- 1Key Laboratory of Real Time Tracing of Brian Circuits in Psychiatry and Neurology (RTBNP_Lab), Nankai University Affiliated Tianjin Fourth Center Hospital, Tianjin Fourth Center Hospital, Tianjin, China
- 2Digital Analysis Center of Psychiatry, Tianjin Fourth Center Hospital, Tianjin, China
- 3Department of Psychiatry and Neurology Imaging-Genetics and Comorbidity Laboratory (PNGC_Lab) of Tianjin Mental Health Center, Tianjin Anding Hospital, Tianjin, China
- 4Department of Psychiatry, The First Affiliated Hospital of Zhengzhou University, Zhengzhou, China
- 5Deep Learning Center of MRI and Genetics, Wenzhou Seventh People’s Hospital, Wenzhou, China
- 6Department of Psychiatry, Nankai University Affiliated Tianjin Fourth Center Hospital, Tianjin Fourth Center Hospital, Tianjin, China
- 7Department of Pharmacology, The First Hospital of Hebei Medical University, Shijiazhuang, China
- 8Department of Psychiatry, Wenzhou Seventh People’s Hospital, Wenzhou, China
Cognitive impairment is highly prevalent in patients with major psychiatric disorders (MPDs), including schizophrenia (SCZ), bipolar disorder, major depressive disorder, in whom it can be highly disruptive to community functioning and worsen prognosis. Previously, genetic factors and cognitive impairments in MPD patients have been examined mostly in isolated circuits rather than in the whole brain. In the present study, genetic, neuroimaging, and psychometric approaches were combined to investigate the relationship among genetic factors, alterations throughout the brain, and cognitive impairments in a large cohort of patients diagnosed with SCZ, with a reference healthy control (HC) group. Single nucleotide polymorphisms (SNPs) in SCZ-risk genes were found to be strongly related to cognitive impairments as well as to gray matter volume (GMV) and functional connectivity (FC) alterations in the SCZ group. Annotating 136 high-ranking SNPs revealed 65 affected genes (including PPP1R16B, GBBR2, PDE4B, CANCNA1C, SLC12AB, SATB2, MAG12, and SATB2). Only one, a PDE4B SNP (rs1006737), correlated with GMV (r = 0:19 p = 0.015) and FC (r = 0.21, p = 0.0074) in SCZ patients. GMV and FC alterations correlated with one another broadly across brain regions. Moreover, the present data demonstrate three-way SNP-FC-GMV associations in patients with SCZ, thus providing clues regarding potential genetic bases of cognition impairments in SCZ. SNP-FC-GMV relationships correlated with visual learning and reasoning dimensions of cognition. These data provide evidence that SCZ-related cognitive impairments may reflect genetically underlain whole-brain structural and functional alterations.
Introduction
Schizophrenia (SCZ), which has a worldwide prevalence of about 1%, is a highly debilitating major psychiatric disorder (MPD) that, in addition to psychosis symptoms, commonly involves impaired cognition (Insel, 2010). Cognition refers to the diverse assembly of abilities that allow an individual to recognize, process, and respond to information (Smeland et al., 2020). Consequently, cognitive impairment alters how one perceives, processes, and reacts to external information, which can lead to bizarre thinking, soundtrack language, and abnormal behavior (González-Blanch et al., 2006). Among patients with MPDs, impaired cognition is seen most prominently in patients with SCZ (60–80%) (González-Blanch et al., 2006; Smeland et al., 2020) though it is also observed in patients with bipolar disorder (Jenkins, 2019; Zhu et al., 2019) and depression (Miskowiak et al., 2016).
Cognitive ability is an important consideration in patients with MPDs because it affects their ability to function within the community (Reilly and Sweeney, 2014). Cognitive impairment has been observed by several groups to precede MPD onset and then to deteriorate within the first 2–3 years that patients are medicated, causing challenges even in remission (Allen et al., 2003; Ivleva et al., 2010; McCleery and Nuechterlein, 2019). The impairment severity appears to stabilize after 5–10 years of treatment (Holthausen et al., 2002; Allen et al., 2003; Keefe et al., 2005; Vaskinn et al., 2014). Cognitive impairment in patients with SCZ specifically has been reported to be accompanied by a 2% loss in gray matter (GM) and a 1% loss in white matter (WM), accounting for a whole-brain volumetric loss of about 2.6% within 2 years of illness onset, followed by relative stability (Vita et al., 2012). SCZ-associated cognitive impairments have been demonstrated in the important realms of attention, memory, language, executive function, and social cognitive function (Nuechterlein et al., 2004), and GM alterations have been related to cognitive impairment and brain functional alterations in schizophrenic patients with cognitive impairments (Jauhar et al., 2022).
There has been a diversity of evidence regarding potential mechanisms of cognitive impairment in MPDs, especially SCZ. For example, hippocampus-prefrontal lobe linking theta oscillations have been shown to be related to cognitive performance in patients with SCZ (Soltani Zangbar et al., 2020). Additionally, the functional magnetic resonance imaging (fMRI) findings in patients with SCZ reported by Luck and Vogel, (2013) revealed hypoactivity in memory-related brain regions and hyperactivity in other brain regions. Zaytseva et al. (2018) related SCZ-related cognitive impairments to localized aberrations in brain activity that lead gradually to the disturbance of whole-brain networks (Zaytseva et al., 2018; Luck et al., 2019). Sutcliffe et al. (2016) suggested that the cognitive impairments may reflect an interaction of risk genes with activity disturbances in the anterior cingulate and dorsolateral prefrontal cortex. These various lines of evidence have in common an indication that cognitive impairments in SCZ reflect a whole-brain systemic disturbance. Hence, elucidating the underlying pathological features should be based on whole-brain systemic theory.
Cognitive impairments in patients with SCZ have been linked to genetic single nucleotide polymorphisms (SNPs) (Eum et al., 2021). For example, in a meta-analysis, Rees et al. (2014) identified five SNPs as risk factors for SCZ-associated cognitive impairment: 1q21.1del; 1q21.1dupl; NRXN1 del; 16p11.2 dup; and 22q11.2 del. Waddington et al. (2021) reported that expression of dysbindin, encoded by the SCZ-risk associated gene DTNBP1 (dystrobrevin-binding protein-1) may be related to cognitive impairments in SCZ, especially impairments affecting working memory, attention, and executive function. In addition, Matosin et al. (2018) reported evidence relating cognitive impairment to aberrant regulation of glutamate metabotropic receptor subtype 5, encoded by GRM5; and Iwamoto and Ouchi (2014) reported that insulin-like growth factor 2 can influence cognitive performance. In their meta-analysis, Bozza et al. (2013) found that loss of the 6p25 locus can induce serious cognitive impairments, especially in speech and language. The authors of these above-mentioned studies all discussed cognitive impairment preceding SCZ onset; furthermore, some even noted cognitive impairments in non-schizophrenic individuals with the identified SNPs who were at high risk of SCZ (i.e., those with a first degree relative with SCZ).
In the last two decades, there has been growth in research focused on investigating cognition impaired in patients with SCZ, most notably including recent studies employing genome-wide association study (GWAS) and neuroimaging techniques and relating detected gene variants and brain alterations to cognitive performance. For example, Bhattacharyya et al. found that 12 SNPs in human accelerated regions were associated with cognitive performance in the patients with SCZ (Maria Teixeira et al., 2020). Chen et al. reported evidence suggesting that the rs3811655 variant allele of TF may act as a moderator of the association between Cu/Zn-SOD activity and cognition (Mancuso et al., 2021), whereas Zakowicz et al. (2022). Reported that the rs1800871 and rs18008729 variant alleles of IL10 were highly predictive of poor cognitive performance in patients with SCZ (Smeland et al., 2017). Fu et al. (2021) reported that the CMIP allele variant rs2287112 was related to cognition ability in patients with SCZ. Based on GWAS data, Smeland et al. (2017) who have related numerous genetic loci to cognitive traits, including intelligence, general cognition, reaction time, and verbal-numerical reasoning identified 21 loci that influence both SCZ and cognitive traits, including 2 loci that influence verbal-numerical reasoning, 6 loci that influence reaction time, and 14 that influence general cognitive function. One particularly relevant SCZ-associated locus was related to two cognitive traits (Jenkins, 2019). Studies using advanced neuroimaging techniques have revealed structural and functional alterations in the brain that can be related to SNPs and cognitive deficits. For example, Dixon et al. reported that decreased GABA or glutamate concentrations in frontal lobe areas correlated with cognitive performance in patients with SCZ (Miskowiak et al., 2016), Picó-Pérez et al. reported that structural aberrations in the neocortex (frontal, parietal, occipital, and temporal lobes) and in subcortical structures (amygdala, nucleus accumbens, caudate, putamen, and pallidum) correlated with cognitive dysfunction in patients with SCZ (Reilly and Sweeney, 2014). Dienel et al. (2022) found that genetic changes associated with altered excitatory signaling in layer 3 pyramidal neurons were associated with cognitive impairments in the prodromal phase of SCZ (Ivleva et al., 2010). Importantly, Mahmoudi et al. (2021) reported that rs58335419, a variable number tandem repeat variant of MIR137, is associated with cognitive impairment and altered cortical morphology in patients with SCZ (McCleery and Nuechterlein, 2019). Schweiger et al. (2019) reported that rs6265, a missense BDNF SNP allele, was associated with impaired cognition in the patients with SCZ (Allen et al., 2003). Yu et al. (2016) reported that the rs1063843 allele of CAMKK2 was disruptive to dorsolateral prefrontal cortex functional activity and associated with reduced cognitive ability in the patients with SCZ. Future neuroimaging studies may help to clarify the aforementioned and other yet to be identified genetic alternations affect the brains of patients with SCZ.
The aim of this study was to use genetic, neuroimaging, and psychometric techniques to explore how genetics may relate to brain alterations and cognitive impairments in patients with SCZ. Brain alterations were assessed in terms of differences in gray matter volume (GMV) and functional connectivity (FC). We hypothesized that: 1) genetic factors can be associated with SCZ-related cognitive impairment; 2) brain structural and functional alterations, represented as GMVs and FCs, can be associated with cognitive impairments; and 3) genetic factors and brain alterations may interact in causing cognitive impairments in patients with SCZ.
Materials and methods
Subjects
We recruited 80 healthy control (HC) subjects and 78 patients with schizophrenia (SCZ) to participate in this study. All participants were recruited from the Psychiatric Department at Tianjin Fourth Centre Between 1 March 2020 and 28 February 2021. The Ethics committee of Tianjin Fourth Center Hospital approved this study. All participants signed informed consent forms.
The inclusion criteria for the SCZ group were as follows: confirmed diagnosis by two psychiatrists using the SCID (Structured Clinical Interview for DSM Disorders) according to the criteria of DSM-IV (Diagnostic and Statistical Manual of Mental Disorders, Fourth Edition) criteria; 18–35 years old; no diagnostic history of any neurological comorbidity or serious other comorbidity, including diabetes; no history of drug or alcohol dependence; and no contraindications for magnetic resonance scanning.
The HC group participants were matched with SCZ case participants with respect to age and gender, had no history of neurological or mental illness, and no family history of MPD within three generations of immediate relatives (screened with the SCID non-patient edition).
Additionally, to remove the potential influence of abnormal blood glucose on cognitive performance in this study (28, 29), we excluded potential participants meeting the following definition of prediabetes: >5.6 mmol/L or plasma glucose of 2 hours after meal >7.8 mmol/L. After excluding participants meeting either component of this definition (i.e. 45 SCZ patients and 46 HCs), we included 33 SCZ patients and 34 HCs in the final analysis.
Genetic data acquisition
All participants provided blood samples for genetic analysis. Genomic DNA was extracted via the standard protocol (protease K digestion, phenol-chloroform extraction, and ethanol precipitation) and submitted to whole-genome genotyping of 571,054 loci with Infinium™ PsychArray-24 v1.3 BeadChip kits (Illumina China).
Imaging data acquisition
Structural data and functional data were acquired with a 3.0-T Siemens trio scanner at the Medical Imaging Department of Tianjin Fourth Central Hospital. Soft foam pads were fitted to each subject’s head to reduce head movement and scanner noise. All participants were asked to keep their eyes closed and to relax during scanning, but do not fall asleep, and to avoid head movements as much as possible. The scanning parameters for resting fMRI were: repetition time (TR), 2000 ms; echo time (TE), 30 ms; flip angel, 90°; matrix, 64 × 64; field of view (FOV), 220 × 220; slice thickness, 4 cm; and voxel size 3.4 × 3.4 × 3.4. The scanning parameters for structural magnetic resonance image were: TR, 2530 ms; TE, 3.4 ms; flip angel, 7°; matrix, 256 × 256; FOV, 256 × 256; slice thickness, 1.0 cm; and voxel size 1 × 1 × 1 (Zhuo et al., 2017).
Imaging data preprocessing
Structural data were analyzed by voxel-based morphology in SPM8 software (www.fil.Ion.UCL. Ac.uk/SPM)/Software/spm). Voxel-based morphology data preprocessing included the following steps: 1) three-dimensional images were registered in Montreal Neurological Institute standard space; 2) brain tissue within the images was divided into GM, WM, and cerebrospinal fluid (CSF) sections according to a priori probability and image gray information; 3) spatial registration-related brain volume alterations were corrected by modulation; 4) Gaussian smoothing was applied to suppress the signal-to-noise ratio. Subsequently, to adapt to the correlation analysis algorithm, subject’s GMV voxel values were aligned in a row vector to obtain a characteristic matrix integrated by the row vectors of all subjects within a group (Zhuo et al., 2017).
GMV determination
GMVs were determined in SPM8 software (http://www.fil.ion.ucl.ac.uk/spm/software/spm8/). In brief, the standard unified segmentation model was used for segmentation of structural MR images into GM, WM, and CSF. Following an initial affine registration of the GM concentration map into the MNI space, diffeomorphic anatomical registration with the exponentiated lie algebra technique was used to warp GM concentration images nonlinearly, and then the imaging data were resampled at a cubic voxel size of 3 mm3. GMVs were calculated voxel-wise by multiplying the GM concentration map by the non-linear determinants from the spatial normalization step. GMV images were smoothed with a full-width at half maximum cubic Gaussian kernel (6 mm3) and subjected to spatial preprocessing to obtain smoothed GM maps for statistical analyses (Zhuo et al., 2017).
FC analysis
FC analyses were conducted in SPM8 (http://www.fil.ion.ucl.ac.uk/spm/software/spm8/). Briefly, the first ten volumes were eliminated. The functional images were slice-time corrected, realigned, normalized to a standard MNI space, and re-sliced to a 3-mm slice thickness. Twenty-four motion parameters, together with linear drift, CSF, and WM signals, were then regressed out, filtered (0.01–0.08 Hz), and smoothed with a full-width half of maximum of 6 mm × 6 mm × 6 mm. We defined 256 FC matrix elements and binary FC matrix elements based on the 246 Brainnetome regions, including subcortical nuclei. The 1% most-positive FCs were retained and then their associated network properties were examined with the Brain Connectivity Toolbox as described by Meunier et al. (2020). We calculated FC modularity according to the binary FC matrix (Tohka et al., 2016) to ensure consistency across groups. Subsequently, the binary FC matrix then corresponded to eight Yeo networks based on module segregation index values by Hsu et al. (2020) method. Intranetwork and internetwork connections in and among the eight Yeo networks were quantitated as described in detail in our recent work (Xuan et al., 2022).
Global functional connectivity density calculation
We calculated a gFCD value for each voxel using an in-house Linux script. FCs were compared across voxels with Pearson’s linear correlation analysis (correlation coefficient threshold, R > 0.6). On the basis of the whole-brain GMV, the gFCD of each voxel (×0) was calculated with a growth algorithm in accordance with the total FC [k (x0)] between x0 and all other voxels. To improve the normality of the gFCD value distribution, each gFCD value was divided by the mean gFCD of all included voxels. During gFCD determination, FCD maps were smoothed with a 6 mm × 6 mm × 6 mm Gaussian kernel to minimize functional anatomy differences as described previously (Yao et al., 2021).
Association among GMV, FC, and gFCD calculation
Combination analysis was employed to confirm the predominance of SNP-GM-FC correlation and the existence of group differences and to test whether other factors, such as group information, do interfere with inter-modal correlation. Pair-wise inter-modal correlations were determined after controlling for age, gender, education level, and group (Li et al., 2021).
Data analysis
Inter-group variances in MCCB scores and GMVs were detected with analyses of variance (ANOVAs). Inter-group differences in FC and gFCD were detected with student test. Pearson correlational analyses were conducted across variables. Statistical tests were conducted in SPSS version 21.0 (IBM, United States).
Results
Cognitive impairments
ANOVAs demonstrated that group (SCZ vs. HC) had a main effect on all eight MCCB dimension subscores and the MCCB composite score (Table 1).
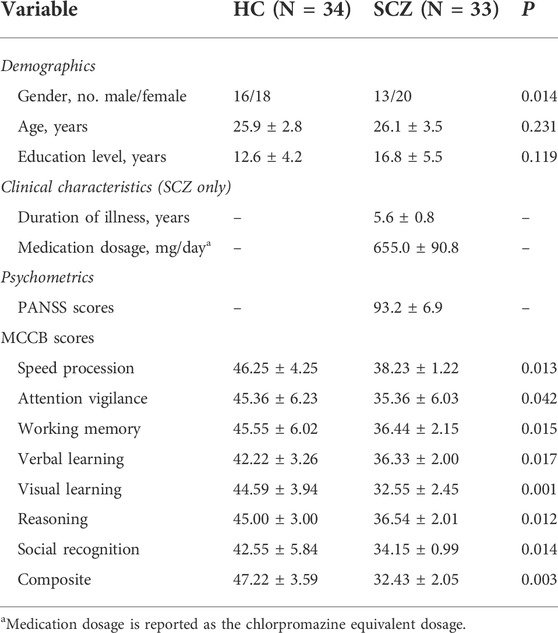
TABLE 1. Reporting and comparison of demographics, clinical characteristics, and psychometric variables across schizophrenia (SCZ) and healthy control (HC) groups.
GMV alterations
ANOVAs showed that, compared to the HC group, the SCZ group had significantly decreased GMVs in the hippocampus, temporal lobe, amygdala, and cerebellum.
FC and gFCD alterations
Compared to the HC group, the SCZ group had decreased FC between the postcentral gyrus and amygdala, increased FC between the parietal lobe and cerebellum (Figure 1), increased gFCD in the orbital frontal lobe, medial prefrontal cortex, parietal lobe, and right temporal lobe, and decreased gFCD in the left temporal lobe and bilateral hippocampus. Follow-up region-of-interest analyses of brain regions with aberrant gFCD in the SCZ group demonstrated that basal ganglia FC with the postcentral gyrus and left temporal lobe was decreased in the SCZ group while basal ganglia FC with the parietal lobe and occipital lobe was increased in the SCZ group (Figure 2), with the latter increases correlating significantly with PANSS negative symptoms scores in the SCZ group (Figure 3).
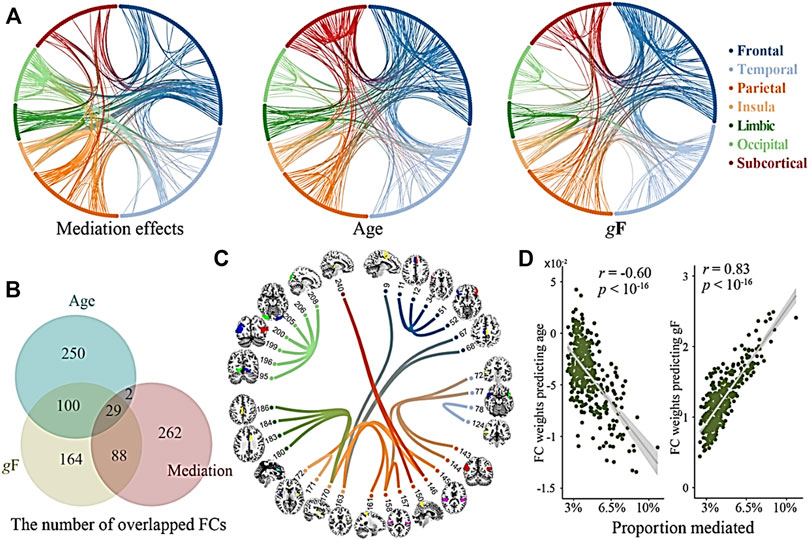
FIGURE 1. Coupling of GMV and gFCD alterations. (A) The mediations effects of GMV and gFCD when age and global structural connection (gF) are regressed out. (B) The numbers of overlapped GMV and gFCD connections (age, mediation effects, and gF are regress out). (C) Overlapped GMV and gFCD are associated with cognitive deficits. (D) Proportion mediated of age and global structural connections (gF) in the correlations of total functional connectivity (FC).
Genetic alterations
The genetic data were preprocessed in PLINK, applying quality control procedures, and overlapped with PGC’s SCZ2 database. Applying a significance threshold of p < 0.01 yielded 4,937 SNPs. Discrete numbers were assigned to the categorical genotypes: 0, no minor allele; 1, one minor allele; and 2, two minor alleles. SNP components were transformed to Z scores and visualized at |Z| > 2. Applying this cutoff yielded 258 SNPs, of which 136 were high-ranking, involving 101 genes, including SATB2, GABBR2, PDE4B, and CACNA1C, among others (Table 2; Figure 2).
Association analysis
None of the analyzed demographic factors (age, gender, educational level) had a significant relationship with FC or GMV alterations in the SCZ group (data not shown). Regarding clinical factors, SCZ illness duration correlated with FC or GMV alterations while anti-psychotic agent dose exposure did not (data not shown). Surprisingly, gFCD values did not associate with FC or GMV alterations.
Compared to HCs, the SCZ patients had significant incidences of SNPs in PDE4B, SATB2, SLC12A8, EGF, CACNA1C, MAG12, GABBR2, CACAN1C, PPP1R16B (Figure 3). Only the SNP that was associated with the rs1006737 allele of PDE4B correlated significantly with GMV (r = 0:19; p = 0.015) and FC (r = 0.21, p = 0.0074) in SCZ patients after controlling for age, gender, education level, and group (Bonferroni corrected, Figure 2).
SNP components were transformed to Z scores and visualized at |Z| > 2. This threshold revealed 136 risk SNPs (see Manhattan plot of these data in Figure 2). Annotation of these 136 SNPs indicated that they were associated with 65 genes, notably including PPP1R16B, GBBR2, PDE4B, CANCNA1C, SLC12AB, SATB2, MAG12, and SATB2. One PDE4B SNP, namely that associated with the rs1006737 allele, correlated significantly with GMV (r = 0:19 p = 0.015) and FC (r = 0.21, p = 0.0074) in the SCZ patient group after controlling for age, gender, education level, and group (Bonferroni corrected, Figure 2). None of SNPs correlated with gFCD values.
GMVs with a high gFCD (rank order: left temporal lobe > right orbital frontal lobe > left hippocampus > left occipital lobe > right cerebellum) correlated with five MCCB dimension subscores (Figure 3). With respect to positive correlations, GMVs correlated most strongly with speed procession subscores (r = 0.69) and most weakly with social recognition subscores (r = 0.59). With respect to negative correlations, GMVs in the prefrontal lobe correlated negatively with visual learning subscores while GMVs in the anterior cingulate cortex correlated negatively with reasoning subscores, thus relating GMV-gFCD to cognitive impairments.
In summary, combining GWAS and fMRI techniques we found that rs1006737 an SNP allele of PDE4B, associated with GMVs within the hippocampus, temporal lobe, amygdala, and cerebellum in patients with SCZ. Interestingly, our data also demonstrated that the presence of the SNP allele rs1006737 was associated with decreased postcentral gyrus–amygdala FC and increased parietal lobe–cerebellum FC. Additionally, these FCs also correlated with altered GMVs and rs1006737.
Discussion
In the present study we employed an integrated research strategy encompassing genotyping, cognitive function testing, and neuroimaging to investigate the impact of genetic variations on brain structure and function in patients with SCZ and thus produced evidence that may help to explain why cognitive impairments occur in this patient population. Overall, the present study supports the neurodevelopmental hypothesis of SCZ, which posits that stress events may alter the expression of predisposing SCZ-susceptibility genes and thereby lead to the pathogenesis of a schizophrenic episode (Vaskinn et al., 2014).
SZ is a highly heritable MPD in which a large number of SCZ-susceptibility genetic variations have been identified and characterized, especially since genome-wide association analysis has become feasible and affordable. We observed significant GMV reductions in the hippocampus, temporal lobe, amygdala, and cerebellum that were associated with SNPs in SZ-susceptibility genes including GBBR2, SATB2, CACNA1C, and PDE4B, which together with EGF have been identified as the top-contributing SNPs in SZ (Chen et al., 2019; Zhuo et al., 2019). Notably, these genes have been related to molecular pathways essential to cognitive functioning, including chemical synapses, cell junctions, and neuron projections and thus their alteration may contribute to the development of cognitive impairments in SCZ (Zhuo et al., 2020).
Our data demonstrating that SNPs in known SCZ-susceptibility genes, such as those listed above, can be associated with alterations in brain structure, as indexed with GMVs, and brain FC suggest that innate susceptibility genes may induce brain functional aberrations when the brain’s functional capacities cannot compensate for deficits, leading to structural alterations, such as GMV reduction (Berrettini, 2003). Moreover, our data demonstrating that SNPs that have been related to cognitive deficits were observed in our patients with SCZ support the view that SCZ-related cognitive deficits precede clinical schizophrenia onset (Green, 1996; Bowie and Harvey, 2006; Schaefer et al., 2013; Luo et al., 2018).
Importantly, the present data demonstrate three-way SNP- GMV-FC associations in patients with SCZ, thus providing clues regarding potential genetic bases of cognition impairments in SCZ. This work, together with prior studies (Jenkins, 2019), provides empirical evidence in support of a three-way SNP- GMV-FC interaction underlying cognitive impairment in SCZ. Given that impaired cognition is observed in other MPDs (McCleery and Nuechterlein, 2019), it would be of interest to examine how SNP- GMV-FC relationships in SCZ may compare to SNP- GMV-FC relationships in other MPDs, especially with respect to cognitive deficit severity, which has been shown to be worse in male schizophrenia patients, versus in female patients, and in patients with early onset of schizophrenia symptoms (McCleery and Nuechterlein, 2019).
SNP- GMV- FC relationships did not correlated with any dimensions of the MCCB is an unexpectedly. However, it is unclear why gFCD did not associate significantly with other brain indices in our study. If gFCD reflects connection number, then this lack of correlation may mean that SCZ patients may experience FC strength alterations without overall changes in connection quantity. This possibility remains to be examined in future research.
Limitations
There are several limitations to our study. First, the patients all had chronic SCZ (illness duration >5 years). Over the course of a long-term mental, illness, cognitive impairments could be influenced by many factors. Hence, in a future study, first-episode patients and high-risk individuals (e.g., first-degree relatives of MPD patients) should be examined to elucidate the trajectory of cognitive impairment. Second, our patients were all being treated with high-dosage anti-psychotic agents, which themselves can affect cognitive performance. Third, although we used genetic techniques to test the relationship between SNPs and cognitive impairments, cognitive performance may be influenced by additional environmental factors. Fourth, our data do not explain the phenomenon of SNP- GM-FC bi-directional correlations with MCCB dimension sub-scores.
Conclusion
The present findings support the hypotheses that SCZ-related cognitive impairments are related to genetic factors (H1) and brain structural and functional alterations (H2). Furthermore, these findings are consistent with the hypothesis that genetic factors and brain alterations may interact in causing cognitive impairments in the patients with SCZ (H3). The biological mechanisms by which genetic factor-related whole brain structural and functional alterations lead to SCZ-associated cognitive impairments remain to be elucidated.
Data availability statement
The original contributions presented in the study are included in the article/supplementary material, further inquiries can be directed to the corresponding authors.
Ethics statement
The studies involving human participants were reviewed and approved by Tianjin Fourth Center Hospital Committee of IRB. The patients/participants provided their written informed consent to participate in this study. Written informed consent was obtained from the individual(s) for the publication of any potentially identifiable images or data included in this article.
Author contributions
CZ, HT, JC, and QL: conceptualization, methodology, software, analysis, investigation, writing/original draft preparation. LY and QZ: software, analysis, writing/reviewing, and editing. GC, LC, and CZ: software, investigation, writing/reviewing, and editing. XS and CZ: conceptualization and supervision.
Funding
This work was supported by grants from the National Natural Science Foundation of China (81871052, 82171503), the Key Projects of the Natural Science Foundation of Tianjin (17JCZDJC35700), the Tianjin Health Bureau Foundation (2014KR02), and the Tianjin Science and Technology Bureau (15JCYBJC50800).
Acknowledgments
We would like to thank all participants for their contributions to this study.
Conflict of interest
The authors declare that the research was conducted in the absence of any commercial or financial relationships that could be construed as a potential conflict of interest.
Publisher’s note
All claims expressed in this article are solely those of the authors and do not necessarily represent those of their affiliated organizations, or those of the publisher, the editors and the reviewers. Any product that may be evaluated in this article, or claim that may be made by its manufacturer, is not guaranteed or endorsed by the publisher.
References
Allen, D. N., Goldstein, G., and Warnick, E. (2003). A consideration of neuropsychologically normal schizophrenia. J. Int. Neuropsychol. Soc. 9, 56–63. doi:10.1017/s135561770391006x
Berrettini, W. (2003). Evidence for shared susceptibility in bipolar disorder and schizophrenia. Am. J. Med. Genet. C Semin. Med. Genet. 123, 59–64. doi:10.1002/ajmg.c.20014
Bowie, C. R., and Harvey, P. D. (2006). Cognitive deficits and functional outcome in schizophrenia. Neuropsychiatr. Dis. Treat. 2, 531–536. doi:10.2147/nedt.2006.2.4.531
Bozza, M., Bernardini, L., Novelli, A., Brovedani, P., Moretti, E., Canapicchi, R., et al. (2013). 6p25 interstitial deletion in two dizygotic twins with gyral pattern anomaly and speech and language disorder. Eur. J. Paediatr. Neurol. 17, 225–231. doi:10.1016/j.ejpn.2012.09.008
Chen, M., Zhuo, C. J., Ji, F., Li, G. Y., and Ke, X. Y. (2019). Brain function differences in drug-naïve first-episode auditory verbal hallucination-schizophrenia patients with versus without insight. Chin. Med. J. 132, 2199–2205. doi:10.1097/cm9.0000000000000419
Dienel, S. J., Schoonover, K. E., and Lewis, D. A. (2022). Cognitive dysfunction and prefrontal cortical circuit alterations in schizophrenia: Developmental trajectories. Biol. Psychiatry S0006-3223 (22), 01072. doi:10.1016/j.biopsych.2022.03.002
Eum, S., Hill, S. K., Alliey-Rodriguez, N., Stevenson, J. M., Rubin, L. H., Lee, A. M., et al. (2021). Genome-wide association study accounting for anticholinergic burden to examine cognitive dysfunction in psychotic disorders. Neuropsychopharmacology 46, 1802–1810. doi:10.1038/s41386-021-01057-8
Fu, Y., Ren, X., Bai, W., Yu, Q., Sun, Y., Yu, Y., et al. (2021). Association between C-Maf-inducing protein gene rs2287112 polymorphism and schizophrenia. PeerJ 9, e11907. PMID: 34484985; PMCID: PMC8381876. doi:10.7717/peerj.11907
González-Blanch, C., Alvarez-Jiménez, M., Rodríguez-Sánchez, J. M., Pérez-Iglesias, R., Vázquez-Barquero, J. L., Crespo-Facorro, B., et al. (2006). Cognitive functioning in the early course of first-episode schizophrenia spectrum disorders: Timing and patterns. Eur. Arch. Psychiatry Clin. Neurosci. 256, 364–371. doi:10.1007/s00406-006-0646-6
Green, M. F. (1996). What are the functional consequences of neurocognitive deficits in schizophrenia? Am. J. Psychiatry 153, 321–330. doi:10.1176/ajp.153.3.321
Holthausen, E. A., Wiersma, D., Sitskoorn, M. M., Hijman, R., Dingemans, P. M., Schene, A. H., et al. (2002). Schizophrenic patients without neuropsychological deficits: Subgroup, disease severity or cognitive compensation? Psychiatry Res. 112, 1–11. doi:10.1016/s0165-1781(02)00184-1
Hsu, A., Yeo, Z. Y., Rauber, R., Sun, J., Kim, Y., Raghavan, S., et al. (2020). ClimActor, harmonized transnational data on climate network participation by city and regional governments. Sci. Data 7, 374. doi:10.1038/s41597-020-00682-0
Ivleva, E. I., Morris, D. W., Moates, A. F., Suppes, T., Thaker, G. K., Tamminga, C. A., et al. (2010). Genetics and intermediate phenotypes of the schizophrenia--bipolar disorder boundary. Neurosci. Biobehav. Rev. 34, 897–921. doi:10.1016/j.neubiorev.2009.11.022
Iwamoto, T., and Ouchi, Y. (2014). Emerging evidence of insulin-like growth factor 2 as a memory enhancer: A unique animal model of cognitive dysfunction with impaired adult neurogenesis. Rev. Neurosci. 25, 559–574. doi:10.1515/revneuro-2014-0010
Jauhar, S., Johnstone, M., and McKenna, P. J. (2022). Schizophrenia. Lancet 399, 473–486. doi:10.1016/s0140-6736(21)01730-x
Jenkins, A. C. (2019). Rethinking cognitive load: A default-mode network perspective. Trends Cogn. Sci. 23, 531–533. doi:10.1016/j.tics.2019.04.008
Keefe, R. S., Eesley, C. E., and Poe, M. P. (2005). Defining a cognitive function decrement in schizophrenia. Biol. Psychiatry 57, 688–691. doi:10.1016/j.biopsych.2005.01.003
Li, H. J., Zhang, C., Hui, L., Zhou, D. S., Li, Y., Zhang, C. Y., et al. (2021). Novel risk loci associated with genetic risk for bipolar disorder among han Chinese individuals: A genome-wide association study and meta-analysis. JAMA Psychiatry 78, 320–330. doi:10.1001/jamapsychiatry.2020.3738
Luck, S. J., Leonard, C. J., Hahn, B., and Gold, J. M. (2019). Is attentional filtering impaired in schizophrenia? Schizophr. Bull. 45, 1001–1011. doi:10.1093/schbul/sbz045
Luck, S. J., and Vogel, E. K. (2013). Visual working memory capacity: From psychophysics and neurobiology to individual differences. Trends Cogn. Sci. 17, 391–400. doi:10.1016/j.tics.2013.06.006
Luo, N., Tian, L., Calhoun, V. D., Chen, J., Lin, D., Vergara, V. M., et al. (2018). Exploring different impaired speed of genetic-related brain function and structures in schizophrenic progress using multimodal analysis. Annu. Int. Conf. IEEE Eng. Med. Biol. Soc. 2018, 4126–4129. doi:10.1109/embc.2018.8513313
Mahmoudi, E., Atkins, J. R., Quidé, Y., Reay, W. R., Cairns, H. M., Fitzsimmons, C., et al. (2021). The MIR137 VNTR rs58335419 is associated with cognitive impairment in schizophrenia and altered cortical morphology. Schizophr. Bull. 47, 495–504. doi:10.1093/schbul/sbaa123
Mancuso, E., Spiga, R., Rubino, M., Averta, C., Rotundo, S., Segura-Garcìa, C., et al. (2021). Effects of Alpha-2-HS-glycoprotein on cognitive and emotional assessment in prediabetic and diabetic subjects. J. Affect. Disord. 282, 700–706. doi:10.1016/j.jad.2020.12.135
Maria Teixeira, M., Passos, V., Barreto, S., Inês Schmidt, M., Duncan, B., Beleigoli, A., et al. (2020). Markers of adiposity, insulin resistance, prediabetes and cognitive function at baseline of the Brazilian Longitudinal Study of Adult Health (ELSA - brasil). Diabetes Res. Clin. Pract. 170, 108499. doi:10.1016/j.diabres.2020.108499
Matosin, N., Halldorsdottir, T., and Binder, E. B. (2018). Understanding the molecular mechanisms underpinning gene by environment interactions in psychiatric disorders: The FKBP5 model. Biol. Psychiatry 83, 821–830. doi:10.1016/j.biopsych.2018.01.021
McCleery, A., and Nuechterlein, K. H. (2019). Cognitive impairment in psychotic illness: Prevalence, profile of impairment, developmental course, and treatment considerations. Dialogues Clin. Neurosci. 21, 239–248. doi:10.31887/DCNS.2019.21.3/amccleery
Meunier, D., Pascarella, A., Altukhov, D., Jas, M., Combrisson, E., Lajnef, T., et al. (2020). NeuroPycon: An open-source python toolbox for fast multi-modal and reproducible brain connectivity pipelines. Neuroimage 219, 117020. doi:10.1016/j.neuroimage.2020.117020
Miskowiak, K. W., Ott, C. V., Petersen, J. Z., and Kessing, L. V. (2016). Systematic review of randomized controlled trials of candidate treatments for cognitive impairment in depression and methodological challenges in the field. Eur. Neuropsychopharmacol. 26, 1845–1867. doi:10.1016/j.euroneuro.2016.09.641
Nuechterlein, K. H., Barch, D. M., Gold, J. M., Goldberg, T. E., Green, M. F., Heaton, R. K., et al. (2004). Identification of separable cognitive factors in schizophrenia. Schizophr. Res. 72, 29–39. doi:10.1016/j.schres.2004.09.007
Rees, E., Walters, J. T., Georgieva, L., Isles, A. R., Chambert, K. D., Richards, A. L., et al. (2014). Analysis of copy number variations at 15 schizophrenia-associated loci. Br. J. Psychiatry 204, 108–114. doi:10.1192/bjp.bp.113.131052
Reilly, J. L., and Sweeney, J. A. (2014). Generalized and specific neurocognitive deficits in psychotic disorders: Utility for evaluating pharmacological treatment effects and as intermediate phenotypes for gene discovery. Schizophr. Bull. 40, 516–522. doi:10.1093/schbul/sbu013
Schaefer, J., Giangrande, E., Weinberger, D. R., and Dickinson, D. (2013). The global cognitive impairment in schizophrenia: Consistent over decades and around the world. Schizophr. Res. 150, 42–50. doi:10.1016/j.schres.2013.07.009
Schweiger, J. I., Bilek, E., Schäfer, A., Braun, U., Moessnang, C., Harneit, A., et al. (2019). Effects of BDNF Val66Met genotype and schizophrenia familial risk on a neural functional network for cognitive control in humans. Neuropsychopharmacology 44, 590–597. doi:10.1038/s41386-018-0248-9
Smeland, O. B., Frei, O., Dale, A. M., and Andreassen, O. A. (2020). The polygenic architecture of schizophrenia-rethinking pathogenesis and nosology. Nat. Rev. Neurol. 16, 366–379. doi:10.1038/s41582-020-0364-0
Smeland, O. B., Frei, O., Kauppi, K., Hill, W. D., Li, W., Wang, Y., et al. (2017). Identification of genetic loci jointly influencing schizophrenia risk andthe cognitive traitsof verbal-numerical reasoning, reaction time, and general cognitive function. JAMA Psychiatry 74, 1065–1075. doi:10.1001/jamapsychiatry.2017.1986
Soltani Zangbar, H., Ghadiri, T., Seyedi Vafaee, M., Ebrahimi Kalan, A., Fallahi, S., Ghorbani, M., et al. (2020). Theta oscillations through hippocampal/prefrontal pathway: Importance in cognitive performances. Brain Connect. 10, 157–169. doi:10.1089/brain.2019.0733
Sutcliffe, G., Harneit, A., Tost, H., and Meyer-Lindenberg, A. (2016). Neuroimaging intermediate phenotypes of executive control dysfunction in schizophrenia. Biol. Psychiatry. Cogn. Neurosci. Neuroimaging 1, 218–229. doi:10.1016/j.bpsc.2016.03.002
Tohka, J., Bellec, P., Grova, C., and Reilhac, A. (2016). Simulation and validation in brain image analysis. Comput. Intell. Neurosci. 2016, 1041058. doi:10.1155/2016/1041058
Vaskinn, A., Ueland, T., Melle, I., Agartz, I., Andreassen, O. A., Sundet, K., et al. (2014). Neurocognitive decrements are present in intellectually superior schizophrenia. Front. Psychiatry 5, 45. doi:10.3389/fpsyt.2014.00045
Vita, A., De Peri, L., Deste, G., and Sacchetti, E. (2012). Progressive loss of cortical gray matter in schizophrenia: A meta-analysis and meta-regression of longitudinal MRI studies. Transl. Psychiatry 2, e190. doi:10.1038/tp.2012.116
Waddington, J. L., Nkire, N., and Russell, V. (2021). Schizophrenia vis-à-vis dimensional-spectrum concepts of psychotic illness: Has an answer been 'hiding in plain sight. Schizophr. Res. 242, 144–146. doi:10.1016/j.schres.2021.11.012
Xuan, R., Gao, J., Lin, Q., Yue, W., Liu, T., Hu, S., et al. (2022). Mitochondrial DNA diversity of Mesocricetus auratus and other cricetinae species among cricetidae family. Biochem. Genet. 2022, 1–14. doi:10.1007/s10528-022-10195-7
Yao, Y., Guo, W., Zhang, S., Yu, H., Yan, H., Zhang, H., et al. (2021). Cell type-specific and cross-population polygenic risk score analyses of MIR137 gene pathway in schizophrenia. iScience 24, 102785. doi:10.1016/j.isci.2021.102785
Yu, P., Chen, X., Zhao, W., Zhang, Z., Zhang, Q., Han, B., et al. (2016). Effect of rs1063843 in the CAMKK2 gene on the dorsolateral prefrontal cortex. Hum. Brain Mapp. 37, 2398–2406. doi:10.1002/hbm.23181
Zakowicz, P., Pawlak, J., Kapelski, P., Wiłkość-Dębczyńska, M., Szałkowska, A., and Twarowska-Hauser, J. (2022). Genetic association study reveals impact of interleukin 10 polymorphisms on cognitive functions in schizophrenia. Behav. Brain Res. 419, 113706. doi:10.1016/j.bbr.2021.113706
Zaytseva, Y., Fajnerová, I., Dvořáček, B., Bourama, E., Stamou, I., Šulcová, K., et al. (2018). Theoretical modeling of cognitive dysfunction in schizophrenia by means of errors and corresponding brain networks. Front. Psychol. 9, 1027. doi:10.3389/fpsyg.2018.01027
Zhu, Y., Womer, F. Y., Leng, H., Chang, M., Yin, Z., Wei, Y., et al. (2019). The relationship between cognitive dysfunction and symptom dimensions across schizophrenia, bipolar disorder, and major depressive disorder. Front. Psychiatry 10, 253. doi:10.3389/fpsyt.2019.00253
Zhuo, C., Ji, F., Lin, X., Tian, H., Wang, L., Liu, S., et al. (2020). Without insight accompanied with deteriorated brain functional alterations in healthy individuals with auditory verbal hallucinations: A pilot study. Brain Imaging Behav. 14, 2553–2558. doi:10.1007/s11682-019-00207-3
Zhuo, C., Xu, Y., Zhang, L., Jing, R., and Zhou, C. (2019). The effect of dopamine antagonist treatment on auditory verbal hallucinations in healthy individuals is clearly influenced by COMT genotype and accompanied by corresponding brain structural and functional alterations: An artificially controlled pilot study. Front. Genet. 10, 92. doi:10.3389/fgene.2019.00092
Keywords: schizophrenia, SNP, cognitive impairment, GMV, FC
Citation: Zhuo C, Tian H, Chen J, Li Q, Yang L, Zhang Q, Chen G, Cheng L, Zhou C and Song X (2022) Associations of cognitive impairment in patients with schizophrenia with genetic features and with schizophrenia-related structural and functional brain changes. Front. Genet. 13:880027. doi: 10.3389/fgene.2022.880027
Received: 20 February 2022; Accepted: 29 June 2022;
Published: 19 August 2022.
Edited by:
Edyta Agnieszka Pawlak, Hirszfeld Institute of Immunology and Experimental Therapy (PAS), PolandReviewed by:
Li Wenqiang, Xinxiang Medical University, ChinaThomas Frodl, University Hospital RWTH Aachen, Germany
Copyright © 2022 Zhuo, Tian, Chen, Li, Yang, Zhang, Chen, Cheng, Zhou and Song. This is an open-access article distributed under the terms of the Creative Commons Attribution License (CC BY). The use, distribution or reproduction in other forums is permitted, provided the original author(s) and the copyright owner(s) are credited and that the original publication in this journal is cited, in accordance with accepted academic practice. No use, distribution or reproduction is permitted which does not comply with these terms.
*Correspondence: Chuanjun Zhuo, chuanjunzhuotjmh@163.com; Xueqin Song, fccsongxq@zzu.edu.cn
†These authors have contributed equally to this work and share first authorship