- 1Shanghai Skin Disease Hospital, School of Medicine, Tongji University, Shanghai, China
- 2Department of Dermatology, Yueyang Hospital of Integrated Traditional Chinese and Western Medicine, Shanghai University of Traditional Chinese Medicine, Shanghai, China
- 3Institute of Dermatology, Shanghai Academy of Traditional Chinese Medicine, Shanghai, China
- 4School of Rehabilitation Science, Shanghai University of Traditional Chinese Medicine, Shanghai, China
Background: Psoriasis is a chronic and immune-mediated skin disorder that currently has no cure. Pyroptosis has been proved to be involved in the pathogenesis and progression of psoriasis. However, the role pyroptosis plays in psoriasis remains elusive.
Methods: RNA-sequencing data of psoriasis patients were obtained from the Gene Expression Omnibus (GEO) database, and differentially expressed pyroptosis-related genes (PRGs) between psoriasis patients and normal individuals were obtained. A principal component analysis (PCA) was conducted to determine whether PRGs could be used to distinguish the samples. PRG and immune cell correlation was also investigated. Subsequently, a novel diagnostic model comprising PRGs for psoriasis was constructed using a random forest algorithm (ntree = 400). A receiver operating characteristic (ROC) analysis was used to evaluate the classification performance through both internal and external validation. Consensus clustering analysis was used to investigate whether there was a difference in biological functions within PRG-based subtypes. Finally, the expression of the kernel PRGs were validated in vivo by qRT-PCR.
Results: We identified a total of 39 PRGs, which could distinguish psoriasis samples from normal samples. The process of T cell CD4 memory activated and mast cells resting were correlated with PRGs. Ten PRGs, IL-1β, AIM2, CASP5, DHX9, CASP4, CYCS, CASP1, GZMB, CHMP2B, and CASP8, were subsequently screened using a random forest diagnostic model. ROC analysis revealed that our model has good diagnostic performance in both internal validation (area under the curve [AUC] = 0.930 [95% CI 0.877–0.984]) and external validation (mean AUC = 0.852). PRG subtypes indicated differences in metabolic processes and the MAPK signaling pathway. Finally, the qRT-PCR results demonstrated the apparent dysregulation of PRGs in psoriasis, especially AIM2 and GZMB.
Conclusion: Pyroptosis may play a crucial role in psoriasis and could provide new insights into the diagnosis and underlying mechanisms of psoriasis.
1 Introduction
Psoriasis is regarded as an immune-mediated, inflammatory skin disease (Demir Pektas et al., 2018), affecting more than 100 million people worldwide; estimates of its prevalence vary from 0.51 to 11.43% (Michalek et al., 2017). Comorbidities associated with psoriasis include cardiovascular disease (Tangtatco and Lara-Corrales, 2017), chronic obstructive pulmonary disease (Ungprasert et al., 2016), and metabolic syndrome (Armstrong et al., 2013), which can affect a patient’s quality of life and pose significant challenges for the medical field.
Psoriasis is thought to progress as a result of abnormal keratinocyte cell death in the skin (Ramirez et al., 2012). The unique keratinization process of psoriasis is that late differentiation markers are abolished because of premature cell death (Iizuka et al., 2004), including pyroptosis, apoptosis, necrosis, necroptosis, and ferroptosis. Pyroptosis-related genes (PRGs) have been shown to have a strong association with aberrant keratinocyte keratinization in vitro (Lachner et al., 2017). Pyroptosis is a novel, inflammation-dependent type of programmed cell death (Fang et al., 2020), encompassing the classical caspase-1 pathway and the non-classical caspase-4/5/11 pathway (Song et al., 2021) (Figure 1). As studies continue, the non-classical pathway, caspase-11-mediated pyroptosis has been found to contribute to the pathogenesis of psoriasis (Kenealy et al., 2019). Multiple pieces of evidence indicated that PRGs are differentially expressed in psoriasis, such as GSDMD, caspase-1/2, IL-1 family genes (Lachner et al., 2017), NLRP1, NLRP3 (Deng et al., 2019), and AIM2 (Ciążyńska et al., 2021). These findings suggest that pyroptosis may serve as a potential prognostic or therapeutic target in psoriasis.
Due to the limitations of current investigational techniques, however, relevant gene expression studies have often been confined to one or two PRGs, despite psoriasis being a polygenically mediated disorder (Georgescu et al., 2019). A comprehensive understanding of PRG characteristics will be of great importance in elucidating the underlying mechanisms and predicting the response of psoriasis to immunotherapy. Hence, there is an urgent need to establish a predictive screening model to identify potential PRGs of psoriasis with a high predictive accuracy.
Various machine learning models have shown encouraging performance in biomarker prediction studies (Izadyyazdanabadi et al., 2018). The random forest model, one of the best conventional machine learning approaches (Jha et al., 2018) (Douville et al., 2021), is based on the principle of ensemble learning (Sessa et al., 2020), which has previously shown a high predictive accuracy (62–71%) in modeling (Tran et al., 2019) and provide variable importance estimates than classifiers. The random forest method has advantages even in uninformative predictor of random forest missing data mechanism (Moore et al., 2015). Despite the potential usefulness of random forest, its application in psoriasis PRG regulators have not been reported.
Here, we comprehensively evaluated the regulation of PRGs. First, the differential expressions of PRGs between psoriasis and normal samples was analyzed. Second, a machine learning model of psoriasis was established using a random forest algorithm to innovatively identify potential therapeutic approaches. Third, the relationship between pyroptosis and the immune system was investigated. Subsequently, we validated the mRNA expression of PRGs in vivo. Therefore, this research provides potential targets for the diagnostic and treatment of patients with psoriasis.
2 Methods
2.1 Psoriasis patients’ dataset
2.1.1 mRNA expression profile of psoriasis patients
The mRNA expression profiles of psoriasis patients and control were downloaded from the Gene Expression Omnibus (GEO) database (Barrett et al., 2013) (http://www.ncbi.nlm.nih.gov/geo/). The data acquisition criteria were as follows: all participants of the included studies were human; samples were not based on cell lines; sample type should be skin tissue; patients should be diagnosed with psoriasis; datasets should be complete data for analysis; ethical approval was obtained; and the control group had neither systemic nor autoimmune diseases and no relevant family history. GSE114286 was obtained as the training cohort, and the other three datasets (GSE14905, GSE109248, and GSE117239) were obtained for external validation analyses, the basic information for which is shown in Table 1.
2.1.2 Dataset characteristics
The R package limma was adopted to identify the differentially expressed genes (DEGs) between psoriasis and normal samples. To correct for false-positive results, Benjamini and Hochberg’s (1995) false discovery rate (FDR) method was used by default. A fold change of >1.5, a p-value <0.05, and an FDR <0.05 were set as the cutoffs to screen DEGs (Yu et al., 2012).
2.2 Characteristics of PRGs
2.2.1 PRGs obtained
Two pyroptosis gene sets were acquired (REACTOME_PYROPTOSIS and GOBP_PYROPTOSIS), and a total of 39 PRGs were obtained from the Molecular Signatures Database (MSigDB) (Liberzon et al., 2015) (https://www.gsea-msigdb.org/gsea/msigdb/index.jsp).
2.2.2 PRG internal correlation analysis and protein–protein interaction (PPI) analysis
For correlation analysis among PRGs, the Spearman correlation function of the R package was used. The range of the correlation coefficient of a pair (r) was from 1 to -1; r values close to 1 or -1 indicate a strong positive or negative correlation between genes, respectively.
A protein–protein interaction (PPI) network analysis was performed to identify potential interactions among PRGs. The STRING database (Szklarczyk et al., 2017) (https://string-db.org/) was used to obtain interaction pairs for proteins related to pyroptosis with the highest confidence (interaction minimum >0.9). Cytoscape software (Shannon et al., 2003) (version 3.9.0) was then used to display the PPI network.
2.3 Identification of differentially expressed PRGs
2.3.1 DE mRNA levels of PRGs
Pyroptosis-related genes were extracted, and we screened the PRGs using the R package limma, with p < 0.05, |Fold change|>1.5 as the conditions. The heatmap was created using the R packages pheatmap and ggplot2 to show differentially expressed PRGs in patients with psoriasis compared with normal control samples. We also examined the effectiveness of PRGs in other skin diseases.
2.3.2 The ability of differentially expressed (DE) PRGs to discriminate samples by principal component analysis (PCA)
To determine whether PRGs were suitable for distinguishing psoriasis from normal tissue samples, PCA was used to determine the genotyping consistency using the R package mixomics.
2.4 PRGs and immune cell correlation representation
Pearson correlation were performed for PRGs and immune cell abundance using the cor function in R, and then the pheatmap function was used to draw a heatmap. If the grid on the right image is marked with *, it means that the absolute value of the correlation between the pyroptosis gene and immune cells is >0.5.
2.5 Diagnostic model using a random forest algorithm
2.5.1 Establishing the random forest model
We used a machine learning diagnostic model, the random forest, to screen candidate psoriasis-specific genes from PRGs using the R package randomForest (Liaw and Wiener, 2002). Random forest is an ensemble method for the training and prediction of samples based on multiple classification trees. The best split among all variables was used for each node, and the best among a random subset of predictors was used for the random forest. The parameter optimization is initially performed using randomly generated parameter sets. As computation time increases with increasing ntree, we used ntree = 400 in our modeling. The training set contained three quarters of the samples in each repetition. The procedure was repeated 100 times by random sampling.
2.5.2 Internal validation
Conventionally, the internal validation set should include one quarter of the samples in each repetition. Ten-fold cross-validation was performed as an internal validation method to confirm the predictive performance of our diagnostic model for psoriasis based on PRGs. The receiver operating characteristic (ROC) curves were generated, and the area under the curves (AUC) were derived as the internal cross-validation, using the pROC package in R to evaluate the discriminative ability.
2.5.3 External validation
The universality and reliability of the random forest diagnostic model were independently validated against an external validation cohort, including the GSE14905, GSE109248, and GSE117239 datasets, whose characteristics are shown in Table 1. The ROC analysis procedure was performed.
2.6 Consensus clustering analysis of PRGs
We performed consensus clustering to investigate whether there was a difference within PRG-based subtypes, such as psoriasis-related functions and pathways. Consensus clustering is an unsupervised clustering algorithm used to identify subgroup members and verify subgroups based on resampling.
2.6.1 Identification of pyroptosis-related subtypes
The classification of patients into subtypes based on PRGs was calculated using the R packages consensusclusterplus and pheatmap, which were employed for consensus unsupervised clustering analysis. The box plots were created using the R software package ggplot2 (Yi et al., 2020). The criteria included cumulative distribution function (CDF) curve increasing smoothly. The k value was adopted to describe the number of clusters, from 2 to 8 and finally set k = 3 as the optimal subtype number because of the highest delta area score.
2.6.2 DEGs based on PRG subtypes
The consensus clustering classified psoriasis into three distinct subtypes based on PRGs. We next identified DEGs among the different clusters using the limma package. Considering the insufficient sample size in cluster 3 (sample duplicates <3), we focused on the first two clusters.
2.6.3 Biological functions based on PRG subtypes
To explore the differentiation in biological characteristics and potential pathways between PRG-based subtypes, the Gene Ontology (GO) and Kyoto Encyclopedia of Genes and Genomes (KEGG) pathways among clusters were investigated using the R package clusterProfiler (Kursa, 2014), with an FDR cutoff of <0.01.
2.7 Experimental verification
2.7.1 Animal preparation
Twelve male C57BL/6 mice, aged 6–7 weeks, weighing 22–25 g, provided by Shanghai Medical Experimental Animal Center (permission no. SCXK(Hu)2018-0003, Shanghai, China) were used as experimental animals. They were housed in a specific pathogen free (SPF) facility, at a temperature of 21–25°C, 16–8 h light–dark cycle, free water, and standard diet provided by Shanghai Pu Lu Tong Biological Technology Co., Ltd. The Yueyang Hospital of Integrated Traditional Chinese and Western Medicine of Shanghai University of Traditional Chinese Medicine Ethics Committee approved the animal experiment (No. YYLAC-2020-078-3) (Supplementary File S1).
2.7.2 Imiquimod (IMQ)-induced psoriasis-like mice model establishment and intervention
After shaving the hair of mice in a 2 × 2 cm2 square area, the animals were randomly separated into two groups:
1) Control group (NC), received a topical dose (62.5 mg) of petroleum jelly.
2) Psoriasiform modeling group (IMQ), received a topical dose (62.5 mg) of IMQ cream (5%) for 6 h prior to intragastric administration of saline (1.8 g/kg).
All treatments were applied once per day for 12 consecutive days from the date of application of IMQ (day 0). Mice were fasted and allowed to drink water for 12 h prior to sample collection. The animals were euthanized by CO2 inhalation (day 12), and back and ear tissues were collected for further experiments.
2.7.3 qRT-PCR
To detect the mRNA expression level of PRGs selected by the random forest algorithm, qRT-PCR was used. Skin tissue homogenates from psoriasis-like mice were taken and applied Trizol reagent (Beyotime, China) protocol for total RNA extraction, whose concentration and purity were determined using an ultraviolet spectrophotometer and assessed via agarose gel electrophoresis. The relative quantitative method (2−ΔΔCT) was applied. Reverse transcriptase was used to prepare cDNA. The results were normalized to GAPDH. Primers were designed and synthesized (Table 2).
2.7.4 Statistical methods
All experimental data were analyzed using the statistical software SPSS 24.0 (IBM Corp., Armonk International Business Machines, New York, United States). Data are presented as mean ± standard deviation (SD). Comparisons between two groups were conducted using one-way ANOVA. Significant differences among two groups were indicated by **p < 0.01, *p < 0.05, or ***p < 0.001, two-sided.
3 Results
A flow diagram representing this work is shown in Figure 2. First, the characteristic information and gene expression profiles of psoriasis cases and healthy controls in the GSE114286, GSE14905, GSE109248, and GSE117239 datasets were obtained. Using the GSE114286 dataset, we identified several differentially expressed PRGs, and PCA analysis indicated that the PRGs could distinguish psoriasis from normal tissue samples. Then, a correlation analysis between PRGs and immune cell were conducted as well.
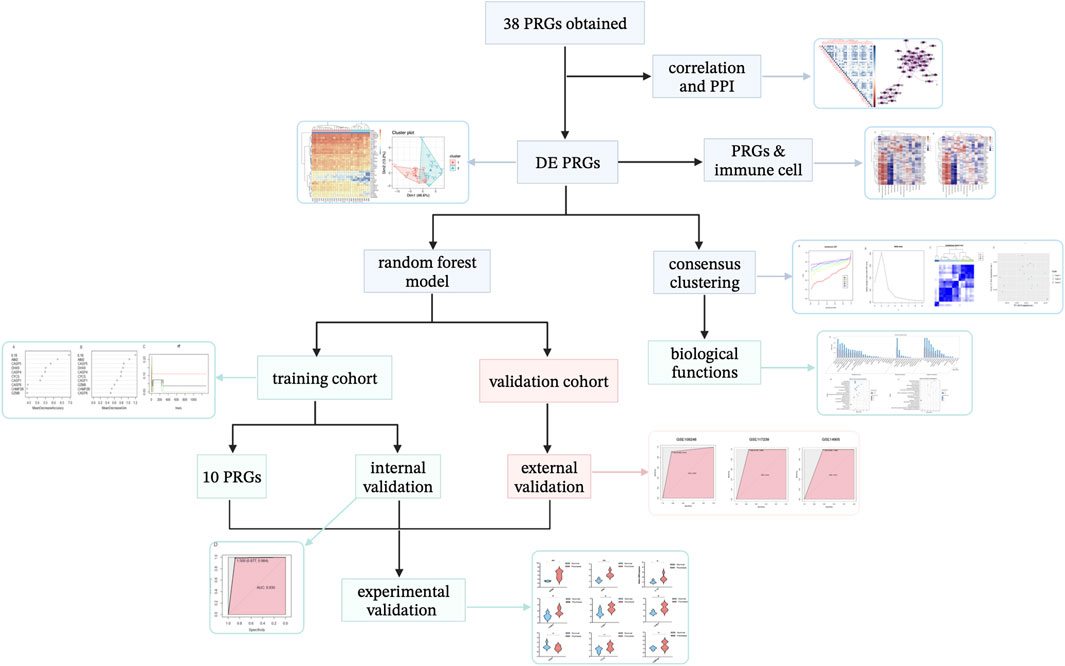
FIGURE 2. Article framework and workflow. PRGs, pyroptosis-related genes. PPI, protein–protein interaction. DE, differentially expressed.
Subsequently, we developed a novel diagnostic model for psoriasis based on PRGs using a machine learning random forest method, which screened 10 potential PRG biomarkers. GSE14905, GSE109248, and GSE117239 were used for external validation.
To discover whether there was a difference between subtypes of PRGs, we used consensus clustering analysis to divide the samples into three subtypes. The particular biological characteristics among clusters were investigated.
Finally, experimental validation was performed using IMQ-induced psoriasis-like mice and qRT-PCR analysis.
3.1 The transcriptional regulator landscape in psoriasis patients
The training cohort, the GSE98793 dataset, included 2,139 DEGs comparing psoriasis patients with healthy individuals and 39 PRGs were presented respectively (Supplementary Figure S1A). The external validation cohort (GSE14905, GSE109248, and GSE117239) included 1,959 DEGs (Supplementary Figure S1B), the basic information of which is shown in Table 1.
3.2 PRG characteristics
A total of 39 PRGs were obtained from MSigDB, and the annotation analysis is shown in Supplementary Figure S2. We attempted to demonstrate the PRGs correlation, and the results indicated that the PRGs have positive connection, especially AIM2, ACSP1, CASP8, and GZMB (Figure 3A). The interaction relationships of these PRGs regulators were exhibited as a PPI network, which reminded us that the PRGs have strong protein-protein interaction, especially in CHMPs family or CASPs family (Figure 3B). Among all nodes, CASP1 and IL1B had the highest degree of protein interactions in the PPI network. These results indicated that expression imbalances of PRGs played critical roles in psoriasis.
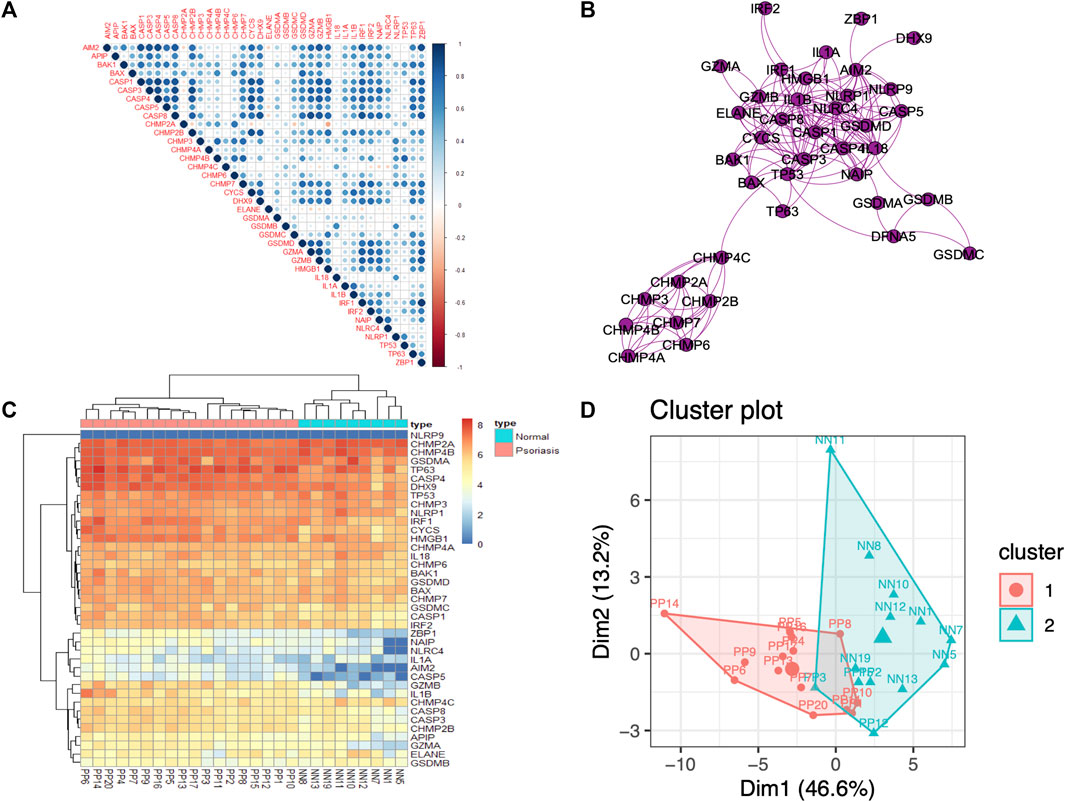
FIGURE 3. PRGs correlation and interaction analysis, and DE PRGs identification between psoriasis and normal samples (A). Spearman correlation analysis of the 39 differentially expressed PRGs; blue represents a positive correlation, red represents a negative correlation, and the darker the color, the stronger the correlation. (B). Protein–protein interaction (PPI) analysis of the 39 differentially expressed PRGs. (C). Heatmap of the 39 differentially expressed PRGs in psoriasis and normal samples. (D). Principal component analysis (PCA) of PRGs.
3.3 Identification of DE PRGs
3.3.1 PRGs are differentially expressed in psoriasis
To investigate the contribution of PRGs to the pathogenesis of psoriasis, the mRNA expression of PRGs was compared between psoriasis and normal samples (Figure 3C). Furthermore, the results of differences on PRGs in other skin disorders, including psoriatic arthritis (GSE61281), atopic dermatitis (GSE124700 and GSE60709), and acne (GSE53795 and GSE6475), indicated that PRGs could be biomarkers of psoriasis (Supplementary Table S1).
3.3.2 DE PRGs have the ability to discriminate psoriasis samples
The PCA results were used to determine distinctiveness-based confidence measures of PRGs (Dim1 = 46.6%, Dim2 = 13.2%), which indicated that PRGs have the ability to clearly distinguish psoriasis from normal samples (Figure 3D).
3.4 PRGs and immune cell correlation analysis
Emerging evidence has indicated there is crosstalk between pyroptosis and immune responses (Orning et al., 2019). In Figure 4, T cells CD4 memory activated show the strongest positive correlation with TP63 (r = 0.69), while mast cells resting and NAIP are the most negatively correlated pair (r = -0.64). The CIBERSORT and immune cell abundance between psoriasis and normal is shown in Supplementary Figure S3.
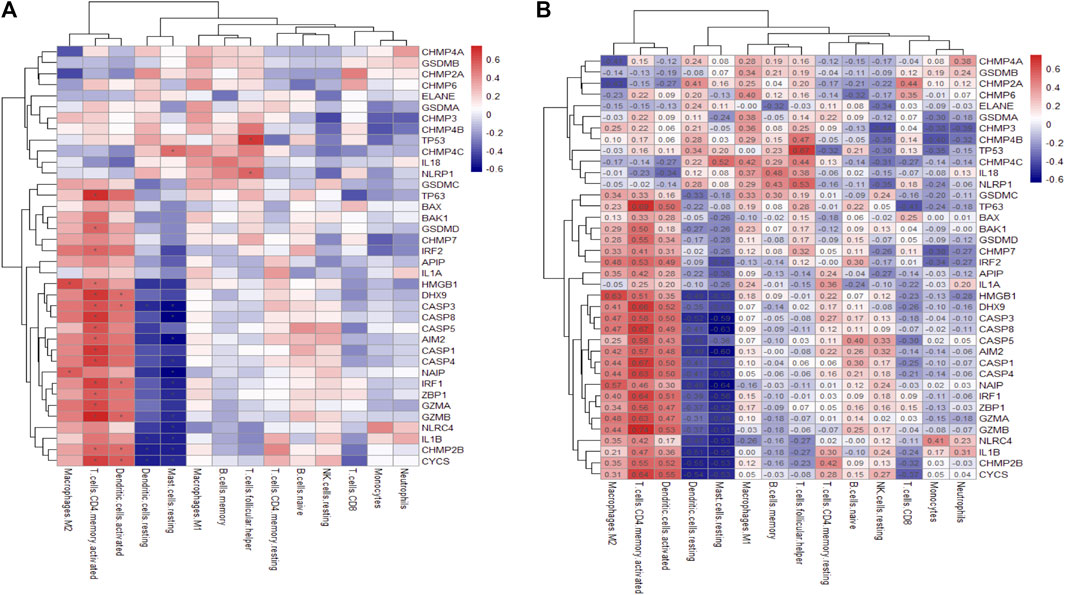
FIGURE 4. Heatmap of the differentially expressed PRGs in various immune cells. (A). PRG correlation map with immune cells, where the horizontal axis represents PRGs and the vertical axis represents immune cells. (B) The correlation map marked with absolute value. Asterisks represent levels of significance *p < 0.05.
3.5 Random forest model mediated by PRGs
3.5.1 Ten feature DE PRGs were screened out random forest model
The random forest algorithm was used to rank the importance of prognostic PRGs. Applying the selection criteria previously used, we shortlisted 10 PRGs by mean decrease accuracy and mean decrease gini (Figures 5A–C). Interleukin 1 beta (IL-1β) was the most prominent pyroptosis eigengene of psoriasis, followed by absent in melanoma 2 (AIM2) and caspase 5 (CASP5). DExH-Box helicase 9 (DHX9) and caspase 4 (CASP4) contain slightly more predominance than cytochrome C, somatic (CYCS), and caspase 1 (CASP1).
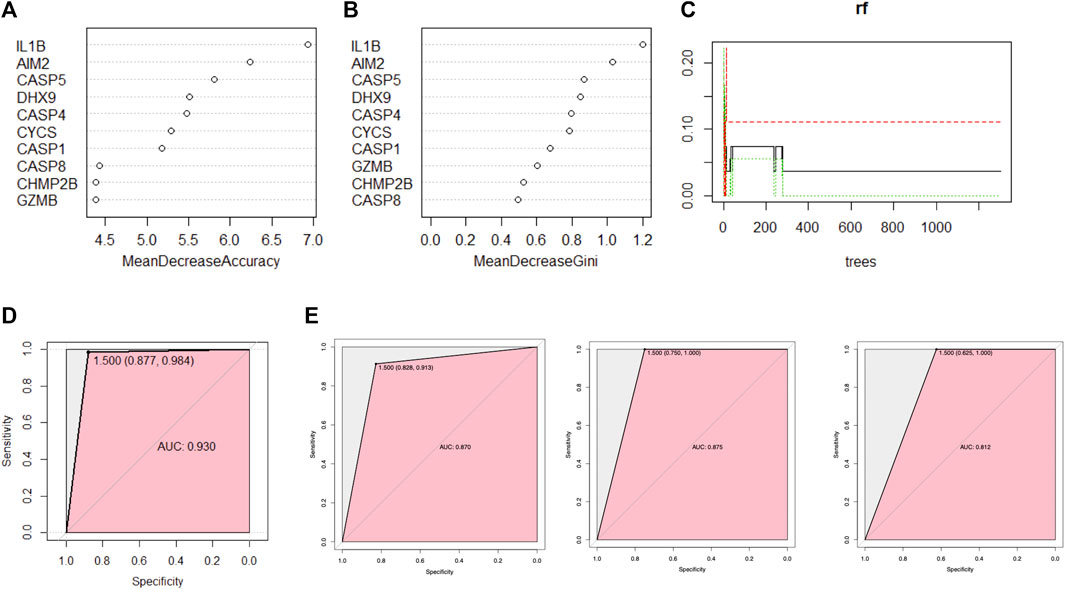
FIGURE 5. A random forest algorithm was used for ranking the importance of prognostic PRGs. (A,B) The importance of PRGs using the scores returned by the random forest model. (C) Parameter optimization was initially performed using randomly generated parameter sets, ntree = 400 was selected in the modeling, and stability was achieved when 100 random samples were taken, three quarters of the dataset was grouped as the training set and the remaining one quarter as the validation set in each repetition. (D) The receiver operating characteristic (ROC) curve (area under the curve (AUC) = 0.93) was generated by cross-internal validation. (E) ROC curve on independent external validation, from left to right are GSE117239, GSE109248, and GSE14905, respectively.
3.5.2 Internal validation
The model demonstrated good discrimination by performing cross-validation in an ROC curve in internal validation [AUC = 0.930 (0.877, 0.984)] (Figure 5D), which indicated the excellent performance of the current random forest model.
3.5.3 External validation
The ROC curve analysis was subsequently used for independent external validation, with the value of the AUC representing the consistency of the model. The results showed that the AUC was 0.870 (95% CI 0.828–0.913) for GSE109248, 0.875 (95% CI 0.750–1.000) for GSE117239, and 0.812 (95% CI 0.625–1.000) for GSE14905 (Figure 5E), which indicated the model had a good predictive performance. In summary, this random forest model showed reliable predictive power in the external discrimination test (mean AUC = 0.852).
3.6 Consensus clustering analysis of PRGs
3.6.1 Three pyroptosis subtypes of psoriasis obtained
To investigate the association between the PRGs and psoriasis subtypes, we used a consensus clustering algorithm in the training cohort. When variable (k) = 3, the psoriasis patients could be well separated into three subtypes of 39 PRGs (Figures 6A–C) with the highest intragroup correlations and the lowest intergroup correlations. Cluster 1 included nine patients and cluster 2 included seven patients, leaving two psoriasis patients in cluster 3. The PCA results showed these three clusters were significantly different from each other, especially cluster 1 and cluster 2 (Figure 6D).
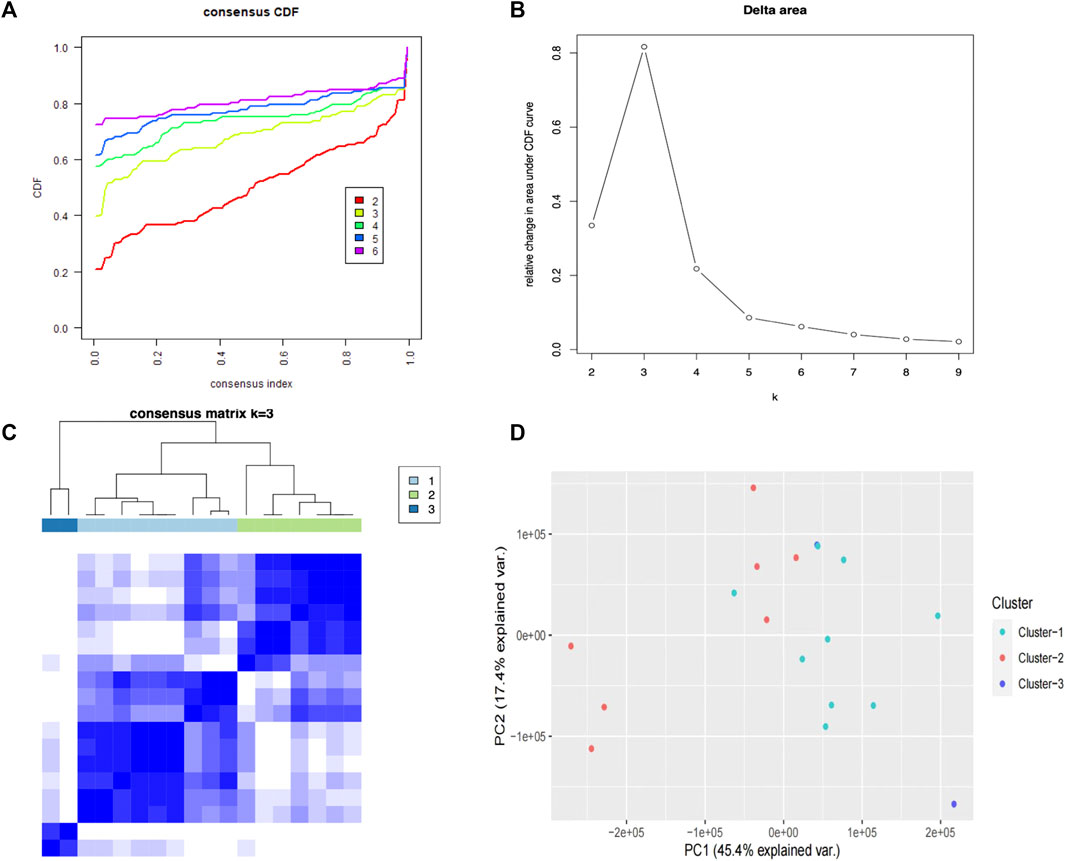
FIGURE 6. Consensus clustering analysis of PRGs. (A) The cumulative distribution function (CDF) curve for each category number k compared with k -1.(B) Delta area curve of consensus clustering, indicating the relative change in area under the CDF. The horizontal axis represents the category number k, and the vertical axis represents the relative change in area under the CDF curve. (C) A heatmap showing the consensus clustering solution (k = 3) for 39 PRGs in three clusters. (D) The PCA results show these three clusters were significantly differentiated, especially clusters 1 and 2.
3.6.2 Differential genes and biological functions based on PRGs subtype
To investigate the differences in biological functions between cluster 1 and cluster 2, we initially calculated the differentially expressed mRNAs (Supplementary Table S2). Next, we performed the GO and KEGG enrichment analyses, which revealed the enrichment of metabolic processes (Figures 7A,B), the MAPK signaling pathway, FoxO signaling, and the RAGE signaling pathway (Figure 7D).
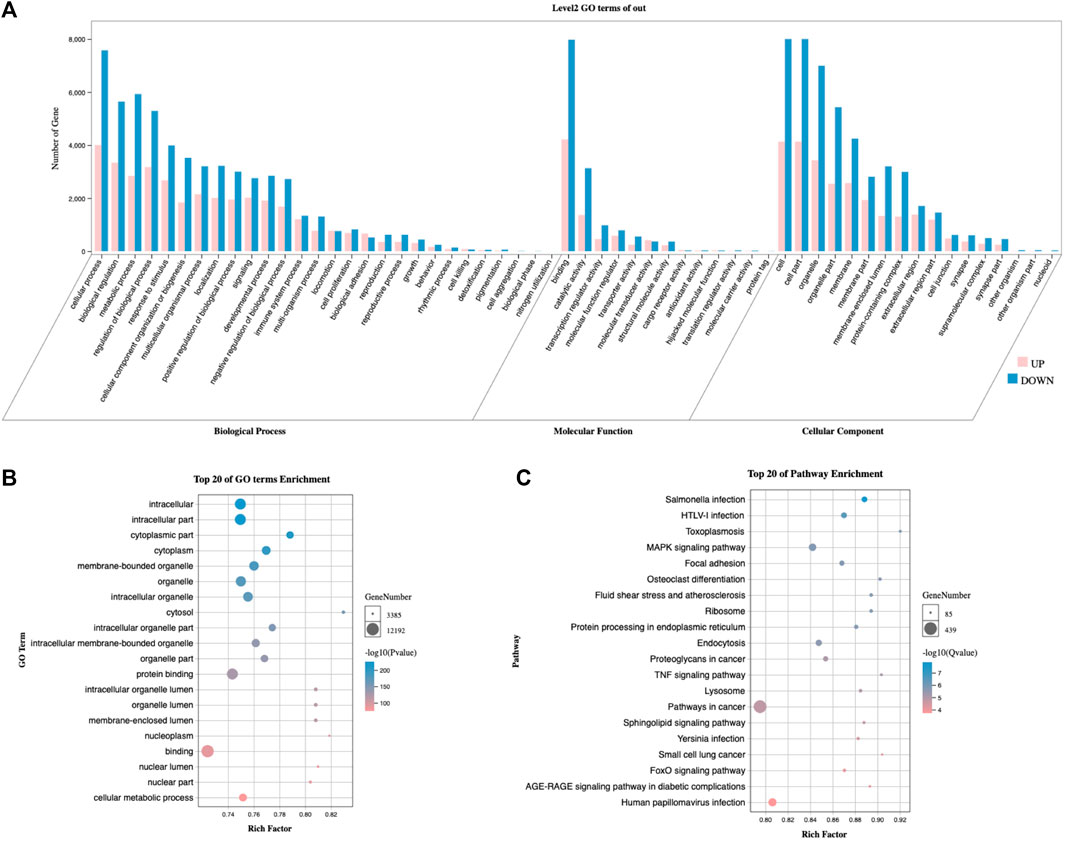
FIGURE 7. Comparison of biological functions analysis between clusters 1 and 2. (A) The secondary classification of GO, including biological processes, molecular functions, and cellular components. (B) Bubble map of the GO analysis, ranked by p-value. (C) Bubble map of KEGG signaling pathways, ranked by Q-value. GO, Gene Ontology. KEGG, Kyoto Encyclopedia of Genes and Genomes.
3.7 qRT-PCR-verified expression of PRGs in IMQ-induced psoriasis-like mice
To further demonstrate the results of the random forest model, we validated the nine target mRNAs in psoriatic mice, except for CASP5, which is not present in mice. In contrast with the normal group, significant changes were observed by qRT-PCR in the expression of several PRGs in the psoriasis group.
The most obvious significance of PRGs expression was observed in Granzyme B (GZMB) (Figure 8), which was dramatically elevated in inflammatory skin disorders; it is known for its pro-apoptotic function, as well as epithelial barrier disruption (Turner et al., 2019). Psoriasis has a high levels of AIM2, the activation of which initiates the assembly of the inflammasome, triggering the maturation and secretion of the cytokine IL-1β (Lugrin and Martinon, 2018, 2). Here, we revealed the high expression of the cysteinyl aspartate protease (caspase, or CASP) gene family, including CASP1, CASP4, and CASP8, which play significant roles in programmed cell death, inflammation, and immunity (Hong et al., 2020). However, no statistical significance was shown on DHX9, CYCS, and CHMP2B, which should be accomplished in future research using large scale clinical samples.
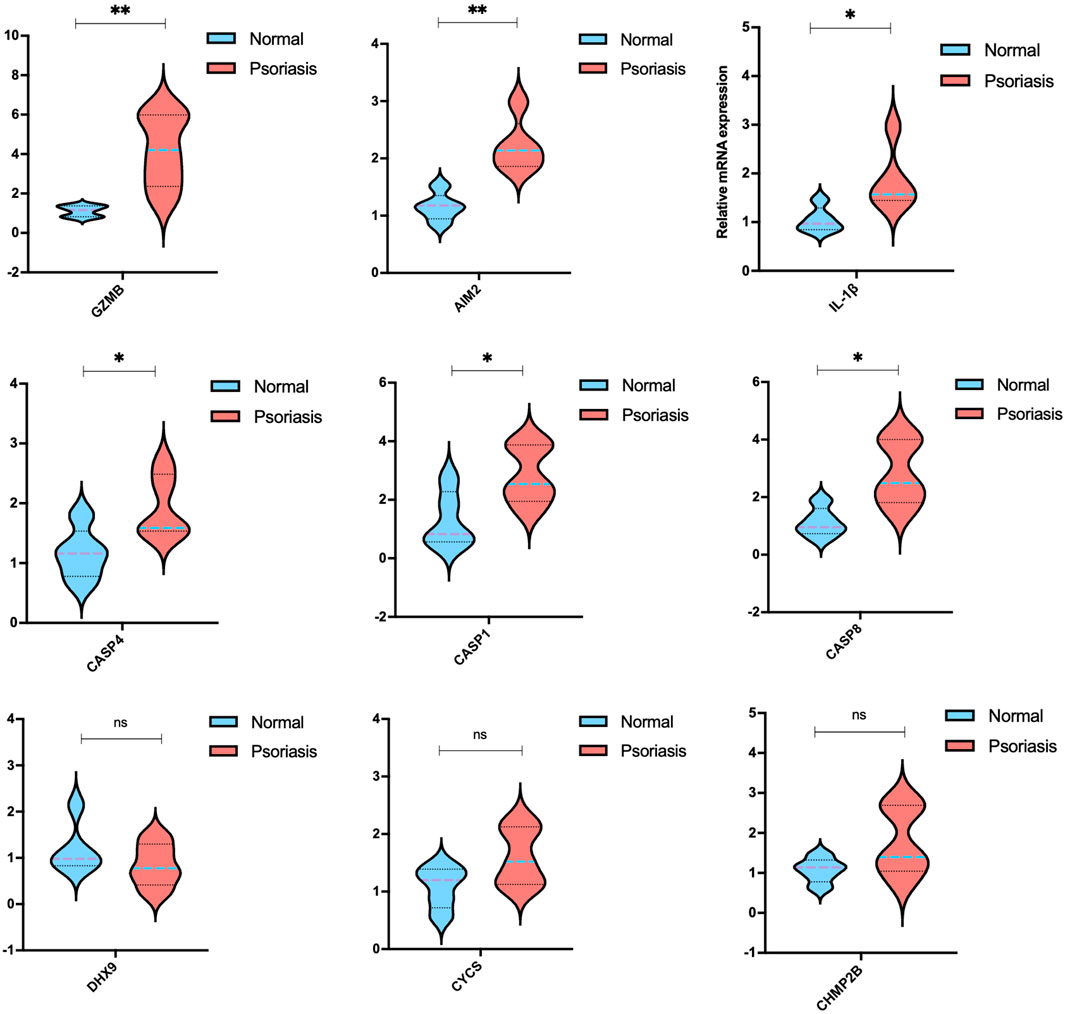
FIGURE 8. mRNA levels of the candidate PRGs of imiquimod (IMQ)-induced psoriasis-like mice compared with the control group (n = 5). The data are expressed as means ± SD. Four skin lesions in each group were included in the analysis. *p < 0.05, **p < 0.01, compared with the control group.
4 Discussion
Many studies have reported the indispensable role pyroptosis plays in psoriasis (Wang et al., 2020). Whereas most research focused on one or two PRGs in vivo or vitro, for instance, the pathogenesis of psoriasis is closely related to caspase-1, IL-1β, IL-18, or GSDMD. The contribution degree of PRGs in psoriasis are still elucidated.
Here, we identified several differentially expressed PRGs, and PCA indicated that these PRGs could distinguish psoriasis from normal tissue samples. Subsequently, we developed a novel diagnostic model for psoriasis based on PRGs, using the random forest machine learning method, which involved screening 10 potential PRG biomarkers, followed by internal and external validation. We also looked for correlations between immune cells and PRGs. To discover whether there was a difference between subtypes of PRGs, consensus clustering analysis was used to divide the samples into three subtypes. The particular biological characteristics among clusters were observed. Finally, experimental validation was performed using IMQ-induced psoriasis-like mice and qRT-PCR analysis.
Random forest, a machine learning algorithm, was the main predictive model used in our study to reveal contribution degree of PRGs in psoriasis. It is an ensemble machine learning algorithm based on decision trees, with strong anti-noise ability and good robustness, and can be used for feature selection based on features with a high prediction accuracy; it is widely used for a variety of classification and regression problems (Moore et al., 2015). We identified 10 regulators based on feature values from the random forest algorithm. Subsequent experimental validation provided further evidence that mRNA expression of AIM2, CASP4, CASP1, CASP8, and GZMB was significantly upregulated in IMQ-induced mice.
Pyroptosis is regulated by the classical caspase-1 pathway and the non-classical caspase-4/5/11 pathway (Xiang and Yang, 2020). Upon stimulation by pathogenic microbes, intracellular pattern recognition receptors (PRRs), such as AIM2, assemble into caspase-1-dependent inflammasomes via apoptosis-related specific protein pattern recognition receptors. Next, the activated caspase-1 cleaves the inflammatory cytokine IL-1β and IL-18 precursor, making it an active cytokine, which is the classical pathway of pyroptosis (Chen et al., 2018). Pyroptosis is characterized by the cellular production of IL-1β (Wagner et al., 2017). Also, the tendency of the numerical results to meet an agreement with our experiments. Our model revealed consistencies with clinical observations that the augmented protein levels of IL-1β in skin tissue are associated with psoriatic lesional area and severity (Cai et al., 2019) (Su et al., 2018, 3) (Yu et al., 2021) (Ciążyńska et al., 2021, 2). Enhanced expression of IL-1β mRNA has been observed in vivo, in IMQ-induced mice (Shou et al., 2021) (Deng et al., 2019) (Tang et al., 2021). This tendency was in accordance with the result of one previous cell experiment (Kim et al., 2021). Aberrant or excessive activation of the classical caspase-1 path is associated with many autoinflammatory, autoimmune, and metabolic diseases (Shi et al., 2015). One study revealed that inflammasome sensors, NLRP3, NLRP1, CASP1, and AIM2, enhanced expression in psoriasis patients (Verma et al., 2021). An in vivo study demonstrated significantly augmenting protein expression of AIM2 in IMQ-induced psoriasis-like mouse model (Chung et al., 2020).
The non-classical pathway of pyroptosis mediated by caspase-4 and caspase-5 was identified as kernel regulators in psoriasis by a random forest algorithm in the current study. Consistent with our findings, the non-classical inflammasome is a complex of bacterial lipopolysaccharide together with caspase-4, caspase-5, and caspase-11 (Liu and Lieberman, 2017), which has been proved to directly activated GSDMD-mediates pyroptosis process. After stimulation of cells with lipopolysaccharide from gram-negative bacteria, caspase-4/5/11 directly binds to lipid A of lipopolysaccharide or activates caspase-1 via the NLRP3 inflammasome pathway, which cleaves GSDMD to become GSDMD N-terminal and GSDMD C-terminal, which in turn causes cell membrane rupture and lysis as well as the release of pyroptosis-related factors such as IL-18 and IL-1β (Liu and Lieberman, 2017). Notably, the augmented levels of CASP8 and GAMB were observed in the psoriasis-like dermatitis model, although their roles in the pathogenesis of psoriasis have not yet been investigated.
Accumulating evidence suggests that pyroptosis-related regulators play a crucial role in psoriasis. Intervention in the pyroptosis-related pathway could be a potential target for the treatment of psoriasis. Our study had some limitations. First, due to the lack of information about clinical outcomes, we were unable to directly evaluate the prognostic value of the current model, therefore, further studies are needed. Second, achieving practical strategies for translating psoriasis risk-associated genetic variants into functional annotations and clinical applications remains challenging.
5 Conclusion
In summary, we used a machine learning model and experimental verification to investigate the role of PRGs in the pathogenesis of psoriasis. Through the experimental verification of 10 PRGs obtained by machine learning, it was found that the mRNA expression of IL-1β, AIM2, CASP4, CASP1, CASP8, GZMB, especially IL-1β, CASP1, CASP8, and GZMB, was ameliorated to alleviate the skin lesions IMQ-induced psoriasis-like mice, which provided potential therapeutic targets.
Data availability statement
The datasets presented in this study can be found in online repositories. Further inquiries can be directed to the corresponding authors.
Ethics statement
The animal study was reviewed and approved by Yueyang Hospital, affiliated to Shanghai University of Traditional Chinese Medicine Ethics Committee.
Author contributions
J-KS, YZ, and X-YF conceived and designed the study. YL and LK provided administrative support. Y-RC and YL collected and assembled the data. J-SJ and YR performed the experimental validation. J-KS and YZ analyzed and interpreted the data. BL and Y-WX supported resources and validation. All authors wrote the manuscript and approved the final version of the manuscript.
Funding
This study was supported by the National Key Research and Development Program of China (No. 2018YFC1705305), the NSFC of China (No. 81973860, 81904214, 82004235, 82174383), the Three-Year Action Plan of Shanghai Municipality for further acceleration of the development of Chinese medicine [No. ZY (2018-2020)-FWTX-4010, ZY (2018-2020)-FWTX-1008, ZY (2021-2023)-0302], the Dermatology Department of Traditional Chinese Medicine, Clinical Key Specialty Construction Project of Shanghai (No. shslczdzk05001), Shanghai Municipal Commission of Economy and Information Technology, Shanghai Artificial Intelligence Innovation and Development Project-Intelligent Dermatology Clinic Based on Modern TCM Diagnostic Technology (No. 2020-RGZN-02038) the Young Talent Support Project of Chinese Association of Traditional Chinese Medicine (No. 2021-QNRC2-A10), the Xinglin Youth Scholar of Shanghai University of Traditional Chinese Medicine (no. RY411.33.10), the Shanghai Sailing Program (no. 21YF1448100, 22YF1450000, 22YF1441300), the Clinical Transformation Incubation Program in Hospital (lczh 2021-05), and the Peiran Scholar of Shanghai University of Traditional Chinese Medicine (no. 2015811038).
Conflict of interest
The authors declare that the research was conducted in the absence of any commercial or financial relationships that could be construed as a potential conflict of interest.
Publisher’s note
All claims expressed in this article are solely those of the authors and do not necessarily represent those of their affiliated organizations, or those of the publisher, the editors and the reviewers. Any product that may be evaluated in this article, or claim that may be made by its manufacturer, is not guaranteed or endorsed by the publisher.
Supplementary material
The Supplementary Material for this article can be found online at: https://www.frontiersin.org/articles/10.3389/fgene.2022.850108/full#supplementary-material
SUPPLEMENTARY FILE S1 | The laboratory animal ethics review approval of Yueyang Hospital of Integrated Traditional Chinese and Western Medicine of Shanghai University of Traditional Chinese Medicine Ethics Committee.
SUPPLEMENTARY TABLE S1 | PRG mRNA expression level of other skin disease-related datasets: atopic dermatitis, psoriatic arthritis, and acne).
SUPPLEMENTARY TABLE S2 | Differentially expressed mRNAs between PRGs-mediated cluster 1 and cluster 2 of psoriasis samples.
SUPPLEMENTARY FIGURE S1 | Differentially expressed mRNAs between psoriasis and normal samples. (A) Volcano plot of the training cohort of differentially expressed mRNAs. (B) Heatmap of the training cohort of differentially expressed mRNAs. (C) Volcano plot of the validation cohort of differentially expressed mRNAs. (D) Heatmap of the training cohort of differentially expressed mRNAs.
SUPPLEMENTARY FIGURE S2 | The gene annotation analysis of the landscape of 39 PRGs using Metascape. (A). The top-20 representative terms of gene annotation of PRGs. (B) A network based on subsets, colored by its terms ID. (C) A network based on subsets, colored by its p-values.
SUPPLEMENTARY FIGURE S3 | The CIBERSORT (A) and immune cell abundance (B) of psoriasis compared with normal samples.
References
Armstrong, A. W., Harskamp, C. T., and Armstrong, E. J. (2013). Psoriasis and metabolic syndrome: a systematic review and meta-analysis of observational studies. J. Am. Acad. Dermatol. 68, 654–662. doi:10.1016/j.jaad.2012.08.015
Barrett, T., Wilhite, S. E., Ledoux, P., Evangelista, C., Kim, I. F., Tomashevsky, M., et al. (2013). NCBI GEO: archive for functional genomics data sets--update. Nucleic Acids Res. 41, D991–D995. doi:10.1093/nar/gks1193
Benjamini, Y., and Hochberg, Y. (1995). Controlling the false discovery rate: A practical and powerful approach to multiple testing. J. Royal Stat. Soc. Ser. B 57, 289–300. doi:10.1111/J.2517-6161.1995.TB02031.X
Cai, Y., Xue, F., Quan, C., Qu, M., Liu, N., Zhang, Y., et al. (2019). A critical role of the IL-1β-IL-1R signaling pathway in skin inflammation and psoriasis pathogenesis. J. Invest. Dermatol. 139, 146–156. doi:10.1016/j.jid.2018.07.025
Chen, S., Mei, S., Luo, Y., Wu, H., Zhang, J., Zhu, J., et al. (2018). Gasdermin family: a promising therapeutic target for stroke. Transl. Stroke Res. 9, 555–563. doi:10.1007/s12975-018-0666-3
Chung, I.-C., Yuan, S.-N., OuYang, C.-N., Hu, S.-I., Lin, H.-C., Huang, K.-Y., et al. (2020). EFLA 945 restricts AIM2 inflammasome activation by preventing DNA entry for psoriasis treatment. Cytokine 127, 154951. doi:10.1016/j.cyto.2019.154951
Ciążyńska, M., Olejniczak-Staruch, I., Sobolewska-Sztychny, D., Narbutt, J., Skibińska, M., Lesiak, A., et al. (2021). The role of NLRP1, NLRP3, and AIM2 inflammasomes in psoriasis: review. Int. J. Mol. Sci. 22, 5898. doi:10.3390/ijms22115898
Demir Pektas, S., Pektas, G., Tosun, K., Dogan, G., Neselioglu, S., Erel, O., et al. (2018). Evaluation of erythroid disturbance and thiol-disulphide homeostasis in patients with psoriasis. Biomed. Res. Int. 2018, 9548252. doi:10.1155/2018/9548252
Deng, G., Chen, W., Wang, P., Zhan, T., Zheng, W., Gu, Z., et al. (2019). Inhibition of NLRP3 inflammasome-mediated pyroptosis in macrophage by cycloastragenol contributes to amelioration of imiquimod-induced psoriasis-like skin inflammation in mice. Int. Immunopharmacol. 74, 105682. doi:10.1016/j.intimp.2019.105682
Douville, N. J., Douville, C. B., Mentz, G., Mathis, M. R., Pancaro, C., Tremper, K. K., et al. (2021). Clinically applicable approach for predicting mechanical ventilation in patients with COVID-19. Br. J. Anaesth. 126, 578–589. doi:10.1016/j.bja.2020.11.034
Fang, Y., Tian, S., Pan, Y., Li, W., Wang, Q., Tang, Y., et al. (2020). Pyroptosis: a new frontier in cancer. Biomed. Pharmacother. 121, 109595. doi:10.1016/j.biopha.2019.109595
Georgescu, S.-R., Tampa, M., Caruntu, C., Sarbu, M.-I., Mitran, C.-I., Mitran, M.-I., et al. (2019). Advances in understanding the immunological pathways in psoriasis. Int. J. Mol. Sci. 20, 739. doi:10.3390/ijms20030739
Hong, W., Gu, Y., Guan, R., Xie, D., Zhou, H., Yu, M., et al. (2020). Pan-cancer analysis of the CASP gene family in relation to survival, tumor-infiltrating immune cells and therapeutic targets. Genomics 112, 4304–4315. doi:10.1016/j.ygeno.2020.07.026
Iizuka, H., Takahashi, H., Honma, M., and Ishida-Yamamoto, A. (2004). Unique keratinization process in psoriasis: late differentiation markers are abolished because of the premature cell death. J. Dermatol. 31, 271–276. doi:10.1111/j.1346-8138.2004.tb00672.x
Izadyyazdanabadi, M., Belykh, E., Mooney, M. A., Eschbacher, J. M., Nakaji, P., Yang, Y., et al. (2018). Prospects for theranostics in neurosurgical imaging: empowering confocal laser endomicroscopy diagnostics via deep learning. Front. Oncol. 8, 240. doi:10.3389/fonc.2018.00240
Jha, D., Ward, L., Paul, A., Liao, W., Choudhary, A., Wolverton, C., et al. (2018). ElemNet: deep learning the chemistry of materials from only elemental composition. Sci. Rep. 8, 17593. doi:10.1038/s41598-018-35934-y
Kenealy, S., Manils, J., Raverdeau, M., Munoz-Wolf, N., Barber, G., Liddicoat, A., et al. (2019). Caspase-11-Mediated cell death contributes to the pathogenesis of imiquimod-induced psoriasis. J. Invest.. Dermatol. 139, 2389. doi:10.1016/j.jid.2019.05.010
Kim, N., Lee, S., Kang, J., Choi, Y.-A., Jang, Y. H., Jeong, G.-S., et al. (2021). Cudraxanthone D ameliorates psoriasis-like skin inflammation in an imiquimod-induced mouse model via inhibiting the inflammatory signaling pathways. Molecules 26, 6086. doi:10.3390/molecules26196086
Kursa, M. B. Robustness of random forest-based gene selection methods. BMC Bioinf. 15, 8. doi:10.1186/1471-2105-15-8
Lachner, J., Mlitz, V., Tschachler, E., and Eckhart, L. (2017). Epidermal cornification is preceded by the expression of a keratinocyte-specific set of pyroptosis-related genes. Sci. Rep. 7, 17446. doi:10.1038/s41598-017-17782-4
Liberzon, A., Birger, C., Thorvaldsdóttir, H., Ghandi, M., Mesirov, J. P., Tamayo, P., et al. (2015). The Molecular Signatures Database (MSigDB) hallmark gene set collection. Cell Syst. 1, 417–425. doi:10.1016/j.cels.2015.12.004
Liu, X., and Lieberman, J. (2017). A mechanistic understanding of pyroptosis: the fiery death triggered by invasive infection. Adv. Immunol. 135, 81–117. doi:10.1016/bs.ai.2017.02.002
Lugrin, J., and Martinon, F. (2018). The AIM2 inflammasome: sensor of pathogens and cellular perturbations. Immunol. Rev. 281, 99–114. doi:10.1111/imr.12618
Michalek, I. M., Loring, B., and John, S. M. (2017). A systematic review of worldwide epidemiology of psoriasis. J. Eur. Acad. Dermatol. Venereol. 31, 205–212. doi:10.1111/jdv.13854
Moore, B. L., Aitken, S., and Semple, C. A. (2015). Integrative modeling reveals the principles of multi-scale chromatin boundary formation in human nuclear organization. Genome Biol. 16, 110. doi:10.1186/s13059-015-0661-x
Orning, P., Lien, E., and Fitzgerald, K. A. (2019). Gasdermins and their role in immunity and inflammation. J. Exp. Med. 216, 2453–2465. doi:10.1084/jem.20190545
Ramirez, V. P., Gurevich, I., and Aneskievich, B. J. (2012). Emerging roles for TNIP1 in regulating post-receptor signaling. Cytokine Growth Factor Rev. 23, 109–118. doi:10.1016/j.cytogfr.2012.04.002
Sessa, M., Khan, A. R., Liang, D., Andersen, M., and Kulahci, M. (2020). Artificial intelligence in pharmacoepidemiology: a systematic review. Part 1—overview of knowledge discovery techniques in artificial intelligence. Front. Pharmacol. 11, 1028. doi:10.3389/fphar.2020.01028
Shannon, P., Markiel, A., Ozier, O., Baliga, N. S., Wang, J. T., Ramage, D., et al. (2003). Cytoscape: a software environment for integrated models of biomolecular interaction networks. Genome Res. 13, 2498–2504. doi:10.1101/gr.1239303
Shi, J., Zhao, Y., Wang, K., Shi, X., Wang, Y., Huang, H., et al. (2015). Cleavage of GSDMD by inflammatory caspases determines pyroptotic cell death. Nature 526, 660–665. doi:10.1038/nature15514
Shou, Y., Yang, L., Yang, Y., and Xu, J. (2021). Inhibition of keratinocyte ferroptosis suppresses psoriatic inflammation. Cell Death Dis. 12, 1009. doi:10.1038/s41419-021-04284-5
Song, W., Ren, J., Xiang, R., Kong, C., and Fu, T. (2021). Identification of pyroptosis-related subtypes, the development of a prognosis model, and characterization of tumor microenvironment infiltration in colorectal cancer. Oncoimmunology 10, 1987636. doi:10.1080/2162402X.2021.1987636
Su, F., Xia, Y., Huang, M., Zhang, L., and Chen, L. (2018). Expression of NLPR3 in psoriasis is associated with enhancement of interleukin-1β and caspase-1. Med. Sci. Monit. 24, 7909–7913. doi:10.12659/MSM.911347
Szklarczyk, D., Morris, J. H., Cook, H., Kuhn, M., Wyder, S., Simonovic, M., et al. (2017). The STRING database in 2017: quality-controlled protein-protein association networks, made broadly accessible. Nucleic Acids Res. 45, D362–D368. doi:10.1093/nar/gkw937
Tang, Z.-L., Zhang, K., Lv, S.-C., Xu, G.-W., Zhang, J.-F., Jia, H.-Y., et al. (2021). LncRNA MEG3 suppresses PI3K/AKT/mTOR signalling pathway to enhance autophagy and inhibit inflammation in TNF-α-treated keratinocytes and psoriatic mice. Cytokine 148, 155657. doi:10.1016/j.cyto.2021.155657
Tangtatco, J. A. A., and Lara-Corrales, I. (2017). Update in the management of pediatric psoriasis. Curr. Opin. Pediatr. 29, 434–442. doi:10.1097/MOP.0000000000000517
Tran, T. M., Guha, R., Portugal, S., Skinner, J., Ongoiba, A., Bhardwaj, J., et al. (2019). A molecular signature in blood reveals a role for p53 in regulating malaria-induced inflammation. Immunity 51, 750. doi:10.1016/j.immuni.2019.08.009
Turner, C. T., Lim, D., and Granville, D. J. (2019). Granzyme B in skin inflammation and disease. Matrix Biol. 75 (76), 126–140. doi:10.1016/j.matbio.2017.12.005
Ungprasert, P., Srivali, N., and Thongprayoon, C. (2016). Association between psoriasis and chronic obstructive pulmonary disease: a systematic review and meta-analysis. J. Dermatol. Treat. 27, 316–321. doi:10.3109/09546634.2015.1107180
Verma, D., Fekri, S. Z., Sigurdardottir, G., Bivik Eding, C., Sandin, C., Enerbäck, C., et al. (2021). Enhanced inflammasome activity in patients with psoriasis promotes systemic inflammation. J. Invest.. Dermatol. 141, 586–595.e5. doi:10.1016/j.jid.2020.07.012
Wagner, R. D., Johnson, S. J., Danielsen, Z. Y., Lim, J.-H., Mudalige, T., Linder, S., et al. (2017). Polyethylene glycol-functionalized poly (Lactic Acid-co-Glycolic Acid) and graphene oxide nanoparticles induce pro-inflammatory and apoptotic responses in Candida albicans-infected vaginal epithelial cells. PLoS One 12, e0175250. doi:10.1371/journal.pone.0175250
Wang, Q., Wang, Y., Ding, J., Wang, C., Zhou, X., Gao, W., et al. (2020). A bioorthogonal system reveals antitumour immune function of pyroptosis. Nature 579, 421–426. doi:10.1038/s41586-020-2079-1
Xiang, Z., and Yang, J. (2020). The therapeutic potential of miR-135b in myocardial infarction: anti-inflammatory trials may be enlightening. Int. J. Cardiol. 312, 99. doi:10.1016/j.ijcard.2020.03.030
Yi, L., Wu, G., Guo, L., Zou, X., and Huang, P. (2020). Comprehensive analysis of the PD-L1 and immune infiltrates of m6A RNA methylation regulators in head and neck squamous cell carcinoma. Mol. Ther. Nucleic Acids 21, 299–314. doi:10.1016/j.omtn.2020.06.001
Yu, G., Wang, L.-G., Han, Y., and He, Q.-Y. (2012). clusterProfiler: an R Package for comparing biological themes among gene clusters. OMICS 16, 284–287. doi:10.1089/omi.2011.0118
Keywords: pyroptosis, pyroptosis-related genes, psoriasis, random forest algorithm, machine learning
Citation: Song J-K, Zhang Y, Fei X-Y, Chen Y-R, Luo Y, Jiang J-S, Ru Y, Xiang Y-W, Li B, Luo Y and Kuai L (2022) Classification and biomarker gene selection of pyroptosis-related gene expression in psoriasis using a random forest algorithm. Front. Genet. 13:850108. doi: 10.3389/fgene.2022.850108
Received: 07 January 2022; Accepted: 04 July 2022;
Published: 30 August 2022.
Edited by:
Huabing Li, Shanghai Jiao Tong University, ChinaReviewed by:
Ananta Paine, University of Rochester, United StatesAlejandro Caceres, Instituto Salud Global Barcelona (ISGlobal), Spain
Copyright © 2022 Song, Zhang, Fei, Chen, Luo, Jiang, Ru, Xiang, Li, Luo and Kuai. This is an open-access article distributed under the terms of the Creative Commons Attribution License (CC BY). The use, distribution or reproduction in other forums is permitted, provided the original author(s) and the copyright owner(s) are credited and that the original publication in this journal is cited, in accordance with accepted academic practice. No use, distribution or reproduction is permitted which does not comply with these terms.
*Correspondence: Yue Luo, moon_ms.luo@hotmail.com; Le Kuai, kuaile@shyueyanghospital.com
†These authors have contributed equally to this work