClusterProfiler, Q. Y. (2012). clusterProfiler: an R package for comparing biological themes among gene clusters. Omi. A J. Integr. Biol. 16, 284–287. doi:10.1089/omi.2011.0118
Zhang, G., Yao, X., Wang, C., Wang, D., and Wei, G. (2019). Transcriptome analysis reveals the mechanism underlying improved glutathione biosynthesis and secretion in Candida utilis during selenium enrichment. J. Biotechnol. 304, 89–96. doi:10.1016/j.jbiotec.2019.08.015
Zhu, G. D., Xie, L. M., Su, J. W., Cao, X. J., Yin, X., Li, Y. P., et al. (2022). Identification of differentially expressed genes and signaling pathways with Candida infection by bioinformatics analysis. Eur. J. Med. Res. 27, 43. doi:10.1186/s40001-022-00651-w
Ziello, J. E., Jovin, I. S., and Huang, Y. (2007). Hypoxia-inducible factor (HIF)-1 regulatory pathway and its potential for therapeutic intervention in malignancy and ischemia. Yale J. Biol. Med. 80, 51–60.
Zu, G., Sun, K., Zu, X., Han, T., and Huang, H. (2021). Mechanism of quercetin therapeutic targets for Alzheimer disease and type 2 diabetes mellitus. Sci. Rep. 111 11, 22959–23011. doi:10.1038/s41598-021-02248-5
Keywords: host-pathogen interaction, correlation network, RNA-sequencing, immune response, candida albican, WGCNA, transplantation
Citation: Naik S and Mohammed A (2022) Coexpression network analysis of human candida infection reveals key modules and hub genes responsible for host-pathogen interactions. Front. Genet. 13:917636. doi: 10.3389/fgene.2022.917636
Received: 11 April 2022; Accepted: 08 November 2022;
Published: 22 November 2022.
Edited by:
Mallana Gowdra Mallikarjuna, Indian Agricultural Research Institute (ICAR), IndiaReviewed by:
Shiyu Song, Nanjing University, ChinaLuis Antonio Pérez-García, Autonomous University of San Luis Potosí, Mexico
Copyright © 2022 Naik and Mohammed. This is an open-access article distributed under the terms of the Creative Commons Attribution License (CC BY). The use, distribution or reproduction in other forums is permitted, provided the original author(s) and the copyright owner(s) are credited and that the original publication in this journal is cited, in accordance with accepted academic practice. No use, distribution or reproduction is permitted which does not comply with these terms.
*Correspondence: Akram Mohammed, amoham18@uthsc.edu