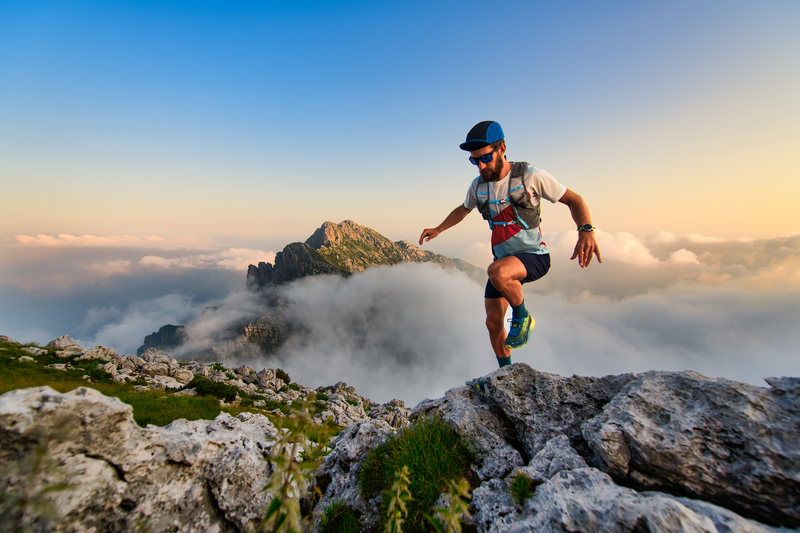
94% of researchers rate our articles as excellent or good
Learn more about the work of our research integrity team to safeguard the quality of each article we publish.
Find out more
ORIGINAL RESEARCH article
Front. Genet. , 09 August 2022
Sec. Neurogenomics
Volume 13 - 2022 | https://doi.org/10.3389/fgene.2022.884348
This article is part of the Research Topic An Insight into Multiomics Analysis of dementia disorders View all 9 articles
A correction has been applied to this article in:
Frontiers Corrigendum Template for Authors
Dementia is a clinical syndrome characterized by progressive cognitive decline, and the symptoms could be gradual, persistent, and progressive. In the present study, we investigated 47 genes that have been linked to dementia. Compositional, selectional, and mutational forces were seen to be involved. Nucleotide components that influenced A- and GC-affected codon usages bias at all three codon positions. The influence of these two compositional constraints on codon usage bias (CUB) was positive for nucleotide A and negative for GC. Nucleotide A also experienced the highest mutational force, and GC-ending codons were preferred over AT-ending codons. A high bias toward GC-ending codons enhances the gene expression level, evidenced by the positive association between CAI- and GC-ending codons. Unusual behavior of the TTG codon showing an inverse relationship with the GC-ending codon and negative influence of gene expression, behavior contrary to all other GC-ending codons, shows an operative selectional force. Furthermore, parity analysis, higher translational selection value, preference of GC-ending codons over AT-ending codons, and association of gene length with gene expression refer to the dominant role of selection pressure with compositional constraint and mutational force-shaping codon usage.
Dementia, a collection of illnesses characterized by a loss in cognitive ability that affects activities of daily living and social functioning, is one of the most severe worldwide health and social care concerns of the twenty-first century. Dementia affects approximately 50 million people globally, and the number is anticipated to rise by 2050, with one new case occurring every 3 s. Because of the rising number of people living with dementia, its significant social and economic impact, and the lack of a solution, countries must endeavor to reduce modifiable dementia risk factors (Dementia, 2022). Dementia is defined as losing two or more cognitive abilities that produce functional impairment but not alertness or attention. The deterioration in cognition distinguishes it from lifelong intellectual disability and learning problems, both present from birth and manifest in infancy. Dementia is a syndrome characterized by various brain diseases that cause memory, language, understanding, and judgment impairments (Kindell et al., 2017). Alzheimer’s disease (AD), vascular dementia, dementia with Lewy bodies, and frontotemporal dementia are the most frequent kinds of dementia (Hinz and Geschwind, 2017; Oh and Rabins, 2019). Despite breakthroughs in our understanding of the etiology, neuropathophysiology, and treatment of diverse types of dementia, these disorders continue to be significant and growing health concerns globally. Dementia affects many Parkinson’s disease patients, with a point prevalence of roughly 30%. Significant deficits characterize the executive, visuospatial, attention, and memory (Hanagasi et al., 2017). In dementia, neuropsychiatric symptoms are practically universal. The vast majority of persons with dementia will have at least one neuropsychiatric symptom throughout their illness (Radue et al., 2019). Interventions and care can dramatically improve the quality of life for people with dementia, their families, and society. There is a tangible link between cardiovascular disease, especially hypertension, and dementia (Cheng, 2017). A new study conducted in three French cities discovered a link between seven vascular risk factors and the probability of dementia (Hachinski, 2019). People with dementia are pre-dominantly susceptible to COVID-19 because of their oldness, multi-morbidity, and difficulties in keeping physical separation (Livingston et al., 2020).
In recent decades, tremendous progress has been made toward understanding the molecular genetics of neurodegenerative dementias and identifying the pathologically aggregating proteins implicated. It became possible mainly due to advances in sequencing technology and bioinformatics approaches (Hinz and Geschwind, 2017). Defects in specific genes that form abnormal proteins lead to unusual brain changes that cause neurodegenerative diseases.
Genome-wide association studies (GWAS) indicated the APOE as a decisive genetic risk factor (Harold et al., 2009; Lambert et al., 2009; Seshadri et al., 2010). UBQLN2 is another gene that encodes for ubiquitin-like protein ubiquitin two and is responsible for dominantly inherited, chromosome-X-linked amyotrophic lateral sclerosis (ALS). ALS, a paralytic disorder, results from motor-neuron degeneration in the brain and spinal cord (Deng et al., 2011). Frontotemporal lobar degeneration (FLD) is a degenerative disorder that is a genetically and pathologically heterogeneous neurodegenerative disorder. After AD, it is the most common cause of neurodegeneration, and a higher proportion of this disease is genetic causes. Mutations are present in six unrelated genes that are directly involved in FLD. Out of six, three more frequent genes are the tau gene MAPT, the progranulin gene GRN, and the hexanucleotide repeat expansions C9ORF72, TADRP, VCP, and CHMP2B are the other four genes (Paulson and Igo, 2011). Other GWAS indicated CLU gene encoding for clusterin, a protein involved in modulating the inflammatory response, as a potential risk factor, and its level is found elevated in AD patients (Lambert et al., 2009). ApoE, CLU, CR1, CD33, ABCA7, and MS4A are considered genes responsible for the late onset of AD genes (Bates et al., 2009). A large study conducted in South China encompassing 1795 patients with neurodegenerative dementias and pathogenic variants of PSEN1, PSEN2, APP, MAPT, GRN, CHCHD10, TBK1, VCP, HTRA1, OPTN, SQSTM1, and SIGMAR1 genes and abnormal repeat expansions in C9orf72 and HTT was observed, and among all, PSEN1 gene was mutated frequently (Jiao et al., 2021). The mutated genes result in the production of abnormal proteins. Amino acids are building blocks for proteins. Each amino acid is represented by a codon, a three-base sequence of nitrogenous bases. Synonymous variants with either decreasing or increasing RSCU scores in two (MLST8 and RHOB) and six genes (FLG2, CHD6, CD244, FLG-AS1, SERPINB5, and GTF3C1), respectively, have been found associated with entorhinal cortical thickness. In addition, also rare synonymous variants of MLST8 and RHOB genes were associated with the whole-brain cortical thickness (Miller et al., 2018).
Except for methionine and tryptophan, all amino acids are encoded by two or more than two codons, referred to as synonymous codons. However, synonymous codons are not used equal, with the preference of a few codons over others and termed codon usage bias (CUB). CUB has been discovered as a species-specific phenomenon. In mammals, the neutral concept and the selection–mutation–drift balance model are the two major theories to explain the origin of CUB. However, after the entire genome sequencing of numerous organisms, these two ideas were insufficient to explain the CUB phenomena. Other factors influencing CUB include the GC content (Newman et al., 2016), gene length (Duret and Mouchiroud, 1999), RNA and protein structures (Zhou et al., 2016), physical properties of encoded protein (Chen et al., 2017), environmental stress (Arella et al., 2021), tRNA population (Sabi and Tuller, 2014), etc. This research would reveal the molecular details of imperative genes participating in the regular operation of the central nervous system. In CUB, the GC content plays a crucial role in the bending, thermostability, and converting ability of B DNA to Z DNA. Newman et al. (2016) (Newman et al., 2016) found that after optimizing the codons, the improved rate of protein expression is not attributed to the enhanced translation rate but to improved transcription. An enhancement in transcription is owing to the enhanced guanine–cytosine (GC) content following codon optimization (Uddin and Chakraborty, 2019).
Furthermore, research suggests that synonymous variations can affect gene regulation pathways, particularly those connected to Alzheimer’s. Codon bias occurs when some synonymous codons are chosen over others. When it comes to codons utilized more or less frequently in the genome, bias may occur. Optimal and non-optimal codons, which have more significant and weaker codon and anti-codon interactions, can also cause a bias (Miller et al., 2018).
In the present study, we investigated the effects of various factors, including nucleotide composition, expression patterns, physical properties of the protein, length, and compositional constraints at various codon positions, CAI, length, and role of selectional and mutational pressure on the codon usage of 47 genes related to dementia. The genes envisaged here had a direct or indirect effect on neuronal health in case of improper functioning. Table 1 describes the occurrence of diseases if these genes are malfunctioning. The set of genes envisaged is involved in at least 42 diseased conditions, including but not limited to amyotrophic lateral sclerosis, Alzheimer’s disease, neurodegeneration with brain iron accumulation, Parkinson’s disease, frontotemporal lobar degeneration, cerebral amyloid angiopathy, lateral meningocele syndrome, Lewy body dementia, etc. The study will help determine various forces that drive the codon usage in genes involved in dementia.
Based on the information available at NCBI Genetic Testing Registry, 47 gene sequences involved in dementia (list of genes given in Table 1) were retrieved. Genetic testing is commercially available for all these genes from Amsterdam UMC Genome Diagnostics, Amsterdam University Medical Center, Netherlands, in conditions/phenotype of Alzheimer’s disease types 1–4, ABri amyloidosis, ADan amyloidosis, and amyotrophic lateral sclerosis. A total of 54 genes was available, and out of them, we took 47 gene sequences based on qualifying criteria. All the coding sequences were qualified based on nucleotides in triplicate, the absence of in-frame stop codons, and the lack of ambiguous nucleotides. Sequences less than 150 base pairs were also omitted.
The nucleotide composition analysis was done for genes related to dementia. Individually overall, %A, %T, %G, and %C nucleotide compositions were determined. Also, their compositions at all the three positions of codons were determined. Percent GC3 and the average composition of %GC at the first and second positions of codon (%GC12) were determined for neutrality analysis. Nucleotide composition at the third codon position was used for determining the AT bias [A3/(A3 + T3)] and GC bias [G3/(G3 + C3)] calculations to be included in parity analysis. The analysis was done using CAIcal, a web-based server available at http://genomes.urv.es/CAIcal (CAI calculator, 2022).
Sixteen dinucleotide combinations are possible with four nucleotides, but their appearance is biased in any genome. The ratio of the obtained-to-expected frequency is called the odds ratio. An odds ratio below 0.78 is called under-representation, and above 1.23, it is called over-representation of a dinucleotide (Butt et al., 2014).
Among 64 codons (nucleotide triplets) in the standard genetic code, except for three stop codons (TAA, TAG, TGA), methionine, and tryptophan (Belalov and Lukashev, 2013), all amino acids are encoded by two or more than two triplets and are termed as synonymous codons. All the synonymous codons are not used equally, referred to as codon usage bias (CUB). The ratio observed to the expected frequency of a codon coding for an amino acid is termed as the relative synonymous codon usage (RSCU) value. RSCU analysis was done by CodonW 1.4.4 software available at http://codonw.sourceforge.net. The codons with RSCU values above 1.6 are called over-represented and below 0.6 are called under-represented (Kumar et al., 2021).
Codon adaptation index measures the level of expression of a protein and the adaptiveness of a gene to the host. It is also a measure of CUB for a DNA/RNA sequence and can quantify the codon usage similarities between a gene and a reference set (Puigbò et al., 2008). Its values range between 0 and 1. If a gene always uses the most frequently used synonymous codon from the reference set, in such case, the CAI value will be 1. In contrast, the usage of the least frequently used synonymous codon from the reference set will result in a CAI value of zero. CAI values were obtained by the software developed by Bourret et al. (2019) (Bourret et al., 2019). As a reference set, the RSCU analysis of 40662582 codons belonging to 93487 coding sequences from Homo sapiens available at the codon usage database https://www.kazusa.or.jp/codon/ (Codon Usage Database, 2022) was used.
It is a directional estimate of CUB and computed as the sum of the chi-square values of the codon families within the gene normalized by peptide length termed as scaled chi-square (). For each gene, SCS was calculated. Its value ranges between 0 and 1, and a higher value shows higher bias (Chu and Wei, 2019).
The physical properties of proteins affect several of the properties of the protein and its biological functions. To determine the relationship of the physical properties of proteins with CUB, a correlation analysis was carried out between various protein indices (PIs or isoelectric point, instability index, aliphatic index, and hydrophobicity, frequency of acidic, basic, neutral amino acids, GRAVY and AROMA; total nine) and SCS. The protein properties were calculated using Protparam Expasy (Gasteiger et al., 2005) and peptide2 tool available at Peptide 2.0 Inc. GRAVY and AROMA values were calculated using the software developed by Bourret et al. (2019) (Bourret et al., 2019). GRAVY expresses features of both hydrophobicity and hydrophilicity, and it ranges between -2 and +2. A positive value is an indicator of a more hydrophobic protein and vice versa. AROMA indicates the frequency of aromatic amino acids. Hydrophobicity measures a protein’s solubility and plays a role in the protein–protein interactions. The aliphatic index is a suggestive of volume gained by aliphatic side chains. An instability index with an aliphatic index reveals the stability of a protein (Khandia et al., 2021). The isoelectric point is a value where no net electric charge on protein is present, and solubility is minimal (ScienceDirect Topics, 2022).
AT skew, GC skew, purine skew, pyrimidine skew, amino skew, and keto skew are determinants of compositional skews and are calculated using the formula proposed by Wu et al. (2021). Cumulative GC and AT skews are calculated as a sum of (G-C)/(G + C) and (A-T)/(A + T), respectively (Grigoriev, 1998). Likewise, keto-amino or purine-pyrimidine skews were obtained by making appropriate replacements (Powdel et al., 2010).
To generate a regression plot, the mean %GC12 and %GC3 were plotted on the Y- and X-axes. The neutrality plot measures the mutational force or the neutrality primarily. When the slope is 1, codon usage is solely driven by mutational forces (Guan et al., 2018). Conversely, a slope deviation from 1 indicates other forces like selection pressure while shaping codon usage in any organism.
A parity rule 2 (PR2) bias was calculated to determine the disparity of the usage of AT or GC at the third position of the codon. A plot is made by taking the average AT bias [A3/(A3 + T3)] as the ordinate and GC bias [G3/(G3 + C3)] as the abscissa (K handia et al., 2019), and a scatter plot is made. At the center of the plot, where the value is zero, A = T and C = G in a strand (Yang et al., 2015).
A plot between the overall nucleotide content and nucleotide content at the third codon position was plotted for all the four nucleotides (%A3-%A, %T3-%T, %C3-%C, %G3-%G). It is indicative of the effect of mutational force on the composition of the gene.
Translational selection (P2) is a measure of the codon–anticodon association and the translational efficacy of a gene (Bennetzens and Hall, 1982). Translational selection P2 was calculated using the formula
where W = A or U, S = C or G, and Y = C or U.
And the values above 0.5 are indicative of a bias favoring translational selection (A U et al., 2019).
Compositional analysis revealed that among all nucleotide compositions, the %GC3 content was highly variable, ranging between 30.08 and 86.68%. Overall, the average percentage composition analysis revealed that %GC1 and %GC3 compositions were almost equal (57.17 and 58.51%, respectively), while the %GC2 composition was the least (43.21%). The average nucleotide composition for %A and %C nucleotides was almost equal (25.09 and 25.12%, respectively), with the maximum for %C (27.84%) and least for %T (21.94). %T1 was the least at codon position one (T composition at codon position one and likewise), and %A3 had a trend of low appearance in composition (Figure 1).
Figure 1. A nucleotide compositional analysis figure shows the trend of the compositional constraints. The nucleotide contents are sorted here as per their increasing values and do not depict their content in a single gene.
The SCS correlated only with the frequency of acidic amino acids. It had a positive association with the frequency of acidic amino acids (r = 0.373, p < 0.001). The correlation was absent for any of the other eight tested indices in the present study.
The effect of compositional disproportion on CUB was observed by correlating the AT skew, GC skew, purine skew, pyrimidine skew, amino skew, and keto skew with SCS. A significantly strong positive relationship between CUB and purine skew (r = 0.535, p < 0.001), pyrimidine skew (r = 0.407,p < 0.01), amino skew (r = 0.370, p < 0.05), and keto skew (r = 0.535,p < 0.001) was found.
Correlation analysis was carried out between the nucleotide content and SCS values (Table 2). Nucleotide A has a positive correlation with CUB at all three positions of codons. Conversely, %GC composition had a negative association with CUB at all codon positions. At other codon positions, also a few correlations were observed. Overall analysis revealed that compositional constraints affect CUB.
Out of nine protein indices studied, %GC2 had a significant relationship with six indices (both positive and negative) while %G2 and %T2 had a relationship (both positive and negative) with five indices each. %GC2 had a positive association with PI (r = 0.356, p < 0.05), instability index (r = 0.413, p < 0.01), and frequency of neutral amino acid (r = 0.686, p < 0.05), while a negative association with aliphatic index (r = -0.644, p < 0.001), hydrophobicity (r = -0.361, p < 0.05), and frequency of acidic amino acid (r = −0.554, p < 0.001). Alike GC2, %G2 also had a negative association with aliphatic index (r = −.656, p < 0.001), hydrophobicity (r = −0.646, p < 0.001), and frequency of acidic amino acid (r = −0.501, p < 0.001), but a positive association with only PI (r = 0.497, p < 0.001) and frequency of neutral amino acid (r = 0.852, p < 0.001). %T2 had a positive relationship with aliphatic index (r = 0.938, p < 0.001) and hydrophobicity (r = 0.747, p < 0.001), while a negative relationship with PI (r = −0.548, p < 0.001), instability index (r = −0.427, p < 0.01), and frequency of neutral amino acid (r = −0.324, p < 0.05). %T1, %T3, %G3, %GC1, and %GC3 had no relationship with any of the protein indices tested in the present study. GRAVY has a positive association with %T1 (r = 0.340, p < 0.05) and %T2 (r = 0.801, p < 0.001), while a negative association with %A2 (r = −0.521, p < 0.001) and %G2 (r = −0.341, p < 0.05). AROMA has a positive association with nucleotide T at all the three positions of codon (%T1-r = 0.665, p < 0.001; %T2-r = 0.419, p < 0.01 and %T3- (r = 0.304, p < 0.05). AROMA had a negative association with %C2 (r = −0.498, p < 0.001), %G1 (r = −0.288, p < 0.05), %G3 (r = −0.304, p < 0.05), and %GC1 (r = −0.476, p < 0.01).
Analysis of the trend of dinucleotides in different genes associated with dementia showed that dinucleotides ApG, CpA, and TpG were either over or randomly represented (odds ratio >0.78). In contrast, CpG, GpT, and TpA dinucleotides were either under or randomly expressed based on the odds ratio (odds ratio <1.6). An analysis of the relationship between the dinucleotide odds ratio and CUB revealed that CUB has a significant positive association with ApA (r = 0.358, p < 0.05), ApC (r = 0.292, p < 0.05), ApT (r = 0.456, p < 0.01), TpA (r = 0.484, p < 0.001), and TpT (r = 0.466, p < 0.001) dinucleotides, while a negative relationship with CpG (r = −0.456, p < 0.001), GpC (r = −0.468, p < 0.001), and GpG (r = −0.621, p < 0.001) dinucleotides. There was no correlation between CpG and TpG dinucleotides (r = −0.091, p = 0.542).
RSCU pattern analysis indicated the preference of GC-ending codons over AT-ending codons. Nucleotide CTG and GTG were over-represented in 74.46 and 68.08% of genes, respectively. GTA, CAA, CTA, ATA, TTA, CGT, GCG, ACG, CCG, and TCG codons were under-represented in 68.085, 59.57%, 72.34%, 70.216, 68.08, 53.19, 72.34, 57.44, 70.21, and 78.72% of genes, respectively. When RSCU values of 47 genes were correlated with SCS, SCS was found positively associated with a few AT-ending codons while negatively associated with some of the GC-ending codons (data not shown here).
To understand the trend of gene expression with AT- and CG-ending codons, the correlation analysis between RSCU values of genes and CAI (Figure 2) was performed. CAI value of genes is given in (Table 3).
Figure 2. Correlation analysis of RSCU values of a codon and CAI value. A positive correlation is blue circles, while a negative correlation is depicted as red circles. The level of significance was at 0.05%.
The analysis revealed that gene expression was negatively correlated with all the AT-ending codons while positively correlated with GC-ending codons. Clustering of multivariate data based on the RSCU analysis revealed that codon TTG was clustered with GC-ending codons (Figure 3).
Figure 3. A cluster analyses of multivariate data showed the clustering of TTG with AT-ending codons.
Codon TTG was inversely related to gene expression and surprisingly showed a negative association with GC-ending codons. Codons CGT and AGG had correlations only with two codons out of 59 (excluding trp, met, and stop codons). Codon CGT had a positive affiliation with AGT (r = 0.448, p < 0.05) and negative with TCC (r = −0.307, p < 0.05), while AGG codon had a positive affiliation with CCG (r = 0.303, p < 0.05) and negative with CGC (r = −0.358, p < 0.05). Here, the determination of codon correlation is important since codon correlation tends to accelerate the translation process compared to anti-correlated codons, and translation speed may be primarily explained based on codon correlation (Cannarozzi et al., 2010).
GC composition can be used as both a good indicator of CUB (Shen et al., 2015a) and the extent of base composition (Das and Roy, 2021a). The unusual behavior of codons CGT and AGG can be further investigated for the impact posed by compositional bias. GC3 is a good indicator of compositional bias (Deka and Chakraborty, 2016); therefore, regression analysis between RSCU values of CGT and AGG, and %GC3 composition was done to evaluate the effect of compositional bias (Figure 4). We took two more codons, CTG and CTA, the most over-represented and under-represented codons. The R2 values here explain the percent variation in the RSCU value by the GC3 component. CTG and CTA, the most over-represented and under-represented codons, respectively, in the set of 47 genes, showed R2 values = 0.632 and 0.396, indicating that 63.2 and 39.6% variations in the CTG and CTA codon could be explained by %GC3 composition. Contrary to this, codons CGT and AGG could explain only 0.74 and 1.96% variations in %GC3, which is negligible. Furthermore, the regression analysis of these codons with AT3 revealed the same results. Hence, bias in these two codons CGT and AGG is not influenced by compositional constraints (Table 4).
Percent GC12 and %GC3 had a positive correlation (r = 0.622, p < 0.001). A neutrality plot is drawn to precisely determine which force is the major force affecting codon usage and quantify it. The neutrality plot indicated that the relative neutrality is 24.52%, while the relative constraint was 75.48% for GC3 (Kumar et al., 2021). The GC12 content was affected by mutation pressure and natural selection with a ratio of = 24.52/75.48 = 0.324 (Figure 5).
Figure 5. Regression plot analysis between GC3 and GC12 exhibiting the mutational and selection forces.
The mean value of AT bias [A3/(A3 + T3)] was 0.498 ± 0.071 and GC bias [G3/(G3 + C3)] was 0.442 ± 0.076. The plot indicated that T and C are preferred over A and G (Zhang et al., 2018). When the overall nucleotide skew was observed, a positive value was obtained for both the AT and GC skews. Furthermore, the positive skew value indicated nucleotide A dominance over T and G over C. Cumulatively, the parity and skew analysis results indicate that overall A and G nucleotides are dominant, while T and C nucleotides are dominant at the third codon position.
The regression analysis between the overall composition and composition at the third position of codon indicates the mutational pressure (Uddin and Chakraborty, 2019), and it affected nucleotide A the maximum (67.19%), while it affected nucleotide G the least (37.15%). Nucleotides T and C contributed 40.13 and 51.36% to mutation, respectively (Figure 6).
Correspondence analysis on RSCU values of genes involved in dementia revealed a scattered distribution of genes, showing variability in codon usage. Axis 1 contributed for 41.58% and axis 2 contributed for 6.66% variation. Axis 1 contributed the maximum for variation, and both the GC- and AT-ending genes were located near axis 1, which indicates the effect of both the AT-ending and GC-ending codons on CUB (Lu, 2005). The genes had a major distribution along the first axis, suggesting other factors like selectional force on the codon usage of genes related to dementia (Wei et al., 2014). A biplot analysis revealed that codons AGA and CTG along the first axis and AGG and CGC along the second axis influenced CUB.
CAI is a measure of the codon expression level of any gene, and higher CAI values reveal a higher expression level. CAI value was the highest for the CTSD gene (CAI = 0.849) and the least for the VPS13A gene (CAI = 0.673). A correlation analysis was done between the AT, GC, purine, pyrimidine, keto, and amino skews, and CAI to determine the possible connection between the gene expression level and nucleotide disproportion. The analysis revealed that except AT and GC skews, the gene expression level is negatively influenced by purine (r = −0.777, p < 0.001), pyrimidine (r = −0.805, p < 0.001), amino (r = −0.735, p < 0.001), and keto (r = −0.754, p < 0.001) skews. There was no correlation between the CAI and SCS, indicative of no effect of expression levels of genes on CUB. With the length of the protein, the CAI had a negative association (r = −0.303, p < 0.05), and it decreased with the increasing length of the protein. The protein expressivity in the genes associated with dementia is positively influenced by GC3 (r = 0.851, p < 0.001), while negative influenced by %GC12 (r = −0.303, p < 0.05). The GC3 content can be considered an indicator representing the extent of bias in nucleotide composition (Sablok et al., 2011). The association of GC3 with CAI indicated the effects of nucleotide bias on gene expression. CAI had a statistically significant (p < 0.05) negative association with AT-ending codons (all codons except CGA, CGT, TGT), while a significant positive association (p < 0.05) with GC-ending codons except codon AGG.
A P2 value higher than 0.5 indicated a bias toward the translational selection (A U et al., 2019). In the present study, the P2 value was 1.01, indicating a strong translation efficacy toward selectional force.
The nucleotide composition gene is a crucial determinant of many of the properties and CUB. The composition might affect a protein’s physical properties, like stability and various functions that could be ascribed by the secondary structure [36]. In the present study, we found the highest percentage of %C and the lowest percentage of %T, while %A and %C nucleotides were almost equal. A lower occurrence of C and G nucleotides has been observed by Franzo et al. (2021) (Franzo et al., 2021). The GC content at the three positions is documented to be variable, and Song et al. (2017) (Song et al., 2017) reported the order %GC3>%GC2>%GC1 in peramine-coding genes of Epichloë species; however, in a highly expressed gene, a higher GC2 content was reported. In the present case, the average percent analysis of composition revealed variability in the % GC composition according to the position of the codon, and %GC1 and %GC3 compositions were almost equal while %GC2 composition was least.
Protein properties like GRAVY and AROMA are linked to the nucleotide composition, and CUB indicated that codon variations affect protein properties (Huang et al., 2017). CUB was negatively associated with the aliphatic index in the spike protein gene of infectious bronchitis virus (Makhija and Kumar, 2015), while positively associated with GRAVY and AROMO in genes of Ginkgo biloba (He et al., 2016). Protein indices like GRAVY and AROMA are the indices of natural selection (K handia et al., 2019). In the 47 genes associated with dementia, out of nine envisaged protein indices, only one physical property, viz. the frequency of neutral amino acid, was found linked with CUB, depicting that the effect of physical properties on CUB is at a low level in our study. Various nucleotide skews, including AT skew (A-T/A + T), GC skew (G-C/G + C), purine skew (A-G/A + G), pyrimidine skew (T-C/T + C), amino skew (A-C/A + C), and keto skew (T-G/T + G), explain nucleotide composition disproportion and nucleotide skew impacts on CUB. Correlation analysis between CUB and various skews revealed an association between the skews and CUB (Chakraborty et al., 2019). A positive association of CUB (SCS) with purine, pyrimidine, amino, and keto skew was observed in the present study. So, it can be inferred that codon bias will also increase with the increasing disproportion in nucleotide composition.
Codon usage bias correlates with GC composition and, generally, a very low or very high GC composition refers to a greater codon usage bias (Wan et al., 2004). In the present study, nucleotide A has a positive association with CUB at all the three positions of codons, and the GC content had a negative relationship with CUB at all three positions of the codon. Other researchers also found a relationship between codon bias and nucleotide composition suggestive of mutational force acting on codon bias (Deb et al., 2020). CpC dinucleotide abundance has been reported with the fine-tuning of gene expression also (Khandia et al., 2022). The positive association of odds ratio of A/T-containing dinucleotides (ApA, ApC, ApT, TpA, and TpT) and the positive association of odds ratio of G/C-containing dinucleotides (CpG, GpC, and GpG) with CUB indicated the presence of selectional forces in shaping codon usage.
Under-representation of CpG and TpA, dinucleotides are common in eukaryotes, and for CpG in mammalian genomes, the occurrence is at one-fifth of their expected frequency is supported by experimental pieces of evidence (Simmen, 2008). Our study also found under-represented CpG and TpA dinucleotides, apparently resulting from selectional forces (Munjal et al., 2020). The under-representation of CpG can be understood because in the CpG context, cytosine is found methylated in eukaryotes and becomes 5-methylcytosine. Methylated cytosine undergoes rapid deamination to give rise to thymidine. In further rounds of replication, in the case of endogenous mismatch repair enzymes failing in repairing this, CpG dinucleotide changes into TpG, or CpA (if the mutation occurred on the opposite strand) (Salser, 1978). TpA under-representation is present throughout the eukaryotic genomes (Karlin and Mrázek, 1997) and is attributed to the fact that TpA in the mRNA sequence (UpA) is prone to be a target by cellular RNases. Also, under-representation could be described based on its presence in two of three stop codons (Kumar et al., 2021). If we try to link the CpG deficit to the TpG excess, we found either over-representation or random representation of TpG dinucleotide in the genes evaluated in the present study. Further, if the CpG deficit is solely attributed to TpG conversion, there must be a negative correlation between CpG and TpG contents, but no such relation was observed in the present study. So, it further questions the theory of CpG methylation behind the CpG deficit.
Even though we did not find a correlation between the CpG and TpG/CpA, TpG dinucleotide-containing codons CTG and GTG were over-represented in 74.46 and 68.08% genes, respectively, while all CpG-containing codons (CGT, GCG, ACG, CCG, and TCG) were under-represented (in 53.19, 72.34, 57.44, 70.21, and 78.72% genes respectively). CTG codon has been over-represented in genes common in primary immunodeficiencies and cancer (Khandia et al., 2021). The same has been depicted in genes associated with brain iron accumulation (Alqahtani et al., 2021) (Alqahtani et al., 2021). With that, A/T-ending codons positively influenced CUB and G/C-ending codons negatively influenced CUB coupled with the fact that AT-ending codons were preferred, while GC-ending codons were not preferred. It indicated that with the usage of highly preferred codons, CUB is also increased. Biplot analysis revealed that AGA and CTG along the first axis and AGG and CGC along the second axis influenced CUB the most. In the study of Yang et al. (2010), other codons, including CGC (Arg), AGC (Ser), and GGC (Gly), were also found to be critical in influencing codon usage bias.
Codon usage bias correlates with GC composition, and with increasing GC composition, GC ending codon preference also increases (Wan et al., 2004). However, codon TTG behaves differently, and when GC ending codons positively influence gene expression, TTG showed an inverse relationship. Also, at one end, where all the GC ending codons show a positive relation with GC-ending codons, TTG showed a positive relationship with AT-ending codons. In the studies of Alqahtani et al. (2021), TTG has been shown to have an inverse relationship with the GC content, the gene set associated with neurodegeneration with iron accumulation. A codon preference affects the expression level of individual genes and the gene translation level of other proteins present in cells (Frumkin et al., 2018). This behavior cannot be explained based on compositional bias. The result follows the works of Kliman and Bernal (2005), who found in a high expression dataset of human genes, an exaggerated T→C transition rates are attributed to a higher number of CTG codons at the expense of TTG codons. Also, a decline in TTG numbers is observed in highly expressed genes suggestive of operative selection force.
Percent GC3 composition indicates CUB (Shen et al., 2015b) and base composition constraint (Das and Roy, 2021b). Regression analysis was done for unusual behavior displaying codons CGT and AGG, whether their CUB is driven by the compositional constraint. Moreover, CTG and CTA, the most over-represented and under-represented codons in the set of 47 genes, regressed with %GC3 as a reference. On the one hand, where 63.2 and 39.6% variations in the RSCU of CTG and CTA could be explained based on %GC3, CGT and AGG codons could explain only 0.74 and 1.14% variations in %GC3 indicative of a very poor association with compositional constraint.
CAI is a measure of the gene expression level, and highly expressed genes have CAI values near 1. Also, values close to 1 indicate that codons with highly RSCU values are used in the gene (K handia et al., 2019). The CAI value is independent of protein length and depends on amino acid frequency (Xia, 2007). However, in the present study, we found a negative association of CAI with the length of the protein, referring to the selection force favoring shorter proteins over larger proteins. Opposite results were obtained by Huang et al. (2017), who reported significant positive correlations of CAI with gene length in Taenia multiceps. GC3 was found to positively influence CAI, indicating the effect of nucleotide composition on gene expression (Sablok et al., 2011). CAI was negatively associated with AT-ending codons, while positively associated with highly preferred GC-ending codons.
Gene expression level is influenced by nucleotide disproportion also. Kleerebezem et al., 2003 suggested the effect of skewness in CUB. A negative GC skew value refers to the richness of C nucleotides over G, and likewise, a negative AT skew refers to the richness of T over A nucleotides (Nath Choudhury et al., 2017). In the cp genes, Zhang et al. (2013) reported more frequent usage of pyrimidines than purine at the third position of codons. In the present study, CAI was found to have no association with AT or GC skews but a significant (p < 0.05) negative correlation with a purine (A-G/A + G), pyrimidine (T-C/T + C), amino (A-C/A + C), and keto skews (T-G/T + G) suggests an effect of compositional disproportion on the gene expression level. A significant correlation of protein indices GRAVY and AROMO with the codon compositions at the third codon positions (A3s, T3s, G3s, C3s, and GC3s) showed natural selection as the principal force in shape codon usage in the evolution of canine distemper virus (Wang et al., 2020). In our study, all the relationships between nucleotide composition and protein indices were found (positive, negative, and no correlations), indicating the imperative role of selectional forces.
From the studies of various researchers, it is evident that CUB in any organism is the result of selectional and mutational forces and compositional constraints. Mutational force is the key force in shaping CUB in the Gallus genome (Rao et al., 2011), while compositional constraints are imperative in hemagglutinin gene in the H1N1 subtype of influenza A virus (Deka and Chakraborty, 2014). Sometimes, a combination of forces is involved (Chen et al., 2014; Trotta, 2016; Yengkhom et al., 2019; Khandia et al., 2021). Other forces, including mutational drift (Bulmer, 1991) and tRNA availability (Rocha, 2004), also affect codon usage.
A neutrality plot is generated to determine the decisive force between mutation and selection in CUB. When a correlation between GC12 and GC3 is present, it indicates mutational force likely working on all the codon positions (Jenkins and Holmes, 2003). In our study, a positive relationship between GC12 and GC3 indicated the presence of mutational pressure. Our results are in concordance with Uddin (2020), who also reported the same in genes associated with anxiety in humans. The regression plot indicated a strong selectional force where relative neutrality (mutational force) was 24.52%, while the relative constraint (natural selection) was 75.48%. The dominance of T and C over A and G in the parity plot again highlighted the role of selectional forces. The translational selection value greater than 0.5 in the present study also signified the importance of selectional pressure.
The regression analysis between the overall composition and composition at the third position of the codon is indicative of the role of mutational pressure acting in shaping the nucleotide composition [56]. In the present study, mutational forces affected the nucleotide A composition the maximum (67.19%). In comparison, it affected nucleotide G least (37.15%), inferring the effects of mutational force in determining the composition of a gene. A greater contribution of nucleotides G and A (68.48 and 71.19%, respectively) has been observed in the Anelloviridae genomes (Deb et al., 2021).
Overall, the analysis indicated the presence of mutational and selectional forces and compositional constraints in shaping codon usage. Nucleotide compositions greatly affected the bias and nucleotide A positively affected, and GC compositions negatively affected CUB at all codon positions. Mutational force affected nucleotide A the maximum and overall contributed 67.19% to the CUB. Based on the RSCU analysis, it is clear that GC-ending codons are over-represented and positively influence the CUB. Highly biased composition and over-representation of GC-ending codons were associated with higher expression levels. Based on the neutrality plot, parity plot, under-representation of TpA and CpG, and over the presentation of TpG dinucleotide, the preference of GC-ending codons over AT-ending codons, the high value of P2 and the relationship of gene expression with gene length, and unusual behavior of TTG codon, all point toward the dominant role of selectional force over mutational force.
The datasets presented in this study can be found in online repositories. The names of the repository/repositories and accession number(s) can be found below: https://www.ncbi.nlm.nih.gov/gtr/.
Conceptualization, RK and NP; methodology, RK and NP; software, RK and NP; validation, RK, TA, and AMA; formal analysis, RK, TA, and AMA; investigation, RK, NP, and AA; resources, RK, TA, AMA, AAM, KC, and MAK; experimentation, data curation, RK and NP; writing—original draft preparation, RK and NP; writing—review and editing, RK, TA, and AMA; visualization, RK, TA, and AMA; supervision, RK; project administration, RK, TA, and AMA; funding acquisition, RK, TA, AMA, AAM, KC, and MAK. All authors have read and agreed to the published version of the article. All authors read and agreed on the final version of the article.
“This research was funded by Small Research Grant Program, grant number SRP/275/1442.”
The authors declare that the research was conducted in the absence of any commercial or financial relationships that could be construed as a potential conflict of interest.
All claims expressed in this article are solely those of the authors and do not necessarily represent those of their affiliated organizations, or those of the publisher, the editors, and the reviewers. Any product that may be evaluated in this article, or claim that may be made by its manufacturer, is not guaranteed or endorsed by the publisher.
The authors are thankful to their respective universities for providing the requirements to conduct the study.
A. U, N. P, S. C., Paul, N., and Chakraborty, S. (2019). The codon usage pattern of genes involved in ovarian cancer. Ann. N. Y. Acad. Sci. 1440 (1), 67–78. doi:10.1111/nyas.14019
Alqahtani, T., Khandia, R., Puranik, N., Alqahtani, A. M., Almikhlafi, M. A., Algahtany, M. A., et al. (2021). Leucine encoding codon TTG shows an inverse relationship with GC content in genes involved in neurodegeneration with iron accumulation. J. Integr. Neurosci. 20 (4), 905–918. doi:10.31083/j.jin2004092
Arella, D., Dilucca, M., and Giansanti, A. (2021). Codon usage bias and environmental adaptation in microbial organisms. Mol. Genet. Genomics. 296 (3), 751–762. doi:10.1007/s00438-021-01771-4
Bates, K. A., Verdile, G., Li, Q. X., Ames, D., Hudson, P., Masters, C. L., et al. (2009). Clearance mechanisms of alzheimer’s amyloid-beta peptide: Implications for therapeutic design and diagnostic tests. Mol. Psychiatry 14 (5), 469–486. doi:10.1038/mp.2008.96
Belalov, I. S., and Lukashev, A. N. (2013). Causes and implications of codon usage bias in RNA viruses. PLoS One 8 (2), e56642. doi:10.1371/journal.pone.0056642
Bennetzens, J. L., and Hall, B. D. (1982). Codon selection in yeast. J. Biol. Chem. 257 (6), 3026–3031. doi:10.1016/s0021-9258(19)81068-2
Bourret, J., Alizon, S., and Bravo, I. G. (2019). COUSIN (COdon usage similarity INdex): A normalized measure of codon usage preferences. Genome Biol. Evol. 11 (12), 3523–3528. doi:10.1093/gbe/evz262
Bulmer, M. (1991). The selection-mutation-drift theory of synonymous codon usage. Genetics 129 (3), 897–907. doi:10.1093/genetics/129.3.897
Butt, A. M., Nasrullah, I., and Tong, Y. (2014). Genome-wide analysis of codon usage and influencing factors in chikungunya viruses. PLoS One 9 (3), e90905. doi:10.1371/journal.pone.0090905
CAI calculator. Available at: http://genomes.urv.es/CAIcal/.[cited 2022 Apr 16].
Cannarozzi, G., Cannarrozzi, G., Schraudolph, N. N., Faty, M., von Rohr, P., Friberg, M. T., et al. (2010). A role for codon order in translation dynamics. Cell. 141 (2), 355–367. doi:10.1016/j.cell.2010.02.036
Chakraborty, S., Deb, B., Barbhuiya, P. A., and Uddin, A. (2019). Analysis of codon usage patterns and influencing factors in Nipah virus. Virus Res. 263, 129–138. doi:10.1016/j.virusres.2019.01.011
Chen, H., Sun, S., Norenburg, J. L., and Sundberg, P. (2014). Mutation and selection cause codon usage and bias in mitochondrial genomes of ribbon worms (Nemertea). PLoS One 9 (1), e85631. doi:10.1371/journal.pone.0085631
Chen, Y., Li, X., Chi, X., Wang, S., Ma, Y., Chen, J., et al. (2017). Comprehensive analysis of the codon usage patterns in the envelope glycoprotein E2 gene of the classical swine fever virus. PLoS One 12 (9), e0183646. doi:10.1371/journal.pone.0183646
Cheng, S. T. (2017). Dementia caregiver burden: A research update and critical analysis. Curr. Psychiatry Rep. 19 (9), 64. doi:10.1007/s11920-017-0818-2
Chu, D., and Wei, L. (2019). Nonsynonymous, synonymous and nonsense mutations in human cancer-related genes undergo stronger purifying selections than expectation. BMC Cancer 19 (1), 1–359. doi:10.1186/s12885-019-5572-x
Codon Usage Database. Available at: https://www.kazusa.or.jp/codon/.[cited 2022 Apr 16].
Das, J. K., and Roy, S. (2021). Comparative analysis of human coronaviruses focusing on nucleotide variability and synonymous codon usage patterns. Genomics 113 (4), 2177–2188. doi:10.1016/j.ygeno.2021.05.008
Das, J. K., and Roy, S. (2021). Comparative analysis of human coronaviruses focusing on nucleotide variability and synonymous codon usage patterns. Genomics 113 (4), 2177–2188. doi:10.1016/j.ygeno.2021.05.008
Deb, B., Uddin, A., and Chakraborty, S. (2020). Codon usage pattern and its influencing factors in different genomes of hepadnaviruses. Arch. Virol. 165 (3), 557–570. doi:10.1007/s00705-020-04533-6
Deb, B., Uddin, A., and Chakraborty, S. (2021). Genome-wide analysis of codon usage pattern in herpesviruses and its relation to evolution. Virus Res. 292, 198248. doi:10.1016/j.virusres.2020.198248
Deka, H., and Chakraborty, S. (2014). Compositional constraint is the key force in shaping codon usage bias in Hemagglutinin gene in H1N1 Subtype of influenza A virus. Int. J. Genomics 2014, 349139. doi:10.1155/2014/349139
Deka, H., and Chakraborty, S. (2016). Insights into the usage of nucleobase triplets and codon context pattern in five influenza A virus subtypes. J. Microbiol. Biotechnol. 26 (11), 1972–1982. doi:10.4014/jmb.1605.05016
Dementia. Available at: https://www.who.int/news-room/fact-sheets/detail/dementia.[cited 2022 Apr 16]
Deng, H. X., Chen, W., Hong, S. T., Boycott, K. M., Gorrie, G. H., Siddique, N., et al. (2011). Mutations in UBQLN2 cause dominant X-linked juvenile and adult-onset ALS and ALS/dementia. Nature 477 (7363), 211–215. doi:10.1038/nature10353
Duret, L., and Mouchiroud, D. (1999). Expression pattern and, surprisingly, gene length shape codon usage in Caenorhabditis, Drosophila, and Arabidopsis. Proc. Natl. Acad. Sci. U. S. A. 96 (8), 4482–4487. doi:10.1073/pnas.96.8.4482
Franzo, G., Tucciarone, C. M., Legnardi, M., and Cecchinato, M. (2021). Effect of genome composition and codon bias on infectious bronchitis virus evolution and adaptation to target tissues. BMC Genomics 22 (1), 244. doi:10.1186/s12864-021-07559-5
Frumkin, I., Lajoie, M. J., Gregg, C. J., Hornung, G., Church, G. M., Pilpel, Y., et al. (2018). Codon usage of highly expressed genes affects proteome-wide translation efficiency. Proc. Natl. Acad. Sci. U. S. A. 115 (21), E4940–E4949. doi:10.1073/pnas.1719375115
Gasteiger, E., Hoogland, C., Gattiker, A., Duvaud, S., Wilkins, M. R., Appel, R. D., et al. (2005). “Protein identification and analysis tools on the ExPASy server,” in The proteomics protocols handbook. Editor J. M. Walker (Totowa, NJ: Humana Press), 571–607. (Springer Protocols Handbooks). Available from. doi:10.1385/1-59259-890-0:571[cited 2022 Apr 13]
Grigoriev, A. (1998). Analyzing genomes with cumulative skew diagrams. Nucleic Acids Res. 26 (10), 2286–2290. doi:10.1093/nar/26.10.2286
Guan, D. L., Ma, L. B., Khan, M. S., Zhang, X. X., Xu, S. Q., Xie, J. Y., et al. (2018). Analysis of codon usage patterns in Hirudinaria manillensis reveals a preference for GC-ending codons caused by dominant selection constraints. BMC Genomics 19 (1), 542. doi:10.1186/s12864-018-4937-x
Hachinski, V. (2019). Dementia: New vistas and opportunities. Neurol. Sci. 40 (4), 763–767. doi:10.1007/s10072-019-3714-1
Hanagasi, H. A., Tufekcioglu, Z., and Emre, M. (2017). Dementia in Parkinson’s disease. J. Neurol. Sci. 374, 26–31. doi:10.1016/j.jns.2017.01.012
Harold, D., Abraham, R., Hollingworth, P., Sims, R., Gerrish, A., Hamshere, M. L., et al. (2009). Genome-wide association study identifies variants at CLU and PICALM associated with Alzheimer’s disease. Nat. Genet. 41 (10), 1088–1093. doi:10.1038/ng.440
He, B., Dong, H., Jiang, C., Cao, F., Tao, S., Xu, L. A., et al. (2016). Analysis of codon usage patterns in Ginkgo biloba reveals codon usage tendency from A/U-ending to G/C-ending. Sci. Rep. 6, 35927. doi:10.1038/srep35927
Hinz, F. I., and Geschwind, D. H. (2017). Molecular genetics of neurodegenerative dementias. Cold Spring Harb. Perspect. Biol. 9 (4), a023705. doi:10.1101/cshperspect.a023705
Huang, X., Xu, J., Chen, L., Wang, Y., Gu, X., Peng, X., et al. (2017). Analysis of transcriptome data reveals multifactor constraint on codon usage in Taenia multiceps. BMC Genomics 18 (1), 308. doi:10.1186/s12864-017-3704-8
Jenkins, G. M., and Holmes, E. C. (2003). The extent of codon usage bias in human RNA viruses and its evolutionary origin. Virus Res. 92 (1), 1–7. doi:10.1016/s0168-1702(02)00309-x
Jiao, B., Liu, H., Guo, L., Xiao, X., Liao, X., Zhou, Y., et al. (2021). The role of genetics in neurodegenerative dementia: A large cohort study in South China. NPJ Genom. Med. 6 (1), 69. doi:10.1038/s41525-021-00235-3
Khandia, R., Singhal, S., Kumar, U., Ansari, A., Tiwari, R., Dhama, K., et al. (2019). Analysis of nipah virus codon usage and adaptation to hosts. Front. Microbiol. 10, 886. doi:10.3389/fmicb.2019.00886
Karlin, S., and Mrázek, J. (1997). Compositional differences within and between eukaryotic genomes. Proc. Natl. Acad. Sci. U. S. A. 94 (19), 10227–10232. doi:10.1073/pnas.94.19.10227
Khandia, R., Ali Khan, A., Alexiou, A., Povetkin, S. N., and Verevkina, M. N. (2022). Codon usage analysis of pro-apoptotic bim gene isoforms. J. Alzheimers Dis. 28, 1711–1725. doi:10.3233/JAD-215691
Khandia, R., Alqahtani, T., and Alqahtani, A. M. (2021). Genes common in primary immunodeficiencies and cancer display overrepresentation of codon CTG and dominant role of selection pressure in shaping codon usage. Biomedicines 9 (8), 1001. doi:10.3390/biomedicines9081001
Kindell, J., Keady, J., Sage, K., and Wilkinson, R. (2017). Everyday conversation in dementia: A review of the literature to inform research and practice. Int. J. Lang. Commun. Disord. 52 (4), 392–406. doi:10.1111/1460-6984.12298
Kleerebezem, M., Boekhorst, J., van Kranenburg, R., Molenaar, D., Kuipers, O. P., Leer, R., et al. (2003). Complete genome sequence of Lactobacillus plantarum WCFS1. Proc. Natl. Acad. Sci. U. S. A. 100 (4), 1990–1995. doi:10.1073/pnas.0337704100
Kliman, R. M., and Bernal, C. A. (2005). Unusual usage of AGG and TTG codons in humans and their viruses. Gene 352, 92–99. doi:10.1016/j.gene.2005.04.001
Kumar, U., Khandia, R., Singhal, S., Puranik, N., Tripathi, M., Pateriya, A. K., et al. (2021). Insight into codon utilization pattern of tumor suppressor gene EPB41L3 from different mammalian species indicates dominant role of selection force. Cancers (Basel) 13 (11), 2739. doi:10.3390/cancers13112739
Lambert, J. C., Heath, S., Even, G., Campion, D., Sleegers, K., Hiltunen, M., et al. (2009). Genome-wide association study identifies variants at CLU and CR1 associated with Alzheimer’s disease. Nat. Genet. 41 (10), 1094–1099. doi:10.1038/ng.439
Livingston, G., Huntley, J., Sommerlad, A., Ames, D., Ballard, C., Banerjee, S., et al. (2020). Dementia prevention, intervention, and care: 2020 report of the lancet commission. Lancet 396 (10248), 413–446. doi:10.1016/S0140-6736(20)30367-6
Lu, Z., Zhou, T., Gu, W., Ma, J., and Sun, X. (2005). Analysis of synonymous codon usage in H5N1 virus and other influenza A viruses. Biosystems. 81 (1), 77–86. doi:10.1016/j.biosystems.2005.03.002
Makhija, A., and Kumar, S. (2015). Analysis of synonymous codon usage in spike protein gene of infectious bronchitis virus. Can. J. Microbiol. 61 (12), 983–989. doi:10.1139/cjm-2015-0418
Miller, J. E., Shivakumar, M. K., Risacher, S. L., Saykin, A. J., Lee, S., Nho, K., et al. (2018). Codon bias among synonymous rare variants is associated with Alzheimer’s disease imaging biomarker. Pac. Symp. Biocomput. 23, 365–376.
Munjal, A., Khandia, R., Shende, K. K., and Das, J. (2020). Mycobacterium lepromatosis genome exhibits unusually high CpG dinucleotide content and selection is key force in shaping codon usage. Infect. Genet. Evol. 84, 104399. doi:10.1016/j.meegid.2020.104399
Nath Choudhury, M., Uddin, A., and Chakraborty, S. (2017). Codon usage bias and its influencing factors for Y-linked genes in human. Comput. Biol. Chem. 69, 77–86. doi:10.1016/j.compbiolchem.2017.05.005
Newman, Z. R., Young, J. M., Ingolia, N. T., and Barton, G. M. (2016). Differences in codon bias and GC content contribute to the balanced expression of TLR7 and TLR9. Proc. Natl. Acad. Sci. U. S. A. 113 (10), E1362–E1371. doi:10.1073/pnas.1518976113
Oh, E. S., and Rabins, P. V. (2019). Dementia. Ann. Intern. Med. 171 (5), ITC33–ITC48. doi:10.7326/AITC201909030
Paulson, H. L., and Igo, I. (2011). Genetics of dementia. Semin. Neurol. 31 (5), 449–460. doi:10.1055/s-0031-1299784
Powdel, B. R., Borah, M., and Ray, S. K. (2010). Strand-specific mutational bias influences codon usage of weakly expressed genes in Escherichia coli. Genes cells. 15 (7), 773–782. doi:10.1111/j.1365-2443.2010.01417.x
Puigbò, P., Bravo, I. G., and Garcia-Vallvé, S. E-C. A. I. (2008). E-CAI: A novel server to estimate an expected value of codon adaptation index (eCAI). BMC Bioinforma. 9, 65. doi:10.1186/1471-2105-9-65
Radue, R., Walaszek, A., and Asthana, S. (2019). Neuropsychiatric symptoms in dementia. Handb. Clin. Neurol. 167, 437–454. doi:10.1016/B978-0-12-804766-8.00024-8
Rao, Y., Wu, G., Wang, Z., Chai, X., Nie, Q., Zhang, X., et al. (2011). Mutation bias is the driving force of codon usage in the Gallus gallus genome. DNA Res. 18 (6), 499–512. doi:10.1093/dnares/dsr035
Rocha, E. P. C. (2004). Codon usage bias from tRNA’s point of view: Redundancy, specialization, and efficient decoding for translation optimization. Genome Res. 14 (11), 2279–2286. doi:10.1101/gr.2896904
Sabi, R., and Tuller, T. (2014). Modelling the efficiency of codon-tRNA interactions based on codon usage bias. DNA Res. 21 (5), 511–526. doi:10.1093/dnares/dsu017
Sablok, G., Nayak, K. C., Vazquez, F., and Tatarinova, T. V. (2011). Synonymous codon usage, GC(3), and evolutionary patterns across plastomes of three pooid model species: Emerging grass genome models for monocots. Mol. Biotechnol. 49 (2), 116–128. doi:10.1007/s12033-011-9383-9
Salser, W. (1978). Globin mRNA sequences: Analysis of base pairing and evolutionary implications. Cold Spring Harb. Symp. Quant. Biol. 42 (Pt 2), 985–1002. doi:10.1101/sqb.1978.042.01.099
ScienceDirect Topics Isoelectric point - an overview. Available at: https://www.sciencedirect.com/topics/nursing-and-health-professions/isoelectric-point.[cited 2022 Apr 16].
Seshadri, S., Fitzpatrick, A. L., Ikram, M. A., DeStefano, A. L., Gudnason, V., Boada, M., et al. (2010). Genome-wide analysis of genetic loci associated with Alzheimer disease. JAMA 303 (18), 1832–1840. doi:10.1001/jama.2010.574
Shen, W., Wang, D., Shi, M., Ma, L., Zhang, Y., Zhao, Z., et al. (2015). GC3-biased gene domains in mammalian genomes. Bioinforma. Oxf. Engl. 31 (19), 3081–3084. doi:10.1093/bioinformatics/btv329
Shen, W., Wang, D., Ye, B., Shi, M., Ma, L., Zhang, Y., et al. (2015). GC3-biased gene domains in mammalian genomes. Bioinformatics 31 (19), 3081–3084. doi:10.1093/bioinformatics/btv329
Simmen, M. W. (2008). Genome-scale relationships between cytosine methylation and dinucleotide abundances in animals. Genomics 92 (1), 33–40. doi:10.1016/j.ygeno.2008.03.009
Song, H., Liu, J., Song, Q., Zhang, Q., Tian, P., Nan, Z., et al. (2017). Comprehensive analysis of codon usage bias in seven Epichloë species and their peramine-coding genes. Front. Microbiol. 8, 1419. doi:10.3389/fmicb.2017.01419
Trotta, E. (2016). Selective forces and mutational biases drive stop codon usage in the human genome: A comparison with sense codon usage. BMC Genomics 17, 366. doi:10.1186/s12864-016-2692-4
Uddin, A., and Chakraborty, S. (2019). Codon usage pattern of genes involved in central nervous system. Mol. Neurobiol. 56 (3), 1737–1748. doi:10.1007/s12035-018-1173-y
Uddin, A. (2020). Compositional features and codon usage pattern of genes associated with anxiety in human. Mol. Neurobiol. 57 (12), 4911–4920. doi:10.1007/s12035-020-02068-0
Wan, X. F., Xu, D., Kleinhofs, A., and Zhou, J. (2004). Quantitative relationship between synonymous codon usage bias and GC composition across unicellular genomes. BMC Evol. Biol. 4, 19. doi:10.1186/1471-2148-4-19
Wang, X., Xu, W., Fan, K., Chiu, H. C., and Huang, C. (2020). Codon usage bias in the H gene of canine distemper virus. Microb. Pathog. 149, 104511. doi:10.1016/j.micpath.2020.104511
Wei, L., He, J., Jia, X., Qi, Q., Liang, Z., Zheng, H., et al. (2014). Analysis of codon usage bias of mitochondrial genome in Bombyx mori and its relation to evolution. BMC Evol. Biol. 14 (1), 262. doi:10.1186/s12862-014-0262-4
Wu, Y., Jin, L., Li, Y., Zhang, D., Zhao, Y., Chu, Y., et al. (2021). The nucleotide usages significantly impact synonymous codon usage in Mycoplasma hyorhinis. J. Basic Microbiol. 61 (2), 133–146. doi:10.1002/jobm.202000592
Xia, X. (2007). An improved implementation of codon adaptation index. Evol. Bioinform. Online. 3, 117693430700300. doi:10.1177/117693430700300028
Yang, J., Zhu, T. Y., Jiang, Z. X., Chen, C., Wang, Y. L., Zhang, S., et al. (2010). Codon usage biases in Alzheimer’s disease and other neurodegenerative diseases. Protein Pept. Lett. 17 (5), 630–645. doi:10.2174/092986610791112666
Yang, X., Ma, X., Luo, X., Ling, H., Zhang, X., Cai, X., et al. (2015). Codon usage bias and determining forces in Taenia solium genome. Korean J. Parasitol. 53 (6), 689–697. doi:10.3347/kjp.2015.53.6.689
Yengkhom, S., Uddin, A., and Chakraborty, S. (2019). Deciphering codon usage patterns and evolutionary forces in chloroplast genes of Camellia sinensis var. assamica and Camellia sinensis var. sinensis in comparison to Camellia pubicosta. J. Integr. Agric. 18 (12), 2771–2785. doi:10.1016/s2095-3119(19)62716-4
Zhang, R., Zhang, L., Wang, W., Zhang, Z., Du, H., Qu, Z., et al. (2018). Differences in codon usage bias between photosynthesis-related genes and genetic system-related genes of chloroplast genomes in cultivated and wild solanum species. Int. J. Mol. Sci. 19 (10), E3142. doi:10.3390/ijms19103142
Zhang, Z., Dai, W., and Dai, D. (2013). Synonymous codon usage in TTSuV2: Analysis and comparison with TTSuV1. PLoS One 8 (11), e81469. doi:10.1371/journal.pone.0081469
Keywords: dementia, GC composition, compositional constraint, codon usage, nucleotide skew
Citation: Alqahtani T, Khandia R, Puranik N, Alqahtani AM, Alghazwani Y, Alshehri SA, Chidambaram K and Kamal MA (2022) Codon Usage is Influenced by Compositional Constraints in Genes Associated with Dementia. Front. Genet. 13:884348. doi: 10.3389/fgene.2022.884348
Received: 26 February 2022; Accepted: 18 April 2022;
Published: 09 August 2022.
Edited by:
Prachi Srivastava, Amity University Uttar Pradesh, IndiaCopyright © 2022 Alqahtani, Khandia, Puranik, Alqahtani, Alghazwani, Alshehri, Chidambaram and Kamal. This is an open-access article distributed under the terms of the Creative Commons Attribution License (CC BY). The use, distribution or reproduction in other forums is permitted, provided the original author(s) and the copyright owner(s) are credited and that the original publication in this journal is cited, in accordance with accepted academic practice. No use, distribution or reproduction is permitted which does not comply with these terms.
*Correspondence: Rekha Khandia, UmVraGEua2hhbmRpYUBidWJob3BhbC5hYy5pbg==, YnUucmVraGEua2hhbmRpYUBnbWFpbC5jb20=
Disclaimer: All claims expressed in this article are solely those of the authors and do not necessarily represent those of their affiliated organizations, or those of the publisher, the editors and the reviewers. Any product that may be evaluated in this article or claim that may be made by its manufacturer is not guaranteed or endorsed by the publisher.
Research integrity at Frontiers
Learn more about the work of our research integrity team to safeguard the quality of each article we publish.