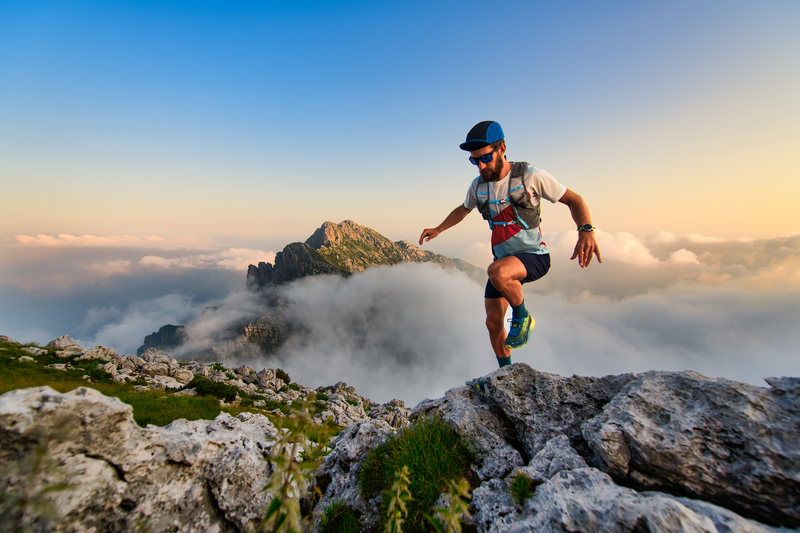
94% of researchers rate our articles as excellent or good
Learn more about the work of our research integrity team to safeguard the quality of each article we publish.
Find out more
ORIGINAL RESEARCH article
Front. Genet. , 12 July 2022
Sec. Genetics of Common and Rare Diseases
Volume 13 - 2022 | https://doi.org/10.3389/fgene.2022.890007
Objective: To explore potential causal genetic variants and genes underlying the pathogenesis of uterine leiomyomas (ULs).
Methods: We conducted the summary data-based Mendelian randomization (SMR) analyses and performed functional mapping and annotation using FUMA to examine genetic variants and genes that are potentially involved in the pathogenies of ULs. Both analyses used summarized data of a recent genome-wide association study (GWAS) on ULs, which has a total sample size of 244,324 (20,406 cases and 223,918 controls). We performed separate SMR analysis using CAGE and GTEx eQTL data.
Results: Using the CAGE eQTL data, our SMR analysis identified 13 probes tagging 10 unique genes that were pleiotropically/potentially causally associated with ULs, with the top three probes being ILMN_1675156 (tagging CDC42, PSMR = 8.03 × 10−9), ILMN_1705330 (tagging CDC42, PSMR = 1.02 × 10−7) and ILMN_2343048 (tagging ABCB9, PSMR = 9.37 × 10−7). Using GTEx eQTL data, our SMR analysis did not identify any significant genes after correction for multiple testing. FUMA analysis identified 106 independent SNPs, 24 genomic loci and 137 genes that are potentially involved in the pathogenesis of ULs, seven of which were also identified by the SMR analysis.
Conclusions: We identified many genetic variants, genes, and genomic loci that are potentially involved in the pathogenesis of ULs. More studies are needed to explore the exact underlying mechanisms in the etiology of ULs.
Uterine leiomyomas (ULs), also called myomas or uterine fibroids, are benign tumors in the smooth muscle tissue in myometrium (Bulun, 2013; Stewart et al., 2016). The overall prevalence of UL is about 70% in women of reproductive age, and approximately 25% of UL patients suffer from apparent clinical symptoms and require treatment (Stewart et al., 2017). ULs are the most prevalent benign tumor in female reproductive tract and the leading indication for hysterectomy. ULs represent a major cause of morbidity in women of childbearing age and account for excessive menstrual bleeding, pelvic pain or pressure, infertility, and pregnancy complications (Commandeur et al., 2015). To date, the only definitive treatment for ULs, including the familial subtype, is hysterectomy, which creates a great challenge if fertility preservation is desired. ULs also cause tremendous economic burden. For example, the annual cost of ULs in the US alone, including direct medical costs and indirect financial losses, is estimated to be up to $34.4 billion, higher than the combined cost of breast and colon cancer (Cardozo et al., 2012).
UL is a complex, multi-factorial gynecological benign disease with highly variable tumor size, tumor location and clinical manifestations. Many factors have been reported to be associated with the risk of ULs, including biological, demographic, reproductive and lifestyle factors (Parazzini, 2006; Sparic et al., 2016; Wise and Laughlin-Tommaso, 2016). Furthermore, previous studies also suggested that genetics plays an important role in the pathogenesis of ULs. For example, African-American women, or generally women with African origin, are more predisposed to develop ULs, with a prevalence as high as 80% (Day Baird et al., 2003), suggesting that ethnicity-specific factors, potentially ethnicity-specific genetic structure, may underlie the pathogenesis of ULs. Familial clustering between first-degree relatives and twins was also observed as well as multiple inherited syndromes in which fibroid development occurred (Luoto et al., 2000; Tomlinson et al., 2002). Moreover, many genome-wide association study (GWAS) and candidate gene studies have identified several genetic variants/loci associated with the susceptibility of ULs (Cha et al., 2011; Hellwege et al., 2017; Rafnar et al., 2018; Välimäki et al., 2018; Edwards et al., 2019; Gallagher et al., 2019). However, the role of putative risk factors and the underlying biological mechanisms underpinning ULs remain largely unclear, which has contributed to the slow progress in the development of effective treatment options for ULs. More studies are needed to explore genetic variants/genes that are potentially causally associated with ULs to better understand the pathogenesis of ULs.
Mendelian randomization (MR) uses genetic variants as the proxy to randomization. Recently, it has been widely adopted to explore pleiotropic/potentially causal effect of an exposure on various outcomes (e.g., ULs) (Davey Smith and Hemani, 2014). Confounding and reverse causation, which are commonly encountered in traditional association studies, can be greatly reduced by MR. This method has been successful in identifying gene expression probes or DNA methylation loci that are pleiotropically/potentially causally associated with various phenotypes, such as neuropathologies of Alzheimer’s disease and severity of COVID-19 (Liu et al., 2021a; Liu et al., 2021b).
In this paper, we attempted to prioritize genes that are potentially causally associated with ULs through a summary data-based MR (SMR) approach. We also performed functional mapping and annotation to further explore genetic variants and genomic loci that are potentially involved in the pathogenesis of ULs.
The GWAS summarized data for ULs were provided by a recent genome-wide association meta-analysis of ULs (Gallagher et al., 2019). The results were based on meta-analyses of ULs using data from four population-based cohorts (Women’s Genome Health Study, United Kingdom Biobank, Queensland Institute of Medical Research, and North Finnish Birth Cohort), with a total sample size of 244,324 (20,406 cases and 223,918 controls). Genotyping was done on different platforms, and imputation was performed using the reference panel from the 1000 Genomes Project European dataset (1000G EUR) Phase 3 or the Haplotype Reference Consortium (HRC) panel. For each cohort, logistic regression or linear mixed model association analysis was done, assuming an additive genetic model and adjusting for age, BMI, and/or the first five principal components, and/or array type, as appropriate. The GWAS summarized data can be downloaded at http://ftp.ebi.ac.uk/pub/databases/gwas/summary_statistics/GCST009001-GCST010000/GCST009158/.
The SMR analyses used cis-eQTL genetic variants as the instrumental variables (IVs) for gene expression. We performed separate SMR analysis using eQTL data from two sources. Specifically, we used the CAGE eQTL summarized data for whole blood (Lloyd-Jones et al., 2017), which included 2,765 participants, and the V7 release of the GTEx eQTL summarized data for uterus, which included 70 participants (Consortium, 2020). The eQTL data can be downloaded at https://cnsgenomics.com/data/SMR/#eQTLsummarydata.
The SMR analyses, based on the principle of MR (Davey Smith and Ebrahim, 2003), used cis-eQTL as the IV, gene expression as the exposure and ULs as the outcome. It was essentially a two-sample MR analysis which jointly analyzed summarized GWAS data and eQTL data from different samples to test for pleiotropic association between gene expression and a ULs. Detailed information regarding the SMR method was reported elsewhere (Zhu et al., 2016). The analyses were done using the software SMR, and we followed an approach similar to the one adopted in our previous publication (Liu et al., 2021b). The existence of linkage in the observed association was assessed using the heterogeneity in dependent instruments (HEIDI) test. PHEIDI<0.05 means rejection of the null hypothesis. That is, the observed association could be due to two distinct genetic variants in high linkage disequilibrium with each other. We adopted the default settings in SMR [e.g., PeQTL <5 × 10−8 and minor allele frequency (MAF) > 0.01] and used false discovery rate (FDR) to adjust for multiple testing (for full details of the default settings, see Supplementary Table S1). The SMR analytic process is illustrated in Figure 1.
FIGURE 1. Flow chart for the SMR analyses. (A) SMR analysis using CAGE eQTL data from blood; and (B) SMR analysis using GTEx eQTL data. CAGE, Consortium for the Architecture of Gene Expression; eQTL, expression quantitative trait loci; GWAS, genome--wide association studies; GTEx, Genotype--Tissue Expression; LD, linkage disequilibrium; SMR, summary data--based Mendelian randomization; SNP, single nucleotide polymorphism.
To better understand the genetic mechanisms underlying ULs, we also conducted a FUMA analysis to functionally map and annotate the genetic association, again using the GWAS summarized results of ULs. FUMA is an on-line platform that integrates information from multiple resources for easy implementation of post-GWAS analysis, such as functional annotation and gene prioritization (Watanabe et al., 2017). It has two processes, SNP2GENE, which annotates SNPs regarding their biological functions and maps them to genes, and GENE2FUNC, which annotates the mapped genes in biological contexts. In SNP2GENE, we performed both positional mapping and eQTL mapping using GTEx v8 of whole blood and uterus. We selected all types of genes in gene prioritization and adopted the default settings otherwise (e.g., maximum p-value of lead SNPs being 5 × 10−8 and r2 threshold for independent significant SNPs being 0.6). In GENE2FUNC, we adopted the default settings (e.g., using FDR to correct for multiple testing in the gene-set enrichment analysis).
Data cleaning and statistical/bioinformatical analysis was performed using R version 4.1.2 (https://www.r-project.org/), SMR (https://cnsgenomics.com/software/smr/), and FUMA (https://fuma.ctglab.nl/).
In the SMR analyses, the CAGE eQTL has a much larger number of participants than that of the GTEx eQTL data (2,765 vs. 70), so is the number of eligible probes (8,523 vs. 999). After checking allele frequencies among the datasets and LD pruning, there were more than six million eligible SNPs in each SMR analysis. In the FUMA analysis, about 8.6 million SNPs were used as the input. The detailed information was shown in Table 1.
Using the CAGE eQTL data, our SMR analysis identified 13 probes tagging 10 unique genes that were pleiotropically/potentially causally associated with ULs, with the top three probes being ILMN_1675156 (tagging CDC42, PSMR = 8.03 × 10−9), ILMN_1705330 (tagging CDC42, PSMR = 1.02 × 10−7) and ILMN_2343048 (tagging ABCB9, PSMR = 9.37 × 10−7; Table 2). There were three probes tagging CDC42 (Figure 2) and two probes tagging ABCB9 (Figure 3) that showed significant pleiotropic association with ULs. Using GTEx eQTL data, we did not identify any genes that were pleiotropically/potentially causally associated with ULs after correction for multiple testing (Table 2).
TABLE 2. The top hit probes identified in SMR analysis*.
FIGURE 2. Pleiotropic association of CDC42 with ULs using CAGE eQTL. Top plot, grey dots represent the --log10(p values) for SNPs from the GWAS of ULs, with solid rhombuses indicating that the probes pass HEIDI test. Middle plot, eQTL results. Bottom plot, location of genes tagged by the probes. CAGE, Consortium for the Architecture of Gene Expression; eQTL, expression quantitative trait loci; GWAS, genome--wide association studies; GTEx, Genotype--Tissue Expression; HEIDI, heterogeneity in dependent instruments; SMR, summary data--based Mendelian randomization; SNP, single nucleotide polymorphism; ULs, uterine leiomyomas.
FIGURE 3. Pleiotropic association of ABCB9 and MPHOSPH9 with ULs using CAGE eQTL data. Top plot, grey dots represent the log10(p values) for SNPs from the GWAS of ULs, with solid rhombuses indicating that the probes pass HEIDI test. Middle plot, eQTL results. Bottom plot, location of genes tagged by the probes. CAGE, Consortium for the Architecture of Gene Expression; eQTL, expression quantitative trait loci; GWAS, genome--wide association studies; GTEx, Genotype--Tissue Expression; HEIDI, heterogeneity in dependent instruments; SMR, summary data--based Mendelian randomization; SNP, single nucleotide polymorphism; ULs, uterine leiomyomas.
FUMA analysis identified 106 independent significant SNPs, 33 lead SNPs (Supplementary Tables S2–S4), and 24 genomic risk loci (Figure 4; Supplementary Table S5). In addition, FUMA identified 137 genes that were potentially involved in the pathogenesis of ULs (Supplementary Table S6). These 137 genes are distributed in 20 genomic risk loci, with four genomic risk loci containing no identified genes (Figure 4 and Supplementary Table S6). Of the 137 identified genes, 7 were also identified by SMR analysis, including CDC42, SLC38A1, ABCB9, MPHOSPH9, SBNO1, MRPS31 and CD68. Expression of the prioritized genes in 30 tissues can be found in Supplementary Table S7 and Supplementary Figure S1.
FIGURE 4. Genetic risk loci identified by FUMA analysis using GWAS data on ULs. Genomic risk loci are displayed in the format of “chromosome:start position--end position” on the Y axis. For each genomic locus, histograms from left to right depict the size, the number of candidate SNPs, the number of mapped genes (using positional mapping and eQTL mapping), and the number of genes known to be located within the genomic locus, respectively. CAGE, Consortium for the Architecture of Gene Expression; eQTL, expression quantitative trait loci; GWAS, genome--wide association studies; GTEx, Genotype--Tissue Expression; SNP, single nucleotide polymorphism; ULs, uterine leiomyomas.
Gene-set enrichment analysis (GSEA) was undertaken to test the possible biological mechanisms of the 137 candidate genes implicated in ULs (Supplementary Table S8). A total of 96 gene sets with an adjusted p < 0.05 were identified. We found strong enrichment signals related with uterine fibroids (adjusted p = 2.00 × 10−51). In addition, we also found enrichment of sex-related signals such as GO_REGULATION_OF_GONAD_DEVELOPMENT (adjusted p = 0.023), endometriosis (adjusted p = 7.3 × 10−4), sex hormone-binding globulin levels (adjusted p = 0.003), and sex hormone levels (adjusted p = 0.024; Supplementary Table S8).
In this study, we conducted SMR and FUMA analysis to prioritize SNPs and genes to better understand the genetic mechanisms underlying ULs. We identified multiple genetic variants, genes, genomic risk loci and gene sets that may be involved in the pathogenesis of ULs. These findings provided helpful leads to a better understanding of the pathogenesis of ULs and highlight potential therapeutic targets for the treatment of ULs.
Several probes tagging CDC42 (cell division control protein 42 homolog) showed significant pleiotropic association with ULs in the SMR analysis using CAGE eQTL data (Table 2). This gene was also identified by the FUMA analysis. CDC42 is a member of the Rho family and is implicated in a variety of cellular functions including cell cycle progression, survival, transcription, actin cytoskeleton organization, and membrane trafficking (Huang et al., 2019). CDC42 has been linked to multiple human cancers and is involved in the initiation of many cellular responses during oncogenic processes, such as transition from epithelial to mesenchymal, cell-cycle progression, migration/invasion, tumor growth, angiogenesis, and oncogenic transformation (Qadir et al., 2015; Maldonado and Dharmawardhane, 2018). Several studies reported that CDC42 might also play an essential role in the pathogenesis of fibroid. For example, genome-wide analysis revealed that the 1p36.12 region, where CDC42/WNT4 is located, was associated with uterine fibroids (Rafnar et al., 2018; Edwards et al., 2019; Gallagher et al., 2019). Interestingly, the genetic variant rs10917151 in CDC42/WNT4 seems to have ancestry-specific effect on the risk of uterine fibroids. Specifically, the A allele was associated with a reduced risk of uterine fibroids in women of African ancestry (OR = 0.84) and an increased risk in women of European ancestry (OR = 1.16) (Edwards et al., 2019). Since rs10917151 has been reported to be involved in hormone-related traits (e.g., endometriosis and endometrial cancer), it probably plays a role in the development of leiomyomas via influencing hormone metabolism (Rafnar et al., 2018). Meanwhile, another study showed that the deregulation of CDC42 influences fibroblasts activation which is essential in the pathogenesis of ULs (Zheng et al., 2014). Given the fact that increased cellular proliferation is present in fibroid compared with the adjacent uterine tissue and the function of CDC42 in influencing cell cycle, further investigation is needed to elucidate the role of CDC42 in the development of leiomyoma and the potential of this gene as a promising target for the prevention and treatment of ULs.
We also found that two probes tagging ABCB9 (ATP binding cassette subfamily B member 9) showed significant pleotropic association with ULs in the SMR analysis using CAGE eQTL data. This gene was also identified in the FUMA analysis. ABCB9 belongs to the superfamily of ATP-binding cassette (ABC) transporters which fulfill diverse physiological functions in different cellular localizations ranging from plasma membrane to intracellular membranous compartments (Linton, 2007). ABCB9, located on 12q24.31, is an antigen processing-like (TAPL) transporter that has been found to be involved in the development and progression of various malignant tumors, such as ovarian cancer and non-small cell lung cancer (Dong et al., 2014; Ween et al., 2015). The genetic variant rs2270788 in ABCB9 was found to be associated with both the risk and tumor size of ULs in African American participants (Aissani et al., 2015). ABCB9 was downregulated in women with a high level of progesterone serum (>1.5 ng/ml), compared with women with a lower level of progesterone serum (<1.5 ng/ml) (Labarta et al., 2011). Since progesterone is a major promoter of leiomyoma development and growth (Kim and Sefton, 2012), the role of ABCB9 in fibroids in general, and its function in progesterone-driven growth of leiomyomas in particular, needs further exploration.
A recent GWAS study on ULs also performed two-sample Mendelian randomization analysis (Gallagher et al., 2019). However, the MR analysis was different from our SMR analyses in that their objective was to examine the causality of genetic association between UL and heavy menstrual bleeding (HMB). Their study identified 29 independent loci for ULs, with 27 of them on the autosomal chromosomes while our FUMA analysis identified a total of 24 genomic loci on the autosomal chromosomes. The definition of genomic locus was different between their approach and FUMA: their genomic locus was defined as regions of the genome containing all SNPs in LD (r2 > 0.6) with the index SNPs (independent SNPs, i.e., SNPs in low LD (r2 < 0.1) with nearby (≤500 kb) significantly associated SNPs), with any adjacent regions within 250 kb of one another being combined and classified as a single locus. In FUMA, independent significant SNPs (p < 5 × 10−8 and independent from each other at r2 < 0.6) were first identified. Then, all known SNPs in LD (r2 > 0.6) with one of the independent SNPs were included, using the pre-calculated LD structure based on 1000G. As a results, SNPs that were not originally in the GWAS results could also be included. This may partly explain the difference in the findings.
Findings from our SMR and FUMA analyses are not consistent, although we did identify several genes that were significant in both the SMR and FUMA analyses (Table 2 and Supplementary Table S3). This is likely due to the different focuses of the two types of analyses. The SMR analyses attempted to identify genes whose expressions were causally associated with ULs by using genetic variants as the instrumental variables (Zhu et al., 2016). By contrast, the FUMA analysis focused on genetic variants to characterize and annotate significant SNPs which were then mapped to genes for subsequent functional annotation by integrating knowledge from multiple resources (Watanabe et al., 2017). In one word, SMR focused on potentially causal association of gene expression, while FUMA attempted to pinpoint the most likely causal genetic variants and genes without differentiating whether the causal function is through gene expression or not.
There are three core assumptions underlying the SMR analyses: 1) the genotype is associated with gene expression; 2) the genotype is not associated with confounding factors that bias the associations between gene expression and ULs; and 3) the genotype is related to ULs only via its association with gene expression. Concern is minimal, moderate or cannot be directly verified for these assumptions. Specifically, for assumption 1, the SMR analyses adopted a p-value threshold of 5 × 10−8 to select the top associated eQTL. Therefore, we believe that the genetic variants we selected indeed showed strong association with gene expression and the concern of weak instrument is minimal. Assumption 2 is often based on biological belief that the genotype will not be associated with socioeconomic and behavioral characteristics that commonly confound the effects of exposure (i.e., gene expression) on the outcome (i.e., ULs) (Lawlor et al., 2008). This assumption could not be verified directly because we used summarized data for the SMR analyses. Violation of assumption 3 (i.e., horizontal pleiotropy) may distort MR results. It was found that horizontal pleiotropy can be detected in over 48% significant causal relationships in MR and introduced distortions as high as 201% in the causal estimates in MR. It can induce false-positive causal relationships in up to 10% of relationships (Verbanck et al., 2018). We did observe that the HEIDI test was significant for some of the observed associations, implying the existence of horizontal pleiotropy (Table 2). Caution should be exerted in interpretation of the findings in the presence of pleiotropy.
Our study has limitations. Although we identified multiple genes, such as CDC42 and ABCB9, in pleiotropic association with ULs, we cannot directly compare expression of the identified genes between ULs patients and the normal control due to a lack of relevant gene expression data. Future studies are needed to examine changes in gene expression of the identified genes and further explore the possible pathogenic mechanisms. The incidence of ULs varies among ethnicities, implying the existence of ethnic-specific genetic architecture. However, the GWAS summarized data used in our analyses were based on participants of European ancestry. Moreover, ethnicity-specific gene expression and eQTL data are unavailable. As such, our findings might not be generalized to other ethnicities, and more studies comparing expression of the identified genes among different ethnicities are warranted. Previous research demonstrated that the SMR approach had good performance with a sample size of 1,000 and 10,000 for QTL summarized data and GWAS summarized data, respectively (Zhu et al., 2016). Therefore, power should not be a big concern for the SMR analysis using CAGE eQTL data which utilized eQTL data from 2,765 subjects and GWAS summarized data from 244,324 subjects. However, the SMR analysis using GTEx eQTL data might suffer from insufficient power with eQTL data from only 70 subjects. The number of eligible probes used in the SMR analyses was limited, especially in the analysis using GTEx eQTL (eligible probes = 999). We further checked and found that there were only 482 probes that were common in both GTEx eQTL data and the CAGE eQTL data, and none of the significant probes identified by using the CAGE eQTL data were included in the eligible probes in the GTEx eQTL data. Taken together, we believe that limited sample size and eligible probes was the major reason of null findings of the SMR analysis using GTEx eQTL data, and that we could not rule out the possibility of missing some important genes that were not tagged in the eQTL data. In addition, the FDR approach to correct for multiple testing resulted in additional possibilities of missing important genes.
We identified many genetic variants, genes and genomic loci that are potentially involved in the pathogenesis of ULs. Biological validation of some of the identified genes is highly needed in future research, especially of the seven genes that were identified by both the SMR analysis using CAGE eQTL data and the FUMA analysis.
All data generated or analyzed during this study are publicly available as specified in the methods section of this paper. Specifically, the eQTL data can be downloaded at https://cnsgenomics.com/data/SMR/#eQTLsummarydata, and the GWAS summarized data can be downloaded at http://ftp.ebi.ac.uk/pub/databases/gwas/summary_statistics/GCST009001-GCST010000/GCST009158/.
The manuscript was written through contributions of all authors. YD, JY, and LZ designed the study. YD, YZ, and JY analyzed data and performed interpretation. YD, XL, and JY wrote the initial draft, and SM, JY, and LZ contributed to writing subsequent versions of the manuscript. All authors reviewed the study findings and read and approved the final version before submission.
The study was supported by the Non-profit Central Research Institute Fund of Chinese Academy of Medical Sciences (NO. 2020-PT320-003). JY’s research was supported by NIH/NIA grants P30AG10161, R01AG15819, R01AG17917, R01AG033678, R01AG36042, U01AG61356, and 1RF1AG064312– 01.
The authors declare that the research was conducted in the absence of any commercial or financial relationships that could be construed as a potential conflict of interest.
All claims expressed in this article are solely those of the authors and do not necessarily represent those of their affiliated organizations, or those of the publisher, the editors and the reviewers. Any product that may be evaluated in this article, or claim that may be made by its manufacturer, is not guaranteed or endorsed by the publisher.
The Supplementary Material for this article can be found online at: https://www.frontiersin.org/articles/10.3389/fgene.2022.890007/full#supplementary-material
Aissani, B., Zhang, K., and Wiener, H. (2015). Follow-up to Genome-wide Linkage and Admixture Mapping Studies Implicates Components of the Extracellular Matrix in Susceptibility to and Size of Uterine Fibroids. Fertil. Steril. 103 (2), 528–534. doi:10.1016/j.fertnstert.2014.10.025
Bulun, S. E. (2013). Uterine Fibroids. N. Engl. J. Med. 369 (14), 1344–1355. doi:10.1056/nejmra1209993
Cardozo, E. R., Clark, A. D., Banks, N. K., Henne, M. B., Stegmann, B. J., and Segars, J. H. (2012). The Estimated Annual Cost of Uterine Leiomyomata in the United States. Am. J. Obstet. Gynecol. 206 (3), 211–219. doi:10.1016/j.ajog.2011.12.002
Cha, P.-C., Takahashi, A., Hosono, N., Low, S.-K., Kamatani, N., Kubo, M., et al. (2011). A Genome-wide Association Study Identifies Three Loci Associated with Susceptibility to Uterine Fibroids. Nat. Genet. 43 (5), 447–450. doi:10.1038/ng.805
Commandeur, A. E., Styer, A. K., and Teixeira, J. M. (2015). Epidemiological and Genetic Clues for Molecular Mechanisms Involved in Uterine Leiomyoma Development and Growth. Hum. Reprod. Update 21 (5), 593–615. doi:10.1093/humupd/dmv030
Consortium, G. (2020). The GTEx Consortium Atlas of Genetic Regulatory Effects across Human Tissues. Science 369 (6509), 1318–1330. doi:10.1126/science.aaz1776
Davey Smith, G., and Ebrahim, S. (2003). 'Mendelian Randomization': Can Genetic Epidemiology Contribute to Understanding Environmental Determinants of Disease? Int. J. Epidemiol. 32 (1), 1–22. doi:10.1093/ije/dyg070
Davey Smith, G., and Hemani, G. (2014). Mendelian Randomization: Genetic Anchors for Causal Inference in Epidemiological Studies. Hum. Mol. Genet. 23 (R1), R89–R98. doi:10.1093/hmg/ddu328
Day Baird, D., Dunson, D. B., Hill, M. C., Cousins, D., and Schectman, J. M. (2003). High Cumulative Incidence of Uterine Leiomyoma in Black and White Women: Ultrasound Evidence. Am. J. Obstetrics Gynecol. 188 (1), 100–107. doi:10.1067/mob.2003.99
Dong, Z., Zhong, Z., Yang, L., Wang, S., and Gong, Z. (2014). MicroRNA-31 Inhibits Cisplatin-Induced Apoptosis in Non-small Cell Lung Cancer Cells by Regulating the Drug Transporter ABCB9. Cancer Lett. 343 (2), 249–257. doi:10.1016/j.canlet.2013.09.034
Edwards, T. L., Giri, A., Hellwege, J. N., Hartmann, K. E., Stewart, E. A., Jeff, J. M., et al. (2019). A Trans-ethnic Genome-wide Association Study of Uterine Fibroids. Front. Genet. 10, 511. doi:10.3389/fgene.2019.00511
Gallagher, C. S., Makinen, N., Mäkinen, N., Harris, H. R., Rahmioglu, N., Uimari, O., et al. (2019). Genome-wide Association and Epidemiological Analyses Reveal Common Genetic Origins between Uterine Leiomyomata and Endometriosis. Nat. Commun. 10 (1), 4857. doi:10.1038/s41467-019-12536-4
Hellwege, J. N., Jeff, J. M., Wise, L. A., Gallagher, C. S., Wellons, M., Hartmann, K. E., et al. (2017). A Multi-Stage Genome-wide Association Study of Uterine Fibroids in African Americans. Hum. Genet. 136 (10), 1363–1373. doi:10.1007/s00439-017-1836-1
Huang, Q. Y., Lai, X. N., Qian, X. L., Lv, L. C., Li, J., Duan, J., et al. (2019). Cdc42: A Novel Regulator of Insulin Secretion and Diabetes-Associated Diseases. Int. J. Mol. Sci. 20 (1). doi:10.3390/ijms20010179
Kim, J. J., and Sefton, E. C. (2012). The Role of Progesterone Signaling in the Pathogenesis of Uterine Leiomyoma. Mol. Cell. Endocrinol. 358 (2), 223–231. doi:10.1016/j.mce.2011.05.044
Labarta, E., Martinez-Conejero, J. A., Alama, P., Horcajadas, J. A., Pellicer, A., Simon, C., et al. (2011). Endometrial Receptivity Is Affected in Women with High Circulating Progesterone Levels at the End of the Follicular Phase: a Functional Genomics Analysis. Hum. Reprod. 26 (7), 1813–1825. doi:10.1093/humrep/der126
Lawlor, D. A., Harbord, R. M., Sterne, J. A. C., Timpson, N., and Davey Smith, G. (2008). Mendelian Randomization: Using Genes as Instruments for Making Causal Inferences in Epidemiology. Stat. Med. 27 (8), 1133–1163. doi:10.1002/sim.3034
Linton, K. J. (2007). Structure and Function of ABC Transporters. Physiology 22, 122–130. doi:10.1152/physiol.00046.2006
Liu, D., Wang, Y., Jing, H., Meng, Q., and Yang, J. (2021). Mendelian Randomization Integrating GWAS and mQTL Data Identified Novel Pleiotropic DNA Methylation Loci for Neuropathology of Alzheimer's Disease. Neurobiol. Aging 97, 18–27. doi:10.1016/j.neurobiolaging.2020.09.019
Liu, D., Yang, J., Feng, B., Lu, W., Zhao, C., and Li, L. (2021). Mendelian Randomization Analysis Identified Genes Pleiotropically Associated with the Risk and Prognosis of COVID-19. J. Infect. 82 (1), 126–132. doi:10.1016/j.jinf.2020.11.031
Lloyd-Jones, L. R., Holloway, A., McRae, A., Yang, J., Small, K., Zhao, J., et al. (2017). The Genetic Architecture of Gene Expression in Peripheral Blood. Am. J. Hum. Genet. 100 (2), 371. doi:10.1016/j.ajhg.2017.01.026
Luoto, R., Kaprio, J., Rutanen, E.-M., Taipale, P., Perola, M., and Koskenvuo, M. (2000). Heritability and Risk Factors of Uterine Fibroids - the Finnish Twin Cohort Study. Maturitas 37 (1), 15–26. doi:10.1016/s0378-5122(00)00160-2
Maldonado, M. d. M., and Dharmawardhane, S. (2018). Targeting Rac and Cdc42 GTPases in Cancer. Cancer Res. 78 (12), 3101–3111. doi:10.1158/0008-5472.can-18-0619
Parazzini, F. (2006). Risk Factors for Clinically Diagnosed Uterine Fibroids in Women Around Menopause. Maturitas 55 (2), 174–179. doi:10.1016/j.maturitas.2006.01.013
Qadir, M. I., Parveen, A., and Ali, M. (2015). Cdc42: Role in Cancer Management. Chem. Biol. Drug Des. 86 (4), 432–439. doi:10.1111/cbdd.12556
Rafnar, T., Gunnarsson, B., Stefansson, O. A., Sulem, P., Ingason, A., Frigge, M. L., et al. (2018). Variants Associating with Uterine Leiomyoma Highlight Genetic Background Shared by Various Cancers and Hormone-Related Traits. Nat. Commun. 9 (1), 3636. doi:10.1038/s41467-018-05428-6
Sparic, R., Mirkovic, L., Malvasi, A., and Tinelli, A. (2016). Epidemiology of Uterine Myomas: A Review. Int. J. Fertil. Steril. 9 (4), 424–435. doi:10.22074/ijfs.2015.4599
Stewart, E. A., Laughlin-Tommaso, S. K., Catherino, W. H., Lalitkumar, S., Gupta, D., and Vollenhoven, B. (2016). Uterine Fibroids. Nat. Rev. Dis. Prim. 2, 16043. doi:10.1038/nrdp.2016.43
Stewart, E., Cookson, C., Gandolfo, R., and Schulze-Rath, R. (2017). Epidemiology of Uterine Fibroids: a Systematic Review. BJOG Int. J. Obstet. Gy 124 (10), 1501–1512. doi:10.1111/1471-0528.14640
Tomlinson, I. P., Alam, N. A., Rowan, A. J., Barclay, E., Jaeger, E. E., Kelsell, D., et al. (2002). Germline Mutations in FH Predispose to Dominantly Inherited Uterine Fibroids, Skin Leiomyomata and Papillary Renal Cell Cancer. Nat. Genet. 30 (4), 406–410. doi:10.1038/ng849
Välimäki, N., Kuisma, H., Pasanen, A., Heikinheimo, O., Sjöberg, J., Bützow, R., et al. (2018). Genetic Predisposition to Uterine Leiomyoma Is Determined by Loci for Genitourinary Development and Genome Stability. Elife 7. doi:10.7554/eLife.37110
Verbanck, M., Chen, C.-Y., Neale, B., and Do, R. (2018). Detection of Widespread Horizontal Pleiotropy in Causal Relationships Inferred from Mendelian Randomization between Complex Traits and Diseases. Nat. Genet. 50 (5), 693–698. doi:10.1038/s41588-018-0099-7
Watanabe, K., Taskesen, E., van Bochoven, A., and Posthuma, D. (2017). Functional Mapping and Annotation of Genetic Associations with FUMA. Nat. Commun. 8 (1), 1826. doi:10.1038/s41467-017-01261-5
Ween, M. P., Armstrong, M. A., Oehler, M. K., and Ricciardelli, C. (2015). The Role of ABC Transporters in Ovarian Cancer Progression and Chemoresistance. Crit. Rev. Oncology/Hematology 96 (2), 220–256. doi:10.1016/j.critrevonc.2015.05.012
Wise, L. A., and Laughlin-Tommaso, S. K. (2016). Epidemiology of Uterine Fibroids: From Menarche to Menopause. Clin. Obstet. Gynecol. 59 (1), 2–24. doi:10.1097/grf.0000000000000164
Zheng, L.-H., Cai, F.-F., Ge, I., Biskup, E., and Cheng, Z.-P. (2014). Stromal Fibroblast Activation and Their Potential Association with Uterine Fibroids (Review). Oncol. Lett. 8 (2), 479–486. doi:10.3892/ol.2014.2225
Keywords: uterine leiomyomas, expression quantitative trait loci, summary Mendelian randomization, genome-wide association study, functional mapping
Citation: Dai Y, Liu X, Zhu Y, Mao S, Yang J and Zhu L (2022) Exploring Potential Causal Genes for Uterine Leiomyomas: A Summary Data-Based Mendelian Randomization and FUMA Analysis. Front. Genet. 13:890007. doi: 10.3389/fgene.2022.890007
Received: 04 March 2022; Accepted: 10 June 2022;
Published: 12 July 2022.
Edited by:
Kui Zhang, Michigan Technological University, United StatesReviewed by:
Fengxia Xue, Tianjin Medical University General Hospital, ChinaCopyright © 2022 Dai, Liu, Zhu, Mao, Yang and Zhu. This is an open-access article distributed under the terms of the Creative Commons Attribution License (CC BY). The use, distribution or reproduction in other forums is permitted, provided the original author(s) and the copyright owner(s) are credited and that the original publication in this journal is cited, in accordance with accepted academic practice. No use, distribution or reproduction is permitted which does not comply with these terms.
*Correspondence: Lan Zhu, emh1X2p1bGllQHZpcC5zaW5hLmNvbQ==
Disclaimer: All claims expressed in this article are solely those of the authors and do not necessarily represent those of their affiliated organizations, or those of the publisher, the editors and the reviewers. Any product that may be evaluated in this article or claim that may be made by its manufacturer is not guaranteed or endorsed by the publisher.
Research integrity at Frontiers
Learn more about the work of our research integrity team to safeguard the quality of each article we publish.