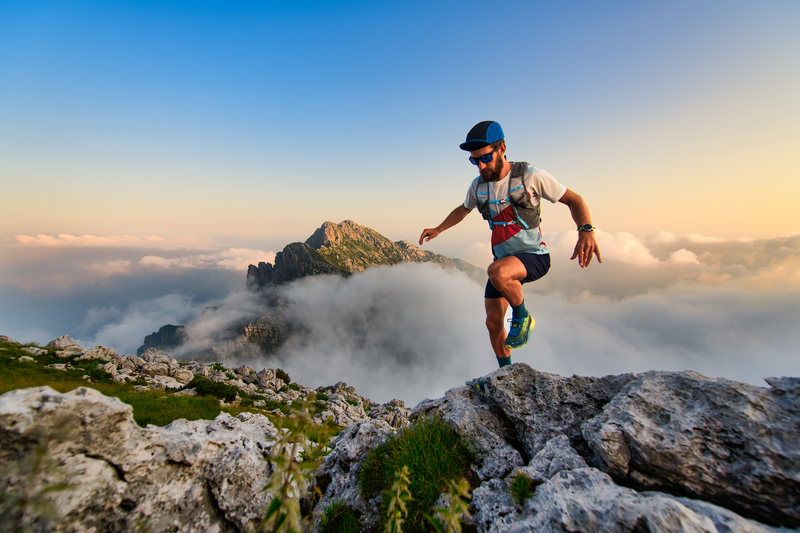
95% of researchers rate our articles as excellent or good
Learn more about the work of our research integrity team to safeguard the quality of each article we publish.
Find out more
EDITORIAL article
Front. Genet. , 31 March 2022
Sec. Human and Medical Genomics
Volume 13 - 2022 | https://doi.org/10.3389/fgene.2022.896032
This article is part of the Research Topic Clinical Genome Sequencing: Bioinformatics Challenges and Key Considerations View all 6 articles
Editorial on the Research Topic
Clinical Genome Sequencing: Bioinformatics Challenges and Key Considerations
Next generation sequencing (NGS) has been increasingly used to generate mutation, transcriptome and epigenomic profiles, as well demonstrated by The Cancer Genome Atlas (TCGA) (Tomczak et al., 2015) and the International Cancer Genome Consortium (ICGC) in major cancer types (Milius et al., 2014). It is evident that utilizing NGS-based omics data, individually or in combination, along with clinical metadata, can foster the development of robust biomarkers, such as tumor mutational burden, gene mutation and expression signature, and the classification of disease subtypes, thus benefiting patients in diagnosis, risk evaluation and potentially individualized therapy. In practice, however, prioritization on causal variants and genes still faces key challenges in data processing, harmonization, and clinical interpretation. Misinterpretation of genetic testing results remains a major bottleneck in cases of challenges (Farmer et al., 2021). This topic covers research articles that, as we described below, aimed to identify potentially functional variants and genes, or to build models for risk prediction.
Nomogram is a predictive model that is widely used to predict individual’s risk of recurrence, metastases and overall survival (Balachandran et al., 2015). To build a nomogram for early-stage hepatocellular carcinoma (HCC), Huang et al. downloaded transcriptome, mutation and clinical data for patients from a single cohort in TCGA and another four in ICGC. Cox regression analysis identified seven significant variables, including mutation status of TP53, MACF1, EYS and DOCK2, that were used to build the nomogram. The patients were then divided into low-versus high-risk group, with the former being associated with a better overall survival. Focused analysis of the cohort from TCGA revealed clear differences between the two risk groups in the abundance for seven of the 22 tumor-infiltrating hematopoietic cell subpopulations (Newman et al., 2015); also, the low-risk group had significantly lower Tumor Immune Dysfunction and Exclusion (TIDE) scores (Jiang et al., 2018), suggestive of a better immunotherapy response. This study demonstrated a risk stratification nomogram that is potentially linked to the infiltrating immune cell composition in HCC.
Starting with a public RNA-seq data of 117 Ewing sarcoma (ES) patients, Zhou et al. first calculated, for each sample, an immune enrichment score across each of the 28 infiltrating immune cell subpopulations (Jia et al., 2018), followed by unsupervised sample clustering. Two clusters with the highest and lowest overall score were retained. Of the differentially expressed genes (DEGs) between the two clusters, 862 formed a distinct immune-related module that showed the strongest negative correlation with immune score (estimated via the ESTIMATE package). About 10% (85 genes) were DEGs between normal skeletal muscle tissue and ES. They focused on NPM1 (nucleophosmin 1) involved in DNA repair and cell proliferation, showing that its mRNA and protein expression levels were markedly higher in ES cell lines compared to mesenchymal stem cells. The higher mRNA expression correlated with lower immune score, TIDE score and PD-L1 expression, as well as worse prognosis in ES. Importantly, NSC348884, a nucleophosmin inhibitor (Qi et al., 2008), can induce apoptosis in treated ES cells. This work recapitulates the previous finding that NPM1, a drug-targetable gene, is a prognostic biomarker in ES (Kikuta et al., 2009).
Through total RNA and miRNA sequencing, Wang et al. identified mRNAs, IncRNAs, and miRNAs differentially expressed between acute myeloid leukemia (AML) patients and healthy subjects. They used RAID, a comprehensive RNA-associated interaction database (Yi et al., 2017), to predict mRNAs and lncRNAs targeted by the differential miRNAs. The analysis revealed a potential network of the top 25 hub mRNAs with 15 miRNAs and 12 lncRNAs, including at least four mRNAs and two lncRNAs that are associated with overall survival. Notably, the expression of CCL5 and lncRNA UCA1, known to play key roles in the proliferation of AML, correlated with the fraction of infiltrating immune and stromal cells (Yoshihara et al., 2013). The analysis also revealed a novel interaction between UCA1 and miR-16-5p, expanding the known UCA1-miRNA crosstalk in AML (Sun et al., 2018). Together, this study supports CCL5 and UCA1 as potential diagnostic biomarkers in AML.
Biomarker discovery often relies on the integration of different datasets. In ulcerative colitis (UC), Chen et al. selected six microarray gene expression data from GEO, including 22–162 patients and 11–21 controls. After batch effect correction, 231–436 DEGs were identified from each dataset, with only 79 DEGs in common by a simple intersection approach. To effectively integrate the results, the authors applied the robust rank aggregation (RRA) method, which is robust to outliers and noises (Kolde et al., 2012), on the ranked DEG lists. Of the 208 RRA-identified DEGs, six hub genes were selected and confirmed to be upregulated in a UC mouse model. Indeed, these six genes are known to be associated with UC. Thus, to extract biological signatures shared across multiple datasets, one should consider robust meta-analysis approaches for high reproducibility.
Finally, Shestak et al. reported the genetic test of a 14-year-old female athlete, who was suspected to have long QT syndrome (LQTS). WES identified a rare mutation (c.647C > T, p. S216L, chr3:38655522-38655522) in the non-canonical exon 6 of SCN5A. SCN5A is a cardiac ion channel gene implicated in multiple cardiac diseases, with conclusive evidence for its causation in congenital LQTS (Adler et al., 2020). The clinical report, however, mistook this variant for the one previously reported in the canonical exon 6 (c.647C > T, p. S216L, chr3:38655290-38655290) (Marangoni et al., 2011), leading to misinterpretation. Subsequent Sanger sequencing confirmed a lack of mutation in canonical exon 6. Two more tests were ordered, and both identified the mutation only in the non-canonical exon 6. First, DNA was sequenced in a targeted panel of 11 genes including SCN5A, followed by Sanger sequencing validation. Second, Sanger sequencing revealed the mutation in the mother, but not in the father. The variant was classified as benign, suggesting negative result of the genetic testing. This study highlights the importance of variant validation. Obviously, the collaboration between clinicians and bioinformaticians is vital for genetic counseling. With the ongoing efforts, we are expecting the development of systems for accurately prioritizing causal variants and genes in accelerating biomarker discovery.
All authors listed have made a substantial contribution to the work and approved it for publication.
This work is supported by the Mayo Clinic Center for Individualized Medicine.
The authors declare that the research was conducted in the absence of any commercial or financial relationships that could be construed as a potential conflict of interest.
All claims expressed in this article are solely those of the authors and do not necessarily represent those of their affiliated organizations, or those of the publisher, the editors and the reviewers. Any product that may be evaluated in this article, or claim that may be made by its manufacturer, is not guaranteed or endorsed by the publisher.
Adler, A., Novelli, V., Amin, A. S., Abiusi, E., Care, M., Nannenberg, E. A., et al. (2020). An International, Multicentered, Evidence-Based Reappraisal of Genes Reported to Cause Congenital Long QT Syndrome. Circulation 141, 418–428. doi:10.1161/circulationaha.119.043132
Balachandran, V. P., Gonen, M., Smith, J. J., and Dematteo, R. P. (2015). Nomograms in Oncology: More Than Meets the Eye. Lancet Oncol. 16, e173–e180. doi:10.1016/s1470-2045(14)71116-7
Farmer, M. B., Bonadies, D. C., Pederson, H. J., Mraz, K. A., Whatley, J. W., Darnes, D. R., et al. (2021). Challenges and Errors in Genetic Testing. Cancer J. 27, 417–422. doi:10.1097/ppo.0000000000000553
Jia, Q., Wu, W., Wang, Y., Alexander, P. B., Sun, C., Gong, Z., et al. (2018). Local Mutational Diversity Drives Intratumoral Immune Heterogeneity in Non-small Cell Lung Cancer. Nat. Commun. 9, 5361. doi:10.1038/s41467-018-07767-w
Jiang, P., Gu, S., Pan, D., Fu, J., Sahu, A., Hu, X., et al. (2018). Signatures of T Cell Dysfunction and Exclusion Predict Cancer Immunotherapy Response. Nat. Med. 24, 1550–1558. doi:10.1038/s41591-018-0136-1
Kikuta, K., Tochigi, N., Shimoda, T., Yabe, H., Morioka, H., Toyama, Y., et al. (2009). Nucleophosmin as a Candidate Prognostic Biomarker of Ewing's Sarcoma Revealed by Proteomics. Clin. Cancer Res. 15, 2885–2894. doi:10.1158/1078-0432.ccr-08-1913
Kolde, R., Laur, S., Adler, P., and Vilo, J. (2012). Robust Rank Aggregation for Gene List Integration and Meta-Analysis. Bioinformatics 28, 573–580. doi:10.1093/bioinformatics/btr709
Marangoni, S., Di Resta, C., Rocchetti, M., Barile, L., Rizzetto, R., Summa, A., et al. (2011). A Brugada Syndrome Mutation (p.S216L) and its Modulation by p.H558R Polymorphism: Standard and Dynamic Characterization. Cardiovasc. Res. 91, 606–616. doi:10.1093/cvr/cvr142
Milius, D., Dove, E. S., Chalmers, D., Dyke, S. O. M., Kato, K., Nicolás, P., et al. (2014). The International Cancer Genome Consortium's Evolving Data-protection Policies. Nat. Biotechnol. 32, 519–523. doi:10.1038/nbt.2926
Newman, A. M., Liu, C. L., Green, M. R., Gentles, A. J., Feng, W., Xu, Y., et al. (2015). Robust Enumeration of Cell Subsets from Tissue Expression Profiles. Nat. Methods 12, 453–457. doi:10.1038/nmeth.3337
Qi, W., Shakalya, K., Stejskal, A., Goldman, A., Beeck, S., Cooke, L., et al. (2008). NSC348884, a Nucleophosmin Inhibitor Disrupts Oligomer Formation and Induces Apoptosis in Human Cancer Cells. Oncogene 27, 4210–4220. doi:10.1038/onc.2008.54
Sun, M. D., Zheng, Y. Q., Wang, L. P., Zhao, H. T., and Yang, S. (2018). Long Noncoding RNA UCA1 Promotes Cell Proliferation, Migration and Invasion of Human Leukemia Cells via Sponging miR-126. Eur. Rev. Med. Pharmacol. Sci. 22, 2233–2245. doi:10.26355/eurrev_201804_14809
Tomczak, K., Czerwińska, P., and Wiznerowicz, M. (2015). Review the Cancer Genome Atlas (TCGA): an Immeasurable Source of Knowledge. Wspólczesna Onkologia 1A, 68–77. doi:10.5114/wo.2014.47136
Yi, Y., Zhao, Y., Li, C., Zhang, L., Huang, H., Li, Y., et al. (2017). RAID v2.0: an Updated Resource of RNA-Associated Interactions across Organisms. Nucleic Acids Res. 45, D115–d118. doi:10.1093/nar/gkw1052
Keywords: bioinformatics, biomarker, next-generation sequencing, nomogram, RNA sequencing, microarray, whole-exome sequencing
Citation: Tian S, Tu ZJ, Yan H and Klee EW (2022) Editorial: Clinical Genome Sequencing: Bioinformatics Challenges and Key Considerations. Front. Genet. 13:896032. doi: 10.3389/fgene.2022.896032
Received: 14 March 2022; Accepted: 16 March 2022;
Published: 31 March 2022.
Edited and reviewed by:
Stephen J. Bush, University of Oxford, United KingdomCopyright © 2022 Tian, Tu, Yan and Klee. This is an open-access article distributed under the terms of the Creative Commons Attribution License (CC BY). The use, distribution or reproduction in other forums is permitted, provided the original author(s) and the copyright owner(s) are credited and that the original publication in this journal is cited, in accordance with accepted academic practice. No use, distribution or reproduction is permitted which does not comply with these terms.
*Correspondence: Shulan Tian, dGlhbi5zaHVsYW5AbWF5by5lZHU=; Eric W. Klee, S2xlZS5FcmljQG1heW8uZWR1
Disclaimer: All claims expressed in this article are solely those of the authors and do not necessarily represent those of their affiliated organizations, or those of the publisher, the editors and the reviewers. Any product that may be evaluated in this article or claim that may be made by its manufacturer is not guaranteed or endorsed by the publisher.
Research integrity at Frontiers
Learn more about the work of our research integrity team to safeguard the quality of each article we publish.