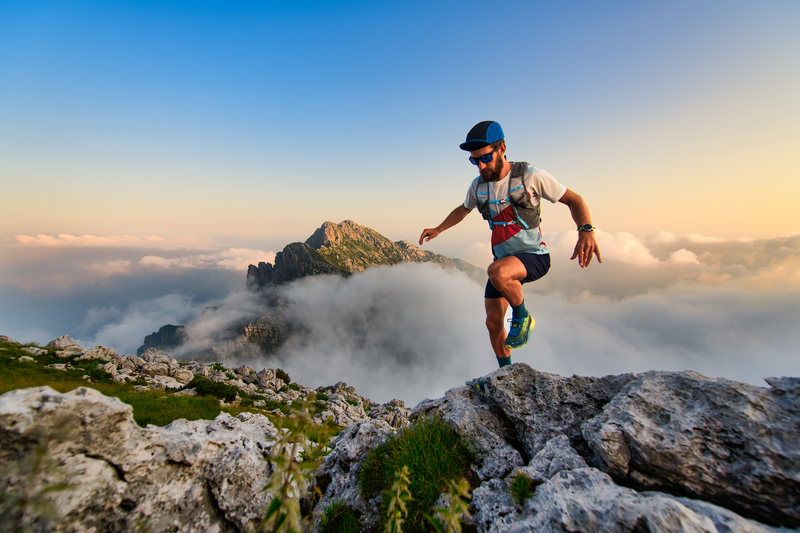
95% of researchers rate our articles as excellent or good
Learn more about the work of our research integrity team to safeguard the quality of each article we publish.
Find out more
ORIGINAL RESEARCH article
Front. Genet. , 26 August 2022
Sec. Computational Genomics
Volume 13 - 2022 | https://doi.org/10.3389/fgene.2022.911801
Non-small-cell lung cancer (NSCLC) is the second most common cancer worldwide, and most deaths are associated with epithelial–mesenchymal transition (EMT). Therefore, this study aimed to explore the role of EMT-related transcriptomic profiles in NSCLC and the effect of EMT-based signatures on clinical diagnosis, prognosis, and treatment responses for patients with NSCLC. After integrating the transcriptomics and clinicopathological data, we first constructed EMT clusters (C1 and C2) using machine learning algorithms, found the significant relationship between EMT clusters and survival outcomes, and then explored the impact of EMT clusters on the tumor heterogeneity, drug efficiency, and immune microenvironment of NSCLC. Prominently, differential-enriched tumor-infiltrated lymphocytes were found between EMT clusters, especially the macrophages and monocyte. Next, we identified the most significantly down-regulated gene SFTA2 in the EMT clusters C2 with poor prognosis. Using RT-qPCR and RNA-seq data from the public database, we found prominently elevated SFTA2 expression in NSCLC tissues compared with normal lung tissues, and the tumor suppressor role of SFTA2 in 82 Chinese patients with NSCLC. After Cox regression and survival analysis, we demonstrated that higher SFTA2 expression in tumor samples significantly predicts favorable prognosis of NSCLC based on multiple independent cohorts. In addition, the prognostic value of SFTA2 expression differs for patients with lung adenocarcinoma and squamous cell carcinoma. In conclusion, this study demonstrated that the EMT process is involved in the malignant progression and the constructed EMT clusters exerted significant predictive drug resistance and prognostic value for NSCLC patients. In addition, we first identified the high tumoral expression of SFTA2 correlated with better prognosis and could serve as a predictive biomarker for outcomes and treatment response of NSCLC patients.
Lung cancer is the second most common cancer worldwide (Akram et al., 2018). Non-small-cell lung cancer (NSCLC) accounts for 85% of all lung cancer cases, and the global incidence of NSCLC is estimated to be approximately 2 million new cases per year (Zhou et al., 2021). Most patients are diagnosed at an advanced stage of the cancer (Kwan and Chowdhury, 2021), suffering from a poor prognosis and high risk of death. With poor efficacy of monotherapy, targeted therapy with specific inhibitors is now the most promising first-line cancer therapy on the market (Osmani et al., 2018). Numerous studies related to the discovery of candidate biomarkers for lung cancer have been published (Gong et al., 2020). However, none of these biomarkers are widely used in clinical practices, and the function of these candidate signatures still lacks validation in well-designed large-scale studies (Rybarczyk-Kasiuchnicz et al., 2021).
The vast majority of cancer-related deaths, such as hepatocellular carcinoma and breast cancer (Saxena et al., 2021; Gao et al., 2022), are due to metastatic spread of cancer cells, a process aided by epithelial–mesenchymal transition (EMT) (Atay, 2020; Kitz et al., 2021). EMT refers to the biological process in which epithelial cells are transformed into cells with a mesenchymal phenotype through specific programs (Zhang et al., 2022). In the process of EMT, the polarity of epithelial cells is lost, contact with surrounding cells and stromal cells is reduced, interaction between cells is reduced, and ability of cell migration and motility is enhanced (Ramesh et al., 2020). At the same time, the cell phenotype changes and the expression level of E-cadherin decreased, resulting in reduced cell adhesion, allowing cells to acquire the characteristics of easy invasion and metastasis (Wu et al., 2020).
EMT also plays a very important role in the malignant evolution and migration capacities of tumors (Pastushenko and Blanpain, 2019; Xu et al., 2020a; Liu et al., 2021). EMT enables tumor cells in the primary site to acquire the ability to move and invade and also enables tumor cells to escape from apoptosis induced by certain factors, increasing their antiapoptotic ability (Singh et al., 2018; Bakir et al., 2020). Tumor cells exuding from blood vessels or lymphatics could revert to an epithelial state through mesenchymal–epithelial transition and then proliferate to form large and even macroscopic secondary tumors. For example, transient inhibition or overexpression of fatty acid synthase significantly regulated kidney cancer cells proliferation and migration by regulating the EMT process (Xu et al., 2020b). Many EMT-related signaling pathways and transcription factors have been confirmed to be involved in the drug resistance of NSCLC (Mahmood et al., 2017). Inhibition of tumor EMT signaling can delay tumor drug resistance, thereby improving efficacy and reducing toxicity (Chae et al., 2018; Wu et al., 2021). Therefore, this study aimed to investigate the effect of EMT on NSCLC and the EMT-related hub gene. We speculate that the expression of the EMT pathway is related to the prognosis and clinical treatments in NSCLC patients.
Raw counts of RNA-sequencing data and corresponding clinical information from 1,000 NSCLC patients were obtained from The Cancer Genome Atlas (TCGA) database (https://portal.gdc.cancer.gov/) as a training cohort. After we excluded patients with unclear clinicopathological information and missing overall survival information, we finally included 1,000 NSCLC patients with present survival, clinicopathological information, and transcriptomic data. In addition, 1,144 NSCLC patients with clinical follow-up information were enrolled in GSE19188, GSE30219, GSE29013, GSE50081, and GSE37745 cohorts from the Gene Expression Omnibus (GEO) data sets (https://www.ncbi.nlm.nih.gov/geo/) as testing cohorts. In addition, we enrolled 82 NSCLC patients with available survival and clinicopathological data from tissue bank of the Affiliated Hospital of Youjiang Medical University for Nationalities (Youjiang cohort, Youjiang, China) as a validation cohort.
Among 1,000 patients with NSCLC from the TCGA database, we integrated the level 3 RNA-seq data, extracted expression profiles of EMT process, and collected corresponding clinical and pathological data. Performing a consistency analysis using “ConsensusClusterPlus” and “pheatmap” R package, we observed the best unsupervised groups (less than 6) and performed clustering heat maps according to the progression-free survival. The gene expression heatmap retains genes with SD > 0.1. If the number of input genes is more than 1,000, it will extract the top 25% genes after sorting the SD.
We obtained a variety of immune infiltrating lymphocytes with different degrees of infiltration using the CIBERSORT algorithm (https://cibersort.stanford.edu) (Rusk, 2019). The differentially infiltrated lymphocytes were evaluated using the Wilcoxon rank sum test. The Tumor Immune Dysfunction and Exclusion (TIDE) and the semi-inhibitory concentration (IC50) tests were used to detect tumor heterogeneity between the two groups (Yang et al., 2013; Jiang et al., 2018).
A TissueScan® qPCR cDNA array analysis (Origene, Rockville, MD, United States) was performed on 48 human tissues using SFTA2-specific primers, according to the manufacturer’s protocol. RNA was extracted from lung cancer and adjacent normal lung tissues, and a SFTA2 q-PCR analysis was performed with normalization for GAPDH. Primers used were SFTA2 forward: GGAGTCTTTTCTGACAAATTCCTC and reverse: GGTGTTGAGATCTTGCATGGTGG.
The Kaplan–Meier method was used to assess the significance of progression-free survival (PFS) and overall survival (OS) by using the Kaplan–Meier plotter (http://kmplot.com/analysis/index). Univariate and multivariate Cox regression analyses were performed to analyze the impact of SFTA2 expression, age, gender, and pathological TNM stages on overall survival. The forest was used to show the p-value, HR, and 95% CI of each variable through the “forestplot” R package.
To illustrate the statistically significance of differential expressed genes (DEGs) between EMT clusters, the “Limma” R package was used to compare the differences among NSCLC samples with threshold as follows: |Log2 (fold change)| > 1 and p-value < 0.05. Functional enrichment of all DEGs was performed using the KEGG database in a bubble chart.
All statistical analyses were performed on R software and GraphPad Prism (Version 8.0). Group comparisons were determined by two-tailed t test and one-way ANOVA. Spearman’s correlation analysis was applied to determine significant correlation between linear variables. A survival analysis was visualized using the Kaplan–Meier curves. A univariate Cox regression analysis was performed using the R package “survival”. All tests with p-values < 0.05 were considered statistically significance.
Based on 1,000 patients with NSCLC from the TCGA cohort, with unsupervised clustering, we prominently divided the samples into EMT Cluster1 and EMT Cluster 2, according to the EMT activity of NSCLC samples (Figures 1A,B). In order to further compare the differences between C1 group and C2, we selected some clinical and pathological factors, such as gender, race, TNM stage, and smoking status (Figure 1C). As shown in Table 1, there were significantly increased samples of progression, female, advanced pathological TNM stages, and non-smoking in EMT C2. Interestingly, we found more patients with smoking history in C2 than those in C1, suggesting that smoking is not significantly related to the EMT pathway in NSCLC patients.
FIGURE 1. Construction of EMT clusters (C1 and C2) using machine learning algorithms. (A) The principal component analysis (PCA) was applied to explore differences in the expressed genes between the two groups (C1 and C2). Unsupervised clustering was used to classify NSCLC patients into groups (C1 and C2). (B) The heatmap of consistent clustering results shows the expression of EMT in each sample in C1 and C2 groups. Red represents high expression and blue represents low expression. (C) We investigated the distribution of some clinical characteristics in the two groups and analyzed significant p-values by using the chi-square test. (D) Kaplan–Meier survival curve analysis using median patient samples.
Moreover, a progression-free survival analysis showed that the prognosis of the group with EMT C1 was markedly better (log-rank p = 3.31e-05, HR = 0.629), with median time of 6.1 month in C1 and 3.0 month in C2 (Figure 1D). In addition, we then evaluated the differential expression of traditional EMT markers, including CDH1, CDH2, CLDN1, SNAI1, SNAI2, TGFB1, TWIST1, VIM, ZEB1, and ZEB2, between the EMT clusters. The findings suggested significant elevated expression of CDH1, CLND1, SNAI2, TGFB1, and TWIST1 in the EMT Cluster 1 and significant increased expression of CDH2, SNAI1, VIM, ZEB1, and ZEB2 in EMT Cluster 2 (Supplementary Figure S1). Overall, EMT clusters significantly correlated with clinicopathological indicators and predicts PFS in 1000 NSCLC patients from the TCGA database.
After understanding the differential activity of the EMT process in EMT clusters, we focused on the impact of EMT clusters on tumor immune microenvironment and clinical treatment. We screened the aggregation of important immune cells in both groups and found that activity of macrophage M1 cells and resting mast cells were significantly higher in C2. However, in the C1 group, there were significantly enriched macrophage M2, monocytes, and activated mast cell (Figure 2A). Since M1 mainly secretes proinflammatory factors and M2 expression inhibits inflammatory factors, which plays a role in inhibiting inflammatory response and tissue repair, the better prognosis of the EMT C1 group may be related to the immune-excluded microenvironment. Though the C1 has a better prognosis and a more positive immune response, we compared and analyzed immune checkpoints and found that the C2 had higher expression of immune checkpoints, such as CTLA2, HAVCR2, LAG3, PDCD1, TIGIT, and SIGLEC15 (Figure 2B). These finding suggested that immunotherapy could be a potential treatment selection for NSCLC patients in the EMT C2 group.
FIGURE 2. EMT clusters divide differential tumor-infiltrated lymphocytes and immune microenvironment. (A) We found the connection between different immune cells and EMT. Red represents positive correlation while blue represents negative correlation. (B) Expression distribution of immune checkpoint molecules in C1 and C2 groups.
The TIDE score showed that the score of C2 was significantly lower than that in the C1 group, indicating that the EMT cluster 2 samples had lower heterogeneity and all tumor cells could have strong sensitivity to the given treatment (Figure 3). In addition, patients in EMT cluster 2 could receive favorable responses to immune checkpoint inhibitors and easier to obtain precise treatment strategies. Then we evaluated the effect of targeted therapy (first-generation EGFR tyrosine kinase inhibitors) and chemotherapy using the semi-inhibitory concentration (IC50) method, an important indicator for evaluating drug efficacy or sample treatment response (version cgp 2016). As shown in Supplementary Figure 2, the results indicated that the IC50 values of EGFR tyrosine kinase inhibitors gefitinib, erlotinib, and cisplatin in EMT Cluster 1 were significantly higher lower than EMT Cluster 2 (p < 0.0001), revealing that NSCLC patients in EMT Cluster one received better clinical responses to tyrosine kinase inhibitors or chemotherapy.
FIGURE 3. Intratumoral heterogeneity of NSCLC. The TIDE test was used to predict response to immune checkpoint inhibition therapy.
Comparing the differential transcriptional profiles of EMT clusters, we listed down-regulated and up-regulated DEGs in EMT C2 (Supplementary Table S1). The most significantly down-regulated gene is SFTA2, a tumor suppressor gene, and the significantly up-regulated genes included KRT5, CLCA2, TP63, and so on in the volcano and clustering plot (Figure 4A). A functional enrichment analysis with samples from the KEGG database showed that the up-regulated genes were mainly involved in signaling pathways regulating pluripotency of stem cells and the cell cycle, while the down-regulated genes were mainly involved in Staphylococcus aureus infection, complement and coagulation cascades, and cell adhesion molecules pathway (Figure 4B).
FIGURE 4. Function enrichment analysis of EMT clusters and the identification of tumor-suppressor SFTA2. (A) The graphs show the differentially expressed genes in the C1 and C2 groups, with up-regulated genes in red and down-regulated genes in blue. (B) We performed functional enrichment analysis to show the pathways involved in differentially up-regulated and down-regulated genes in the KEGG database.
Furthermore, we compared the expression level of SFTA2 in tumor and normal tissues. To further illustrate the accuracy and reliability of the biomarker, we analyzed the expression level of the SFTA2 in human organs, and the results showed that the SFTA2 expression was most significantly expressed in lungs, followed by the esophagus (Supplementary Figures S3A,B).
We then found that the expression of SFAT2 was significantly lower in tumor than that in normal tissues in NSCLC and squamous cell carcinoma (LUSC) cohorts, whereas the expression in adenocarcinoma (LUAD) was lower in normal tissue (Figure 5A). Table 2 shows the association of SFTA2 with clinicopathological factors, with increased samples of progression, female, non-white, advanced pTNM stage, and smoking in the SFTA2 high expression group. Next, we performed univariate and multivariate Cox regression analyses enrolling clinical and pathological indicators. The results suggested that the T stage, N stage, and SFTA2 were significantly associated with patient prognosis, indicating that SFTA2 could be a valuable independent biomarker of NSCLC (Figure 5B). Finally, with multiple independent cohorts and integrated cohort from the GEO database, we found that patients in the high SFTA2 expression group experienced better outcomes than that of SFTA2 low expression in cohorts like GSE30219 (n = 293, p = 0.0022, HR = 0.57), GSE33745 (n = 196, p = 0.01, HR = 0.64), GSE50081 (n = 181, p = 0.023, HR = 0.5), and GSE19188 (n = 82, p = 0.059, HR = 0.56) (Figure 5C).
FIGURE 5. Potential implications of SFTA2 expression in outcomes of NSCLC. (A) We compared the expression of SFTA2 in normal and tumor tissues in NSCLC and subgroups of LUAD and LUSC. (B) We performed univariate and multivariate regression analyses to find the factors related to the survival of NSCLC patients. (C) We utilized independent cohort samples to study the relationship between SFTA2 expression and survival prognosis.
TABLE 2. Clinical and pathological features according to the differential SFTA2 expression in 1,000 NSCLC patients from the TCGA database.
Next, we assessed mRNA expression of STFA2 in NSCLC tissues and normal tissues. The results validated our previous findings and showed significantly elevated expression level of SFTA2 in 47 paired tumor compared with normal tissues (p < 0.001) (Figure 6A). Then a total of 82 patients from the Youjiang cohort were enrolled in this study to validate our hypothesis. The clinical and pathological baseline data for patients with NSCLC in line with SFTA2 expression is shown in Supplementary Table S2. We found that SFTA2 serves as a significant tumor suppressor gene and could significantly predict prognosis for patients with lung adenocarcinoma (Figure 6B). The Kaplan–Meier survival analysis and the log-rank test indicated that low expression of SFTA2 significantly predicted poor OS for 82 Chinese patients with NSCLC from the real-world Jiaxing cohort (p = 0.0212, HR = 0.431). In addition, for patients with lung adenocarcinoma from the TCGA database, we found that decreased SFTA2 expression was not markedly associated with PFS (p = 0.059) while significantly predicted poorer OS (p = 0.0066). For patients with lung squamous cell carcinoma, elevated SFTA2 expression was not markedly associated with PFS (p = 0.310) while significantly predicted poorer OS (p = 0.01) (Supplementary Figure S4)
FIGURE 6. Expression and prognostic validation of SFTA2 expression in outcomes of NSCLC patients from the real-world cohort. (A) We compared the expression of SFTA2 in normal tissues (n = 47) and NSCLC (n = 47) tissues using Student’s t test. (B) Kaplan–Meier survival analysis and log-rank test reveal the prognostic value of SFTA2 expression in 82 patients from the Jiaxing cohort.
As one of the most common cancers in the world, lung cancer is characterized by late diagnosis, poor prognosis, and high mortality (Dundr et al., 2021; Zhao et al., 2022; Zheng et al., 2022). NSCLC is more common compared to small-cell lung cancer (SCLC), accounting for 85% of lung cancer patients (Fintelmann et al., 2015). Since there is no cure for NSCLC, the best supportive treatment is usually used for palliative treatment (NSCLC Meta-Analyses Collaborative Group, 2008). In NSCLC, driver mutations that activate the EGFR represent the most common actionable therapeutic alteration. EGFR tyrosine kinase inhibitors (TKIs) are the standard of care for first-line treatment of advanced or metastatic EGFR-mutant NSCLC. Early-stage treatment modalities include first-generation (1G; erlotinib, gefitinib), second-generation (2G; afatinib, dacomitinib), or third-generation (3G; osimertinib) EGFR TKIs, alone or in combination with other therapies. However, despite a high response rate of 80%, resistance inevitably develops after a median of 10–17 months (1–4 months) (Zhong et al., 2013).
The most common mechanism of resistance to 1G and 2G EGFR TKIs is the EGFR T790M “gatekeeper” mutation, which occurs in 50%–60% of patients (Yang et al., 2022a). Osimertinib is highly selective for T790M and was originally developed and approved for the treatment of T790M-positive (T790M+) resistance (Yang et al., 2022b). The mechanisms of resistance in the remaining patients, as well as those treated with 3G EGFR TKIs, are thought to be diverse and include activation of alternative signaling pathways, such as MET or HER2 amplification, or phenotypic changes of the EMT process, which is also a key cellular phenomenon involved in tumor metastasis and progression (Erin et al., 2020; Vokes et al., 2022). Overall, T790M-negative (T790M−) EGFR TKIs resistance patients remain a population with significant precision medical need.
It has been revealed that the EMT pathway and related transcription factors have been confirmed to be involved in cancer progression and resistance of EGFR TKIs NSCLC patients (Li et al., 2018; Meyer-Schaller et al., 2019). Therefore, we first divided the patients into two groups: C1 and C2. The study found that the difference between the EMT C1 and C2 groups was significant and was related to factors such as gender and clinical T and N stages. More importantly, we are the first to discover that prognosis of NSCLC patients with significantly associated with EMT. Since studies have shown that EMT cells can promote immune exclusion and deviation (Romeo et al., 2019). We then studied the immune cells related to EMT and the effects of EMT on the immune checkpoints. It was found that there were more active immune cells in the EMT C1 group, corresponding to the better prognosis of patients, while in the EMT C2 group, the expression of immune checkpoints was high, indicating that these patients might have better effect of receiving specific target immunotherapy.
Previous studies have interrogated the transcriptional profiles of TKI-resistant tumors and have suggested fundamental transcriptional differences in T790M+ and T790M− resistance mechanisms. Notably, the expression of lung adenocarcinoma marker genes such as SFTA2 and SFTA3 was widespread and almost completely lost in cancer cells of T790M− tumors (Kim et al., 2020; Chua et al., 2021). In order to explore the deeper association mechanism between the EMT and the prognosis of NSCLC, we compared the DEGs in the two groups and found that SFTA2 was significantly down-regulated in the C2 group. The overall expression of SFTA2 in lung cancer samples was lower in tumor tissues than that in normal lung tissues, and the low expression of SFTA2 was significantly related to the poor progression-free survival of NSCLC patients. Interestingly, Mittal et al. (2012) characterized SFTA2 as a novel secretory peptide highly expressed in the lung and is modulated by lipopolysaccharide. SFTA2 was also identified as prognostic genes with significantly correlation with the pathological stages of pancreatic ductal adenocarcinoma in an integrated transcriptome meta-analysis (Atay, 2020). So far, we have verified that SFTA2 gene could be used as an independent signature to predict the prognosis and TKIs resistance of lung cancer.
However, there still exist some limitations of this study. We did not include real-world cohort samples to study the comparison of EMT in tumor tissues and normal tissues, but we analyzed the differences between the EMT C1 and C2 groups and the corresponding prognosis through a large number of independent cohorts, revealing the significance of EMT in the prognosis of the patient.
In conclusion, this study revealed the EMT process involved in the malignant progression of NSCLC. The construction of EMT clusters showed significant predictive value for NSCLC patients. In addition, we first identified the high expression of SFTA2 associated with better prognosis could serve as predictive biomarker for outcomes, dysregulation of TME, and immunotherapy response for NSCLC patients.
The original contributions presented in the study are included in the article/Supplementary Material; further inquiries can be directed to the corresponding authors.
A written informed consent was obtained from the individual(s) for the publication of any potentially identifiable images or data included in this article.
Conceptualization, data curation, and formal analysis: NL, ZZ, and XL. Investigation, methodology, resources, and software: NL, ZZ, and XL. Supervision, validation, and visualization: NL and ZZ. Original draft and Editing: NL, ZZ, and XL.
We are grateful to all patients for their dedicated participation in the current study. We are grateful to the grants from the Key Discipline of Jiaxing Respiratory Medicine Construction Project (No.2019-zc-04).
The authors declare that the research was conducted in the absence of any commercial or financial relationships that could be construed as a potential conflict of interest.
All claims expressed in this article are solely those of the authors and do not necessarily represent those of their affiliated organizations, or those of the publisher, the editors, and the reviewers. Any product that may be evaluated in this article, or claim that may be made by its manufacturer, is not guaranteed or endorsed by the publisher.
The Supplementary Material for this article can be found online at: https://www.frontiersin.org/articles/10.3389/fgene.2022.911801/full#supplementary-material
Akram, A., Khalil, S., Halim, S. A., Younas, H., Iqbal, S., and Mehar, S. (2018). Therapeutic uses of HSP90 inhibitors in non-small cell lung carcinoma (NSCLC). Curr. Drug Metab. 19 (4), 335–341. doi:10.2174/1389200219666180307122441
Atay, S. (2020). Integrated transcriptome meta-analysis of pancreatic ductal adenocarcinoma and matched adjacent pancreatic tissues. PeerJ 8, e10141. doi:10.7717/peerj.10141
Bakir, B., Chiarella, A. M., Pitarresi, J. R., and Rustgi, A. K. (2020). EMT, MET, plasticity, and tumor metastasis. Trends Cell Biol. 30 (10), 764–776. doi:10.1016/j.tcb.2020.07.003
Chae, Y., Chang, S., Ko, T., Anker, J., Agte, S., Iams, W., et al. (2018). Epithelial-mesenchymal transition (EMT) signature is inversely associated with T-cell infiltration in non-small cell lung cancer (NSCLC). Sci. Rep. 8 (1), 2918. doi:10.1038/s41598-018-21061-1
Chua, K. P., Teng, Y. H. F., Tan, A. C., Takano, A., Alvarez, J. J. S., Nahar, R., et al. (2021). Integrative profiling of T790M-negative EGFR-mutated NSCLC reveals pervasive lineage transition and therapeutic opportunities. Clin. Cancer Res. 27 (21), 5939–5950. doi:10.1158/1078-0432.CCR-20-4607
Dundr, P., Matej, R., Nemejcova, K., Bartu, M., and Struzinska, I. (2021). Predictive testing in non-small cell lung carcinoma. Klin. Onkol. 34, 29–34. doi:10.48095/ccko2021S29
Erin, N., Grahovac, J., Brozovic, A., and Efferth, T. (2020). Tumor microenvironment and epithelial mesenchymal transition as targets to overcome tumor multidrug resistance. Drug Resist. Updat. 53, 100715. doi:10.1016/j.drup.2020.100715
Fintelmann, F., Bernheim, A., Digumarthy, S. R., Lennes, I. T., Kalra, M. K., Gilman, M. D., et al. (2015). The 10 pillars of lung cancer screening: rationale and logistics of a lung cancer screening program. Radiographics 35 (7), 1893–1908. doi:10.1148/rg.2015150079
Gao, Z., Zhong, M., Ye, Z., Wu, Z., Xiong, Y., Ma, J., et al. (2022). PAK3 promotes the metastasis of hepatocellular carcinoma by regulating EMT process. J. Cancer 13 (1), 153–161. doi:10.7150/jca.61918
Gong, B., Kao, Y., Zhang, C., Sun, F., Gong, Z., and Chen, J. (2020). Identification of hub genes related to carcinogenesis and prognosis in colorectal cancer based on integrated bioinformatics. Mediat. Inflamm. 2020, 5934821. doi:10.1155/2020/5934821
Jiang, P., Gu, S., Pan, D., Fu, J., Sahu, A., Hu, X., et al. (2018). Signatures of T cell dysfunction and exclusion predict cancer immunotherapy response. Nat. Med. 24 (10), 1550–1558. doi:10.1038/s41591-018-0136-1
Kim, N., Kim, H. K., Lee, K., Hong, Y., Cho, J. H., Choi, J. W., et al. (2020). Single-cell RNA sequencing demonstrates the molecular and cellular reprogramming of metastatic lung adenocarcinoma. Nat. Commun. 11 (1), 2285. doi:10.1038/s41467-020-16164-1
Kitz, J., Lefebvre, C., Carlos, J., Lowes, L. E., and Allan, A. L. (2021). Reduced Zeb1 expression in prostate cancer cells leads to an aggressive partial-EMT phenotype Associated with altered global methylation patterns. Int. J. Mol. Sci. 22 (23), 12840. doi:10.3390/ijms222312840
Kwan, T., and Chowdhury, E. (2021). Clinical outcomes of chemotherapeutic molecules as single and multiple agents in advanced non-small-cell lung carcinoma (NSCLC) patients. Med. Kaunas. Lith. 57 (11), 1252. doi:10.3390/medicina57111252
Li, C., Shen, Z., Zhou, Y., and Yu, W. (2018). Independent prognostic genes and mechanism investigation for colon cancer. Biol. Res. 51 (1), 10. doi:10.1186/s40659-018-0158-7
Liu, J., Cui, G., Shen, S., Gao, F., Zhu, H., and Xu, Y. (2021). Establishing a prognostic signature based on epithelial-mesenchymal transition-related genes for endometrial cancer patients. Front. Immunol. 12, 805883. doi:10.3389/fimmu.2021.805883
Mahmood, M., Ward, C., Muller, H. K., Sohal, S. S., and Walters, E. H. (2017). Epithelial mesenchymal transition (EMT) and non-small cell lung cancer (NSCLC): a mutual association with airway disease. Med. Oncol. 34 (3), 45. doi:10.1007/s12032-017-0900-y
Meyer-Schaller, N., Cardner, M., Diepenbruck, M., Saxena, M., Tiede, S., Luond, F., et al. (2019). A hierarchical regulatory landscape during the multiple stages of EMT. Dev. Cell 48 (4), 539–553. doi:10.1016/j.devcel.2018.12.023
Mittal, R. A., Hammel, M., Schwarz, J., Heschl, K. M., Bretschneider, N., Flemmer, A. W., et al. (2012). SFTA2--a novel secretory peptide highly expressed in the lung--is modulated by lipopolysaccharide but not hyperoxia. PLoS One 7 (6), e40011. doi:10.1371/journal.pone.0040011
NSCLC Meta-Analyses Collaborative Group (2008). Chemotherapy in addition to supportive care improves survival in advanced non-small-cell lung cancer: a systematic review and meta-analysis of individual patient data from 16 randomized controlled trials. J. Clin. Oncol. 26 (28), 4617–4625. doi:10.1200/JCO.2008.17.7162
Osmani, L., Askin, F., Gabrielson, E., and Li, Q. K. (2018). Current WHO guidelines and the critical role of immunohistochemical markers in the subclassification of non-small cell lung carcinoma (NSCLC): moving from targeted therapy to immunotherapy. Semin. Cancer Biol. 52, 103–109. doi:10.1016/j.semcancer.2017.11.019
Pastushenko, I., and Blanpain, C. (2019). EMT transition states during tumor progression and metastasis. Trends Cell Biol. 29 (3), 212–226. doi:10.1016/j.tcb.2018.12.001
Ramesh, V., Brabletz, T., and Ceppi, P. (2020). Targeting EMT in cancer with repurposed metabolic inhibitors. Trends Cancer 6 (11), 942–950. doi:10.1016/j.trecan.2020.06.005
Romeo, E., Caserta, C. A., Rumio, C., and Marcucci, F. (2019). The vicious cross-talk between tumor cells with an EMT phenotype and cells of the immune system. Cells 8 (5), E460. doi:10.3390/cells8050460
Rybarczyk-Kasiuchnicz, A., Ramlau, R., and Stencel, K. (2021). Treatment of brain metastases of non-small cell lung carcinoma. Int. J. Mol. Sci. 22 (2), E593. doi:10.3390/ijms22020593
Saxena, M., Hisano, M., Neutzner, M., Diepenbruck, M., Ivanek, R., Sharma, K., et al. (2021). The long non-coding RNA ET-20 mediates EMT by impairing desmosomes in breast cancer cells. J. Cell Sci. 134, jcs258418. doi:10.1242/jcs.258418
Singh, M., Yelle, N., Venugopal, C., and Singh, S. K. (2018). EMT: mechanisms and therapeutic implications. Pharmacol. Ther. 182, 80–94. doi:10.1016/j.pharmthera.2017.08.009
Vokes, N. I., Chambers, E., Nguyen, T., Coolidge, A., Lydon, C. A., Le, X., et al. (2022). Concurrent TP53 mutations facilitate resistance evolution in EGFR-mutant lung adenocarcinoma. J. Thorac. Oncol. 17 (6), 779–792. doi:10.1016/j.jtho.2022.02.011
Wu, T., Tang, C., Tao, R., Yong, X., Jiang, Q., and Feng, C. (2021). PD-L1-Mediated immunosuppression in oral squamous cell carcinoma: Relationship with macrophage infiltration and epithelial to mesenchymal transition markers. Front. Immunol. 12, 693881. doi:10.3389/fimmu.2021.693881
Wu, X., Xin, Z., Zou, Z., Lu, C., Yu, Z., Feng, S., et al. (2020). SRY-related high-mobility-group box 4: Crucial regulators of the EMT in cancer. Semin. Cancer Biol. 67, 114–121. doi:10.1016/j.semcancer.2019.06.008
Xu, H., Xu, W. H., Ren, F., Wang, J., Wang, H. K., Cao, D. L., et al. (2020). Prognostic value of epithelial-mesenchymal transition markers in clear cell renal cell carcinoma. Aging (Albany NY) 12 (1), 866–883. doi:10.18632/aging.102660
Xu, W., Hu, X., Anwaier, A., Wang, J., Liu, W., Tian, X., et al. (2020). Fatty acid synthase correlates with prognosis-related abdominal adipose distribution and metabolic disorders of clear cell renal cell carcinoma. Front. Mol. Biosci. 7, 610229. doi:10.3389/fmolb.2020.610229
Yang, J. C., Ohe, Y., Chiu, C-H., Ou, X., Cantarini, M., Jänne, P. A., et al. (2022). Osimertinib plus selumetinib in EGFR-mutated non-small cell lung cancer after progression on EGFR-TKIs: a phase ib, open-label, multicenter trial (TATTON Part B). Clin. Cancer Res., OF1–OF10. doi:10.1158/1078-0432.CCR-21-4329
Yang, J. C., Ohe, Y., Chiu, C-H., Ou, X., Cantarini, M., Jänne, P. A., et al. (2022). Osimertinib plus selumetinib in EGFR-mutated, non-small cell lung cancer after progression on EGFR-TKIs: a phase 1b, open-label, multicenter trial (TATTON Part B). Clin. Cancer Res., OF1–OF10. doi:10.1158/1078-0432.CCR-21-4329
Yang, W., Soares, J., Greninger, P., Edelman, E. J., Lightfoot, H., Forbes, S., et al. (2013). Genomics of drug sensitivity in cancer (GDSC): a resource for therapeutic biomarker discovery in cancer cells. Nucleic Acids Res. 41, D955–D961. doi:10.1093/nar/gks1111
Zhang, G., Li, Z., Dong, J., Zhou, W., Zhang, Z., Que, Z., et al. (2022). Acacetin inhibits invasion, migration and TGF-β1-induced EMT of gastric cancer cells through the PI3K/Akt/Snail pathway. BMC Complement. Med. Ther. 22 (1), 10. doi:10.1186/s12906-021-03494-w
Zhao, Y., Sun, P., Xiao, J., Jin, L., Ma, N., Li, Z., et al. (2022). International patterns and trends of childhood and adolescent cancer, 1978-2012. J. Natl. Cancer Cent. 2 (2), 78–89. doi:10.1016/j.jncc.2022.02.001
Zheng, R., Zhang, S., Zeng, H., Wang, S., Sun, K., Chen, R., et al. (2022). Cancer incidence and mortality in China, 2016. J. Natl. Cancer Cent. 2, 1–9. doi:10.1016/j.jncc.2022.02.002
Zhong, C., Liu, H., Jiang, L., Zhang, W., and Yao, F. (2013). Chemotherapy plus best supportive care versus best supportive care in patients with non-small cell lung cancer: a meta-analysis of randomized controlled trials. PLoS One 8 (3), e58466. doi:10.1371/journal.pone.0058466
Keywords: epithelial–mesenchymal transition (EMT), non-small-cell lung cancer (NSCLC), SFTA2, prognosis, tumor microenvironment
Citation: Li N, Zhai Z, Chen Y and Li X (2022) Transcriptomic and immunologic implications of the epithelial–mesenchymal transition model reveal a novel role of SFTA2 in prognosis of non-small-cell lung carcinoma. Front. Genet. 13:911801. doi: 10.3389/fgene.2022.911801
Received: 03 April 2022; Accepted: 21 July 2022;
Published: 26 August 2022.
Edited by:
Hong Zhu, Zhejiang University, ChinaReviewed by:
Xiaofeng Liu, Tianjin Medical University Cancer Institute and Hospital, ChinaCopyright © 2022 Li, Zhai, Chen and Li. This is an open-access article distributed under the terms of the Creative Commons Attribution License (CC BY). The use, distribution or reproduction in other forums is permitted, provided the original author(s) and the copyright owner(s) are credited and that the original publication in this journal is cited, in accordance with accepted academic practice. No use, distribution or reproduction is permitted which does not comply with these terms.
*Correspondence: Yuanbiao Chen, MjMxODQyMTI2MEBxcS5jb20=; Xiaofeng Li, bGl4aWFvZmVuZzIwMTAxMkAxMjYuY29t
Disclaimer: All claims expressed in this article are solely those of the authors and do not necessarily represent those of their affiliated organizations, or those of the publisher, the editors and the reviewers. Any product that may be evaluated in this article or claim that may be made by its manufacturer is not guaranteed or endorsed by the publisher.
Research integrity at Frontiers
Learn more about the work of our research integrity team to safeguard the quality of each article we publish.