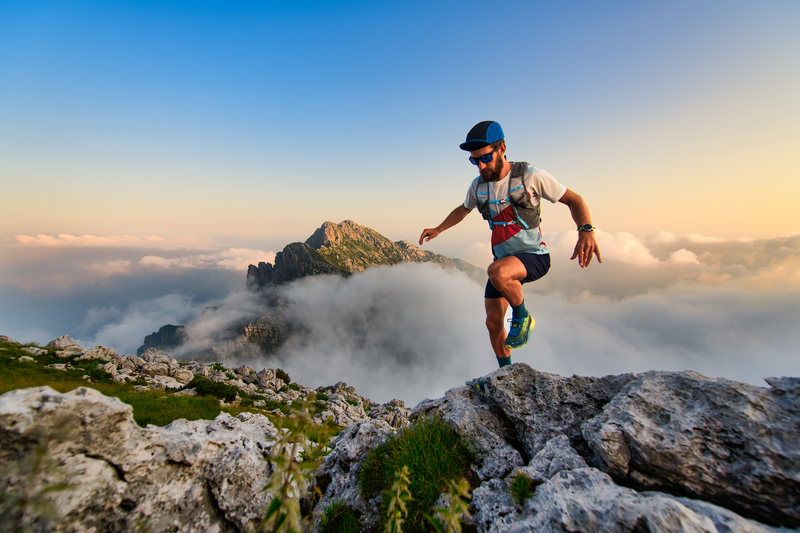
95% of researchers rate our articles as excellent or good
Learn more about the work of our research integrity team to safeguard the quality of each article we publish.
Find out more
ORIGINAL RESEARCH article
Front. Genet. , 14 April 2022
Sec. Genomics of Plants and the Phytoecosystem
Volume 13 - 2022 | https://doi.org/10.3389/fgene.2022.876482
There has been no systematic identification and screening of candidate reference genes for normalization of quantitative real-time PCR (qRT-PCR) results in Rhododendron delavayi to date. Therefore, the present study used GAPDH, Act, EF1, Tub-, Tub-5, UEC1, TATA, TATA-2, UEP, TIP41, and Ubiquitin to predict their stabilities on different aboveground tissues (matured leaves (ML), stem tips (STM), and flower buds (FB)) at different developmental stages (young and adult plants) using five statistical algorithms: Delta Ct method, BestKeeper, geNorm, Normfinder, and RefFinder. The findings were confirmed using ML obtained from plants that had been stressed by drought. By using RefFinder with ML samples collected under drought conditions, it was determined that the top five most stable reference genes were GAPDH > UEC1 > Actin > Tubulin- > Tubulin—5, whereas the least stable reference gene was Ubiquitin. In addition, under control conditions, UEC1, UEC2, Actin, and GAPDH were selected as the highest stable potential reference genes at the juvenile stage of R. delavayi with ML and STM. When ML and STM were combined with drought-stressed samples, TIP41, GAPDH, or their combination proved to be the most effective qRT-PCR primers. The findings will aid in the improvement of the precision and reliability of qRT-PCR data and laying the groundwork for future gene functional studies in R. delavayi.
Rhododendron delavayi Franch possesses very attractive flowers and good resistance to arid and cold climates contributing to a high demand in the Chinese market and other Southeastern Asian countries, such as Burma, India, Thailand, and Vietnam (Zhang et al., 2017). It has medicinal properties such as antioxidant, anti-inflammatory, anticarcinogenic, and antibacterial properties making it a suitable candidate for the treatment of diabetes, arthritis, headache, and hypertension (Cao et al., 2004; Qiang et al., 2011; Zhou et al., 2014). R. delavayi is widely distributed and cultivated throughout southwest China and grows at a wide altitudinal range between 1,200 and 3,200 m (Ma, 2015; Zhang et al., 2017).
The draft genome of R. delavayi was published in 2017 by Zhang et al. (2017), and since then, a number of molecular studies have been conducted on this species (Cai et al., 2019; Sun et al., 2021). For instance, Sun et al. (2021) functionally characterized dihydroflavonol4-reductase–designated RdDFR1 and revealed its involvement in flower color formation in R. delavayi. In addition, Cai et al. (2019) performed physiological and transcriptomic analyses to identify key metabolic pathways involved in drought tolerance in R. delavayi and further conducted quantitative reverse transcription polymerase chain reaction (qRT-PCR) with ten differentially expressed genes detected from the prominent Kyoto Encyclopedia of Genes and Genomes (KEGG) enriched pathways.
Omics, such as genomics and transcriptomics, and their application have been essential in revealing several key genes and metabolic pathways modulating numerous traits of agronomic and medicinal importance and aiding marker-assisted breeding in crop species (Channale et al., 2021; Cui et al., 2021; Maritim et al., 2021; Xiao et al., 2021; Zenda et al., 2021). One of the requisites for genomics and transcriptomics is qRT-PCR which measures changes in gene expression (Phillips et al., 2009; Köhsler et al., 2020). The prominence of qRT-PCR is due to its precision, accuracy, convenience, speed, and sensitivity (Wang et al., 2016). However, qRT-PCR requires a housekeeping/reference gene to act as an internal control to normalize the expression level of target genes (Bustin et al., 2009; Gao et al., 2017). Preferred housekeeping genes must be stably expressed in organisms under varied biotic and abiotic conditions (Zhu et al., 2014) and in different tissues (Janská et al., 2013) but not affected by the stage of development of the organism (Huis et al., 2010) and endogenous or exogenous factors (Janská et al., 2013).
The previously mentioned background suggests the need to screen and select appropriate reference gene(s) to accelerate the breeding process in R. delavayi. A number of reference genes for qRT-PCR have been reported to include glyceraldehyde-3-phosphate dehydrogenase (GAPDH), actin (Act), ribosomal protein S3 (RPS3), elongation factor 1-alpha (EF1α), tubulin-beta (Tub-β), TATA binding protein (TATA), Ubiquitin-conjugating enzymes E2-28 and E2-32 (UEC1 and UEC2, respectively), and many others (Fan et al., 2013; Gao et al., 2017; Jin et al., 2019; Yang et al., 2019; Li et al., 2021). To the best of our knowledge, there has not been any report on the selection and validation of reference genes in R. delavayi; therefore, the present study used eleven well-known reference genes: GAPDH, Act, EF1α, Tub-β, Tub-β5, UEC1, UEC2, TATA, UEP (Ubiquitin domain–containing protein), TIP41 (TIP41-like family protein), and Ubiquitin (Ubiquitin-40S ribosomal protein S27a) to profile their expression in different tissues at varied developmental stages under control and drought conditions. In addition, we utilized a well-known gene– 9-cis-expoxycarotenoid dioxygenase1 (NCED1) involved in water stress (Changan et al., 2018) to study their stabilities under drought conditions either individually or combined tissues with five statistical algorithms: Delta Ct method (Silver et al., 2006), BestKeeper (Pfaffl et al., 2004), geNorm (Vandesompele et al., 2002), Normfinder (Andersen et al., 2004), and RefFinder (Xie et al., 2012). The results would provide valuable information and lay the foundation for further functional validation of gene expression by qRT-PCR and biosynthetic pathway and metabolic engineering in R. delavayi.
This study utilized 3-year-old potted, healthy young plants of R. delavayi that had not reached reproductive growth stage as young plants (Figure 1A). The young plants were well-watered during the study and tissues: matured leaves (ML) and stem tips (STM) were sampled on the sampling dates presented in Table 1. On the other hand, the adult plants of R. delavayi were used in this study in the Kunming Jindian Scenic Area (Figure 1B), 1980 m above sea level, longitude and latitude of 102°43′ 5.99″E and 25°02′ 20.00″N, respectively, and tissues such as ML and flower bud (FB) were sampled at the dates given in Table 1.
FIGURE 1. Samples of R. delavayi plants used in this study. (A) Three-year-old potted, healthy young plants of R. delavayi that had not reached the reproductive growth stage. (B) Adult plants of R. delavayi were used in this study in the Kunming Jindian Scenic Area. (C-G). Young plants under 9, 10, 11, 12, and 13 days drought stress, respectively, with severe leaf wilting at 11th, 12th, and 13th day of stress. (H) Fully recovered plants after rewatering from 13 days of drought stress.
To validate the expression of the selected candidate reference genes, some of the young plants of R. delavayi received no water for 9, 10, 11, 12, and 13 days during the study (Figures 1C–G, respectively). After the 13th day drought treatment, the plants were well-watered, leading to full recovery as evidenced in Figure 1H. MLs were sampled from the recovered plant at periods shown in Table 1. Specifically, tissue sampling was carried out sequentially simultaneously each day (10:30–11:00 a.m.), once per day for four consecutive days with three replicates.
Total RNA was extracted following the manufacturer’s instructions with the RNAprep Pure Plant Kit (TianGen, Gen). The total RNA integrity was assessed via electrophoresis with 1% agarose gel. The quality and concentrations of RNA were measured by using a NanoDrop 2000 Spectrophotometer (Thermo Scientific Wilmington, DE, United States). First-strand cDNA was synthesized by reverse transcription of 300 ng total RNA with PrimeScript II First Strand cDNA Synthesis Kit Mix (Biotek, Beijing, China) with oligo (dT) primers in a final volume of 20 μL following the manufacturer’s protocols. All the cDNA samples were stored at −20°C for later use.
The full length sequences of eleven candidate housekeeping genes used in several previous studies in other crop species were used to blast R. delavayi’s whole-genome sequencing data released by Zhang et al. (2017) through nucleotide blast option (BlastN) using BioEdit software (Hall, 1999). Gene-specific primers for qRT-PCR analysis were designed using Primer Premier 6 standalone version (www.premierbiosoft.com), according to the sequences of the 11 candidate reference genes obtained from the R. delavayi genome (Table 2). The primers were synthesized by Tsingke Biotechnology Co., Ltd (Beijing, China).
TABLE 2. Details of primer pairs used in real-time fluorescence quantitative PCR for candidate genes and validation of genes in R. delavayi.
To detect the specificity and amplification efficiency of each pair of primers developed, we conducted RT-PCR in a 20-μl system using a Mastercycler nexus GSXI PCR apparatus (Eppendorf AG, Hamburg, Germany) following the manufacturer’s instructions. Thus, 60 ng of the synthesized cDNA, 2 μl rTaq buffer (TakaRa, Dalian, China), 0.4 μM of two primer pairs equally and thoroughly mixed, 200 μM each dNTP, and 1 U rTaq (TaKaRa, Dalian, China) were used. The amplification program and product evaluation were conducted following the conditions outlined by Jin et al. (2019). The dissociation curve was generated by melting the amplicons from 60–95°C.
All qRT-PCR experiments were performed in three biological replicates and three technical replicates. A no template control and reverse transcription negative control were also performed. The relative gene expression levels were computed using the 2-∆∆Ct method (Livak and Schmittgen, 2001).
Five tools– Delta Ct method (Silver et al., 2006), BestKeeper (Pfaffl et al., 2004), geNorm (Vandesompele et al., 2002), Normfinder (Andersen et al., 2004), and RefFinder (Xie et al., 2012) were used to compute the stabilities of the individual genes. Briefly, the Delta CT method was used to select the optimal reference gene by comparing the relative expression of “pairs of genes” within each sample (Silver et al., 2006). BestKeeper works with ranking of candidate reference genes based on standard deviations and coefficients of variation (CVs) (Pfaffl et al., 2004). geNorm was used to compute the normalization factor to determine the optimal number of reference genes following the pairwise variation (V) between different candidate genes, thus Vn/Vn+1. When the ratio is less than 0.15, it implies that the number of internal reference gene combinations in this group can maintain the accurate normalization of the data to some extent (Vandesompele et al., 2002). The Normfinder algorithm is a model-based approach which ranks the candidate reference genes by the stability value, of which the gene with the lowest value represents the most stable reference gene (Andersen et al., 2004). Last, the RefFinder (https://www.heartcure.com.au/reffinder/?type=reference accessed on 22/12/2021) assigns an appropriate weight of the four methods elaborated previously to a single gene and computes the geometric average of their weights for the overall final ranking (Xie et al., 2012).
The NCED1 copy in the R. delavayi genome (DUH017412.1), which catalyzes oxidative cleavage of 9-cis-epoxycarotenoids neoxanthin and violaxanthin to xanthoxin, is a key step in the biosynthesis of abscisic acid (ABA) in higher plants (Changan et al., 2018). The most and least stable reference gene and the combination of the two–three best reference genes were used to normalize the expression profile of DUH017412.1 in the tissues sampled at a young stage with ML. The relative expression was conducted with the comparative 2-∆∆Ct method (Livak and Schmittgen, 2001).
Data obtained via qRT-PCR were subjected to analysis of variance (ANOVA), and post hoc mean separation was carried out by Duncan’s Multiple Range Test at p < 0.05 using GenStat software (Version 12; https://www.vsni.co.uk/). Means were visualized via GraphPadPrism (Version 7; https://www.graphpad.com/). Transcriptome data of the candidate reference genes and NCED1 were log2 transformed and heat-mapped with the pheatmap package in R (Kolde, 2012).
We blasted the genome of R. delavayi earlier published by Zhang et al. (2017) with 11 candidate reference genes listed in Tables 2–3. The melting curves of the 11 candidate reference genes showed that all primer pairs designed present a single peak (Supplementary Figure S1), which validates the specificity of primers. The amplicon lengths of the 11 candidate reference genes ranged from 103 bp in Tubulin-β-5 to 257 bp in TIP41 (Table 3, Supplementary Figure S2). On the other hand, the minimum (90.30%) and maximum (105.60%) amplification efficiency was observed in UEC1 and Actin, respectively (Table 3). Strong correlation coefficients (R = 1) were recorded for the eleven candidate reference genes. The linear equation predicted based on Ct for the primers’ specificity is shown in Table 3. From this background, the primers were used for further experiments aimed at screening the 11 candidate reference genes along with one reference gene (NCED1) for their stabilities.
TABLE 3. Primers used in this study and their amplification efficiency and characteristics for eleven candidate reference genes in R. delavayi.
The transcript abundance of the eleven candidate reference genes in matured leaves (ML) and stem tips (STM) under control and stress conditions sampled from young R. delavayi and ML and flower buds (FB) from adult R. delavayi were determined by Ct values from qRT-PCR experiments (Figures 2A–E). The results revealed that the candidate reference genes showed a wide range of transcription level across all the test samples under control and drought stress conditions at different sampling times (Figures 3A–E). The ML sample from young R. delavayi had transcript abundance ranging from 21.09 in GAPDH to 27.12 in Tubulin-β-5 (Figure 2A). Consistently, GAPDH had the least Ct values across the seven sampling times in ML sampled from young R. delavayi, while TIP41 had the highest Ct values from ML1–ML4; the remaining three sampling times (ML5–ML7) had Tubulin-β-5 recording the highest Ct values (Figure 2A). In the case of the STM, GAPDH and TIP41 had the least (20.16–20.79) and maximum (26.33–26.68) Ct values, respectively (Figure 2B).
FIGURE 2. Threshold cycle (Ct) of the 11 candidate reference genes in young and adult R. delavayi. (A) Young matured leaves (ML) (sampled at 9/22/2017, 10/8/2017, 11/8/2017, 12/8/2017, 1/8/2018, 1/23/2018, and 2/8/2018 denoted ML1, ML2, ML3 ML4, ML5, ML6, and ML7, respectively). (B) Stem tips sampled from young plant (at 9/22/2017, 10/8/2017, and 11/8/2017 denoted STM-1, STM-2, and STM-3, respectively). (C) Drought stress samples (sampled at 9, 10, 11, 12, and 13 days after drought stress (DS) condition denoted B1-9, B1-10, B1-11, B1-12, and B1-13, respectively). (D) Adult ML (sampled at 9/22/2017, 10/8/2017, 12/8/2017, 1/8/2018, 1/23/2018, 2/8/2018, and 2/26/2018 denoted ML8, ML9, ML11, ML12, ML13, ML14, and ML15, respectively). (E) Flower bud (FB) (sampled at 9/22/2017, 10/8/2017, 12/8/2017, 1/8/2018, 1/23/2018, 2/8/2018, and 2/26/2018 denoted FB8, FB9, FB11, FB12, FB13, FB14, and FB15, respectively).
FIGURE 3. Threshold cycle (Ct) of the 11 candidate reference genes in the aboveground parts of young and matured R. delavayi plant. (A) Young plant (matured leaves (ML) + stem tips (STM) + under drought stress (DS) condition. (B) Matured plant (ML + STM + flower buds (FB)). Average of the 2 years (2017 and 2018 sampling periods). Lower and upper lines on the box represent the minimum and maximum Ct, respectively. The lower and upper parts of the box represent 25 and 75% percentile, respectively, and the line in the middle of the box represents mean Cq for each gene. Boxes with a common alphabet indicate no significant difference (p > 0.05), while those with no common alphabet indicate significant difference (p < 0.05) with Post hoc mean separation by Duncan’s Multiple Range Test.
Under drought stress condition, GAPDH had the least Ct values (20.88–22.44), while Tubulin-β-5 exhibited the highest Ct values (32.24–33.29) in the ML sampled from young R. delavayi (Figure 2C). This suggests that environmental factors such as drought could alter the transcript abundance of candidate reference genes, indicating the relevance of our study.
In addition to the previously mentioned data, we sampled ML and FB from adult R. delavayi to evaluate the expression of the eleven candidate reference genes via Ct values. With ML from adult plants, Tubulin-β-5 had the highest Ct values (28.71–31.40), while either Ubiquitin and GAPDH or UEP had the least Ct values (20.58–23.77) across the eight sampling times (Figure 2D). A similar trend was observed in FB as in the ML, but the highest Ct values were observed with either Tubulin-β-5 or TIP41 (Figure 2E).
We further pooled the Ct values recorded previously and treated each as a replicate for each of the candidate reference genes and subjected to ANOVA. It was observed that significant variation existed among the tissues from young (plus under drought stress) and adult R. delavayi (Figures 3A,B). The Ct values of Tubulin-β-5 had transcript abundance in the young R. delavayi under control and drought stress with wide variation in the range and mean ± standard deviation (20.52–33.29 and 27.32 ± 4.48), which statistically differed from TIP41 (25.23–28.20 and 26.70 ± 0.90) and TATA (24.67–29.26 and 26.96 ± 1.80), while the mean Ct values recorded by GAPDH (20.96 ± 0.84) and Ubiquitin (22.53 ± 1.06) were statistically similar (Figure 3A). With exception of TATA, Tubulin-β-5 and TIP41 and GAPDH (20.96 ± 0.84) and Ubiquitin in adult R. delavayi followed similar statistical order as observed in young R. delavayi (Figure 3B).
In order to give credence to the transcript abundance assessed by Ct values for the eleven candidate reference genes and one reference gene (NCED1), we retrieved gene expression based on fragments per kilobase of transcript per million (FPKM) mapped reads from two project data available on the NCBI with accession numbers: PRJNA476831 (dormant bud) and PRJNA503304 (drought) (Cai et al., 2019). The FPKM was log2-transformed and heat-mapped with the Pheatmap package in R (Kolde, 2012). It was observed that Actin, EF1α, Ubiquitin, and UEP consistently exhibited the highest FPKM across the dormant buds, while the least FPKM was observed with NCED1 (Figure 4A).
FIGURE 4. Gene (eleven candidate reference genes and NCDE1) expression based fragments per kilobase of transcript per million mapped reads (log2 transformation) in R. delavayi. (A) Dormant buds from the study with accession SRA = PRJNA476831 (available at https://www.ncbi.nlm.nih.gov/bioproject/476831), pre-dormancy (Bud-pre, 07/20/2015), para-dormancy (Bud-para, 10/29/2015), endo-dormancy (Bud-endo, 12/02/2015), eco-dormancy (Bud-eco, 12/27/2015), and dormancy-release (Bud-rele, 01/14/2016)). (B) Drought stress condition from the study of Cai et al. (2019) (SRA = PRJNA503304) (four experimental scenarios were designed: normal irrigation (WW), stopping irrigation for 5 days (WS), stopping irrigation for 9 days (SS), and re-watering for 6 h (REC) after 10 days of drought. Rewatering was only carried out on 10 plants. The other five plants were continued under no irrigation for subsequent electron microscopic observation. All the measurements were carried out at the end of each timepoint).
Under drought conditions, Actin, EF1α, and Ubiquitin persistently had the highest transcript abundances under the five conditions evaluated under drought treatments: normal irrigation (WW), stopping irrigation for 5 days (WS), stopping irrigation for 9 days (SS), stopping irrigation for 10 days, and re-watering for 6 h (REC) after 10 days of drought, whereas the reference gene (NCED1) had the transcript abundance in the order of SS > WS > WW > RC. This trend gives credence to our use of NCED1 as the reference gene to validate our results under drought.
Under drought condition, the best five stable candidate reference genes followed the order GAPDH > UEC1 > Actin > Tubulin-β > Tubulin-β-5 and were predicted by the Ct method for ML (Table 4), whereas the BestKeeper method predicted TATA, UEC2, TIP41, and Tubulin-β-5 as the four most stable candidate reference genes. The geNorm predicted Actin together with UEC1 genes as the most stable followed by GAPDH > UEC1 > Actin > Tubulin-β (Table 4). The GAPDH followed by UEC1, Tubulin-β > Tubulin-β-5, and Actin were predicted as the best five stable candidate reference genes by Normfinder. By integrating the results from Ct, BestKeeper, geNorm, and Normfinder, the RefFinder predicted GAPDH > UEC1 > Actin > Tubulin-β-5 as the four most stable candidate reference genes under drought stress condition with ML as the tissue. Consistently among the five tools used for stability analysis of the candidate reference genes, Ubiquitin was among the last two most stable genes (Table 4).
TABLE 4. Stability ranking of 11 candidate reference genes with matured leaf sampled from a 3-year-old young R. delavayi under drought stress.
The use of young R. delavayi with both ML and STM under control and ML from drought stress condition was considered, and the Ct method predicted UEC1, Actin, UEC2, and GAPDH as the four most stable candidate reference genes (Table 5). In addition, GAPDH, TIP41, Ubiquitin, and UEP were predicted as the best candidate reference genes by the BestKeeper method, while the geNorm method predicted UEC2+TATA as the best candidate reference gene followed by UEC1 > Actin > EF1α. Normfinder predicted that UEC1 > Actin > UEC1>EF1α as the four stable genes. The results from RefFinder prediction for the best four candidate reference genes followed similarly with the Ct method with minor rearrangement. From the five models, Tubulin-β-5 was ranked the least stable which is consistent with Figure 4A, suggesting that the wider the range of Ct values, the lesser the stability will be.
TABLE 5. Stability ranking of 11 candidate reference genes with tissues (matured leaf and stem) and under drought conditions (matured leaf) from young R. delavayi.
TIP41 was predicted as the most stable candidate reference gene in the tissues of adult R. delavayi (ML + FB) followed UEP, UEC1, and GAPDH by the Ct method (Table 6). Conversely, the BestKeeper method classified EF1α, UCE2, GAPDH, and UEP as the top four stable candidate reference genes, but in the case of geNorm, GAPDH + UEP, TIP41, Ubiquitin, and UEC2 were considered the best four candidate reference genes. The first four best candidate reference genes predicted by Normfinder followed a similar trend as that of the Ct method (Table 5). A comprehensive analysis by RefFinder revealed UEP, TIP41, GAPDH, and EF1α as the best candidate reference genes (Table 6).
TABLE 6. Stability ranking of 11 candidate reference genes with tissues (matured leaf and flower bud) sampled from adult R. delavayi.
qRT-PCR is usually conducted on different tissues at varied developmental stages and conditions (control and stressed); hence, we pooled the Ct values together to predict their stability in both young (control-ML + STM and under DS) and adult (ML + FB) R. delavayi (Table 7). The Ct method predicted TIP41 as the most stable candidate reference gene followed by Actin, UEC1, and GAPDH. Equally, GAPDH, EF1α, TIP41, and UEC2 were predicted as the best four candidate reference genes by the BestKeeper method (Table 6). geNorm recommended GAPDH + TIP41 as the best gene pair followed by UEC1, Actin, and EF1α, whereas Normfinder predicted Actin, TIP41, UEC1, and GAPDH as the best four candidate reference genes (Table 7). The five statistical algorithms ranked Tubulin-β-5 as the most unstable candidate reference gene, suggesting that the higher the average Ct values, the poorer the stability will be.
TABLE 7. Stability ranking of 11 candidate reference genes with tissues from young (matured leaf and stem) and under drought (matured leaf) and adult (matured leaf and flower bud) of R. delavayi.
In order to evaluate the reliability of candidate reference genes, we selected two most stable (Actin and UEC1) and most unstable (UEC2 and Ubiquitin) either individually or in combination from the geNorm model and normalized their Ct values with NCED1 to obtain their relative expression level in matured leaf samples at five sampling times from young R. delavayi (Figure 5). Mostly, the expression patterns of most stable and unstable genes were similar with minor discrepancies. For example, at B1-9 sampling time, either one or a combination of the most unstable candidate reference genes (UEC2 and Ubiquitin) had significantly different relative expressions, of which either was statistically higher and different from the two most stable (Actin and UEC1) (Figure 5). However, contrary trends were observed at B1-10. As evidenced by standard deviation at each sampling time, UEC2 and Ubiquitin, either individually or in combination, were unstable when used, confirming the results of the geNorm model under drought condition (Table 4). This result highlights the need to select a suitable reference gene for gene expression studies.
FIGURE 5. Relative expression of selected genes: most stable (Actin + UEC1) and most unstable (UEC2 + TIP41) relative to the reference gene (NCED1) under drought stress condition of matured leaves of young R. delavayi plant. Sampling times consisted of 9, 10, 11, 12, and 13 days of drought stress (no watering) condition denoted B1-9, B1-10, B1-11, B1-12, and B1-13, respectively. The error bars represent standard deviations computed on three replications. Different letters of the same colors of the lines represent significant difference (p < 0.05) at each time point based on Duncan’s Multiple Range Test.
Though the draft genome of R. delavayi was announced in 2017 by Zhang et al. (2017), there has been no report on screening reference genes for qRT-PCR experiments, unlike other crops such as soybean (Gao et al., 2017), barley (Janská et al., 2013), flax (Huis et al., 2010), bamboo (Fan et al., 2013), rice (Almas and Kamrodi, 2018), and tomato (Cheng et al., 2017). Gene expression analysis by qRT-PCR in plants of economic, nutritional, and health importance has gained increasing attention in recent years (Vandesompele et al., 2002; Almas and Kamrodi, 2018; Ahiakpa et al., 2021; Sun et al., 2021; Xiao et al., 2021) as a result of its high sensitivity, quantitative accuracy, low cost, and high throughput (Bustin, 2002; Radonić et al., 2004). One of the determinants of successful and meaningful qRT-PCR operation is the selection and reliability of reference genes which function to normalize the qRT-PCR experiment to ensure that results are both statistically significant and biologically meaningful (González-Aguilera et al., 2016).
To screen and select a number of candidate genes for functional validation in R. delavayi via gene overexpression, virus-induced gene silencing (VIGS) and clustered regularly interspaced short palindromic repeats (CRISPR)/CRISPR-associated protein (Cas) (CRSIPR/Cas) technology (Zhang et al., 2022) from our transcriptomics dataset were carried out, and our research group attempted to validate the expression profile of RNA-sequencing (RNA-seq) data by qRT-PCR; however, the challenge of the reference gene was eminent; hence, we decided to screen 11 known reference genes reported in other crops (Fan et al., 2013; Gao et al., 2017; Yang et al., 2019; Jin et al., 2019). First, we blasted the eleven known reference genes with the draft genome of R. delavayi and designed primer pairs for each of the genes (Table 2). One of the requirements for primer pairs for qRT-PCR is the specificity of the primers for a specific gene (David et al., 2017; Bustin and Huggett, 2017). Second, we performed primer-specificity experiments for the 11 candidate reference genes via melting curve and analyzed amplification efficiencies and correlation coefficients which had single peak (Supplementary Figure S1), 90.30–105.60% and 0.993–0.998 (Table 3), respectively. These primer specificity results revealed that all 11 pairs of primers meet the criteria for qRT-PCR experiments (Gao et al., 2017).
We subsequently screened the 11 candidate reference genes in matured leaves, stem tips, and flower buds at two developmental stages (young and adult) (Figures 2A,B, d–e). It was observed that the 11 candidate reference genes have varied expression values (Ct) in different tissues and developmental stages. For instance, GAPDH was among the genes with least transcript abundance in all the tissues at the two different developmental stages (Figures 2A,B, d–e; Figures 3A,B). This according to Gao et al. (2017) suggests that the cDNA sample with more abundance reaches the threshold at lower Ct value, which leads to a higher gene expression level. The wide variation in Ct values is consistent with studies in Camellia sinensis and Brassica napus. Thus, the expression of the housekeeping genes varies considerably under different conditions and in different tissues (Hao et al., 2014; Yang et al., 2014).
R. delavayi is well known as an alpine evergreen ornamental plant, in which water shortage impedes its growth and development in urban gardens (Cai et al., 2019). With this in mind, our research group has initiated studies to unravel the molecular mechanism underlying drought tolerance/susceptibility in R. delavayi with the aim of developing more drought-resilient genotypes. Therefore, the eleven candidate reference genes were screened for their stability under drought stress. GAPDH is a multifunctional enzyme that plays an important role in abiotic stress including drought and plant development (Zhang et al., 2019). Consistently, across the five sampling times (B1–9 to B1–13), GAPDH had the least Ct value suggesting its highest gene expression among the eleven candidate reference genes. The study of Zhang et al. (2019) explained that overexpression of GAPDH encoded the gene TaGAPC1 to enhance drought tolerance in transgenic Arabidopsis; therefore, we speculate that GAPDH (DUH011791.1) could be the candidate gene for drought tolerance in R. delavayi, as it also showed higher transcript abundance under drought stress (stopping irrigation for 9 days, SS) in the RNA-seq of Cai et al. (2019) (Figure 4B). A similar observation was made in maize and Arabidopsis (Russell and Sachs, 1992; Yang et al., 1993) under polyethylene glycol, hydrogen peroxide, and abscisic acid stress.
It is established that the ideal reference gene should exhibit a consistent expression level across all tested tissues or conditions (Bustin, 2002; Udvardi et al., 2008). To establish stability in the expression of the eleven candidate reference genes, we applied five statistical algorithms, of which RefFinder is a user-friendly web-based comprehensive tool developed for evaluating and screening reference genes from extensive experimental datasets (Xie et al., 2012) and integrates the four other computational programs (Delta Ct method (Silver et al., 2006)– BestKeeper (Pfaffl et al., 2004), geNorm (Vandesompele et al., 2002), and Normfinder (Andersen et al., 2004)). The ranking values of these programs indicate the most stable (least value), moderately stable (average), and least stable (highest value). Under drought condition with matured leaves as tissue, the five programs largely predicted GAPDH as the most stable reference gene together with UEC1 and Actin, while the least was Ubiquitin (Table 4). These corroborate findings by Nicot et al. (2005), Garg et al. (2010), Li et al. (2012), and Müller et al. (2015). The essential roles these genes play in basic cellular progress may have accounted for their stable expression. However, Mao et al. (2021) reported that GAPDH had the least stability in pineapple (Ananas comosus var bracteatus) in response to hormone stimuli. The further validation of our results with two of the most stable candidate reference genes (UEC1 and Actin) and two least stable genes (UEC2 and TIP41), which were normalized by one well-known gene under drought, showed that NCDE1 was consistent with our stability analysis (Table 4; Figure 5). This indicates that RNA-seq data from other projects provide clues on the expression of genes in tissues and conditions for their inclusion in qRT-PCR experiments. However, there is a need to conduct optimization experiments to confirm and select ideal reference genes for qRT-PCR.
On the contrary, RefFinder ranked UEC1, UEC2, Actin, and GAPDH as the topmost stable candidate reference genes at the young stage of R. delavayi with matured leaves and stem tips under control and drought stress conditions (matured leaves) and Tubulin-β-5 as the least stable (Table 5). This indicates that no reference gene is adequate in all experimental settings and tissues and in all stages of development (Mao et al., 2021), which suggests that reference genes should be thoroughly evaluated prior to specific experimental conditions and use (Cheng et al., 2017). The ranking of Tubulin-β-5 partly corroborates this finding, while GAPDH contrasts with the findings of Li et al. (2020).
TIP41 has been considered a stable internal control gene for Caragana intermedia (Zhu et al., 2013) and in tall fescus (Festuca arundinacea Schreb) under several abiotic stresses such as salinity, drought, cold, and heat (Yang et al., 2015). This gene (TIP41) together with GAPDH was stably ranked among the topmost four potential reference genes by the five statistical models (Vandesompele et al., 2002; Andersen et al., 2004; Pfaffl et al., 2004; Silver et al., 2006; Xie et al., 2012) (Table 6-7) with matured leaves and flower buds and young (+drought stress) and adult combined from R. delavayi. Thus, either TIP41 or GAPDH/their combination could be exploited for qRT-PCR experiments in R. delavayi. However, the criteria for the ranking among potential reference genes largely remain unknown; whether the differences are caused by the genes themselves or the difference caused by the specific amplified fragments that affect the amplification experiment warrants further investigations. In addition, future studies should include the use of several tissues such as root from drought and other abiotic stresses conditions to study the stabilities of the eleven and other novel candidate reference genes.
The present study represents the first systematic identification and screening of potential reference genes as internal controls for normalization of qRT-PCR results in R. delavayi varied above-ground tissues under drought stress conditions. From our results, GAPDH (DUH011791.1) could be recommended as a reference gene for drought stress experiments using developed leaves as the experimental tissue. Furthermore, under control conditions, the topmost stable candidate reference genes at the young stage of R. delavayi with developed leaves and stem tips were anticipated to be UEC1, UEC2, Actin, and GAPDH, with UEC1 expected to be the most stable candidate reference gene. qRT-PCR experiments in R. delavayi could be improved by combining young and adult organs. TIP41 or GAPDH/their combination could be further validated and used for qRT-PCR experiments in R. delavayi. The findings presented here not only identify the most appropriate reference genes for qRT-PCR analysis in R. delavayi, but they also provide referable guidelines for other plant species that do not have documented or validated reference genes for qRT-PCR analysis.
The datasets presented in this study can be found in online repositories. The names of the repository/repositories and accession number(s) can be found in the article/Supplementary Material.
Conceptualization: LZ and JW; methodology: GD; software: YC; validation: MZ and YC; formal analysis: LZ and YC; investigation: MZ; resources, GD; writing—original draft preparation: LZ and YC; writing—review and editing: JW and GD; supervision: JW; project administration and funding acquisition: LZ. All authors have read and agreed to the published version of the manuscript.
This research was financially supported by the National Natural Science Foundation of China (31760231), the Science and Technology Talent and Platform Program of Yunnan Province (202105AC160017), and the Construction of International Flower Technology Innovation Center and Industrialization of Achievements (2019ZG006).
The authors declare that the research was conducted in the absence of any commercial or financial relationships that could be construed as a potential conflict of interest.
All claims expressed in this article are solely those of the authors and do not necessarily represent those of their affiliated organizations, or those of the publisher, the editors, and the reviewers. Any product that may be evaluated in this article, or claim that may be made by its manufacturer, is not guaranteed or endorsed by the publisher.
The Supplementary Material for this article can be found online at: https://www.frontiersin.org/articles/10.3389/fgene.2022.876482/full#supplementary-material
Ahiakpa, J. K., Magdy, M., Karikari, B., Munir, S., Mumtaz, M. A., Tamim, S. A., et al. (2021). Genome-Wide Identification and Expression Profiling of Tomato Invertase Genes Indicate Their Response to Stress and Phytohormones. J. Plant Growth Regul., 1–18. doi:10.1007/s00344-021-10384-5
Almas, D. E., and Kamrodi, A. R. (2018). Validation of Appropriate Reference Genes for Real-Time Quantitative PCR Gene Expression Analysis in Rice Plants Exposed to Metal Stresses. Russ. J. Plant Physiol. 65, 890–897. doi:10.1134/S102144371806002X
Andersen, C. L., Jensen, J. L., and Ørntoft, T. F. (2004). Normalization of Real-Time Quantitative Reverse Transcription-PCR Data: a Model-Based Variance Estimation Approach to Identify Genes Suited for Normalization, Applied to Bladder and colon Cancer Data Sets. Cancer Res. 64, 5245–5250. doi:10.1158/0008-5472.can-04-0496
Bustin, S., and Huggett, J. (2017). qPCR Primer Design Revisited. Biomol. Detect. Quant. 14, 19–28. doi:10.1016/j.bdq.2017.11.001
Bustin, S. A., Benes, V., Garson, J. A., Hellemans, J., Huggett, J., Kubista, M., et al. (2009). The MIQE Guidelines: Minimum Information for Publication of Quantitative Real-Time PCR Experiments. Clin. Chem. 55, 611–622. doi:10.1373/clinchem.2008.112797
Bustin, S. (2002). Quantification of mRNA Using Real-Time Reverse Transcription PCR (RT-PCR): Trends and Problems. J. Mol. Endocrinol. 29, 23–39. doi:10.1677/jme.0.0290023
Cai, Y.-F., Wang, J.-H., Zhang, L., Song, J., Peng, L.-C., and Zhang, S.-B. (2019). Physiological and Transcriptomic Analysis Highlight Key Metabolic Pathways in Relation to Drought Tolerance in Rhododendron Delavayi. Physiol. Mol. Biol. Plants 25, 991–1008. doi:10.1007/s12298-019-00685-1
Cao, Y., Chu, Q., and Ye, J. (2004). Chromatographic and Electrophoretic Methods for Pharmaceutically Active Compounds in Rhododendron Dauricum. J. Chromatogr. B 812, 231–240. doi:10.1016/s1570-0232(04)00549-5
Changan, S. S., Ali, K., Kumar, V., Garg, N. K., and Tyagi, A. (2018). Abscisic Acid Biosynthesis under Water Stress: Anomalous Behavior of the 9-Cis-Epoxycarotenoid Dioxygenase1 (NCED1) Gene in rice. Biol. Plant 62, 663–670. doi:10.1007/s10535-018-0807-2
Channale, S., Kalavikatte, D., Thompson, J. P., Kudapa, H., Bajaj, P., Varshney, R. K., et al. (2021). Transcriptome Analysis Reveals Key Genes Associated with Root-Lesion Nematode Pratylenchus Thornei Resistance in Chickpea. Sci. Rep. 11, 17491. doi:10.1038/s41598-021-96906-3
Cheng, Y., Bian, W., Pang, X., Yu, J., Ahammed, G. J., Zhou, G., et al. (2017). Genome-Wide Identification and Evaluation of Reference Genes for Quantitative RT-PCR Analysis during Tomato Fruit Development. Front. Plant Sci. 8, 1440. doi:10.3389/fpls.2017.01440
Cui, X., Deng, J., Huang, C., Tang, X., Li, X., Li, X., et al. (2021). Transcriptomic Analysis of the Anthocyanin Biosynthetic Pathway Reveals the Molecular Mechanism Associated with Purple Color Formation in Dendrobium Nestor. Life 11, 113. doi:10.3390/life11020113
David, F. P. A., Rougemont, J., and Deplancke, B. (2017). GETPrime 2.0: Gene- and Transcript-specific qPCR Primers for 13 Species Including Polymorphisms. Nucleic Acids Res. 45, D56–D60. doi:10.1093/nar/gkw913
Fan, C., Ma, J., Guo, Q., Li, X., Wang, H., and Lu, M. (2013). Selection of Reference Genes for Quantitative Real-Time PCR in Bamboo (Phyllostachys Edulis). PLoS One 8, e56573. doi:10.1371/journal.pone.0056573
Gao, M., Liu, Y., Ma, X., Shuai, Q., Gai, J., and Li, Y. (2017). Evaluation of Reference Genes for Normalization of Gene Expression Using Quantitative RT-PCR under Aluminum, Cadmium, and Heat Stresses in Soybean. PLoS One 12, e0168965. doi:10.1371/journal.pone.0168965
Garg, R., Sahoo, A., Tyagi, A. K., and Jain, M. (2010). Validation of Internal Control Genes for Quantitative Gene Expression Studies in Chickpea (Cicer Arietinum L.). Biochem. Biophys. Res. Commun. 396, 283–288. doi:10.1016/j.bbrc.2010.04.079
González-Aguilera, K. L., Saad, C. F., Chávez Montes, R. A., Alves-Ferreira, M., and de Folter, S. (2016). Selection of Reference Genes for Quantitative Real-Time RT-PCR Studies in Tomato Fruit of the Genotype MT-Rg1. Front. Plant Sci. 7, 1386. doi:10.3389/fpls.2016.01386
Hall, T. A. (1999). BioEdit: A User-Friendly Biological Sequence Alignment Editor and Analysis Program for Windows 95/98/NT. Nucleic Acids Symp. Ser. 41, 95–98.
Hao, X., Horvath, D., Chao, W., Yang, Y., Wang, X., and Xiao, B. (2014). Identification and Evaluation of Reliable Reference Genes for Quantitative Real-Time PCR Analysis in Tea Plant (Camellia Sinensis (L.) O. Kuntze). Int. J. Mol. Sci. 15, 22155–22172. doi:10.3390/ijms151222155
Huis, R., Hawkins, S., and Neutelings, G. (2010). Selection of Reference Genes for Quantitative Gene Expression Normalization in Flax (Linum usitatissimum L.). BMC Plant Biol. 10, 71. doi:10.1186/1471-2229-10-71
Janská, A., Hodek, J., Svoboda, P., Zámečník, J., Prášil, I. T., Vlasáková, E., et al. (2013). The Choice of Reference Gene Set for Assessing Gene Expression in Barley (Hordeum Vulgare L.) under Low Temperature and Drought Stress. Mol. Genet. Genomics 288, 639–649. doi:10.1007/s00438-013-0774-4
Jin, Y., Liu, F., Huang, W., Sun, Q., and Huang, X. (2019). Identification of Reliable Reference Genes for qRT-PCR in the Ephemeral Plant Arabidopsis Pumila Based on Full-Length Transcriptome Data. Sci. Rep. 9, 8408. doi:10.1038/s41598-019-44849-1
Köhsler, M., Leitsch, D., Müller, N., and Walochnik, J. (2020). Validation of Reference Genes for the Normalization of RT-qPCR Gene Expression in Acanthamoeba Spp. Sci. Rep. 10, 10362. doi:10.1038/s41598-020-67035-0
Kolde, R. (2012). Pheatmap: Pretty Heatmaps. R Package Version 1.0.10. Available at: https://CRAN.R-project.org/package=pheatmap (Accessed December 18, 2021).
Li, X.-S., Yang, H.-L., Zhang, D.-Y., Zhang, Y.-M., and Wood, A. J. (2012). Reference Gene Selection in the Desert Plant Eremosparton Songoricum. Int. J. Mol. Sci. 13, 6944–6963. doi:10.3390/ijms13066944
Li, L., Li, N., Fang, H., Qi, X., and Zhou, Y. (2020). Selection and Validation of Reference Genes for Normalisation of Gene Expression in Glehnia Littoralis. Sci. Rep. 10, 7374. doi:10.1038/s41598-020-63917-5
Li, M., Li, X., Wang, C., Li, Q., Zhu, S., Zhang, Y., et al. (2021). Selection and Validation of Reference Genes for qRT-PCR Analysis of Rhopalosiphum Padi (Hemiptera: Aphididae). Front. Physiol. 12, 494. doi:10.3389/fphys.2021.663338
Livak, K. J., and Schmittgen, T. D. (2001). Analysis of Relative Gene Expression Data Using Real-Time Quantitative PCR and the 2−ΔΔCT Method. Methods 25, 402–408. doi:10.1006/meth.2001.1262
Ma, Y. P. (2015). A New Species of Rhododendron (Ericaceae) from Shangri-La. NW Yunnan, China. Phytotaxa 238, 293–297. doi:10.11646/phytotaxa.238.3.10
Mao, M., Xue, Y., He, Y., Zhou, X., Hu, H., Liu, J., et al. (2021). Validation of Reference Genes for Quantitative Real-Time PCR Normalization in Ananas Comosus Var. Bracteatus during Chimeric Leaf Development and Response to Hormone Stimuli. Front. Genet. 12, 2004. doi:10.3389/fgene.2021.716137
Maritim, T. K., Masand, M., Seth, R., and Sharma, R. K. (2021). Transcriptional Analysis Reveals Key Insights into Seasonal Induced Anthocyanin Degradation and Leaf Color Transition in Purple tea (Camellia Sinensis (L.) O. Kuntze). Sci. Rep. 11, 1244. doi:10.1038/s41598-020-80437-4
Müller, O. A., Grau, J., Thieme, S., Prochaska, H., Adlung, N., Sorgatz, A., et al. (2015). Genome-Wide Identification and Validation of Reference Genes in Infected Tomato Leaves for Quantitative RT-PCR Analyses. PLoS ONE 10, e0136499. doi:10.1371/journal.pone.0136499
Nicot, N., Hausman, J.-F., Hoffmann, L., and Evers, D. (2005). Housekeeping Gene Selection for Real-Time RT-PCR Normalization in Potato during Biotic and Abiotic Stress. J. Exp. Bot. 56, 2907–2914. doi:10.1093/jxb/eri285
Pfaffl, M. W., Tichopad, A., Prgomet, C., and Neuvians, T. P. (2004). Determination of Stable Housekeeping Genes, Differentially Regulated Target Genes and Sample Integrity: BestKeeper - Excel-based Tool Using Pair-wise Correlations. Biotechnol. Lett. 26, 509–515. doi:10.1023/B:BILE.0000019559.84305.47
Phillips, M. A., D’Auria, J. C., Luck, K., and Gershenzon, J. (2009). Evaluation of Candidate Reference Genes for Real-Time Quantitative PCR of Plant Samples Using Purified cDNA as Template. Plant Mol. Biol. Rep. 27, 407–416. doi:10.1007/s11105-008-0072-1
Qiang, Y., Zhou, B., and Gao, K. (2011). Chemical Constituents of Plants from the Genus Rhododendron. Chem. Biodiversity 8, 792–815. doi:10.1002/cbdv.201000046
Radonić, A., Thulke, S., Mackay, I. M., Landt, O., Siegert, W., and Nitsche, A. (2004). Guideline to Reference Gene Selection for Quantitative Real-Time PCR. Biochem. Biophys. Res. Commun. 313, 856–862. doi:10.1016/j.bbrc.2003.11.177
Russell, D. A., and Sachs, M. M. (1992). Protein Synthesis in Maize during Anaerobic and Heat Stress. Plant Physiol. 99, 615–620. doi:10.1104/pp.99.2.615
Silver, N., Best, S., Jiang, J., and Thein, S. L. (2006). Selection of Housekeeping Genes for Gene Expression Studies in Human Reticulocytes Using Real-Time PCR. BMC Mol. Biol. 7, 33. doi:10.1186/1471-2199-7-33
Sun, W., Zhou, N., Wang, Y., Sun, S., Zhang, Y., Ju, Z., et al. (2021). Characterization and Functional Analysis of RdDFR1 Regulation on Flower Color Formation in Rhododendron Delavayi. Plant Physiol. Biochem. 169, 203–210. doi:10.1016/j.plaphy.2021.11.016
Udvardi, M. K., Czechowski, T., and Scheible, W.-R. (2008). Eleven Golden Rules of Quantitative RT-PCR. Plant Cell 20, 1736–1737. doi:10.1105/tpc.108.061143
Vandesompele, J., De Preter, K., Pattyn, F., Poppe, B., Van Roy, N., De Paepe, A., et al. (2002). Accurate Normalization of Real-Time Quantitative RT-PCR Data by Geometric Averaging of Multiple Internal Control Genes. Genome Biol. 3, 00341. doi:10.1186/gb-2002-3-7-research0034
Wang, Y., Liu, J., Wang, X., Liu, S., Wang, G., Zhou, J., et al. (2016). Validation of Suitable Reference Genes for Assessing Gene Expression of MicroRNAs in Lonicera japonica. Front. Plant Sci. 7, 1101. doi:10.3389/fpls.2016.01101
Xiao, Y., Karikari, B., Wang, L., Chang, F., and Zhao, T. (2021). Structure Characterization and Potential Role of Soybean Phospholipases A Multigene Family in Response to Multiple Abiotic Stress Uncovered by CRISPR/Cas9 Technology. Environ. Exp. Bot. 188, 104521. doi:10.1016/j.envexpbot.2021.104521
Xie, F., Xiao, P., Chen, D., Xu, L., and Zhang, B. (2012). miRDeepFinder: a miRNA Analysis Tool for Deep Sequencing of Plant Small RNAs. Plant Mol. Biol. 80, 75–84. doi:10.1007/s11103-012-9885-2
Yang, Y., Kwon, H. B., Peng, H. P., and Shih, M. C. (1993). Stress Responses and Metabolic Regulation of Glyceraldehyde-3-Phosphate Dehydrogenase Genes in Arabidopsis. Plant Physiol. 101, 209–216. doi:10.1104/pp.101.1.209
Yang, H., Liu, J., Huang, S., Guo, T., Deng, L., and Hua, W. (2014). Selection and Evaluation of Novel Reference Genes for Quantitative Reverse Transcription PCR (qRT-PCR) Based on Genome and Transcriptome Data in Brassica Napus L. Gene 538, 113–122. doi:10.1016/j.gene.2013.12.057
Yang, Z., Chen, Y., Hu, B., Tan, Z., and Huang, B. (2015). Identification and Validation of Reference Genes for Quantification of Target Gene Expression with Quantitative Real-Time PCR for Tall Fescue under Four Abiotic Stresses. PLoS One 10, e0119569. doi:10.1371/journal.pone.0119569
Yang, T., Gu, B., Xu, G., Shi, Y., Shen, H., Rao, R., et al. (2019). Identification of Candidate Reference Genes for qRT-PCR Normalization Studies of Salinity Stress and Injury in Onchidium Reevesii. PeerJ 7, e6834. doi:10.7717/peerj.6834
Zenda, T., Liu, S., Dong, A., Li, J., Wang, Y., Liu, X., et al. (2021). Omics-Facilitated Crop Improvement for Climate Resilience and Superior Nutritive Value. Front. Plant Sci. 12, 2770. doi:10.3389/fpls.2021.774994
Zhang, L., Xu, P., Cai, Y., Ma, L., Li, S., Li, S., et al. (2017). The Draft Genome Assembly of Rhododendron Delavayi Franch. Var. Delavayi. Giga Sci. 6, 1–11. doi:10.1093/gigascience/gix076
Zhang, L., Xu, Z., Ji, H., Zhou, Y., and Yang, S. (2019). TaWRKY40 Transcription Factor Positively Regulate the Expression of TaGAPC1 to Enhance Drought Tolerance. BMC Genomics 20, 795. doi:10.1186/s12864-019-6178-z
Zhang, M., Liu, S., Wang, Z., Yuan, Y., Zhang, Z., Liang, Q., et al. (2022). Progress in Soybean Functional Genomics over the Past Decade. Plant Biotechnol. J. 20, 256–282. doi:10.1111/pbi.13682
Zhou, W., Oh, J., Li, W., Kim, D. W., Yang, M. H., Jang, J.-H., et al. (2014). Chemical Constituents of the Korean Endangered Species Rhododendron Brachycarpum. Biochem. Syst. Ecol. 56, 231–236. doi:10.1016/j.bse.2014.06.003
Zhu, J., Zhang, L., Li, W., Han, S., Yang, W., and Qi, L. (2013). Reference Gene Selection for Quantitative Real-Time PCR Normalization in Caragana Intermedia under Different Abiotic Stress Conditions. PLoS One 8, e53196. doi:10.1371/journal.pone.0053196
Keywords: gene function, genomics, housekeeping gene, marker-assisted breeding, transcriptomics
Citation: Zhang L, Cai Y, Zhang M, Du G and Wang J (2022) Selection and Evaluation of Candidate Reference Genes for Quantitative Real-Time PCR in Aboveground Tissues and Drought Conditions in Rhododendron Delavayi. Front. Genet. 13:876482. doi: 10.3389/fgene.2022.876482
Received: 15 February 2022; Accepted: 17 March 2022;
Published: 14 April 2022.
Edited by:
Vinod K. K, Indian Agricultural Research Institute (ICAR), IndiaReviewed by:
Pankaj Bhardwaj, Central University of Punjab, IndiaCopyright © 2022 Zhang, Cai, Zhang, Du and Wang. This is an open-access article distributed under the terms of the Creative Commons Attribution License (CC BY). The use, distribution or reproduction in other forums is permitted, provided the original author(s) and the copyright owner(s) are credited and that the original publication in this journal is cited, in accordance with accepted academic practice. No use, distribution or reproduction is permitted which does not comply with these terms.
*Correspondence: Guanghui Du, ZGdoMjAxMkB5bnUuZWR1LmNu; Jihua Wang, d2poMDUwNUBnbWFpbC5jb20=
†These authors have contributed equally to this work
Disclaimer: All claims expressed in this article are solely those of the authors and do not necessarily represent those of their affiliated organizations, or those of the publisher, the editors and the reviewers. Any product that may be evaluated in this article or claim that may be made by its manufacturer is not guaranteed or endorsed by the publisher.
Research integrity at Frontiers
Learn more about the work of our research integrity team to safeguard the quality of each article we publish.