- 1Institute of Wheat Research, Shanxi Agricultural University/ State Key Laboratory of Sustainable Dryland Agriculture, Linfen, China
- 2College of Agricultural Economics and Management, Shanxi Agricultural University, Taiyuan, China
- 3Biotechnology Research Institute, Chinese Academy of Agricultural Sciences, Beijing, China
- 4Institute of Crop Science and Resource Conservation (INRES), Crop Science, University of Bonn, Bonn, Germany
- 5Department of Agronomy and Crop Physiology, Institute for Agronomy and Plant Breeding, Justus Liebig University Giessen, Giessen, Germany
Chlorophyll content of the flag leaf is an important trait for drought resistance in wheat under drought stress. Understanding the regulatory mechanism of flag leaf chlorophyll content could accelerate breeding for drought resistance. In this study, we constructed a recombinant inbred line (RIL) population from a cross of drought-sensitive variety DH118 and drought-resistant variety Jinmai 919, and analyzed the chlorophyll contents of flag leaves in six experimental locations/years using the Wheat90K single-nucleotide polymorphism array. A total of 29 quantitative trait loci (QTLs) controlling flag leaf chlorophyll were detected with contributions to phenotypic variation ranging from 4.67 to 23.25%. Twelve QTLs were detected under irrigated conditions and 18 were detected under dryland (drought) conditions. Most of the QTLs detected under the different water regimes were different. Four major QTLs (Qchl.saw-3B.2, Qchl.saw-5A.2, Qchl.saw-5A.3, and Qchl.saw-5B.2) were detected in the RIL population. Qchl.saw-3B.2, possibly more suitable for marker-assisted selection of genotypes adapted to irrigated conditions, was validated by a tightly linked kompetitive allele specific PCR (KASP) marker in a doubled haploid population derived from a different cross. Qchl.saw-5A.3, a novel stably expressed QTL, was detected in the dryland environments and explained up to 23.25% of the phenotypic variation, and has potential for marker-assisted breeding of genotypes adapted to dryland conditions. The stable and major QTLs identified here add valuable information for understanding the genetic mechanism underlying chlorophyll content and provide a basis for molecular marker–assisted breeding.
Introduction
Chlorophyll is the key element for photosynthesis, which captures light energy to drive electron transfer to its reaction center. Chlorophyll content is positively correlated with photosynthetic efficiency (Avenson et al., 2005), directly affecting the accumulation of photosynthates (Guo et al., 2008; Zhang et al., 2009a). Under abiotic stress situations such as drought, high temperature, salinization, and heavy metal presence, genotypes with higher chlorophyll content maintain higher photosynthetic capacity that helps to maintain higher yield achievement (Vijayalakshmi et al., 2010; Kumar et al., 2012; Ilyas et al., 2014; Talukder et al., 2014; Awlachew et al., 2016; Gupta et al., 2020; Bhoite et al., 2021; Borjigin et al., 2021). Photosynthetic activity in the flag leaves of wheat contributes about 50% to the grain yield (Verma et al., 2004; Zhu et al., 2016). Drought stress at the grain-filling stage is a common occurrence in wheat crops. This leads to accelerated degradation of chlorophyll in photosynthetic organs such as leaves, reduced photosynthetic rate, and decreased photosynthetic efficiency (Yang B. et al., 2016), hence lower fixation and assimilation of CO2 (Yang D. et al., 2016) leading to restricted dry matter accumulation and grain development (Farooq et al., 2014). Therefore, the chlorophyll content in flag leaves is regarded as an indicator of drought resistance in wheat under drought stress (Farooq et al., 2014; Barakat et al., 2015). Molecular studies on the genetic regulation of flag leaf chlorophyll content are therefore of considerable significance for maintaining and improving yield potential under drought stress conditions.
Synthesis and degradation of chlorophyll is a complex biological process, which not only involves many genes and cellular metabolic pathways, but is also readily affected by internal and external environments. Quantitative trait locus (QTL) analysis and gene cloning following construction of high-density genetic linkage maps is an effective way to study the genetics of chlorophyll (Verma et al., 2004; Thomas and Ougham, 2014; Rasheed et al., 2020). In rice, more than 900 QTLs affecting chlorophyll content have been identified by QTL mapping (Ye, 2016). More than 120 leaf color–related genes have been cloned (Yang et al., 2020), among which 14 were involved in chlorophyll synthesis. These included OsCAO1 encoding a chlorophyll oxygenase (Yang Y. et al., 2016); OsCHLH, OsCHLD, and OsCHLI encoding subunits of a magnesium-chelating enzyme (Jung et al., 2003; Zhou et al., 2012; Zhang et al., 2015); and YGL1 encoding a chlorophyll synthase (Liu et al., 2016). In addition, eight genes related to stay green were cloned in rice, including a DYE1-encoded light capture complex I subunit (Yamatani et al., 2018), EF8 encoding a HAP3 subunit of the HAP complex (Feng et al., 2014), and SGR that is involved in decomposition of chlorophyll (Morita et al., 2009). Some of these cloned genes have been successfully applied to rice breeding. Chen et al. (2020) found that overexpression of chloroplast gene D1 increased rice biomass by 20.6–22.9% and yield of transgenic rice by 8.1–21.0% under field conditions. Thus, identification of major QTLs/genes related to chlorophyll synthesis and degradation in grain crops could have application in wheat breeding.
The wheat genome is about 17 Gb, 80–90% of which are highly repetitive sequences. Studies on the genetic mechanisms regulating chlorophyll lag behind those in model crops such as rice (Sultana et al., 2021). Moreover, the studies that have been reported involved different wheat populations and growth stages (Zhang et al., 2009a; Ilyas et al., 2014; Yu et al., 2014; Yang B. et al., 2016). The 82 reported QTLs controlling chlorophyll content were distributed across all 21 chromosomes (Quarrie et al., 2006; Zhang et al., 2009a; Zhang et al., 2009b; Kumar et al., 2010; Vijayalakshmi et al., 2010; Ilyas et al., 2014; Saleh et al., 2014; Barakat et al., 2015; Li et al., 2015; Yang D. et al., 2016; Gupta et al., 2017; Shi et al., 2017; Yan et al., 2020).
As chlorophyll content is affected by water availability and environmental conditions, there are few stably expressed major QTLs (Yang D. et al., 2016). Most studies involved widely dispersed SSR markers and there are no reports on the application of QTL for chlorophyll content in wheat breeding. A few major stay green QTLs have been fine-mapped (Li et al., 2018; Wang et al., 2020a; Gupta et al., 2020). For example, the F2 population involving early senescence mutant M114 with significantly reduced chlorophyll content in flag leaves was analyzed by BSR-Seq, and the els1 gene was located in the WGGB303–WGGB305 marker interval of 2BS, with 1.5 cM genetic distance (Li et al., 2018). Wang et al. (2020b) analyzed the inheritance of F2 population constructed with premature senescence mutant LF2099 and Chinese Spring, and mapped the els2 gene to the marker interval of 2BIP09–2BIP14 on 2BL, and its genetic distance was 0.95 cM. There is no report on map-based cloning of genes regulating wheat chlorophyll content.
Genes Tackx4, Tabas1-B1, and TaPPH-7A contributing to chlorophyll content in wheat were identified by homologous cloning in wheat. Chang et al. (2015) cloned the Tackx4 allele encoding a cytokinin oxidase on chromosome 3A and validated it using a Jing411 × Hongmangchun 21 RIL population. A major QTL co-segregating with Tackx4 contributed 8.9–20.1% to chlorophyll content in four environments. Zhu et al. (2016) cloned Tabas1-B1 encoding 2-cys peroxiredoxin BAS1 on chromosome 2B and identified a major co-segregating QTL that contributed 9.0–19.2% of the variation in chlorophyll content in three environments. Wang et al. (2019) cloned TaPPH-7A encoding a pheophorbide hydrolase on chromosome 7A and found that it was closely related to the chlorophyll content of flag leaves in plants grown under drought stress. However, none of these genes was validated by transgenesis. Clearly, synthesis and degradation of chlorophyll is a complex biological process involving many genes, but currently only a few major QTLs and genes related to chlorophyll content have been reported in wheat. Thus, different research approaches and populations to map QTL are of value for a better understanding of the genetics of chlorophyll content.
In this study, the chlorophyll content of flag leaves was analyzed by QTL analysis of a DH118 × Jinmai 919 RIL population grown in six environments with different moisture conditions and validated in a Jinchun 7 × Jinmai 919 DH population to 1) identify stable QTLs that regulate chlorophyll content in flag leaves and 2) study the effects of contrasting moisture availability on the QTLs with the objective of obtaining markers for wheat breeding.
Materials and Methods
Plant Materials and Plot Design
The populations with Jinmai 919 as a same parent included 165 F10 RILs from cross DH118 × Jinmai 919 (DJ) and 168 doubled haploid (DH) lines from Jinchun 7 × Jinmai 919 (JJ). DH118, a high-yielding variety selected for irrigated conditions by the Institute of Wheat Research, Shanxi Agricultural University, has dark green leaves and high chlorophyll content (Figure 1A). Jinmai 919 developed by the Institute of Wheat Research, Shanxi Agricultural University, has strong drought resistance, light green leaves, and good stay green characteristics (Figure 1A). Bred by the Institute of Maize Research, Shanxi Academy of Agricultural Sciences, Jinchun 7 is also a high-yielding variety for irrigated conditions. The DJ population was used for QTL mapping, and the JJ population was used for validating QTLs identified in the mapping population.
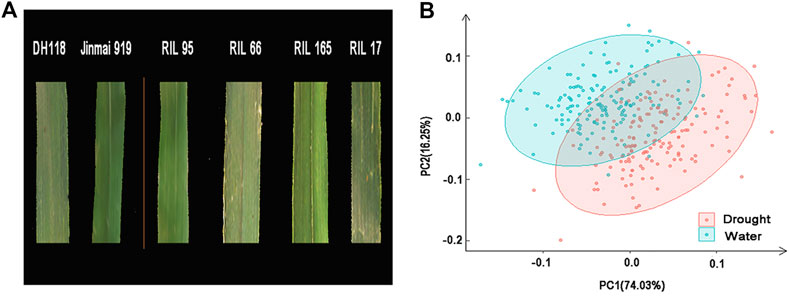
FIGURE 1. Phenotypic characteristic of the DJ population. (A) Phenotypes of the parents and selected RILs. (B) Principal components analysis (PCA) of chlorophyll content of flag leaves estimated for RILs grown under irrigated (water) and dryland (drought) conditions. Percentage variance accounted for by PC1 and PC2 is indicated in parentheses.
The DJ population was planted at Yaodu Experimental Station (36°08′N, 111°52′E, YD) and Hancun Experimental Station (36°25′N, 111°67′E, HC) at Linfen in Shanxi province in 2018–2019, 2019–2020, and 2020–2021. Plants were grown under irrigated and dryland (drought stressed) conditions in each year providing six environments designated as E1 (well-watered, 2019 YD), E2 (well-watered, 2020 YD), E3 (well-watered, 2021 YD), E4 (drought stressed, 2019 HC), E5 (drought stressed, 2020 HC), and E6 (drought stressed, 2021 HC). The JJ population was planted under the environmental conditions of E1, E2, E3, E4, and E5. The field design for both populations consisted of randomized complete blocks with three replications. Each plot consisted of two 1.5 m rows spaced 0.3 m apart at 21 seeds per row. After sowing, the Hancun site relied on natural precipitation during the whole growth period, 132 mm, 154 mm, and 147 mm in 2018–2019, 2019–2020, and 2020–2021, respectively; the Yaodu site was irrigated.
Phenotypic Evaluation and Data Analysis
Ten plants flowering on the same day were randomly selected from each line at 10 days after flowering. The chlorophyll contents of flag leaves were measured using a SPAD-502 (Konica-Minolta, Japan) chlorophyll meter at 7:00 to 10:00 h. Each leaf was measured three times—at the base, mid-region, and tip—and the average value was used for analysis (Yang B. et al., 2016). Average values were also determined for each environment.
SPSS 21.0 software (SPSS, Chicago, IL, USA; http://www.spss.com) was used to perform Student’s t-tests, correlation analysis, and ANOVA comparing phenotypic data from the two environments. SAS (SAS Institute, Cary, NC, USA; https://www.sas.com) was applied for calculating best linear unbiased predictions (BLUPs) and broad sense heritabilities (H2).
High-Density Genetic Linkage Map Construction and QTL Mapping
DNA was extracted from all RILs and DH lines and respective parents using the CTAB method (Vijayalakshmi et al., 2010). The RIL population was genotyped with the Infinium wheat SNP 90K iSelect assay (Illumina Inc., San Diego, CA, USA) developed by the International Wheat SNP Consortium (Wang et al., 2014). IciMapping v4.0 (https://www.isbreeding.net) was used to construct a high-density genetic linkage map (Li et al., 2021). SNP markers with no recombination were placed into a single bin using the “BIN” function in IciMapping V4.0. The final markers were chosen with a minimum percentage of missing data and sorted into different groups with LOD thresholds ≤8 by the “Grouping” function in JoinMap 4.0 (Li et al., 2021).
The QTLs were detected using WinQTLCart version 2.5 (https://brcwebportal.cos.ncsu.edu/qtlcart/WQTLCart.htm) based on the composite interval mapping method. QTLs were proclaimed significant at logarithm of odds (LOD) scores >2.5. The QTL contributing more than 10% to phenotypic variation in a certain environment (including BLUP) and detected in three environments (including BLUP) was considered as a stable and major QTL. QTLs less than 1 cM apart or sharing common flanking markers were treated as a single locus. The QTLs were named according to McCouch et al. (1997). The closest marker sequences flanking QTL were compared with the Chinese Spring reference genome sequence in the wheat multiomics website database (http://wheatomics.sdau.edu.cn/jbrowse-1.12.3-release/?data=Chinese_Spring1.0) to determine the physical locations of the QTL.
Marker Development and Validation of Major QTLs
To develop kompetitive allele specific PCR (KASP) tags from the peak marker SNP sequence of the major QTLs, two specific primers (F1/F2) and a universal primer (R) were designed for each SNP. An F1 tail that could bind to induce FAM fluorescence and an F2 tail that could bind to induce HEX fluorescence were added to the specific sequences. KASP primers were designed by Polymarker (http://www.polymarker.info/) and synthesized by Beijing Jiacheng Biotechnology Co., Ltd. (Supplementary Table S1). The developed KASP markers were used in PCR to detect previously identified QTLs in the JJ population as a means of validation. Following genotyping, the validation population was divided into two groups and differences in chlorophyll content of flag leaves between the groups were assessed by t-tests in SAS V8.0.
Gene Prediction Within QTL
Genes within the target region of major QTL were obtained using the genome browser (JBrowse) on the Triticeae Multi-omics website (http://wheatomics.sdau.edu.cn/). The GO (gene ontology) database and R package cluster profiler were applied for functional annotation and enrichment analysis of genes in the QTL regions. Identification of orthologs in wheat and rice was conducted using the Triticeae-Gene Tribe website (http://wheat.cau.edu.cn/TGT/). The expVIP public database (http://www.wheat-expression.com/) was used to search for expression data of genes in eight tissues and organs, perform log2 conversion processing, and analyze the expression patterns of candidate genes.
Results
Analysis of Phenotypic Data
The chlorophyll contents of flag leaves of DH118 and Jinmai 919 ranged from 52.16 to 60.22 and 48.80 to 58.42, respectively, across the six environments. The chlorophyll content of DH118 was consistently higher than that of Jinmai 919, and the difference was significant in E1 and E6 (p <0.05), and highly significant in E2 and E5 (p <0.01) (Table 1). The correlation of chlorophyll contents among different environments for the RIL population was highly significant (p <0.01), and correlation coefficients ranged from 0.303 to 0.711 (Supplementary Table S2). The H2 of chlorophyll content was 0.90, indicating that chlorophyll content was largely determined by genetic factors. Principal component analysis showed that environmental factors had considerable influence on phenotypic values, and drought stress increases the phenotypic variation (Figure 1B). Chlorophyll content of the RIL population was mostly between the two parents under E2, E3, E4, E5, and E6 environments, showing a continuous distribution. Bidirectional transgressive segregation was also observed in chlorophyll content among the RIL population under E1 condition (Table 1).
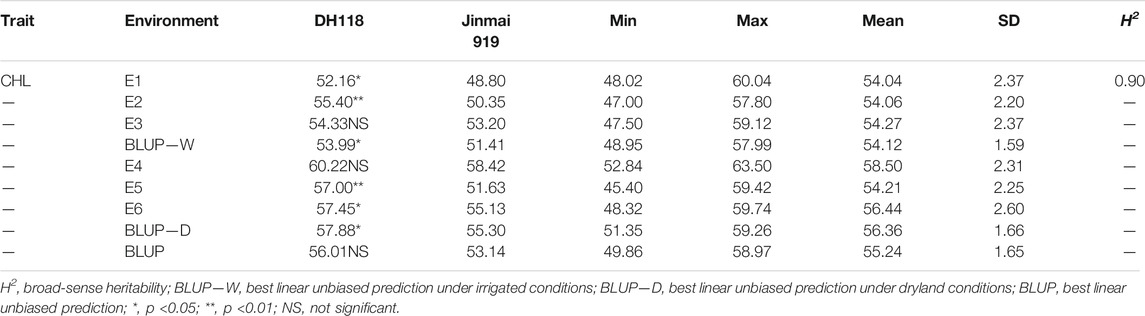
TABLE 1. Chlorophyll contents in flag leaves in parents and RILs derived from cross DH118 × Jinmai 919 in six environments
Linkage Map Construction
A high-density genetic linkage map for the RIL population was constructed by using Wheat90k SNP chip. The total length of the map was 5,858.63 cM with an average genetic distance of 1.65 cM, including 3,553 SNP markers and covering all 21 chromosomes (Table 2). The numbers of SNP markers in the A, B, and D genomes were 1,395, 1,880, and 278, respectively, and the linkage lengths were 2,394.29, 2,953.31, and 511.03 cM, with average distances between markers of 1.72, 1.57, and 1.84 cM, respectively (Table 2). The D genome had the lowest marker coverage; the longest linkage group was 673.66 cM for chromosome 5B, and the shortest was 23.30 cM for chromosome 4D.
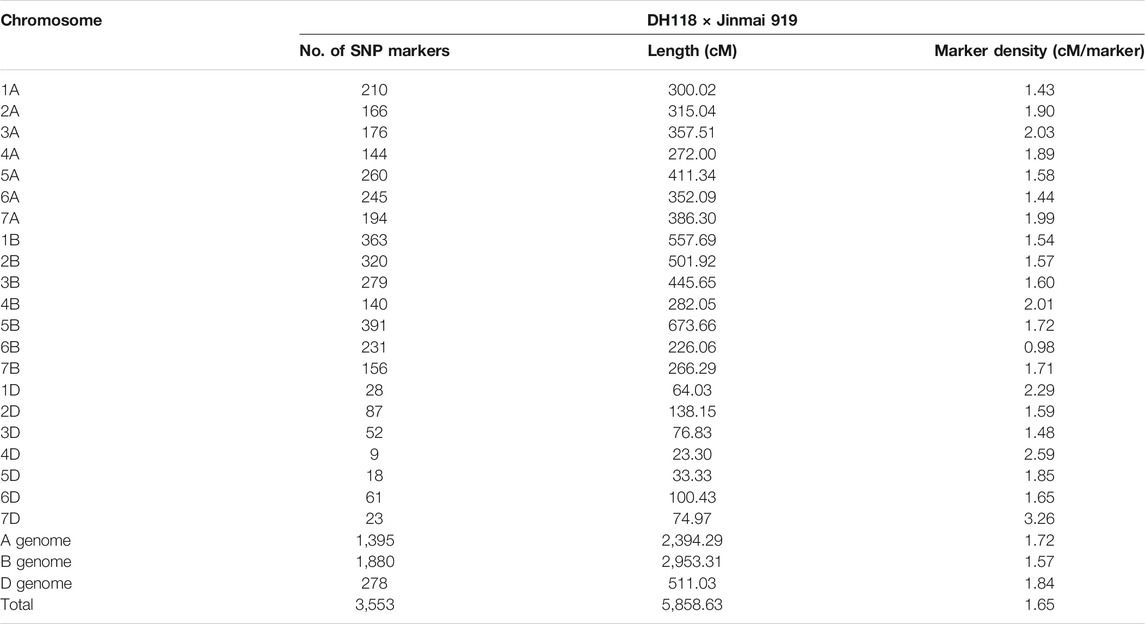
TABLE 2. Summary of linkage group and marker statistics obtained from a 90K SNP chip analysis of the DH118×Jinmai 919 RIL population
QTL Mapping for Chlorophyll Content under Different Environments
A total of 29 QTLs for chlorophyll content were detected on chromosomes 1B, 2A, 2B, 2D, 3A, 3B, 4B, 5A, 5B, 6B, 7A, and 7B. The LOD scores ranged from 2.58 to 10.70 and individual QTL explained 4.67–23.25% of the phenotypic variation in different environments (Table 3). Favorable alleles of 20 QTLs were derived from DH118 and favorable alleles of 9 QTLs were derived from Jinmai 919.
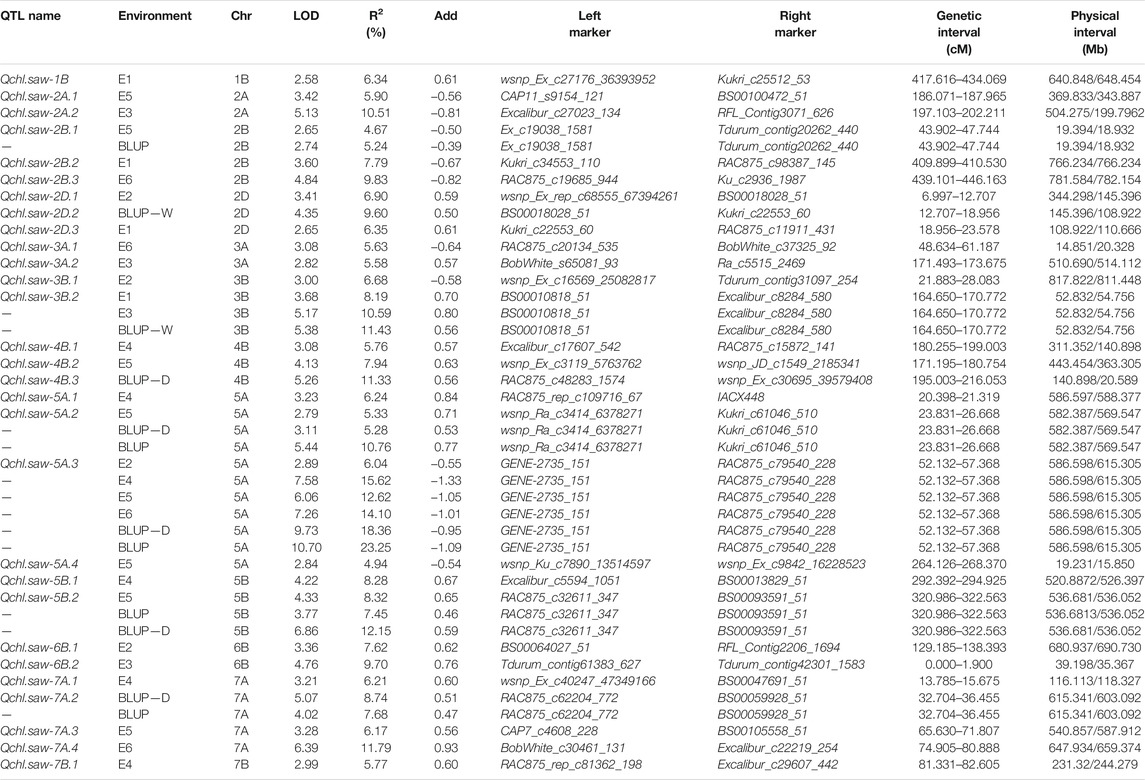
TABLE 3. Quantitative trait loci (QTL) for chlorophyll content detected in the DH118 × Jinmai 919 RIL population grown under different water regimes
Four major QTLs (Qchl.saw-3B.2, Qchl.saw-5A.2, Qchl.saw-5A.3, and Qchl.saw-5B.2) for chlorophyll content were detected on chromosomes 3B, 5A, and 5B, respectively. Qchl.saw-5A.3 was detected in E2, E4, E5, E6, BLUP—D, and BLUP. The LOD values ranged from 2.89 to 10.70 and the QTL explained 6.04–23.25% of the phenotypic variation. The positive allele for Qchl.saw-5A.3 was contributed by Jinmai 919 (Table 3). Qchl.saw-3B.2 was detected in E1, E3, and BLUP—W, explaining 8.19–11.43% of the phenotypic variation. Qchl.saw-5A.2 was detected in E5, BLUP—D, and BLUP, explaining 5.28–10.76% of the phenotypic variation (Table 3). Qchl.saw-5B.2 was detected in E5, BLUP—D, and BLUP, and explained 7.45–12.15% of the phenotypic variation. The positive alleles for Qchl.saw-3B.2, Qchl.saw-5A.2, and Qchl.saw-5B.2 were contributed by DH118 (Table 3).
Additive Effects of the Major QTLs Qchl.saw-3B.2, Qchl.saw-5A.2, Qchl.saw-5A.3, and Qchl.saw-5B.2 on Chlorophyll Content
Analysis of the additive effects of the four major QTLs showed that the number of favorable alleles increased chlorophyll content (Figure 2A, Supplementary Table S3). No RIL with all four favorable alleles was detected. The average chlorophyll content of RILs with three favorable alleles increased by 3.11–3.81 (5.91–7.24%) compared with RILs with no favorable allele. Among combinations, the average chlorophyll content of RILs with favorable alleles of Qchl.saw-3B.2, Qchl.saw-5A.2, and Qchl.saw-5A.3 was the highest at 7.24% above that of lines with no favorable allele (Supplementary Table S3). In addition, the average chlorophyll content of lines with only Qchl.saw-5A.3 allele in RIL population was higher than that of other lines with only one favorable allele (Figure 2B), indicating that the allele of Qchl.saw-5A.3 had the highest genetic effect on chlorophyll content.
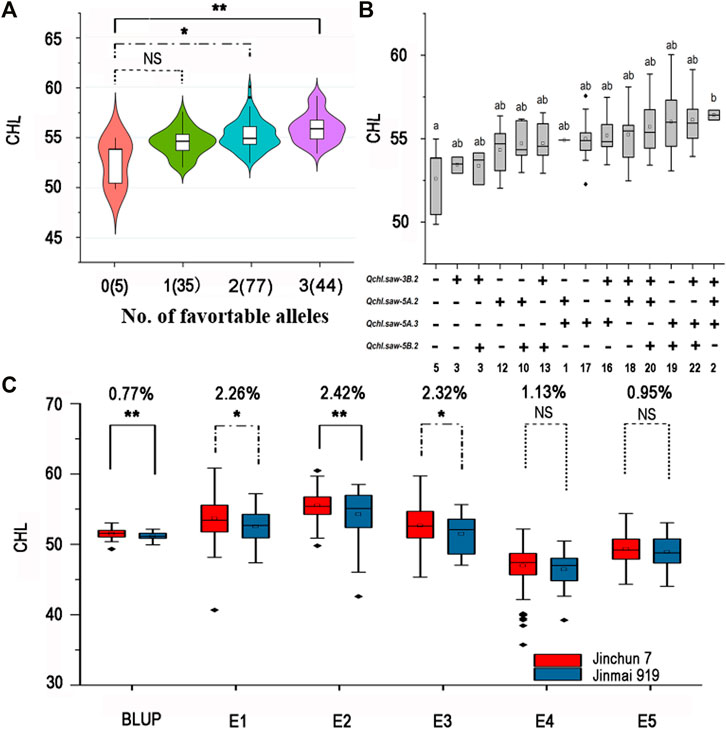
FIGURE 2. Additive effects and validation of major QTL. (A) Relationship of numbers of favorable alleles and chlorophyll content in the DJ population. (B) Linear regressions between the additive effects of QTL and chlorophyll content in the DJ population. Numbers of lines carrying the corresponding number of favorable alleles are shown in brackets. The letter above the bars indicates comparison results at the significant level 0.05 and respectively. “+” and “−” represent lines with and without the favorable alleles. (C) Validation of Qchl.saw-3B.2 in JJ population. * and ** represent significance at p < 0.05 and p < 0.01, respectively. NS represents not significant.
Validation of the Major QTL Qchl.saw-3B.2 in the JJ Population
To validate the four major QTLs, KASP markers for each QTL were used to evaluate their effects on chlorophyll content in the JJ population. The KASP markers for Qchl.saw-5A.2 and Qchl.saw-5A.3 were not polymorphic between Jinchun 7 and Jinmai 919. The effect of Qchl.saw-5B.2 did not differ significantly between two contrasting phenotypic groups in JJ population. The effect of Qchl.saw-3B.2 was significant (p <0.05) in E1 and E3, and highly significant (p <0.01) in E2 (Figure 2C). The chlorophyll content of lines with the favorable Qchl.saw-3B.2 allele was higher than that without this allele, and the difference varied from 0.95 to 2.26% across environments.
Candidate Genes in the Intervals of the Four Major QTLs
A total of 1,207 genes were identified in the four major QTLs; 73 genes in Qchl.saw-3B.2 (52.83–54.76 Mb), 368 in Qchl.saw-5A.2 (569.55–582.39 Mb), 735 in Qchl.saw-5A.3 (586.59–615.30 Mb), and 31 in Qchl.saw-5B.2 (536.05–536.68 Mb) (Table 3; Supplementary Table S4). According to gene functional annotations in the Gene Ontology (GO) public database, 174 of these genes are involved in chlorophyll metabolism and drought stress (Supplementary Table S5; Supplementary Figure S1). Analysis of gene expression in various tissues identified 18 candidate genes related to chlorophyll metabolism (Table 4).
These 18 genes were divided into three categories according to their function. The first category was related to the composition of chloroplasts. TraesCS5A02G420700 related to chloroplast thylakoid membrane, and TraesCS5A02G377000 related to chloroplast membrane formation and the homologous gene TraesCS5A02G423000 of pRRFNR14 (Os03g0784700) in rice involved in the process of chloroplast composition (Aoki and Ida, 1994). The second category was related to eight new genes of chlorophyll photosynthesis, including TraesCS5A02G414400, TraesCS5A02G378700 (OsLOX2), TraesCS5A02G373600, TraesCS5A02G424100, TraesCS5A02G376700, TraesCS5A02G369500, TraesCS5A02G392300, and TraesCS5B02G356300 (OsUgp1) (Huang et al., 2014; E et al., 2015). These genes participated in photosystem I reaction center subunit III, ATP binding, metal ion binding, and transferase activity. The third kind of genes responded to drought stress by regulating photorespiration, mediating auxin response, and participating in the regulation of ABA signal transduction pathway, such as rice homologous gene GLO1, OsIAA13/OsIAA1, OsSAPK8, and OsUBC9 (Thakur et al., 2001; Zhang et al., 2012; Xu et al., 2013; E et al., 2015). We also identified three novel genes TraesCS5A02G411200, TraesCS5A02G374500, and TraesCS5A02G426100 that responded to drought stress by redox reaction, activation of enzyme activity, and ATP binding (Table 4).
Discussion
Comparison with Previous Research Results
According to reviews by Gupta et al. (2017, 2020), a total of 82 QTLs controlling chlorophyll content were identified in previous studies. These QTLs were distributed across all 21 chromosomes and explained 2.7–59.1% of the phenotypic variation, but most of these QTLs were different. The reasons could be due to 1) different methods of chlorophyll measurement that cause differences in phenotypic values, e.g., some studies used a spectrophotometer (Zhang et al., 2009b) and others used a chlorophyll meter, leading to differences in QTL analysis results (Bhusal et al., 2018); 2) chlorophyll content is a complex quantitative trait and genes controlling leaf chlorophyll are expressed differently at different developmental stages (Yang D. et al., 2016), and different measurement periods will inevitably lead to different identified genes; 3) due to different types of populations and molecular markers, it is not easy to compare results across different genetic backgrounds.
In this study, 29 QTLs controlling chlorophyll content in flag leaves were located on 12 chromosomes, most of which were A and B genome chromosomes with only three detected in the D genome. Similar results were reported in previous studies (Zhang et al., 2009b; Yang D. et al., 2016). We detected four stably expressed major QTLs on chromosomes 3B (Qchl.saw-3B.2), 5A (Qchl.saw-5A.2 and Qchl.saw-5A.3), and 5B (Qchl.saw-5B.2), with contribution rates of 5.28–23.25% to the variation in chlorophyll content. These QTLs still need further validation before application in marker-assisted selection (Ahmed et al., 2021).
Fourteen, seven, and nine QTLs for chlorophyll content were located on chromosomes 3B, 5A, and 5B, respectively, in previous studies (Table 5). The three major QTLs controlling chlorophyll content of flag leaves identified in our study were consistent with results of previous studies. The major QTL Qchl.saw-3B.2 on chromosome 3B was in the interval 52.83–54.75 Mb. Kumar et al. (2010) reported a major QTL QSg.bhu-3B for flag leaf senescence in the same region, explaining 17.9% of the variation in stay green phenotypic, and Puttamadanayaka et al. (2020) reported QChl.iari_3B that controlled chlorophyll content. The QTLs in our study spanned shorter physical distances and are therefore more conducive for gene cloning. Qchl.saw-5A.2 was in the range 569.54–582.38 Mb. Puttamadanayaka et al. (2020) reported QChl.iari_5A for chlorophyll content spanned by AX-94531685 (567.52 Mb) and AX-94726381 (582.96 Mb). In the same region, Wang et al. (2017) detected three major QTLs controlling 1,000-grain weight, and their adjacent markers were BS00073670_51, wsnp_Ex_c1138_2185522, and Tdurum_contig71499_211, respectively. Yang et al. (2019) cloned a TaGL3-5A allele that conferred larger grain size based on homology with rice. Many studies have confirmed the high correlation between chlorophyll content and yield-related traits (Zhang et al., 2009b; Vijayalakshmi et al., 2010). Although there was no investigation of yield-related traits in this study, we have co-located QTL/genes for chlorophyll content, 1,000-grain weight, and grain size in the same interval with previous studies and confirmed the correlation between chlorophyll content and yield-related traits. The major QTL Qchl.saw-5B.2 on chromosome 5B was located in the interval 536.05–536.68 Mb, which coincided with chlorophyll content QTL Qspad.acs-5B.4 spanned by Xwmc415 and Xwmc508 reported by Yang et al. (2016). Qchl.saw-5A.3 with the strongest genetic effect in our study was in the chromosome 5A interval 586.59–615.30 Mb (Figures 3A–C). Given no previous report of gene for chlorophyll content in this interval, Qchl.saw-5A.3 is a novel QTL.
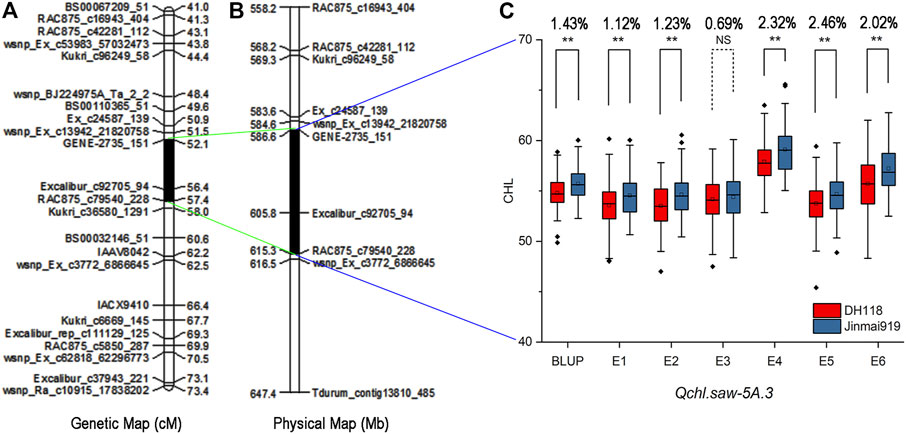
FIGURE 3. Genetic map of the major QTL Qchl.saw-5A.3 and its effect. (A) Genetic map of Qchl.saw-5A.3 for chlorophyll content. (B) Physical map of flanking markers of Qchl.saw-5A.3. (C) Effect of the QTL shown as box plots calculated after dividing the DJ population into two classes based on the flanking markers. * and **, p <0.05 and p <0.01, respectively; NS, not significant.
Effect of Environment on Expression of QTL for Chlorophyll Content
Synthesis and degradation of chlorophyll are complex biological processes and regulation likely differs under different water regimes (Yang D. et al., 2016). Under irrigated conditions, higher chlorophyll content could ensure fixation of more photosynthetic assimilates (Zhang et al., 2009b). Under drought stress conditions, stay green is closely related to higher yield (Verma et al., 2004; Thomas and Ougham, 2014). Drought-tolerant genotypes usually have higher chlorophyll content, and chlorophyll degrades more slowly under drought stress (Kumar et al., 2012; Lopes and Reynolds, 2012).
In this study, QTL analysis of chlorophyll content in flag leaves under irrigated and dryland (drought stressed) conditions was made using a RIL population derived from a cross between a variety DH118 recommended for irrigated conditions and drought-resistant variety Jinmai 919. Twelve QTLs were detected under irrigated conditions (E1, E2, E3 and BULP—W), and 18 QTLs were identified under drought stress (E4, E5, E6 and BULP—D) (Table 3). The number of QTLs under drought stress was much more than that under well-watered conditions, showing that environmental stress could induce to express genes originally keeping silent under irrigated conditions to reduce plant damages from environmental stress (Yang et al., 2007; Guo et al., 2008; Vijayalakshmi et al., 2010; Christopher et al., 2018). In addition, it was not difficult to find that there were some differences in QTL mapping data between the well-watered and drought stress, which implied that there were different QTL expression patterns under different water regimes (Yang et al., 2007; Yang B. et al., 2016; Xu et al., 2017; Hassan et al., 2018; Christopher et al., 2021). It also implies that different QTLs should be used for marker-assisted breeding of wheat varieties under irrigated conditions and dryland. For example, the Qchl.saw-3B.2 detected in this study was not only confirmed to be stably expressed without the influence of genetic background, but also detected under several well-watered conditions, which may be more suitable for molecular marker–assisted selection of varieties under irrigated conditions. In addition, Kumar et al. (2012) and Hassan et al. (2018) considered that the major QTL detected under drought stress may contain genes that contribute to drought resistance and have the application potential to increase yield under drought stress. In our study, three major QTLs (Qchl.saw-5A.2, Qchl.saw-5A.3, and Qchl.saw-5B.2) were detected in drought stress environments. Qchl.saw-5A.3 could be detected in all drought stress environments (E4, E5, and E6), and the contribution rate to phenotype was 6.04–23.25% (Table 3), which may be more suitable for marker-assisted selection breeding of drought-resistant varieties. In short, this study used high-density chips for QTL mapping, and the SNP and KASP markers of four major QTLs could be applied to the next development of molecular markers under different water conditions.
Data Availability Statement
The datasets presented in this study can be found in online repositories. The names of the repository/repositories and accession number(s) can be found in the article/Supplementary Material.
Author Contributions
SY, LQ, and JuZ designed the experiment and wrote the article. BY and XW carried out the experiments. HW and YF analyzed the data. JiZ, BW, XZ, and CY did the field experiments. All authors contributed to the article and approved the final article to publish.
Funding
This study was supported by the Research Program Sponsored by State Key Laboratory of Sustainable Dryland Agriculture (No. 202001-3), the Science Research of Shanxi Academy of Agricultural Sciences (No. YGJPY 2008), the Basic Research Plan of Shanxi Province (No. 20210302124505), the Project funded by Shanxi Key Laboratory of Crop Genetics and Molecular Improvement (No. KFJJ 2019-02), and the Agricultural Science Research of Shanxi Academy of Agricultural Sciences (YCX2020YQ34).
Conflict of Interest
The authors declare that the research was conducted in the absence of any commercial or financial relationships that could be construed as a potential conflict of interest.
Publisher’s Note
All claims expressed in this article are solely those of the authors and do not necessarily represent those of their affiliated organizations, or those of the publisher, the editors, and the reviewers. Any product that may be evaluated in this article, or claim that may be made by its manufacturer, is not guaranteed or endorsed by the publisher.
Acknowledgments
We thank Jianli Chen of the University of Idaho for critical advice on the design of these experiments.
Supplementary Material
The Supplementary Material for this article can be found online at: https://www.frontiersin.org/articles/10.3389/fgene.2022.832898/full#supplementary-material
References
Ahmed, A. A. M., Mohamed, E. A., Hussein, M. Y., and Sallam, A. (2021). Genomic Regions Associated with Leaf Wilting Traits under Drought Stress in Spring Wheat at the Seedling Stage Revealed by GWAS. Environ. Exp. Bot. 184, 104393. doi:10.1016/j.envexpbot.2021.104393
Aoki, H., and Ida, S. (1994). Nucleotide Sequence of a Rice Root Ferredoxin-Nadp+ Reductase cDNA and its Induction by Nitrate. Biochim. Biophys. Acta (Bba) - Bioenerg. 1183, 553–556. doi:10.1016/0005-2728(94)90085-x
Avenson, T. J., Cruz, J. A., Kanazawa, A., and Kramer, D. M. (2005). Regulating the Proton Budget of Higher Plant Photosynthesis. Proc. Natl. Acad. Sci. 102, 9709–9713. doi:10.1073/pnas.0503952102
Awlachew, Z. T., Singh, R., Kaur, S., Bains, N. S., and Chhuneja, P. (2016). Transfer and Mapping of the Heat Tolerance Component Traits of Aegilops speltoides in Tetraploid Wheat Triticum durum. Mol. Breed. 36, 78. doi:10.1007/s11032-016-0499-2
Ballesteros, D. C., Mason, R. E., Addison, C. K., Andrea Acuña, M., Nelly Arguello, M., Subramanian, N., et al. (2015). Tolerance of Wheat to Vegetative Stage Soil Waterlogging Is Conditioned by Both Constitutive and Adaptive QTL. Euphytica 201, 329–343. doi:10.1007/s10681-014-1184-3
Barakat, M., Saleh, M., Al-Doss, A., Moustafa, K., Elshafei, A., and Al-Qurainy, F. (2015). Identification of New SSR Markers Linked to Leaf Chlorophyll Content, Flag Leaf Senescence and Cell Membrane Stability Traits in Wheat under Water Stressed Condition. Acta Biologica Hungarica 66, 93–102. doi:10.1556/ABiol.66.2015.1.8
Bhoite, R., Si, P., Siddique, K. H. M., and Yan, G. (2021). Comparative Transcriptome Analyses for Metribuzin Tolerance Provide Insights into Key Genes and Mechanisms Restoring Photosynthetic Efficiency in Bread Wheat (Triticum aestivum L.). Genomics 113, 910–918. doi:10.1016/j.ygeno.2021.02.004
Bhusal, N., Sharma, P., Sareen, S., and Sarial, A. K. (2018). Mapping QTLs for Chlorophyll Content and Chlorophyll Fluorescence in Wheat under Heat Stress. Biol. Plant 62, 721–731. doi:10.1007/s10535-018-0811-6
Borjigin, C., Schilling, R. K., Jewell, N., Brien, C., Sanchez-Ferrero, J. C., Eckermann, P. J., et al. (2021). Identifying the Genetic Control of Salinity Tolerance in the Bread Wheat Landrace Mocho de Espiga Branca. Funct. Plant Biol. 48, 1148–1160. doi:10.1071/fp21140
Chang, C., Lu, J., Zhang, H.-P., Ma, C.-X., and Sun, G. (2015). Copy Number Variation of Cytokinin Oxidase Gene Tackx4 Associated with Grain Weight and Chlorophyll Content of Flag Leaf in Common Wheat. Plos One 10, e0145970. doi:10.1371/journal.pone.0145970
Chen, J.-H., Chen, S.-T., He, N.-Y., Wang, Q.-L., Zhao, Y., Gao, W., et al. (2020). Nuclear-Encoded Synthesis of the D1 Subunit of Photosystem II Increases Photosynthetic Efficiency and Crop Yield. Nat. Plants 6, 570–580. doi:10.1038/s41477-020-0629-z
Christopher, M., Chenu, K., Jennings, R., Fletcher, S., Butler, D., Borrell, A., et al. (2018). QTL for Stay-Green Traits in Wheat in Well-Watered and Water-Limited Environments. Field Crops Res. 217, 32–44. doi:10.1016/j.fcr.2017.11.003
Christopher, M., Paccapelo, V., Kelly, A., Macdonald, B., Hickey, L., Richard, C., et al. (2021). QTL Identified for Stay-Green in a Multi-Reference Nested Association Mapping Population of Wheat Exhibit Context Dependent Expression and Parent-Specific Alleles. Field Crops Res. 270, 108181. doi:10.1016/j.fcr.2021.108181
E, Z., Zhang, Y., Li, T., Wang, L., and Zhao, H. (2015). Characterization of the Ubiquitin-Conjugating Enzyme Gene Family in Rice and Evaluation of Expression Profiles under Abiotic Stresses and Hormone Treatments. Plos One 10, e0122621. doi:10.1371/journal.pone.0122621
Farooq, M., Hussain, M., and Siddique, K. H. M. (2014). Drought Stress in Wheat during Flowering and Grain-Filling Periods. Crit. Rev. Plant Sci. 33, 331–349. doi:10.1080/07352689.2014.875291
Feng, Z., Zhang, L., Yang, C., Wu, T., Lv, J., Chen, Y., et al. (2014). EF8 Is Involved in Photoperiodic Flowering Pathway and Chlorophyll Biogenesis in Rice. Plant Cell Rep 33, 2003–2014. doi:10.1007/s00299-014-1674-8
Guo, P., Baum, M., Varshney, R. K., Graner, A., Grando, S., and Ceccarelli, S. (2008). QTLs for Chlorophyll and Chlorophyll Fluorescence Parameters in Barley under Post-Flowering Drought. Euphytica 163, 203–214. doi:10.1007/s10681-007-9629-6
Gupta, P. K., Balyan, H. S., and Gahlaut, V. (2017). QTL Analysis for Drought Tolerance in Wheat: Present Status and Future Possibilities. Agronomy 7, 5. doi:10.3390/agronomy7010005
Gupta, P. K., Balyan, H. S., Sharma, S., and Kumar, R. (2020). Genetics of Yield, Abiotic Stress Tolerance and Biofortification in Wheat (Triticum aestivum L.). Theor. Appl. Genet. 133, 1569–1602. doi:10.1007/s00122-020-03583-3
Hassan, F. S. C., Solouki, M., Fakheri, B. A., Nezhad, N. M., and Masoudi, B. (2018). Mapping QTLs for Physiological and Biochemical Traits Related to Grain Yield under Control and Terminal Heat Stress Conditions in Bread Wheat (Triticum aestivum L.). Physiol. Mol. Biol. Plants 24, 1231–1243. doi:10.1007/s12298-018-0590-8
Huang, J., Cai, M., Long, Q., Liu, L., Lin, Q., Jiang, L., et al. (2014). OsLOX2, a Rice Type I Lipoxygenase, Confers Opposite Effects on Seed Germination and Longevity. Transgenic Res. 23, 643–655. doi:10.1007/s11248-014-9803-2
Ilyas, M., Ilyas, N., Arshad, M., Kazi, A. G., Kazi, A. M., and Waheed, A. (2014). QTL Mapping of Wheat Doubled Haploids for Chlorophyll Content and Chlorophyll Fluorescence Kinetics under Drought Stress Imposed at Anthesis Stage. Pak. J. Bot. 46, 1889–1897.
Jung, K.-H., Hur, J., Ryu, C.-H., Choi, Y., Chung, Y.-Y., Miyao, A., et al. (2003). Characterization of a Rice Chlorophyll-Deficient Mutant Using the T-DNA Gene-Trap System. Plant Cell Physiol 44, 463–472. doi:10.1093/pcp/pcg064
Kumar, S., Sehgal, S. K., Kumar, U., Prasad, P. V. V., Joshi, A. K., and Gill, B. S. (2012). Genomic Characterization of Drought Tolerance-Related Traits in Spring Wheat. Euphytica 186, 265–276. doi:10.1007/s10681-012-0675-3
Kumar, U., Joshi, A. K., Kumari, M., Paliwal, R., Kumar, S., and Röder, M. S. (2010). Identification of QTLs for Stay Green Trait in Wheat (Triticum aestivum L.) in the 'Chirya 3' × 'Sonalika' Population. Euphytica 174, 437–445. doi:10.1007/s10681-010-0155-6
Li, F., Wen, W., Liu, J., Zhai, S., Cao, X., Liu, C., et al. (2021). Genome-Wide Linkage Mapping for Canopy Activity Related Traits Using Three RIL Populations in Bread Wheat. Euphytica 217, 67. doi:10.1007/s10681-021-02797-w
Li, H., Tong, Y., Li, B., Jing, R., Lu, C., and Li, Z. (2010). Genetic Analysis of Tolerance to Photo-Oxidative Stress Induced by High Light in Winter Wheat (Triticum aestivum L.). J. Genet. Genomics 37, 399–412. doi:10.1016/s1673-8527(09)60058-8
Li, H., Wang, G., Zheng, Q., Li, B., Jing, R., and Li, Z. (2014). Genetic Analysis of Biomass and Photosynthetic Parameters in Wheat Grown in Different Light Intensities. J. Integr. Plant Biol. 56, 594–604. doi:10.1111/jipb.12174
Li, H., Ye, G., and Wang, J. (2007). A Modified Algorithm for the Improvement of Composite Interval Mapping. Genetics 175, 361–374. doi:10.1534/genetics.106.066811
Li, M., Li, B., Guo, G., Chen, Y., Xie, J., Lu, P., et al. (2018). Mapping a Leaf Senescence Gene Els1 by BSR-Seq in Common Wheat. Crop J. 6, 236–243. doi:10.1016/j.cj.2018.01.004
Li, X.-M., He, Z.-H., Xiao, Y.-G., Xia, X.-C., Trethowan, R., Wang, H.-J., et al. (2015). QTL Mapping for Leaf Senescence-Related Traits in Common Wheat under Limited and Full Irrigation. Euphytica 203, 569–582. doi:10.1007/s10681-014-1272-4
Liu, H., Li, Q., Yang, F., Zhu, F., Sun, Y., Tao, Y., et al. (2016). Differential Regulation of Protochlorophyllide Oxidoreductase Abundances by VIRESCENT 5A (OsV5A) and VIRESCENT 5B (OsV5B) in Rice Seedlings. Plant Cell Physiol 57, 2392–2402. doi:10.1093/pcp/pcw151
Lopes, M. S., and Reynolds, M. P. (2012). Stay-Green in Spring Wheat Can Be Determined by Spectral Reflectance Measurements (Normalized Difference Vegetation Index) Independently from Phenology. J. Exp. Bot. 63, 3789–3798. doi:10.1093/jxb/ers071
Maulana, F., Huang, W., Anderson, J. D., and Ma, X.-F. (2020). Genome-Wide Association Mapping of Seedling Drought Tolerance in Winter Wheat. Front. Plant Sci. 11, 573786. doi:10.3389/fpls.2020.573786
Mccouch, S. R., Chen, X., Panaud, O., Temnykh, S., Xu, Y., Cho, Y. G., et al. (1997). Microsatellite Marker Development, Mapping and Applications in Rice Genetics and Breeding. Plant Mol. Biol. 35, 89–99. doi:10.1023/a:100571143147410.1007/978-94-011-5794-0_9
Morita, R., Sato, Y., Masuda, Y., Nishimura, M., and Kusaba, M. (2009). Defect in Non-Yellow Coloring 3, an α/β Hydrolase-Fold Family Protein, Causes a Stay-Green Phenotype during Leaf Senescence in Rice. Plant J. 60, 1110. doi:10.1111/j.1365-313X.2009.04019.x
Puttamadanayaka, S., Harikrishna, , Balaramaiah, M., Biradar, S., Parmeshwarappa, S. V., Sinha, N., et al. (2020). Mapping Genomic Regions of Moisture Deficit Stress Tolerance Using Backcross Inbred Lines in Wheat (Triticum aestivum L.). Sci. Rep. 10, 21646. doi:10.1038/s41598-020-78671-x
Quarrie, S., Pekic Quarrie, S., Radosevic, R., Rancic, D., Kaminska, A., Barnes, J. D., et al. (2006). Dissecting a Wheat QTL for Yield Present in a Range of Environments: from the QTL to Candidate Genes. J. Exp. Bot. 57, 2627–2637. doi:10.1093/jxb/erl026
Rasheed, A., Takumi, S., Hassan, M. A., Imtiaz, M., Ali, M., Morgunov, A. I., et al. (2020). Appraisal of Wheat Genomics for Gene Discovery and Breeding Applications: a Special Emphasis on Advances in Asia. Theor. Appl. Genet. 133, 1503–1520. doi:10.1007/s00122-019-03523-w
Saleh, M. S., Al-Doss, A. A., Elshafei, A. A., Moustafa, K. A., Al-Qurainy, F. H., and Barakat, M. N. (2014). Identification of New TRAP Markers Linked to Chlorophyll Content, Leaf Senescence, and Cell Membrane Stability in Water-Stressed Wheat. Biol. Plant 58, 64–70. doi:10.1007/s10535-013-0351-z
Shi, S., Azam, F. I., Li, H., Chang, X., Li, B., and Jing, R. (2017). Mapping QTL for Stay-Green and Agronomic Traits in Wheat under Diverse Water Regimes. Euphytica 213, 246. doi:10.1007/s10681-017-2002-5
Shirdelmoghanloo, H., Taylor, J. D., Lohraseb, I., Rabie, H., Brien, C., Timmins, A., et al. (2016). A QTL on the Short Arm of Wheat (Triticum aestivum L.) Chromosome 3B Affects the Stability of Grain Weight in Plants Exposed to a Brief Heat Shock Early in Grain Filling. Bmc Plant Biol. 16, 100. doi:10.1186/s12870-016-0784-6
Sultana, N., Islam, S., Juhasz, A., and Ma, W. (2021). Wheat Leaf Senescence and its Regulatory Gene Network. Crop J. 9, 703–717. doi:10.1016/j.cj.2021.01.004
Tahmasebi, S., Heidari, B., Pakniyat, H., and Mcintyre, C. L. (2017). Mapping QTLs Associated with Agronomic and Physiological Traits under Terminal Drought and Heat Stress Conditions in Wheat (Triticum aestivum L.). Genome 60, 26–45. doi:10.1139/gen-2016-0017
Talukder, S. K., Babar, M. A., Vijayalakshmi, K., Poland, J., Prasad, P. V. V., Bowden, R., et al. (2014). Mapping QTL for the Traits Associated with Heat Tolerance in Wheat (Triticum aestivum L.). Bmc Genet. 15, 97. doi:10.1186/s12863-014-0097-4
Thakur, J. K., Tyagi, A. K., and Khurana, J. P. (2001). OsIAA1, an Aux/IAA cDNA from Rice, and Changes in its Expression as Influenced by Auxin and Light. DNA Res. 8, 193–203. doi:10.1093/dnares/8.5.193
Thomas, H., and Ougham, H. (2014). The Stay-Green Trait. J. Exp. Bot. 65, 3889–3900. doi:10.1093/jxb/eru037
Verma, V., Foulkes, M. J., Worland, A. J., Sylvester-Bradley, R., Caligari, P. D. S., and Snape, J. W. (2004). Mapping Quantitative Trait Loci for Flag Leaf Senescence as a Yield Determinant in Winter Wheat under Optimal and Drought-Stressed Environments. Euphytica 135, 255–263. doi:10.1023/b:Euph.0000013255.31618.14
Vijayalakshmi, K., Fritz, A. K., Paulsen, G. M., Bai, G., Pandravada, S., and Gill, B. S. (2010). Modeling and Mapping QTL for Senescence-Related Traits in Winter Wheat under High Temperature. Mol. Breed. 26, 163–175. doi:10.1007/s11032-009-9366-8
Wang, H., Wang, S., Chang, X., Hao, C., Sun, D., and Jing, R. (2019). Identification of TaPPH-7A Haplotypes and Development of a Molecular Marker Associated with Important Agronomic Traits in Common Wheat. Bmc Plant Biol. 19, 296. doi:10.1186/s12870-019-1901-0
Wang, N., Xie, Y., Li, Y., Wu, S., Li, S., Guo, Y., et al. (2020a). High-Resolution Mapping of the Novel Early Leaf Senescence Gene Els2 in Common Wheat. Plants 9, 698. doi:10.3390/plants9060698
Wang, N., Xie, Y. Z., Li, Y. Z., Wu, S. N., Wei, H. S., and Wang, C. S. (2020b). Molecular Mapping of a Novel Early Leaf-Senescence Gene Els2 in Common Wheat by SNP Genotyping Arrays. Crop Pasture Sci. 71, 356–367. doi:10.1071/cp19435
Wang, S.-X., Zhu, Y.-L., Zhang, D.-X., Shao, H., Liu, P., Hu, J.-B., et al. (2017). Genome-Wide Association Study for Grain Yield and Related Traits in Elite Wheat Varieties and Advanced Lines Using SNP Markers. Plos One 12, e0188662. doi:10.1371/journal.pone.0188662
Wang, S., Wong, D., Forrest, K., Allen, A., Chao, S., Huang, B. E., et al. (2014). Characterization of Polyploid Wheat Genomic Diversity Using a High‐Density 90 000 Single Nucleotide Polymorphism Array. Plant Biotechnol. J. 12, 787–796. doi:10.1111/pbi.12183
Xu, M.-R., Huang, L.-Y., Zhang, F., Zhu, L.-H., Zhou, Y.-L., and Li, Z.-K. (2013). Genome-Wide Phylogenetic Analysis of Stress-Activated Protein Kinase Genes in Rice (OsSAPKs) and Expression Profiling in Response to Xanthomonas oryzae Pv. Oryzicola Infection. Plant Mol. Biol. Rep. 31, 877–885. doi:10.1007/s11105-013-0559-2
Xu, Y.-F., Li, S.-S., Li, L.-H., Ma, F.-F., Fu, X.-Y., Shi, Z.-L., et al. (2017). QTL Mapping for Yield and Photosynthetic Related Traits under Different Water Regimes in Wheat. Mol. Breed. 37. doi:10.1007/s11032-016-0583-7
Yamatani, H., Kohzuma, K., Nakano, M., Takami, T., Kato, Y., Hayashi, Y., et al. (2018). Impairment of Lhca4, a Subunit of LHCI, Causes High Accumulation of Chlorophyll and the Stay-Green Phenotype in Rice. J. Exp. Bot. 69, 1027–1035. doi:10.1093/jxb/erx468
Yan, X., Wang, S., Yang, B., Zhang, W., Cao, Y., Shi, Y., et al. (2020). QTL Mapping for Flag Leaf-Related Traits and Genetic Effect of QFLW-6A on Flag Leaf Width Using Two Related Introgression Line Populations in Wheat. Plos One 15, e0229912. doi:10.1371/journal.pone.0229912
Yang, B., Yan, X., Wang, H., Li, X., Ma, H., Wang, S., et al. (2016a). Dynamic QTL Analysis of Chlorophyll Content during Grain Filling Stage in Winter Wheat (Triticum aestivum L.). Rom. Agric. Res. 33, 77–85.
Yang, D.-L., Jing, R.-L., Chang, X.-P., and Li, W. (2007). Quantitative Trait Loci Mapping for Chlorophyll Fluorescence and Associated Traits in Wheat (Triticum aestivum). J. Integr. Plant Biol. 49, 646–654. doi:10.1111/j.1744-7909.2007.00443.x
Yang, D., Li, M., Liu, Y., Chang, L., Cheng, H., Chen, J., et al. (2016b). Identification of Quantitative Trait Loci and Water Environmental Interactions for Developmental Behaviors of Leaf Greenness in Wheat. Front. Plant Sci. 7, 273. doi:10.3389/fpls.2016.00273
Yang, J., Zhou, Y., Wu, Q., Chen, Y., Zhang, P., Zhang, Y., et al. (2019). Molecular Characterization of a Novel TaGL3-5A Allele and its Association with Grain Length in Wheat (Triticum aestivum L.). Theor. Appl. Genet. 132, 1799–1814. doi:10.1007/s00122-019-03316-1
Yang, Y. R., Huang, Q. Q., Zhao, Y. A., Tang, J. Y., and Liu, X. (2020). Advances on Gene Isolation and Molecular Mechanism of Rice Leaf Color Genes. J. Plant Genet. Resour. 21, 794–803.
Yang, Y., Xu, J., Huang, L., Leng, Y., Dai, L., Rao, Y., et al. (2016c). PGL, Encoding Chlorophyllide a Oxygenase 1, Impacts Leaf Senescence and Indirectly Affects Grain Yield and Quality in Rice. Exbotj 67, 1297–1310. doi:10.1093/jxb/erv529
Ye, W. (2016). Fine Mapping Leaf Chlorophyll Content QTL qFCC7L& Cloning and Function Analysis of Leaf Color Gene WSL12. dissertation/doctor’s thesis (Zhejiang: Zhejiang University).
Yu, M., Mao, S.-l., Chen, G.-y., Liu, Y.-x., Li, W., Wei, Y.-m., et al. (2014). QTLs for Waterlogging Tolerance at Germination and Seedling Stages in Population of Recombinant Inbred Lines Derived from a Cross between Synthetic and Cultivated Wheat Genotypes. J. Integr. Agric. 13, 31–39. doi:10.1016/s2095-3119(13)60354-8
Zhang, H., Liu, L., Cai, M., Zhu, S., Zhao, J., Zheng, T., et al. (2015). A Point Mutation of Magnesium Chelatase OsCHLI Gene Dampens the Interaction between CHLI and CHLD Subunits in Rice. Plant Mol. Biol. Rep. 33, 1975–1987. doi:10.1007/s11105-015-0889-3
Zhang, K., Fang, Z., Liang, Y., and Tian, J. (2009a). Genetic Dissection of Chlorophyll Content at Different Growth Stages in Common Wheat. J. Genet. 88, 183–189. doi:10.1007/s12041-009-0026-x
Zhang, K., Zhang, Y., Chen, G., and Tian, J. (2009b). Genetic Analysis of Grain Yield and Leaf Chlorophyll Content in Common Wheat. Cereal Res. Commun. 37, 499–511. doi:10.1556/crc.37.2009.4.3
Zhang, Z., Lu, Y., Zhai, L., Deng, R., Jiang, J., Li, Y., et al. (2012). Glycolate Oxidase Isozymes Are Coordinately Controlled by GLO1 and GLO4 in Rice. Plos One 7, e39658. doi:10.1371/journal.pone.0039658
Zhou, S., Sawicki, A., Willows, R. D., and Luo, M. (2012). C-Terminal Residues of Oryza sativa GUN4 Are Required for the Activation of the ChlH Subunit of Magnesium Chelatase in Chlorophyll Synthesis. Febs Lett. 586, 205–210. doi:10.1016/j.febslet.2011.12.026
Zhu, X.-F., Zhang, H.-P., Hu, M.-J., Wu, Z.-Y., Jiang, H., Cao, J.-J., et al. (2016). Cloning and Characterization of Tabas1-B1 Gene Associated with Flag Leaf Chlorophyll Content and Thousand-Grain Weight and Development of a Gene-Specific Marker in Wheat. Mol. Breed. 36, 142. doi:10.1007/s11032-016-0563-y
Keywords: wheat, drought, chlorophyll, flag leaf, quantitative trait locus
Citation: Yang B, Wen X, Wen H, Feng Y, Zhao J, Wu B, Zheng X, Yang C, Yang S, Qiao L and Zheng J (2022) Identification of Genetic Loci Affecting Flag Leaf Chlorophyll in Wheat Grown under Different Water Regimes. Front. Genet. 13:832898. doi: 10.3389/fgene.2022.832898
Received: 10 December 2021; Accepted: 25 January 2022;
Published: 15 March 2022.
Edited by:
Pengtao Ma, Yantai University, ChinaCopyright © 2022 Yang, Wen, Wen, Feng, Zhao, Wu, Zheng, Yang, Yang, Qiao and Zheng. This is an open-access article distributed under the terms of the Creative Commons Attribution License (CC BY). The use, distribution or reproduction in other forums is permitted, provided the original author(s) and the copyright owner(s) are credited and that the original publication in this journal is cited, in accordance with accepted academic practice. No use, distribution or reproduction is permitted which does not comply with these terms.
*Correspondence: Sanwei Yang, ysvysv@163.com; Ling Qiao, qiaolingsmile@163.com; Jun Zheng, sxnkyzj@126.com
†These authors have contributed equally to this work and share first authorship