- 1Department of Urology, Tongji Hospital, Tongji Medical College, Huazhong University of Science and Technology, Wuhan, China
- 2Department of Gastroenterology, Union Hospital, Tongji Medical College, Huazhong University of Science and Technology, Wuhan, China
- 3Department of Geriatrics, Tongji Hospital, Tongji Medical College, Huazhong University of Science and Technology, Wuhan, China
Prostate cancer is one of the most common malignancies in males. Despite the recent development of advanced diagnostic platforms and treatment, patients with metastatic disease still have a poor five-year survival rate. Cancer metastasis is correlated with the characteristics of the tumor microenvironment and is significantly associated with patient prognosis. In this study, we obtained mutated genes with significant differences between primary and metastatic prostate cancer from the COSMIC database. Unsupervised consensus clustering was used based on the 1,051 genes obtained, and two PCa clusters were identified, which exhibited different prognostic outcomes and immune characteristics. Next, we generated a scoring system and evaluated the prognostic value of riskscore and its potential to aid treatment decisions in clinical practice. The riskscore could be applied to predict patients’ response to immunotherapy and sensitivity to Docetaxel. In conclusion, this study performed an integrated analysis of mutated genes between primary and metastatic prostate cancer and provides a novel assessment scheme to precisely select treatment strategies.
Introduction
Prostate cancer (PCa) is the second most common type of cancer diagnosed in males (Sung et al., 2021). While several patients run an indolent course, most patients present with high-risk localized, locally advanced, or metastatic cancer (Teo et al., 2019). Despite localized prostate cancer exhibiting long-term survival, metastatic prostate cancer remains largely incurable even after intensive treatment (Wang et al., 2018). It has been reported that more than ninety percent of cancer-related deaths result from metastasis, and most prostate cancer patients die from metastasis (Rycaj et al., 2017). Therefore, exploring those genes with significant differences between primary and metastatic prostate cancer may help us to predict the prognosis of patients and formulate a more effective treatment regimen.
Metastasis is processed by the mechanisms by which tumor cells invade local tissues, reach the circulation, and colonize distant organs (López-Soto et al., 2017). Recent research has suggested that immune cells can regulate these steps of metastasis by influencing the extracellular matrix (ECM) (Blomberg et al., 2018). ECM remodeling can facilitate metastasis by influencing the architecture of the surrounding tissue to favor tumor cell invasion (Ghajar and Bissell, 2008) or allowing the release and diffusion of the pro-tumoral signaling molecules (Cox and Erler, 2011). Currently, the clinical successes of immunotherapy, such as immune checkpoint blockade, have revolutionized cancer therapeutics, and astonishing clinical responses have been achieved in several types of cancers (van den Bulk et al., 2018). Immunotherapy results in long-term durable remission in some advanced cancer patients (Ganesh et al., 2019). However, a large proportion of patients cannot benefit from checkpoint blockade. Therefore, how to choose suitable treatment is critical in clinical practice, and the development of immunotherapy calls for a better understanding of the influence of immune regulation on metastasis to enhance the treatment efficacy for patients with metastatic disease.
COSMIC, the Catalogue Of Somatic Mutations In Cancer, contains the most detailed and comprehensive materials of somatic mutations in human cancer (Tate et al., 2019). Its latest release includes almost 6 million coding mutations across 1.4 million tumor samples. In this study, we downloaded mutation data and corresponding sample features and performed Chi-square test to identify those genes with significant differences in mutation frequencies between primary and metastatic prostate cancer. Then, we filtered these genes by the univariate Cox regression method, performed unsupervised clustering method and identified two PCa clusters based on these mutated genes. Comprehensive analysis revealed that the two subclasses were significantly different in progression-free survival, characteristics of the immune microenvironment and the expression of immune checkpoint genes. Moreover, we extracted the feature genes to construct a riskscore by principal component analysis and evaluated the prognostic value of the riskscore and its potential to aid treatment decisions in clinical practice.
Materials and Methods
Data Collection and Pre-Processing
Transcriptional data (read counts), clinical characteristics and somatic mutation data were acquired from the TCGA database (Supplementary Table S1). Next, we downloaded four datasets with the same platform (Affymetrix GPL570) from the GEO database: GSE69223 (Meller et al., 2016) (N = 30), GSE32448 (Derosa et al., 2012) (N = 80), GSE55945 (Arredouani et al., 2009) (N = 21), and GSE46602 (Mortensen et al., 2015) (N = 50). Thereafter, we adjusted the background by using the “RMA” algorithm of the “affy” R package (Gautier et al., 2004) and removed the batch effect by the “ComBat” algorithm of the “sva” package (Leek et al., 2012). Therefore, we can merge the four datasets as the validation cohort. Moreover, GSE21034 (Taylor et al., 2010) (N = 370) was utilized to validate the prognostic value.
Identification of Mutated Genes Between Primary and Metastatic Prostate Cancer
COSMIC is currently the broadest database of mutations in human cancer (Forbes et al., 2017). COSMIC mutation data (Genome Screens) and corresponding sample features were downloaded, and we estimated the frequency of each mutation site. Chi-square test was applied to discover the mutated genes with significant differences between primary and metastatic prostate cancer. Next, we executed prognostic analysis for each gene discovered by univariate Cox regression, and the genes related to prognosis with p-value < 0.05 were extracted for further analysis.
Identification of PCa Subclasses
Unsupervised consensus clustering of the obtained genes was executed by using the k-means algorithm in the “ConsensusClusterPlus” package (Wilkerson and Hayes, 2010), which was repeated 1,000 times to ensure the stability of the classification. Survival differences between the two clusters were visualized by Kaplan–Meier curves. To explore the molecular characteristics of the two clusters. The “c2.cp.kegg.v7.4.symbols” gene set was downloaded from the MSigDB database, and we applied the “GSVA” package (Hänzelmann et al., 2013) to perform the GSVA analysis.
Immune Infiltration Levels Between PCa Subclasses
The “ssGSEA” method was performed to estimate the infiltration degrees of 28 immune cells by using the “GSVA” package. Estimate is commonly used to calculate scores reflecting the infiltration levels of immune cells and stromal cells in the tumor microenvironment by the package “estimate” (Yoshihara et al., 2013). We applied the estimate algorithm to calculate the ImmuneScore and StromalScore of each sample. Additionally, the correlation between the expression of immune checkpoint genes and androgen receptor between the two clusters was estimated.
Generation of Riskscore
The Pearson correlation coefficients of mutated genes with the identified PCa subclasses were estimated, and the signature genes A and B were obtained based on the correlation coefficients. Then, we applied the Boruta algorithm to the positively and negatively correlated genes to select feather genes. Finally, principal component analysis (PCA) was performed to estimate the first principal components of signature genes A and B. We defined the riskscore of each sample as follows:
Correlation Between Clinical Parameters, Immune Infiltration and Riskscore
The difference in the riskscore in patients stratified by clinical parameters was estimated to expound the effect of the riskscore on cancer progression. Moreover, immune cell infiltration, ImmuneScore, StromalScore and the expression of immune checkpoint genes were also assessed between the high- and low-risk groups.
Tumor Mutation Burden Analysis
Tumor mutation burden (TMB) has been demonstrated as a predictive biomarker to identify whether patients with cancer can respond to immune checkpoint inhibitors well (Merino et al., 2020). Here, we explored the correlation between TMB and riskscore. Furthermore, we divided patients into four subgroups based on the median value of riskscore and TMB. Survival differences of the four subgroups were visualized by Kaplan–Meier curves.
Benefits of the Riskscore to Aid Treatment Decisions
Since the comparison of the expression of different immune checkpoint genes between the high- and low-risk groups was performed, here we used an immunotherapeutic cohort (IMvigor210 cohort) as a validation cohort (Mariathasan et al., 2018). We first evaluated the influence of the riskscore on the prognosis of patients treated with immunotherapy. Then, the riskscore of patients with different clinical statuses after treatment were compared. Finally, transcriptional data of tumor cell lines and IC50 values of antitumor drugs from the GDSC database were used to perform the drug sensitivity analysis by using the “pRRophetic” package (Geeleher et al., 2014).
Statistical Analysis
All analyses were performed in RStudio 4.0.4. Correlation analysis was computed by the Spearman method. Student’s t test and the Wilcoxon test were applied for two-group comparisons. Correspondingly, the Kruskal–Wallis test and one-way ANOVA were used for multiple groups. Statistical significance was defined as p-value < 0.05.
Results
Genetic Alterations in Prostate Cancer
The roadmap of this study is illustrated in Figure 1. The top 20 mutated genes for prostate cancer are shown in Figure 2A, and the mutation frequencies are displayed next to the gene name. Furthermore, Figures 2B,C shows the distribution of different types of mutations for prostate cancer. Missense substitution (88.07%), synonymous substitution (49.48%) and nonsense substitution (37.91%) were the main types of mutations, and the substitution mutations mainly included G > A (72.93%), C > T (72.42%), A > G (64.57%), and G > T (61.27%). Moreover, the Manhattan plot depicted mutation sites that had significant differences between primary and metastatic prostate cancer (Figure 2D).
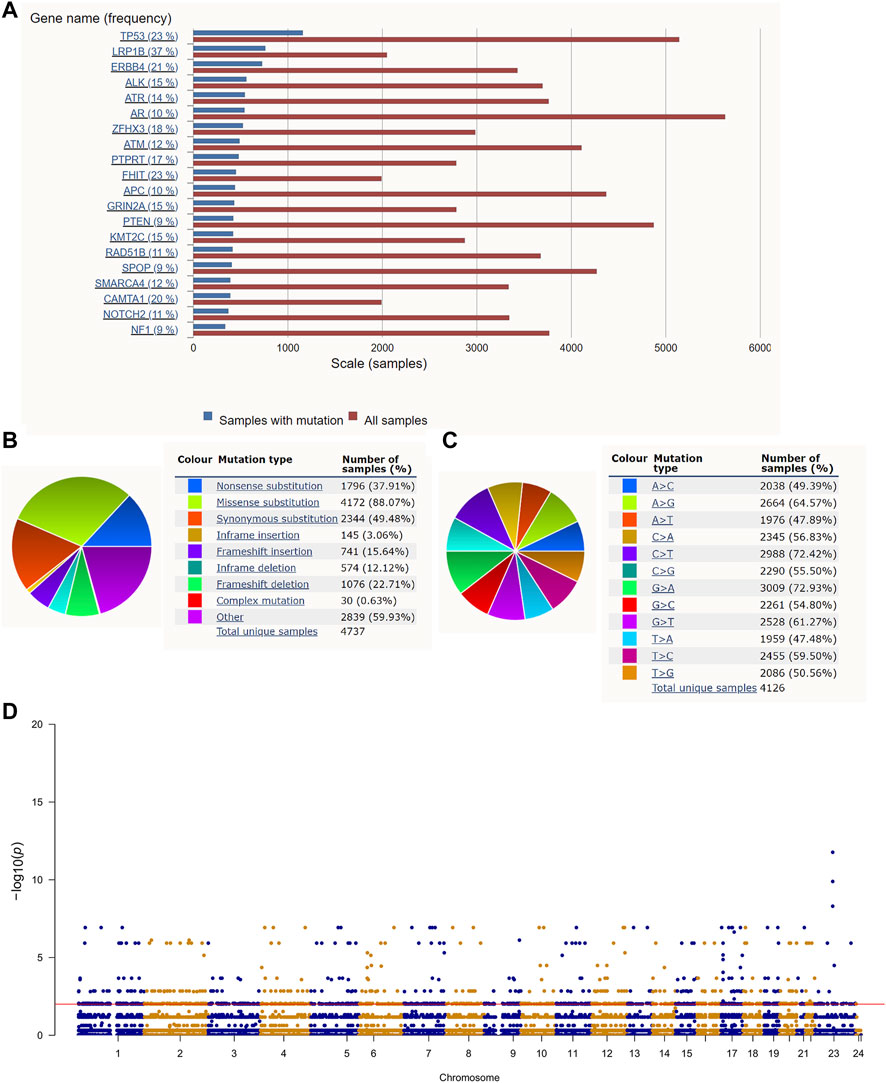
FIGURE 2. Gene mutation in prostate cancer: (A) The top 20 mutated genes in prostate cancer. (B,C) The distribution of different types of mutations in prostate cancer. (D) The Manhattan plot of mutation sites that have significantly different mutation frequencies between primary and metastatic prostate cancer.
Identification of PCa Subclasses
We obtained 3,716 mutated genes with significant differences between primary and metastatic prostate cancer by the Chi-square test (Supplementary Table S2). Thereafter, we explored the prognostic value of these genes for progression-free survival (PFS) by the univariate Cox method, and 1,051 genes were extracted (Supplementary Table S3) for further analysis.
After comprehensive consideration of CDF curves and delta area, we chose k = 2 as the optimal cluster number for the clustering (Figure 3). Finally, 308 patients were assigned to Cluster 1, and 187 patients were assigned to Cluster 2. We also applied t-SNE dimension reduction, and the results suggested that the discrimination among subgroups was decent (Supplementary Figure S1). Survival curves suggested that Cluster 2 had a significant survival advantage compared with Cluster 1 (Figure 3C). Moreover, GSVA analysis and limma analysis (log FC > 0.2, adjusted p-value < 0.05) were performed. Significant differences in pathways related to cancer progression, such as the ERBB signaling pathway and VEGF signaling pathway, and pathways associated with the immune response, such as the B cell receptor signaling pathway and T cell receptor signaling pathway, were observed between the two clusters (Figure 3D).
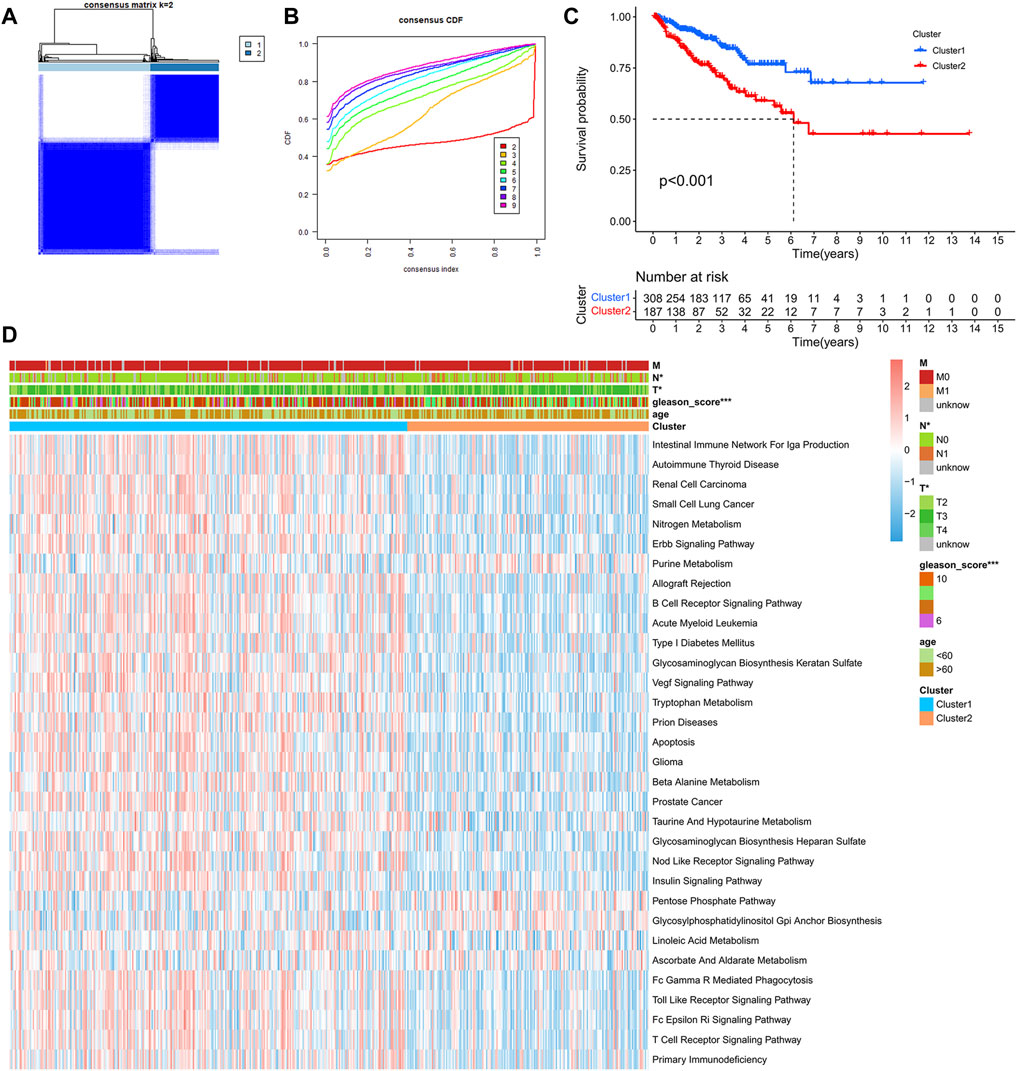
FIGURE 3. Identification of PCa subclasses by unsupervised consensus clustering: (A) Matrix heatmap of the K-means clustering using 1,051 mutated genes between primary and metastatic prostate cancer. (B) CDF curve of the clustering result. (C) Kaplan–Meier survival curve of PFS between different clusters. (D) Heatmap of GSVA enrichment based on KEGG pathways between different clusters. (*p < 0.05, **p < 0.01, ***p < 0.001).
In order to delve into the immune-related characteristics of the two clusters, the infiltration levels of immune cells were compared between the two clusters. A significant difference was observed in the infiltration degree of all immune cells, and all immune cells infiltration were lower in Cluster 2 (Figure 4A). Moreover, the results indicated that both the StromalScore and ImmuneScore of Cluster 2 were significantly lower compared with Cluster 1 (Figure 4B). What’s more, the expression of immune checkpoint genes, including CTLA4, PD-1, PD-L1 and PD-L2, appeared to be decreased in Cluster 2 (Figures 4C–F). However, the expression of AR was higher in Cluster 2 than in Cluster 1 (Figure 4G), which is consistent with the poor survival of Cluster 2.
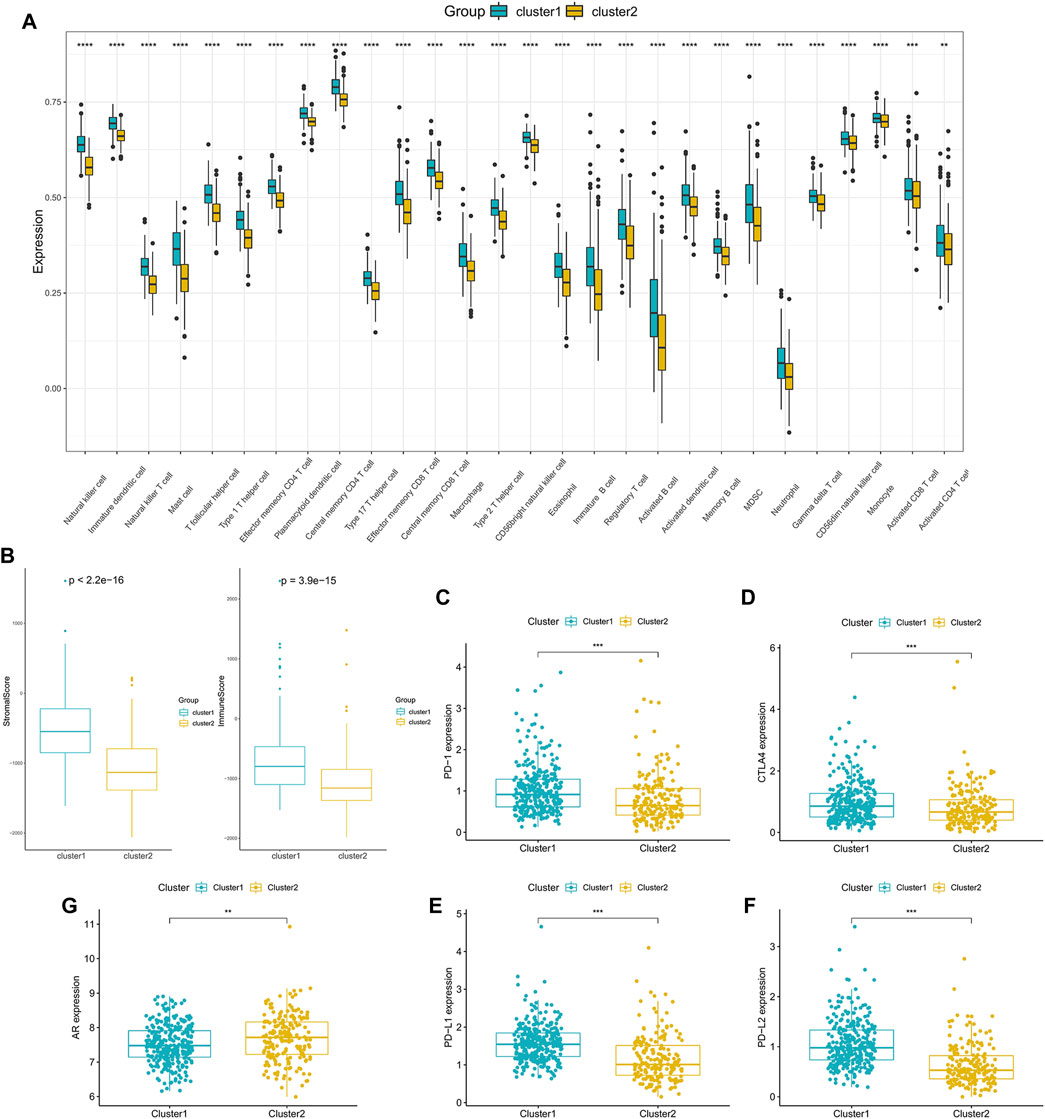
FIGURE 4. Tumor microenvironment characteristics of different PCa subclasses: (A) The proportions of TME cells in the two clusters. (B) ImmuneScore and StromalScore of the two subgroups. (C–F) The expression of the immune checkpoint genes PD-1 (C), CTLA4 (D), PD-L1 (E) and PD-L2 (F) between the two clusters. (G) The expression of AR in the two clusters. (*p < 0.05, **p < 0.01, ***p < 0.001, ****p < 0.0001).
Construction of the Riskscore For Each Sample and the Prognostic Value
Previous results indicated that the subclass was closely associated with the prognosis and immune infiltration levels of patients. However, this population-based classification cannot be directly used in clinical practice. Therefore, we constructed a scoring system to estimate the riskscore to predict the outcome of the patients and aid in making treatment decisions. After performing the Boruta algorithm, 230 genes that were positively and negatively correlated to the subclass were defined as signature genes A and B (Supplementary Table S4). The riskscore was acquired by conducting PCA on each signature gene (Supplementary Table S5). Patients were classified into high- and low-risk groups according to the cut-off point gained by the “survminer” package (Supplementary Figure S2). The results of survival analysis showed that patients in the high-risk group had lower PFS than those in the low-risk group (Figure 5A). As shown in Figure 5B, the high-risk group possessed a higher proportion of death. In the validation cohort GSE21034, patients with higher riskscore also showed a significantly shorter median PFS (Figure 5C). Moreover, it was observed that the riskscore was elevated in the high-risk clinical group with the progression of tumor (Figure 5D), and patients who achieved complete response after treatment had a significantly lower riskscore than other outcomes (Supplementary Figure S3).
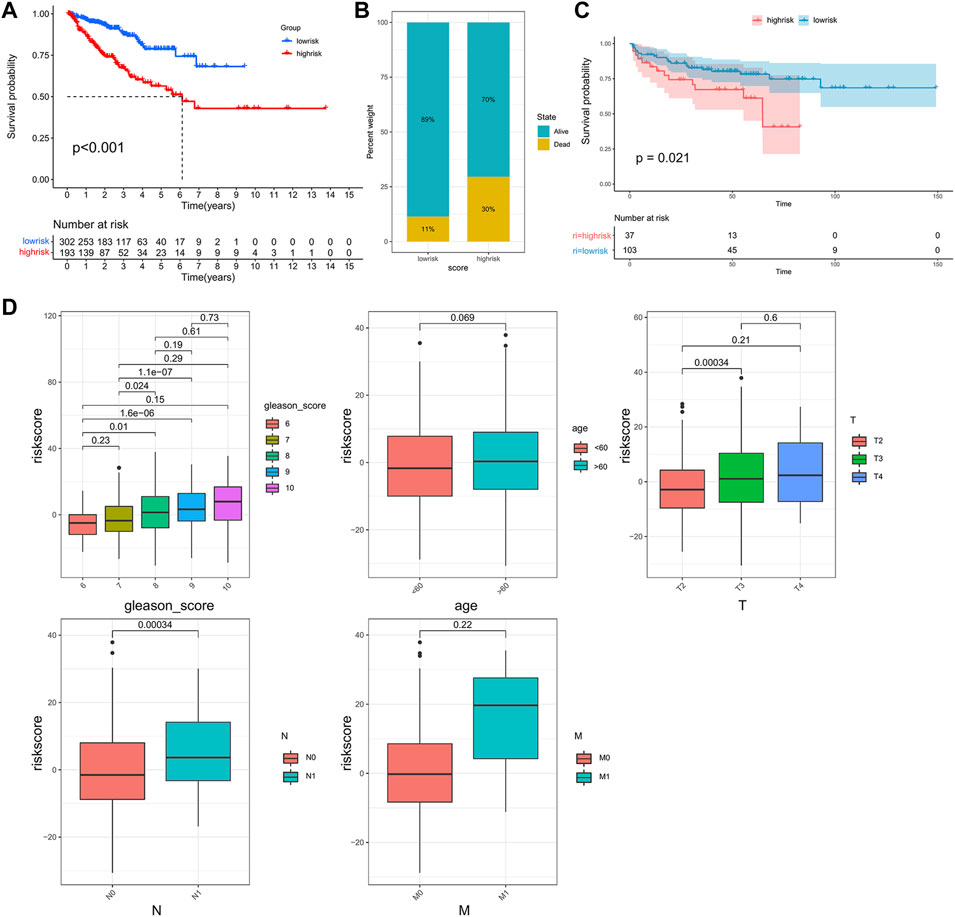
FIGURE 5. Construction of the riskscore and prognosis analysis. (A) Kaplan–Meier survival curve between high- and low-risk subgroups in the TCGA cohort. (B) The proportion of the survival rate between the high- and low-risk subgroups. (C) Kaplan–Meier survival curve between the high- and low-risk subgroups in the validation cohort, GSE21034. (D) The differences in riskscore between patients with different clinicopathological parameters (Gleason score, age, T stage, N stage, M stage).
Relationship Between Riskscore and TMB
TMB is emerging as a potential biomarker to predict the patients response to immune checkpoint inhibitors (Chan et al., 2019). Here, we evaluated the association between the riskscore and TMB. As shown in Figure 6A, patients in the high-risk group had a higher TMB than those in the low-risk group, and patients with a higher TMB had lower PFS (Figure 6B). Moreover, the correlation analysis indicated that the riskscore was positively associated with TMB (Figure 6C). Next, we combined the riskscore and TMB to divide patients into four subgroups. The patients with a high riskscore and high TMB had the shortest median PFS, and patients with a low riskscore and low TMB performed the best prognosis (Figure 6D). Finally, the mutation status of genes with high mutation frequencies in the high- and low-risk groups was visualized (Supplementary Figure S4).
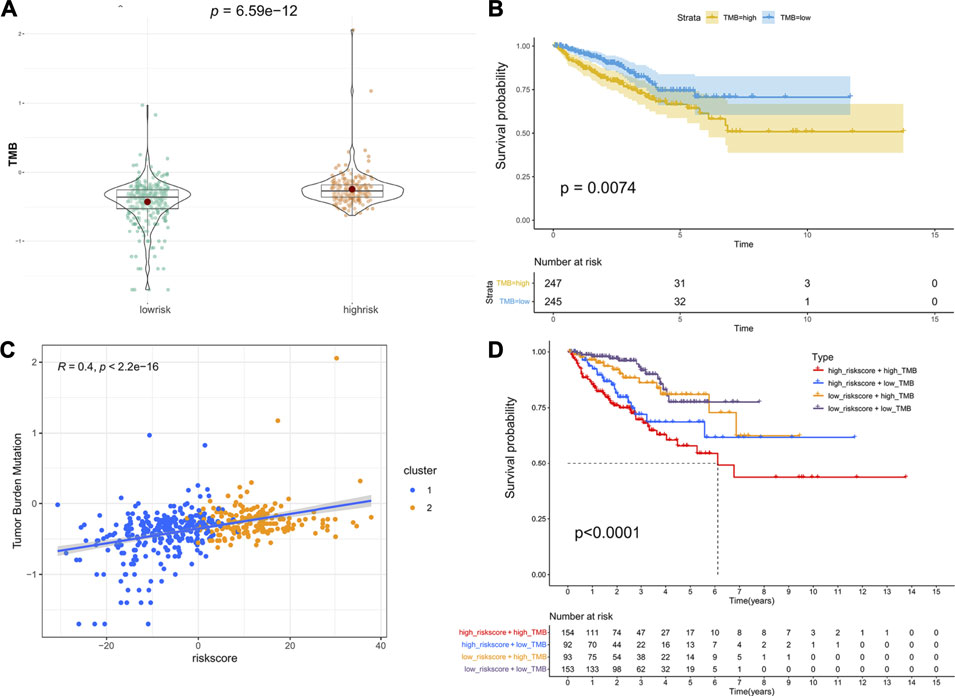
FIGURE 6. Association between riskscore and TMB: (A) The difference in TMB between the high- and low-risk groups. (B) Kaplan–Meier survival curves of PFS between the high- and low-TMB groups. (C) The correlation of riskscore and TMB. (D) Kaplan–Meier survival curves of PFS among the four subgroups stratified by riskscore and TMB.
Correlation Between Immunotherapy Reactivity, Drug Sensitivity and Riskscore
Anticancer immunotherapies involving immune checkpoint inhibitors have emerged as new therapeutic regimens (O’Donnell et al., 2019). The tumor microenvironment (TME) was proven to be tightly linked to tumor progression and metastasis (Brassart-Pasco et al., 2020) and can blunt the therapeutic response, thus affecting the clinical outcome (Wu and Dai, 2017). To further explore the correlations between patients’ response to immunotherapy and riskscore, we first compared the immune cell infiltration levels between high- and low-risk groups, and the results indicated that compared with the low-risk patients, the infiltration levels of 28 immune cells in the high-risk patients were significantly downregulated (Figure 7A).
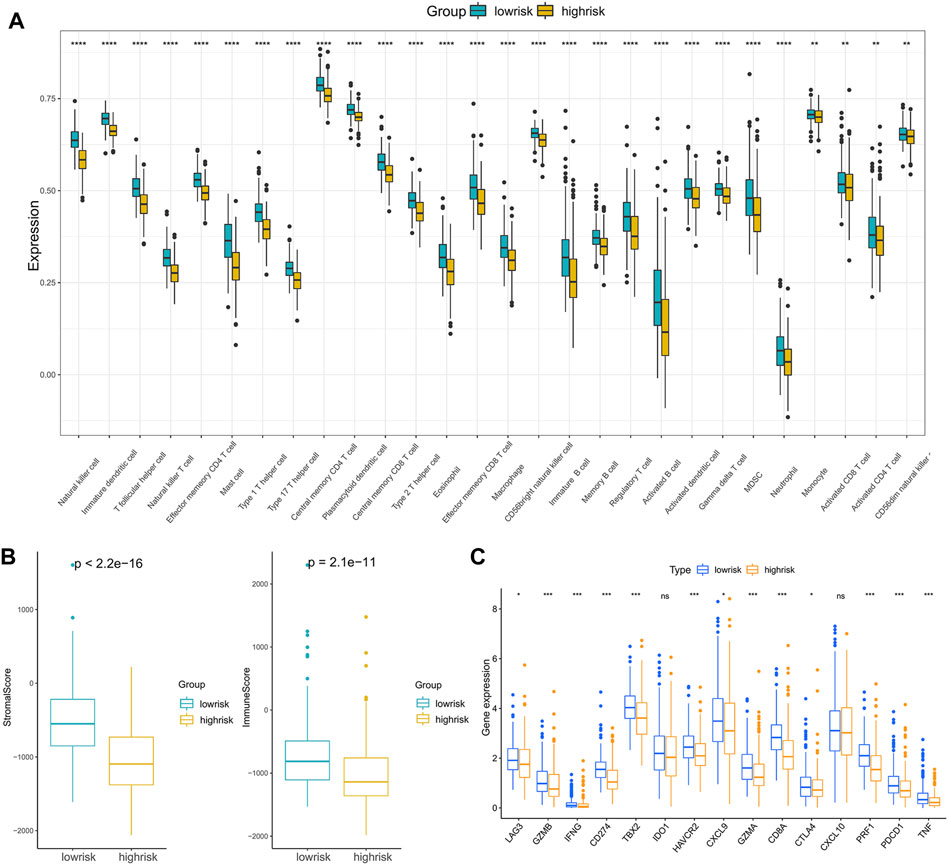
FIGURE 7. Analysis of the correlation between the riskscore and immune cell infiltration: (A) The immune cell infiltration levels between the high- and low-risk subgroups. (B) ImmuneScore and StromalScore between the high- and low-risk subgroups. (C) The expression of immune checkpoint genes in the high- and low-risk subgroups. (*p < 0.05, **p < 0.01, ***p < 0.001, ****p < 0.0001).
Moreover, infiltration estimation for TCGA was downloaded from TIMER 2.0 (Li et al., 2020), which includes several methods, such as XCELL, TIMER, QUANTISEQ, MCPCOUNTER, EPIC, and CIBERSORT. We correlated the immune cell infiltration levels and the riskscore, and the results also suggested that the riskscore was negatively related to most of the immune cells (Supplementary Figure S5). Additionally, the StromalScore and ImmuneScore of the high-risk patients were significantly lower than low-risk patients as well (Figure 7B). Furthermore, the associations between immune checkpoint inhibitor genes and the riskscore were evaluated. The expression of ICI genes, such as CTLA4, PD-1, PD-L1 and LAG3, were downregulated in the high-risk groups compared with the low-risk groups (Figure 7C). Finally, we validated the performance of the riskscore in the IMvigor210 cohort. As shown in Figure 8A, the low-risk patients still showed a significant survival advantage compared with high-risk patients. We evaluated the differences in riskscore among patients with different responses to immunotherapy. Patients with complete response (CR) had the lowest riskscore while patients performed progressive disease (PD) had the highest riskscore (Figure 8B). These results suggested that patients in the high-risk group may not have a satisfying response to immunotherapy.
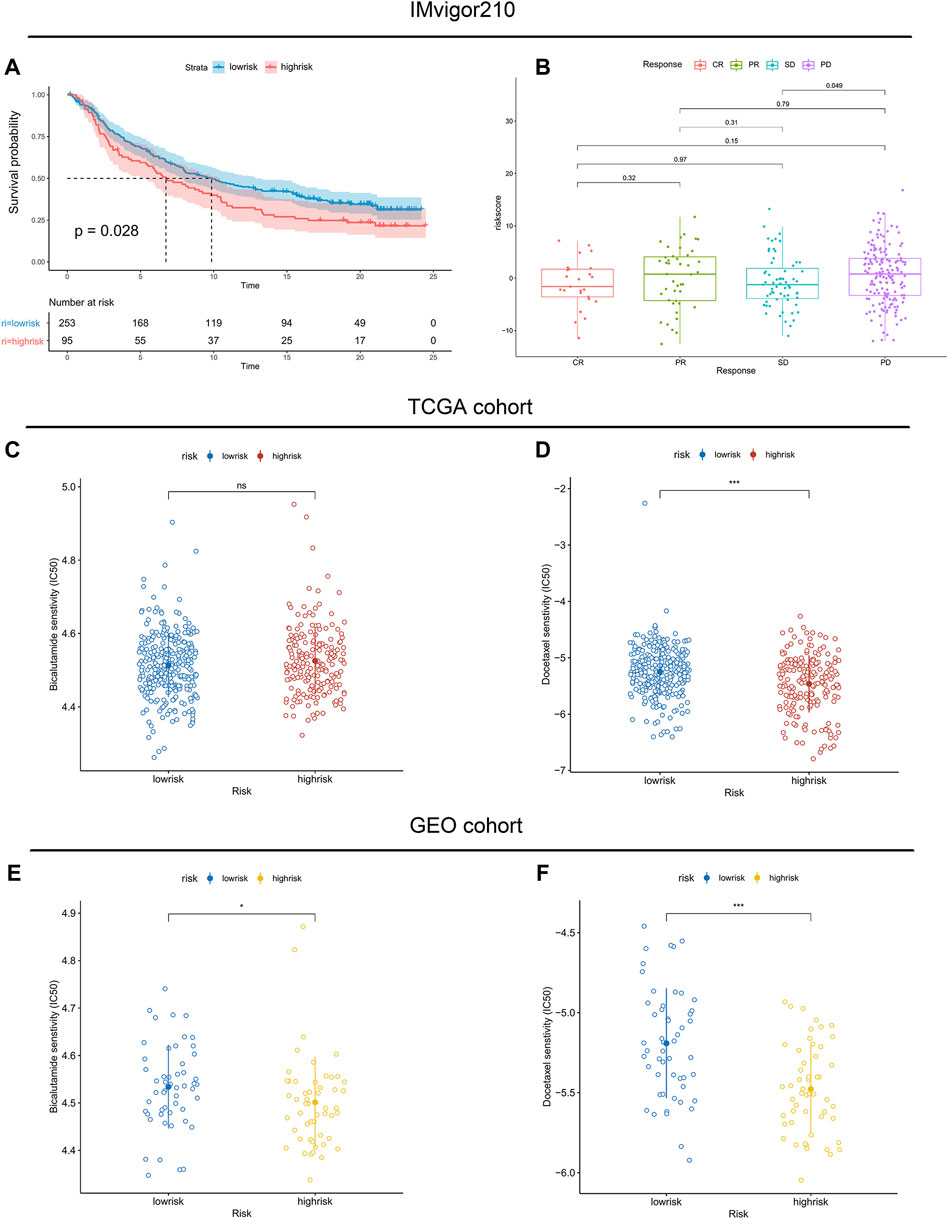
FIGURE 8. Association between immunotherapy reactivity, drug sensitivity and riskscore. (A) Kaplan–Meier survival curve between high- and low-risk subgroups in the IMvigor210 cohort. (B) The difference in riskscore among patients with different responses to immunotherapy. (C,E) The predicted IC50 values of Bicalutamide in the TCGA cohort and validated GEO cohort. (D,F) The predicted IC50 values of Docetaxel in the TCGA cohort and validated GEO cohort.
Considering that androgen deprivation therapy and chemotherapy still play vital roles in the treatment of prostate cancer. We used the GDSC database to explore the association between the riskscore and drug sensitivity. The results revealed little difference in the predicted IC50 of Bicalutamide between the high- and low-risk groups (Figures 8C,E). However, both in the TCGA and GEO cohorts, significant differences were observed in the predicted IC50 of Docetaxel between the high- and low-risk groups (Figures 8D,F). The IC50 of Docetaxel was significantly lower in the high-risk group, suggesting that these patients are sensitive to Docetaxel.
Discussion
Prostate cancer affects millions of men all over the world, and accounts for 7% of newly diagnosed cancers in men worldwide (Rebello et al., 2021). While the prognosis of localized PCa has a good 5-years survival rate, the 5-years survival rate of metastatic PCa decreases significantly to only 30% (Siegel et al., 2020). It is well-known that the growth and progression of prostate cancer are significantly influenced by androgen, and androgen deprivation is an effective treatment strategy which is widely used in clinical practice (Marques et al., 2005). However, among patients with metastatic disease, a substantial proportion will develop metastatic castration-resistant prostate cancer (mCRPC), which is not sensitive to androgen deprivation therapy. Therefore, the long-term prognosis for patients with mCRPC is extremely poor (Henríquez et al., 2021). On that account, it is critical to unearth the mechanism of metastasis of prostate cancer, which may assist us in predicting the prognosis of patients and forming a desirable therapeutic regimen. Although multitudinous studies have explored the correlation of some specific genes in tumor metastasis, few studies have focused on the overall mutated genes between primary and metastatic prostate cancer. Therefore, we used the COSMIC database, which contains the most detailed resource of somatic mutations in human cancer, and executed the Chi-square test to obtain mutated genes with significant differences between primary and metastatic prostate cancer.
In this study, unsupervised clustering method was conducted, and two subclasses of PCa were obtained. Then, we comprehensively assessed the two clusters of prostate cancer and explored their biological characteristics. We observed that significant differences existed between the two clusters in some carcinogenic activation signaling pathways, such as the ErbB signaling pathway (Wang, 2017) and Vegf signaling pathway (Apte et al., 2019), and pathways associated with the immune response, such as the B cell receptor signaling pathway and T cell receptor signaling pathway. Moreover, the results indicated that all the immune cell infiltration levels and the expression of immune checkpoint genes were lower in Cluster 2, which was associated with poorer survival. We supposed that the poor prognosis of patients in Cluster 2 was due to tumor immune escape.
Next, we evaluated the riskscore of each patient by using the “Boruta” algorithm and PCA analysis. Its prognostic value was demonstrated both in TCGA and GEO cohorts. Since cancer develops as a result of somatic mutation and clonal selection (Martincorena et al., 2017), herein, we correlated riskscore and TMB and found a significant positive correlation between riskscore and TMB. Moreover, we assessed the mutation status of genes with high mutation frequencies in the high- and low-risk groups. It was observed that the high-risk groups contained more mutated samples and more mutation types.
Androgen deprivation therapy is a standard treatment used in all stages of recurrent prostate cancer. However, patients will develop CRPC eventually (Gamat and McNeel, 2017). In the past, the consensus was that immunotherapy might be ineffective in prostate cancer due to the immunosuppressive microenvironment (Chakravarty et al., 2020). However, with the recent development of advanced molecular diagnostic platforms, immunotherapy has revolutionized the treatment of prostate cancer and is re-emerging as a practicable option for patients, especially for CRPC (Cha et al., 2020). Nevertheless, a key challenge for immunotherapies is that these treatments have serious adverse effects, including autoimmunity and nonspecific inflammation (Riley et al., 2019). Additionally, many patients appear to have innate or acquired resistance to immunotherapies (O’Donnell et al., 2019). Therefore, it is critical to find reliable validated biomarkers to predict the immunotherapy responsiveness of patients. In fact, it is obvious that using a single biomarker to predict benefit from immunotherapy strategies is unstable. Consequently, we extracted 230 feature genes to construct the riskscore. According to the results, all immune cell infiltration levels were higher in the low-risk groups, and immune checkpoint genes used in immune checkpoint blockade therapy, such as PD-1, CTLA4 and PD-L1, were also more highly expressed in the low-risk groups. Therefore, we suppose that patients identified as having a low riskscore may benefit from the therapeutic strategy combining immune checkpoint blockade therapy, while patients are diagnosed with a high riskscore. Since open-access data of prostate cancer cohorts accepting immunotherapy are rare, we used patients in the IMvigor210 cohort for preliminary validation. We observed that patients who reacted as complete response had the lowest riskscore while patients performed progressive disease had the highest riskscore, which is consistent with the trends of expression of immune checkpoints.
Considering it is unrealistic that utilizing immunotherapy alone can dramatically change the outcome of prostate cancer right now, the combination of conventional cytotoxic agents, androgen deprivation therapy and personalized immunotherapy is more appropriate for patients. We used the GDSC database, which is the largest public resource for information on drug sensitivity in cancer cells (Yang et al., 2013), to predict the IC50 values of drugs for treating prostate cancer. We observed that the high-risk group was more sensitive to Docetaxel than the low-risk group. In fact, Docetaxel was the first systemic therapy to demonstrate survival benefit in mCRPC and became the standard of care for mCRPC in 2004 (Teo et al., 2019). It is still recommended as a first-line treatment for mCRPC in the latest EAU guidelines (Cornford et al., 2021). Therefore, it seems reasonable that patients identified with high riskscores had a higher sensitivity to Docetaxel. Bicalutamide is a competitive androgen receptor antagonist that leads to prostate cell apoptosis and the inhibition of prostate cancer growth (Wellington and Keam, 2006). We also evaluated the IC50 values of Bicalutamide in the high- and low-risk groups. However, there were few differences in the predicted IC50 of Bicalutamide between the high- and low-risk groups. Regrettably, limited by the data currently available in the GDSC database, we could not evaluate the IC50 values of Abiraterone and Enzalutamide in this study. As second-generation androgen receptor inhibitors, they have already been recommended by the latest EAU guidelines (Cornford et al., 2021). Numerous studies have demonstrated that the second-generation androgen receptor inhibitor is associated with improved outcomes compared with bicalutamide in CRPC (Penson et al., 2016; Naiki et al., 2021; Ueda et al., 2021; Vaishampayan et al., 2021). Therefore, the drug sensitivity of Abiraterone and Enzalutamide between high- and low-risk groups needs to be further explored. Moreover, due to the lack of available immunotherapy cohorts of prostate cancer, preliminary validation was performed in the IMvigor210 cohort for bladder cancer. The ability of the riskscore to predict the immunotherapy response of patients still needs further validation in immunotherapy cohorts of prostate cancer.
Conclusion
In summary, we selected mutated genes with significant differences between primary and metastatic prostate cancer from the COSMIC database and identified two PCa clusters that exhibited different prognostic outcomes and immune characteristics. For a better application in clinical practice, we constructed a scoring system and evaluated the prognostic value of the riskscore and its potential to aid treatment decisions. The riskscore could be applied to predict patients’ response to immunotherapy and sensitivity to Docetaxel. The results suggested that immunotherapy may benefit patients in the low-risk group, while Docetaxel is more effective for patients identified in the high-risk group.
Website
TCGA database: https://portal.gdc.cancer.gov/; GEO database: https://www.ncbi.nlm.nih.gov/geo/; COSMIC database: https://cancer.sanger.ac.uk/cosmic; MSigDB database: https://www.gsea-msigdb.org/gsea/msigdb/; GDSC database: https://www.cancerrxgene.org/.
Data Availability Statement
The original contributions presented in the study are included in the article/Supplementary Material, further inquiries can be directed to the corresponding author.
Author Contributions
QL proposed the conception and designed the roadmap of this research. JM provided administrative support. XX, BC, GS, and KZ performed the statistical analyses and interpreted the data. All authors read and approved the final manuscript.
Funding
This work was supported by grants from the National Natural Science Foundation of China (grant number 81902619) and the National Natural Science Foundation of Hubei Province (2020CFB591).
Conflict of Interest
The authors declare that the research was conducted in the absence of any commercial or financial relationships that could be construed as a potential conflict of interest.
Publisher’s Note
All claims expressed in this article are solely those of the authors and do not necessarily represent those of their affiliated organizations, or those of the publisher, the editors and the reviewers. Any product that may be evaluated in this article, or claim that may be made by its manufacturer, is not guaranteed or endorsed by the publisher.
Acknowledgments
We thank TCGA (https://portal.gdc.cancer.gov/) and GEO (https://www.ncbi.nlm.nih.gov/geo/) databases.
Supplementary Material
The Supplementary Material for this article can be found online at: https://www.frontiersin.org/articles/10.3389/fgene.2022.877086/full#supplementary-material
Abbreviations
COSMIC, The Catalogue of Somatic Mutations in Cancer; ECM, Extracellular matrix; FC, Fold change; GDSC, Genomics of Drug Sensitivity in Cancer; GEO, Gene Expression Omnibus; GSVA, Gene set variation analysis; PCa, Prostate cancer; PCA, principal components analysis; ROC, Receiver operating characteristic; ssGSEA, Single sample gene set enrichment analysis; TCGA, The Cancer Genome Atlas; TMB, Tumor mutation burden; TME, Tumor microenvironment.
References
Apte, R. S., Chen, D. S., and Ferrara, N. (2019). VEGF in Signaling and Disease: Beyond Discovery and Development. Cell 176, 1248–1264. doi:10.1016/j.cell.2019.01.021
Arredouani, M. S., Lu, B., Bhasin, M., Eljanne, M., Yue, W., Mosquera, J.-M., et al. (2009). Identification of the Transcription Factor Single-Minded Homologue 2 as a Potential Biomarker and Immunotherapy Target in Prostate Cancer. Clin. Cancer Res. 15, 5794–5802. doi:10.1158/1078-0432.CCR-09-0911
Blomberg, O. S., Spagnuolo, L., and de Visser, K. E. (2018). Immune Regulation of Metastasis: Mechanistic Insights and Therapeutic Opportunities. Dis. Model. Mech. 11, dmm036236. doi:10.1242/dmm.036236
Brassart-Pasco, S., Brézillon, S., Brassart, B., Ramont, L., Oudart, J.-B., and Monboisse, J. C. (2020). Tumor Microenvironment: Extracellular Matrix Alterations Influence Tumor Progression. Front. Oncol. 10, 397. doi:10.3389/fonc.2020.00397
Cha, H.-R., Lee, J. H., and Ponnazhagan, S. (2020). Revisiting Immunotherapy: A Focus on Prostate Cancer. Cancer Res. 80, 1615–1623. doi:10.1158/0008-5472.CAN-19-2948
Chakravarty, D., Huang, L., Kahn, M., and Tewari, A. K. (2020). Immunotherapy for Metastatic Prostate Cancer: Current and Emerging Treatment Options. Urol. Clin. North Am. 47, 487–510. doi:10.1016/j.ucl.2020.07.010
Chan, T. A., Yarchoan, M., Jaffee, E., Swanton, C., Quezada, S. A., Stenzinger, A., et al. (2019). Development of Tumor Mutation burden as an Immunotherapy Biomarker: Utility for the Oncology Clinic. Ann. Oncol. 30, 44–56. doi:10.1093/annonc/mdy495
Cornford, P., van den Bergh, R. C. N., Briers, E., Van den Broeck, T., Cumberbatch, M. G., De Santis, M., et al. (2021). EAU-EANM-ESTRO-ESUR-SIOG Guidelines on Prostate Cancer. Part II-2020 Update: Treatment of Relapsing and Metastatic Prostate Cancer. Eur. Urol. 79, 263–282. doi:10.1016/j.eururo.2020.09.046
Cox, T. R., and Erler, J. T. (2011). Remodeling and Homeostasis of the Extracellular Matrix: Implications for Fibrotic Diseases and Cancer. Dis. Model. Mech. 4, 165–178. doi:10.1242/dmm.004077
Derosa, C. A., Furusato, B., Shaheduzzaman, S., Srikantan, V., Wang, Z., Chen, Y., et al. (2012). Elevated Osteonectin/SPARC Expression in Primary Prostate Cancer Predicts Metastatic Progression. Prostate Cancer Prostatic Dis. 15, 150–156. doi:10.1038/pcan.2011.61
Forbes, S. A., Beare, D., Boutselakis, H., Bamford, S., Bindal, N., Tate, J., et al. (2017). COSMIC: Somatic Cancer Genetics at High-Resolution. Nucleic Acids Res. 45, D777–D783. doi:10.1093/nar/gkw1121
Gamat, M., and McNeel, D. G. (2017). Androgen Deprivation and Immunotherapy for the Treatment of Prostate Cancer. Endocr. Relat. Cancer 24, T297–T310. doi:10.1530/ERC-17-0145
Ganesh, K., Stadler, Z. K., Cercek, A., Mendelsohn, R. B., Shia, J., Segal, N. H., et al. (2019). Immunotherapy in Colorectal Cancer: Rationale, Challenges and Potential. Nat. Rev. Gastroenterol. Hepatol. 16, 361–375. doi:10.1038/s41575-019-0126-x
Gautier, L., Cope, L., Bolstad, B. M., and Irizarry, R. A. (2004). affy--analysis of Affymetrix GeneChip Data at the Probe Level. Bioinformatics 20, 307–315. doi:10.1093/bioinformatics/btg405
Geeleher, P., Cox, N., and Huang, R. S. (2014). pRRophetic: an R Package for Prediction of Clinical Chemotherapeutic Response from Tumor Gene Expression Levels. PLoS One 9, e107468. doi:10.1371/journal.pone.0107468
Ghajar, C. M., and Bissell, M. J. (2008). Extracellular Matrix Control of Mammary Gland Morphogenesis and Tumorigenesis: Insights from Imaging. Histochem. Cel Biol. 130, 1105–1118. doi:10.1007/s00418-008-0537-1
Hänzelmann, S., Castelo, R., and Guinney, J. (2013). GSVA: Gene Set Variation Analysis for Microarray and RNA-Seq Data. BMC Bioinformatics 14, 7. doi:10.1186/1471-2105-14-7
Henríquez, I., Roach, M., Morgan, T. M., Bossi, A., Gómez, J. A., Abuchaibe, O., et al. (2021). Current and Emerging Therapies for Metastatic Castration-Resistant Prostate Cancer (mCRPC). Biomedicines 9, 1247. doi:10.3390/biomedicines9091247
Leek, J. T., Johnson, W. E., Parker, H. S., Jaffe, A. E., and Storey, J. D. (2012). The Sva Package for Removing Batch Effects and Other Unwanted Variation in High-Throughput Experiments. Bioinformatics 28, 882–883. doi:10.1093/bioinformatics/bts034
Li, T., Fu, J., Zeng, Z., Cohen, D., Li, J., Chen, Q., et al. (2020). TIMER2.0 for Analysis of Tumor-Infiltrating Immune Cells. Nucleic Acids Res. 48, W509–W514. doi:10.1093/nar/gkaa407
López-Soto, A., Gonzalez, S., Smyth, M. J., and Galluzzi, L. (2017). Control of Metastasis by NK Cells. Cancer Cell 32, 135–154. doi:10.1016/j.ccell.2017.06.009
Mariathasan, S., Turley, S. J., Nickles, D., Castiglioni, A., Yuen, K., Wang, Y., et al. (2018). TGFβ Attenuates Tumour Response to PD-L1 Blockade by Contributing to Exclusion of T Cells. Nature 554, 544–548. doi:10.1038/nature25501
Marques, R. B., Erkens-Schulze, S., de Ridder, C. M., Hermans, K. G., Waltering, K., Visakorpi, T., et al. (2005). Androgen Receptor Modifications in Prostate Cancer Cells upon Long-Termandrogen Ablation and Antiandrogen Treatment. Int. J. Cancer 117, 221–229. doi:10.1002/ijc.21201
Martincorena, I., Raine, K. M., Gerstung, M., Dawson, K. J., Haase, K., Van Loo, P., et al. (2017). Universal Patterns of Selection in Cancer and Somatic Tissues. Cell 171, 1029–1041. doi:10.1016/j.cell.2017.09.042
Meller, S., Meyer, H.-A., Bethan, B., Dietrich, D., Maldonado, S. G., Lein, M., et al. (2016). Integration of Tissue Metabolomics, Transcriptomics and Immunohistochemistry Reveals ERG- and gleason Score-specific Metabolomic Alterations in Prostate Cancer. Oncotarget 7, 1421–1438. doi:10.18632/oncotarget.6370
Merino, D. M., McShane, L. M., Fabrizio, D., Funari, V., Chen, S.-J., White, J. R., et al. (2020). Establishing Guidelines to Harmonize Tumor Mutational burden (TMB): In Silico Assessment of Variation in TMB Quantification across Diagnostic Platforms: Phase I of the Friends of Cancer Research TMB Harmonization Project. J. Immunother. Cancer 8, e000147. doi:10.1136/jitc-2019-000147
Mortensen, M. M., Høyer, S., Lynnerup, A.-S., Ørntoft, T. F., Sørensen, K. D., Borre, M., et al. (2015). Expression Profiling of Prostate Cancer Tissue Delineates Genes Associated with Recurrence after Prostatectomy. Sci. Rep. 5, 16018. doi:10.1038/srep16018
Naiki, T., Takahara, K., Ito, T., Nakane, K., Sugiyama, Y., Koie, T., et al. (2021). Comparison of Clinical Outcomes between Androgen Deprivation Therapy with Up-Front Abiraterone and Bicalutamide for Japanese Patients with LATITUDE High-Risk Prostate Cancer in a Real-World Retrospective Analysis. Int. J. Clin. Oncol. 27, 592–601. doi:10.1007/s10147-021-02071-y
O’Donnell, J. S., Teng, M. W. L., and Smyth, M. J. (2019). Cancer Immunoediting and Resistance to T Cell-Based Immunotherapy. Nat. Rev. Clin. Oncol. 16, 151–167. doi:10.1038/s41571-018-0142-8
Penson, D. F., Armstrong, A. J., Concepcion, R., Agarwal, N., Olsson, C., Karsh, L., et al. (2016). Enzalutamide versus Bicalutamide in Castration-Resistant Prostate Cancer: The STRIVE Trial. J. Clin. Oncol. 34, 2098–2106. doi:10.1200/JCO.2015.64.9285
Rebello, R. J., Oing, C., Knudsen, K. E., Loeb, S., Johnson, D. C., Reiter, R. E., et al. (2021). Prostate Cancer. Nat. Rev. Dis. Primers 7, 9. doi:10.1038/s41572-020-00243-0
Riley, R. S., June, C. H., Langer, R., and Mitchell, M. J. (2019). Delivery Technologies for Cancer Immunotherapy. Nat. Rev. Drug Discov. 18, 175–196. doi:10.1038/s41573-018-0006-z
Rycaj, K., Li, H., Zhou, J., Chen, X., and Tang, D. G. (2017). Cellular Determinants and Microenvironmental Regulation of Prostate Cancer Metastasis. Semin. Cancer Biol. 44, 83–97. doi:10.1016/j.semcancer.2017.03.009
Siegel, R. L., Miller, K. D., and Jemal, A. (2020). Cancer Statistics, 2020. CA Cancer J. Clin. 70, 7–30. doi:10.3322/caac.21590
Sung, H., Ferlay, J., Siegel, R. L., Laversanne, M., Soerjomataram, I., Jemal, A., et al. (2021). Global Cancer Statistics 2020: GLOBOCAN Estimates of Incidence and Mortality Worldwide for 36 Cancers in 185 Countries. CA Cancer J. Clin. 71, 209–249. doi:10.3322/caac.21660
Tate, J. G., Bamford, S., Jubb, H. C., Sondka, Z., Beare, D. M., Bindal, N., et al. (2019). COSMIC: the Catalogue of Somatic Mutations in Cancer. Nucleic Acids Res. 47, D941–D947. doi:10.1093/nar/gky1015
Taylor, B. S., Schultz, N., Hieronymus, H., Gopalan, A., Xiao, Y., Carver, B. S., et al. (2010). Integrative Genomic Profiling of Human Prostate Cancer. Cancer Cell 18, 11–22. doi:10.1016/j.ccr.2010.05.026
Teo, M. Y., Rathkopf, D. E., and Kantoff, P. (2019). Treatment of Advanced Prostate Cancer. Annu. Rev. Med. 70, 479–499. doi:10.1146/annurev-med-051517-011947
Ueda, T., Shiraishi, T., Ito, S., Ohashi, M., Matsugasumi, T., Yamada, Y., et al. (2021). Abiraterone Acetate versus Bicalutamide in Combination with Gonadotropin Releasing Hormone Antagonist Therapy for High Risk Metastatic Hormone Sensitive Prostate Cancer. Sci. Rep. 11, 10094. doi:10.1038/s41598-021-89609-2
Vaishampayan, U. N., Heilbrun, L. K., Monk, P., Tejwani, S., Sonpavde, G., Hwang, C., et al. (2021). Clinical Efficacy of Enzalutamide vs Bicalutamide Combined with Androgen Deprivation Therapy in Men with Metastatic Hormone-Sensitive Prostate Cancer. JAMA Netw. Open 4, e2034633. doi:10.1001/jamanetworkopen.2020.34633
van den Bulk, J., Verdegaal, E. M., and de Miranda, N. F. (2018). Cancer Immunotherapy: Broadening the Scope of Targetable Tumours. Open Biol. 8, 180037. doi:10.1098/rsob.180037
Wang, G., Zhao, D., Spring, D. J., and DePinho, R. A. (2018). Genetics and Biology of Prostate Cancer. Genes Dev. 32, 1105–1140. doi:10.1101/gad.315739.118
Wang, Z. (2017). ErbB Receptors and Cancer. Methods Mol. Biol. 1652, 3–35. doi:10.1007/978-1-4939-7219-7_1
Wellington, K., and Keam, S. J. (2006). Bicalutamide 150mg. Drugs 66, 837–850. doi:10.2165/00003495-200666060-00007
Wilkerson, M. D., and Hayes, D. N. (2010). ConsensusClusterPlus: a Class Discovery Tool with Confidence Assessments and Item Tracking. Bioinformatics 26, 1572–1573. doi:10.1093/bioinformatics/btq170
Wu, T., and Dai, Y. (2017). Tumor Microenvironment and Therapeutic Response. Cancer Lett. 387, 61–68. doi:10.1016/j.canlet.2016.01.043
Yang, W., Soares, J., Greninger, P., Edelman, E. J., Lightfoot, H., Forbes, S., et al. (2013). Genomics of Drug Sensitivity in Cancer (GDSC): a Resource for Therapeutic Biomarker Discovery in Cancer Cells. Nucleic Acids Res. 41, D955–D961. doi:10.1093/nar/gks1111
Keywords: metastasis, mutation, prostate cancer, treatment decision, unsupervised consensus clustering
Citation: Li Q, Xiao X, Chen B, Song G, Zeng K and Miao J (2022) Identification of a Gene Signature to Aid Treatment Decisions by Integrated Analysis of Mutated Genes Between Primary and Metastatic Prostate Cancer. Front. Genet. 13:877086. doi: 10.3389/fgene.2022.877086
Received: 16 February 2022; Accepted: 25 March 2022;
Published: 12 April 2022.
Edited by:
Apeng Chen, Lanzhou Veterinary Research Institute (CAAS), ChinaReviewed by:
Atrayee Bhattacharya, Dana–Farber Cancer Institute, United StatesNeeti Sharma, Ajeenkya D Y Patil University, India
Copyright © 2022 Li, Xiao, Chen, Song, Zeng and Miao. This is an open-access article distributed under the terms of the Creative Commons Attribution License (CC BY). The use, distribution or reproduction in other forums is permitted, provided the original author(s) and the copyright owner(s) are credited and that the original publication in this journal is cited, in accordance with accepted academic practice. No use, distribution or reproduction is permitted which does not comply with these terms.
*Correspondence: Jianping Miao, miaojianping888@hotmail.com