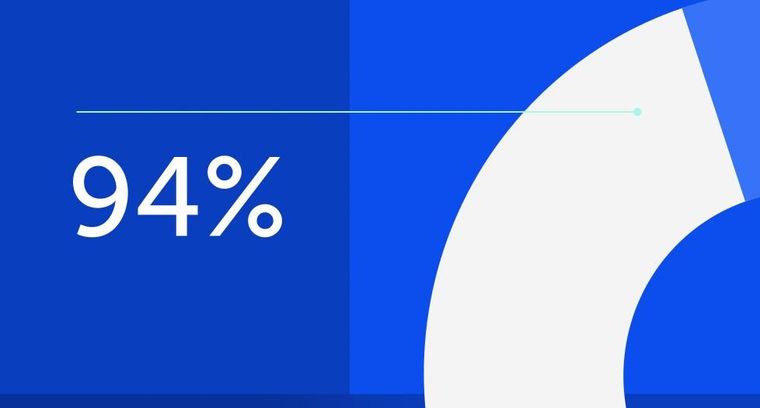
94% of researchers rate our articles as excellent or good
Learn more about the work of our research integrity team to safeguard the quality of each article we publish.
Find out more
ORIGINAL RESEARCH article
Front. Genet., 27 May 2022
Sec. Cancer Genetics and Oncogenomics
Volume 13 - 2022 | https://doi.org/10.3389/fgene.2022.828456
Endometrial cancer (EC) is the gynecological tumor with the highest incidence. In recent years, it has been proved that necroptosis is a method of cell death related to EC. However, the expression of necroptosis-related miRNA in EC and its correlation with prognosis still ill-defined. Use the Cancer Genome Atlas (TCGA) cohort to obtain prognostic data and related clinical data for ECs and normal endometrium tissues. In this study, we identified three necroptotic regulatory miRNAs that are necroptosis-related and survival-related miRNAs (DENSMs) between normal endometrium tissues and EC from 13 necroptosis-related miRNAs. The three DENSMs signature was built to develop prognostic model and classified all EC patients into a high or low risk group. EC patients in the low-risk group showed significantly higher survival possibilities than those in the high-risk group (p = 0.0242), and the risk score was found to be an independent prognosis factor for predicting the OS of EC patients (p = 0.0254) in multivariate Cox regression. Gene Ontology (GO) and Kyoto Encyclopedia of Genes and Genomes (KEGG) analysis showed dephosphorylation, microtubule, protein serine/threonine kinase activity, PI3K-Akt signaling pathway and MAPK signaling pathway are closely related to it. In conclusion, the risk prediction model based on necroptosis-related miRNAs can effectively predict the prognosis of EC patients.
Endometrial cancer (EC) is the most common gynecological cancer in developed countries and ranks second in developing countries (Bray et al., 2018). In 2018, there will be an estimated 382,000 new cases and 89,900 deaths worldwide (Bray et al., 2018). With the decline in birth rate and the prevalence of obesity, the incidence rate has risen significantly (Bray et al., 2018). Most of the primary cases of EC patients were surgically removed, and the prognosis was good, with a 5-years survival rate of more than 70% (Uccella et al., 2016). However, vaginal or pelvic EC recurrence, usually metastasized to a distant location, still occurs 15%–20% of cases after surgery (Connor and Rose, 2018). Despite progress in surgical techniques, chemotherapy or radiation, the data show an increasing mortality rate of EC (Balch et al., 2010; Siegel et al., 2021; Sung et al., 2021). Therefore, a thorough prognostic assessment is urgently needed. Recent studies have shown that necroptosis plays an important role in the formation and development of EC, suggesting that necroptosis-related miRNAs can be used as prognostic markers of EC (Ghanekar and Sadasivam, 2019; López-Janeiro et al., 2021).
Necroptosis is a cell death caused by regulation of independent of caspase. Necroptosis is mainly composed of protein kinase 1 receptor (RIPK1), protein kinase 3 receptor (RIPK3) and mixed lineage kinase domain-like protein (MLKL) (Choi et al., 2019) (Choi et al., 2019). Necroptosis is a programmed necrosis (Galluzzi and Kromer, 2008; Christofferson and Yuan, 2010; Wallach et al., 2016; Galluzzi et al., 2018; Green 2019). The morphological characteristics of necrotic apoptotic cells include crisis of intracellular energy metabolism, incomplete cell membrane and release of inflammatory factors (Pasparakis and Vandenabeele, 2015). Necroptosis plays an important role in the emergence and development of the metastasis of cancer, cerebrovascular diseases and a variety of diseases (Galluzzi et al., 2017; Choi et al., 2019; Tang et al., 2020; Xia et al., 2020). Necroptosis has positive and negative effects in malignant tumors (Su et al., 2015; Galluzzi et al., 2018; Frank and Vince, 2019; Liu et al., 2021). On the one hand, necroptosis can promote tumor metastasis and progression alone or in combination with other factors, and on the other hand, necroptosis acts as a protection against tumor development when apoptosis is disrupted (Su et al., 2015; Frank and Vince 2019; Liu et al., 2021). In this study we examined the influence of necroptosis on the prognosis of ECs.
MicroRNAs (miRNAs) are short non-coding RNAs 19-24 nucleotides in length that play a role in regulating gene expression through antisense complementation or specific mRNA complementation (Vasilatou et al., 2015). Many studies have shown that a large number of miRNAs are abnormally expressed in a large number of tumors and participate in the occurrence and development of tumors as oncogenes or tumor suppressor genes (Bueno et al., 2008; Torres et al., 2013). Previous research tried to establish a connection between miRNA and EC. For example, has-mir-337-3p, let-7b, and miR135a are considered to have the potential as non-invasive biomarkers (Sun et al., 2017). Although there have been many reports on the influence of miRNAs on the formation and development of EC (Sun et al., 2017; Jiang et al., 2018; Sun et al., 2018; Wang Q. et al., 2020), there are no reports on the use of necroptosis miRNAs as molecular markers to predict the prognosis of EC patients. Therefore, whether the miRNAs associated with necroptosis is related to the prognosis of patients with EC remains unclear. It is very important to study miRNAs associated with necroptosis as molecular markers to predict the prognosis of EC. However, there are few studies on the prognostic value of miRNAs associated with necroptosis in EC and even other cancers (Chen et al., 2022; Zhang et al., 2022). Zhang et al. reported that necroptosis-related signatures as an independent prognostic model for head and neck squamous cell carcinoma (Zhang et al., 2022). Chen et al. showed they used necroptosis-related lncRNA to establish novel prognostic signature and predict the immunotherapy response in breast cancer (Chen et al., 2022).
To solve the above problems, in this study we obtained the clinical information and miRNAs profile of normal endometrium and EC tissue from the Cancer Genome Atlas (TCGA). From previously published literature, we have identified and classified necroptosis-related miRNAs (Liu et al., 2021). In this study, we developed a prognostic model to understand the expression levels of miRNA necroptosis in EC and normal endometrial tissues, and to study the prognostic value of miRNAs. Kaplan-Meier curve (KM), area under the curve (AUC), and forest plot show that these models have good predictive effects. Finally, predict the target gene by the different miRNAs expressed, and analyze the functional enrichment of the target gene to study the potential mechanism of these miRNAs.
As of 31 August 2021, we have obtained miRNA and clinical feature data for 546 EC and 33 normal tissue patients from the TCGA database (https://portal.gdc.cancer.gov/repository). The clinicopathological characteristics of the patients in the TCGA database are shown in Table 1. The list of 13 necrotizing miRNA comes from earlier literature (Liu et al., 2021) and is showed as follow: miR-495, miR-331-3p, miR-15a, miR-148a-3p, miR-7-5p, miR-141-3p, miR-425-5p, miR-200a-5p, miR-210, miR-223-3p, miR-500a-3p, miR-181-5p, miR-16-5p. Then extract th e miRNA expression matrix of necroptosis, and perform data matching, data filtering, and data correction. At the same time, relevant clinical data are also filtered and matched for subsequent use. All data are standardized using the cross-array scaling function of the limma R/Bioconductor package. In addition, our research strictly complies with the TCGA data access policy and publication guidelines. The RNA-seq data of the miRNA target genes were downloaded from the GEO database (https://www.ncbi.nlm.nih.gov/geo/, ID: GSE17025).
To accurately assess the prognostic value of necroptosis-related miRNAs, we used the “Limma” package to find differentially expressed necroptosis-related miRNAs. The different expressed miRNAs were filtered according to the standards (|log2FC| ≥ 0 and FDR <0.05). Then, KM curves were used to draw survival curves for the differentially expressed necroptosis-related miRNAs, in order to find the differentially expressed necroptosis-related and survival-related miRNAs (DENSMs). We finally identified a total of three DENSMs for the development of prognostic models and the next step of analysis. Then we divided the patient data of TCGA into two groups according to the ratio of 2:1, one is training cohort for building the prognostic model, and the other is validation cohort for model validation. A total of 364 patients were assigned to the training cohort and 182 patients were assigned to the validation cohort. The clinicopathological characteristics of the training cohort and validation cohort patients are shown in Table 1. The use of DENSMs to calculate risk scores in training cohort is the key to constructing prognostic models. We extracted many clinical characteristics of EC patients from the TCGA database, including age, grade, and stage. These clinical variable data are combined with risk scores in our prognostic model for further analysis. Then, univariate and multivariate Cox regression models are used to further analyze the risk score to determine whether the risk score can be an independent prognostic factor. The predictive ability of the model is determined by drawing the Receiver Operating Characteristics (ROC) curve to calculate the area under the ROC curve (AUC). The “survminer”, “survival”and “time- ROC” R packages were employed to perform a 1-year, 3-years and 5-years ROC curve analysis. According to the calculation of risk score, we divided training cohort EC patients into low-risk and high-risk subgroups by the median of risk score. KM curve analysis compares the overall survival (OS) time of the two subgroups, and Cox regression compares the risk ratio of the two subgroups. Then, we used the validation cohort for validation. And group high-risk and low-risk according to the risk score obtained in training cohort. Then, KM survival time analysis, ROC analysis, univariate and multivariate cox regression analysis were performed on the patients in the validation cohort.
Based on these DENSMs, we utilized three miRNA target gene prediction sites (miRDB, http://mirdb.org; miRTarBase, http://mirtarbase.mbc.nctu.edu.tw; TargetScan, http://www.targetscan.org) to predict the target genes of these 3 miRNAs respectively. Use cytoscape software to display miRNA-target genes network. Gene notology (GO) and the Kyoto Encyclopedia of Genes and Genomes (KEGG) analyses were performed by applying the “clusterProfiler” package (Yu et al., 2012). We further analyzed and validated some miRNA-targeted genes’ expression levels in the GEO database (GSE17025). Further, we analyzed the survival time of some miRNA-targeted genes in the TCGA database.
In order to compare the difference in miRNA expression levels between normal and EC tissues, we used a one-way analysis of variance. However, when comparing categorical variables, we chose to use the Pearson chi-square test. The Kaplan-Meier method of two-sided log-rank test was used to compare the OS of subgroups of patients. Univariate and multivariate Cox regression models are used to evaluate the independent prognostic value of risk models. All statistical analyses were accomplished with R software (v4.0.5).
We obtained a total of 33 normal tissues and 546 tumor tissues in the TCGA database, and compared the expression levels of 13 miRNAs related to necroptosis. We found out six differentially expressed necroptosis-related miRNAs (p < 0.05). Among them, 6 miRNAs (miR-141-3p, miR-200a-5p, miR-16-5p, miR-425-5p, miR-331-3p, and miR-7-5p) were all enriched in the tumor group. The expression levels of these miRNAs were shown as a heat map (Figure 1A) and box plots (Figure 1B).
FIGURE 1. Expressions of the six differentially expressed necroptosis-related miRNAs. (A) Heatmap (green: low expression level; red: high expression level) of the differentially expressed necroptosis-related miRNAs between the normal (N, brilliant blue) and the tumor tissues (T, red). (B) Box plot of the differentially expressed necroptosis-related miRNAs between the normal (blue) and the tumor tissues (red). ***p < 0.001.
KM method was applied to assess survival time data of six differentially expressed miRNAs. Analysis demonstrated higher expression levels of miR-16-5p (p = 0.03678, Figure 2A), miR-331-3p (p = 0.01587, Figure 2B) and miR-425-5p (p = 0.00748, Figure 2C) were all correlated with shorter survival time. In addition, there was no significant correlation between the expression levels of miR-7-5p (Figure 2D), miR-141-3p (Figure 2E) and miR-200a-5p (Figure 2F) and survival time. Preliminary analysis of three DENSMs using univariate Cox regression analysis. The miR-425-5p met the criteria of p < 0.05 which increased the risk with HR = 1.2035 (1.0157–1.4261) (p = 0.0324, Figure 3A). In addition to this, the miR-16-5p and miR-331-3p met the criteria of p < 0.15 which increase the risk with HR = 1.2011 (0.9432–1.5296) and 1.1604 (0.9704–1.3877) respectively (Figure 3A). Then we divided the patient data of TCGA into two groups according to the ratio of 2:1, one is training cohort for building the prognostic model, and the other is validation cohort for model validation. Based on the above 3 DENSMs, we constructed a prognostic model. The risk score was calculated as follows: risk score = (−0.04199*miR-16-5p exp.) + (0.10270*miR-331-3p exp.) + (0.06461 *miR-425-5p exp.). According to the median score calculated using the risk score formula, 364 patients in training cohort were divided equally into a low-risk and a high-risk subgroup. There is a significant difference between the OS time of patients in the low-risk group and the high-risk group (p = 0.0242, Figure 3B) in training cohort. In addition, age (p = 0.0196, Figure 3C) and stage (p < 0.001, Figure 3D) also showed a correlation with survival time in training cohort. Using time-dependent ROC analysis to assess the sensitivity and specificity of the predictive model, we found that the AUC were 0.713 for 1 year, 0.593 for 3 years and 0.639 for 5 years (Figure 4A) in training cohort. Then we used ROC analysis to evaluate the sensitivity and specificity for risk score, age, grade and stage, we found that the AUC were 0.7197 for risk score, 0.5382 for age, 0.6516 for grade and 0.6889 for stage at 1 year (Figure 4B) in training cohort.
FIGURE 2. Kaplan–Meier curves of the OS for six miRNAs. (A) Kaplan–Meier curves of the OS for miR-16-5p. (B) Kaplan–Meier curves of the OS for miR-331-3p. (C) Kaplan–Meier curves of the OS for miR-425-5p. (D) Kaplan–Meier curves of the OS for miR-7-5p. (E) Kaplan–Meier curves of the OS for miR-141-3p. (F) Kaplan–Meier curves of the OS for miR-200a-5p.
FIGURE 3. Construction of the risk score model. (A) Univariate cox regression analysis of OS for three DENSMs in TCGA cohort. (B) Kaplan–Meier curves for the OS of patients in the high- and low-risk groups in training cohort. (C) Kaplan–Meier curves for the OS of patients in the high- and low-age groups in training cohort. (D) Kaplan–Meier curves for the OS of patients in the high- and low-stage groups in training cohort.
FIGURE 4. ROC curves of the risk score (A) ROC curves demonstrated the predictive efficiency of the risk score at 1, 3 and 5 years in train cohort. (B) ROC curves demonstrated the predictive efficiency of the risk score, age, grade and stage at 1 year in training cohort.
Univariate and multivariate Cox regression analysis are used to assess whether the risk score derived from the model can be used as an independent predictor. Univariate Cox regression analysis showed that risk score was a predictor of low survival rate in training cohort (HR = 1.8755, 95% CI: 1.0872–3.2353, p = 0.0238, Figure 5A). Multivariate regression analysis also implied that risk score was an independent prognostic factor after adjusting for other confounding factors (HR = 1.8669, 95% CI: 1.0800–3.2270, p = 0.0254, Figure 5B) for patients with EC in training cohort.
FIGURE 5. Cox regression analysis of the risk score in training cohort. (A) Univariate regression analysis of the risk score in training cohort. (B) Multivariate regression analysis of the risk score in training cohort. (Stage: FIGO stage, I and II vs. III and IV).
To further validate the risk model, we used the validation cohort for validation. Based on the risk score of the training cohort, 182 patients in validation cohort were classified into the high- and low-risk group. KM analysis showed a significant difference between the OS time of patients in the low-risk group and the high-risk group (p = 0.0038, Figure 6A) in validation cohort. ROC analysis showed that the AUC were 0.586 for 1 year, 0.607 for 3 years and 0.707 for 5 years (Figure 6B) in validation cohort. Univariate Cox regression analysis showed that risk score was a predictor of low survival rate in validation cohort (HR = 3.1148, 95% CI: 1.3855–7.0027, p = 0.0060, Figure 6C). Multivariate regression analysis also implied that risk score was an independent prognostic factor after adjusting for other confounding factors (HR = 3.3408, 95% CI: 1.4449–7.7245, p = 0.0048, Figure 6D) in validation cohort.
FIGURE 6. Validation of the risk model in validation cohort. (A) Kaplan–Meier curves for the OS of patients in the high- and low-risk groups in validation cohort. (B) ROC curves demonstrated the predictive efficiency of the risk score at 1, 3 and 5 years in validation cohort. (C) Univariate regression analysis of the risk score in validation cohort. (D) Multivariate regression analysis of the risk score in validation cohort. (Stage: FIGO stage, I and II vs. III and IV).
Further examine the differences of functions and pathways in the three DENSMs, we utilized three miRNA target gene prediction sites (miRDB, http://mirdb.org; miRTarBase, http://mirtarbase.mbc.nctu.edu.tw; TargetScan, http://www.targetscan.org) to predict the target genes of these three miRNAs respectively. Finally, through these three DENSMs, we found 493 target genes (Figure 7A). The network constructed by the three DENSMs and their 493 target genes were exhibited (Figure 7B). Then based on these 493 target genes, GO enrichment analysis and KEGG pathway analysis were performed. GO analysis is divided into three parts, namely biological process, cell composition and molecular function analysis. Biological processes are mainly related to dephosphorylation, histone modification and covalent chromatin modification. Cellular components are mainly related to microtubules, vacuolar membranes and early endosomes. The molecular function is mainly related to protein serine/threonine kinase activity (Figure 8A). The KEGG results indicated that the target genes were mainly related with the PI3K-Akt signaling pathway and MAPK signaling pathway (Figure 8B). This means that the DENSMs are likely to be related to these functional pathways. We further analyzed and validated some miRNA-targeted genes. We obtained 91 EC patients and 12 normal controls from the GEO database (GSE17025) for expression analysis. We show the expression of three differentially expressed miRNA-targeted genes, CCNE1 (Figure 9A), FKBP1A (Figure 9B) and TPT1 (Figure 9C). Further, we analyzed the survival time of these three genes in the TCGA database. KM analysis showed that CCNE1 (Figure 9D), FKBP1A (Figure 9E) and TPT1 (Figure 9F) were significantly correlated with OS.
FIGURE 7. MiRNA target genes of the three DENSMs. (A) The Venn diagram of DENSMs by three prediction websites (miRDB, miRTarBase, TargetScan). (B) The network of DENSMs made by Cytoscape.
FIGURE 8. Functional analysis based on the MiRNA target genes of the three DENSMs. (A) Bubble graph for GO enrichment for DENSMs target genes (the bigger bubble means the more genes enriched, and the increasing depth of red means the differences were more obvious; q-value: the adjusted p-value). (B) Barplot graph for KEGG pathways for DENSMs target genes (the longer bar means the more genes enriched, and the increasing depth of red means the differences were more obvious).
FIGURE 9. Expression and survival analysis of three MiRNA target genes. (A) Box plot of the differentially expressed MiRNA target genes (CCNE1) between the normal (blue) and the tumor tissues (red) in GEO database. (B) Box plot of the differentially expressed MiRNA target genes (FKBP1A) between the normal (blue) and the tumor tissues (red) in GEO database. (C) Box plot of the differentially expressed MiRNA target genes (TPT1) between the normal (blue) and the tumor tissues (red) in GEO database. (D) Kaplan–Meier curves of the OS for CCNE1 in GEO database. (E) Kaplan–Meier curves of the OS for FKBP1A in GEO database. (F) Kaplan–Meier curves of the OS for TPT1in GEO database. *p < 0.05; **p < 0.01.
Although there have been some articles using genetic models to predict the prognosis of EC patients (Wang H. et al., 2020; Zhang et al., 2020a; Wang X. et al., 2021; Weijiao et al., 2021), there is no literature that systematically uses necroptosis-related miRNAs to predict the prognosis of EC patients. As far as we know, this study is the first to systematically use necroptosis-related miRNAs to predict the prognosis of EC patients. In this study, we adopted three DENSMs (miR-16-5p, miR-331-3p and miR-425-5p) to construct prognostic models. As to our prognostic models, KM survival analysis, univariate Cox regression, multivariate Cox regression and the AUC all illustrated our models had a great predictive performance. Additionally, the risk scores of the OS model could be considered as independent predictive indicators. At the same time, we also analyzed the performance of clinical variables including age, grade, and stage in KM analysis and ROC curve analysis. It can be seen that stage is still a good prognostic indicator. In addition, we performed further validation of the prognostic model using the validation cohort. In the validation cohort, the predictive effect of the prognostic model in EC was very good, both in KM prognostic analysis, univariate and multivariate cox regression and ROC curve analysis. This model can provide a new reference for prognostic risk stratification assessment and treatment strategy selection for EC patients.
Necroptosis is a new type of programmed necrosis (Galluzzi et al., 2017; Choi et al., 2019). It has two opposite effects in antitumor and protumor (Su et al., 2015; Tang et al., 2020). In the present study, we successfully constructed a prognostic model based on three DENSMs. These three DENSMs have been found to be related to ECs. MiR-331-3p has been reported to play an important role in the EMT of ECs of which has been shown to related to survival in our study (Zmarzły et al., 2021). Nikola Zmarzły et al. found that the Wnt signaling is disrupted in endometrial cancer, which may be due to miR-331-3p activity (Zmarzły et al., 2021). MiR-16-5p may also play an important role in the occurrence and development of EC. Piotr Januszyk et al. found that significant changes in the expression of miR-16 with Drug Resistance in Endometrial Cancer Cells Treated with Salinomycin (Januszyk et al., 2021). Xiyin Wang et al. found that the expression of miR-16 has also changed significantly with Pten and Dicer1 lost in the mouse uterus causes poorly differentiated endometrial adenocarcinoma (Wang X. et al., 2020). Priti Kumari et al. reported when looking for Diagnostic potential of differentially regulated microRNAs among endometriosis, endometrioid ovarian cancer, and endometrial cancer, they found that miR-16 can be used as one of the important markers (Kumari et al., 2021). In addition, GO functional enrichment and KEGG signaling pathway analysis showed that differentially expressed necroptosis-related miRNAs were involved in some cancer-related signaling pathway such as dephosphorylation, histone modification, covalent chromatin modification, the PI3K-Akt signaling pathway and MAPK signaling pathway. In summary, these differently expressed necroptosis-related miRNAs are involved in many important biological functions and signaling pathways in connection with ECs. At the same time, we analyzed the expression levels of some miRNA target genes in the GEO database, and found that some of them had obvious expression differences. We then further analyzed the prognosis of these differentially expressed miRNA target genes in the TCGA database, and found that some of them also had significant prognostic differences. We showed three representative miRNA target genes in the results, CCNE1, FKBP1A, TPT1. Xu et al. found that CCNE1 copy number is a biomarker for response to combination WEE1-ATR inhibition in endometrial cancer (Xu et al., 2021). FKBP1A has been shown to have a clear role in other cancers including prostate cancer and head and neck squamous cell carcinoma (Zhang et al., 2019b; Patel et al., 2022). Zhang et al. found that TPT1 was a hub gene in EC and there were some studies had reported that TPT1 had role in some cancers (Zhang and Wang, 2019a; Zhang and Wang, 2020b; Wang N. et al., 2021). These miRNA target genes can be a direction guide for our future EC research to better explore the relationship between EC and necroptosis and its pathogenesis.
There are some limitations in this study. First of all, all the data in this study came from TCGA database, and the total sample size was relatively insufficient. Larger clinical samples are needed to validate the conclusions of this study. Secondly, due to the lack of more data on clinical variables, we are unable to conduct a more comprehensive clinical analysis. Thirdly, the molecular mechanism of necroptosis affecting the prognosis of endometrial cancer patients and its significance for clinical translational therapy need to be further studied.
In this study, a risk prediction model of necroptosis-related miRNAs was successfully constructed, which can effectively predict the prognosis of EC patients. This model can provide biomarkers and therapeutic targets for accurate treatment of endometrial cancer in the future.
The original contributions presented in the study are included in the article/Supplementary Material, further inquiries can be directed to the corresponding author.
HS designed the study, conducted the analyses, and drafted the original manuscript. TL revised the paper and refined the language. JS and DL edited the figures. XL, HX, and WL revised the paper. KW and HY supervised the entire study and made recommendations. All authors have read and contributed to the vision of the manuscript and approved the submitted version.
This study was supported by Tianjin Science and Technology Plan (no. 20YFZCSY00040) and the Starting Foundation of MD/Ph.D. and Introduced Talent issued by Tianjin Medical University Cancer Institute and Hospital (no. B2113).
The authors declare that the research was conducted in the absence of any commercial or financial relationships that could be construed as a potential conflict of interest.
All claims expressed in this article are solely those of the authors and do not necessarily represent those of their affiliated organizations, or those of the publisher, the editors and the reviewers. Any product that may be evaluated in this article, or claim that may be made by its manufacturer, is not guaranteed or endorsed by the publisher.
We thank the TCGA project for its valuable contributions to EC research. We thank Risinger JH, Chandran U, Allard J, Maxwell GL, and their teams for sharing their data in GEO database.
Balch, C., Matei, D. E., Huang, T. H.-M., and Nephew, K. P. (2010). Role of Epigenomics in Ovarian and Endometrial Cancers. Epigenomics 2 (3), 419–447. doi:10.2217/epi.10.19
Bray, F., Ferlay, J., Soerjomataram, I., Siegel, R. L., Torre, L. A., and Jemal, A. (2018). Global Cancer Statistics 2018: GLOBOCAN Estimates of Incidence and Mortality Worldwide for 36 Cancers in 185 Countries. CA A Cancer J. Clin. 68 (6), 394–424. doi:10.3322/caac.21492
Bueno, M. J., de Castro, I. P., and Malumbres, M. (2008). Control of Cell Proliferation Pathways by microRNAs. Cell Cycle 7 (20), 3143–3148. doi:10.4161/cc.7.20.6833
Chen, F., Yang, J., Fang, M., Wu, Y., Su, D., and Sheng, Y. (2022). Necroptosis‐related lncRNA to Establish Novel Prognostic Signature and Predict the Immunotherapy Response in Breast Cancer. Clin. Lab. Anal. 36, e24302. doi:10.1002/jcla.24302
Choi, M. E., Price, D. R., Ryter, S. W., and Choi, A. M. K. (2019). Necroptosis: a Crucial Pathogenic Mediator of Human Disease. JCI Insight 4 (15). doi:10.1172/jci.insight.128834
Christofferson, D. E., and Yuan, J. (2010). Necroptosis as an Alternative Form of Programmed Cell Death. Curr. Opin. Cell Biol. 22 (2), 263–268. doi:10.1016/j.ceb.2009.12.003
Connor, E. V., and Rose, P. G. (2018). Management Strategies for Recurrent Endometrial Cancer. Expert Rev. Anticancer Ther. 18 (9), 873–885. doi:10.1080/14737140.2018.1491311
Frank, D., and Vince, J. E. (2019). Pyroptosis versus Necroptosis: Similarities, Differences, and Crosstalk. Cell Death Differ. 26 (1), 99–114. doi:10.1038/s41418-018-0212-6
Galluzzi, L., Vitale, I., Aaronson, S. A., Abrams, J. M., Adam, D., Agostinis, P., et al. (2018). Molecular Mechanisms of Cell Death: Recommendations of the Nomenclature Committee on Cell Death 2018. Cell Death Differ. 25 (3), 486–541. doi:10.1038/s41418-017-0012-4
Galluzzi, L., Kepp, O., Chan, F. K.-M., and Kroemer, G. (2017). Necroptosis: Mechanisms and Relevance to Disease. Annu. Rev. Pathol. Mech. Dis. 12, 103–130. doi:10.1146/annurev-pathol-052016-100247
Galluzzi, L., and Kroemer, G. (2008). Necroptosis: a Specialized Pathway of Programmed Necrosis. Cell 135 (7), 1161–1163. doi:10.1016/j.cell.2008.12.004
Ghanekar, Y., and Sadasivam, S. (2019). In Silico analysis Reveals a Shared Immune Signature in CASP8-Mutated Carcinomas with Varying Correlations to Prognosis. PeerJ 7, e6402. doi:10.7717/peerj.6402
Green, D. R. (2019). The Coming Decade of Cell Death Research: Five Riddles. Cell 177 (5), 1094–1107. doi:10.1016/j.cell.2019.04.024
Januszyk, P., Januszyk, K., Wierzbik-Strońska, M., Boroń, D., and Grabarek, B. (2021). Analysis of the Differences in the Expression of mRNAs and miRNAs Associated with Drug Resistance in Endometrial Cancer Cells Treated with Salinomycin. Curr Pharm Biotechnol 22 (4), 541–548. doi:10.2174/1389201021666200629151008
Jiang, T., Sui, D., You, D., Yao, S., Zhang, L., Wang, Y., et al. (2018). RETRACTED ARTICLE: MiR-29a-5p Inhibits Proliferation and Invasion and Induces Apoptosis in Endometrial Carcinoma via Targeting TPX2. Cell Cycle 17 (10), 1268–1278. doi:10.1080/15384101.2018.1475829
Liu, Y., Chen, Q., Zhu, Y., Wang, T., Ye, L., Han, L., et al. (2021). Non-coding RNAs in Necroptosis, Pyroptosis and Ferroptosis in Cancer Metastasis. Cell Death Discov. 7 (1), 210. doi:10.1038/s41420-021-00596-9
López-Janeiro, Á., Ruz-Caracuel, I., Ramón-Patino, J. L., De Los Ríos, V., Villalba Esparza, M., Berjón, A., et al. (2021). Proteomic Analysis of Low-Grade, Early-Stage Endometrial Carcinoma Reveals New Dysregulated Pathways Associated with Cell Death and Cell Signaling. Cancers 13 (4), 794. doi:10.3390/cancers13040794
Pasparakis, M., and Vandenabeele, P. (2015). Necroptosis and its Role in Inflammation. Nature 517 (7534), 311–320. doi:10.1038/nature14191
Patel, D., Dabhi, A. M., Dmello, C., Seervi, M., Sneha, K. M., Agrawal, P., et al. (2022). FKBP1A Upregulation Correlates with Poor Prognosis and Increased Metastatic Potential of HNSCC. Cell Biol. Int. 46 (3), 443–453. doi:10.1002/cbin.11741
Sharma, I., Kumari, P., Saha, S., Srinivasan, R., and Minhas, P. (2021). Diagnostic Potential of Differentially Regulated microRNAs Among Endometriosis, Endometrioid Ovarian Cancer, and Endometrial Cancer. J. Can. Res. Ther. 17 (4), 1003–1011. doi:10.4103/jcrt.JCRT_969_19
Siegel, R. L., Miller, K. D., Fuchs, H. E., and Jemal, A. (2021). Cancer Statistics, 2021. CA A Cancer J. Clin. 71 (1), 7–33. doi:10.3322/caac.21654
Su, Z., Yang, Z., Xu, Y., Chen, Y., and Yu, Q. (2015). Apoptosis, Autophagy, Necroptosis, and Cancer Metastasis. Mol. Cancer 14, 48. doi:10.1186/s12943-015-0321-5
Sun, H., Yan, L., Tu, R., Zhang, Y., Ma, L., Tang, W., et al. (2017). Expression Profiles of Endometrial Carcinoma by Integrative Analysis of TCGA Data. Gynecol. Obstet. Invest. 82 (1), 30–38. doi:10.1159/000445073
Sun, X., Dongol, S., Qiu, C., Xu, Y., Sun, C., Zhang, Z., et al. (2018). miR-652 Promotes Tumor Proliferation and Metastasis by Targeting RORA in Endometrial Cancer. Mol. Cancer Res. 16 (12), 1927–1939. doi:10.1158/1541-7786.Mcr-18-0267
Sung, H., Ferlay, J., Siegel, R. L., Laversanne, M., Soerjomataram, I., Jemal, A., et al. (2021). Global Cancer Statistics 2020: GLOBOCAN Estimates of Incidence and Mortality Worldwide for 36 Cancers in 185 Countries. CA A Cancer J. Clin. 71 (3), 209–249. doi:10.3322/caac.21660
Tang, R., Xu, J., Zhang, B., Liu, J., Liang, C., Hua, J., et al. (2020). Ferroptosis, Necroptosis, and Pyroptosis in Anticancer Immunity. J. Hematol. Oncol. 13 (1), 110. doi:10.1186/s13045-020-00946-7
Torres, A., Torres, K., Pesci, A., Ceccaroni, M., Paszkowski, T., Cassandrini, P., et al. (2013). Diagnostic and Prognostic Significance of miRNA Signatures in Tissues and Plasma of Endometrioid Endometrial Carcinoma Patients. Int. J. Cancer 132 (7), 1633–1645. doi:10.1002/ijc.27840
Uccella, S., Bonzini, M., Palomba, S., Fanfani, F., Ceccaroni, M., Seracchioli, R., et al. (2016). Impact of Obesity on Surgical Treatment for Endometrial Cancer: A Multicenter Study Comparing Laparoscopy vs Open Surgery, with Propensity-Matched Analysis. J. Minim. Invasive Gynecol. 23 (1), 53–61. doi:10.1016/j.jmig.2015.08.007
Vasilatou, D., Sioulas, V. D., Pappa, V., Papageorgiou, S. G., and Vlahos, N. F. (2015). The Role of miRNAs in Endometrial Cancer. Epigenomics 7 (6), 951–959. doi:10.2217/epi.15.41
Wallach, D., Kang, T.-B., Dillon, C. P., and Green, D. R. (2016). Programmed Necrosis in Inflammation: Toward Identification of the Effector Molecules. Science 352 (6281), aaf2154. doi:10.1126/science.aaf2154
Wang, H., Ma, X., Liu, J., Wan, Y., Jiang, Y., Xia, Y., et al. (2020a). Prognostic Value of an Autophagy-Related Gene Expression Signature for Endometrial Cancer Patients. Cancer Cell Int. 20, 306. doi:10.1186/s12935-020-01413-6
Wang, N., Yang, Y., Jia, G.-Z., Wang, K., Zhou, S., Zhang, B., et al. (2021a). Long Non-coding RNA Down Syndrome Cell Adhesion Molecule-Anti-Sense 1 Promotes Gastric Carcinoma Cell Proliferation and Migration by Regulating the miR-204/TPT1 axis. Hum. Exp. Toxicol. 40 (12_Suppl. l), S187–s195. doi:10.1177/09603271211036037
Wang, Q., Xu, K., Tong, Y., Dai, X., Xu, T., He, D., et al. (2020b). Novel miRNA Markers for the Diagnosis and Prognosis of Endometrial Cancer. J. Cell Mol. Med. 24 (8), 4533–4546. doi:10.1111/jcmm.15111
Wang, X., Dai, C., Ye, M., Wang, J., Lin, W., and Li, R. (2021b). Prognostic Value of an Autophagy-Related Long-Noncoding-RNA Signature for Endometrial Cancer. Aging 13 (4), 5104–5119. doi:10.18632/aging.202431
Wang, X., Wendel, J. R. H., Emerson, R. E., Broaddus, R. R., Creighton, C. J., Rusch, D. B., et al. (2020c). Pten and Dicer1 Loss in the Mouse Uterus Causes Poorly Differentiated Endometrial Adenocarcinoma. Oncogene 39 (40), 6286–6299. doi:10.1038/s41388-020-01434-5
Weijiao, Y., Fuchun, L., Mengjie, C., Xiaoqing, Q., Hao, L., Yuan, L., et al. (2021). Immune Infiltration and a Ferroptosis-Associated Gene Signature for Predicting the Prognosis of Patients with Endometrial Cancer. Aging 13 (12), 16713–16732. doi:10.18632/aging.203190
Xia, X., Lei, L., Wang, S., Hu, J., and Zhang, G. (2020). Necroptosis and its Role in Infectious Diseases. Apoptosis 25 (3-4), 169–178. doi:10.1007/s10495-019-01589-x
Xu, H., George, E., Kinose, Y., Kim, H., Shah, J. B., Peake, J. D., et al. (2021). CCNE1 Copy Number Is a Biomarker for Response to Combination WEE1-ATR Inhibition in Ovarian and Endometrial Cancer Models. Cell Rep. Med. 2 (9), 100394. doi:10.1016/j.xcrm.2021.100394
Yu, G., Wang, L.-G., Han, Y., and He, Q.-Y. (2012). clusterProfiler: an R Package for Comparing Biological Themes Among Gene Clusters. OMICS A J. Integr. Biol. 16 (5), 284–287. doi:10.1089/omi.2011.0118
Zhang, J., Gao, S., Zhang, Y., Yi, H., Xu, M., Xu, J., et al. (2020a). MiR-216a-5p Inhibits Tumorigenesis in Pancreatic Cancer by Targeting TPT1/mTORC1 and Is Mediated by LINC01133. Int. J. Biol. Sci. 16 (14), 2612–2627. doi:10.7150/ijbs.46822
Zhang, J., Wang, Z., Zhao, R., An, L., Zhou, X., Zhao, Y., et al. (2020b). An Integrated Autophagy-Related Gene Signature Predicts Prognosis in Human Endometrial Cancer. BMC Cancer 20 (1), 1030. doi:10.1186/s12885-020-07535-4
Zhang, X., and Wang, Y. (2019a). Identification of Hub Genes and Key Pathways Associated with the Progression of Gynecological Cancer. Oncol. Lett. 18 (6), 6516–6524. doi:10.3892/ol.2019.11004
Zhang, Y., Zhang, D., Lv, J., Wang, S., and Zhang, Q. (2019b). LncRNA SNHG15 Acts as an Oncogene in Prostate Cancer by Regulating miR-338-3p/FKBP1A axis. Gene 705, 44–50. doi:10.1016/j.gene.2019.04.033
Zhang, Z., Hu, X., Qiu, D., Sun, Y., and Lei, L. (2022). Development and Validation of a Necroptosis-Related Prognostic Model in Head and Neck Squamous Cell Carcinoma. J. Oncol. 2022, 1–15. doi:10.1155/2022/8402568
Zmarzły, N., Hermyt, E., Kruszniewska-Rajs, C., Gola, J., Witek, A., Mazurek, U., et al. (2021). Expression Profile of EMT-Related Genes and miRNAs Involved in Signal Transduction via the Wnt Pathway and Cadherins in Endometrial Cancer. Curr Pharm Biotechnol 22 (12), 1663–1671. doi:10.2174/1389201021666201218125900
Keywords: necroptosis, miRNA, endometrial cancer, prognosis, biomarker
Citation: Song H, Li T, Sheng J, Li D, Liu X, Xiao H, Yu H, Liu W, Wang K and Chen Y (2022) Necroptosis-Related miRNA Biomarkers for Predicting Overall Survival Outcomes for Endometrial Cancer. Front. Genet. 13:828456. doi: 10.3389/fgene.2022.828456
Received: 03 December 2021; Accepted: 26 April 2022;
Published: 27 May 2022.
Edited by:
Yogesh Singh, University of Tübingen, GermanyReviewed by:
Gisela Ceballos, Instituto Nacional de Medicina Genómica (INMEGEN), MexicoCopyright © 2022 Song, Li, Sheng, Li, Liu, Xiao, Yu, Liu, Wang and Chen. This is an open-access article distributed under the terms of the Creative Commons Attribution License (CC BY). The use, distribution or reproduction in other forums is permitted, provided the original author(s) and the copyright owner(s) are credited and that the original publication in this journal is cited, in accordance with accepted academic practice. No use, distribution or reproduction is permitted which does not comply with these terms.
*Correspondence: Ying Chen, bHljaGVueWluZzIwMDRAMTI2LmNvbQ==
†These authors have contributed equally to this work
Disclaimer: All claims expressed in this article are solely those of the authors and do not necessarily represent those of their affiliated organizations, or those of the publisher, the editors and the reviewers. Any product that may be evaluated in this article or claim that may be made by its manufacturer is not guaranteed or endorsed by the publisher.
Research integrity at Frontiers
Learn more about the work of our research integrity team to safeguard the quality of each article we publish.