- Regenerative Medicine Clinic, State Key Laboratory of Experimental Hematology, National Clinical Research Center for Blood Diseases, Haihe Laboratory of Cell Ecosystem, Institute of Hematology & Blood Diseases Hospital, Chinese Academy of Medical Sciences & Peking Union Medical College, Tianjin, China
Acute myeloid leukemia (AML) is a genetically heterogeneous hematological malignancy with poor prognosis. We explored the RNA sequence data and clinical information of AML patients from The Cancer Genome Atlas (TCGA) and Genotype-Tissue Expression (GTEx) database to search for the core molecule for prognosis. The DC-STAMP expression was significantly higher in AML patients, which was linked to old age, unfavorable cytogenetic risk, and death (all p < 0.05). Furthermore, it was revealed that high DC-STAMP expression was an independent unfavorable factor for overall survival (OS) by univariate analysis [hazard ratio (HR): 2.683; 95% confidence interval (CI): 1.723–4.178; p < 0.001] and multivariate analysis (HR: 1.733; 95% CI: 1.079–2.781; p = 0.023). The concordance index (C-index 0.734, 95% CI: 0.706–0.762), calibration curves, and decision curve analysis showed the certain predictive accuracy of a nomogram model based on multivariate analysis for OS. In addition, we found that the differentially expressed gene (DEG) enrichment pathways of high- and low-DC-STAMP expression group enrichment pathways were focused on channel activity and platelet alpha granule by the Gene Ontology (GO) and Kyoto Encyclopedia of Genes and Genomes (KEGG), while gene set enrichment analysis (GSEA) pathways were mainly involved in mTORC1 signaling and TNF-α signaling via the NF-kB pathway. Moreover, a protein–protein interaction (PPI) network demonstrated that DC-STAMP interacted with two hub genes (PPBP and PF4), which were highly regulated and associated with poor survival. Finally, high DC-STAMP expression showed a significantly positive correlation with four immune cell [NK CD56 (dim) cells, macrophages, cytotoxic cells, and CD8 (+) T cells] infiltration and high level of immune checkpoint genes (PDCD1, CD274, CTLA-4, and TIGIT). Therefore, our results suggest that high expression of DC-STAMP predicts adverse outcomes for AML patients.
Introduction
Acute myeloid leukemia (AML) is a malignant clonal disease originating from hematopoietic stem cells (HSCs) or myeloid progenitors characterized by inhibiting cellular differentiation and proliferation of blast cells (Puram et al., 2016; Assi et al., 2019). Although most patients received traditional chemotherapy and allogeneic hematopoietic stem cell transplantation (allo-HSCT), more than 70% of the patients failed to achieve the desired effects (Chen et al., 2019). Based on some genetic abnormalities, the risk stratification system of AML has been refined and some AML patients tend to have deeper remission and longer survival through molecular targeted therapy (Estey, 2016; Stone et al., 2017; Kayser and Levis, 2018; DiNardo et al., 2020). However, there are limited numbers of reliable biomarkers for indicating the prognosis of AML and guiding therapy selection (Campos et al., 1993; Tzifi et al., 2012; Fröhling et al., 2002; Patel et al., 2012). Therefore, a much more in-depth and comprehensive research of the molecular abnormalities including genetic mutations and validation would aid in designing effective targeted therapies for AML.
Dendritic cell (DC)-specific transmembrane protein (DC-STAMP), also called TM7SF4, is a seven-transmembrane receptor protein, which is predominantly expressed in myeloid DC, macrophages, and osteoclasts (Hartgers et al., 2000; Yagi et al., 2005). However, DC-STAMP mRNA expression is low in hematopoietic stem and progenitor cells (HSCPs) and monocytes (Eleveld-Trancikova et al., 2008). It plays a role in the limitation of myeloid cell differentiation, regulation of the antigen presentation activity of DC, and maintenance of immune tolerance (Eleveld-Trancikova et al., 2008; Sawatani et al., 2008; Eleveld-Trancikova et al., 2010). A recent study demonstrated that the DC-STAMP was considered as an important molecule promoting the development and progression of multiple myeloma (Silvestris et al., 2011), whereas its role in AML is completely unknown.
In this research, we used not only the cox regression analysis but also a nomogram model, calibration curves, and a decision curve analysis (DCA) to assess the predictive effect of the DC-STAMP on AML patients based on TCGA database. In addition, we performed three types of enrichment analyses, protein–protein interaction (PPI), and a correlation analysis of immune infiltration or immune checkpoints to detect the pathogenic molecular mechanisms of the DC-STAMP. Our findings revealed the prognostic value of the DC-STAMP and may provide novel insights into the gene marker of leukemogenesis.
Materials and Methods
Data Source
The transcripts per million (TPM) reads format RNA-seq data of TCGA and GTEx were collected by the toil process from the UCSC XENA browser (https://xenabrowser.net/datapages/) (Vivian et al., 2017; Consortium, 2020; Goldman et al., 2020). The data of 173 cases of AML patients and 70 cases of normal people were extracted from TCGA’s LAML project and GTEx, respectively. The RNA-seq data of the TPM format was performed for an intrasample comparison after log2 transformation. The clinical data of AML patients were downloaded from TCGA (https://tcga-data.nci.nih.gov/), and 153 patients’ data were eligible for inclusion by removing patients without clinical data.
Differential Gene Expression Analysis
We used the median values of DC-STAMP mRNA expression to divide the AML patients into low and high DC-STAMP expression groups. The differentially expressed genes (DEGs) of the aforementioned two groups were identified by comparing the RNA-seq data of the HTSeq-count format by the DESeq2R package (Love et al., 2014). DEGs were defined as an absolute log2 fold change (|log2 FC|) >1.0 with an adjusted p value < 0.05.
Functional and Pathway Enrichment Analysis
The Gene Ontology (GO) functional gene annotation analysis is a common method used for the enrichment analysis of large-scale genes, including the biological process (BP), cellular component (CC), and molecular function (MF) (Gene Ontology Consortium, 2021). The Kyoto Encyclopedia of Genes and Genomes (KEGG) is a widely used database for information storage of genomes, biological pathways, and diseases and drugs (Kanehisa et al., 2021). We performed the GO and KEGG analyses of DEGs in AML using the R package clusterProfiler (Yu et al., 2012).
Gene Set Enrichment Analysis
GSEA is a method that evaluates the correlation between gene expression and phenotype from a pre-defined gene set and determines the relative contribution (Subramanian et al., 2005). We downloaded hallmark gene sets (h.all.v7.2. symbols.gmt) from the MsigDB and utilized the R package clusterProfiler to conduct the GSEA (Yu et al., 2012; Szklarczyk et al., 2019). It was considered a statistical significance when the p value was less than 0.05.
Construction of Protein–Protein Interaction Network
Known proteins and predicted protein–protein interaction were investigated by using the STRING website (https://string-db.org/) (Szklarczyk et al., 2019), which contained 9.6 million proteins and 138 million protein–protein interactions from 2,031 species. In this research, we used the STRING database to construct a PPI network of encoding DEGs, then visualized the results and screened hub genes using the Cytoscape software (version 3.7.1) (Shannon et al., 2003). We further performed the ggplot2 package in R to investigate the association of DC-STAMP expression with hub genes by the correlation heatmap.
Correlation Analysis of Immune Infiltration and Immune Checkpoint Genes
We applied the ssGSEA algorithm from the GSVA package (version 1.34.0) to estimate the Pearson correlation coefficient between DC-STAMP expression and immune cells and the association of the DC-STAMP with the abundance of the 24 types of infiltrated immune cells (Bindea et al., 2013; Hänzelmann et al., 2013). The involved immune cells were activated dendritic cells (aDCs), B cells, CD8 (+) T cells, cytotoxic cells, DCs, eosinophils, immature DCs (iDCs), macrophages, mast cells, neutrophils, NK CD56 (bright) cells, NK CD56 (dim) cells, NK cells, plasmacytoid DCs (pDCs), T cells, T helper cells, T central memory (Tcm) cells, T effector memory (Tem) cells, T follicular helper (Tfh) cells, T gamma delta (Tgd) cells, Th1 cells, Th17 cells, Th2 cells, and regulatory T (Treg) cells. We next performed the ggplot2 package in R to investigate the association of DC-STAMP expression with specific immune cells and widely discussed immune checkpoint genes (PDCD1, CD274, CTLA-4, LAG-3, TIGIT, and HAVCR2) by the scatter plot.
Statistical Methods
All statistical analyses were completed in R programming (https://www.r-project.org/, version 3.6.3). The effectiveness of the DC-STAMP in distinguishing AML from normal samples was assessed by the receiver operating characteristic (ROC) curve analysis using the pROC software. The difference between clinical features and DC-STAMP expression was detected by Wilcoxon rank sum tests and Kruskal–Wallis tests. The correlation of clinical features between low and high DC-STAMP expression was performed by the Pearson χ2 test. Survival curves were constructed using the Kaplan–Meier (KM) plot. The prognostic risk factors were identified by univariate analyses and the multivariate Cox regression analysis, then, independent factors were recruited for building the final nomogram prognostic model. Additionally, we used calibration and DCA to assess the predictive power of the nomogram model. The nomogram plot and calibration curve were established by using the RMS package in R and the DCA curve was constructed by using the survival package and stdca.R. in R. All tests were two-sided, and p < 0.05 was considered to be of statistical significance. The research and analysis flowchart is presented in Figure 1.
Results
High Expression of DC-STAMP is Linked to Unfavorable Clinical Characteristics in Acute Myeloid Leukemia
We compared the difference in DC-STAMP mRNA expression among AML, normal, and other malignancies samples by using the RNA-seq database. Remarkably, DC-STAMP expression was upregulated in multiple malignancies (Figure 2A), especially in AML (p < 0.05, Figure 2B). Moreover, the power of the expression difference was 0.672 (95% confidence interval, CI = 0.610–0.735, Figure 2C) by the AUC value of the ROC curve analysis. Hence, we are interested in the clinical implications of DC-STAMP expression in AML patients. A total of 153 AML patients with clinical information from TCGA were analyzed in the study. As shown in Figures 2D–G, DC-STAMP expression was associated with old age (p < 0.01, Figure 2D), unfavorable cytogenetic risk (p < 0.001, Figure 2E), NPM1 positive mutation (p < 0.05, Figure 2F), and death (p < 0.001, Figure 2G), no association with French-American-British (FAB) classifications (Supplementary Figure S1). Furthermore, when patients were grouped by low and high mRNA expression, a strong correlation was found in old age (p = 0.004), unfavorable cytogenetic risk category (p < 0.001), normal, +8, del (7) karyotype (p = 0.008), M2, M5 FAB subtypes (p = 0.037), and death (p = 0.001, Table 1), but no association with gender, white blood cell count, and FLT3, IDH1, RAS, and NPM1 mutations. Together, high DC-STAMP expression was closely related to poor clinical characteristics.
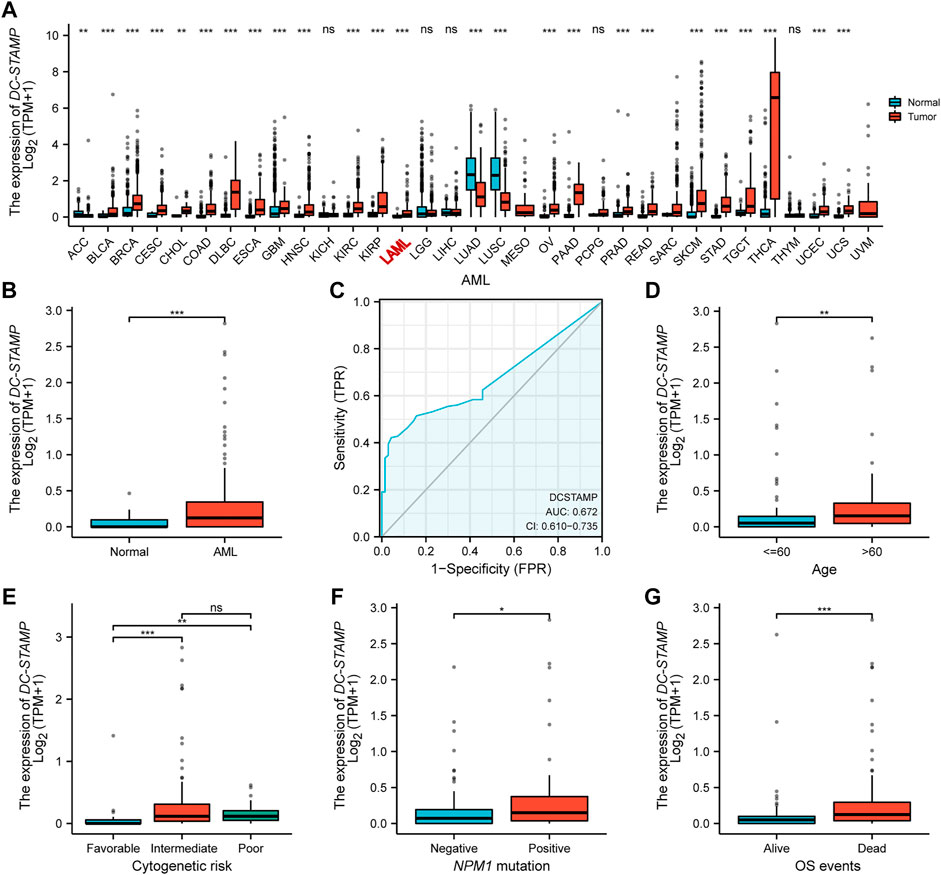
FIGURE 2. High DC-STAMP expression was related with adverse clinical features. (A) Level of DC-STAMP expression in different tumors from TCGA and GTEx database. (B) Expression levels of DC-STAMP in AML (n = 173) and normal samples (n = 70). (C) Receiver operating characteristic analysis (ROC) of DC-STAMP in AML. Clinical characteristics including (D) age, (E) cytogenetic risk classification, (F) NPM1 mutation, and (G) OS events (n = 153). Analysis between two groups of unpaired samples: Wilcoxon rank sum test, analysis among multiple groups of samples: Kruskal–Wallis rank sum test (ns p ≥ 0.05, *p < 0.05, **p < 0.01, ***p < 0.001).
High DC-STAMP Expression Predicts Worse Prognosis
We plotted OS curves by the KM method to identify the effect of the DC-STAMP on the outcomes in AML patients. Patients with a high expression presented a shorter OS than those with a low expression (p < 0.001, Figure 3A). We further use the univariate and multivariate Cox regression analyses to identify the value of the DC-STAMP for survival. By the univariate analysis, high DC-STAMP expression was associated with shorter OS [hazard radio, (HR): 2.683; 95% confidence interval (CI):1.723–4.178; p < 0.001, Supplementary Table S1]. Simultaneously, both age (HR: 3.333; 95% CI: 2.164–5.134; p < 0.001) and unfavorable cytogenetic risk (Intermediate: HR: 2.957; 95% CI; 1.498–5.836; p = 0.002, Poor: HR: 4.157; 95% CI: 1.944–8.893; p < 0.001) were related with poor OS. Then, we included the aforementioned significant univariable factors (p < 0.1) in the multivariate analysis and found that age (HR: 2.548; 95% CI: 1.601–4.055; p < 0.001), poor cytogenetic risk (HR: 2.293; 95% CI: 1.024–5.135; p = 0.044), and high DC-STAMP expression (HR: 1.733; 95% CI: 1.079–2.781; p = 0.023) were also independent prognostic factors, respectively. In detail, we drew forest plots to present the aforementioned results of the Cox regression analysis (Figures 3B,C, Supplementary Table S1).
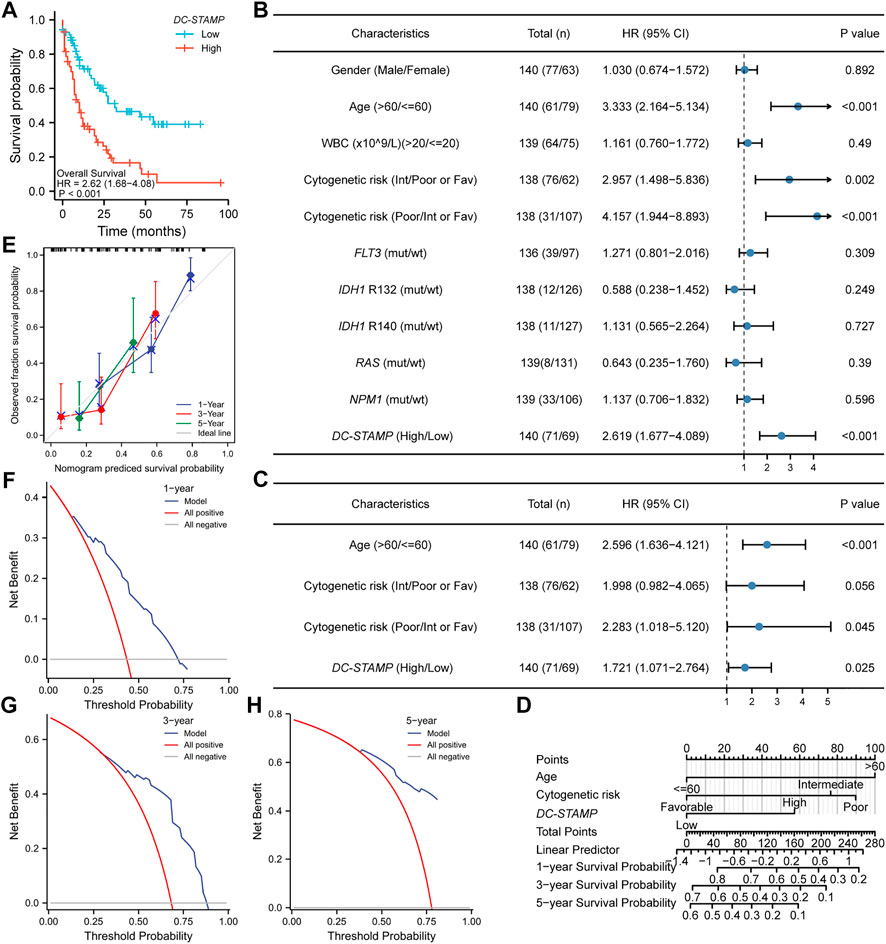
FIGURE 3. Poor prognostic value of DC-TAMP expression in AML. (A) KM curve analysis of overall survival (OS) between high and low DC-STAMP expression groups. (B) Univariate analyses of OS showed by forest plot. (C) Multivariate analyses of OS showed by forest plot. (D) Nomogram integrates DC-STAMP and other prognostic factors in AML. (E) Calibration curve of nomogram. The DCA curves of nomogram (F) by 1 year, (G) 3 years, and (H) 5 years(mut; mutation, wt; wild type, Int; intermediate, Fav; favorable).
Furthermore, to provide a quantitative prediction of the outcomes in AML patients, we constructed a nomogram plot using age, cytogenetic risk, and DC-STAMP expression (Figure 3D). The concordance index (C-index) for predicting the OS was 0.734 (95% CI: 0.706–0.762), indicating that the nomogram had a certain predictive accuracy for OS. Additionally, we performed calibration curves and DCA to evaluate the predictive performance of the nomogram model. The calibration curves presented consistency between the predicted OS of the nomogram and the actual proportion of OS at 1-, 3-, and 5-year (Figure 3E). Moreover, the DCA curves also verified the clinical utility of the predictive nomogram (Figures 3F–H). In summary, this nomogram model had an accurate ability to predict the patients’ survival.
Differentially Expressed Gene Enrichment Analysis Reveals the Dysfunctional Signaling Pathway
We further explored the potential mechanisms in AML patients. Firstly, we identified the DEGs between high and low DC-STAMP expression. In total, 610 DEGs were obtained and shown in volcano plots (Figure 4A), including 260 upregulated genes and 350 downregulated genes (|log2FC| >1, adjusted p value < 0.05).
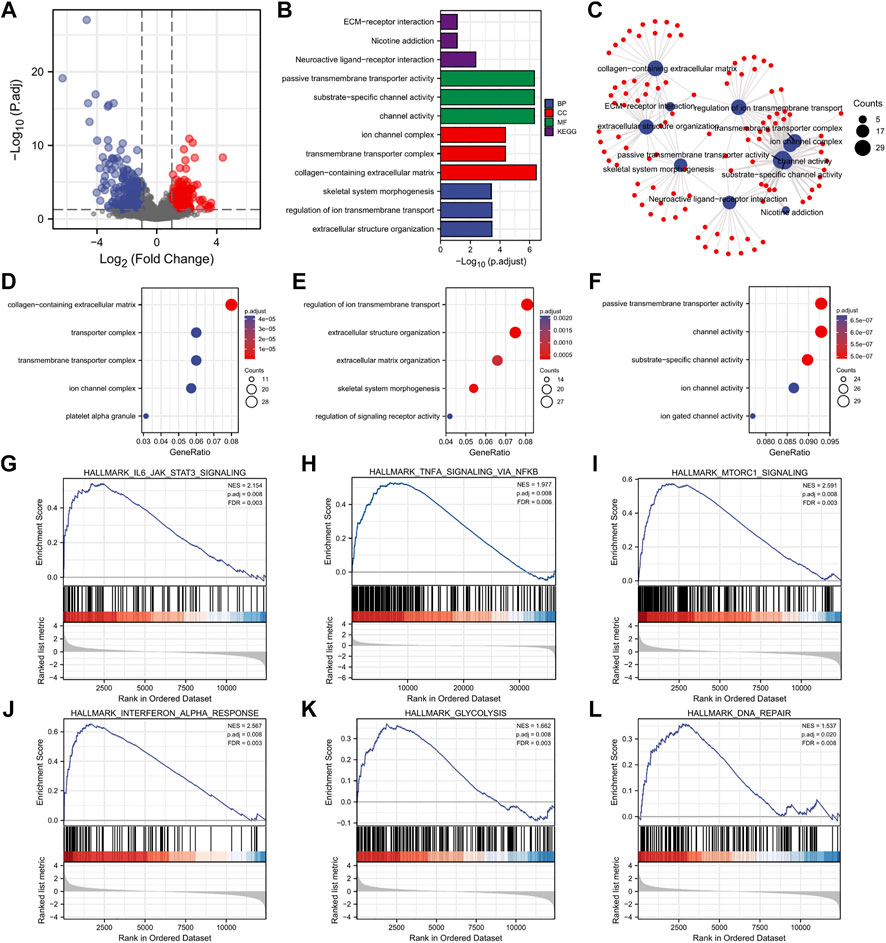
FIGURE 4. DEG analysis and functional enrichment of high and low DC-STAMP expression groups in AML. (A) Volcano plot of DEGs. (B) GO and KEGG pathway of DEGs. (C) Interactive analysis with result of GO and KEGG analyses. (D–F) GO enrichment analysis of DEGs. (G–L) GSEA of DEGs. (G) Enrichment of genes in IL6-JAK-STAT3 signaling pathway. (H) Enrichment of genes in inflammatory pathway. (I) Enrichment of genes in mTORC1 signaling pathway. (J) Enrichment of genes in interferon alpha response pathway. (K) Enrichment of genes in glycolysis signaling pathway. (L) Enrichment of genes in DNA repair signaling pathway.
Next, we performed the GO and KEGG analyses to investigate the biological function of the aforementioned DEGs and obtained the top 15 GO enrichment items (CC five items, BP five items, and MF five items) and top five KEGG pathways (Figures 4B,D–F and Supplementary Table S2). Briefly, the channel activity, platelet alpha granule, extracellular structure organization, regulation of ion transmembrane transport, neuroactive ligand-receptor interaction, and extracellular matrix (ECM)–receptor interactions were the most enriched sets.
Moreover, to better understand the mutual connection, we performed an interactive analysis derived from the results of GO and KEGG analyses. It showed that the numbers of enrichment genes were channel activity (counts = 29), passive transmembrane transporter activity (counts = 29), collagen-containing extracellular matrix (counts = 28), substrate-specific channel activity (counts = 28), regulation of ion transmembrane transport (counts = 27), extracellular structure organization (counts = 25), transmembrane transporter complex (counts = 21), ion channel complex (counts = 20), neuroactive ligand-receptor interaction (counts = 19), and skeletal system morphogenesis (counts = 18) (Figure 4C).
Finally, the GSEA was used to investigate the enrichment pathway of DC-STAMP expression, and a total of 28 significant pathways were enriched (Table 2). Interestingly, some pathways, such as IL6-JAK-STAT3 signaling, mTORC1 signaling, TNF-α signaling via NF-κB, INF-γ response, glycolysis, and DNA repair (Figures 4J–L) were reported to correlate with leukemogenesis (Steelman et al., 2008; Park et al., 2010; Binder et al., 2018; Molina et al., 2018; Gabellier et al., 2020; Grants et al., 2020).
Identification of Hub Genes Connected With DC-STAMP
As indicated in Figure 5A, the PPI network of 358 encoding DEGs was constructed to determine the hub genes. The top 15 hub genes were selected by the maximum neighborhood component (MNC), density of maximum neighborhood component (DMNC), and maximal clique centrality (MCC) algorithms, respectively (Figures 5B–D). Therefore, we observed four hub genes (SELP, SERPINE1, PF4, and PPBP) shared from the aforementioned three gene lists. We analyzed the association between the DC-STAMP and four hub genes. It indicated that the DC-STAMP has significant positive correlations with SELP (p < 0.001, correlation coefficient: 0.364), PF4 (p < 0.001, correlation coefficient: 0.39), and PPBP (p < 0.001, correlation coefficient: 0.406) (Figure 5E). In contrast, the DC-STAMP and SERPINE1 were negatively correlated (p = 0.005, correlation coefficient: 0.228) (Figure 5E). Finally, an analysis of the relationship between the four hub genes and clinical prognosis in AML patients revealed that only PF4 and PPBP were expressed at a high level, which was associated with poor outcomes (Figures 5F–I).
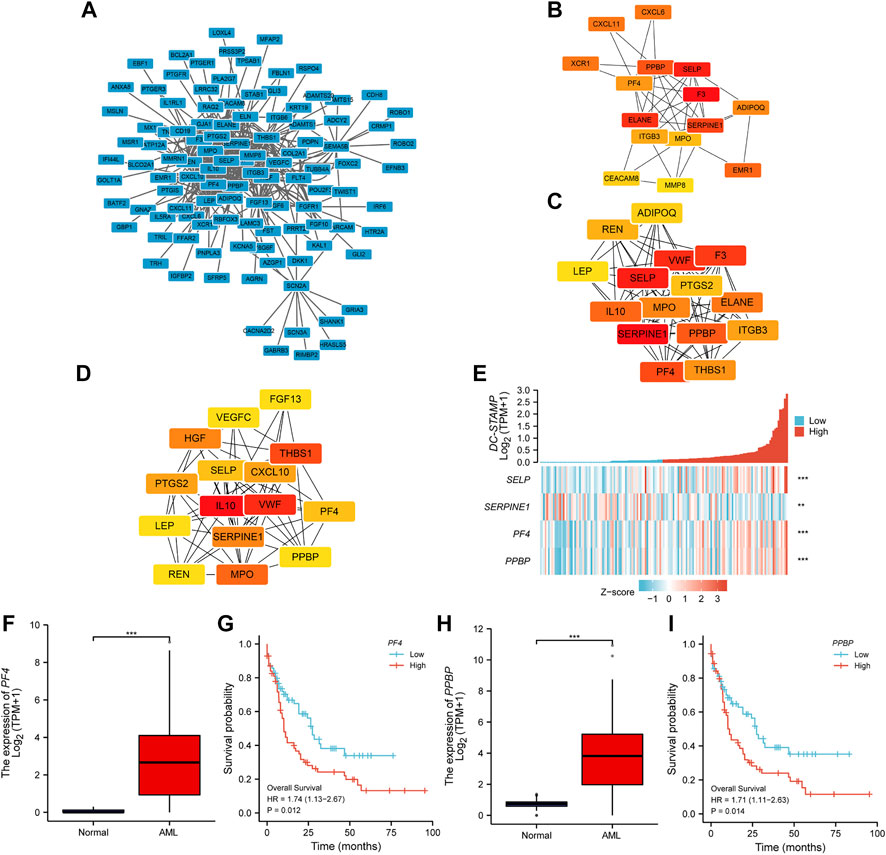
FIGURE 5. PPI network construction and clinical significance of hub genes. (A) The PPI network of 358 encoding DEGs. (B–D) Top 15 hub genes were selected respectively by (B) MNC, (C) DMNC, and (D) MCC. (E) Association of DC-STAMP with four hub genes (SELP, SFRPINE1, PF4, and PPBP). (F) Expression levels of PF4 in AML (n = 173) and normal subjects (n = 70). (G) Different OS between high- and low-PF4 expression levels shown by KM curves. (H) Expression levels of PPBP in AML (n = 173) and normal subjects (n = 70). (I) Different OS between high- and low-PPBP expression levels shown by KM curves. (*p < 0.05, **p < 0.01, ***p < 0.001).
Correlation Analysis Between DC-STAMP and Immune Cell or Immune Checkpoint Molecules
To describe the association of DC-STAMP expression with immune infiltration in AML, we systematically evaluated 24 kinds of infiltrated immune cells. The result showed that the level of DC-STAMP expression had a significant positive correlation with the infiltrating level of NK CD56 (dim) cells, macrophages, cytotoxic cells, and CD8 (+) T cells (Figure 6A). The details of a quantified analysis by Spearman’s correlation are shown in Figures 6B–E. Furthermore, we analyzed the relationship between DC-STAMP expressions and widely discussed immune checkpoint genes (PDCD1, CD274, CTLA-4, LAG-3, TIGIT, and HAVCR2). As shown in Figure 6F, the level of DC-STAMP gene expression was significantly and positively correlated with PDCD1, CD274, CTLA-4, and TIGIT. The specific correlation analysis is shown in Figures 6G–J.
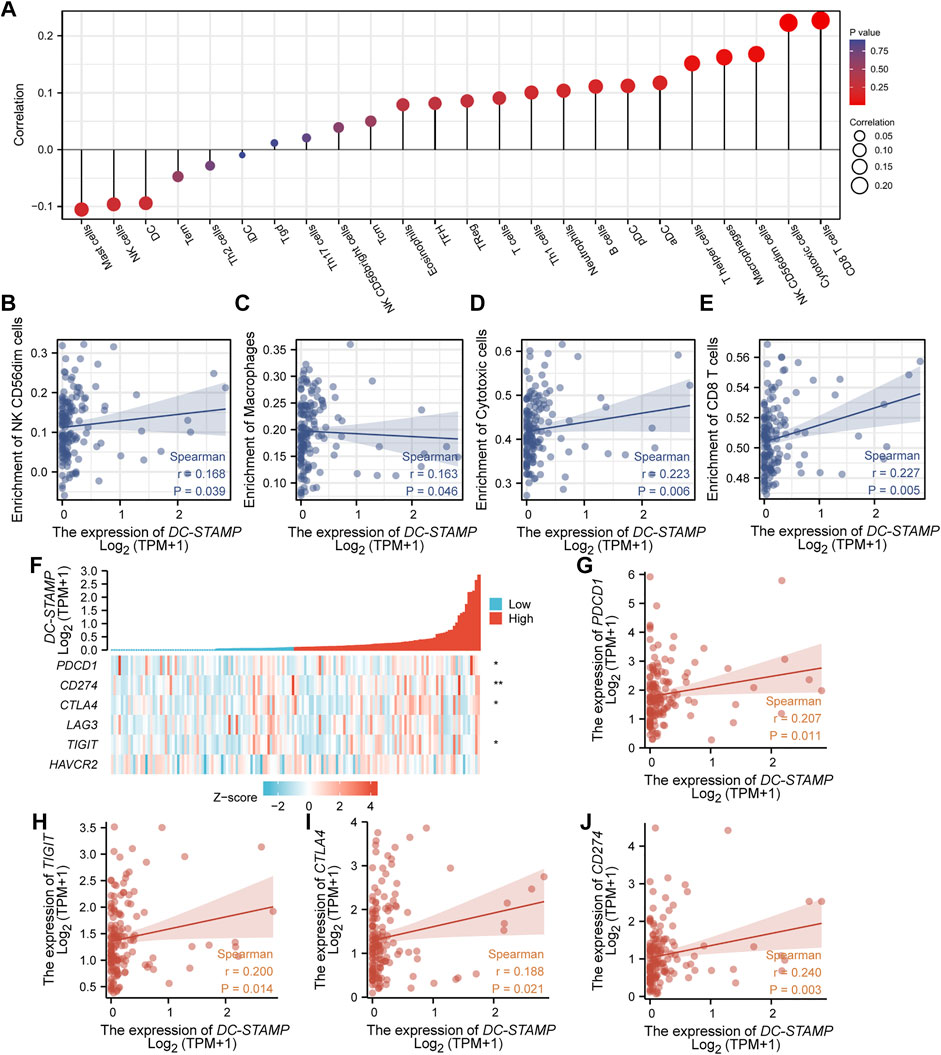
FIGURE 6. Correlation analysis between the level of DC-STAMP gene expression and immune cell infiltration or immune checkpoint molecules. (A) Association between DC-STAMP expression and 24 kinds of infiltrated immune cells. (B–E) Using Spearman’s correlation in quantified analysis of the correlation of DC-STAMP expression with infiltrating level of (B) NK CD56 (dim) cells, (C) macrophages, (D) cytotoxic cells and (E) and CD8 (+) T cells. (F) Association of DC-STAMP with five immune checkpoint molecules (PDCD1, CD274, CTLA-4, LAG-3, TIGIT, and HAVCR2). (G–J) Using Spearman’s correlation in quantified analysis of the correlation of DC-STAMP expression with (G) PDCD1, (H) TIGIT, (I) CTLA-4, and (J) CD274 (r was Spearman’s correlation coefficient) (*p < 0.05, **p < 0.01, ***p < 0.001).
Discussion
AML is a hematological neoplastic disease and involves many different molecular genetic abnormalities. The DC-STAMP is considered to be a receptor protein, which functions by promoting DC antigen-presentation and osteoclast activation. Only few studies have revealed that overexpression of the DC-STAMP would influence the differentiation of myeloid lineage cells (Eleveld-Trancikova et al., 2008). It is also reported that normal HSCPs remain at the low level of the DC-STAMP (Eleveld-Trancikova et al., 2008; Eleveld-Trancikova et al., 2010). According to a recent review, a high DC-STAMP expression level may have potential pathogenic impacts on myeloid malignancies. However, it is still unknown whether DC-STAMP has an impact on AML.
Based on the aforementioned situation, we first investigated the association between the DC-STAMP and clinical features of AML by using TCGA database. As expected, an upregulated DC-STAMP expression was observed, and a high level of the DC-STAMP gene was correlated with adverse clinical characteristics and poor survival. Thus, it was consistent with the hypothesis that an abnormally high level of DC-STAMP expression blocked the differentiation of HSCs in AML patients.
Additionally, we preliminarily explored the pathogenic molecular mechanisms of the DC-STAMP by using various bioinformatics analyses. Expectedly, we found DEG enrichment pathways were focused on the molecular transport process and platelet alpha granule by GO and KEGG, while GSEA pathways were involved in mTORC1 signaling, TNF-α signaling via NF-κB, and inflammatory and DNA repair pathways. A previous research work reported that the activation of mTORC1 signaling promotes the proliferation and survival of the leukemic clones (Steelman et al., 2008; Park et al., 2010) and cytotoxicity in AML cells from the selective AMPK agonist (GSK621) because of mTORC1 activation which was through the eIF2α/ATF4 signaling pathway (Sujobert et al., 2015). Another study also revealed that the mTORC1 pathway had a correlation with easy relapse and disease progression in AML (Oki et al., 2021). Grants et al. (2020) mentioned that NF-κB, IL6, and TNF were a kind of potential drivers of HSC dysfunction, activating inflammatory signaling in myeloid malignancy. As we know, proinflammatory factors were linked to blast cell growth, and the dysregulation of cytokine signaling contributed to a beneficial AML microenvironment (Binder et al., 2018). Therefore, we think that the effect of the DC-STAMP on potential pathogens is probably associated with the aforementioned signaling pathways.
Furthermore, we obtained two hub genes (PF4 and PPBP) with poor OS through different PPI calculation methods and survival analyses. It has been reported that PF4 and PPBP belonged to the CXC chemokine family and played roles in platelet activation, platelet degranulation, immune response to infection, activation of neutrophils and monocytes, and tumorigenesis. (Yan et al., 1994; Martí et al., 2002; Schaffner, 2005; Strieter et al., 2006; Sakurai et al., 2016). These two genes also had been implicated in acute megakaryocytic leukemia, lung adenocarcinoma, gastric cancer, and several autoimmune disorders, including rheumatoid arthritis and Crohn’s disease (Ulivi et al., 2013; Pelleri et al., 2014; Takeyama et al., 2015; Pucci et al., 2016; Xia et al., 2017; Wu et al., 2021). Although the DC-STAMP, PF4, and PPBP had links with tumor-associated immune response, the mechanisms of the synergistic effects of their interaction remain unclear. A more in-depth detection is needed to explore this complex correlation in AML patients in future.
Finally, when analyzing the relationship between DC-STAMP expression and immune cell infiltration, we found that the high DC-STAMP group was inclined to harbor more immune cells with cytotoxic effects. As previously demonstrated, the DC-STAMP promoted the most efficient CD4 (+) and CD8 (+) T-cell responses in vitro (Moulin et al., 2012). Moreover, AML patients had a trend toward increased mature NK cells (NK CD56 (dim) cells) (Tang et al., 2020). We also found DC-STAMP expression had a correlation with PDCD1, CD274, CTLA-4, and TIGIT which were exhaustion markers of T cells and considered a dysfunction of anti-tumor immunity (Noviello et al., 2019; Wang et al., 2021). A recent study showed that PD1-positive/CD8-positive T cells were higher in relapsed AML patients, compared with newly diagnosed AML patients (Williams et al., 2019). This result may suggest that the DC-STAMP was closely related to the immune escape of AML. However, the detailed pathological mechanism of the DC-STAMP remains unknown and needs further exploration in the future. The research of DC-STAMP expression or the relationship between the DC-STAMP and immune checkpoints would be a benefit for the discovery of new immunotherapeutic targets to improve the survival of AML patients.
However, our study still has the following limitations that cannot be ignored. Firstly, we investigate the diagnostic effect of the DC-STAMP because of the publicly available TCGA AML database and this observation needs to be subsequently validated in larger independent cohorts. Secondly, although this research comprehensively describes the impact of the DC-STAMP level on the survival of AML patients, it lacks the exploration of DC-STAMP pathogenic mutations. Lastly, all the interactions between the DC-STAMP and AML-associated immune response lack functional validation and detection of the potential molecular mechanisms. Therefore, further laboratory work is required to make up for the aforementioned shortcomings.
Conclusion
In this research, it was shown that high expression of DC-STAMP has an adverse effect on the overall survival of AML patients and is linked to both AML-associated pathway activation and special immune cells or checkpoints, which suggests that high expression of DC-STAMP may be a potential independent prognostic factor and an immunotherapeutic target for AML. This finding could help clinicians decide on optimal regimens and explore new targeted therapies for AML patients.
Data Availability Statement
All data were collected and downloaded from TCGA and GTEx database using the following links: Clinical data of AML was downloaded from TCGA: https://portal.gdc.cancer.gov/projects/TCGA-LAML RNA-seq data of TCGA and GTEx was collected from UCSC XENA browser: https://xenabrowser.net/datapages/.
Ethics Statement
Written and informed consent was obtained from the individual(s) for the publication of any potential identifiable images or data included in this article.
Author Contributions
QL, LZ, WW, and JS conceived the study. QL, LZ, and WW. collected and analyzed the data and wrote the paper. QL, LZ, WW, QL, and LF, contributed to the data collection and analysis. JS designed the research and gave approval of the final manuscript.
Funding
This work was supported by the Tianjin Municipal Science and Technology Commission Major Project (18ZXDBSY00070), the National Natural Science Foundation of China (81670120), and CAMS Innovation Fund for Medical Sciences (2021-I2M-1-073).
Conflict of Interest
The authors declare that the research was conducted in the absence of any commercial or financial relationships that could be construed as a potential conflict of interest.
Publisher’s Note
All claims expressed in this article are solely those of the authors and do not necessarily represent those of their affiliated organizations, or those of the publisher, the editors, and the reviewers. Any product that may be evaluated in this article, or claim that may be made by its manufacturer, is not guaranteed or endorsed by the publisher.
Supplementary Material
The Supplementary Material for this article can be found online at: https://www.frontiersin.org/articles/10.3389/fgene.2022.876689/full#supplementary-material
Supplementary Figure S1 | DC-STAMP expression has no association with French-American-British (FAB) classifications.
Supplementary Table S1 | Univariate and multivariate Cox regression analyses.
Supplementary Table S2 | GO enrichment analysis and KEGG pathway analysis.
References
Assi, S. A., Imperato, M. R., Coleman, D. J. L., Pickin, A., Potluri, S., Ptasinska, A., et al. (2019). Subtype-Specific Regulatory Network Rewiring in Acute Myeloid Leukemia. Nat. Genet. 51 (1), 151–162. doi:10.1038/s41588-018-0270-1
Bindea, G., Mlecnik, B., Tosolini, M., Kirilovsky, A., Waldner, M., Obenauf, A. C., et al. (2013). Spatiotemporal Dynamics of Intratumoral Immune Cells Reveal the Immune Landscape in Human Cancer. Immunity 39 (4), 782–795. doi:10.1016/j.immuni.2013.10.003
Binder, S., Luciano, M., and Horejs-Hoeck, J. (2018). The Cytokine Network in Acute Myeloid Leukemia (AML): A Focus on Pro- and Anti-Inflammatory Mediators. Cytokine Growth Factor. Rev. 43, 8–15. doi:10.1016/j.cytogfr.2018.08.004
Campos, L., Rouault, J., Sabido, O., Oriol, P., Roubi, N., Vasselon, C., et al. (1993). High Expression of Bcl-2 Protein in Acute Myeloid Leukemia Cells Is Associated with Poor Response to Chemotherapy. Blood 81 (11), 3091–3096. doi:10.1182/blood.v81.11.3091.3091
Chen, X., Glytsou, C., Zhou, H., Narang, S., Reyna, D. E., Lopez, A., et al. (2019). Targeting Mitochondrial Structure Sensitizes Acute Myeloid Leukemia to Venetoclax Treatment. Cancer Discov. 9 (7), 890–909. doi:10.1158/2159-8290.CD-19-0117
Consortium, G. T. (2020). The GTEx Consortium Atlas of Genetic Regulatory Effects across Human Tissues. Science 369 (6509), 1318–1330. doi:10.1126/science.aaz1776
DiNardo, C. D., Jonas, B. A., Pullarkat, V., Thirman, M. J., Garcia, J. S., Wei, A. H., et al. (2020). Azacitidine and Venetoclax in Previously Untreated Acute Myeloid Leukemia. N. Engl. J. Med. 383 (7), 617–629. doi:10.1056/NEJMoa2012971
Eleveld-Trancikova, D., Janssen, R. A. J., Hendriks, I. A. M., Looman, M. W. G., Moulin, V., Jansen, B. J. H., et al. (2008). The DC-Derived Protein DC-STAMP Influences Differentiation of Myeloid Cells. Leukemia 22 (2), 455–459. doi:10.1038/sj.leu.2404910
Eleveld-Trancikova, D., Sanecka, A., van Hout-Kuijer, M. A., Looman, M. W. G., Hendriks, I. A. M., Jansen, B. J. H., et al. (2010). DC-STAMP Interacts with ER-Resident Transcription Factor LUMAN Which Becomes Activated during DC Maturation. Mol. Immunol. 47 (11-12), 1963–1973. doi:10.1016/j.molimm.2010.04.019
Estey, E. (2016). Acute Myeloid Leukemia - Many Diseases, Many Treatments. N. Engl. J. Med. 375 (21), 2094–2095. doi:10.1056/NEJMe1611424
Fröhling, S., Schlenk, R. F., Breitruck, J., Benner, A., Kreitmeier, S., Tobis, K., et al. (2002). Prognostic Significance of Activating FLT3 Mutations in Younger Adults (16 to 60 years) with Acute Myeloid Leukemia and normal Cytogenetics: A Study of the AML Study Group Ulm. Blood 100 (13), 4372–4380. doi:10.1182/blood-2002-05-1440
Gabellier, L., Bret, C., Bossis, G., Cartron, G., and Moreaux, J. (2020). DNA Repair Expression Profiling to Identify High-Risk Cytogenetically Normal Acute Myeloid Leukemia and Define New Therapeutic Targets. Cancers 12 (10), 2874. doi:10.3390/cancers12102874
Gene Ontology Consortium (2021). The Gene Ontology Resource: Enriching a GOld Mine. Nucleic Acids Res. 49 (D1), D325–D334. doi:10.1093/nar/gkaa1113
Goldman, M. J., Craft, B., Hastie, M., Repečka, K., McDade, F., Kamath, A., et al. (2020). Visualizing and Interpreting Cancer Genomics Data via the Xena Platform. Nat. Biotechnol. 38 (6), 675–678. doi:10.1038/s41587-020-0546-8
Grants, J. M., Wegrzyn, J., Hui, T., O’Neill, K., Shadbolt, M., Knapp, D. J. H. F., et al. (2020). Altered microRNA Expression Links IL6 and TNF-Induced Inflammaging with Myeloid Malignancy in Humans and Mice. Blood 135 (25), 2235–2251. doi:10.1182/blood.2019003105
Hänzelmann, S., Castelo, R., and Guinney, J. (2013). GSVA: Gene Set Variation Analysis for Microarray and RNA-Seq Data. BMC Bioinformatics 14, 7. doi:10.1186/1471-2105-14-7
Hartgers, F. C., Vissers, J. L. M., Looman, M. W. G., Zoelen, C. v., Huffine, C., Figdor, C. G., et al. (2000). DC-STAMP, a Novel Multimembrane-Spanning Molecule Preferentially Expressed by Dendritic Cells. Eur. J. Immunol. 30 (12), 3585–3590. doi:10.1002/1521-4141(200012)30:12<3585::aid-immu3585>3.0.co;2-y
Kanehisa, M., Sato, Y., and Kawashima, M. (2021). KEGG Mapping Tools for Uncovering Hidden Features in Biological Data. Protein Sci. 31, 47–53. doi:10.1002/pro.4172
Kayser, S., and Levis, M. J. (2018). Advances in Targeted Therapy for Acute Myeloid Leukaemia. Br. J. Haematol. 180 (4), 484–500. doi:10.1111/bjh.15032
Love, M. I., Huber, W., and Anders, S. (2014). Moderated Estimation of Fold Change and Dispersion for RNA-Seq Data with DESeq2. Genome Biol. 15 (12), 550. doi:10.1186/s13059-014-0550-8
Martí, F., Bertran, E., Llucià, M., Villén, E., Peiró, M., Garcia, J., et al. (2002). Platelet Factor 4 Induces Human Natural Killer Cells to Synthesize and Release Interleukin-8. J. Leukoc. Biol. 72 (3), 590–597. doi:10.1189/jlb.72.3.590
Molina, J. R., Sun, Y., Protopopova, M., Gera, S., Bandi, M., Bristow, C., et al. (2018). An Inhibitor of Oxidative Phosphorylation Exploits Cancer Vulnerability. Nat. Med. 24 (7), 1036–1046. doi:10.1038/s41591-018-0052-4
Moulin, V., Morgan, M. E., Eleveld-Trancikova, D., Haanen, J. B. A. G., Wielders, E., Looman, M. W. G., et al. (2012). Targeting Dendritic Cells with Antigen via Dendritic Cell-Associated Promoters. Cancer Gene Ther. 19 (5), 303–311. doi:10.1038/cgt.2012.2
Noviello, M., Manfredi, F., Ruggiero, E., Perini, T., Oliveira, G., Cortesi, F., et al. (2019). Bone Marrow Central Memory and Memory Stem T-Cell Exhaustion in AML Patients Relapsing after HSCT. Nat. Commun. 10 (1), 1065. doi:10.1038/s41467-019-08871-1
Oki, T., Mercier, F., Kato, H., Jung, Y., McDonald, T. O., Spencer, J. A., et al. (2021). Imaging Dynamic mTORC1 Pathway Activity In Vivo Reveals Marked Shifts that Support Time-Specific Inhibitor Therapy in AML. Nat. Commun. 12 (1), 245. doi:10.1038/s41467-020-20491-8
Park, S., Chapuis, N., Tamburini, J., Bardet, V., Cornillet-Lefebvre, P., Willems, L., et al. (2010). Role of the PI3K/AKT and mTOR Signaling Pathways in Acute Myeloid Leukemia. Haematologica 95 (5), 819–828. doi:10.3324/haematol.2009.013797
Patel, J. P., Gönen, M., Figueroa, M. E., Fernandez, H., Sun, Z., Racevskis, J., et al. (2012). Prognostic Relevance of Integrated Genetic Profiling in Acute Myeloid Leukemia. N. Engl. J. Med. 366 (12), 1079–1089. doi:10.1056/NEJMoa1112304
Pelleri, M. C., Piovesan, A., Caracausi, M., Berardi, A. C., Vitale, L., and Strippoli, P. (2014). Integrated Differential Transcriptome Maps of Acute Megakaryoblastic Leukemia (AMKL) in Children with or without Down Syndrome (DS). BMC Med. Genomics 7, 63. doi:10.1186/s12920-014-0063-z
Pucci, F., Rickelt, S., Newton, A. P., Garris, C., Nunes, E., Evavold, C., et al. (2016). PF4 Promotes Platelet Production and Lung Cancer Growth. Cel Rep. 17 (7), 1764–1772. doi:10.1016/j.celrep.2016.10.031
Puram, R. V., Kowalczyk, M. S., de Boer, C. G., Schneider, R. K., Miller, P. G., McConkey, M., et al. (2016). Core Circadian Clock Genes Regulate Leukemia Stem Cells in AML. Cell 165 (2), 303–316. doi:10.1016/j.cell.2016.03.015
Sakurai, K., Fujiwara, T., Hasegawa, S., Okitsu, Y., Fukuhara, N., Onishi, Y., et al. (2016). Inhibition of Human Primary Megakaryocyte Differentiation by Anagrelide: A Gene Expression Profiling Analysis. Int. J. Hematol. 104 (2), 190–199. doi:10.1007/s12185-016-2006-2
Sawatani, Y., Miyamoto, T., Nagai, S., Maruya, M., Imai, J., Miyamoto, K., et al. (2008). The Role of DC-STAMP in Maintenance of Immune Tolerance through Regulation of Dendritic Cell Function. Int. Immunol. 20 (10), 1259–1268. doi:10.1093/intimm/dxn082
Schaffner, A. (2005). Regulated Expression of Platelet Factor 4 in Human Monocytes-Rrole of PARs as a Quantitatively Important Monocyte Activation Pathway. J. Leukoc. Biol. 78 (1), 202–209. doi:10.1189/jlb.0105024
Shannon, P., Markiel, A., Ozier, O., Baliga, N. S., Wang, J. T., Ramage, D., et al. (2003). Cytoscape: A Software Environment for Integrated Models of Biomolecular Interaction Networks. Genome Res. 13 (11), 2498–2504. doi:10.1101/gr.1239303
Silvestris, F., Ciavarella, S., Strippoli, S., and Dammacco, F. (2011). Cell Fusion and Hyperactive Osteoclastogenesis in Multiple Myeloma. Adv. Exp. Med. Biol. 714, 113–128. doi:10.1007/978-94-007-0782-5_5
Steelman, L. S., Abrams, S. L., Whelan, J., Bertrand, F. E., Ludwig, D. E., Bäsecke, J., et al. (2008). Contributions of the Raf/MEK/ERK, PI3K/PTEN/Akt/mTOR and Jak/STAT Pathways to Leukemia. Leukemia 22 (4), 686–707. doi:10.1038/leu.2008.26
Stone, R. M., Mandrekar, S. J., Sanford, B. L., Laumann, K., Geyer, S., Bloomfield, C. D., et al. (2017). Midostaurin Plus Chemotherapy for Acute Myeloid Leukemia with a FLT3 Mutation. N. Engl. J. Med. 377 (5), 454–464. doi:10.1056/NEJMoa1614359
Strieter, R. M., Burdick, M. D., Mestas, J., Gomperts, B., Keane, M. P., and Belperio, J. A. (2006). Cancer CXC Chemokine Networks and Tumour Angiogenesis. Eur. J. Cancer 42 (6), 768–778. doi:10.1016/j.ejca.2006.01.006
Subramanian, A., Tamayo, P., Mootha, V. K., Mukherjee, S., Ebert, B. L., Gillette, M. A., et al. (2005). Gene Set Enrichment Analysis: A Knowledge-Based Approach for Interpreting Genome-wide Expression Profiles. Proc. Natl. Acad. Sci. U.S.A. 102 (43), 15545–15550. doi:10.1073/pnas.0506580102
Sujobert, P., Poulain, L., Paubelle, E., Zylbersztejn, F., Grenier, A., Lambert, M., et al. (2015). Co-Activation of AMPK and mTORC1 Induces Cytotoxicity in Acute Myeloid Leukemia. Cel Rep. 11 (9), 1446–1457. doi:10.1016/j.celrep.2015.04.063
Szklarczyk, D., Gable, A. L., Lyon, D., Junge, A., Wyder, S., Huerta-Cepas, J., et al. (2019). STRING V11: Protein-Protein Association Networks with Increased Coverage, Supporting Functional Discovery in Genome-Wide Experimental Datasets. Nucleic Acids Res. 47 (D1), D607–D613. doi:10.1093/nar/gky1131
Takeyama, H., Mizushima, T., Iijima, H., Shinichiro, S., Uemura, M., Nishimura, J., et al. (2015). Platelet Activation Markers Are Associated with Crohn's Disease Activity in Patients with Low C-Reactive Protein. Dig. Dis. Sci. 60 (11), 3418–3423. doi:10.1007/s10620-015-3745-2
Tang, L., Wu, J., Li, C.-G., Jiang, H.-W., Xu, M., Du, M., et al. (2020). Characterization of Immune Dysfunction and Identification of Prognostic Immune-Related Risk Factors in Acute Myeloid Leukemia. Clin. Cancer Res. 26 (7), 1763–1772. doi:10.1158/1078-0432.ccr-19-3003
Tzifi, F., Economopoulou, C., Gourgiotis, D., Ardavanis, A., Papageorgiou, S., and Scorilas, A. (2012). The Role of BCL2 Family of Apoptosis Regulator Proteins in Acute and Chronic Leukemias. Adv. Hematol. 2012, 524308. doi:10.1155/2012/524308
Ulivi, P., Mercatali, L., Casoni, G.-L., Scarpi, E., Bucchi, L., Silvestrini, R., et al. (2013). Multiple Marker Detection in Peripheral Blood for NSCLC Diagnosis. PLoS ONE 8 (2), e57401. doi:10.1371/journal.pone.0057401
Vivian, J., Rao, A. A., Nothaft, F. A., Ketchum, C., Armstrong, J., Novak, A., et al. (2017). Toil Enables Reproducible, Open Source, Big Biomedical Data Analyses. Nat. Biotechnol. 35 (4), 314–316. doi:10.1038/nbt.3772
Wang, X., Chen, Y., Li, Z., Huang, B., Xu, L., Lai, J., et al. (2021). Single‐Cell RNA‐Seq of T Cells in B‐ALL Patients Reveals an Exhausted Subset with Remarkable Heterogeneity. Adv. Sci. 8 (19), 2101447. doi:10.1002/advs.202101447
Williams, P., Basu, S., Garcia‐Manero, G., Hourigan, C. S., Oetjen, K. A., Cortes, J. E., et al. (2019). The Distribution of T‐cell Subsets and the Expression of Immune Checkpoint Receptors and Ligands in Patients with Newly Diagnosed and Relapsed Acute Myeloid Leukemia. Cancer 125 (9), 1470–1481. doi:10.1002/cncr.31896
Wu, F., Chen, C., and Peng, F. (2021). Potential Association between Asthma, Helicobacter P Infection, and Gastric Cancer. Front. Oncol. 11, 630235. doi:10.3389/fonc.2021.630235
Xia, W., Wu, J., Deng, F.-Y., Wu, L.-F., Zhang, Y.-H., Guo, Y.-F., et al. (2017). Integrative Analysis for Identification of Shared Markers from Various Functional Cells/Tissues for Rheumatoid Arthritis. Immunogenetics 69 (2), 77–86. doi:10.1007/s00251-016-0956-4
Yagi, M., Miyamoto, T., Sawatani, Y., Iwamoto, K., Hosogane, N., Fujita, N., et al. (2005). DC-STAMP Is Essential for Cell-Cell Fusion in Osteoclasts and Foreign Body Giant Cells. J. Exp. Med. 202 (3), 345–351. doi:10.1084/jem.20050645
Yan, Z., Zhang, J., Holt, J., Stewart, G., Niewiarowski, S., and Poncz, M. (1994). Structural Requirements of Platelet Chemokines for Neutrophil Activation. Blood 84 (7), 2329–2339. doi:10.1182/blood.v84.7.2329.2329
Keywords: DC-STAMP, acute myeloid leukemia, prognosis, bioinformatics, TCGA, immune checkpoints
Citation: Liang Q, Zhang L, Wang W, Zhao J, Li Q, Pan H, Gao Z, Fang L and Shi J (2022) High Expression of DC-STAMP Gene Predicts Adverse Outcomes in AML. Front. Genet. 13:876689. doi: 10.3389/fgene.2022.876689
Received: 15 February 2022; Accepted: 21 March 2022;
Published: 27 April 2022.
Edited by:
Ayan Biswas, University of Alabama at Birmingham, United StatesReviewed by:
Ezhilarasi Chendamarai, Washington University in St. Louis, United StatesJing-Dong Zhou, Jiangsu University Affiliated People’s Hospital, China
Copyright © 2022 Liang, Zhang, Wang, Zhao, Li, Pan, Gao, Fang and Shi. This is an open-access article distributed under the terms of the Creative Commons Attribution License (CC BY). The use, distribution or reproduction in other forums is permitted, provided the original author(s) and the copyright owner(s) are credited and that the original publication in this journal is cited, in accordance with accepted academic practice. No use, distribution or reproduction is permitted which does not comply with these terms.
*Correspondence: Jun Shi, c2hpanVuQGloY2Ftcy5hYy5jbg==, b3JjaWQub3JnLzAwMDAtMDAwMi04NTMxLTA0ODM=